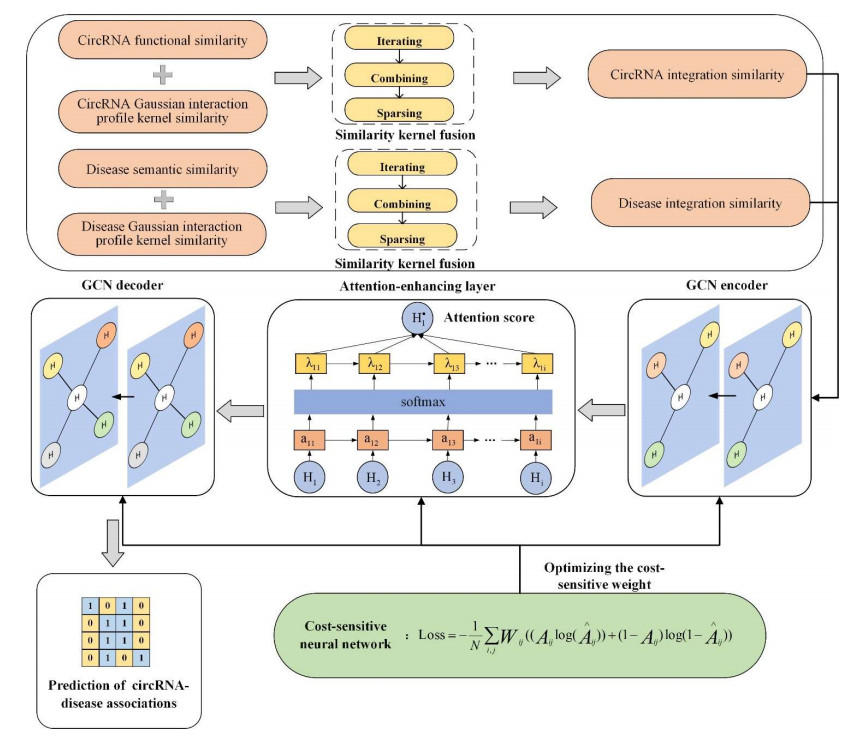
Circular RNAs (circRNAs) constitute a category of circular non-coding RNA molecules whose abnormal expression is closely associated with the development of diseases. As biological data become abundant, a lot of computational prediction models have been used for circRNA–disease association prediction. However, existing prediction models ignore the non-linear information of circRNAs and diseases when fusing multi-source similarities. In addition, these models fail to take full advantage of the vital feature information of high-similarity neighbor nodes when extracting features of circRNAs or diseases. In this paper, we propose a deep learning model, CDA-SKAG, which introduces a similarity kernel fusion algorithm to integrate multi-source similarity matrices to capture the non-linear information of circRNAs or diseases, and construct a circRNA information space and a disease information space. The model embeds an attention-enhancing layer in the graph autoencoder to enhance the associations between nodes with higher similarity. A cost-sensitive neural network is introduced to address the problem of positive and negative sample imbalance, consequently improving our model's generalization capability. The experimental results show that the prediction performance of our model CDA-SKAG outperformed existing circRNA–disease association prediction models. The results of the case studies on lung and cervical cancer suggest that CDA-SKAG can be utilized as an effective tool to assist in predicting circRNA–disease associations.
Citation: Huiqing Wang, Jiale Han, Haolin Li, Liguo Duan, Zhihao Liu, Hao Cheng. CDA-SKAG: Predicting circRNA-disease associations using similarity kernel fusion and an attention-enhancing graph autoencoder[J]. Mathematical Biosciences and Engineering, 2023, 20(5): 7957-7980. doi: 10.3934/mbe.2023345
[1] | Lei Chen, Xiaoyu Zhao . PCDA-HNMP: Predicting circRNA-disease association using heterogeneous network and meta-path. Mathematical Biosciences and Engineering, 2023, 20(12): 20553-20575. doi: 10.3934/mbe.2023909 |
[2] | Huiqing Wang, Sen Zhao, Jing Zhao, Zhipeng Feng . A model for predicting drug-disease associations based on dense convolutional attention network. Mathematical Biosciences and Engineering, 2021, 18(6): 7419-7439. doi: 10.3934/mbe.2021367 |
[3] | Saranya Muniyappan, Arockia Xavier Annie Rayan, Geetha Thekkumpurath Varrieth . DTiGNN: Learning drug-target embedding from a heterogeneous biological network based on a two-level attention-based graph neural network. Mathematical Biosciences and Engineering, 2023, 20(5): 9530-9571. doi: 10.3934/mbe.2023419 |
[4] | Huan Rong, Tinghuai Ma, Xinyu Cao, Xin Yu, Gongchi Chen . TEP2MP: A text-emotion prediction model oriented to multi-participant text-conversation scenario with hybrid attention enhancement. Mathematical Biosciences and Engineering, 2022, 19(3): 2671-2699. doi: 10.3934/mbe.2022122 |
[5] | Jinmiao Song, Shengwei Tian, Long Yu, Qimeng Yang, Qiguo Dai, Yuanxu Wang, Weidong Wu, Xiaodong Duan . RLF-LPI: An ensemble learning framework using sequence information for predicting lncRNA-protein interaction based on AE-ResLSTM and fuzzy decision. Mathematical Biosciences and Engineering, 2022, 19(5): 4749-4764. doi: 10.3934/mbe.2022222 |
[6] | Jie Qiu, Maolin Sun, Chuanshan Zang, Liwei Jiang, Zuorong Qin, Yan Sun, Mingbo Liu, Wenwei Zhang . Five genes involved in circular RNA-associated competitive endogenous RNA network correlates with metastasis in papillary thyroid carcinoma. Mathematical Biosciences and Engineering, 2021, 18(6): 9016-9032. doi: 10.3934/mbe.2021444 |
[7] | Zhi Yang, Kang Li, Haitao Gan, Zhongwei Huang, Ming Shi, Ran Zhou . An Alzheimer's Disease classification network based on MRI utilizing diffusion maps for multi-scale feature fusion in graph convolution. Mathematical Biosciences and Engineering, 2024, 21(1): 1554-1572. doi: 10.3934/mbe.2024067 |
[8] | Zhijing Xu, Jingjing Su, Kan Huang . A-RetinaNet: A novel RetinaNet with an asymmetric attention fusion mechanism for dim and small drone detection in infrared images. Mathematical Biosciences and Engineering, 2023, 20(4): 6630-6651. doi: 10.3934/mbe.2023285 |
[9] | Xiaotong Ji, Dan Liu, Ping Xiong . Multi-model fusion short-term power load forecasting based on improved WOA optimization. Mathematical Biosciences and Engineering, 2022, 19(12): 13399-13420. doi: 10.3934/mbe.2022627 |
[10] | Shuai Cao, Biao Song . Visual attentional-driven deep learning method for flower recognition. Mathematical Biosciences and Engineering, 2021, 18(3): 1981-1991. doi: 10.3934/mbe.2021103 |
Circular RNAs (circRNAs) constitute a category of circular non-coding RNA molecules whose abnormal expression is closely associated with the development of diseases. As biological data become abundant, a lot of computational prediction models have been used for circRNA–disease association prediction. However, existing prediction models ignore the non-linear information of circRNAs and diseases when fusing multi-source similarities. In addition, these models fail to take full advantage of the vital feature information of high-similarity neighbor nodes when extracting features of circRNAs or diseases. In this paper, we propose a deep learning model, CDA-SKAG, which introduces a similarity kernel fusion algorithm to integrate multi-source similarity matrices to capture the non-linear information of circRNAs or diseases, and construct a circRNA information space and a disease information space. The model embeds an attention-enhancing layer in the graph autoencoder to enhance the associations between nodes with higher similarity. A cost-sensitive neural network is introduced to address the problem of positive and negative sample imbalance, consequently improving our model's generalization capability. The experimental results show that the prediction performance of our model CDA-SKAG outperformed existing circRNA–disease association prediction models. The results of the case studies on lung and cervical cancer suggest that CDA-SKAG can be utilized as an effective tool to assist in predicting circRNA–disease associations.
Circular RNAs (circRNAs) constitute a type of covalently closed non-coding RNAs with a loop structure. CircRNAs are resistant to exonuclease digestion and more stable than linear RNAs [1]. Studies have shown that circRNAs have various biological functions, such as regulating the expression of miRNA target genes and affecting protein interactions [2,3,4]. The mutations or dysfunctions of circRNAs can lead to the development of various diseases [5]. Liang et al. [6] found that breast cancer proliferation and progression can be promoted by circCDYL binding of miR-1275-ATG7, suggesting that circCDYL can be a potential molecule for predicting prognosis and treatment response in breast cancer patients. Zhang et al. [7] found that circ_0005015 is significantly upregulated in the fibrovascular membranes of diabetic retinopathy patients, suggesting that circ_0005015 is one of the candidate biomarkers for monitoring diabetic retinopathy. With the development of circRNA research, a variety of circRNAs have been identified as being importantly associated with the generation and development of complex diseases such as gastric cancer, leukemia and diabetes [8,9,10,11]. Therefore, predicting potential circRNA–disease associations is useful for exploring the pathogenesis of complex diseases and identifying additional therapeutic targets and biomarkers to aid disease treatment.
The multi-source similarity fusion algorithm can achieve information complementation between circRNAs similarities and diseases similarities and enrich the features between them, which is important for enhancing the ability to predict circRNA–disease associations [12]. To achieve the fusion of multi-source similarity data, Deepthi and Jereesh [13,14] used an information-filling algorithm to integrate circRNA functional similarity, circRNA Gaussian interaction profile kernel similarity, disease semantic similarity and disease Gaussian interaction profile kernel similarity. The information-filling algorithm replaces missing values with the similarity values of the corresponding nodes in the other remaining similarity matrices for the prediction of circRNA–disease associations. Ma et al. [15] used a linear weighting algorithm to fuse multiple sources of similarity data to predict circRNA–disease associations. Studies have shown that retaining non-linear information of multi-source similarity data facilitates the accuracy of prediction models [16]. However, both the information-filling algorithm and linear weighting algorithm integrate circRNA and disease similarity data in the manner of linear operations. They ignore the information differences existing in circRNA and disease similarity data. Zheng et al. [17] quantified the non-linear relationship of circRNAs by using chaos game representation and demonstrated that capturing the non-linear relationship in data contributed to the prediction of circRNA–disease associations. The similarity kernel fusion algorithm can effectively capture the information differences between circRNAs similarity and diseases similarity by calculating both the neighbor information matrix and the iterative similarity matrix; it then obtains the complex non-linear information effectively [18]. Therefore, we introduce the similarity kernel fusion algorithm to integrate multi-source circRNA and disease similarity data and capture the non-linear information of circRNA and disease. The similarity kernel fusion algorithm can construct more reliable integrated similarity information and construct the circRNA information space and disease information space.
A graph autoencoder (GAE) aggregates neighbor node features to obtain topological information from the graph, and it has shown good performance in predicting circRNA–disease associations. Li et al. [19] proposed GGAECDA for circRNA–disease associations prediction. GGAECDA obtained low-dimensional representations of multi-source similarity data through the use of a graph attention network and random walk with restart algorithm; they used a GAE to extract feature representations of circRNAs and diseases. Wu et al. [20] proposed a deep learning model based on GAE and matrix complementation for the prediction of lncRNA–disease associations. In the above-mentioned model, the graph convolutional network (GCN) layer of the GAE aggregates neighbor information via the adjacency matrix, and it updates the node features to the sum of all neighbor nodes features [21]. However, since all neighbor nodes are given the same weight, important feature information of the higher-similarity neighbor nodes cannot obtain more attention, which restricts the GAE's ability to optimize the feature representation of circRNAs and diseases [22,23]. Therefore, we have embedded an attention-enhancing layer in the GAE to increase the attention of the GAE to high-similarity neighbor features. It enables the adaptive optimization of features and enhances the feature representation capability of the prediction model.
The confirmed human circRNA–disease associations in the CircR2disease dataset are only 650, while there are 50,830 unlabeled samples [13]. Training the model with all unlabeled samples as negative samples may lead to a bias in the prediction results toward classes with a higher number of samples. It will impact the generalization capability of the model and even cause prediction failure [24,25]. To address the imbalance of the samples, Deepthi and Jereesh [13] balanced the positive and negative samples by randomly sampling the negative samples and predicted circRNA–disease associations by using a random forest classifier. Zeng et al. [26] proposed the positive-unlabeled strategy, which uses all positive samples and a subset of unlabeled samples to train the classifier together, training the classifier multiple times to find more reliable negative samples. The above methods improve the recognition rate of positive samples by balancing the number of positive and negative samples. However, they only select the same number of negative samples as positive samples in the full set of negative samples, which cannot take full advantage of all of the data in the dataset; and, their performance relies on the random sampling results. Cost-sensitive neural networks adjust the ratio of majority class to minority class weights in the loss function by re-weighting, instead of balancing the positive and negative samples via random sampling, solving the problem of not fully using all sample data. It has been applied successfully to disease gene identification, compound-protein interactions and so on [27,28,29]. Thus, we introduce a cost-sensitive neural network to address the classification bias of positive and negative samples caused by class imbalance, adjust the weight ratio between samples and improve the generalization capability of the prediction model [30].
Based on the above problems, we propose a deep learning model, CDA-SKAG, with similarity kernel fusion and an attention-enhancing GAE to predict circRNA–disease associations. The framework is shown in Figure 1. In this model, we take circRNA functional similarity, circRNA Gaussian interaction profile kernel similarity, disease semantic similarity and disease Gaussian interaction profile kernel similarity as input. To construct the circRNA information space and disease information space, we introduce the similarity kernel fusion algorithm to integrate multi-source similarity data and obtain non-linear information from the circRNA and disease data. An attention-enhancing GAE is used to capture the circRNA and disease features, enhance the feature weight of neighbor nodes with high similarity and adaptively optimize the circRNA and disease feature components. A cost-sensitive neural network is included to adjust the weight ratio between samples. To validate the predictive performance of CDA-SKAG, we applied a five-fold cross-validation to the CircR2disease dataset and performed case studies on lung and cervical cancers. The experimental results show that the predictive performance of CDA-SKAG is superior to existing circRNA–disease association prediction models. Our model, CDA-SKAG, obtains complex association mechanisms between the circRNA and disease and can be used as an effective tool to assist in predicting circRNA–disease associations.
We collected and downloaded experimentally validated circRNA–disease association data from the CircR2Disease database, which contains 661 circRNAs, 100 diseases and 739 validated circRNA–disease associations [31]. We retained circRNA-disease samples associated with humans and removed redundant entries, resulting in a dataset containing 585 circRNAs, 88 diseases and 650 circRNA–disease associations (hereafter referred to as the circRNA–disease dataset). Let C = {c1, c2, …, cm} and D = {d1, d1, …, dn} be the set of m circRNAs and n diseases in the dataset, respectively. We constructed a binary matrix Y∈Rm∗n of circRNA–disease interactions, where the circRNA–disease association value Y(i,j) = 1 if the association between circRNA i and disease j has been experimentally confirmed, and the value Y(i,j) = 0 if the association between i and j is unknown or unverified.
In this part, we calculate the disease semantic similarity, circRNA functional similarity, circRNA Gaussian interaction profile kernel similarity and disease Gaussian interaction profile kernel similarity as input for the CDA-SKAG model. The dimensions of similarities are shown in Table 1.
Multi-source similarity data | Database | Dimension |
Disease semantic similarity | Mesh | 88 × 88 |
Disease Gaussian interaction profile kernel similarity | CircR2Disease | 88 × 88 |
CircRNA functional similarity | CircR2Disease | 585 × 585 |
CircRNA Gaussian interaction profile kernel similarity | CircR2Disease | 585 × 585 |
Disease semantic similarity is calculated by using the hierarchical ontology method, which calculates the degree of disease similarity based on their relative positional relationships in a directed acyclic graph (DAG) [32]. Based on the description information of the Mesh database for disease relationships, we constructed a DAG to describe the relationships of all diseases. The DAG of disease D includes the ancestor nodes of D and D itself, as well as all direct edges from parent-to-child nodes [32]. According to Wang's method for calculating semantic similarity scores for diseases, we can calculate the semantic similarity scores between each pair of diseases based on their DAGs [33,34]. The detailed formula is as follows:
SD(di,dj)=∑t∈Ndi∩Ndj(Sdi(t)+Sdj(t))∑t∈NdiSdi(t)+∑t∈NdjSdj(t) | (1) |
where Ndi and Ndj denote the ancestral diseases in the graph for disease di and disease dj, respectively. Sdi(t) denotes the semantic value of disease t∈Ndi compared to disease di, and Sdj(t) denotes the semantic value of disease t∈Ndj compared to disease dj. The semantic value of disease d, Sd(t), is defined as follows:
Sd(t)={max{μ∗Sd(d,)d,ϵchildrenof(d)}ift≠d1otherwise | (2) |
CircRNA functional similarity is measured based on the assumption, whereby the higher semantic similarity of the disease group two circRNAs share, the more functionally similar the two circRNAs are [34]. We can calculate the functional similarity of each circRNA pair based on disease semantic similarity according to the method of Deepthi and Jereesh [13], assuming that Di and Dj are the disease groups associated with circRNAs ci and cj, respectively. The circRNA functional similarity matrix can be calculated based on Eqs (3) and (4):
S(ci,cj)=∑1≤q≤|Di|S(dq,Dj)+∑1≤r≤|Dj|S(dr,Di)|Di|+|Dj| | (3) |
S(dq,Dj) represents the calculated similarity with disease dq and disease group Dj:
S(dq,Dj)=max1≤s≤|Dj|(SD(dq,ds)) | (4) |
SD(dq,ds) represents the semantic similarity of dq and ds, where disease dq is related to circRNA ci and disease group Dj is related to circRNA cj. The semantic similarity between diseases and disease groups is calculated by considering disease ontology terms as described in Section 2.2.1.
By obtaining topological information, Gaussian interaction profile kernel similarity is calculated based on the interactions in the circRNA–disease interaction network between nodes [35]. For circRNA ci, the R(ci) value is defined as the ith row of the circRNA–disease association matrix Y. The Gaussian interaction profile kernel similarity between each pair ci and cj is calculated as described by Eqs (5) and (6):
CGS(ci,cj)=exp(−λ||R(ci)−R(cj)||2) | (5) |
λ=11Nc∑Nci=1||R(ci)||2 | (6) |
where CGS(ci,cj) denotes the Gaussian interaction profile kernel similarity between ci and cj. R(ci) and R(cj) respectively denote the ith and jth rows of the association matrix Y; λ is used to control the bandwidth, which denotes the regularized Gaussian interaction profile kernel similarity coefficient constructed based on Nc (the number of circRNAs).
Similarly, the disease Gaussian interaction profile kernel similarity DGS(di,dj) between the two diseases di and dj can be calculated according to Eq (7) and (8), as follows:
DGS(di,dj)=exp(−λ||R(di)−R(dj)||2) | (7) |
λ=11Nd∑Ndi=1||R(di)||2 | (8) |
In our work, we have applied the circRNA functional similarity, circRNA Gaussian interaction profile kernel similarity, disease semantic similarity, disease Gaussian interaction profile kernel similarity and circRNA–disease associations as the input of CDA-SKAG and used CDA-SKAG to learn the features of the circRNAs and diseases. Here, we introduce the similarity kernel fusion algorithm used to integrate the multi-source similarity data; it is purposed to obtain the non-linear information from the circRNA data and disease data. An attention-enhancing GAE is used to acquire the circRNA features and disease features. A cost-sensitive neural network has been introduced to adjust the weight ratio between samples and enhance the generalization capability of the model. The framework of our model, CDA-SKAG, is illustrated in Figure 1.
In this part, we denote the circRNA functional similarity and circRNA Gaussian interaction profile kernel similarity by Sc,m(m=1,2), and Sd,n(n=1,2) denotes the disease semantic similarity and disease Gaussian interaction profile kernel similarity; all four similarities are entered into our model in matrix form. The similarity kernel fusion algorithm fuses the four similarities non-linearly, constructing the circRNA integration similarity matrix S∗c∈Rc∗c and the disease integration similarity matrix S∗d∈Rd∗d. We illustrate the calculation of the similarity kernel fusion algorithm by using the calculation of circRNA integration similarity as a case study, and it is implemented as follows.
First, each circRNA similarity matrix is normalized by using Eq (9).
NSc,m(ci,cj)=Sc,m(ci,cj)∑ck∈CSc,m(ck,cj) | (9) |
NSc,m(ci,cj) denotes the circRNA normalized similarity matrix that satisfies ∑ck∈CNSc,m(ck,cj)=1.
Then, we calculate the contribution of neighbor information for each node in the circRNA similarity matrix by using Eq (10); unrelated nodes are assigned zero to obtain Fc,m.
Fc,m(ci,cj)={Sc,m(ci,cj)∑ck∈NiSc,m(ci,ck)ifcj∈Ni0ifcj∉Ni | (10) |
where Fc,m is a sparse matrix that satisfies ∑cj∈CFc,m(ck,cj)=1, Ni is the set of all neighbors of ci.
We use Eq (11) to calculate the two circRNA similarity matrices (m = 1, 2) separately after t iterations.
SCt+1c,m=α(Fc,m×∑r≠1SCtc,r2×FTc,m)+(1−α)(∑r≠1SC0c,r2) | (11) |
SCt+1c,m is the state matrix of the mth circRNA similarity matrix after t+1 iterations, and SC0c,r denotes the initial state of SCc,r. The transfer probability α is distributed between (0, 1), and the value of α is taken as 0.1.
After Step t+1, we use Eq (12) to calculate the circRNA integration similarity matrix:
Sc=122∑m=1SCt+1c,m | (12) |
In addition, to eliminate the noise in the similarity matrix Sc, we define the following weight matrix wc and calculate the fused circRNA similarity matrix by using Eq (14).
wc(ci,cj)={1ifci∈Nj∧cj∈Ni0ifci∉Nj∧cj∉Ni0.5otherwise | (13) |
S∗c=wc∘Sc | (14) |
Similarly, we normalize the disease matrices to NSd,n(di,dj) and construct the sparse matrix Fd,n(di,dj) for each disease matrix; the two similarity matrices for the diseases can be calculated as SDt+1d,n(n=1,2), respectively. After t+1 iterations, we fuse the two disease similarity matrices as Sd and multiply with the weight matrix wd. We obtain the disease integration similarity, denoted by S∗d∈Rd∗d.
In this part, we design an attention-enhancing GAE for extracting circRNA and disease features. The encoder acquires the embedding feature representations of circRNAs and diseases; the attention-enhancing layer enhances the feature weight of high-similarity neighbors and obtains optimized embedding features; the decoder reconstructs the circRNA–disease association matrix based on the optimized embedding features. It is implemented as follows (extracting circRNA features as an example).
For this model, we use a one-layer GCN as an encoder to extract similarity information from the circRNA and disease information space into the embedding features. For the circRNA information space, we obtain the embedding feature Ec of circRNAs via the encoder:
Ec=Enc(Ac,Y)=tanh(AcYW(0)) | (15) |
Ac=D−12cScD−12c | (16) |
where Y is the circRNA–disease association matrix, SC∈Rnc∗nc denotes the circRNA integration similarity matrix, Ac denotes the regularized circRNA similarity matrix, the normalization is calculated as shown in Eq (16) and Dc∈Rnc∗nc is the degree matrix of the similarity matrix SC. W(i) denotes the weight matrix of the ith neural network layer. The activation function in the encoder is tanh(⋅).
The attention-enhancing layer optimizes the feature representation of node embeddings by calculating the important differences of different neighboring nodes through the use of an attention mechanism [36]. The attention-enhancing layer reinforces the feature representation between highly similar circRNA nodes (Figure 2); moreover, it enables smooth updates of the parameters of the embedding features. We define the loss function LAtt for the attention-enhancing layer in Eq (18), following Gao et al. [37].
L(Hi)=β||Hi−Ei||22−γ∑j∈Niλij||Hi−Hj||22 | (17) |
LAtt=1Nn∑i=1L(Hi) | (18) |
where Ei∈Ec denotes the initial embedding features of node i obtained from the encoder and Hi denotes the updated embedding features of node i in the attention-enhancing layer. In addition, λ denotes the attention score and λij measures the weight of neighbor node j to node i. Ni is the set of all neighbor nodes of node i. The first term in Eq (17) is used for the smoothing update of the embedding features of node i, and the second term makes Hi of node i similar to Hj of neighbor node j. Parameters β and γ are used to balance the weighting factors of the first and second terms on the effect of the embedding features. The embedding features Hi are updated according to the following rules:
H(k+1)i=αEi+β∑j∈NiλijH(k)jα+β∑j∈Niλij | (19) |
The initial value of the embedding feature H(1)i is set to Ei and H(k)i denotes the embedding updated in the kth iteration.
The attention weight between node i and node j is calculated as shown in Eq (21).
aij=Attention(WtHi,WtHj) | (20) |
λij=softmax(aij)=exp(aij)∑x∈Niexp(aix) | (21) |
Attention(⋅) denotes the single-layer feed-forward network for calculating the attention values and Wt denotes the trainable matrix of the hidden layer. We set Hi = H(k)i as the final representation of node i. The value of K was set to 2 in our experiments. The attention-enhancing layer concentrates on extracting features from the nodes of highly similar neighbors so that, as the number of iterations K in each layer increases, nodes will gain more and more information from their more similar neighbors [38].
The decoder consists of a one-layer GCN that reconstructs the prediction matrix with the embedding features obtained from the attention-enhancing layer. The feature embeds Hi from the attention-enhancing layer is combined into a circRNA feature matrix Hc and a disease feature matrix Hd, where Hc∈Rnc∗nc and Hd∈Rnd∗nd, respectively. We feed the embedding feature Hc into the decoder and reconstruct the circRNA–disease association score matrix Dc∈Rnc∗nd. Dc is the prediction of the attention-enhancing GAE based on the circRNA features. The score (ci,dj) of all items in the association matrix represents the probability of association between each circRNA c(i) and each disease d(j). The formula is as follows.
Dc=Dec(Ac,Hc)=sigmoid(AcHcW(1)) | (22) |
W(i) denotes the weight matrix of the ith neural network layer. The activation function of the decoder is sigmoid(⋅).
Finally, we fuse the circRNA association matrix and the disease association matrix to obtain the circRNA–disease association prediction results.
F=δDc+(1−δ)DTd | (23) |
F is the final prediction matrix, where δ ∈ (0, 1) determines the scaling relationship between circRNA features and disease features.
There are only 650 validated positive samples in the circRNA–disease dataset, accounting for 1.26% of all samples [15]. Class imbalance leads to differences in the recognition rates of positive and negative samples. Therefore, we have introduced a cost-sensitive neural network to offset the classification bias caused by class imbalance [30]; the idea is to weaken the impact of sample size differences on classification by increasing the misclassification cost of positive samples. When the model predicts the circRNA–disease associations, the cost-sensitive neural network increases the weight value of positive samples so that the loss value of positive sample misclassification is higher than the loss value of negative samples. It is possible to improve the recognition rate of positive samples in this way. Therefore, we modify the loss function as follows:
L=−1N∑i,jWij⋅(Aij^log(Aij)+(1−Aij)log(1−^Aij)) | (24) |
where Wij denotes the learnable label weight matrix with the same dimensions as the association matrix. The values of the weight matrix are updated by the feed-forward network.
In this study, we used five-fold cross-validation to evaluate the performance of CDA-SKAG. Five statistical metrics were used to evaluate the predictive performance of the proposed model and other comparative models. These metrics include the accuracy (Acc), precision, recall, F1 score and Matthew's correlation coefficient (MCC), which are defined as follows:
Acc=TP+TNTP+TN+FP+FN | (25) |
F1score=2TP∗(TP+FP)∗(TP+FN)2TP+FP+FN | (26) |
Precision=TPTP+FP | (27) |
Recall=TPTP+FN | (28) |
MCC=TP∗TN−FP∗FN√(TP+FN)(TP+FP)(TN+FP)(TN+FN) | (29) |
where TP and TN represent the number of correctly identified results in positive and negative samples, respectively. FP and FN represent the number of incorrectly identified results in positive and negative samples, respectively. Acc represents the proportion of the number of positive and negative samples correctly identified by the model relative to the size of all samples. Precision represents the probability that, of all of the samples with a positive prediction, the true class is also positive. Recall represents the proportion of all positive samples that are correctly predicted by the model, and it is used to assess the ability of the model to identify circRNA–disease association pairs. The circRNA–disease dataset has a problem of sample class imbalance, so we can calculate the F1 score and MCC to measure the accuracy of the predictive model. The F1 score and MCC combine the precision and recall of the model, as well as provide a more accurate evaluation of the predictive performance. In addition, we used the AUC (area under receiver operating characteristics (ROC)) curve and AUPR (area under precision-recall (PR)) curve to evaluate the predictive performance of our model.
In the circRNA–disease association prediction problem, the model parameter settings can affect model predictive performance. In this study, the CDA-SKAG model was implemented using Pytorch 1.8.0. In the process of training the model, we used a cost-sensitive neural network as the loss function and Adam's algorithm as the optimizer to optimize the object function [39]. We set the learning rate to 0.001 and the epoch to 1200 to ensure the stability of the model training process. We performed five-fold cross-validation on the circRNA-disease dataset using CDA-SKAG and plotted the loss curves, the relevant experimental results are shown in Figure A1 of supplementary materials. We set the dropout to 0.5 and the hyperparameter of weight decay to 0.00001 to prevent overfitting of CDA-SKAG. CDA-SKAG consists of similarity kernel fusion, an attention-enhancing GAE and a cost-sensitive neural network, so we will discuss the parameters involved in each component separately in this section.
Convergence is an important factor affecting the fusion of multi-source circRNA and disease similarity data. We concentrate on the value of the number of iterations t. We analyzed the number of iterations to converge for each type of circRNA and disease similarity data. Inspired by the study of Fan et al. [40], we chose to denote the relative errors of circRNA similarities and disease similarities during the iterative process as ECt and EDt, respectively. The number of iterations ranged from 1 to 11 and the step size was 1; ECt and EDt were calculated after each iteration. The convergence process is depicted in Figure 3.
ECt=||SCt+1c,m−SCtc,m||||SCtc,m|| | (30) |
EDt=||Sdt+1d,n−SDtd,n||||SDtd,n|| | (31) |
The results show that the convergence process of the similarity kernel fusion algorithm was fast, with the ECt values reaching 10−10 after six iterations and EDt values reaching 10−10 after ten iterations. The convergence process for two types of circRNA similarities both yielded relative errors of less than 0.01 at the second iteration. The relative errors of circRNA Gaussian interaction profile kernel similarity and circRNA functional similarity reached 10−10 during the sixth and seventh iterations, respectively. Two types of disease similarities obtained a relative error of less than 0.01 at the fifth iteration. The relative errors of disease Gaussian interaction profile kernel similarity and disease semantic similarity reached 10−10 during the 11th iteration. Fan et al. [40] concluded that the relative error of multi-source circRNA and disease similarity data of less than 10−10 could be identified as convergence. Therefore, we selected eleven as the number of iterations for circRNA similarity fusion and disease similarity fusion.
To explore the optimal GCN layer settings for the CDA-SKAG model in this study, we performed a five-fold cross-validation experiment based on the circRNA–disease dataset. Considering the amount of data, when the number of GCN layers exceeded three, it would trigger over-smoothing and impact the predictive performance of CDA-SKAG. Therefore, we set the GCN layers of the GAE to be 1, 2 and 3 layers, respectively, and then recorded the AUC, AUPR, F1 score, Acc, precision, recall and MCC under those different layer setting conditions. The experimental results are shown in Table 2.
Layer | AUC (%) | AUPR (%) | F1score (%) | Acc (%) | Precision (%) | Recall (%) | MCC (%) |
1 | 98.69 | 90.54 | 83.95 | 99.65 | 99.38 | 73.38 | 85.25 |
2 | 96.74 | 64.72 | 61.21 | 99.18 | 77.48 | 51.35 | 62.41 |
3 | 89.90 | 26.26 | 17.57 | 98.82 | 74.31 | 10.22 | 26.47 |
As shown in Table 2, we obtained the highest results when the GCN layer setting was 1. The AUC, AUPR, F1 score, Acc, precision, recall and MCC values all gradually decreased as the number of GCN layers increased. Among them, the AUPR, F1 score and MCC decreased significantly. Over-smoothing appeared when the number of GCN layers exceeded the range of applicability of the dataset [41]. Over-smoothing manifests as the inability of the graph neural network to distinguish the features of different classes of nodes [42]. Too many GCN layers led to over-smoothing of the model, and the predictive model was unable to correctly distinguish between positive and negative samples, reducing the predictive performance of the model. Increasing the number of network layers caused the network to incorrectly identify the nodes' features and reduce the model's predictive capability. CDA-SKAG learned the embeddings, aiming to reconstruct the association matrix to predict the associations of circRNA–disease. Therefore, we selected 1 as the optimal number of layers for the GCN.
To evaluate the impact of the number of iterations of the attention-enhancing layer, we set different iterations K and experimented on the circRNA–disease dataset. K = 1 means that the attention-enhancing layers only calculate the weight values of the node embedding feature without iterating again; K = 2, K = 3 and K = 4 means 1, 2 and 3 iterations, respectively, after calculating the weight of the node embedding feature. We set k to 1, 2, 3 and 4 in the experiment, and the results are shown in Table 3.
Iterations K | AUC (%) | AUPR (%) | F1 score (%) | Acc (%) | Precision (%) | Recall (%) | MCC (%) |
K = 1 | 98.04 | 90.66 | 83.82 | 99.44 | 99.45 | 72.15 | 84.79 |
K = 2 | 98.69 | 90.54 | 83.95 | 99.65 | 99.68 | 73.38 | 85.25 |
K = 3 | 92.93 | 75.76 | 75.36 | 99.50 | 99.68 | 60.46 | 77.56 |
K = 4 | 91.16 | 59.57 | 41.27 | 99.07 | 99.68 | 26.00 | 50.75 |
As we can see in Table 3, with the increase in the number of iterations, the metrics of AUC, F1 score, Acc, precision, recall and MCC increased at first, gradually decreasing after K > 2. The best results were obtained at K = 2. K = 1 indicates that it has calculated the weight value of the node embedding feature and has not reassigned the node embedding feature to optimize the feature combination; thus, the performance of the predictive model still has the possibility to be improved. When the number of iterations K was more than 2, a larger number of iterations generated noise and the node embedding feature was misrepresented during the iterations, impacting the effectiveness of the decoder in reconstructing the circRNA–disease association matrix. Therefore, we chose K = 2 as the optimal number of iterations of the attention-enhancing layer.
We experimented with a five-fold cross-validation of the positive sample weight to explore the optimal parameter selection for the cost-sensitive neural network. We fixed the weight value of negative samples at 1 and set the positive samples with weight values ranging from 1 to 12 respectively. We recorded the corresponding MCC as the evaluation metric. The results are shown in Figure 4.
As shown in Figure 4, the cost-sensitive neural network facilitates balancing the classification bias caused by the number of positive and negative samples. When the positive sample weight was taken as 2, the MCC value was significantly better than the result when the positive sample weight was taken as 1. It is shown that the cost-sensitive neural network can improve the classification accuracy of predictive models for unbalanced datasets and help the models to identify potential circRNA–disease associations more effectively. When the positive sample weight was taken as 5, the model achieved an optimal result of 85.25% for the evaluation metric MCC. As the positive sample weight value exceeded 5, it led to misclassification of some of the negative samples as positive samples and the false positive rate increased, causing the MCC trend to decrease. Therefore, we chose 5 as the positive sample weight value for the subsequent experiments.
In this section, our purpose is to evaluate whether similarity kernel fusion algorithms can integrate circRNA and disease multi-source similarity more effectively. The similarity kernel fusion, information-filling and linear weighting algorithms were each coupled with an attention-enhancing GAE. We conducted a five-fold cross-validation on the circRNA-disease dataset and recorded the AUC, AUPR, F1 score, Acc, precision, recall and MCC values of different fusion strategies; the results are shown in Table 4.
Similarity fusion strategies | AUC (%) | AUPR (%) | F1 score (%) | Acc (%) | Precision (%) | Recall (%) | MCC (%) |
Information-Filling | 89.64 | 20.29 | 27.36 | 97.80 | 23.48 | 32.77 | 26.65 |
Linear Weighting | 91.47 | 36.14 | 43.23 | 98.61 | 52.23 | 42.62 | 42.83 |
Similarity Kernel Fusion | 98.69 | 90.54 | 83.95 | 99.65 | 99.68 | 73.38 | 85.25 |
As can be seen in Table 4, the similarity kernel fusion algorithm achieved the best performance, with higher results for the AUPR, F1 score, precision, recall and MCC than the information-filling and linear weighting algorithms. The similarity kernel fusion algorithm takes into account the influence of neighbor information on node similarity, fuses the non-linear information from different circRNA similarities and disease similarities and achieved a higher accuracy of sample classification compared to the above two linear fusion algorithms. In summary, we have introduced the similarity kernel fusion algorithm to fuse multi-source similarity data non-linearly and provide more accurate feature input for subsequent prediction tasks, helping the model to predict potential circRNA–disease associations more accurately.
To validate the effect of each module in CDA-SKAG for circRNA–disease association prediction, we designed model structural ablation experiments. In our experiments, we composed a baseline model by using a GAE combined with the cross-entropy function to predict circRNA–disease associations. We took the integrated similarity derived from the similarity kernel fusion algorithm as input to the baseline model. The GAE was replaced in the experiments with an attention-enhancing GAE (Att-GAE in Figure 5), and the cross-entropy loss function in the model was replaced with a cost-sensitive neural network to verify the contribution of each module. Each of the model compositions was subjected to a five-fold cross-validation on the circRNA–disease dataset; the results are shown in Figure 5.
The different colors in Figure 5 indicate the different model compositions. Orange represents the baseline model, consists of GAE and cross entropy. Green represents the model that replace GAE with Att-GAE. Comparing the performance indicators of the orange and green models, the figure shows that the F1 score, recall and MCC were improved, indicating that the attention-enhancing GAE has better feature extraction capabilities and can correctly identify more circRNA–disease associations. By comparing the performance indicators of the green and blue models, we can see that the AUPR, F1 score, recall and MCC increased significantly. The F1 score and MCC measured the classification accuracy of the model in the imbalanced dataset, indicating that the cost-sensitive neural network could offset the classification bias caused by class imbalance and improve the accuracy of the model in terms of its ability to identify positive and negative samples.
To evaluate the predictive performance of the models, we compared CDA-SKAG with other existing circRNA–disease association prediction models. We chose six comparison models: DMCCDA [43], DMFCDA [44], GMNN2CD [45], CRPGCN [15], LLCDC [46] and GAMCLDA [20]. Among these models, DMCCDA and GAMCLDA are matrix-completion-based non-coding RNA–disease association prediction models; DMCCDA uses a dual matrix-completion algorithm and GAMCLDA uses a GAE to predict circRNA–disease associations. DMFCDA used a deep neural network to implement a matrix decomposition algorithm for learning the deep features of the circRNA and disease, and for predicting potential associations. LLCDC obtains the reconstructed similarity through locality-constrained linear coding, and it uses label propagation methods to predict the circRNA–disease association matrix. GMNN2CD uses information-filling algorithms as a multi-source similarity fusion method, incorporating graph Markov neural network-extracted features, to predict circRNA–disease associations. CRPGCN uses a linear weighting algorithm to fuse multiple sources of similarity data, and it is combined with a GCN to extract features to predict circRNA–disease associations. We conducted a five-fold cross-validation experiment on the circRNA–disease dataset to compare our model's predictive performance with that of the comparison model; the results are shown in Table 5.
Model | AUC (%) | AUPR (%) | F1 score (%) | Acc (%) | Precision (%) | Recall (%) | MCC (%) |
DMCCDA [43] | 97.71 | 71.75 | 22.9 | 92.56 | 13.17 | 87.54 | 32.28 |
DMFCDA [44] | 91.66 | 55.30 | 44.41 | 97.46 | 30.69 | 90.31 | 48.72 |
GAMCLDA [20] | 94.86 | 72.17 | 24.76 | 92.95 | 14.31 | 91.85 | 34.70 |
GMNN2CD [45] | 94.43 | 60.08 | 60.06 | 99.28 | 99.88 | 42.92 | 65.51 |
CRPGCN [15] | 93.34 | 82.32 | 82.07 | 99.62 | 99.78 | 69.69 | 83.23 |
LLCDC [46] | 94.52 | 83.47 | 74.76 | 99.49 | 99.78 | 59.69 | 77.06 |
CDA-SKAG (Our model) | 98.69 | 90.54 | 83.95 | 99.65 | 99.68 | 73.38 | 85.25 |
As can be seen in Table 5 and Figure 6, CDA-SKAG achieved the highest AUC, AUPR, F1 score, Acc and MCC values. We notice that DMCCDA, DMFCDA and GAMCLDA obtained high recall values. However, the precision values were lower, indicating that these models identified a large number of unconfirmed associations as positive samples in the prediction results. Although more positive samples were identified, a large number of negative samples were misclassified. It suggests that these models failed to obtain the features of negative samples accurately, resulting in a failure to identify circRNA–disease associations accurately. LLCDC uses locality-constrained linear coding, which can retain local information and iteratively update the labels by using label propagation. CDA-SKAG achieved a higher AUPR, MCC and recall than LLCDC. CDA-SKAG not only captures the local information of the nodes, but it also obtains the non-linear features of the nodes themselves, which results in better feature extraction capability. Compared to the graph neural network-based models GMNN2CD and CRPGCN, our CDA-SKAG model obtained better results in terms of the AUC, AUPR, F1 score, Acc, recall and MCC values, whereas the precision metric of CDA-SKAG was equivalent to these two comparison models. It shows that our CDA-SKAG model can not only identify the majority of circRNA–disease associations, but it can also distinguish unrelated circRNA–disease pairs. Thus, CDA-SKAG can predict circRNA–disease associations more effectively.
To demonstrate the effectiveness of CDA-SKAG in predicting novel associations between diseases and circRNAs, we conducted case studies on lung cancer and cervical cancer. In the case studies, we trained CDA-SKAG with known circRNA–disease associations obtained from the CircR2disease database as a dataset and ranked candidate circRNAs for the target diseases based on the prediction scores given by the models in this paper. We selected the top 15 candidate circRNAs and verified their correctness by using the authoritative database circRNA disease [47] or PubMed experimental literature. We have labeled the sources of validation of circRNA–disease associations in the "PMID" columns in Tables 6 and 7.
Rank | Lung cancer | PMID |
1 | hsa_circ_0013958 | PMID: 29241190 |
2 | hsa_circRNA_401977 | PMID: 29241190 |
3 | hsa_circ_0012673 | PMID: 29241190 |
4 | hsa_circRNA_404833 | PMID: 29241190 |
5 | hsa_circ_0043256 | PMID: 29366790 |
6 | hsa_circ_0016760 | PMID: 29620202 |
7 | hsa_circ_0014130 | PMID: 29698681 |
8 | hsa_circ_0007385 | PMID: 29372377 |
9 | hsa_circRNA_006411 | PMID: 29241190 |
10 | hsa_circRNA_100782/circHIPK3/hsa_circ_0000284 | circRNA disease, Circ2 Disease |
11 | hsa_circRNA_104912/hsa_circ_0088442 | circRNA disease |
12 | hsa_circRNA_100855/hsa_circ_0023028 | unconfirmed |
13 | circ-Foxo3/hsa_circ_0006404 | unconfirmed |
14 | hsa_circRNA_100241 | unconfirmed |
15 | hsa_circ_0023404/circRNA_100876/circ-CER | PMID: 28343871 |
Rank | Cervical cancer | PMID |
1 | hsa_circ_0002343 | PMID: 28080204 |
2 | hsa_circ_0069399 | PMID: 28080204 |
3 | hsa_circ_0001187 | PMID: 28282919 |
4 | hsa circ 0031288/circPABPN1 | PMID: 28080204 |
5 | hsa_circRNA_100782/circHIPK3/hsa_circ_0000284 | CircR2disease |
6 | hsa_circ_0008844 | PMID: 28080204 |
7 | hsa_circ_0001212 | PMID: 28080204 |
8 | hsa_circ_0007928 | PMID: 28080204 |
9 | circRNA_102913/hsa_circ_0058058 | unconfirmed |
10 | hsa_circ_0004277 | unconfirmed |
11 | hsa_circ_0004136 | unconfirmed |
12 | hsa_circ_0035381 | unconfirmed |
13 | hsa_circRNA_102683/hsa_circ_0007386 | PMID: 28080204 |
14 | Cir-ITCH/hsa_circ_0001141/hsa_circ_001763 | unconfirmed |
15 | circGFRA1/hsa_circ_005239 | unconfirmed |
The 15 candidate circRNAs are listed in Table 6 as being associated with lung cancer. Two candidate results are included in the databases circRNA disease and CircR2Disease, and they show increased expression levels in lung cancer cells: hsa_circ_0043256 and circHIPK3. In addition, we recognized 10 circRNAs supported by the literature; refer to the list of predictions labeled "PMID" in Table 6. In summary, 12 of the 15 candidate circRNAs for lung cancer predicted by our model CDA-SKAG were confirmed to be associated with the disease.
The 15 candidate circRNAs are listed in Table 7 as being associated with cervical cancer. One candidate result is contained in the CircR2Disease database. The circRNA: hsa_circ_0000284 showed increased expression levels in cervical cancer cells. In addition, we found eight predicted circRNAs supported by the literature. Nine of the 15 candidate circRNAs predicted by CDA-SKAG for cervical cancer were confirmed to be associated with the disease. Thus, CDA-SKAG enables accurate prediction of novel associations between diseases and circRNAs based on the existing experimental results. It implies that CDA-SKAG can capture the complex structure of circRNA–disease association data and is capable of robustly inferring potential associations.
In this paper, we have proposed CDA-SKAG for the prediction of potential circRNA–disease associations, and it is based on a similarity kernel fusion algorithm and an attention-enhancing GAE. CDA-SKAG integrates multi-source similarity data by using a similarity kernel fusion algorithm to construct the circRNA information space and disease information space. An attention-enhancing GAE is used to extract circRNA and disease features and predict potential circRNA–disease associations. A cost-sensitive neural network has been added to resolve the widespread class imbalance in circRNA–disease association datasets. By comparing the performance with existing circRNA–disease association predictive models, the results show that our CDA-SKAG model has comparable or even better predictive performance than existing association-based predictive models. Ablation experiments of the multi-source similarity fusion strategy and model architecture show that the similarity kernel fusion algorithm takes into account the non-linear information of circRNA and disease to obtain more reliable integrated similarity information; the combination of an attention-enhancing GAE and a cost-sensitive neural network can effectively process circRNA and disease features and help to predict circRNA–disease association more accurately. The current version of CDA-SKAG still has certain limitations. First, the model relies on known association data and multi-source similarity, while the variety of multi-source similarity data is limited. Second, present methods are limited by the selection of negative samples, which heavily impacts the prediction results' reliability. Finally, there is still room for improvement of the multi-source similarity fusion strategy. In the future, we can learn important studies to improve the association-based predictive model for the related fields of circRNA, such as lncRNA–miRNA interaction prediction, circRNA–miRNA association prediction and metabolite–disease association prediction [48,49,50,51]. The introduction of a variety of circRNA and disease data, such as miRNA–disease association data, circRNA–miRNA interaction data and the structural embedding feature of circRNA, will enrich the multi-source information of circRNAs and diseases [49,51]. With the continuous development of deep learning research and the increasing amount of data, we believe that deep learning will play a more significant role in predicting circRNA and disease association in the future.
This research was funded by the National Natural Science Foundation of China, grant number 62176177; and the Natural Science Foundation of Shanxi Province, grant number 202203021211121.
The authors declare that there is no conflict of interest.
[1] |
W. R. Jeck, N. E. Sharpless, Detecting and characterizing circular RNAs, Nat. Biotechnol., 32 (2014), 453–461. https://doi.org/10.1038/nbt.2890 doi: 10.1038/nbt.2890
![]() |
[2] |
L. Salmena, L. Poliseno, Y. Tay, L. Kats, P. Pandolfi, A ceRNA hypothesis: the Rosetta Stone of a hidden RNA language, Cell, 146 (2011), 353–358. https://doi.org/10.1016/j.cell.2011.07.014 doi: 10.1016/j.cell.2011.07.014
![]() |
[3] |
Y. Zhang, X. Zhang, T. Chen, J. Xiang, Q. Yin, Y. Xing, Circular intronic long noncoding RNAs, Mol. Cell, 51 (2013), 792–806. https://doi.org/10.1016/j.molcel.2013.08.017 doi: 10.1016/j.molcel.2013.08.017
![]() |
[4] |
C. Wang, C. Han, Q. Zhao, X. Chen, Circular RNAs and complex diseases: from experimental results to computational models, Brief. Bioinform., 22 (2021), 1–27. https://doi.org/10.1093/bib/bbab286 doi: 10.1093/bib/bbab286
![]() |
[5] |
V. M. Conn, V. Hugouvieux, A. Nayak, S. A. Conos, G. Capovilla, G. Cildir, A circRNA from SEPALLATA3 regulates splicing of its cognate mRNA through R-loop formation, Nat. Plants, 3 (2017), 1–5. https://doi.org/10.1038/nplants.2017.53 doi: 10.1038/nplants.2017.53
![]() |
[6] |
G. Liang, Y. Ling, M. Mehrpour, P. E. Saw, Z. Liu, W. Tan, Autophagy-associated circRNA circCDYL augments autophagy and promotes breast cancer progression, Mol Cancer, 19 (2020), 1–16. https://doi.org/10.1186/s12943-020-01152-2 doi: 10.1186/s12943-020-01152-2
![]() |
[7] |
S. Zhang, X. Chen, C. Li, X. Li, Identification and characterization of circular RNAs as a new class of putative biomarkers in diabetes retinopathy, Invest. Ophthalmol. Vis. Sci., 58 (2017), 6500–6509. https://doi.org/10.1167/iovs.17-22698 doi: 10.1167/iovs.17-22698
![]() |
[8] |
C. Ma, X. Wang, F. Yang, Y. Zang, J. Liu, X. Wang, Circular RNA hsa_circ_0004872 inhibits gastric cancer progression via the miR-224/Smad4/ADAR1 successive regulatory circuit, Mol. Cancer, 19 (2020), 1–21. https://doi.org/10.1186/s12943-020-01268-5 doi: 10.1186/s12943-020-01268-5
![]() |
[9] |
M. Jamal, T. Song, B. Chen, M. Faisal, Z. Hong, T. Xie, Recent progress on circular RNA research in acute myeloid leukemia, Front. Oncol., 9 (2019), 1–13. https://doi.org/10.3389/fonc.2019.01108 doi: 10.3389/fonc.2019.01108
![]() |
[10] |
J. Zhang, H. Sun, Roles of circular RNAs in diabetic complications: From molecular mechanisms to therapeutic potential, Gene, 763 (2020), 1–11. https://doi.org/10.1016/j.gene.2020.145066 doi: 10.1016/j.gene.2020.145066
![]() |
[11] |
Z. Mohamed, circRNAs signature as potential diagnostic and prognostic biomarker for diabetes mellitus and related cardiovascular complications, Cells, 9 (2020), 1–19. https://doi.org/10.3390/cells9030659 doi: 10.3390/cells9030659
![]() |
[12] |
Y. Zhou, J. Hu, Z. Shen, W. Zhang, P. Du, LPI-SKF: predicting lncRNA-protein interactions using similarity kernel fusions, Front. Genet., 11 (2020), 1–11. https://doi.org/10.3389/fgene.2020.615144 doi: 10.3389/fgene.2020.615144
![]() |
[13] |
K. Deepthi, A. S. Jereesh, Inferring potential CircRNA–disease associations via deep autoencoder-based classification, Mol. Diagn. Ther, 25 (2021), 87–97. https://doi.org/10.1007/s40291-020-00499-y doi: 10.1007/s40291-020-00499-y
![]() |
[14] |
K. Deepthi, A. S. Jereesh, An ensemble approach for circRNA–disease association prediction based on autoencoder and deep neural network, Gene, 762 (2020), 1–7. https://doi.org/10.1016/j.gene.2020.145040 doi: 10.1016/j.gene.2020.145040
![]() |
[15] |
Z. Ma, Z. Kuang, L. Deng, CRPGCN: predicting circRNA–disease associations using graph convolutional network based on heterogeneous network, BMC Bioinform., 22 (2021), 1–23. https://doi.org/10.1186/s12859-021-04467-z doi: 10.1186/s12859-021-04467-z
![]() |
[16] |
C. Shi, B. Hu, W. Zhao, P. Yu, Heterogeneous information network embedding for recommendation, IEEE Trans. Knowl. Data Eng., 31 (2018), 357–370. https://doi.org/10.1109/TKDE.2018.2833443 doi: 10.1109/TKDE.2018.2833443
![]() |
[17] |
K. Zheng, Z. You, J. Li, L. Wang, Z. Guo, Y. Huang, iCDA-CGR: Identification of circRNA–disease associations based on chaos game representation, PLoS Comput. Biol., 16 (2020), 1–22. https://doi.org/10.1371/journal.pcbi.1007872 doi: 10.1371/journal.pcbi.1007872
![]() |
[18] |
L. Jiang, Y. Ding, J. Tang, F. Guo, MDA-SKF: similarity kernel fusion for accurately discovering miRNA-disease association, Front. Genet., 9 (2018), 1–13. https://doi.org/10.3389/fgene.2018.00618 doi: 10.3389/fgene.2018.00618
![]() |
[19] |
G. Li, Y. Lin, J. Luo, Q. Xiao, C. Liang, GGAECDA: Predicting circRNA–disease associations using graph autoencoder based on graph representation learning, Comput. Biol. Chem., 99 (2022), 1–10. https://doi.org/10.1016/j.compbiolchem.2022.107722 doi: 10.1016/j.compbiolchem.2022.107722
![]() |
[20] |
X. Wu, W. Lan, Q. Chen, Y. Dong, J. Liu, W. Peng, Inferring LncRNA-disease associations based on graph autoencoder matrix completion, Comput. Biol. Chem., 87 (2020), 1–7. https://doi.org/10.1016/j.compbiolchem.2020.107282 doi: 10.1016/j.compbiolchem.2020.107282
![]() |
[21] | T. N. Kipf, M. Welling, Variational graph auto-encoders, arXiv e-prints, 2016, 1–3. https://arXiv.org/abs/1611.07308 |
[22] |
W. Wang, L. Zhang, J. Sun, Q. Zhao, J. Shuai, Predicting the potential human lncRNA–miRNA interactions based on graph convolution network with conditional random field, Brief. Bioinform., 23 (2022), 1–9. https://doi.org/10.1093/bib/bbac463 doi: 10.1093/bib/bbac463
![]() |
[23] | L. Wang, Z. You, D. Huang, J. Li, MGRCDA: Metagraph recommendation method for predicting circRNA–disease association, in IEEE Transactions on Cybernetics, 53 (2023), 67–75. https://doi.org/10.1109/TCYB.2021.3090756 |
[24] | B. Kang, S. Xie, M. Rohrbach, Z. Yan, A. Gordo, Decoupling representation and classifier for long-tailed recognition, in International Conference on Learning Representations, (2019), 1–14. https://arXiv.org/abs/1910.09217 |
[25] |
H. Guo, Y. Li, J. Shang, M. Gu, Y. Huang, B. Gong, Learning from class-imbalanced data: Review of methods and applications, Expert Syst. Appl., 73 (2017), 220–239. https://doi.org/10.1016/j.eswa.2016.12.035 doi: 10.1016/j.eswa.2016.12.035
![]() |
[26] |
X. Zeng, Y. Zhong, W. Lin, Q. Zou, Predicting disease-associated circular RNAs using deep forests combined with positive-unlabeled learning methods, Brief. Bioinform., 21 (2020), 1425–1436. https://doi.org/10.1093/bib/bbz080 doi: 10.1093/bib/bbz080
![]() |
[27] |
P. Yang, X. Li, J. Mei, C. Kwoh, S. Ng, Positive-unlabeled learning for disease gene identification, Bioinformatics, 28 (2012), 2640–2647. https://doi.org/10.1093/bioinformatics/bts504 doi: 10.1093/bioinformatics/bts504
![]() |
[28] |
Z. Cheng, S. Zhou, Y. Wang, H. Liu, J. Guan, Effectively identifying compound-protein interactions by learning from positive and unlabeled examples, IEEE/ACM Trans Comput. Biol. Bioinform., 15 (2016), 1832–1843. https://doi.org/10.1109/TCBB.2016.2570211 doi: 10.1109/TCBB.2016.2570211
![]() |
[29] |
L. Wang, L. Wong, Z. Li, Y. Huang, X. Su, B. Zhao, Z. You, A machine learning framework based on multi-source feature fusion for circRNA–disease association prediction, Brief. Bioinform., 23 (2022), 1–9. https://doi.org/10.1093/bib/bbac388 doi: 10.1093/bib/bbac388
![]() |
[30] | C. Wan, L. Wang, K. Ting, Introducing cost-sensitive neural networks, in Processing of The Second International Conference on information, Communications, and Signal Processing (ICICS 99), (1999), 1–4. |
[31] |
C. Fan, X. Lei, Z. Fang, Q. Jiang, F. Wu, CircR2Disease: a manually curated database for experimentally supported circular RNAs associated with various diseases, Database, 2018 (2018), 1–6. https://doi.org/10.1093/database/bay044 doi: 10.1093/database/bay044
![]() |
[32] |
L. M. Schriml, C. Arze, S. Nadendla, Y. Chang, M. Mazaitis, V. Felix, et al., Disease ontology: a backbone for disease semantic integration, Nucleic Acids Res., 40 (2012), 940–946. https://doi.org/10.1093/nar/gkr972 doi: 10.1093/nar/gkr972
![]() |
[33] |
G. Yu, L. Wang, G. Yan, Q. He, DOSE: an R/Bioconductor package for disease ontology semantic and enrichment analysis, Bioinformatics, 31 (2015), 608–609. https://doi.org/10.1093/bioinformatics/btu684 doi: 10.1093/bioinformatics/btu684
![]() |
[34] |
D. Wang, J. Wang, M. Lu, F. Song, Q. Cui, Inferring the human microRNA functional similarity and functional network based on microRNA-associated diseases, Bioinformatics, 26 (2010), 1644–1650. https://doi.org/10.1093/bioinformatics/btq241 doi: 10.1093/bioinformatics/btq241
![]() |
[35] |
T. V. Laarhoven, S. B. Nabuurs, E. Marchiori, Gaussian interaction profile kernels for predicting drug–target interaction, Bioinformatics, 27 (2011), 3036–3043. https://doi.org/10.1093/bioinformatics/btr500 doi: 10.1093/bioinformatics/btr500
![]() |
[36] | D. Bahdanau, K. Cho, Y. Bengio, Neural machine translation by jointly learning to align and translate, in International Conference on Learning Representations, (2015), 1–15. https://arXiv.org/abs/1409.0473 |
[37] | H. Gao, J. Pei, H. Huang, Conditional random field enhanced graph convolutional neural networks, in Proceedings of the 25th ACM SIGKDD International Conference on Knowledge Discovery & Data Mining, (2019), 276–284. https://doi.org/10.1145/3292500.3330888 |
[38] |
Y. Long, M. Wu, C. K. Kwoh, J. Luo, X. Li, Predicting human microbe–drug associations via graph convolutional network with conditional random field, Bioinformatics, 36 (2020), 4918–4927. https://doi.org/10.1093/bioinformatics/btaa598 doi: 10.1093/bioinformatics/btaa598
![]() |
[39] | D. P. Kingma, J. Ba, Adam: A method for stochastic optimization, in International Conference on Learning Representations, (2014), 1–15. https://arXiv.org/abs/1412.6980 |
[40] |
C. Fan, X. Lei, Y. Pan, Prioritizing CircRNA–disease associations with convolutional neural network based on multiple similarity feature fusion, Front. Genet., 11 (2020), 1–13. https://doi.org/10.3389/fgene.2020.540751 doi: 10.3389/fgene.2020.540751
![]() |
[41] | Q. Li, Z. Han, X. Wu, Deeper insights into graph convolutional networks for semi-supervised learning, Proceed. AAAI, 32 (2018), 3538–3545. https://arXiv.org/abs/1801.07606 |
[42] |
D. Chen, Y. Lin, W. Li, P. Li, J. Zhou, X. Sun, Measuring and relieving the over-smoothing problem for graph neural networks from the topological view, Proceed. AAAI Conf. Artif. Intell., 34 (2020), 3438–3445. https://doi.org/10.1609/aaai.v34i04.5747 doi: 10.1609/aaai.v34i04.5747
![]() |
[43] |
Z. Zuo, R. Cao, P. Wei, J. Xia, C. Zheng, Double matrix completion for circRNA–disease association prediction, BMC Bioinform., 22 (2021), 1–15. https://doi.org/10.1186/s12859-021-04231-3 doi: 10.1186/s12859-021-04231-3
![]() |
[44] |
C. Lu, M. Zeng, F. Zhang, F. Wu, M. Li, J. Wang, Deep matrix factorization improves prediction of human circRNA–disease associations, IEEE J. Biomed. Health Inform., 25 (2020), 891–899. https://doi.org/10.1109/JBHI.2020.2999638 doi: 10.1109/JBHI.2020.2999638
![]() |
[45] |
M. Niu, Q. Zou, C. Wang, GMNN2CD: identification of circRNA–disease associations based on variational inference and graph Markov neural networks, Bioinformatics, 38 (2022), 2246–2253. https://doi.org/10.1093/bioinformatics/btac079 doi: 10.1093/bioinformatics/btac079
![]() |
[46] |
E. Ge, Y. Yang, M. Gang, C. Fan, Q. Zhao, Predicting human disease-associated circRNAs based on locality-constrained linear coding, Genomics, 112 (2020), 1335–1342. https://doi.org/10.1016/j.ygeno.2019.08.001 doi: 10.1016/j.ygeno.2019.08.001
![]() |
[47] |
Z. Zhao, K. Wang, F. Wu, W. Wang, K. Zhang, H. Hu, circRNA disease: a manually curated database of experimentally supported circRNA–disease associations, Cell Death Dis., 9 (2018), 1–2. https://doi.org/10.1038/s41419-018-0503-3 doi: 10.1038/s41419-018-0503-3
![]() |
[48] |
Q. Zhao, Y. Yang, G. Ren, E. Ge, C. Fan, Integrating bipartite network projection and KATZ measure to identify novel circRNA–disease associations, IEEE Trans. Nanobiosci., 18 (2019), 578–584. https://doi.org/10.1109/TNB.2019.2922214 doi: 10.1109/TNB.2019.2922214
![]() |
[49] |
L. Zhang, P. Yang, H. Feng, Q. Zhao, H. Liu, Using network distance analysis to predict lncRNA–miRNA interactions, Interdiscip. Sci. Comput. Life Sci., 13 (2021), 535–545. https://doi.org/10.1007/s12539-021-00458-z doi: 10.1007/s12539-021-00458-z
![]() |
[50] |
F. Sun, J. Sun, Q. Zhao, A deep learning method for predicting metabolite–disease associations via graph neural network, Brief. Bioinform., 23 (2022), 1–11. https://doi.org/10.1093/bib/bbac266 doi: 10.1093/bib/bbac266
![]() |
[51] |
L. Guo, Z. You, L. Wang, C. Yu, B. Zhao, Z. Ren, et al., A novel circRNA-miRNA association prediction model based on structural deep neural network embedding, Brief. Bioinform., 23 (2022), 1–10. https://doi.org/10.1093/bib/bbac391 doi: 10.1093/bib/bbac391
![]() |
1. | Pengli Lu, Wenqi Zhang, Jinkai Wu, AMPCDA: Prediction of circRNA–disease associations by utilizing attention mechanisms on metapaths, 2024, 108, 14769271, 107989, 10.1016/j.compbiolchem.2023.107989 | |
2. | Sanghyuk Roy Choi, Minhyeok Lee, Transformer Architecture and Attention Mechanisms in Genome Data Analysis: A Comprehensive Review, 2023, 12, 2079-7737, 1033, 10.3390/biology12071033 | |
3. | Hanyuan Liu, Xuelin Yao, Ying Zhou, Liang Chen, CircRNA-based therapeutics: Current opinions and clinical potential, 2024, 2, 2959-8745, 100081, 10.59717/j.xinn-med.2024.100081 | |
4. | Zhihao Ma, Guitao Cao, Wenming Cao, Adaptive Meta-Path Selection Based Heterogeneous Spatial Enhancement for circRNA-Disease Associations Prediction, 2025, 29, 2168-2194, 3792, 10.1109/JBHI.2024.3523391 |
Multi-source similarity data | Database | Dimension |
Disease semantic similarity | Mesh | 88 × 88 |
Disease Gaussian interaction profile kernel similarity | CircR2Disease | 88 × 88 |
CircRNA functional similarity | CircR2Disease | 585 × 585 |
CircRNA Gaussian interaction profile kernel similarity | CircR2Disease | 585 × 585 |
Layer | AUC (%) | AUPR (%) | F1score (%) | Acc (%) | Precision (%) | Recall (%) | MCC (%) |
1 | 98.69 | 90.54 | 83.95 | 99.65 | 99.38 | 73.38 | 85.25 |
2 | 96.74 | 64.72 | 61.21 | 99.18 | 77.48 | 51.35 | 62.41 |
3 | 89.90 | 26.26 | 17.57 | 98.82 | 74.31 | 10.22 | 26.47 |
Iterations K | AUC (%) | AUPR (%) | F1 score (%) | Acc (%) | Precision (%) | Recall (%) | MCC (%) |
K = 1 | 98.04 | 90.66 | 83.82 | 99.44 | 99.45 | 72.15 | 84.79 |
K = 2 | 98.69 | 90.54 | 83.95 | 99.65 | 99.68 | 73.38 | 85.25 |
K = 3 | 92.93 | 75.76 | 75.36 | 99.50 | 99.68 | 60.46 | 77.56 |
K = 4 | 91.16 | 59.57 | 41.27 | 99.07 | 99.68 | 26.00 | 50.75 |
Similarity fusion strategies | AUC (%) | AUPR (%) | F1 score (%) | Acc (%) | Precision (%) | Recall (%) | MCC (%) |
Information-Filling | 89.64 | 20.29 | 27.36 | 97.80 | 23.48 | 32.77 | 26.65 |
Linear Weighting | 91.47 | 36.14 | 43.23 | 98.61 | 52.23 | 42.62 | 42.83 |
Similarity Kernel Fusion | 98.69 | 90.54 | 83.95 | 99.65 | 99.68 | 73.38 | 85.25 |
Model | AUC (%) | AUPR (%) | F1 score (%) | Acc (%) | Precision (%) | Recall (%) | MCC (%) |
DMCCDA [43] | 97.71 | 71.75 | 22.9 | 92.56 | 13.17 | 87.54 | 32.28 |
DMFCDA [44] | 91.66 | 55.30 | 44.41 | 97.46 | 30.69 | 90.31 | 48.72 |
GAMCLDA [20] | 94.86 | 72.17 | 24.76 | 92.95 | 14.31 | 91.85 | 34.70 |
GMNN2CD [45] | 94.43 | 60.08 | 60.06 | 99.28 | 99.88 | 42.92 | 65.51 |
CRPGCN [15] | 93.34 | 82.32 | 82.07 | 99.62 | 99.78 | 69.69 | 83.23 |
LLCDC [46] | 94.52 | 83.47 | 74.76 | 99.49 | 99.78 | 59.69 | 77.06 |
CDA-SKAG (Our model) | 98.69 | 90.54 | 83.95 | 99.65 | 99.68 | 73.38 | 85.25 |
Rank | Lung cancer | PMID |
1 | hsa_circ_0013958 | PMID: 29241190 |
2 | hsa_circRNA_401977 | PMID: 29241190 |
3 | hsa_circ_0012673 | PMID: 29241190 |
4 | hsa_circRNA_404833 | PMID: 29241190 |
5 | hsa_circ_0043256 | PMID: 29366790 |
6 | hsa_circ_0016760 | PMID: 29620202 |
7 | hsa_circ_0014130 | PMID: 29698681 |
8 | hsa_circ_0007385 | PMID: 29372377 |
9 | hsa_circRNA_006411 | PMID: 29241190 |
10 | hsa_circRNA_100782/circHIPK3/hsa_circ_0000284 | circRNA disease, Circ2 Disease |
11 | hsa_circRNA_104912/hsa_circ_0088442 | circRNA disease |
12 | hsa_circRNA_100855/hsa_circ_0023028 | unconfirmed |
13 | circ-Foxo3/hsa_circ_0006404 | unconfirmed |
14 | hsa_circRNA_100241 | unconfirmed |
15 | hsa_circ_0023404/circRNA_100876/circ-CER | PMID: 28343871 |
Rank | Cervical cancer | PMID |
1 | hsa_circ_0002343 | PMID: 28080204 |
2 | hsa_circ_0069399 | PMID: 28080204 |
3 | hsa_circ_0001187 | PMID: 28282919 |
4 | hsa circ 0031288/circPABPN1 | PMID: 28080204 |
5 | hsa_circRNA_100782/circHIPK3/hsa_circ_0000284 | CircR2disease |
6 | hsa_circ_0008844 | PMID: 28080204 |
7 | hsa_circ_0001212 | PMID: 28080204 |
8 | hsa_circ_0007928 | PMID: 28080204 |
9 | circRNA_102913/hsa_circ_0058058 | unconfirmed |
10 | hsa_circ_0004277 | unconfirmed |
11 | hsa_circ_0004136 | unconfirmed |
12 | hsa_circ_0035381 | unconfirmed |
13 | hsa_circRNA_102683/hsa_circ_0007386 | PMID: 28080204 |
14 | Cir-ITCH/hsa_circ_0001141/hsa_circ_001763 | unconfirmed |
15 | circGFRA1/hsa_circ_005239 | unconfirmed |
Multi-source similarity data | Database | Dimension |
Disease semantic similarity | Mesh | 88 × 88 |
Disease Gaussian interaction profile kernel similarity | CircR2Disease | 88 × 88 |
CircRNA functional similarity | CircR2Disease | 585 × 585 |
CircRNA Gaussian interaction profile kernel similarity | CircR2Disease | 585 × 585 |
Layer | AUC (%) | AUPR (%) | F1score (%) | Acc (%) | Precision (%) | Recall (%) | MCC (%) |
1 | 98.69 | 90.54 | 83.95 | 99.65 | 99.38 | 73.38 | 85.25 |
2 | 96.74 | 64.72 | 61.21 | 99.18 | 77.48 | 51.35 | 62.41 |
3 | 89.90 | 26.26 | 17.57 | 98.82 | 74.31 | 10.22 | 26.47 |
Iterations K | AUC (%) | AUPR (%) | F1 score (%) | Acc (%) | Precision (%) | Recall (%) | MCC (%) |
K = 1 | 98.04 | 90.66 | 83.82 | 99.44 | 99.45 | 72.15 | 84.79 |
K = 2 | 98.69 | 90.54 | 83.95 | 99.65 | 99.68 | 73.38 | 85.25 |
K = 3 | 92.93 | 75.76 | 75.36 | 99.50 | 99.68 | 60.46 | 77.56 |
K = 4 | 91.16 | 59.57 | 41.27 | 99.07 | 99.68 | 26.00 | 50.75 |
Similarity fusion strategies | AUC (%) | AUPR (%) | F1 score (%) | Acc (%) | Precision (%) | Recall (%) | MCC (%) |
Information-Filling | 89.64 | 20.29 | 27.36 | 97.80 | 23.48 | 32.77 | 26.65 |
Linear Weighting | 91.47 | 36.14 | 43.23 | 98.61 | 52.23 | 42.62 | 42.83 |
Similarity Kernel Fusion | 98.69 | 90.54 | 83.95 | 99.65 | 99.68 | 73.38 | 85.25 |
Model | AUC (%) | AUPR (%) | F1 score (%) | Acc (%) | Precision (%) | Recall (%) | MCC (%) |
DMCCDA [43] | 97.71 | 71.75 | 22.9 | 92.56 | 13.17 | 87.54 | 32.28 |
DMFCDA [44] | 91.66 | 55.30 | 44.41 | 97.46 | 30.69 | 90.31 | 48.72 |
GAMCLDA [20] | 94.86 | 72.17 | 24.76 | 92.95 | 14.31 | 91.85 | 34.70 |
GMNN2CD [45] | 94.43 | 60.08 | 60.06 | 99.28 | 99.88 | 42.92 | 65.51 |
CRPGCN [15] | 93.34 | 82.32 | 82.07 | 99.62 | 99.78 | 69.69 | 83.23 |
LLCDC [46] | 94.52 | 83.47 | 74.76 | 99.49 | 99.78 | 59.69 | 77.06 |
CDA-SKAG (Our model) | 98.69 | 90.54 | 83.95 | 99.65 | 99.68 | 73.38 | 85.25 |
Rank | Lung cancer | PMID |
1 | hsa_circ_0013958 | PMID: 29241190 |
2 | hsa_circRNA_401977 | PMID: 29241190 |
3 | hsa_circ_0012673 | PMID: 29241190 |
4 | hsa_circRNA_404833 | PMID: 29241190 |
5 | hsa_circ_0043256 | PMID: 29366790 |
6 | hsa_circ_0016760 | PMID: 29620202 |
7 | hsa_circ_0014130 | PMID: 29698681 |
8 | hsa_circ_0007385 | PMID: 29372377 |
9 | hsa_circRNA_006411 | PMID: 29241190 |
10 | hsa_circRNA_100782/circHIPK3/hsa_circ_0000284 | circRNA disease, Circ2 Disease |
11 | hsa_circRNA_104912/hsa_circ_0088442 | circRNA disease |
12 | hsa_circRNA_100855/hsa_circ_0023028 | unconfirmed |
13 | circ-Foxo3/hsa_circ_0006404 | unconfirmed |
14 | hsa_circRNA_100241 | unconfirmed |
15 | hsa_circ_0023404/circRNA_100876/circ-CER | PMID: 28343871 |
Rank | Cervical cancer | PMID |
1 | hsa_circ_0002343 | PMID: 28080204 |
2 | hsa_circ_0069399 | PMID: 28080204 |
3 | hsa_circ_0001187 | PMID: 28282919 |
4 | hsa circ 0031288/circPABPN1 | PMID: 28080204 |
5 | hsa_circRNA_100782/circHIPK3/hsa_circ_0000284 | CircR2disease |
6 | hsa_circ_0008844 | PMID: 28080204 |
7 | hsa_circ_0001212 | PMID: 28080204 |
8 | hsa_circ_0007928 | PMID: 28080204 |
9 | circRNA_102913/hsa_circ_0058058 | unconfirmed |
10 | hsa_circ_0004277 | unconfirmed |
11 | hsa_circ_0004136 | unconfirmed |
12 | hsa_circ_0035381 | unconfirmed |
13 | hsa_circRNA_102683/hsa_circ_0007386 | PMID: 28080204 |
14 | Cir-ITCH/hsa_circ_0001141/hsa_circ_001763 | unconfirmed |
15 | circGFRA1/hsa_circ_005239 | unconfirmed |