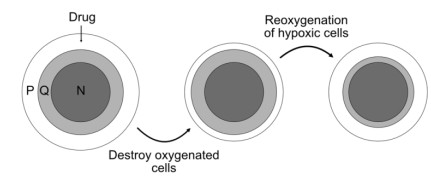
Citation: Bertin Hoffmann, Udo Schumacher, Gero Wedemann. Absence of convection in solid tumors caused by raised interstitial fluid pressure severely limits success of chemotherapy—a numerical study in cancers[J]. Mathematical Biosciences and Engineering, 2020, 17(5): 6128-6148. doi: 10.3934/mbe.2020325
[1] | Alessandro Bertuzzi, Antonio Fasano, Alberto Gandolfi, Carmela Sinisgalli . Interstitial Pressure And Fluid Motion In Tumor Cords. Mathematical Biosciences and Engineering, 2005, 2(3): 445-460. doi: 10.3934/mbe.2005.2.445 |
[2] | Ernesto A. B. F. Lima, Patrick N. Song, Kirsten Reeves, Benjamin Larimer, Anna G. Sorace, Thomas E. Yankeelov . Predicting response to combination evofosfamide and immunotherapy under hypoxic conditions in murine models of colon cancer. Mathematical Biosciences and Engineering, 2023, 20(10): 17625-17645. doi: 10.3934/mbe.2023783 |
[3] | Andreas Wagner, Pirmin Schlicke, Marvin Fritz, Christina Kuttler, J. Tinsley Oden, Christian Schumann, Barbara Wohlmuth . A phase-field model for non-small cell lung cancer under the effects of immunotherapy. Mathematical Biosciences and Engineering, 2023, 20(10): 18670-18694. doi: 10.3934/mbe.2023828 |
[4] | Defne Yilmaz, Mert Tuzer, Mehmet Burcin Unlu . Assessing the therapeutic response of tumors to hypoxia-targeted prodrugs with an in silico approach. Mathematical Biosciences and Engineering, 2022, 19(11): 10941-10962. doi: 10.3934/mbe.2022511 |
[5] | Samantha L Elliott, Emek Kose, Allison L Lewis, Anna E Steinfeld, Elizabeth A Zollinger . Modeling the stem cell hypothesis: Investigating the effects of cancer stem cells and TGF−β on tumor growth. Mathematical Biosciences and Engineering, 2019, 16(6): 7177-7194. doi: 10.3934/mbe.2019360 |
[6] | Lifeng Han, Steffen Eikenberry, Changhan He, Lauren Johnson, Mark C. Preul, Eric J. Kostelich, Yang Kuang . Patient-specific parameter estimates of glioblastoma multiforme growth dynamics from a model with explicit birth and death rates. Mathematical Biosciences and Engineering, 2019, 16(5): 5307-5323. doi: 10.3934/mbe.2019265 |
[7] | Peter Hinow, Philip Gerlee, Lisa J. McCawley, Vito Quaranta, Madalina Ciobanu, Shizhen Wang, Jason M. Graham, Bruce P. Ayati, Jonathan Claridge, Kristin R. Swanson, Mary Loveless, Alexander R. A. Anderson . A spatial model of tumor-host interaction: Application of chemotherapy. Mathematical Biosciences and Engineering, 2009, 6(3): 521-546. doi: 10.3934/mbe.2009.6.521 |
[8] | Hsiu-Chuan Wei . Mathematical modeling of tumor growth: the MCF-7 breast cancer cell line. Mathematical Biosciences and Engineering, 2019, 16(6): 6512-6535. doi: 10.3934/mbe.2019325 |
[9] | Duane C. Harris, Giancarlo Mignucci-Jiménez, Yuan Xu, Steffen E. Eikenberry, C. Chad Quarles, Mark C. Preul, Yang Kuang, Eric J. Kostelich . Tracking glioblastoma progression after initial resection with minimal reaction-diffusion models. Mathematical Biosciences and Engineering, 2022, 19(6): 5446-5481. doi: 10.3934/mbe.2022256 |
[10] | Aftab Ahmed, Javed I. Siddique . The effect of magnetic field on flow induced-deformation in absorbing porous tissues. Mathematical Biosciences and Engineering, 2019, 16(2): 603-618. doi: 10.3934/mbe.2019029 |
Abbreviations: TFIP: Tumor interstitial fluid pressure; DTC: Disseminated tumor cells
There is a remarkable difference between the treatment responses of generalized lymphomas and leukemias on the one hand and solid neoplasms, i.e. cancer, on the other. While the former can be successfully treated with chemotherapy and/or antibody treatment, the latter cannot. This treatment failure in carcinomas has been attributed to the high tumor interstitial fluid pressure (TIFP) observed in most cancers, which is absent in lymphomas and leukemias. TIFP is caused by a fluid imbalance within the tumor mass. In particular, functioning lymphatic vessels are absent within the tumor so that interstitial fluid, normally transported away by the lymphatic vessels, remains in the local area. Two forms of fluid transport within tissues can be distinguished, namely diffusion and convection. Diffusion is the random movement of molecules and particles from a higher concentration region to one of lower concentration which is driven by a concentration gradient. In contrast, convection is the directed flow of particles within the interstitial fluid from the blood-tissue exchange microvessels to the lymphatic drainage system, which is pressure-related. The latter is absent in cancers so transport processes are more or less limited to diffusion [1,2]. For both macromolecules such as antibodies [3,4] and small molecules such as doxorubicin [5] or cisplatin [6], xenograft experiments showed that anti-cancer drugs only penetrate about 100 µm around blood vessels, and therefore target only a minority of cancer cells. As these drugs are transported in the same way as oxygen and nutrients, their access to the tumor cells via the existing blood vessels is accordingly limited. Due to the lack of oxygen and nutrients, a fraction of the oxygenated proliferating cells, those that are more than 100 µm from a blood vessel, switch into a hypoxic and later into a necrotic state.
Due to this low penetration depth, cancers consist mainly of hypoxic and necrotic areas; this leads to treatment resistance because hypoxia enhances chemo- and/or radioresistance of cancer cells [7]. After the removal of oxygenated cells by chemotherapy or radiotherapy, formerly hypoxic cells are resupplied with oxygen and nutrients, leading to an oxygenated state. This process is called reoxygenation, and it also rescues hypoxic cells from becoming necrotic. The process of reoxygenation is utilized during radiotherapy, where small radiation fractions are applied at short intervals, leading to radiosensitization of previously hypoxic tumor areas [8]. Saggar and Tannock investigated chemotherapy-induced reoxygenation using hypoxia markers to quantify the number of cells that are reoxygenated after therapy in MCF-7 and PC-3 xenograft mouse tumor models [9]. They showed that after 24 hours, approximately 90% of the hypoxic cells were already reoxygenated in xenograft primary tumors of both cell lines.
Mathematical models are widely used to describe the increase in tumor volume over time, the spread of metastases, and the effect of different treatment strategies [10,11,12,13,14]. In order to create biologically meaningful translational predictions (e.g., optimal treatment doses), these models have to be calibrated with experimental data, validated with untrained data, and evaluated on predictive performance for known treatments [15]. Mathematical models can also be useful for developing a better understanding of biological mechanisms, which can stimulate new experimental approaches. A variety of mathematical models exist that can describe tumor growth including Gompertz [16,17], exponential [18], von Bertalanffy [19], or the generalized logistic model [20,21]. These models only consider the change in tumor volume over time, not the behavior of individual fractions within the tumor. Ribba et al. published a mathematical model that successfully describes tumor growth and tumor fractions over time based on longitudinal data and histological biomarkers [10].
In order to better understand the limited success of chemotherapy in solid neoplasms, we modeled the effect of limited distribution of chemotherapeutic drugs into the primary tumor by extending this mathematical model [10], adding a pharmacokinetic-pharmacodynamic model [22], and including the reoxygenation of hypoxic cells. In a second step, the effect of chemotherapy on disseminated tumor cells (DTCs) and multicellular metastases was studied. DTCs and small metastases are of particular relevance as they consist only of tissue that is well supplied with nutrients and oxygen and have not built up increased TIFP. Only once metastases have grown to a certain size does TIFP build up, leading to the formation of hypoxic and necrotic tumor fractions. Changes were identified in the overall effectiveness of chemotherapy, which decreased with the progression of the disease. TIFP also has adverse effects on the treatment of metastases and is, therefore, a critical factor that must be considered in developing treatment strategies for malignant tumors.
To investigate the limited convection of chemotherapeutic drugs into the primary tumor, we used experimental data, including tumor size and histological biomarkers, which describe two fractions of the tumor over time: hypoxic and necrotic tissue [10]. In this study 6 × 106 HT29 cells were subcutaneously injected into 15 female athymic nude mice. Tumor size was measured at repeated intervals using a caliper. The percentage of hypoxic and necrotic tissue was determined using immunohistochemistry. The mean values of tumor size (mm) and hypoxic and necrotic fractions (%) for each measured time point were obtained using WebPlotDigitizer [23]. The oxygenated fraction of the tumor was deduced from the hypoxic and necrotic fractions.
Since no metastases were examined in the original study, we used data from an HT29 xenograft mouse model [24], which provided information about the number and size of the metastases at the end of the experiment. In this study 1 × 106 HT29 cells were subcutaneously injected into pore-forming protein and recombination activating gene 2 double knockout (pfp/rag2) mice and in recombination activating gene 2 only knockout (rag2) mice. In pfp/rag2 mice typical metastases (~ 789) consisted of approximately 10–100 tumor cells. In rag2 mice, only disseminated tumor cells were generally found (~ 200). Computer simulation of the metastatic spread proved that metastases stayed dormant for at least 30 days after reaching a size between 10 and 100 cells in the pfp/rag2 group. In contrast, the metastatic cells in the rag2 group entered an initial dormant phase, in which they remained for 30 days before starting to proliferate.
Investigations of human breast (MCF-7) and prostate cancer (PC-3) primary xenograft tumors have shown that chemotherapy induces reoxygenation and repopulation of hypoxic cells [9]. In this study 5 × 106 MCF-7 and 2 × 106 PC-3 cells were subcutaneously injected into female and male athymic nude mice, respectively. The tumor hypoxia marker pimonidazole was given concurrently with doxorubicin. At 24, 48, 72, 96 and 120 hours following initial treatment, a second tumor hypoxia marker EF5 was injected to quantify reoxygenated tissue. The reoxygenation process rescues hypoxic cells from conversion to a necrotic state by resupplying oxygen and nutrients (Figure 1). Approximately 90% of the hypoxic cells were converted to an oxygenated state after 24 hours. After 120 hours, around 100% of formerly hypoxic cells were reoxygenated. The number of reoxygenated cells should depend on the number of eliminated oxygenated cells. For example, if only 20% of the tissue is destroyed during therapy, it is implausible that all hypoxic cells will be converted back into an oxygenated state if the number of cells in the hypoxic tissue is higher than the initial number of cells in the oxygenated tissue. We assume that rescued hypoxic cells are able to proliferate after being converted to an oxygenated state.
An existing tumor growth model was coupled with models of the pharmacokinetics and pharmacodynamics of a cell-cycle non-specific drug such as cisplatin (an alkylating agent), reoxygenation of hypoxic tissue after treatment, and metastasis formation and dormancy behavior (Figure 2).
The mathematical model of tumor growth published by Ribba et al. [10] describes the growth of cancer and its tumor fractions over time. The tumor consists of three main compartments: Oxygenated (P), hypoxic (Q), and necrotic tissue (N). P* represents the total tumor size. The oxygenated tissue follows a generalized logistic equation, where the maximum size is limited by a carrying capacity (K) that describes the maximum tumor size that can be reached with the amount of physical space and nutrients available. Therefore, the carrying capacity depends on the rate of increase in carrying capacity for a specific cell line (b) induced by angiogenic processes. Since we assume that only oxygenated cells are able to proliferate, λP is the growth rate constant for the oxygenated tissue. The parameter s regulates the slowdown of the logistic growth of oxygenated tissues and α reflects the effect of hypoxic stress. Cell transfer from oxygenated tissue (P) to hypoxic tissue (Q) and hypoxic tissue (Q) to necrotic tissue (N) is modeled via the rate constants kPQ and kQN, respectively. The system equations are given by the following:
dPdt=λPP(1−sa)−kPQP,P(t=0)=P0dQdt=kPQP−kQNQ,Q(t=0)=0dNdt=kQNQ,N(t=0)=0dKdt=bP∗,K(t=0)=K0P∗=P+Q+Nands=P∗K | (1) |
We modeled the pharmacokinetics (PK) of a cell-cycle non-specific drug using a two-compartment model. The system is divided into two kinetically different regions: Central (CD) and peripheral (PD) compartment [22]. The central compartment consists of the plasma and tissues where the drug is homogeneously distributed right after the administration. In contrast, drug distribution in the tissue of the peripheral compartment is slower. The intravenous bolus administration is modeled via the addition of the dose to the central drug compartment. The parameters k12 and k21 are rate constants characterizing drug movement between the central and peripheral compartment. ke represents the rate constant for drug elimination (clearance) out of the central compartment, which leads to a drug concentration c(t) that is following an exponential decay function (as illustrated in the "PK/PD" sub-model in Figure 2). The corresponding model equations are given by:
dCDdt=−(k12+ke)∗CD+k21∗PDdPDdt=−k21∗PD+k12∗CD | (2) |
To include the effect (PD) of limited diffusion of chemotherapeutic agents into the primary tumor, the drug only affects cells in the oxygenated layer (P), because drugs cannot diffuse into the hypoxic layer due to the limited penetration depth of 100 µm around blood vessels [5,6]. The parameter k2 represents the drug potency. Treatment was applied on days 10, 20 and 30.
The process of reoxygenation is modeled by the fraction of the hypoxic cells (kQPQ) that is transferred to the oxygenated compartment after treatment, where kQP is the constant transfer rate. This approach is similar to models of the reoxygenation process after chemotherapy or radiotherapy treatment [25,26,27]. Since approximately 90% of hypoxic cells were already reoxygenated after 24 hours in a previous study [9], we assume that the reoxygenation process begins immediately after chemotherapy. Cell transfer from the hypoxic to the oxygenated compartment remains active until the number of cells in the oxygenated layer reaches the number of cells before treatment. This decision is based on experiments showing that chemotherapy does not lead to better blood supply through angiogenesis, but to a reduction in blood vessel density, which is still detectable several days after the therapy ended [28]. Therefore, the number of cells in the oxygenated layer during the reoxygenation process cannot be higher than the number before treatment.
The primary tumor spreads single malignant cells at a colonization rate μ(P∗), which depends on the per day probability m that any individual cell will spread and establish a distant metastasis, the number of cells in the primary tumor P*, and the fractal dimension of blood vessels δ [29]. A fractal dimension of 2 implies that the tumor is superficially supplied with blood vessels, while a value of 3 means a completely supplied tumor.
μ(P∗)=m∗P∗δ/3 | (3) |
A cumulative trapezoidal numerical integration of the colonization rate was performed to derive the time of development of each metastasis from the integrated values. Each time the integrated values reach the next integer value, a new metastasis is created.
The growth of metastases starts from a single cell and follows the growth pattern of the primary tumor. Metastases also consist of oxygenated, hypoxic, and necrotic tissue. Since nutrients are only transported up to 100 µm away from the blood vessels, we assume that the formation of a hypoxic and necrotic layer only begins when the metastasis reaches a threshold diameter of 200 µm with an assumed superficial blood supply. Therefore, the initial values of the rate constants kPQ and kQN were set to 0 until the size of the metastasis reached this threshold.
Malignant cells emitted from the primary tumor can stay dormant for a certain length of time or enter a late dormancy state after they reach a specific size [24]. In this article we focus on the case of metastases entering a late dormancy phase of 30 days. With the chosen treatment strategy, it makes no difference whether 1 or 100 cells are affected since chemotherapy is parameterized in such a way that all treatable cells will be destroyed. Furthermore, all metastases are in a dormant phase at the selected treatment times (days 10, 20 and 30). The growth parameter λP and transfer rate constants (kPQ, kQN) are set to a value of 0 during the time span of dormancy (30 days). Based on previous experiments on metastasis formation, the number of cells at which metastases enter a late dormant state is calculated randomly for each metastasis based on a uniform distribution between 10 and 100 cells [24].
The full mathematical model including tumor growth, chemotherapy treatment, reoxygenation of hypoxic cells, and dormancy of metastases is defined by:
dPdt=Growth⏞λPP(1−sa)−Treatmenteffect⏞k2c(t)P−TransferP→Q⏞kPQP+Reoxygenatedcells⏞kQPQ,P(t=0)=P0dQdt=TransferredcellsP→Q⏞kPQP−TransferQ→N⏞kQNQ−Reoxygenatedcells⏞kQPQ,Q(t=0)=0dNdt=TransferredcellsQ→N⏞kQNQ,N(t=0)=0dKdt=bP∗,K(t=0)=K0P∗=P+Q+N,s=P∗K,μ(P∗)=m∗P∗δ/3 | (4) |
Experimental data was used to determine model parameters and rate constants in order to calibrate the model.
The growth of tumor size in experiments is usually measured as diameter in mm. For computer models the number of cells is required. The diameter of the tumor (mm) was converted to volume (mm3) and multiplied by 106 using the well-established conversion rule (1 mm3 ~ 1 × 106 cells) to determine the number of cells [30]. The number of hypoxic and necrotic cells was calculated from the percentage of tumor fractions at each measuring time point. Least-squares minimization was performed using fmincon from the MATLAB Optimization Toolbox (MATLAB R2019b, The MathWorks Inc., Natick, USA) to obtain all model parameters based on experimental tumor data. The number of initial tumor cells in the oxygenated compartment (P) was set to 6 × 105 cells during the parameter estimation process because our previous study showed that only 10% of the subcutaneously injected tumor cells survive the first 24 hours [31]. Furthermore, fixation of the initial tumor cell number to a well-known value reduces the number of degrees of freedom and improves the parameter estimation results.
Due to the absence of pharmacokinetic and pharmacodynamic data for the experimental data analyzed here, we used determined parameters from Simeoni et al. [32], which describe the drug concentration of the chemotherapy agent paclitaxel over time. We adjusted the drug potency parameter k2 until all cells in the oxygenated fraction of the tumor and metastases were eliminated (in the absence of reoxygenation) to mimic the most effective treatment. Since the cells in the oxygenated layer will be entirely destroyed, the limiting effect of the interstitial fluid pressure on the efficacy of the chemotherapeutic drug can be studied most clearly.
Previous experimental data from human MCF-7 and PC-3 primary xenograft tumors in mice were used to determine the reoxygenation rate kQP of hypoxic cells [9]. These data provide the percentage of reoxygenated cells 24, 48, 72, 96 and 120 hours after the application of chemotherapy. They show that 90 and 100% of hypoxic cells were reoxygenated by 24 and 120 hours, respectively. These percentages represent the mean values from four mice. Based on these percentages, growth data for the reoxygenation process after the application of chemotherapy was generated. For example, 1 × 105 cells were killed by chemotherapy in the oxygenated layer. After 24 hours, 9 × 104 cells (90%) are already reoxygenated. After 120 hours, the oxygenated layer has regained its pre-treatment cell count. The generated data were used to estimate the reoxygenation rate constant kQP using lsqnonlin (trust-region reflective algorithm). The number of cells that are reoxygenated can change depending on the drug concentration in the system. Since we assume that reoxygenation starts immediately after the therapy, reoxygenated cells can also be killed. The reoxygenation process remains active until the number of cells has reached the cell count in the oxygenated layer before treatment.
We assumed a superficial blood supply (fractal dimension α = 2) for the primary tumor due to the fast growth behavior that is observed in typical xenograft experiments. Based on experimental data [24], we adjusted the per day probability m for each cell to spread and establish a distant metastasis until the metastasis count reached a value of 789.
The mathematical model was implemented in MATLAB SimBiology®-Command line (MATLAB R2019b Update 4, 9.7.0.1296695, The MathWorks Inc., Natick, USA). We used the SUNDIALS solver (Suite of Nonlinear and Differential/Algebraic Equation Solvers) to solve the model's ordinary differential equations. The simulation time was set to 50 days. The first step was the parametrization of the primary tumor, the spread of metastases, and reoxygenation based on the model calibration results. After this simulation, the metastasis creation time was calculated based on the colonization rate μ(P∗). The last step was the simulation of each metastasis. This procedure allowed us to examine the course of the individual fractions of each metastasis for further evaluations.
The tumor growth model was calibrated with experimental data, including longitudinal tumor data and histological biomarkers (hypoxic and necrotic fractions). The initial number of surviving engrafted tumor cells after subcutaneous injection (P) was set to 6 × 105 cells. The predictions were in good agreement with the observed values from experimental data (tumor cells R2 = 0.95, hypoxic cells R2 = 0.82, necrotic cells R2 = 0.97). The parameterized model was able to describe the size of the tumor and the dynamics of its hypoxic and necrotic fractions based on the number of cells (Figure 3). The estimated parameters are displayed in Table 1.
Parameter | Description | Value |
P0 | Initial tumor size | 6.00e5 cells* |
K0 | Initial carrying capacity | 1.1193e9 cells |
λP | Growth rate for the oxygenated tissue | 1.8420 day-1 |
kPQ | Transfer rate from oxygenated to hypoxic tissue compartment | 0.3595 day-1 |
kQN | Transfer rate from hypoxic to necrotic tissue compartment | 0.1141 day-1 |
kQP | Transfer rate from hypoxic to oxygenated tissue compartment | 2.2216 day-1 |
b | Rate of increase of carrying capacity | 0.5811 day-1 |
m | Per day probability to spread and establish a distant metastasis | 2.732e-8 (cell day)-1 |
δ | Fractal dimension of blood vessels | 2 |
α | Effect of the hypoxic stress on the growth | 0.1* [10] |
dose | Drug dose | 20 arbitrary unit* |
k12 | Transfer rate from central to peripheral compartment (PK/PD) | 0.0115 day-1 [32] |
k21 | Transfer rate from peripheral to central compartment (PK/PD) | 0.0616 day-1 [32] |
ke | Rate constant for drug elimination (clearance) | 3 day-1 * |
k2 | Drug potency (effectivity) | 2.1 * |
*Known parameters were set to a fixed value during the parameter estimation procedure, which increased the accuracy of the estimates and reduced the number of degrees of freedom. |
Some time after subcutaneous injection of HT29 tumor cells into mice, the engrafted surviving cells form a local tumor nodule and develop hypoxic and necrotic fractions depending on the blood vessel geometry. Experimental tumor data, including histological biomarkers of hypoxic and necrotic tissue, were used to estimate model parameters and rate constants. Figure 4 shows the simulation output of untreated tumor growth including the percentage of tumor fractions over time. According to the model output, the percentage of oxygenated cells decreased significantly over time compared to the tumor size. This finding implies that the tumor consists mainly of hypoxic and necrotic tissue, which is in good agreement with the experimental data [10].
Chemotherapeutic drugs only penetrate about 100 µm around blood vessels, and therefore only target cancer cells within this range [5,6]. The percentage of oxygenated cells in a tumor after chemotherapy could be understood as an indicator of the success of the therapy since hypoxic (and necrotic) cells are chemotherapy resistant [7]. One reason for this resistance is their increased distance from blood vessels, so that no chemotherapeutic drugs can reach these cells [5,6]. Furthermore, this distance also causes nutrient and oxygen deficiency, which slows down the cell cycle and, as a result, the proliferation rate [33]. Therefore, cell-cycle specific drugs are less effective in these cells because they are only active in a particular phase of the cell cycle. We investigated the effectivity of cell-cycle non-specific chemotherapy, which can treat cells in all phases of the cell cycle. Since cell-cycle non-specific drugs destroy more cells than cell-cycle specific drugs, we can focus on the limited distribution of the chemotherapeutic drug.
The drug potency parameter k2 for cell-cycle non-specific chemotherapy was manually adjusted until the number of cells in the oxygenated layer was smaller than one cell layer after treatment in the absence of reoxygenation. A value of k2 = 2.1 was determined for all three treatment time points (days 10, 20 and 30). The number of cells in the oxygenated layer is affected by the occurrence of reoxygenation of hypoxic cells, which is assumed to start immediately after cells are destroyed in the oxygenated layer. This approach is based on our assumption that more cells are destroyed if a sufficient concentration of the chemotherapeutic drug is still present, depending on the PK/PD model's parameterization. Figure 5 shows the results of model simulations of chemotherapy treatment at three different treatment times (days 10, 20 and 30, panels A–F) as well as the percentage distribution of the tumor fractions before and after treatment (panels G–I). Since the formation of the necrotic portion of the tumor is not reversible and treatment of these cells has no effect, the oxygenated and hypoxic fractions represent the potentially treatable part of the tumor. Over time, the treatable part of the tumor became smaller and smaller, and hence a more substantial portion of the tumor could be destroyed with early treatment. However, the fact that penetration of the drug is limited to 100 µm around blood vessels prevents treatment of the hypoxic fraction, even for small tumors. Panels A–C show the course of tumor growth for three different treatment times (days 10, 20 and 30). As shown in panel D (blue dotted lines), the oxygenated cells were killed in the first days after the application of chemotherapy. The decreasing concentration of the drug, combined with reoxygenation of the hypoxic cells and the proliferative behavior of the oxygenated cells, led to recovery of the oxygenated tissue. The hypoxic layer also decreased in size due to the reoxygenation process, as hypoxic cells switched into an oxygenated state (panel E). Since reoxygenation prevented a proportion of hypoxic cells from entering the necrotic state, the growth of the necrotic layer slowed down (panel F). The values for the oxygenated, hypoxic, and necrotic fractions were determined when the drug concentration (central drug) reached a value below 1% of its peak concentration (day 2 post-treatment) in order to compare the tumor fraction distribution before and after therapy. This drug concentration is considered to have a negligible therapeutic effect [34,35]. If treatment commenced on day 10, the proportion of potentially targetable tumor cells was higher than if it starts on day 20 or 30 (panels G–I). The percentage of necrotic tissue before and after treatment provides information about the therapeutic efficacy. The necrotic portion did not grow abruptly after treatment but did represent a higher percentage of the tumor volume since the oxygenated tissue had been killed and a fraction of the hypoxic cells had been reoxygenated. The difference between the necrotic tissue fraction before and after therapy was 49% (G), 47% (H) and 37% (I) on treatment days 10, 20 and 30, respectively. These results imply that the timing of chemotherapy treatment is critical in the presence of interstitial fluid pressure, which leads to the formation of hypoxic and necrotic tumor fractions.
The application of chemotherapy can possibly disrupt the blood vessel geometry [28]. As an effect of the altered geometry, cell transfer from the hypoxic to the oxygenated compartment after chemotherapy might lead to a reduced number of cells after treatment only due to the smaller amount of cells that are in contact with blood vessels. To study this effect, we performed the same simulation with a modified reoxygenation behavior (Figure S1). In this scenario, cells were transferred from the hypoxic to the oxygenated compartment until the number of cells in the oxygenated compartment reached 80% of the cell count before treatment. This percentage represents a reasonable assumption in our view since no experimental data is available. Similar results were obtained in comparison to the simulation with a reoxygenation behavior in which the number of cells is completely restored during the reoxygenation process indicating that the percentage of the oxygenated fraction is of lesser importance.
Inspired by the simulation results for the effects of chemotherapy on the size of the primary tumor, we applied the same procedure to understand its impact on the spread of malignant cells and metastases. The colonization rate µ(P*) was parametrized in such a way that an untreated primary tumor spread and established 789 distant metastases, which was the observed mean number from previous HT29 xenograft experiments [24]. We assumed a superficial blood supply (fractal dimension of 2) due to the rapid growth of the primary tumor. In some of our previous research, the size of metastases at the end of the experiment ranged from 10 to 100 cells, which implied a late dormancy after metastases reached a specific size [24]. Two main scenarios were considered, namely metastases with and without dormancy behavior. Chemotherapy was applied on day 30 in both scenarios with a simulation time of 50 days. In addition, effects of the resection of the primary tumor two days after chemotherapy treatment commenced were simulated for both scenarios.
Figure 6 shows different simulation scenarios to investigate the influence of chemotherapy on metastasis formation. The untreated primary tumor established 789 distant metastases. In contrast, the treated primary tumor, which received chemotherapy on day 30, established 683 distant metastases or disseminated tumor cells due to a reduced spreading behavior because the reduction in the size of the primary tumor P∗ affected the colonization rate μ(P∗). Chemotherapy had no substantial effect on metastases that had already built up interstitial fluid pressure. Only 56 metastases that were smaller than 200 µm in size at the time of commencement of chemotherapy were destroyed (blue bar). In the scenario where metastases switched into a late dormancy state (Figure 7B, C), chemotherapy destroyed 266 metastases. The remaining 417 metastases were disseminated after application of chemotherapy and could not be treated. With an additional resection two days after chemotherapy, 761 distant metastases or disseminated tumor cells were eliminated.
In addition, we studied blood vessel disruption after chemotherapy to analyze its influence on spreading behavior. As in the utilized model of vascular tumor growth [10], the disruption of blood vessels was realized with the formula dKdt=bP∗−ξK, where ξ was set to an arbitrary value of 0.1 during treatment. Since no data about the alteration of the blood vessel geometry is available for the used experiment, the changed fractal dimension of blood vessels after the chemotherapy was estimated from our previous studies [28]. To obtain a biologically realistic value we adjusted the fractal dimension after chemotherapy to the mean value of 1.58. Supplementary Figure S2 represent the same simulation scenarios to investigate the influence of chemotherapy on metastasis formation. In general, the disruption of blood vessels has a positive effect on the spreading behavior of malignant cells, since the primary tumor spread fewer malignant cells. Although surgical removal of the primary tumor stops the tumor's spread, the resistance of the metastases due to the increased interstitial fluid pressure is the most prominent obstacle in the treatment of cancer.
As with the primary tumor, the timing of the initiation of chemotherapy plays an important role in the treatment of metastases, because in small metastases interstitial fluid pressure has not been built up. Interstitial fluid pressure protects metastases from chemotherapy if they are not in a dormant state or have grown larger than 200 µm in diameter, leading to the development of hypoxic and necrotic fractions. Furthermore, the results show that one cycle of chemotherapy is not sufficient to kill all metastases if the primary tumor is not removed by surgery.
Chemotherapy profiles from real published experiments were used [32]. To understand the limitations of the present approach, we generated 25 synthetic parameter sets to simulate different treatment effects on a primary tumor that received chemotherapy on day 30, as previous results (Figure 5) showed that this treatment time point was the most ineffective of the whole series of time points used. The effects on the number and size of distant metastases in the absence and presence of interstitial fluid pressure were also investigated. We performed a local sensitivity analysis (SimBiology® Model Analyzer) of the model to investigate which PK/PD parameters had a significant influence on the growth behavior of the primary tumor or metastases (P*, P, Q and N). Since the anti-cancer drug influenced the size of the primary tumor and the number of metastases, the tumor growth response was simulated for each of the synthetic parameter sets. The local sensitivity analysis of the combined model showed that drug clearance ke (µ = 3.0) and drug potency k2 (µ = 2.1) are the two most sensitive parameters that affect tumor growth behavior. This is especially true for the oxygenated fraction P since the treatment is only effective in this tissue which is limited due to the low penetration depth when diffusion takes place, but convection is absent. The full sensitivity analysis for the combined model is displayed in the supplementary material Figure S3 which increases the understanding of the relationships between input and output parameters in our model for further investigation. We drew parameter values from a joint probability distribution with a standard deviation of θ = 0.20 for both parameters to cover a wide parameter range. To select a subset of samples, random sampling with a rank correlation matrix, where the matrix was an identity matrix, was performed. Simulations were then performed for each parameter set.
Figure 7 shows the distribution of each parameter and simulation outputs for the primary tumor based on the generated synthetic samples. The influence of the different therapies led to an overall reduction in tumor size (P*; range −22 to +8%) at the end of the experiment (simulation time 50 days, treatment on day 30). Compared with the drug potency k2, drug clearance ke had a considerably greater influence, as reduced clearance meant the drug was present within the system and affecting tumor cells for a prolonged period of time. Furthermore, depending on the length of time the drug was present in the system, the oxygenated tissue recovered faster or slower (Figure 7F), which influenced the overall reduction in tumor size. Drug potency k2 can also have a substantial impact but this is limited to the oxygenated compartment due to the low diffusion depth. The various tumor size reductions led to different spreading behaviors as well as different size distributions of those metastases that did not switch into a dormant phase. No differences were found in metastases that switched into a late dormant state after reaching a size of 10 to 100 cells. This result indicates that all metastases present at the time of therapy are killed in the absence of interstitial fluid pressure.
The mathematical tumor growth model presented here and calibrated with longitudinal tumor data and histological biomarkers successfully describes the development of hypoxic and necrotic fractions reported by Ribba et al. [10]. Since no metastases were investigated in the original experiment, we used data from other HT29 xenograft experiments to calibrate the model for the primary tumor to spread and establish distant metastasis [24]. Previous research showed that chemotherapy modifies the geometry and density of the blood vessels [28]. This change was still detectable 15 days post-treatment compared to the control group, and led to a reduction in the number of malignant cells that were able to spread to distant sites. Even if the correct number of metastases is not known for the underlying experimental data, chemotherapy will have a similar effect on the number of metastases because the increased interstitial fluid pressure limits the distribution of the drug. The simulation results clearly showed the impact of interstitial fluid pressure inside the tumor, which results in a barrier to oxygen, nutrients, and drugs.
In cancers, oxygen and nutrients penetrate only about 100 µm around the blood vessels in the presence of increased interstitial fluid pressure. This increased interstitial fluid pressure results in the formation of hypoxic and necrotic fractions once the tumor has reached a specific size. In contrast to large primary tumors, micrometastases are too small for interstitial fluid pressure to build up and the blood supply can reach all cells. This is the equivalent of a fractal dimension of 3 for the blood vessels. Micrometastases consist only of oxygenated cells until their size reaches a certain threshold when the fluid pressure starts to build up. The threshold value for the formation of hypoxic and necrotic layers may vary with different blood vessel geometries. Further investigations of the dynamics of hypoxic and necrotic tissues are important in order to better understand this process. This variation in the threshold may have a negligible effect when metastases grow as rapidly as the primary tumor, leading to a superficial blood supply after several days. Furthermore, metastases may switch into a late dormant state for at least 30 days after reaching a size between 10 and 100 cells [24]. However, this threshold may be important for simulation scenarios that extend far beyond a period of about 50 days.
In a previous HT29 xenograft study, the metastases present at the end of the experiment consisted of 10–100 tumor cells in pfp/rag2 mice and only disseminated tumor cells were generally found in rag2 mice. This difference in the number of metastases was caused by a late dormancy phase of at least 30 days when cells entered a reversible cell cycle arrest in the pfp/rag2 group. The emitted cells in the rag2 group underwent an initial dormancy phase before they were able to proliferate. Cell-cycle specific chemotherapy agents such as doxorubicin that target actively dividing cells cannot kill cells that are in a dormant state because they are not cycling [36]. In contrast, cell-cycle non-specific drugs kill cells in any phase of the cell cycle. In order to be able to affect both types of cell simultaneously, up to five agents could be combined, including cell-cycle specific and cell-cycle non-specific chemotherapy agents (polychemotherapy) [37]. The mathematical model must be modified to model cycling and resting cells in the oxygenated layer to perform simulations of cell-cycle specific treatments [38]. Since cell-cycle non-specific chemotherapeutic agents such as cisplatin were used in our simulations, the simulated treatment was able to kill dormant cells as well. Therefore, simulation results obtaining using cell-cycle specific chemotherapy may show reduced treatment efficacy compared with cell-cycle non-specific chemotherapy.
In this article, a mathematical model is presented to model tumor growth and the dynamics of oxygenated, hypoxic and necrotic tumor fractions. This model was combined with a pharmacokinetic-pharmacodynamic model that describes the behavior of a chemotherapeutic drug over time. We used this combined model as a theoretical tool to investigate the effects of limited distribution of chemotherapy into the primary tumor and metastases as a result of interstitial fluid pressure, which means chemotherapy drugs can only penetrate about 100 µm around blood vessels. The number of cells in the oxygenated tissue increased slowly over time. However, the size of the oxygenated tissue fraction as a percentage of the total tumor size decreased significantly over time, thereby reducing the effect of chemotherapy on the entire tumor. This is due to the limited distribution of the drug, which can only reach oxygenated cells as oxygen and drug diffuse together to the cancer cells. Therefore, early treatment is recommended, especially before surgery, to reduce the base number of oxygenated and hypoxic tumor cells and the vessel density to change the spreading behavior of malignant cells. Similar results were obtained in the treatment of metastases, where interstitial fluid pressure also limits the effect of chemotherapy. The timing of the initiation of chemotherapy is an important factor in the success of the treatment, especially when interstitial fluid pressure is increased. Priority should be given to overcoming the convection barrier to achieve a higher penetration depth for chemotherapeutic drugs [2]. This approach would increase the chances of a cure for solid tumors as a more substantial proportion of the tumor cells would be reached by chemotherapy.
Bertin Hoffmann gratefully acknowledges funding by the European Social Fund through a scholarship from the State Graduate Funding Program (Landesgraduiertenförderung) of the University of Rostock, Germany. The funder did not have any role in the design and conduct of the study, the analysis and interpretation of the data, the decision to publish, or the preparation of the manuscript.
We thank Jenny Cattermole for critical reading and copyediting.
All authors declare no conflicts of interest in this paper.
[1] | T. R. Samatov, V. V. Galatenko, A. Block, M. Y. Shkurnikov, A. G. Tonevitsky, U. Schumacher, Novel biomarkers in cancer: The whole is greater than the sum of its parts, Semin. Cancer Biol., 45 (2017), 50-57. |
[2] | L. C. Böckelmann, U. Schumacher, Targeting tumor interstitial fluid pressure: Will it yield novel successful therapies for solid tumors?, Expert Opin. Ther. Targets, 23 (2019), 1-10. |
[3] | M. Heine, B. Freund, P. Nielsen, C. Jung, R. Reimer, H. Hohenberg, et al., High interstitial fluid pressure is associated with low tumour penetration of diagnostic monoclonal antibodies applied for molecular imaging purposes, PLoS One, 7 (2012), e36258. |
[4] | M. Heine, P. Nollau, C. Masslo, P. Nielsen, B. Freund, O.T. Bruns, et al., Investigations on the usefulness of CEACAMs as potential imaging targets for molecular imaging purposes, PLoS One, 6 (2011), e28030. |
[5] | A. J. Primeau, A. Rendon, D. Hedley, L. Lilge, I. F. Tannock, The distribution of the anticancer drug Doxorubicin in relation to blood vessels in solid tumors, Clin. Cancer Res., 11 (2005), 8782-8788. |
[6] | L. Böckelmann, C. Starzonek, A. C. Niehoff, U. Karst, J. Thomale, H. Schlüter, et al., Detection of doxorubicin, cisplatin and therapeutic antibodies in formalin-fixed paraffin-embedded human cancer cells, Histochem. Cell Biol., 153 (2020), 367-377. |
[7] | J. M. Brown, W. R. Wilson, Exploiting tumour hypoxia in cancer treatment, Nat. Rev. Cancer, 4 (2004), 437-447. |
[8] | F. Pajonk, E. Vlashi, W. H. McBride, Radiation resistance of cancer stem cells: The 4 R's of radiobiology revisited, Stem Cells, 28 (2010), 639-648. |
[9] | J. K. Saggar, I. F. Tannock, Chemotherapy rescues hypoxic tumor cells and induces their reoxygenation and repopulation-an effect that is inhibited by the hypoxia-activated prodrug TH-302, Clin. Cancer Res., 21 (2015), 2107-2114. |
[10] | B. Ribba, E. Watkin, M. Tod, P. Girard, E. Grenier, B. You, et al., A model of vascular tumour growth in mice combining longitudinal tumour size data with histological biomarkers, Eur. J. Cancer, 47 (2011), 479-490. |
[11] | N. D. Evans, R. J. Dimelow, J. W. T. Yates, Modelling of tumour growth and cytotoxic effect of docetaxel in xenografts, Comput. Methods Programs Biomed., 114 (2014), e3-e13. |
[12] | S. Benzekry, A. Gandolfi, P. Hahnfeldt, Global dormancy of metastases due to systemic inhibition of angiogenesis, PLoS One, 9 (2014), e84249. |
[13] | H. Enderling, M. A. J. Chaplain, A. R. A. Anderson, J. S. Vaidya, A mathematical model of breast cancer development, local treatment and recurrence, J. Theor. Biol., 246 (2007), 245-259. |
[14] | A. Bethge, U. Schumacher, G. Wedemann, Simulation of metastatic progression using a computer model including chemotherapy and radiation therapy, J. Biomed. Inform., 57 (2015), 74-87. |
[15] | R. Brady, H. Enderling, Mathematical models of cancer: When to predict novel therapies, and when not to, Bull. Math. Biol., 81 (2019), 3722-3731. |
[16] | A. Akanuma, Parameter analysis of Gompertzian function growth model in clinical tumors, Eur. J. Cancer, 14 (1978), 681-688. |
[17] | A. K. Laird, Dynamics of tumour growth, Br. J. Cancer, 18 (1964), 490-502. |
[18] | V. P. Collins, R. K. Loeffler, H. Tivey, Observations on growth rates of human tumors, AJR Am. J. Roentgenol., 76 (1956), 988-1000. |
[19] | L. von Bertalanffy, Quantitative laws in metabolism and growth, Q. Rev. Biophys., 32 (1957), 217-231. |
[20] | S. Michelson, A. S. Glicksman, J. T. Leith, Growth in solid heterogeneous human colon adenocarcinomas: Comparison of simple logistical models, Cell Tissue Kinet, 20 (1987), 343-355. |
[21] | J. A. Spratt, D. von Fournier, J. S. Spratt, E. E. Weber, Decelerating growth and human breast cancer, Cancer, 71 (1993), 2013-2019. |
[22] | R. H. Reuning, R. A. Sams, R. E. Notari, Role of pharmacokinetics in drug dosage adjustment. I. pharmacologic effect kinetics and apparent volume of distribution of digoxin, J. Clin. Pharmacol .New Drugs, 13 (1973), 127-141. |
[23] | A. Rohatgi, WebPlotDigitizer-Extract Data from Plots, Images, and Maps. Available from: https://automeris.io/WebPlotDigitizer/. |
[24] | T. Brodbeck, N. Nehmann, A. Bethge, G. Wedemann, U. Schumacher, Perforin-dependent direct cytotoxicity in natural killer cells induces considerable knockdown of spontaneous lung metastases and computer modelling-proven tumor cell dormancy in a HT29 human colon cancer xenograft mouse model, Mol. Cancer, 13 (2014), 244. |
[25] | A. V. Chvetsov, L. Dong, J. R. Palta, R. J. Amdur, Tumor-volume simulation during radiotherapy for head-and-neck cancer using a four-level cell population model, Int. J. Radiat. Oncol. Biol. Phys., 75 (2009), 595-602. |
[26] | R. Ruggieri, A. E. Nahum, The impact of hypofractionation on simultaneous dose-boosting to hypoxic tumor subvolumes, Med. Phys., 33 (2006), 4044-4055. |
[27] | K. Nakamura, A. Brahme, Evaluation of fractionation regimens in stereotactic radiotherapy using a mathematical model of repopulation and reoxygenation, Radiat. Med., 17 (1999), 219-225. |
[28] | T. Frenzel, B. Hoffmann, R. Schmitz, A. Bethge, U. Schumacher, G. Wedemann, Radiotherapy and chemotherapy change vessel tree geometry and metastatic spread in a small cell lung cancer xenograft mouse tumor model, PLoS One, 12 (2017), e0187144. |
[29] | K. Iwata, K. Kawasaki, N. Shigesada, A dynamical model for the growth and size distribution of multiple metastatic tumors, J. Theor. Biol., 203 (2000), 177-186. |
[30] | J. S. Spratt, J. S. Meyer, J. A. Spratt, Rates of growth of human solid neoplasms: Part I, J. Surg. Oncol., 60 (1995), 137-146. |
[31] | B. Hoffmann, T. Lange, V. Labitzky, K. Riecken, A. Wree, U. Schumacher, et al., The initial engraftment of tumor cells is critical for the future growth pattern: a mathematical study based on simulations and animal experiments, BMC Cancer, 20 (2020), 524. |
[32] | M. Simeoni, P. Magni, C. Cammia, G. De Nicolao, V. Croci, E. Pesenti, et al., Predictive pharmacokinetic-pharmacodynamic modeling of tumor growth kinetics in xenograft models after administration of anticancer agents, Cancer Res., 64 (2004), 1094-1101. |
[33] | I. F. Tannock, The relation between cell proliferation and the vascular system in a transplanted mouse mammary tumour., Br. J. Cancer, 22 (1968), 258-273. |
[34] | R. Seifert, Basic Knowledge of Pharmacology, Springer International Publishing, 2019. |
[35] | K. D. Tripathi, Essentials of Medical Pharmacology, Jaypee Brothers Medical Publishers, 2013. |
[36] | G. N. Naumov, J. L. Townson, I. C. MacDonald, S. M. Wilson, V. H. C. Bramwell, A. C. Groom, et al., Ineffectiveness of doxorubicin treatment on solitary dormant mammary carcinoma cells or late-developing metastases, Breast Cancer Res. Treat., 82 (2003), 199-206. |
[37] | W. Sun, L. M. Schuchter, Metastatic melanoma, Curr. Treat Options Oncol., 2 (2001), 193-202. |
[38] | J. C. Panetta, J. Adam, A mathematical model of cycle-specific chemotherapy, Math. Comput. Model, 22 (1995), 67-82. |
![]() |
![]() |
1. | Hooman Salavati, Charlotte Debbaut, Pim Pullens, Wim Ceelen, Interstitial fluid pressure as an emerging biomarker in solid tumors, 2022, 1877, 0304419X, 188792, 10.1016/j.bbcan.2022.188792 | |
2. | Alexander V. Zhdanov, Rajannya Sen, Ciaran Devoy, Liang Li, Mark Tangney, Dmitri B. Papkovsky, Analysis of tumour oxygenation in model animals on a phosphorescence lifetime based macro-imager, 2023, 13, 2045-2322, 10.1038/s41598-023-46224-7 | |
3. | Zhaoxuan Huo, Jicai Huang, Yang Kuang, Shigui Ruan, Yuyue Zhang, Oscillations in a tumor–immune system interaction model with immune response delay, 2024, 1477-8599, 10.1093/imammb/dqae016 | |
4. | Gabriela Da Silva André, Céline Labouesse, Mechanobiology of 3D cell confinement and extracellular crowding, 2024, 1867-2450, 10.1007/s12551-024-01244-z | |
5. | Shrey Shah, Gerard G. M. D’Souza, Modeling Tumor Microenvironment Complexity In Vitro: Spheroids as Physiologically Relevant Tumor Models and Strategies for Their Analysis, 2025, 14, 2073-4409, 732, 10.3390/cells14100732 |
Parameter | Description | Value |
P0 | Initial tumor size | 6.00e5 cells* |
K0 | Initial carrying capacity | 1.1193e9 cells |
λP | Growth rate for the oxygenated tissue | 1.8420 day-1 |
kPQ | Transfer rate from oxygenated to hypoxic tissue compartment | 0.3595 day-1 |
kQN | Transfer rate from hypoxic to necrotic tissue compartment | 0.1141 day-1 |
kQP | Transfer rate from hypoxic to oxygenated tissue compartment | 2.2216 day-1 |
b | Rate of increase of carrying capacity | 0.5811 day-1 |
m | Per day probability to spread and establish a distant metastasis | 2.732e-8 (cell day)-1 |
δ | Fractal dimension of blood vessels | 2 |
α | Effect of the hypoxic stress on the growth | 0.1* [10] |
dose | Drug dose | 20 arbitrary unit* |
k12 | Transfer rate from central to peripheral compartment (PK/PD) | 0.0115 day-1 [32] |
k21 | Transfer rate from peripheral to central compartment (PK/PD) | 0.0616 day-1 [32] |
ke | Rate constant for drug elimination (clearance) | 3 day-1 * |
k2 | Drug potency (effectivity) | 2.1 * |
*Known parameters were set to a fixed value during the parameter estimation procedure, which increased the accuracy of the estimates and reduced the number of degrees of freedom. |
Parameter | Description | Value |
P0 | Initial tumor size | 6.00e5 cells* |
K0 | Initial carrying capacity | 1.1193e9 cells |
λP | Growth rate for the oxygenated tissue | 1.8420 day-1 |
kPQ | Transfer rate from oxygenated to hypoxic tissue compartment | 0.3595 day-1 |
kQN | Transfer rate from hypoxic to necrotic tissue compartment | 0.1141 day-1 |
kQP | Transfer rate from hypoxic to oxygenated tissue compartment | 2.2216 day-1 |
b | Rate of increase of carrying capacity | 0.5811 day-1 |
m | Per day probability to spread and establish a distant metastasis | 2.732e-8 (cell day)-1 |
δ | Fractal dimension of blood vessels | 2 |
α | Effect of the hypoxic stress on the growth | 0.1* [10] |
dose | Drug dose | 20 arbitrary unit* |
k12 | Transfer rate from central to peripheral compartment (PK/PD) | 0.0115 day-1 [32] |
k21 | Transfer rate from peripheral to central compartment (PK/PD) | 0.0616 day-1 [32] |
ke | Rate constant for drug elimination (clearance) | 3 day-1 * |
k2 | Drug potency (effectivity) | 2.1 * |
*Known parameters were set to a fixed value during the parameter estimation procedure, which increased the accuracy of the estimates and reduced the number of degrees of freedom. |