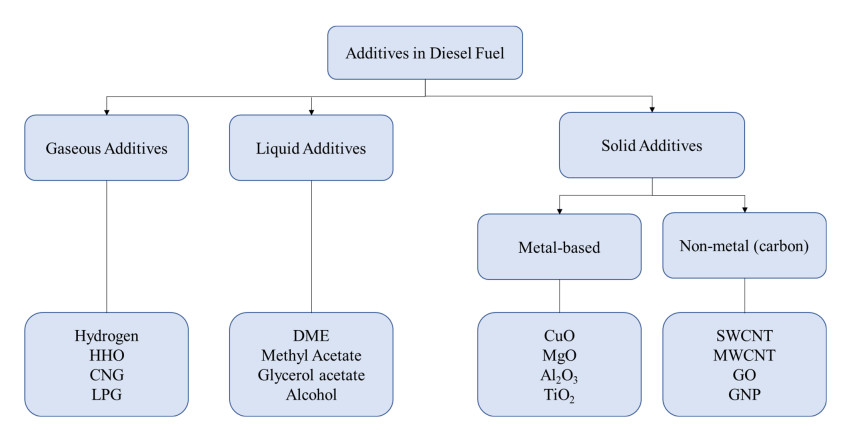
Approximation theory plays a central role in numerical analysis, evolving through a variety of methodologies, with significant contributions from Lebesgue, Weierstrass, Fourier, and Chebyshev approximations. For sufficiently smooth functions, the partial sum of Chebyshev series expansion provides optimal polynomial approximation, making it a preferred choice in many applications. However, existing literature predominantly focuses on Chebyshev interpolation, which requires exact Chebyshev series coefficients. The computation of these exact coefficients is challenging and often impractical for numerical algorithms, limiting their practical utility. Additionally, traditional approaches typically involve polynomials on fixed intervals where the basis functions of the series are defined. In this article, we have generalized Chebyshev polynomial approximation to a broader domain and presented two optimal error estimations for functions of bounded variation, using approximated Chebyshev series coefficients. This aspect is notably absent in current literature. To support our theoretical findings, we conducted numerical experiments and proposed future research directions, particularly in the fields of machine learning and related areas.
Citation: S. Akansha, Aditya Subramaniam. Exploring Chebyshev polynomial approximations: Error estimates for functions of bounded variation[J]. AIMS Mathematics, 2025, 10(4): 8688-8706. doi: 10.3934/math.2025398
[1] | Luz del Carmen Huesca-Espitia, J.L. Sánchez-Salas, Erick R. Bandala . Effect of Coat Layers in Bacillus Subtilis Spores Resistance to Photo-Catalytic Inactivation. AIMS Geosciences, 2017, 3(4): 514-525. doi: 10.3934/geosci.2017.4.514 |
[2] | Hareef Baba Shaeb Kannemadugu, Manish Verma, Dibyendu Dutta, Lianne Rachel Johnson, Srinivasa Rao, Seshasai MVR . Comparison of temperature and humidity profiles retrieved from INSAT-3DR sounder with high resolution radiosonde measurements. AIMS Geosciences, 2021, 7(2): 180-193. doi: 10.3934/geosci.2021011 |
[3] | José Luis Sánchez-Salas, Alejandra Aguilar Ubeda, Beatriz Flores Gómez, Oscar Daniel Máynez Navarro, Miguel Ángel Méndez Rojas, Silvia Reyna Tellez, Erick R. Bandala . Inactivation of Bacterial Spores and Vegetative Bacterial Cells by Interaction with ZnO-Fe2O3 Nanoparticles and UV Radiation. AIMS Geosciences, 2017, 3(4): 498-513. doi: 10.3934/geosci.2017.4.498 |
[4] | Kazuhisa A. Chikita . Environmental factors controlling stream water temperature in a forest catchment. AIMS Geosciences, 2018, 4(4): 192-214. doi: 10.3934/geosci.2018.4.192 |
[5] | Konstantinos X Soulis, Evangelos E Nikitakis, Aikaterini N Katsogiannou, Dionissios P Kalivas . Examination of empirical and Machine Learning methods for regression of missing or invalid solar radiation data using routine meteorological data as predictors. AIMS Geosciences, 2024, 10(4): 939-964. doi: 10.3934/geosci.2024044 |
[6] | Grant W Lowey . Very low-grade metamorphism of the Dezadeash Formation (Jura-Cretaceous): Constraints on the tectonometamorphic history of the Dezadeash flysch basin and implications regarding the tectonic evolution of the Northern Cordillera of Alaska and Yukon. AIMS Geosciences, 2021, 7(3): 355-389. doi: 10.3934/geosci.2021022 |
[7] | Quentin Fiacre Togbévi, Luc Ollivier Sintondji . Hydrological response to land use and land cover changes in a tropical West African catchment (Couffo, Benin). AIMS Geosciences, 2021, 7(3): 338-354. doi: 10.3934/geosci.2021021 |
[8] | Luis M. Sandoval, Philip C. Goodell, Hector Gonzalez-Huizar, Munazzam Ali Mahar . Rayleigh wave group velocity model of the southeast flank of the Rio Grande Rift using Cross-Correlation. AIMS Geosciences, 2018, 4(1): 1-20. doi: 10.3934/geosci.2018.1.1 |
[9] | Adil A Mansoor, Hameed M Abduljabbar . Calculation and determination of radioactivity in the old district of Najaf by using the track detector CR-39 and geographical information systems (GIS) methods. AIMS Geosciences, 2022, 8(4): 709-717. doi: 10.3934/geosci.2022039 |
[10] | Mario Batubara, Masa-yuki Yamamoto, Islam Hosni Hemdan Eldedsouki Hamama, Musthofa Lathif, Ibnu Fathrio . Measurement and characterization of infrasound waves from the March 25, 2023 thunderstorm at the near equatorial. AIMS Geosciences, 2023, 9(4): 652-671. doi: 10.3934/geosci.2023035 |
Approximation theory plays a central role in numerical analysis, evolving through a variety of methodologies, with significant contributions from Lebesgue, Weierstrass, Fourier, and Chebyshev approximations. For sufficiently smooth functions, the partial sum of Chebyshev series expansion provides optimal polynomial approximation, making it a preferred choice in many applications. However, existing literature predominantly focuses on Chebyshev interpolation, which requires exact Chebyshev series coefficients. The computation of these exact coefficients is challenging and often impractical for numerical algorithms, limiting their practical utility. Additionally, traditional approaches typically involve polynomials on fixed intervals where the basis functions of the series are defined. In this article, we have generalized Chebyshev polynomial approximation to a broader domain and presented two optimal error estimations for functions of bounded variation, using approximated Chebyshev series coefficients. This aspect is notably absent in current literature. To support our theoretical findings, we conducted numerical experiments and proposed future research directions, particularly in the fields of machine learning and related areas.
The internal combustion engine has become the foundation for vehicles, agriculture, and military operations as well as electricity generation. Acting as the heart of the vehicle, the internal combustion (IC) engine's function is to give the power that moves the vehicle. The engine itself is comprised of many elements and components, each of which has its own specific role. The primary component of the fuel is hydrocarbons. The composition and properties of it will determine its performance and emission results in the combustion process.
An internal combustion engine that runs on diesel fuel is known as a diesel engine. Diesel engines are commonly found in heavy-duty vehicles such as trucks, cars, and industrial transport that are used on the road. In comparison to S.I engines, C.I diesel engines have better fuel economy and reliability [1]. The excellent thermal efficiency of diesel engines is the primary reason for their vast range of applications, which include transportation, power generation, agricultural forms, and development domains [2].
In any case, compression ignition diesel engines emit massive volumes of pollutants (NOx, soot, CO, UHC, and particulate matter), posing serious health and environmental dangers [3]. The majority of these discharges result from non-ideal combustion cycles, such as fragmented fuel ignition, high temperature and pressing blending product responses, ignition of engine lubricating oil and additives, and burning of non-hydrocarbon diesel fuel components such as sulphur compounds and fuel added substances [4]. In terms of the environment, smoke emissions produced by diesel engines can cause both pollution of water and air, which significantly reduces visibility and contributes to global climate change [5]. It's a different scenario when it comes to health. Dizziness, headaches, and irritation of the eyes, nose, and mouth can occur after a brief exposure to high diesel exhaust emission levels [6]. Long-term exposure raises the risk of cardiovascular illness, cardiopulmonary disease, and respiratory disease, as well as lung cancer [7]. As a result of this, smoke emissions from diesel engines constitute a severe challenge that requires constant forecasting in order to keep them at a minimum, as well as other tactics such as fuel composition alteration, fuel additives, and other alternatives to manage smoke emissions [8].
Asia's rapid development has resulted in extremely high oil demand [9]. It is estimated that the world will consume 105 million barrels of oil per day in 2025 [10]. At this rate, the world is likely to run out of fossil fuels in the next 100 to 50 years [11]. Aside from that, the fuel price is extremely volatile [12]. Furthermore, fuel price fluctuations have a significant impact on economic, fiscal, and income patterns [13]. This is due to the fact that the fuel consumed in goods transportation has an impact on the price of goods [14].
With the conditions listed above, which include a mix of high emissions, high petroleum demand, volatile petroleum prices, and dwindling petroleum resources [15], it is well acknowledged that internal combustion engines must be more efficient [16]. To put it another way, the engine needs to consume less gasoline while producing more power [17]. The engine must also emit fewer pollutants. With these facts in mind, it's clear that we need to limit emissions from combustion engines [18]. As a result, a variety of studies have been done to improve performance and minimise emissions [19,20,21].
Alternative vehicle concepts such as electric vehicles and fuel cells are one way to cut emissions [22]. The less radical option involves employing a combustion engine but with alternative fuels such as biodiesel instead of pure diesel [23]. Another alternative is to add additives to the fuel that is currently being used [24]. The first application of fuel additives was to eliminate knocking in gasoline engines in the early twentieth century [25]. Combustion modifiers, antioxidants, corrosion inhibitors, and deposit control detergents are all examples of fuel additives [26]. Furthermore, gasoline additives can be utilised for a variety of applications, including octane boosters, fuel injector cleaners, and fuel line antifreezes [27]. This study aims to assess the current state of fuel additives in diesel and gasoline engines. In addition to observing the impacts, the study's scope has been expanded to include predicting the outcomes of additive effects on engine performance and emissions.
Currently, the transportation sector is primarily reliant on internal combustion engines, such as diesel and spark-ignition engines. Combustion engines are likely to remain the most important engine for the next 30 years. Every year, the global transportation sector consumes more and more energy. The use of fossil fuels is a major contribution to this fact. Internal combustion engines that run on fossil fuels provide a lot of power to the transportation sector. Despite advancements in alternative fuels, around 90% of people still use traditional fossil fuels, with only a small percentage using renewable fuels [28]. The demand for transportation, industrial, and research services is increasing every day as the world's population grows, resulting in a petroleum fuel shortage. The rapid depletion rate of petroleum fuel, as well as the impact of its emission gases on the environment, has become a major issue for people all over the world.
The literature suggested some popular additives that are in use. Hydrogen [29], CNG [30], LPG, ZnO [31], alcohols, methyl-esters [32], Mn2O3, Al2O3 [33], MWCNT [34], GO, and GNP are the latest additions to the list of materials used as additives. These additives can be classified into gaseous additives, liquid additives, and solid additives. These solid additives can be divided into metal and non-metal additives. This classification can be represented in Figure 1 below:
To understand the subject, the properties of the fuel and additives are observed in detail. The properties of the base fuel (diesel or biodiesel) can be summarised in Table 1, while the properties of gaseous and liquid additives are presented in Table 2.
![]() |
CV [MJ/kg] | Density [kg/m3] | Kinematic Viscosity [mm2/s] | Flash point [℃] |
pure diesel | 44 | 825.96 | 3.96 | 72 |
Biodiesel Chlorella Vulgaris | 38.7 | 860 | 3.7 | 124 |
honge biodiesel HB20 | 42.123 | 835 | 4.12 | 104 |
![]() |
CV [MJ/kg] | Density [kg/m3] | Kinematic Viscosity [mm2/s] |
Flash point [℃] |
hydrogen | 119.93 | 0.0838 | 110 | - |
methanol | 20.30 | 790 | 0.59 | 12 |
ethanol | 27 | 785 | 1.1 | 14 |
CNG | 45.76 | 720 | - | 81.6 |
The carbon-based additives used are carbon nanotubes and graphene. The properties of the materials as in the Table 3 below:
![]() |
CV [MJ/kg] | Density [g/cm3] | Specific Surface Area (m2g-1) | Thermal conductivity (kW/mK) |
SWCNT | 1.3–1.4 | 1315 | 1750–3800 | |
Graphene | 31.82 | 2.267 | 1598 | 5300 |
Many parameters can be used to measure the performance and emissions of a diesel engine. However, after researching many articles in the field, standard parameters used to evaluate engine performance are brake torque, brake power, brake specific fuel consumption (BSFC), and brake thermal efficiency (BTE). Additionally, the emission parameters being observed are carbon monoxide (CO), carbon dioxide (CO2), nitrogen oxides (NOx), and unburned hydrocarbons (HC).
In a study to determine the effect of hydrogen on diesel-biodiesel blend, Tayari [37] added different amounts of hydrogen mixtures to the engine. It was found that hydrogen improved the power performance. Torques in B20H10 and B10H10 were 10.6% and 8.4% higher than the biodiesel blends without hydrogen induction (B20H0 and B10H0). The engine's low power and torque are caused by biodiesel's low heating value and high viscosity. Additional hydrogen to biodiesel improved the power and torque of the engine due to the faster rate of combustion caused by hydrogen. In another research by Ngang [38], the authors conclude that the torque of the diesel-LPG engine increases as the quantity of LPG fuel also increases. The findings strongly support the directly proportional relationship between the amount of LPG fuel introduced and the output torque of the diesel-LPG engine. The power output in the diesel-LPG mode of operation can be controlled by the amount of LPG introduced to the engine. Generally, increasing the LPG mass fraction yields more power. For example, the power of 60% LPG is higher than the power of 50% LPG.
In an experiment by Surya [29], hydrogen enrichment of biodiesel was observed. The fuel used was a honge biodiesel blend (HB20). The hydrogen enrichment was between 10–13 l/min. H-B20-A (Hydrogen 5 l/min + B20 + Al2O3 produced higher power of 6.4%. This combination also reduced specific fuel consumption by 2.72%. In a study to determine the effect of hydrogen on diesel-biodiesel blend, Tayari [37] added different amounts of hydrogen mixtures to the engine. It was found that hydrogen improved the BSFC performance. The average reductions of BSFC for B20H5 and B10H5 were 3.4% and 5.1% compared to the biodiesel blends without hydrogen (B20H0 and B10H0). Biodiesel with hydrogen led to more power generation and increased the combustion efficiency of the engine due to high calorific value and mass flow rate of hydrogen. An example experiment by Akcay [39] indicated that hydrogen addition reduced the BSFC. The result showed With H40 (hydrogen induction of 40 l/min), the BSFCs of diesel fuel tests were decreased by 11.1%, 12.5%, and 7.9%, respectively, for the engine loads of 40, 60, and 80 Nm, as compared with the pure diesel fuel tests. It is seen that BSFC generally exhibits a decrease with the increasing load for each fuel and hydrogen addition. As a result of the increase in the cylinder wall temperatures, the decrease in the ignition delay and, accordingly, the progress of the combustion, BSFCs showed decreasing tendencies [40]. For example, in an experiment using spark-ignition engine, Yilmaz [41] found that the BSFC of alcohol (methanol and ethanol) had been reduced after the induction of hydrogen. The addition of hydrogen reduced the BSFC by around 4% compared to the alcohol-gasoline blend. This is because the lower heating value of hydrogen is very high compared to gasoline. Hydrogen addition increases thermal efficiency and decreases BSFC [42].
The effect of hydrogen addition on thermal efficiency was carried out by Yilmaz [41] in an experiment with alcohol-gasoline in a spark-ignition engine. It was found that the BTE increased with the hydrogen addition. Experimentally, Gnanamoorthi [43] also shows a better BTE (increased by 30.65%) with the addition of hydrogen. Another finding from Tabar [44] is that the BMEP of CNG is 4–14% less than that of gasoline throughout all the engine speeds. CNG has a 10% lower indicated power. When compared to gasoline, CNG has a remarkable 11–39% lower fuel consumption [44]. Sonachalam [45] demonstrated that the brake thermal efficiency (BTE) is increased by about 3.7% at a flow rate of 4 liters per minute (LPM) of acetylene injection in RCCI combustion mode.
An analysis of fuel additive effects on engine emissions was carried out by Gnanamoorthi [43], and it indicated that the emissions of HC, CO, and CO2 were reduced by 22.3%, 14% and 32.74% respectively with the addition of hydrogen. Similarly, another experiment by Selahaddin Orhan Akansu [46] pointed out that hydrogen produced fewer emissions, especially CO, CO2, and HC. This happens because of the chemical composition of hydrogen, which does not have carbon and has high thermal efficiency. However, the emission of NOx increased. The reason for that is the high temperature of the combustion. As argued by Yilmaz [41], the NOx emission has been reduced when hydrogen is introduced into the combustion. The reason for that is the low adiabatic flame temperature of the methanol. The addition of hydrogen by Surya [29] was successful in reducing the CO by 15.91%. However, this experiment produced higher CO2 and NOx by 22.37% and 37.68% respectively.
It was also reported by Yilmaz [41] that CO has been reduced with the addition of hydrogen. The fuel with the lowest CO emission was found to be G85M15H15, with a CO emission of 0.094%. On the other hand, CO2 has been reduced with the addition of hydrogen. The lowest CO2 emission was found to be 12.06% for G85M15H15 fuel. Another example, Sonachalam [45] found that acetylene addition to a diesel engine running with biodiesel shows significant decreases in polluting components such as smoke opacity, oxides of nitrogen (NOx), hydrocarbons (HC) and carbon monoxide (CO) are reduced by about 10%, 7.6%, 13.4% and 28.7%. As reported by [38], LPG addition reduced NOx and HC emission pollutants, despite the fact that the overall in-cylinder temperature increased. This demonstrates that adding LPG to a specific value allows for decreased emissions as long as the mixture remains lean. The ensuing increase in temperature and pressure is compensated for by the mixture becoming richer, cutting emissions and enhancing combustion efficiency.
Figure 2 is a bar chart depicting the various effects of gaseous fuel additives on engine emissions. In the graphic, the percentage value of hydrogen, CNG, and acetylene additions are compared to their base fuels, which are gasoline-ethanol blend, gasoline, and biodiesel, respectively.
Several studies have been undertaken to investigate the impact of liquid additives on engine performance and emissions. Najafi [47] conducted an experiment to estimate the performance and exhaust emissions of a four-cylinder SI engine. The engine operates on the different mixing ratios of ethanol and gasoline blends. A mixture of gasoline and bioethanol derived from potato peels has been employed. 0%, 5%, 10%, 15%, and 20% have been employed. The mixture was called E0, E5, E10, E15, and E20. The engine performance and emissions from the mixture have been compared with the performance and emissions from 100% gasoline. The experiment found that using ethanol blends improved brake power, brake thermal efficiency (BTE), engine torque, and volumetric efficiency. On the other hand, the brake specific fuel consumption (BSFC) decreased. BSFC is defined as the energy consumed per unit power produced considering the calorific value of the fuel. Mohamed Nour [48] identifies the effects of pentanol/hydrous ethanol/diesel blend and octanol/hydrous ethanol/diesel have on compressed-ignition engine. The finding suggests that the addition of octanol to the hydrous ethanol/diesel blends achieves the lower BSFC at all loads.
A deeper investigation by Yesilyurt [49] was performed to compare the engine performance, combustion, and emissions of direct-injected diesel engines fuelled with diesel, with diesel-butanol blend, and diesel-pentanol blend. Yesilyurt reported that the blended fuels produced lower EGT and CO2. However, more O2 is produced. The reason for the observation was the higher oxygen content in the alcohol.
As highlighted by Godwin [50], gasoline blended with alcohol produced lower EGT compared to 100% gasoline. This is because gasoline has the highest calorific value and the lowest latent heat of vaporization, lean mixture formation, and flame propagation. This leads to an increase in cylinder temperature and more gasoline being used.
Another experiment using alcohol was conducted by S. Phuangwongtrakul [51] to study the engine performance. Brake torque and brake specific fuel consumption while a different mixing ratio of ethanol was investigated. The experiment proved that a proper ethanol-gasoline mixing ratio can improve engine torque output while reducing emissions. By adding pentanol to biodiesel, Yesilyurt [52] found the maximum BTE values of the diesel fuel and B20, and B20P5 were found to be at 22.75%, 21.82%, and 20.96% under 750 W engine load condition, respectively. This little change, however, can be considered insignificant.
From the experiment by Najafi [47], the result showed that the amount of CO and HC had been reduced, but CO2 and NOx emissions were higher. This is supported by Dogan in 2017 [53] to identify irreversible processes using energy and exergy analyses. The fuels were blended in various proportions. E0 refers to 100 percent unleaded gasoline, while E10 refers to gasoline blended with 10% ethanol. E20 is ethanol with a concentration of 20%, and E30 is ethanol with a concentration of 30%. The fuel blends were then tested in gasoline engines at various speeds, loads, and under identical conditions for each fuel type. NOx levels were reduced as a result of the experiments and theoretical calculations.
One of the new exploratory investigations by Yesilyurt [49] is the exhaust emission characteristics of a solitary chamber, direct-infusion diesel motor running on biodiesel/diesel/1-butanol and biodiesel/diesel/n-pentanol fuel blends. Yesilyurt found that alcohol blended fuels reduced NOx by 0.56%–2.65%, CO by 6.90%–32.40%, and smoke by 10.47%–44.33%. The authors expressed that higher alcohols, particularly n-pentanol, could be an efficient additive to diminish NOx emissions by thinking about the acquired outcomes.
In another major study, Uslu and Celik [54] utilised diethyl ether (DEE) blends with pure diesel to study the exhaust emission characteristics of a single-cylinder, direct-injection, and air-cooled diesel engine. An experiment was carried out at four engine speeds ranging from 1000 to 1600 RPM with 200 RPM intervals and four engine loads, which were 150,300,500, and 1000-watt halogen lamps. Diesel fuel was mixed with DEE0, DEE2.5, DEE5, DEE7.5, and DEE10, which contained 2.5%, 5%, 7.5%, and 10% by volume of DEE, respectively. According to the author's findings, DEE10 allows NOx emissions to be reduced by 56%.
Another experiment was conducted by Dogan [53] to identify irreversible processes using energy and exergy analyses. The fuels were blended at different ratios. 100% unleaded gasoline is called E0, and gasoline blended with 10% ethanol is called E10. 20% ethanol is called E20 and 30% ethanol is called E30. The fuel blends then applied into gasoline engine with different speeds, under different loads and under the same conditions for each fuel type. The result from the experiments and theoretical calculations showed a reduction in carbon monoxide (CO).
In another major study done by Uslu and Celik [54], it was identified that DEE10 allows NOx emissions to be reduced by 56% compared with diesel fuel, while CO emissions are reduced by about 45%. Dogan [53] conducted another experiment that focused on identifying irreversible processes using energy and exergy analyses. The fuels were mixed in various proportions. E0 refers to 100 percent unleaded gasoline, while E10 refers to gasoline blended with 10% ethanol. E20 is ethanol with a concentration of 20%, and E30 is ethanol with a concentration of 30%. The fuel blends are then applied to gasoline engines at varying speeds, under varying loads, and under the same conditions for each fuel type. Carbon dioxide levels were reduced as a result of the experiments and theoretical calculations (CO2).
In the same vein, the experiments by Chao Chen [55] and Pinzi [56] found that the diesel/alcohol blend was able to reduce soot formation. This phenomenon might happen due to the high oxygen content in alcohol that helps in the burning of soot during combustion. A bar chart is plotted in Figure 3 to visualise the different effects of the liquid fuel additives on the engine emissions.
Solid fuel additives are another important type of additive. Amirabedi [57] went above and beyond by incorporating Mn2O3 into the gasoline-alcohol mixture. She used gasoline that had been diluted with 10% ethanol. She then added 10 ppm and 20 ppm Mn2O3 as additional samples. The outcomes were very promising. In terms of efficiency, performance has improved. This is supported by Jayaraman's [58] findings, which investigate the effects of adding titanium oxide TiO2 to a B20 blend in a CI engine. As a result, specific consumption has declined. At 25% load, B20 + 50 produces the lowest BSFC of 0.46 kg/kw-hr. At 18.16 kg load, the maximum BTE for B20 + 50 + EGR was 32.64 percent. The BTE of B20 with TiO2 is higher than that of normal B20 because the TiO2 improved combustion and released a high heat rate.
Jiangjun Wei [59] investigated the emission of CI engine operation with diesel-methanol blends using Aluminium Oxide Al2O3 as an additive. Among the highlights are reductions in BTE and BSFC of 3.6 and 3.7 percent, respectively. According to Örs [60], adding TiO2 to biodiesel-butanol blends increased brake engine torque and power by 10.20 percent and 9.74 percent, respectively. BSFC, on the other hand, can be reduced by 27.73%. Amirabedi [57] discovered that the performance in terms of power and efficiency has improved. This finding is supported by Soner's findings [61]. In his experiment, adding 50 ppm of Al2O3 and CuO to diesel fuel resulted in increased engine power.
The research by Örs [60] showed that CO and HC have been reduced by around 14.96% and 34.39%, respectively. with the TiO2 addition. However, the emission of CO2 and NO was increased by 5.75% and 3.94%, respectively. Research by Amirabedi [57] achieved a 51.83% reduction of HC by using gasoline with 10% ethanol and 20 ppm Mn2O3 compared to that of baseline gasoline. This is achieved when the engine runs at full load and the speed is set at 2800 rpm. The CO was reduced by 24.09% with respect to the baseline gasoline fuel. However, CO2 emissions increased by 13.27% in this experiment. Using aluminium oxide (Al2O3) as an additive, Jiangjun Wei [62] tested the emissions of a CI engine run with diesel-methanol blends. The results were lower CO and HC of 83.3% and 40.9%. However, the engine emitted a marginally higher NOX of 14.4%. A bar chart is plotted in Figure 4 to visualise the different effects of the solid fuel additives on the engine's emissions.
The section that follows discusses carbon-based fuel additives. This review focuses on carbon nanotubes and graphene. Heydari-Melaney [63] improved the performance of biodiesel-bioethanol mixtures by adding carbon nanotubes (CNT) and increasing torque, power, and BTE by 15.52%, 15.52%, and 13.97%, respectively. BSFC, on the other hand, fell 11.73%. Similarly, Hosseini [64] used CNT as an additive in a CI engine that was powered by a diesel-biodiesel blend. The procedure for the experiment was carried out under full load with various blends and speeds. In his experiment, he discovered that the addition of CNT produced more torque than pure diesel fuel. He also discovered that power increased by 21.84% overall. BTE, on the other hand, was discovered to be decreasing from 1800 to 2800 rpm. This was caused by a decrease in volumetric efficiency. The addition of CNT reduced BSFC as well.
Gad [65] used carbon nanotubes and graphene nanosheets as fuel additives in B20 blends. CNTs and graphene nanosheets are mixed into B20 at concentrations of 25, 50, and 100 ppm to create fuel blends. Thermal efficiency for B20CNT100 and B20CNS100 improved by 8% and 19%, respectively, according to the results. In another significant study, Razzaq [66] investigated the use of graphene and dimethyl carbonate (DMC) as additives in biodiesel. The goal is to investigate the effects on performance and emissions. According to his observations, the BTE increased with a small amount of GNP. However, increasing the amount reduces the BTE. The reason for this could be due to the higher viscosity.
In Hosseini's experiment [64], he discovered that under various conditions, the emission of a fuel blend with CNT additives was reduced by 40.38 percent when compared to neat diesel. In the case of CO2, the average emission increased by 9.88 percent when all fuel blends with CNTs were compared to straight diesel fuel. The HC emissions of a fuel blend containing a CNT additive were reduced in general. In Gad's experiment [65], extremely large reductions in smoke thickness, CO, NOx, HC, and with B20CNT100 are observed, which are 28 percent, 27 percent, 22 percent, and 28 percent, respectively. For smoke thickness, CO, NOx, and HC, B20CNS100 obtained 54%, 47%, 44%, and 52%, respectively.
Tomar and Kumar [67] conducted a similar investigation using a single-cylinder four-stroke diesel engine (Kirloskar) and diesel-Schleichera Oleosa Biodiesel mixes with alumina nanoparticles and multi-walled carbon nanotubes. Based on their findings, the authors believe that the use of alumina and MWCNT-added substances could be an effective fuel reformulation strategy. NOx emissions decrease as a result of the presence of nano-added substances in D80B20C100 fuel, owing to the amazing spongy inclination of MWCNT nanoparticles.
Mei et al. [68] proposed a single-cylinder four-stroke air-cooled common-rail diesel engine operating at 3000 rpm with changing loads of 100%, 75%, 50%, and 25%. The authors used two different nanoparticles, CNTs and molybdenum trioxide (MoO3), and mixed them with clean diesel at concentrations of 50 mg/L and 100 mg/L for each nanoparticle. According to the authors, CNTs have a greater potential than MoO3 to reduce the entire estimated exhaust emission. CO, HC, NOx, and smoke discharge from CNT100 were reduced by 9.6%, 11.4%, 8.9%, and 15.25%, respectively, when compared to the exhaust emission produced by Mo100, which is 6.8%, 7.5%, 5.2%, and 8.3%. The authors also stated that the results could be attributed to CNTs' superior heat conductivity and surface deficiency when compared to MoO3.
In Heydari-Melaney [63] experiment where CNT was mixed into biodiesel-bioethanol mixtures, The CO and UHC reduced by 5.47% and 31.72%, respectively. However, NOx emission increased by 12.22%. According to Razzaq [66], an increase in HC emission was observed compared to that of B30 with the addition of GNPs in biodiesel blends. However, if the concentration in GNP is increased further, the HC emissions are reduced significantly. The CO emission was also increased with the increasing engine speeds. This is valid for all tested blends. The reason for this observation is caused by the difference in air-fuel quantity entering the combustion chamber for different engine operating conditions. Normally, NOx emissions contributed to the high temperature in the cylinder. The high temperature oxidized the nitrogen which is the main component of air sucked by the engine. In Razzaq's experiment, the addition of GNPs in biodiesel blends reduced the NOx emission. This happens because GNP's presence improved the ID period.
Nivin Chacko [69] used GNP in an experiment that also showed a reduction in emissions, particularly NO. El-Seesy [70] discovered a reduction in emissions after using graphene nanoplatelets as an additive in jatropha biodiesel-diesel fuel. NOx, CO, and UHC emissions were reduced by 45 percent, 55 percent, and 50 percent, respectively. Another study by Debbarma discovered that adding 75 ppm of GNP to biodiesel improved engine performance, particularly BTE, BSFC, and combustion behaviour, especially at higher loads [71]. Figure 5 depicts a bar chart that depicts the various effects of carbon-based fuel additives on engine emissions.
In addition, a table to summarise the results from different experiments is depicted in Table 4 below:
Gaseous Additives | ||||||
Ref. | Engine | Fuel | Additive | Method | Performance | Emissions |
[29] | 4-cylinder Diesel | B20 | Al2O3 + H2 | Full load between 1200–2800 rpm | Power ↑ BTE ↑ BSFC ↓ |
CO ↓ CO2 ↑ NOx ↑ |
[37] | 1-cylinder Diesel | B20 |
H2 | Full load. Various speed |
Torque ↑ Power ↑ BTE ↑ |
CO ↓ CO2 ↓ HC ↓ NOx ↑ |
[43] | 1-cylinder Diesel | Diesel | H2 | Constant speed 1500 rpm | BTE ↑ |
CO ↓ CO2 ↓ HC ↓ NOx ↑ |
[45] | 1-cylinder Diesel | Biodiesel | Acetylene | 1500 rpm Different loads |
BTE ↑ |
CO ↓ HC ↓ NOx ↓ |
[38] | 4-cylinder diesel | diesel | LPG | different Speeds and loads | Torque ↑ Power ↑ |
HC ↓ NOx ↓ |
Liquid Additives | ||||||
Ref. | Engine | Fuel | Additive | Method | Performance | Emissions |
[48] | 1-cylinder Diesel | Hydrous ethanol-Diesel | Pentanol and Octanol | Different blends at different loads | Power ↓ BTE ↑ BSFC ↑ |
CO ↓ CO2 ↓ NOx ↓ |
[72] | 1-cylinder Diesel | Diesel | Alcohol | Constant speed 2500 rpm. Different alcohol percentage. | BTE ↓ |
CO ↓ HC ↑ NOx ↑ |
[52] | 1-cylinder Diesel | Biodiesel | Pentanol | 3000 rpm Different engine loads |
BSFC ↑ BTE ↓ |
CO ↓ CO2 ↑ NOx ↓ |
Solid Additives (Metal-based) | ||||||
Ref. | Engine | Fuel | Additive | Method | Performance | Emissions |
[73] | 1-cylinder Diesel |
biodiesel | CuO | Various loads | BTE ↑ BSFC ↓ |
CO ↓ HC ↓ NOx ↑ |
[62] | 1-cylinder Diesel |
Diesel-methanol | Al2O3 | Constant speed 1400 rpm. Different loads | BTE ↑ BSFC ↓ |
CO ↓ HC ↓ NOx ↑ |
[60] | 1-cylinder Diesel |
Waste Cooking Biodiesel | TiO2 | Various engine speeds. Full load | Torque ↑ Power ↑ BSFC ↓ |
CO ↓ CO2 ↑ HC ↓ NO ↑ |
Solid Additives (Carbon-based) | ||||||
Ref. | Engine | Fuel | Additive | Method | Performance | Emissions |
[63] | 1-cylinder Diesel | Diesohol (diesel + alcohol) | CNT | Full load at different rpm | Torque ↑ Power ↑ BTE ↑ BSFC ↓ |
CO ↓ CO2 ↓ HC ↓ NOx ↑ |
[67] | 1-cylinder Diesel | Diesel & Diesel-biodiesel blend | MWCNT | Constant speed 1500 rpm. Different loads | BTE ↑ BSFC ↓ |
CO ↓ NOX↓ |
[64] | 1-cylinder Diesel | Diesel-biodiesel blend | CNT | Full load. Different blends and speed | Power ↑ Torque ↑ BTE ↓ BSFC ↓ |
CO ↓ CO2 ↑ HC ↓ NOX ↑ |
[66] | 1-cylinder Diesel | Palm oil biodiesel | Graphene Oxide Nanoplatelets (GNP) | Varying engine speed. Different blends | BTE ↑ BSFC ↑ |
HC ↓ CO ↑ NOX↓ |
[74] | 1-cylinder Diesel | Jatropha biodiesel | Graphene Nanoplatelets (GNP) | Different Speeds, different loads, different fuel blends | BSFC ↓ | CO ↓ HC ↓ NOX ↓ |
Further, the study extends to the prediction of the effects on the performance and emissions of a diesel engine. The articles with predictions or simulations of the effects are being studied. Different research articles with additional predictions on the additive effects on the performance and emissions are selected. The following is a table summary of the effects that additives have on performance and emissions.
In addition to understanding the effects the additive has on the fuels, the researchers go on further to predict the engine performance and emissions. The most popular method to predict the response of different factors in a study is called response surface methodology (RSM).
According to Table 5, no prediction research has been conducted on the effects of graphene additives on the performance and emissions of diesel engines. As a result, this is an opportunity for research to fill this gap.
No | Base fuel | Additives/blends | Parameters | Prediction Method | Software/Tool | Reference |
Liquid Additives | ||||||
1 | Waste plastic oil | ethanol | HC, CO, SFC | DOE with Full factorial design | Minitab | [75] |
2 | diesel | DME | BTE, BSEC | Double Weibe | - | [76] |
3 | diesel | higher alcohol additive (Decanol) | BSFC, BTE, CO, CO2, HC, NOx | ANN and RSM | Design Expert | [77] |
4 | diesel | pongamia methyl esters | BTE, UHC, NOx | RSM | Minitab | [78] |
Gaseous Additives | ||||||
5 | diesel | CNG | BSFC, BTE, CO, CO2, HC, NOx | RSM | Minitab and Design Expert | [35] |
6 | diesel | LPG | BSEC, BTE | Fuzzy Logic | ANFIS | [79] |
7 | diesel | Hydrogen |
CO, CO2, HC, NOx | DOE with Taguchi | Minitab | [80] |
Solid Additives (Metal) | ||||||
8 | biodiesel | NiO | BSFC NOx, HC, CO |
RSM | Design Expert | [81] |
9 | biodiesel | Alumina nanoparticles | BSFC, BTE, CO, NOx | RSM | Design Expert | [82] |
10 | biodiesel | TiO2, and Al2O3 nanoparticles | BSFC, BTE, CO, NOx | RSM | Design Expert | [83] |
Solid Additives (Carbon based) | ||||||
11 | diesel and biodiesel | MWCNT | BSFC, CO, NOx | RSM | Design Expert | [83] |
12 | diesel and biodiesel | MWCNT | BTE, BSFC, UHC, CO, NOx | RSM | Design Expert | [84] |
13 | fusel oil | nano- biochar | Torque, Power, BSFC, BTE, CO, NOx | RSM | Design Expert | [85] |
According to the review, many different types of fuel additives have been developed. Many additives were available in gaseous, liquid, and solid forms. Many solid materials are being considered as fuel additives as nanotechnology advances. Carbon-based nanomaterials have shown a lot of promise in terms of improved performance and lower emissions. New graphene materials have also been discovered and added to the list of additives; however, the depth of research is still insufficient. More information on various types of engines and configurations would aid us in establishing greater accuracy on this subject.
The financial assistance and laboratory use provided by the RACER Grant, RDU192617, through the Automotive Engineering Centre, Universiti Malaysia Pahang is gratefully acknowledged.
The authors declare no conflicts.
[1] | W. F. Ames, Numerical methods for partial differential equations, Academic press, 2014. |
[2] | J. C. Butcher, Numerical methods for ordinary differential equations, John Wiley & Sons, 2016. |
[3] | J. C. Mason, D. C. Handscomb, Chebyshev polynomials, Chapman & Hall/CRC, 2003. https://doi.org/10.1201/9781420036114 |
[4] | L. N. Trefethen, Approximation theory and approximation practice, Philadelphia: Society for Industrial and Applied Mathematics, 2013. |
[5] | G. Meinardus, Approximation of functions: Theory and numerical methods, Springer Science & Business Media, 13 (2012). |
[6] | H. Attouch, G. Buttazzo, G. Michaille, Variational analysis in Sobolev and BV spaces: Applications to PDEs and optimization, SIAM, 2014. |
[7] | R. F, Gariepy, Functions of bounded variation and free discontinuity problems (oxford mathematical monographs) by luigi ambrosio, nicolo fucso and diego pallara: 434 pp., £ 55.00, isbn 0-19-850254-1 (clarendon press, oxford, 2000), Bulletin of the London Mathematical Society, 33 (2001), 492–512. https://doi.org/10.1017/S0024609301309281 |
[8] | C. M. Dafermos, C. M. Dafermos, Hyperbolic conservation laws in continuum physics, Springer, 3 (2005). https://doi.org/10.1007/978-3-662-49451-6 |
[9] |
B. Bourdin, A. Chambolle, Implementation of an adaptive finite-element approximation of the mumford-shah functional, Numer. Math., 85 (2000), 609–646. https://doi.org/10.1007/PL00005394 doi: 10.1007/PL00005394
![]() |
[10] |
S. Xiang, On the optimal convergence rates of Chebyshev interpolations for functions of limited regularity, Appl. Math. Lett., 84 (2018), 1–7. https://doi.org/10.1016/j.aml.2018.04.009 doi: 10.1016/j.aml.2018.04.009
![]() |
[11] |
F. Dell'Accio, F. Di Tommaso, F. Nudo, Generalizations of the constrained mock-chebyshev least squares in two variables: Tensor product vs total degree polynomial interpolation, Appl. Math. Lett., 125 (2022), 107732. https://doi.org/10.1016/j.aml.2021.107732 doi: 10.1016/j.aml.2021.107732
![]() |
[12] |
F. Dell'Accio, F. Marcellán, F. Nudo, An extension of a mixed interpolation-regression method using zeros of orthogonal polynomials, J. Comput. Appl. Math., 450 (2024), 116010. https://doi.org/10.1016/j.cam.2024.116010 doi: 10.1016/j.cam.2024.116010
![]() |
[13] |
H. Wang, Analysis of error localization of chebyshev spectral approximations, SIAM J. Numer. Anal., 61 (2023), 952–972. https://doi.org/10.1137/22M1481452 doi: 10.1137/22M1481452
![]() |
[14] |
R. Xie, B. Wu, W. Liu, Optimal error estimates for chebyshev approximations of functions with endpoint singularities in fractional spaces, J. Sci. Comput., 96 (2023), 71. https://doi.org/10.1007/s10915-023-02292-5 doi: 10.1007/s10915-023-02292-5
![]() |
[15] |
H. Y. Wang, New error bounds for legendre approximations of differentiable functions, J. Fourier Anal. Appl., 29 (2023), 42. https://doi.org/10.1007/s00041-023-10024-4 doi: 10.1007/s00041-023-10024-4
![]() |
[16] | W. J. Liu, L. L. Wang, H. Y. Li, Optimal error estimates for chebyshev approximations of functions with limited regularity in fractional sobolev-type spaces, Math. Comput., 88 (2019), 2857–2895. |
[17] | M. Hamzehnejad, M. M. Hosseini, A. Salemi, An improved bound on legendre approximation, arXiv Preprint, 2022. https://doi.org/10.48550/arXiv.2207.13477 |
[18] |
X. L. Zhang, J. P. Boyd, Asymptotic coefficients and errors for chebyshev polynomial approximations with weak endpoint singularities: Effects of different bases, Sci. China Math., 66 (2023), 191–220. https://doi.org/10.1007/s11425-021-1974-x doi: 10.1007/s11425-021-1974-x
![]() |
[19] | X. L. Zhang, The mysteries of the best approximation and chebyshev expansion for the function with logarithmic regularities, arXiv Preprint, 2021. https://doi.org/10.48550/arXiv.2108.03836 |
[20] |
X. L. Zhang, Comparisons of best approximations with chebyshev expansions for functions with logarithmic endpoint singularities, Numer. Algorithms, 2023, 1–25. https://doi.org/10.1007/s11075-023-01538-5 doi: 10.1007/s11075-023-01538-5
![]() |
[21] |
D. Occorsio, W. Themistoclakis, On the filtered polynomial interpolation at chebyshev nodes, Appl. Numer. Math., 166 (2021), 272–287. https://doi.org/10.1016/j.apnum.2021.04.013 doi: 10.1016/j.apnum.2021.04.013
![]() |
[22] |
D. Occorsio, G. Ramella, W. Themistoclakis, Lagrange-chebyshev interpolation for image resizing, Math. Comput. Simulat., 197 (2022), 105–126. https://doi.org/10.1016/j.matcom.2022.01.017 doi: 10.1016/j.matcom.2022.01.017
![]() |
[23] |
M. C. De Bonis, D. Occorsio, W. Themistoclakis, Filtered interpolation for solving prandtl's integro-differential equations, Numer. Algorithms, 88 (2021), 679–709. https://doi.org/10.1007/s11075-020-01053-x doi: 10.1007/s11075-020-01053-x
![]() |
[24] | D. I. Shuman, P. Vandergheynst, P. Frossard, Chebyshev polynomial approximation for distributed signal processing, In 2011 International Conference on Distributed Computing in Sensor Systems and Workshops (DCOSS), 2011, 1–8. https://doi.org/10.1109/DCOSS.2011.5982158 |
[25] |
T. T. Lee, J. T. Jeng, The chebyshev-polynomials-based unified model neural networks for function approximation, IEEE T. Syst. Man Cy.-B, 28 (1998), 925–935. https://doi.org/10.1109/3477.735405 doi: 10.1109/3477.735405
![]() |
[26] | M. G. He, Z. W. Wei, J. R. Wen, Convolutional neural networks on graphs with chebyshev approximation, revisited, Adv. Neural Inform. Pro. Syst., 35 (2022), 7264–7276. |
[27] | Z. P. Li, J. Wang, Spectral graph neural networks with generalized laguerre approximation, In: ICASSP 2024-2024 IEEE International Conference on Acoustics, Speech and Signal Processing (ICASSP), 2024, 7760–7764. https://doi.org/10.1109/ICASSP48485.2024.10445856 |
[28] | L. Stankovic, D. Mandic, M. Dakovic, M. Brajovic, B. Scalzo, A. G. Constantinides, Graph signal processing–part ii: Processing and analyzing signals on graphs, arXiv Preprint, 2019. https://doi.org/10.48550/arXiv.1909.10325 |
[29] |
C. C. Tseng, S. L. Lee, Minimax design of graph filter using chebyshev polynomial approximation, IEEE T. Circuits-II, 68 (2021), 1630–1634. https://doi.org/10.1109/TCSII.2021.3065977 doi: 10.1109/TCSII.2021.3065977
![]() |
[30] |
K. O. Geddes, Near-minimax polynomial approximation in an elliptical region, SIAM J. Numer. Anal., 15 (1978), 1225–1233. https://doi.org/10.1137/0715083 doi: 10.1137/0715083
![]() |
[31] |
H. Majidian, On the decay rate of chebyshev coefficients, Appl. Numer. Math., 113 (2017), 44–53. https://doi.org/10.1016/j.apnum.2016.11.004 doi: 10.1016/j.apnum.2016.11.004
![]() |
[32] |
W. M. A. Elhameed, O. M. Alqubori, New results of unified chebyshev polynomials, AIMS Math., 9 (2024), 20058–20088. https://doi.org/10.3934/math.2024978 doi: 10.3934/math.2024978
![]() |
[33] |
W. M. A. Elhameed, H. M. Ahmed, Spectral solutions for the time-fractional heat differential equation through a novel unified sequence of chebyshev polynomials, AIMS Math., 9 (2024), 2137–2166. https://doi.org/10.3934/math.2024107 doi: 10.3934/math.2024107
![]() |
[34] | A. L. Tampos, J. E. C. Lope, Overcoming Gibbs phenomenon in Padé-Chebyshev approximation, Matimyás Mat., 30 (2007), 127–135. |
[35] | T. J. Rivlin, The Chebyshev polynomials, New York: John Wiley & Sons, 1974. |
[36] |
C. Carle, M. Hochbruck, A. Sturm, On leapfrog-chebyshev schemes, SIAM J. Numer. Anal., 58 (2020), 2404–2433. https://doi.org/10.1137/18M1209453 doi: 10.1137/18M1209453
![]() |
[37] | J. J. Glunt, J. A. Siefert, A. F. Thompson, H. C. Pangborn, Error bounds for compositions of piecewise affine approximations, arXiv Preprint, 2024. https://doi.org/10.1016/j.ifacol.2024.07.423 |
[38] |
J. V. Schaftingen, Approximation in sobolev spaces by piecewise affine interpolation, J. Math. Anal. Appl., 420 (2014), 40–47. https://doi.org/10.1016/j.jmaa.2014.05.036 doi: 10.1016/j.jmaa.2014.05.036
![]() |
[39] | S. Lang, Real and functional analysis, New York: Springer, 2012. |
[40] | T. M. Apostol, Mathematical analysis, 2Eds., Addison-Wesley series in mathematics, Reading: Addison-Wesley, 1974. |
[41] |
S. Xiang, X. Chen, H. Wang, Error bounds for approximation in Chebyshev points, Numer. Math., 116 (2010), 463–491. https://doi.org/10.1007/s00211-010-0309-4 doi: 10.1007/s00211-010-0309-4
![]() |
[42] | L. Fox, I. B. Parker, Chebyshev polynomials in numerical analysis, Oxford University Press, 1968. |
1. | Ligia Alves Da Silva, Jiankui Shi, Lívia R. Alves, Laysa Cristina Araújo Resende, Luis Eduardo Antunes Vieira, Joaquim Eduardo Rezende Costa, Jose Paulo Marchezi, Oleksiy V. Agapitov, David Gary Sibeck, Angela Machado dos Santos, Vania Fatima Andrioli, Paulo Ricardo Jauer, Vinicius Deggeroni, Carolina de Sousa do Carmo, Prosper Kwamla Nyassor, Sony Su Chen, Toyese Tunde Ayorinde, Karen Júlia Coldebella Ferreira, Juliano Moro, Chi Wang, Hui Li, Zhengkuan Liu, Space weather effects over SAMA during the extreme geomagnetic storm on May 10-11, 2024: disturbances of the neutral and ionized atmosphere, 2025, 12, 2296-987X, 10.3389/fspas.2025.1550635 |
![]() |
CV [MJ/kg] | Density [kg/m3] | Kinematic Viscosity [mm2/s] | Flash point [℃] |
pure diesel | 44 | 825.96 | 3.96 | 72 |
Biodiesel Chlorella Vulgaris | 38.7 | 860 | 3.7 | 124 |
honge biodiesel HB20 | 42.123 | 835 | 4.12 | 104 |
![]() |
CV [MJ/kg] | Density [kg/m3] | Kinematic Viscosity [mm2/s] |
Flash point [℃] |
hydrogen | 119.93 | 0.0838 | 110 | - |
methanol | 20.30 | 790 | 0.59 | 12 |
ethanol | 27 | 785 | 1.1 | 14 |
CNG | 45.76 | 720 | - | 81.6 |
![]() |
CV [MJ/kg] | Density [g/cm3] | Specific Surface Area (m2g-1) | Thermal conductivity (kW/mK) |
SWCNT | 1.3–1.4 | 1315 | 1750–3800 | |
Graphene | 31.82 | 2.267 | 1598 | 5300 |
Gaseous Additives | ||||||
Ref. | Engine | Fuel | Additive | Method | Performance | Emissions |
[29] | 4-cylinder Diesel | B20 | Al2O3 + H2 | Full load between 1200–2800 rpm | Power ↑ BTE ↑ BSFC ↓ |
CO ↓ CO2 ↑ NOx ↑ |
[37] | 1-cylinder Diesel | B20 |
H2 | Full load. Various speed |
Torque ↑ Power ↑ BTE ↑ |
CO ↓ CO2 ↓ HC ↓ NOx ↑ |
[43] | 1-cylinder Diesel | Diesel | H2 | Constant speed 1500 rpm | BTE ↑ |
CO ↓ CO2 ↓ HC ↓ NOx ↑ |
[45] | 1-cylinder Diesel | Biodiesel | Acetylene | 1500 rpm Different loads |
BTE ↑ |
CO ↓ HC ↓ NOx ↓ |
[38] | 4-cylinder diesel | diesel | LPG | different Speeds and loads | Torque ↑ Power ↑ |
HC ↓ NOx ↓ |
Liquid Additives | ||||||
Ref. | Engine | Fuel | Additive | Method | Performance | Emissions |
[48] | 1-cylinder Diesel | Hydrous ethanol-Diesel | Pentanol and Octanol | Different blends at different loads | Power ↓ BTE ↑ BSFC ↑ |
CO ↓ CO2 ↓ NOx ↓ |
[72] | 1-cylinder Diesel | Diesel | Alcohol | Constant speed 2500 rpm. Different alcohol percentage. | BTE ↓ |
CO ↓ HC ↑ NOx ↑ |
[52] | 1-cylinder Diesel | Biodiesel | Pentanol | 3000 rpm Different engine loads |
BSFC ↑ BTE ↓ |
CO ↓ CO2 ↑ NOx ↓ |
Solid Additives (Metal-based) | ||||||
Ref. | Engine | Fuel | Additive | Method | Performance | Emissions |
[73] | 1-cylinder Diesel |
biodiesel | CuO | Various loads | BTE ↑ BSFC ↓ |
CO ↓ HC ↓ NOx ↑ |
[62] | 1-cylinder Diesel |
Diesel-methanol | Al2O3 | Constant speed 1400 rpm. Different loads | BTE ↑ BSFC ↓ |
CO ↓ HC ↓ NOx ↑ |
[60] | 1-cylinder Diesel |
Waste Cooking Biodiesel | TiO2 | Various engine speeds. Full load | Torque ↑ Power ↑ BSFC ↓ |
CO ↓ CO2 ↑ HC ↓ NO ↑ |
Solid Additives (Carbon-based) | ||||||
Ref. | Engine | Fuel | Additive | Method | Performance | Emissions |
[63] | 1-cylinder Diesel | Diesohol (diesel + alcohol) | CNT | Full load at different rpm | Torque ↑ Power ↑ BTE ↑ BSFC ↓ |
CO ↓ CO2 ↓ HC ↓ NOx ↑ |
[67] | 1-cylinder Diesel | Diesel & Diesel-biodiesel blend | MWCNT | Constant speed 1500 rpm. Different loads | BTE ↑ BSFC ↓ |
CO ↓ NOX↓ |
[64] | 1-cylinder Diesel | Diesel-biodiesel blend | CNT | Full load. Different blends and speed | Power ↑ Torque ↑ BTE ↓ BSFC ↓ |
CO ↓ CO2 ↑ HC ↓ NOX ↑ |
[66] | 1-cylinder Diesel | Palm oil biodiesel | Graphene Oxide Nanoplatelets (GNP) | Varying engine speed. Different blends | BTE ↑ BSFC ↑ |
HC ↓ CO ↑ NOX↓ |
[74] | 1-cylinder Diesel | Jatropha biodiesel | Graphene Nanoplatelets (GNP) | Different Speeds, different loads, different fuel blends | BSFC ↓ | CO ↓ HC ↓ NOX ↓ |
No | Base fuel | Additives/blends | Parameters | Prediction Method | Software/Tool | Reference |
Liquid Additives | ||||||
1 | Waste plastic oil | ethanol | HC, CO, SFC | DOE with Full factorial design | Minitab | [75] |
2 | diesel | DME | BTE, BSEC | Double Weibe | - | [76] |
3 | diesel | higher alcohol additive (Decanol) | BSFC, BTE, CO, CO2, HC, NOx | ANN and RSM | Design Expert | [77] |
4 | diesel | pongamia methyl esters | BTE, UHC, NOx | RSM | Minitab | [78] |
Gaseous Additives | ||||||
5 | diesel | CNG | BSFC, BTE, CO, CO2, HC, NOx | RSM | Minitab and Design Expert | [35] |
6 | diesel | LPG | BSEC, BTE | Fuzzy Logic | ANFIS | [79] |
7 | diesel | Hydrogen |
CO, CO2, HC, NOx | DOE with Taguchi | Minitab | [80] |
Solid Additives (Metal) | ||||||
8 | biodiesel | NiO | BSFC NOx, HC, CO |
RSM | Design Expert | [81] |
9 | biodiesel | Alumina nanoparticles | BSFC, BTE, CO, NOx | RSM | Design Expert | [82] |
10 | biodiesel | TiO2, and Al2O3 nanoparticles | BSFC, BTE, CO, NOx | RSM | Design Expert | [83] |
Solid Additives (Carbon based) | ||||||
11 | diesel and biodiesel | MWCNT | BSFC, CO, NOx | RSM | Design Expert | [83] |
12 | diesel and biodiesel | MWCNT | BTE, BSFC, UHC, CO, NOx | RSM | Design Expert | [84] |
13 | fusel oil | nano- biochar | Torque, Power, BSFC, BTE, CO, NOx | RSM | Design Expert | [85] |
![]() |
CV [MJ/kg] | Density [kg/m3] | Kinematic Viscosity [mm2/s] | Flash point [℃] |
pure diesel | 44 | 825.96 | 3.96 | 72 |
Biodiesel Chlorella Vulgaris | 38.7 | 860 | 3.7 | 124 |
honge biodiesel HB20 | 42.123 | 835 | 4.12 | 104 |
![]() |
CV [MJ/kg] | Density [kg/m3] | Kinematic Viscosity [mm2/s] |
Flash point [℃] |
hydrogen | 119.93 | 0.0838 | 110 | - |
methanol | 20.30 | 790 | 0.59 | 12 |
ethanol | 27 | 785 | 1.1 | 14 |
CNG | 45.76 | 720 | - | 81.6 |
![]() |
CV [MJ/kg] | Density [g/cm3] | Specific Surface Area (m2g-1) | Thermal conductivity (kW/mK) |
SWCNT | 1.3–1.4 | 1315 | 1750–3800 | |
Graphene | 31.82 | 2.267 | 1598 | 5300 |
Gaseous Additives | ||||||
Ref. | Engine | Fuel | Additive | Method | Performance | Emissions |
[29] | 4-cylinder Diesel | B20 | Al2O3 + H2 | Full load between 1200–2800 rpm | Power ↑ BTE ↑ BSFC ↓ |
CO ↓ CO2 ↑ NOx ↑ |
[37] | 1-cylinder Diesel | B20 |
H2 | Full load. Various speed |
Torque ↑ Power ↑ BTE ↑ |
CO ↓ CO2 ↓ HC ↓ NOx ↑ |
[43] | 1-cylinder Diesel | Diesel | H2 | Constant speed 1500 rpm | BTE ↑ |
CO ↓ CO2 ↓ HC ↓ NOx ↑ |
[45] | 1-cylinder Diesel | Biodiesel | Acetylene | 1500 rpm Different loads |
BTE ↑ |
CO ↓ HC ↓ NOx ↓ |
[38] | 4-cylinder diesel | diesel | LPG | different Speeds and loads | Torque ↑ Power ↑ |
HC ↓ NOx ↓ |
Liquid Additives | ||||||
Ref. | Engine | Fuel | Additive | Method | Performance | Emissions |
[48] | 1-cylinder Diesel | Hydrous ethanol-Diesel | Pentanol and Octanol | Different blends at different loads | Power ↓ BTE ↑ BSFC ↑ |
CO ↓ CO2 ↓ NOx ↓ |
[72] | 1-cylinder Diesel | Diesel | Alcohol | Constant speed 2500 rpm. Different alcohol percentage. | BTE ↓ |
CO ↓ HC ↑ NOx ↑ |
[52] | 1-cylinder Diesel | Biodiesel | Pentanol | 3000 rpm Different engine loads |
BSFC ↑ BTE ↓ |
CO ↓ CO2 ↑ NOx ↓ |
Solid Additives (Metal-based) | ||||||
Ref. | Engine | Fuel | Additive | Method | Performance | Emissions |
[73] | 1-cylinder Diesel |
biodiesel | CuO | Various loads | BTE ↑ BSFC ↓ |
CO ↓ HC ↓ NOx ↑ |
[62] | 1-cylinder Diesel |
Diesel-methanol | Al2O3 | Constant speed 1400 rpm. Different loads | BTE ↑ BSFC ↓ |
CO ↓ HC ↓ NOx ↑ |
[60] | 1-cylinder Diesel |
Waste Cooking Biodiesel | TiO2 | Various engine speeds. Full load | Torque ↑ Power ↑ BSFC ↓ |
CO ↓ CO2 ↑ HC ↓ NO ↑ |
Solid Additives (Carbon-based) | ||||||
Ref. | Engine | Fuel | Additive | Method | Performance | Emissions |
[63] | 1-cylinder Diesel | Diesohol (diesel + alcohol) | CNT | Full load at different rpm | Torque ↑ Power ↑ BTE ↑ BSFC ↓ |
CO ↓ CO2 ↓ HC ↓ NOx ↑ |
[67] | 1-cylinder Diesel | Diesel & Diesel-biodiesel blend | MWCNT | Constant speed 1500 rpm. Different loads | BTE ↑ BSFC ↓ |
CO ↓ NOX↓ |
[64] | 1-cylinder Diesel | Diesel-biodiesel blend | CNT | Full load. Different blends and speed | Power ↑ Torque ↑ BTE ↓ BSFC ↓ |
CO ↓ CO2 ↑ HC ↓ NOX ↑ |
[66] | 1-cylinder Diesel | Palm oil biodiesel | Graphene Oxide Nanoplatelets (GNP) | Varying engine speed. Different blends | BTE ↑ BSFC ↑ |
HC ↓ CO ↑ NOX↓ |
[74] | 1-cylinder Diesel | Jatropha biodiesel | Graphene Nanoplatelets (GNP) | Different Speeds, different loads, different fuel blends | BSFC ↓ | CO ↓ HC ↓ NOX ↓ |
No | Base fuel | Additives/blends | Parameters | Prediction Method | Software/Tool | Reference |
Liquid Additives | ||||||
1 | Waste plastic oil | ethanol | HC, CO, SFC | DOE with Full factorial design | Minitab | [75] |
2 | diesel | DME | BTE, BSEC | Double Weibe | - | [76] |
3 | diesel | higher alcohol additive (Decanol) | BSFC, BTE, CO, CO2, HC, NOx | ANN and RSM | Design Expert | [77] |
4 | diesel | pongamia methyl esters | BTE, UHC, NOx | RSM | Minitab | [78] |
Gaseous Additives | ||||||
5 | diesel | CNG | BSFC, BTE, CO, CO2, HC, NOx | RSM | Minitab and Design Expert | [35] |
6 | diesel | LPG | BSEC, BTE | Fuzzy Logic | ANFIS | [79] |
7 | diesel | Hydrogen |
CO, CO2, HC, NOx | DOE with Taguchi | Minitab | [80] |
Solid Additives (Metal) | ||||||
8 | biodiesel | NiO | BSFC NOx, HC, CO |
RSM | Design Expert | [81] |
9 | biodiesel | Alumina nanoparticles | BSFC, BTE, CO, NOx | RSM | Design Expert | [82] |
10 | biodiesel | TiO2, and Al2O3 nanoparticles | BSFC, BTE, CO, NOx | RSM | Design Expert | [83] |
Solid Additives (Carbon based) | ||||||
11 | diesel and biodiesel | MWCNT | BSFC, CO, NOx | RSM | Design Expert | [83] |
12 | diesel and biodiesel | MWCNT | BTE, BSFC, UHC, CO, NOx | RSM | Design Expert | [84] |
13 | fusel oil | nano- biochar | Torque, Power, BSFC, BTE, CO, NOx | RSM | Design Expert | [85] |