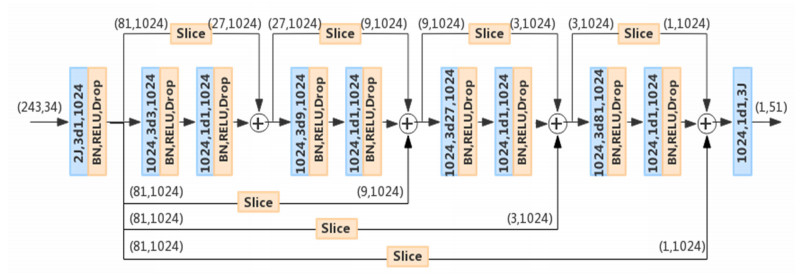
The purpose of this work is to interpret the experiences of students when audience response systems (ARS) were implemented as a strategy for teaching large mathematics lecture groups at university. Our paper makes several contributions to the literature. Firstly, we furnish a basic model of how ARS can form a teaching and learning strategy. Secondly, we examine the impact of this strategy on student attitudes of their experiences, focusing on the ability of ARS to: assess understanding; identify strengths and weaknesses; furnish feedback; support learning; and to encourage participation. Our findings support the position that there is a place for ARS as part of a strategy for teaching and learning mathematics in large groups.
Citation: Christopher C. Tisdell. Embedding opportunities for participation and feedback in large mathematics lectures via audience response systems[J]. STEM Education, 2021, 1(2): 75-91. doi: 10.3934/steme.2021006
[1] | Jaouhra Cherif, Anis Raddaoui, Ghofrane Ben Fraj, Asma Laabidi, Nada Souissi . Escherichia coli's response to low-dose ionizing radiation stress. AIMS Biophysics, 2024, 11(2): 130-141. doi: 10.3934/biophy.2024009 |
[2] | Tika Ram Lamichhane, Hari Prasad Lamichhane . Structural changes in thyroid hormone receptor-beta by T3 binding and L330S mutational interactions. AIMS Biophysics, 2020, 7(1): 27-40. doi: 10.3934/biophy.2020003 |
[3] | Irina A. Zamulaeva, Kristina A. Churyukina, Olga N. Matchuk, Alexander A. Ivanov, Vyacheslav O. Saburov, Alexei L. Zhuze . Dimeric bisbenzimidazoles DB(n) in combination with ionizing radiation decrease number and clonogenic activity of MCF-7 breast cancer stem cells. AIMS Biophysics, 2020, 7(4): 339-361. doi: 10.3934/biophy.2020024 |
[4] | Reham Ebrahim, Aya Abdelrazek, Hamed El-Shora, Abu Bakr El-Bediwi . Effect of ultraviolet radiation on molecular structure and photochemical compounds of Salvia hispanica medical seeds. AIMS Biophysics, 2022, 9(2): 172-181. doi: 10.3934/biophy.2022015 |
[5] | Soad Hasanin, A. G. ELshahawy, Hamed M El-Shora, Abu Bakr El-Bediwi . Gamma radiation effects on vitamins, antioxidant, internal and molecular structure of Purslane seeds. AIMS Biophysics, 2022, 9(3): 246-256. doi: 10.3934/biophy.2022021 |
[6] | Francesca Ballarini, Mario P. Carante, Alessia Embriaco, Ricardo L. Ramos . Effects of ionizing radiation in biomolecules, cells and tissue/organs: basic mechanisms and applications for cancer therapy, medical imaging and radiation protection. AIMS Biophysics, 2022, 9(2): 108-112. doi: 10.3934/biophy.2022010 |
[7] | Mohamed A. Elblbesy . The refractive index of human blood measured at the visible spectral region by single-fiber reflectance spectroscopy. AIMS Biophysics, 2021, 8(1): 57-65. doi: 10.3934/biophy.2021004 |
[8] | Erma Prihastanti, Sumariyah Sumariyah, Febiasasti Trias Nugraheni . Increasing growth of monobulb garlic through the application of corona glow discharge plasma radiation and organic fertilizers. AIMS Biophysics, 2024, 11(1): 85-96. doi: 10.3934/biophy.2024006 |
[9] | Tika Ram Lamichhane, Hari Prasad Lamichhane . Heat conduction by thyroid hormone receptors. AIMS Biophysics, 2018, 5(4): 245-256. doi: 10.3934/biophy.2018.4.245 |
[10] | C. Dal Lin, M. Falanga, E. De Lauro, S. De Martino, G. Vitiello . Biochemical and biophysical mechanisms underlying the heart and the brain dialog. AIMS Biophysics, 2021, 8(1): 1-33. doi: 10.3934/biophy.2021001 |
The purpose of this work is to interpret the experiences of students when audience response systems (ARS) were implemented as a strategy for teaching large mathematics lecture groups at university. Our paper makes several contributions to the literature. Firstly, we furnish a basic model of how ARS can form a teaching and learning strategy. Secondly, we examine the impact of this strategy on student attitudes of their experiences, focusing on the ability of ARS to: assess understanding; identify strengths and weaknesses; furnish feedback; support learning; and to encourage participation. Our findings support the position that there is a place for ARS as part of a strategy for teaching and learning mathematics in large groups.
Human pose estimation is a key technology in the field of computer vision. Its output is the basis of down-stream tasks such as action recognition, visual tracking and action analysis. The early work in human pose estimation was mainly limited to a 2D plane, and the goal is to get the body joints' 2D coordinates from 2D images or videos. In recent years, 3D body pose estimation has become popular because it provides more accurate data with depth information. 3D pose estimation can be categorized into three types, according to the input: from a monocular image [1,2,3,4], from multi-camera images [5,6] and from a depth image [7,8,9]. Monocular 3D human pose estimation is the most popular, and it is widely used in applications such as virtual reality, intelligent video analysis and human-computer interaction.
At present, there are two main branches for monocular 3D human pose estimation. One is the so-called two-stage method, which first estimates the 2D human pose and then lifts it to a 3D human pose. An example is the weakly supervised model [10]. The other is the end-to-end 3D human pose estimation method, which predicts the 3D human pose directly from images or videos. Examples are the adversarial learning method [11], the self-supervised approach [12] and the famous Transformer [13]. Because the human pose shows spatial correlation, some work tried to extract skeleton features in the spatial domain. Liu et al. [14] employed graph networks with weight sharing to do 3D pose estimation. The stacked graph hourglass model [15] tried to capture multi-scale spatial correlation. With the goal of capturing both the spatial and temporal correlation of a human pose, Zhang et al. [16] proposed a spatial-temporal encoder to learn spatial-temporal correlations. In comparison with the two-stage model, the end-to-end model regresses the 3D pose directly from the 2D image, which provides the model with rich information. However, it usually requires the support of large-scale human pose datasets. The 2D pose datasets includes Leeds Sports Pose Dataset (LSP) [17], Frames Labeled In Cinema (FLIC) [18], Max Planck Institut Informatik (MPII) [19] and Microsoft COCO: Common Objects in Context (MSCOCO) [20]. The 3D pose datasets include HumanEva [21], MPI-INF-3DHP and human 3.6M [22]. For 3D human poses, it is a very challenging task to obtain large-scale labels. Therefore, most of the existing data are collected in the laboratory using motion capture systems (such as human 3.6M), and the backgrounds are relatively simple and very limited in number. Due to these limitations, the end-to-end methods usually perform better in some specific scenarios but cannot generalize well to applications in natural scenes. In order to improve the model's generalizability, Gholami et. al. [23] proposed adapting the training data to the test dataset, such as camera viewpoint, position, human actions and body size.
The two-stage model estimates the 3D pose by two steps. It first gets a 2D pose by a 2D detector, and then regresses the 3D pose from the 2D. Obviously, the two-stage model will heavily rely on the 2D detector; however, the 2D pose datasets are more sufficient than 3D and contain much in-the-wild data. Thus, the 2D detector will be trained by more diverse data, and the two-stage model can be expected to show better generalizability. In addition, the two-stage model also has an advantage of low complexity. Therefore, in this paper, we adopt it to predict the 3D human pose with the 2D human skeleton as input.
The main contributions of this work include the following:
1) We design a multi-stage supervision temporal convolution network to capture human dynamics by temporal continuity constraints. In addition, the network is trained in a multi-stage supervision manner to improve the model.
2) We impose that the model is to be consistent with general human pose dynamic knowledge and introduce human body pose geometry to the network training step, so as to improve the model generality.
The task of 3D pose estimation is more challenging than 2D pose estimation, because it needs to regress relative depth between body joints, which suffers from severe ambiguity.
For the two-stage 3D pose estimation models, Martinez et. al. [24] designed a simple multi-layer network and regressed the 3D pose from the 2D pose skeleton. At first, this work analyzed the reasons for low accuracy in 3D pose estimation, and determined two aspects: the low accuracy of the 2D estimation, and the mapping from 2D to 3D. In fact, the 2D detector has achieved very high performance, so this paper focused on estimating the 3D pose from the 2D. Therefore, at second, they designed a very simple and lightweight network, and achieved good performance. Their work demonstrated the effectiveness of the two-stage model.
Based on Martinez's work, Fang et al. [25] extended it by a pose semantic network to code joints' dependency and correlations. Because recurrent neural network is good at learning temporal correlations, it was also introduced in Martinez's model. Hossian and Little [26] used Long short-term memory (LSTM) to capture the temporal continuity and achieved accuracy improvement. However, this model cannot deal with long-term sequential data, because it will lead to the gradient vanishing and gradient explosion problems.
Temporal convolution networks provide a new way to capture temporal continuity, so they are also used in the field of pose estimation. WaveNet [27] proved the convolution model's advantages in capturing temporal information. WaveNet is constructed by 1D convolution, and it can prevent the problems of gradient vanishing and gradient explosion. In addition, it is of high efficiency, because it can process temporal data in parallel. Based on this model, Pavllo et. al. [28] designed a temporal convolution model to estimate 3D body pose. This model generates the 2D pose sequence first by the 2D detector and then estimates the 3D pose. In comparison with WaveNet, the temporal convolution model is advantaged in learning the implicit kinematics knowledge. Instead of estimating 3D human pose from monocular images, videos can provide temporal information to improve accuracy and robustness. Several works [29,30,31] utilized spatial-temporal relationships and constraints such as bone-length and left-right symmetry to improve performance. In this paper, we employed the temporal convolution model and improved it in two ways: First, we design a multi-stage supervision model to further explore the periodic motion pattern; second, we introduce the prior geometry knowledge to generalize the model.
The performance of data-driven model is limited by the dataset, so prior knowledge is imposed to the deep models in many computer vision fields [32,33,34]. With the goal of decreasing depth ambiguity, some work tried to introduce human geometric knowledge into the 3D pose estimation model. Belagiannis et. al. [35] imposed kinematic constraints on the translation and rotation between body parts in the 3D pictorial model, and the symmetric body parts are constrained not to collide with each other. Ronchi et. al. [36] imposed limb length loss and measured the difference in length between the predicted limb and the predefined reference length. In fact, we can develop many kinematic constraints, such as limb lengths, limb length proportions, joint angles, occlusion constraints, appearance constraints and temporal smoothness constraints. Kinematics is crucial prior knowledge for the deep models, and it constrains the model predictions to be reasonable when measured by body geometry.
In the temporal domain, human movement always shows continuity according to human kinematics. If we can capture the temporal continuity, it will provide important information for the 3D pose estimation model. In this paper, we employed the temporal convolution network to model the periodical human kinematics.
The model structure is shown in Figure 1. The network takes a 2D human pose sequence of size 243×34 (17 joints' 2D pose coordinates, and 243 is the sequence length) as input. The input sequence is passed through four same consecutive modules, which are composed of a 1D convolution with 3 convolution kernels and 1024 output channels, batch normalization layer, Rectified Linear Unit (ReLu) and dropout. Each module is added by a residual connection, as shown in the upper part of Figure 1. The dimension of the input data is directly reduced through a specific slice function (Slice), and the results are added to the output data of the module. The channel number is 1024 in the module. Each module contains two different convolution layers. The first convolution layer applies extended convolution, which is mainly used to extract data features. The kernel size is 3, and the expansion rate is 3 (3d3 in the figure). With the increase of modules, the kernel size is fixed, but the expansion rate increases exponentially. As shown in this figure, the expansion rate of the second module is 9, while it is 27 for the third and 81 for the last. Different from the first convolution layer, the kernel size for the second one is 1, which is used to increase the depth of the network and improve the nonlinearity. At the end of the network, a convolution layer is applied to output 3D human posture.
In order to avoid the gradient vanishing problem, we add residual connection to enhance the gradient propagation. As shown in Figure 1, we add multi-level residual connection to supervise the network in multiple stages. In the process of forward propagation, multi-level residual connection enables shallow features to be directly propagated to the upper layer. The features of the shallow layer are combined with high-level features as input to the next layer. Combining features at different levels helps to reduce network degradation and improve network generalization performance. In the process of back propagation, the gradient can be transmitted to the lower layer faster without too much intermediate weight matrix transformation, so it can effectively alleviate gradient vanishing. The time-series convolution network based on multi-stage supervision makes the feature information more smoothly spread in the forward and backward directions, so the network has better optimization performance, which will further improve the model accuracy.
The input is the joints' 2D coordinates of the consecutive frames x2d(x∈R2n), and the model will output the joints' 3D coordinate estimations (y3d,y∈R3n). The loss function is defined as the Euclidean distance between the estimated 3D pose and the ground-truth (yt,y∈R3n):
Lmpjpe=1N∑N||y3d−yt|| | (1) |
in which N is the batch size and is set to be 1024 in our case. We use gradient descent to optimize the model and exponential decay to update the learning rate. The learning rate is set to be 0.001, and the decay rate is 0.95.
Relying on joints' coordinates only tends to cause ambiguity when restoring 3D coordinates from 2D. Therefore, the geometric prior knowledge of human kinematics is introduced in this part. We employed the distance Lp−mpjpe between the ground-truth coordinates and the estimated joints' coordinates after translation, rotation and scale transformation.
Lp−mpjpe=1N∑N||T(y3d)−yt|| | (2) |
where T is the transformation operation. In addition, we also introduced the geometry consistency as constraints, including bone length symmetry and proportions. The skeleton of a normal human body is symmetrical: for example, the bone length of the left shoulder is the same as that of the right shoulder.
The bone length symmetry constraint:
Lsym=∑i1|Si|∑e∈Si(le−lsym(e))2 | (3) |
The bones connecting human joints are divided into four groups S={Sarm,Sleg,Sshoulder,Ship}. Sarm includes left and right upper arms and left and right lower arms. Sleg includes the left and right thighs and the left and right calves. Sshoulder contains the left shoulder bone and the right shoulder bone, and Ship contains the left hip bone and the right hip bone. le is the length of the selected bone. The length of the bone at the symmetrical position is obtained through the sym function. For example, if le is the right thigh, lsym(e) will be the left thigh. lsym controls the bone length by calculating the error of each group of bones and constrains the bone length at symmetrical positions.
The bone length proportions constraint:
Lrat=∑i1|Ri|∑e∈Ri(le¯le−¯ri)2 | (4) |
¯ri=1|Ri|∑e∈Rile¯le | (5) |
The bones are also grouped into four groups R={Rarm,Rleg,Rshoulder,Rhip}. le is the bone length, ¯le is its corresponding mean length in its datasets. The proportion le/¯le should be consistent in the same group. ¯ri is the mean proportion in group i.
The constraints and the accuracy loss are integrated as the final loss:
L=λ1Lmpjpe+λ2Lp−mpjpe+λ3Lsym+λ4Lrat | (6) |
in which λ1,λ2,λ3,λ4 are the weights of each constraint.
We tested our method on the Human3.6M and MPI-INF-3DHP datasets. The Human3.6M dataset contains 15 actions of 11 testers, with a total of 3.6 million video frames. For the task of 3D human pose estimation, there are mainly three standard evaluation protocols based on this dataset: Protocol 1 (MPJPE) is the average joint position error in millimeters, which is the Euclidean distance between the predicted joint position and the real position. Protocol 2 (P-MPJPE) is the error after the predicted joint position is aligned with the real position after translation, rotation and retraction. Protocol 3 (N-MPJPE) is the error after aligning the predicted joint position with the real position only after scaling. Among the three protocols, Protocol 1 (MPJPE) is the most widely used. However, for the method of predicting 3D human pose based on sequence, absolute position error cannot measure the smoothness of prediction over time. In order to evaluate this, Pavllo et al. [28] measured the joint velocity error (MPJVE), which is a time-based velocity motion measurement and the first-order derivative of MPJPE's 3D pose error. For the Human 3.6M dataset, we employed the 17 joint skeletons, used 5 testers (S1, S5, S6, S7, S8) for training and 2 testers (S9, S11) for testing and trained a general model for 15 actions. The MPI-INF-3DHP test set [37] provides images in three different scenarios: studio with a green screen (GS), studio without green screen (noGS) and outdoor scene (Out- door). We use this dataset to test the generalization ability of our model and use 3D-PCK and AUC as evaluation metrics.
In this section, we employ the 2D ground-truth data (2D skeletons of 243 frames) as the input to the second stage. The channel number is set to be 1024. As shown in Tables 1–3, the proposed model with multi-stage intermediate supervision achieved lower error when evaluated by all three protocols.
Dir. | Disc. | Eat | Greet | Phone | Photo | Pose | Purch. | |
Pavllo et al. [28] | 26.0 | 29.8 | 24.6 | 27.0 | 25.8 | 29.4 | 29.2 | 26.7 |
Ours | 25.7 | 29.2 | 25.1 | 27.0 | 25.8 | 30.4 | 28.7 | 25.9 |
Sit. | SitD. | Smoke | Wait | WalkD | Walk | WalkT | Avg | |
Pavllo et al. [28] | 31.7 | 34.6 | 27.4 | 27.3 | 27.9 | 21.5 | 21.8 | 27.4 |
Ours | 30.7 | 35.0 | 27.2 | 26.8 | 27.7 | 21.4 | 22.6 | 27.3 |
Dir. | Disc. | Eat | Greet | Phone | Photo | Pose | Purch. | |
Pavllo et al. [28] | 26.0 | 29.8 | 24.6 | 27.0 | 25.8 | 29.4 | 29.2 | 26.7 |
Ours | 25.7 | 29.2 | 25.1 | 27.0 | 25.8 | 30.4 | 28.7 | 25.9 |
Sit. | SitD. | Smoke | Wait | WalkD | Walk | WalkT | Avg | |
Pavllo et al. [28] | 31.7 | 34.6 | 27.4 | 27.3 | 27.9 | 21.5 | 21.8 | 27.4 |
Ours | 30.7 | 35.0 | 27.2 | 26.8 | 27.7 | 21.4 | 22.6 | 27.3 |
Dir. | Disc. | Eat | Greet | Phone | Photo | Pose | Purch. | |
Pavllo et al. [28] | 36.0 | 39.1 | 31.4 | 35.6 | 33.5 | 38.0 | 40.5 | 34.7 |
Ours | 34.5 | 37.4 | 31.7 | 34.8 | 33.5 | 39.1 | 39.2 | 33.2 |
Sit. | SitD. | Smoke | Wait | WalkD | Walk | WalkT | Avg | |
Pavllo et al. [28] | 41.8 | 41.4 | 34.9 | 36.8 | 34.5 | 26.4 | 27.1 | 35.5 |
Ours | 40.1 | 40.9 | 33.9 | 35.4 | 34.3 | 26.6 | 27.7 | 34.8 |
Table 1 shows the evaluation by Protocol 1 (MPJPE). The proposed method shows performance improvement on most actions, even for some relatively difficult actions, such as "sitting", "sittingD", and "Discussion". Averagely, the error is reduced by about 1 mm, and the prediction accuracy is increased by 2.7%. Table 2 shows the evaluation by Protocol 2 (P-MPJPE). The proposed model achieved 0.1 mm error reduction and about 1 mm for the actions of "Sitting". Table 3 is based on Protocol 3 (N-MPJPE), where the proposed model achieved about 0.7 mm error reduction and about 1.7 mm for the actions of "Sitting" and "Discussion". The proposed model also achieved lower error in joint velocity, as shown in Table 4, which means better temporal smoothness.
Dir. | Disc. | Eat | Greet | Phone | Photo | Pose | Purch. | |
Pavllo et al. [28] | 1.92 | 1.97 | 1.48 | 2.27 | 1.42 | 1.79 | 1.85 | 2.16 |
Ours | 1.92 | 1.94 | 1.45 | 2.24 | 1.39 | 1.75 | 1.80 | 2.11 |
Sit. | SitD. | Smoke | Wait | WalkD | Walk | WalkT | Avg | |
Pavllo et al. [28] | 1.11 | 1.53 | 1.40 | 1.59 | 2.68 | 2.29 | 1.91 | 1.83 |
Ours | 1.07 | 1.49 | 1.37 | 1.56 | 2.64 | 2.27 | 1.90 | 1.79 |
In order to test performance of regressing the 3D pose directly from the 2D image, we employed a Cascade Pyramid Network (CPN) [38] as the 2D detector in the two-stage model, and the predicted 2D skeletons' sequence is input to the 3D estimator. Table 5 compares the proposed model with the state-of-the-art models, where "U" is the model with multi-stage supervision, and "U+L" is the model with multi-stage supervision and geometry constraints. Our method achieved the best result on almost all the actions. For the actions "Phone" and "Photo", our model performs worse than the baseline model. For these two actions, the kinematics feature is not as obvious as other actions in both spatial and temporal domain, especially for the action "Phone, " so our model did not show advantages. For the action "Photo, " the "U+L" model performs better than "U" model, which means the geometry constraints are effective for this action.
Dir. | Disc. | Eat | Greet | Phone | Photo | Pose | Purch. | |
Martinez et al. [24] | 51.8 | 56.2 | 58.1 | 59.0 | 69.5 | 78.4 | 55.2 | 58.1 |
Sun et al. [39] | 52.8 | 54.8 | 54.2 | 54.3 | 61.8 | 67.2 | 53.1 | 53.6 |
Fang et al. [25] | 50.1 | 54.3 | 57.0 | 57.1 | 66.6 | 73.3 | 53.4 | 55.7 |
Pavlakos et al. [40] | 48.5 | 54.4 | 54.4 | 52.0 | 59.4 | 65.3 | 49.9 | 52.9 |
Yang et al. [41] | 51.5 | 58.9 | 50.4 | 57.0 | 62.1 | 65.4 | 49.8 | 52.7 |
Luvizon et al. [42] | 49.2 | 51.6 | 47.6 | 50.5 | 51.8 | 60.3 | 48.5 | 51.7 |
Hossain and Little [26] | 48.4 | 50.7 | 57.2 | 55.2 | 63.1 | 72.6 | 53.0 | 51.7 |
Lee et al. [43] | 40.2 | 49.2 | 47.8 | 52.6 | 50.1 | 75.0 | 50.2 | 43.0 |
Pavllo et al. [28] | 45.2 | 46.7 | 43.3 | 45.6 | 48.1 | 55.1 | 44.6 | 44.3 |
Liu et al. [14] | 46.3 | 52.2 | 47.3 | 50.7 | 55.5 | 67.1 | 49.2 | 46.0 |
Xu and Takano [15] | 45.2 | 49.9 | 47.5 | 50.9 | 54.9 | 66.1 | 48.5 | 46.3 |
Ours (U) | 44.6 | 46.5 | 43.0 | 45.4 | 48.4 | 57.3 | 43.9 | 43.7 |
Ours (U+L) | 44.3 | 46.1 | 42.5 | 45.2 | 48.4 | 56.0 | 43.9 | 43.5 |
Sit. | SitD. | Smoke | Wait | WalkD. | Walk | WalkT | Avg. | |
Martinez et al. [24] | 74.0 | 94.6 | 62.3 | 59.1 | 65.1 | 49.5 | 52.4 | 62.9 |
Sun et al. [39] | 71.7 | 86.7 | 61.5 | 53.4 | 61.6 | 47.1 | 53.4 | 59.1 |
Fang et al. [25] | 72.8 | 88.6 | 60.3 | 57.7 | 62.7 | 47.5 | 50.6 | 60.4 |
Pavlakos et al. [40] | 65.8 | 71.1 | 56.6 | 52.9 | 60.9 | 44.7 | 47.8 | 56.2 |
Yang et al. [41] | 69.2 | 85.2 | 57.4 | 58.4 | 43.6 | 60.1 | 47.7 | 58.6 |
Luvizon et al. [42] | 61.5 | 70.9 | 53.7 | 48.9 | 57.9 | 44.4 | 48.9 | 53.2 |
Hossain and Little [26] | 66.1 | 80.9 | 59.0 | 57.3 | 62.4 | 46.6 | 49.6 | 58.3 |
Lee et al. [43] | 55.8 | 73.9 | 54.1 | 55.6 | 58.2 | 43.3 | 43.3 | 52.8 |
Pavllo et al. [28] | 57.3 | 65.8 | 47.1 | 44.0 | 49.0 | 32.8 | 33.9 | 46.8 |
Liu et al. [14] | 60.4 | 71.1 | 51.5 | 50.1 | 54.5 | 40.3 | 43.7 | 52.7 |
Xu and Takano [15] | 59.7 | 71.5 | 51.4 | 48.6 | 53.9 | 39.9 | 44.1 | 51.9 |
Ours (U) | 56.6 | 64.3 | 47.0 | 43.9 | 49.2 | 32.7 | 33.7 | 46.7 |
Ours (U+L) | 56.7 | 64.6 | 45.6 | 43.6 | 48.9 | 32.6 | 33.7 | 46.4 |
With predicted 2D pose as input, the model shows less accuracy than the model with ground-truth 2D pose as input. As shown in Table 5, the average prediction error is reduced by about 2.3%. In comparison, the "U+L" model shows better performance than the "U" model, and averagely achieved 0.8% improvement. The model with "U+L" achieved the lowest error on almost all the actions. We can see that the "U+L" model has the same number of parameters as the "U" model but better generalization ability.
We applied the model trained on Human 3.6m to the MPI-INF-3DHP dataset, to test the model's generalizability. Table 6 shows the results and comparison with the state-of-the-art models. Trained only on the Human 3.6M dataset, our model shows good generalizability, due to the general knowledge being data independent.
Training Data | GS | noGS | Outdoor | All (PCK) | All (AUC) | |
Martinez et al. [24] | Human3.6M | 49.8 | 42.5 | 31.2 | 42.5 | 17.0 |
Zhou et al. [44] | Human3.6M+MPII | 75.6 | 71.3 | 80.3 | 75.3 | 38.0 |
Xu and Takano [15] | Human3.6M | 81.5 | 81.7 | 75.2 | 80.1 | 45.8 |
Ours (U) | Human 3.6M | 81.7 | 81.6 | 75.4 | 80.2 | 46.0 |
Ours (U+L) | Human3.6M | 81.6 | 81.7 | 75.0 | 80.1 | 45.8 |
In addition to the above quantitative experimental results, we also visualize the 3D pose results for the Human3.6M dataset. Figure 2 shows the prediction effects of some actions, including eating, talking on the phone and smoking. It can be seen from the figure that the proposed model effectively restores the human pose in 3D space with a high prediction accuracy.
For 3D pose estimation, this paper proposed developing human kinematics in two ways. First, we employed the temporal convolution network to extract the temporal continuity and supervised by constructing multi-stage intermediate connections to alleviate gradient vanishing. Second, we introduced geometry constraints to improve the model generalizability. When tested on two public datasets, the proposed model showed comparable performance with the state-of-the-art models. Developing human kinematics is important information for a data-driven model. This paper presents a preliminary study, and developing more kinematics will provide the data-driven model with more effective prior knowledge, which is also our future work.
We would like to thank for the support by the Science and Technology Development Program of Jilin Province (20220101102JC) and Jilin Province Professional Degree Postgraduate Teaching Case Construction Project.
The authors declare there is no conflict of interest.
[1] | Likert scales and data analyses. Quality Progress (2007) 40: p. 64-65. |
[2] | Archer, M.S., Bhaskar, R., Collier, A., Lawson, T., Norrie, A. Critical Realism: Essential Readings. 2009, London, UK: Routledge. |
[3] | Bagley, S.F., Improving student success in calculus: a comparison of four college calculus classes[dissertation]. 2014, San Diego State University: San Diego, USA. |
[4] | Baker, J.W., The 'classroom flip': using web course management tools to become the guide by the side, in Selected Papers from the 11th International Conference on College Teaching and Learning. 2001, Florida Community College at Jacksonville: Jacksonville (FL), p. 9–17. |
[5] | Banks, D.A., Audience Response Systems in Higher Education: Applications and Cases. 2006, Hershey, PA, USA: Information Science Publishing. |
[6] | Berends, M., Survey methods in educational research, in Handbook of Complementary Methods in Education Research, J.L. Green, G. Camilli, P.B. Elmore, Ed. 2006, Lawrence Erlbaum Associates. p. 623-640. Retrieved from http://psycnet.apa.org/record/2006-05382-038 |
[7] | Bligh, D.A., What's the Use of Lectures? 1972, Harmondsworth, UK: Penguin Books. |
[8] | Bonwell, C.C., Eison, J.A., Active Learning: Creating Excitement in the Classroom. 2005, San Francisco, USA: Jossey-Bass. |
[9] | Box, G.E., Robustness in the Strategy of Scientific Model Building. 1979, Ft. Belvoir: Defense Technical Information Center. Retrieved from http://www.dtic.mil/docs/citations/ADA070213 |
[10] |
Exploring student perceptions, learning outcome and gender differences in a flipped mathematics course. British Journal of Educational Technology (2016) 47: p. 1096-1112. ![]() |
[11] | Codecogs. Retrieved from https://www.codecogs.com/latex/eqneditor.php?latex=D |
[12] | Coe, R., Waring, M., Hedges, L.V., Arthur, J., Research Methods and Methodologies in Education. 2017, Los Angeles, CA: SAGE. |
[13] | Cohen, J., Statistical Power Analysis for the Behavioral Sciences. 1988, Abingdon-on-Thames, UK: Routledge. |
[14] | Things I have learned (so far). Am Psychol (1990) p. 1304-1312. |
[15] | Cohen, L., Manion, L., Morrison, K., Research Methods in Education. 2018, London: Routledge. |
[16] | Creswell, J.W., Qualitative Inquiry and Research Design: Choosing among Five Approaches. 2007, Thousand Oaks, CA: Sage. |
[17] | Cronhjort, M., Filipsson, L., Weurlander, M., Improved engagement and learning in flipped-classroom calculus. Teaching Mathematics and its Applications: An International Journal of the IMA, 2018. 37(3): p. 113–121. doi: 10.1093/teamat/hrx007. |
[18] | Day A.L., Case study research, in Research Methods & Methodologies in Education, 2nd ed. R. Coe, M. Waring, L.V. Hedges, J. Arthur, Ed. 2017, Los Angeles, CA: SAGE. p. 114-121. |
[19] | Duncan, D., Clickers in the Classroom: How to Enhance Science Teaching Using Classroom Response Systems. 2005, San Francisco, CA: Pearson Education. |
[20] |
Instructor perceptions of using a mobile-phone-based free classroom response system in first-year statistics undergraduate courses. International Journal of Mathematical Education in Science and Technology (2012) 43: p. 1041-1056. ![]() |
[21] |
Mobile-phone-based classroom response systems: Students' perceptions of engagement and learning in a large undergraduate course. International Journal of Mathematical Education in Science and Technology (2013) 44: p. 1160-1174. ![]() |
[22] | Google, Create forms. 2017. Retrieved from https://www.google.com.au/forms/about/ |
[23] | Higher Education Research & Development Society of Australasia, HERDSA Fellowship Scheme Handbook. 2014, Milperra, NSW: HERSDSA. Retrieved from https://www.herdsa.org.au/sites/default/files/Fellowship\%20Handbook_6_5_2014.pdf |
[24] |
Implementing a flipped classroom approach in a university numerical methods mathematics course. International Journal of Mathematical Education in Science and Technology (2017) 48: p. 485-498. ![]() |
[25] |
On flipping the classroom in large first year calculus courses. International Journal of Mathematical Education in Science and Technology (2015) 46: p. 508-520. ![]() |
[26] |
'Pretty lights' and maths! Increasing student engagement and enhancing learning through the use of electronic voting systems. Computers & Education (2009) 53: p. 189-199. ![]() |
[27] | Kline, R.B., Beyond Significance Testing: Reforming Data Analysis Methods in Behavioral Research. 2004, Washington, DC: American Psychological Association, p. 95. |
[28] |
Mathematics education and mobile technologies. Math Ed Res J (2016) 28: p. 1-7. ![]() |
[29] | Lomen, D.O., Robinson, M.K., Using ConcepTests in single and multivariable calculus, in Electronic Proceedings of the Sixteenth Annual International Conference on Technology in Collegiate Mathematics. 2005. Retrieved October 17, 2017 from http://archives.math.utk.edu/ICTCM/i/16/S107.html |
[30] |
Student learning and perceptions in a flipped linear algebra course. International Journal of Mathematical Education in Science and Technology (2014) 45: p. 317-324. ![]() |
[31] |
Flipping the calculus classroom: an evaluative study. Teaching Mathematics and its Applications (2016) 35: p. 187-201. ![]() |
[32] | Markie, P., Rationalism vs. Empiricism. 2017. Retrieved from https://plato.stanford.edu/entries/rationalism-empiricism/ |
[33] |
Student performance and attitudes in a collaborative and flipped linear algebra course. International Journal of Mathematical Education in Science and Technology (2015) 47: p. 653-673. ![]() |
[34] |
Expectations and implementations of the flipped classroom model in undergraduate mathematics courses. International Journal of Mathematical Education in Science and Technology (2015) 46: p. 968-978. ![]() |
[35] | von Neumann, J., The mathematician, in Works of the Mind, R.B., Haywood Ed. 1947, Chicago: University of Chicago Press. p. 180-196. |
[36] | Novak, J., Kensington-Miller, B., Evans, T., Flip or flop? Students' perspectives of a flipped lecture in mathematics. International Journal of Mathematical Education in Science and Technology, 2017. 48(5): p. 647-658. doi: 10.1080/0020739x.2016.1267810. |
[37] | Center for Educational Research and Innovation, Giving Knowledge for Free: The Emergence of Open Educational Resources. Retrieved from http://www.oecd.org/edu/ceri/38654317.pdf |
[38] |
On flipping first-semester calculus: a case study. International Journal of Mathematical Education in Science and Technology (2016) 47: p. 573-582. ![]() |
[39] | Robson, L., Guide to Evaluating the Effectiveness of Strategies for Preventing Work Injuries: How to Show Whether a Safety Intervention Really Works. 2001, Cincinnati, OH: DHHS. |
[40] |
Smartphones as audience response systems for lectures and seminars. Analytical and Bioanalytical Chemistry (2018) 410: p. 1609-1613. ![]() |
[41] |
New effect size rules of thumb. Journal of Modern Applied Statistical Methods (2009) 8: p. 467-474. ![]() |
[42] | Shadish, W.R., Cook, T.D., Campbell, D.T., Experimental and Quasi-experimental Designs for Generalized Causal Inference. 2001, Belmont, CA: Wadsworth Cengage Learning. |
[43] | Analyzing and interpreting data from Likert-type scales. Journal of Graduate Medical Education (2013) 5: p. 541-542. |
[44] |
Critical perspectives of pedagogical approaches to reversing the order of integration in double integrals. International Journal of Mathematical Education in Science and Technology (2017) 48: p. 1285-1292. ![]() |
[45] |
Pedagogical alternatives for triple integrals: moving towards more inclusive and personalized learning. International Journal of Mathematical Education in Science and Technology (2018) 49: p. 792-801. ![]() |
[46] |
On Picard's iteration method to solve differential equations and a pedagogical space for otherness. International Journal of Mathematical Education in Science and Technology (2019) 50: p. 788-799. ![]() |
[47] | Schoenfeld's problem-solving models viewed through the lens of exemplification. For the Learning of Mathematics (2019) 39: p. 24-26. |
[48] | Trochim, W.M.K., The Research Methods Knowledge Base. 2006. Retrieved from https://www.socialresearchmethods.net/kb/positvsm.php |
[49] | University of Edinburgh. What is digital education? 2019. Retrieved from https://www.ed.ac.uk/institute-academic-development/learning-teaching/staff/digital-ed/what-is-digital-education |
[50] | Wang, V.C., Handbook of Research on e-learning Applications for Career and Technical Education: Technologies for Vocational Training. 2009, Hershey, PA: IGI Global. |
[51] | Wasserman, N., Norris, S., Carr, T., Comparing a "flipped" instructional model in an undergraduate Calculus Ⅲ course, in Proceedings of the 16th Annual Conference on Research in Undergraduate Mathematics Education; S. Brown, G. Karakok, K.H. Roh, M. Oehrtman Ed..2013, Denver, CO. |
[52] | Yin, R.K., Case Study Research: Design and Methods. 4th ed. 2009, Thousand Oaks, CA: Sage. |
1. | Akankshya Priyadarshini, Mihir Kumar Sutar, Sarojrani Pattnaik, Investigation of mechanical properties on functionally graded material reinforced with graphene nanoplatelets for biomedical applications, 2024, 0927-6440, 1, 10.1080/09276440.2024.2406164 | |
2. | T. S. Mohan Kumar, Sharnappa Joladarashi, S. M. Kulkarni, Saleemsab Doddamani, Influence of sea sand reinforcement on the static and dynamic properties of functionally graded epoxy composites, 2024, 33, 1026-1265, 1609, 10.1007/s13726-024-01340-7 |
Dir. | Disc. | Eat | Greet | Phone | Photo | Pose | Purch. | |
Pavllo et al. [28] | 26.0 | 29.8 | 24.6 | 27.0 | 25.8 | 29.4 | 29.2 | 26.7 |
Ours | 25.7 | 29.2 | 25.1 | 27.0 | 25.8 | 30.4 | 28.7 | 25.9 |
Sit. | SitD. | Smoke | Wait | WalkD | Walk | WalkT | Avg | |
Pavllo et al. [28] | 31.7 | 34.6 | 27.4 | 27.3 | 27.9 | 21.5 | 21.8 | 27.4 |
Ours | 30.7 | 35.0 | 27.2 | 26.8 | 27.7 | 21.4 | 22.6 | 27.3 |
Dir. | Disc. | Eat | Greet | Phone | Photo | Pose | Purch. | |
Pavllo et al. [28] | 26.0 | 29.8 | 24.6 | 27.0 | 25.8 | 29.4 | 29.2 | 26.7 |
Ours | 25.7 | 29.2 | 25.1 | 27.0 | 25.8 | 30.4 | 28.7 | 25.9 |
Sit. | SitD. | Smoke | Wait | WalkD | Walk | WalkT | Avg | |
Pavllo et al. [28] | 31.7 | 34.6 | 27.4 | 27.3 | 27.9 | 21.5 | 21.8 | 27.4 |
Ours | 30.7 | 35.0 | 27.2 | 26.8 | 27.7 | 21.4 | 22.6 | 27.3 |
Dir. | Disc. | Eat | Greet | Phone | Photo | Pose | Purch. | |
Pavllo et al. [28] | 36.0 | 39.1 | 31.4 | 35.6 | 33.5 | 38.0 | 40.5 | 34.7 |
Ours | 34.5 | 37.4 | 31.7 | 34.8 | 33.5 | 39.1 | 39.2 | 33.2 |
Sit. | SitD. | Smoke | Wait | WalkD | Walk | WalkT | Avg | |
Pavllo et al. [28] | 41.8 | 41.4 | 34.9 | 36.8 | 34.5 | 26.4 | 27.1 | 35.5 |
Ours | 40.1 | 40.9 | 33.9 | 35.4 | 34.3 | 26.6 | 27.7 | 34.8 |
Dir. | Disc. | Eat | Greet | Phone | Photo | Pose | Purch. | |
Pavllo et al. [28] | 1.92 | 1.97 | 1.48 | 2.27 | 1.42 | 1.79 | 1.85 | 2.16 |
Ours | 1.92 | 1.94 | 1.45 | 2.24 | 1.39 | 1.75 | 1.80 | 2.11 |
Sit. | SitD. | Smoke | Wait | WalkD | Walk | WalkT | Avg | |
Pavllo et al. [28] | 1.11 | 1.53 | 1.40 | 1.59 | 2.68 | 2.29 | 1.91 | 1.83 |
Ours | 1.07 | 1.49 | 1.37 | 1.56 | 2.64 | 2.27 | 1.90 | 1.79 |
Dir. | Disc. | Eat | Greet | Phone | Photo | Pose | Purch. | |
Martinez et al. [24] | 51.8 | 56.2 | 58.1 | 59.0 | 69.5 | 78.4 | 55.2 | 58.1 |
Sun et al. [39] | 52.8 | 54.8 | 54.2 | 54.3 | 61.8 | 67.2 | 53.1 | 53.6 |
Fang et al. [25] | 50.1 | 54.3 | 57.0 | 57.1 | 66.6 | 73.3 | 53.4 | 55.7 |
Pavlakos et al. [40] | 48.5 | 54.4 | 54.4 | 52.0 | 59.4 | 65.3 | 49.9 | 52.9 |
Yang et al. [41] | 51.5 | 58.9 | 50.4 | 57.0 | 62.1 | 65.4 | 49.8 | 52.7 |
Luvizon et al. [42] | 49.2 | 51.6 | 47.6 | 50.5 | 51.8 | 60.3 | 48.5 | 51.7 |
Hossain and Little [26] | 48.4 | 50.7 | 57.2 | 55.2 | 63.1 | 72.6 | 53.0 | 51.7 |
Lee et al. [43] | 40.2 | 49.2 | 47.8 | 52.6 | 50.1 | 75.0 | 50.2 | 43.0 |
Pavllo et al. [28] | 45.2 | 46.7 | 43.3 | 45.6 | 48.1 | 55.1 | 44.6 | 44.3 |
Liu et al. [14] | 46.3 | 52.2 | 47.3 | 50.7 | 55.5 | 67.1 | 49.2 | 46.0 |
Xu and Takano [15] | 45.2 | 49.9 | 47.5 | 50.9 | 54.9 | 66.1 | 48.5 | 46.3 |
Ours (U) | 44.6 | 46.5 | 43.0 | 45.4 | 48.4 | 57.3 | 43.9 | 43.7 |
Ours (U+L) | 44.3 | 46.1 | 42.5 | 45.2 | 48.4 | 56.0 | 43.9 | 43.5 |
Sit. | SitD. | Smoke | Wait | WalkD. | Walk | WalkT | Avg. | |
Martinez et al. [24] | 74.0 | 94.6 | 62.3 | 59.1 | 65.1 | 49.5 | 52.4 | 62.9 |
Sun et al. [39] | 71.7 | 86.7 | 61.5 | 53.4 | 61.6 | 47.1 | 53.4 | 59.1 |
Fang et al. [25] | 72.8 | 88.6 | 60.3 | 57.7 | 62.7 | 47.5 | 50.6 | 60.4 |
Pavlakos et al. [40] | 65.8 | 71.1 | 56.6 | 52.9 | 60.9 | 44.7 | 47.8 | 56.2 |
Yang et al. [41] | 69.2 | 85.2 | 57.4 | 58.4 | 43.6 | 60.1 | 47.7 | 58.6 |
Luvizon et al. [42] | 61.5 | 70.9 | 53.7 | 48.9 | 57.9 | 44.4 | 48.9 | 53.2 |
Hossain and Little [26] | 66.1 | 80.9 | 59.0 | 57.3 | 62.4 | 46.6 | 49.6 | 58.3 |
Lee et al. [43] | 55.8 | 73.9 | 54.1 | 55.6 | 58.2 | 43.3 | 43.3 | 52.8 |
Pavllo et al. [28] | 57.3 | 65.8 | 47.1 | 44.0 | 49.0 | 32.8 | 33.9 | 46.8 |
Liu et al. [14] | 60.4 | 71.1 | 51.5 | 50.1 | 54.5 | 40.3 | 43.7 | 52.7 |
Xu and Takano [15] | 59.7 | 71.5 | 51.4 | 48.6 | 53.9 | 39.9 | 44.1 | 51.9 |
Ours (U) | 56.6 | 64.3 | 47.0 | 43.9 | 49.2 | 32.7 | 33.7 | 46.7 |
Ours (U+L) | 56.7 | 64.6 | 45.6 | 43.6 | 48.9 | 32.6 | 33.7 | 46.4 |
Training Data | GS | noGS | Outdoor | All (PCK) | All (AUC) | |
Martinez et al. [24] | Human3.6M | 49.8 | 42.5 | 31.2 | 42.5 | 17.0 |
Zhou et al. [44] | Human3.6M+MPII | 75.6 | 71.3 | 80.3 | 75.3 | 38.0 |
Xu and Takano [15] | Human3.6M | 81.5 | 81.7 | 75.2 | 80.1 | 45.8 |
Ours (U) | Human 3.6M | 81.7 | 81.6 | 75.4 | 80.2 | 46.0 |
Ours (U+L) | Human3.6M | 81.6 | 81.7 | 75.0 | 80.1 | 45.8 |
Dir. | Disc. | Eat | Greet | Phone | Photo | Pose | Purch. | |
Pavllo et al. [28] | 26.0 | 29.8 | 24.6 | 27.0 | 25.8 | 29.4 | 29.2 | 26.7 |
Ours | 25.7 | 29.2 | 25.1 | 27.0 | 25.8 | 30.4 | 28.7 | 25.9 |
Sit. | SitD. | Smoke | Wait | WalkD | Walk | WalkT | Avg | |
Pavllo et al. [28] | 31.7 | 34.6 | 27.4 | 27.3 | 27.9 | 21.5 | 21.8 | 27.4 |
Ours | 30.7 | 35.0 | 27.2 | 26.8 | 27.7 | 21.4 | 22.6 | 27.3 |
Dir. | Disc. | Eat | Greet | Phone | Photo | Pose | Purch. | |
Pavllo et al. [28] | 26.0 | 29.8 | 24.6 | 27.0 | 25.8 | 29.4 | 29.2 | 26.7 |
Ours | 25.7 | 29.2 | 25.1 | 27.0 | 25.8 | 30.4 | 28.7 | 25.9 |
Sit. | SitD. | Smoke | Wait | WalkD | Walk | WalkT | Avg | |
Pavllo et al. [28] | 31.7 | 34.6 | 27.4 | 27.3 | 27.9 | 21.5 | 21.8 | 27.4 |
Ours | 30.7 | 35.0 | 27.2 | 26.8 | 27.7 | 21.4 | 22.6 | 27.3 |
Dir. | Disc. | Eat | Greet | Phone | Photo | Pose | Purch. | |
Pavllo et al. [28] | 36.0 | 39.1 | 31.4 | 35.6 | 33.5 | 38.0 | 40.5 | 34.7 |
Ours | 34.5 | 37.4 | 31.7 | 34.8 | 33.5 | 39.1 | 39.2 | 33.2 |
Sit. | SitD. | Smoke | Wait | WalkD | Walk | WalkT | Avg | |
Pavllo et al. [28] | 41.8 | 41.4 | 34.9 | 36.8 | 34.5 | 26.4 | 27.1 | 35.5 |
Ours | 40.1 | 40.9 | 33.9 | 35.4 | 34.3 | 26.6 | 27.7 | 34.8 |
Dir. | Disc. | Eat | Greet | Phone | Photo | Pose | Purch. | |
Pavllo et al. [28] | 1.92 | 1.97 | 1.48 | 2.27 | 1.42 | 1.79 | 1.85 | 2.16 |
Ours | 1.92 | 1.94 | 1.45 | 2.24 | 1.39 | 1.75 | 1.80 | 2.11 |
Sit. | SitD. | Smoke | Wait | WalkD | Walk | WalkT | Avg | |
Pavllo et al. [28] | 1.11 | 1.53 | 1.40 | 1.59 | 2.68 | 2.29 | 1.91 | 1.83 |
Ours | 1.07 | 1.49 | 1.37 | 1.56 | 2.64 | 2.27 | 1.90 | 1.79 |
Dir. | Disc. | Eat | Greet | Phone | Photo | Pose | Purch. | |
Martinez et al. [24] | 51.8 | 56.2 | 58.1 | 59.0 | 69.5 | 78.4 | 55.2 | 58.1 |
Sun et al. [39] | 52.8 | 54.8 | 54.2 | 54.3 | 61.8 | 67.2 | 53.1 | 53.6 |
Fang et al. [25] | 50.1 | 54.3 | 57.0 | 57.1 | 66.6 | 73.3 | 53.4 | 55.7 |
Pavlakos et al. [40] | 48.5 | 54.4 | 54.4 | 52.0 | 59.4 | 65.3 | 49.9 | 52.9 |
Yang et al. [41] | 51.5 | 58.9 | 50.4 | 57.0 | 62.1 | 65.4 | 49.8 | 52.7 |
Luvizon et al. [42] | 49.2 | 51.6 | 47.6 | 50.5 | 51.8 | 60.3 | 48.5 | 51.7 |
Hossain and Little [26] | 48.4 | 50.7 | 57.2 | 55.2 | 63.1 | 72.6 | 53.0 | 51.7 |
Lee et al. [43] | 40.2 | 49.2 | 47.8 | 52.6 | 50.1 | 75.0 | 50.2 | 43.0 |
Pavllo et al. [28] | 45.2 | 46.7 | 43.3 | 45.6 | 48.1 | 55.1 | 44.6 | 44.3 |
Liu et al. [14] | 46.3 | 52.2 | 47.3 | 50.7 | 55.5 | 67.1 | 49.2 | 46.0 |
Xu and Takano [15] | 45.2 | 49.9 | 47.5 | 50.9 | 54.9 | 66.1 | 48.5 | 46.3 |
Ours (U) | 44.6 | 46.5 | 43.0 | 45.4 | 48.4 | 57.3 | 43.9 | 43.7 |
Ours (U+L) | 44.3 | 46.1 | 42.5 | 45.2 | 48.4 | 56.0 | 43.9 | 43.5 |
Sit. | SitD. | Smoke | Wait | WalkD. | Walk | WalkT | Avg. | |
Martinez et al. [24] | 74.0 | 94.6 | 62.3 | 59.1 | 65.1 | 49.5 | 52.4 | 62.9 |
Sun et al. [39] | 71.7 | 86.7 | 61.5 | 53.4 | 61.6 | 47.1 | 53.4 | 59.1 |
Fang et al. [25] | 72.8 | 88.6 | 60.3 | 57.7 | 62.7 | 47.5 | 50.6 | 60.4 |
Pavlakos et al. [40] | 65.8 | 71.1 | 56.6 | 52.9 | 60.9 | 44.7 | 47.8 | 56.2 |
Yang et al. [41] | 69.2 | 85.2 | 57.4 | 58.4 | 43.6 | 60.1 | 47.7 | 58.6 |
Luvizon et al. [42] | 61.5 | 70.9 | 53.7 | 48.9 | 57.9 | 44.4 | 48.9 | 53.2 |
Hossain and Little [26] | 66.1 | 80.9 | 59.0 | 57.3 | 62.4 | 46.6 | 49.6 | 58.3 |
Lee et al. [43] | 55.8 | 73.9 | 54.1 | 55.6 | 58.2 | 43.3 | 43.3 | 52.8 |
Pavllo et al. [28] | 57.3 | 65.8 | 47.1 | 44.0 | 49.0 | 32.8 | 33.9 | 46.8 |
Liu et al. [14] | 60.4 | 71.1 | 51.5 | 50.1 | 54.5 | 40.3 | 43.7 | 52.7 |
Xu and Takano [15] | 59.7 | 71.5 | 51.4 | 48.6 | 53.9 | 39.9 | 44.1 | 51.9 |
Ours (U) | 56.6 | 64.3 | 47.0 | 43.9 | 49.2 | 32.7 | 33.7 | 46.7 |
Ours (U+L) | 56.7 | 64.6 | 45.6 | 43.6 | 48.9 | 32.6 | 33.7 | 46.4 |
Training Data | GS | noGS | Outdoor | All (PCK) | All (AUC) | |
Martinez et al. [24] | Human3.6M | 49.8 | 42.5 | 31.2 | 42.5 | 17.0 |
Zhou et al. [44] | Human3.6M+MPII | 75.6 | 71.3 | 80.3 | 75.3 | 38.0 |
Xu and Takano [15] | Human3.6M | 81.5 | 81.7 | 75.2 | 80.1 | 45.8 |
Ours (U) | Human 3.6M | 81.7 | 81.6 | 75.4 | 80.2 | 46.0 |
Ours (U+L) | Human3.6M | 81.6 | 81.7 | 75.0 | 80.1 | 45.8 |