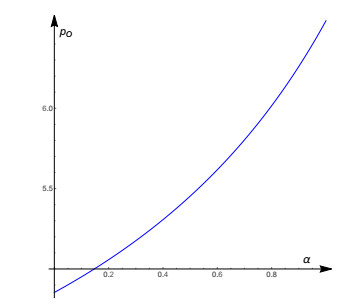
Artificial intelligence (AI) and generative AI (GenAI) have sparked confusion and concern regarding their impact on education. Beyond the assessment integrity risks that currently draw the most attention, technologies such as ChatGPT, Copilot, and Gemini have also been identified as tools that can support learning. Project work, especially when there is no single correct solution, provides a great opportunity for integration, fostering technology knowledge and higher learning standards. However, no AI-integration framework for project-based work is available, resulting in a limited understanding of how AI integration can occur or be maximized. To address this, a collaborative effort of 16 educators from 9 Australian universities has led to the development of a generic AI implementation framework, built upon the CDIO approach. With a focus on engineering education, this framework can be adapted to other project-based learning contexts, where educators can pick and choose the relevant implementation items as needed. This framework is called the Project-work Artificial Intelligence Integration Framework (PAIIF), and its development and structure are outlined here. Initial implementations have shown the effectiveness of promoting reflection and guidance on where and how AI integration can occur.
Citation: Sasha Nikolic, Zach Quince, Anna Lidfors Lindqvist, Peter Neal, Sarah Grundy, May Lim, Faham Tahmasebinia, Shannon Rios, Josh Burridge, Kathy Petkoff, Ashfaque Ahmed Chowdhury, Wendy S.L. Lee, Rita Prestigiacomo, Hamish Fernando, Peter Lok, Mark Symes. Project-work Artificial Intelligence Integration Framework (PAIIF): Developing a CDIO-based framework for educational integration[J]. STEM Education, 2025, 5(2): 310-332. doi: 10.3934/steme.2025016
[1] | Cahya Sutowo, Galih Senopati, Andika W Pramono, Sugeng Supriadi, Bambang Suharno . Microstructures, mechanical properties, and corrosion behavior of novel multi-component Ti-6Mo-6Nb-xSn-xMn alloys for biomedical applications. AIMS Materials Science, 2020, 7(2): 192-202. doi: 10.3934/matersci.2020.2.192 |
[2] | Kipkurui N Ronoh, Fredrick M Mwema, Stephen A Akinlabi, Esther T Akinlabi, Nancy W Karuri, Harrison T Ngetha . Effects of cooling conditions and grinding depth on sustainable surface grinding of Ti-6Al-4V: Taguchi approach. AIMS Materials Science, 2019, 6(5): 697-712. doi: 10.3934/matersci.2019.5.697 |
[3] | Daniela Šuryová, Igor Kostolný, Roman Koleňák . Fluxless ultrasonic soldering of SiC ceramics and Cu by Bi–Ag–Ti based solder. AIMS Materials Science, 2020, 7(1): 24-32. doi: 10.3934/matersci.2020.1.24 |
[4] | Muhammad Awwaluddin, Sri Hastuty, Djoko Hadi Prajitno, Makmuri, Budi Prasetiyo, Yudi Irawadi, Jekki Hendrawan, Harry Purnama, Eko Agus Nugroho . Effect of Yttrium on corrosion resistance of Zr-based alloys in Ringer's lactate solution for biomaterial applications. AIMS Materials Science, 2024, 11(3): 565-584. doi: 10.3934/matersci.2024028 |
[5] | Harish Mooli, Srinivasa Rao Seeram, Satyanarayana Goteti, Nageswara Rao Boggarapu . Optimal weld bead profiles in the conduction mode LBW of thin Ti–6Al–4V alloy sheets. AIMS Materials Science, 2021, 8(5): 698-715. doi: 10.3934/matersci.2021042 |
[6] | Jie Sun, Tzvetanka Boiadjieva-Scherzer, Hermann Kronberger, Kevin Staats, Johannes Holinka, Reinhard Windhager . Surface modification of Ti6Al4V alloy for implants by anodization and electrodeposition. AIMS Materials Science, 2019, 6(5): 713-729. doi: 10.3934/matersci.2019.5.713 |
[7] | Muhammad Awwaluddin, Djoko Hadi Prajitno, Wisnu Ari Adi, Maman Kartaman, Tresna P. Soemardi . Mechanical properties and corrosion behavior of novel β-type biomaterial Zr-6Mo-4Ti-xY alloys in simulated body fluid Ringer's lactate solution for implant applications. AIMS Materials Science, 2020, 7(6): 887-901. doi: 10.3934/matersci.2020.6.887 |
[8] | Nikolay A. Voronin . Analysis of the mechanisms of deformation of topocomposites by modeling of the indentation load-displacement curves. AIMS Materials Science, 2019, 6(3): 397-405. doi: 10.3934/matersci.2019.3.397 |
[9] | Liang Zhao, Weimian Guan, Jiwen Xu, Zhiyuan Sun, Maoda Zhang, Junjie Zhang . Atomistic investigation of effect of twin boundary on machinability in diamond cutting of nanocrystalline 3C-SiC. AIMS Materials Science, 2024, 11(6): 1149-1164. doi: 10.3934/matersci.2024056 |
[10] | Valeri S. Harutyunyan, Ashot P. Aivazyan, Andrey N. Avagyan . Stress field of a near-surface basal screw dislocation in elastically anisotropic hexagonal crystals. AIMS Materials Science, 2017, 4(6): 1202-1219. doi: 10.3934/matersci.2017.6.1202 |
Artificial intelligence (AI) and generative AI (GenAI) have sparked confusion and concern regarding their impact on education. Beyond the assessment integrity risks that currently draw the most attention, technologies such as ChatGPT, Copilot, and Gemini have also been identified as tools that can support learning. Project work, especially when there is no single correct solution, provides a great opportunity for integration, fostering technology knowledge and higher learning standards. However, no AI-integration framework for project-based work is available, resulting in a limited understanding of how AI integration can occur or be maximized. To address this, a collaborative effort of 16 educators from 9 Australian universities has led to the development of a generic AI implementation framework, built upon the CDIO approach. With a focus on engineering education, this framework can be adapted to other project-based learning contexts, where educators can pick and choose the relevant implementation items as needed. This framework is called the Project-work Artificial Intelligence Integration Framework (PAIIF), and its development and structure are outlined here. Initial implementations have shown the effectiveness of promoting reflection and guidance on where and how AI integration can occur.
The models involving entry-exit decisions apply to many situations, as stated in [1,2]. Such models may be described simply as follows. A firm decides when to invest in or when to abandon a project that can bring profit. There is plenty of literature on models concerning how to schedule the timing under the assumption that exiting the project does not take time, so we do not list them in detail, but refer to [1,2,3,4,5,6,7,8,9]. The ideas of entry-exit decisions apply to many concrete issues, for example, relationships of globalization and entrepreneurial entry-exit [10], when to invest or expand a start-up firm [11], when to enter or exit stock markets [12], and how to make a schedule for buying carbon emission rights [13].
In practice, the exiting process may take a long time, so ignoring it is not reasonable. For example, Brexit took more than 3 years, from June 23, 2016 to January 31, 2020 (https://www.britannica.com/topic/Brexit). The models [14,15] take account of the time, but with equal output rates in the regular production and exit periods. It is an apparent feature that the output rate of the project is usually reduced during the exit period. In this paper, we introduce a parameter to describe the output rate during the exit period and completely discuss the effects of output reduction on entry-exit decisions, under the assumption that the commodity price of the project follows a geometric Brownian motion.
We use the optimal stopping theory to carry out the study and obtain the explicit expressions of the optimal activating time and start time of the exit, which is one of the contributions of the paper. With these explicit expressions in hand, we can carefully analyze the effects of output reduction on entry-exit decisions, which is another contribution of the paper.
If the optimal choice is never to exit the project, the output reduction has no effect on the optimal entry-exit decision.
If the optimal exit is in a finite time, the situation becomes complicated. However, we obtain complete criterions, which determine the effects of output reduction on entry-exit decisions (see Section 4).
We outline the structure of this paper. In Section 2, we describe the model in detail. In Section 3, we determine an optimal entry-exit decision. In Section 4, we discuss the effects of output reduction during exit period on entry-exit decisions. Some conclusions are drawn in Section 5.
Assume that the price process P of one unit product follows
dP(t)=μP(t)dt+σP(t)dB(t)andP(0)=p, | (2.1) |
where μ∈R, σ,p>0, and B is a one dimensional standard Brownian motion, which denotes uncertainty. In this paper, all times are stopping times w.r.t. the filtration generated by the Brownian motion B.
Since involving the construction period leads to complicated calculations and distracts us from analyzing the effects of reduction on entry-exit decisions, and the effects of the construction period have been discussed in [3,15], we assume that there is no construction period (it may happen when the firm buys a project). The firm activates the project at time τI with the entry cost KI and completes the abandonment of the project during the time interval [τO,τO+δ], with the exit cost valued at KO at time τO+δ. Without loss of generality, we assume that the firm produces one unit product per unit time during the period [τI,τO] at the marginal cost C and α (0≤α≤1) unit products per unit time during the time interval of exiting the project [τO,τO+δ].
To answer the two questions, what time is optimal to activate the project and what time is optimal to start the abandonment procedure, we solve the optimization problem
J(p)=supτI≤τOEp[∫τOτIexp(−rt)(P(t)−C)dt+α∫τO+δτOexp(−rt)(P(t)−C)dt−exp(−rτI)KI−exp(−r(τO+δ))KO]. | (2.2) |
We call stopping times τI and τO the activating times and start times of the exit, respectively, and we call the function J the maximal expected present value of the project.
If r≤μ, some straight calculations show us that
Ep[∫+∞0exp(−rt)(P(t)−C)dt]=+∞. |
Thus, we obtain the following result.
Theorem 3.1. Assume that r≤μ, then τ∗I: =0 is an optimal activating time and τ∗O: =+∞ is an optimal start time of the exit, i.e., the firm should never exit the project. In addition, the function J in (2.2) is given by J≡+∞.
In the rest of this section, we assume r>μ.
Taking τI=τO: =0 in (2.2), we have
J(p)≥−KI+α(1−exp((μ−r)δ))r−μp−exp(−rδ)KO−αCr(1−exp(−rδ)), |
thus, in the remains of this section, we always assume that
rKI+exp(−rδ)rKO+α(1−exp(−rδ))C≥0 |
to avoid arbitrage opportunities.
Let λ1 and λ2 be the solutions to the equation
r−μλ−12σ2λ(λ−1)=0 |
with λ1<λ2, then we have λ1<0 and λ2>1.
Theorem 3.2. Assume that r>μ. The following are true:
(ⅰ) If
(1−α)C+(αC−rKO)exp(−rδ)≤0, |
then (τ∗I,τ∗O) is a solution to (2.2), where
τ∗I=inf{t:t>0,P(t)≥pI} |
and τ∗O=+∞. Here,
pI=λ2λ2−1(r−μ)(Cr+KI). |
In addition,
J(p)={Bpλ2,ifp<pI,pr−μ−Cr−KI,ifp≥pI, |
where
B=pI1−λ2λ2(r−μ). |
(ⅱ) If
(1−α)C+(αC−rKO)exp(−rδ)>0 |
and
α(1−exp((μ−r)δ))pO−αC(1−exp(−rδ))−exp(−rδ)rKO−rKI≤0, | (3.1) |
where
pO=λ1λ1−1r−μ1−α+αexp((μ−r)δ)((1−α)Cr+exp(−rδ)(αCr−KO)), |
then (τ∗I,τ∗O) is a solution to (2.2), where
τ∗I=inf{t:t>0,P(t)≥pI} |
and
τ∗O=inf{t:t>τ∗I,P(t)≤pO}. |
Here, pI is the largest solution of the algebraic equation
A(λ2−λ1)pλ1I+(λ2−1)r−μpI−λ2(Cr+KI)=0. |
In addition,
J(p)={Bpλ2,ifp<pI,Apλ1+pr−μ−Cr−KI,ifp≥pI, |
where
A=1−α+αexp((μ−r)δ)λ1(μ−r)pO1−λ1 |
and
B=λ1λ−12Apλ1−λ2I+pI1−λ2λ2(r−μ). |
Remark 3.3. We propose condition (3.1) to eliminate the possibility that the firm enters the project at a trigger price lower than the optimal trigger price of the exit, i.e., the firm enters the project and then immediately decides to exit the project.
In light of [14, Theorems 3.1 and 5.2], we have the following Lemma 3.4, which serves as preparation for the proof of Theorem 3.2.
Lemma 3.4. If (τ∗1,τ∗2) is a solution to the optimization problem
˜J(p):=supτI≤τOEp[∫τOτIexp(−rt)(P(t)−C)dt−exp(−rτI)KI−exp(−rτO)(l1P(τO)+l0)], | (3.2) |
it is also a solution to (2.2) and J(x)=˜J(x), where
l1:=α(1−exp((μ−r)δ))μ−r |
and
l0:=αCr(1−exp(−rδ))+exp(−rδ)KO. |
Proof. By the strong Markov property of the process {(s+t,P(t)),t≥0}, where s∈R, we have
Ep[∫τOτIexp(−rt)(P(t)−C)dt+α∫τO+δτOexp(−rt)(P(t)−C)dt−exp(−rτI)KI−exp(−r(τO+δ))KO]=Ep[∫τOτIexp(−rt)(P(t)−C)dt+αexp(−rτO)EP(τO)[∫δ0exp(−rt)(P(t)−C)dt] −exp(−rτI)KI−exp(−r(τO+δ))KO]. |
Thus, we need to calculate
αEP(τO)[∫δ0exp(−rt)(P(t)−C)dt]−exp(−rδ)KO=α∫δ0exp(−rt)(exp(μt)P(τO)−C)dt−exp(−rδ)KO=−l1P(τO)−l0. |
The proof is complete.
Remark 3.5. The proof of Lemma 3.4 has an economic meaning as follows. We first discount the benefit during the abandonment period to time τO, then discount this value to time zero.
With the help of Lemma 3.4, we can prove Theorem 3.2.
Proof of Theorem 3.2. (1) By Lemma 3.4, we solve problem (3.2),
supτI≤τOEp[∫τOτIexp(−rt)(P(t)−C)dt−exp(−rτI)KI−exp(−rτO)(l1P(τO)+l0)]=supτI≤τOEp[exp(−rτI)∫τO−τI0exp(−rt)(P(t+τI)−C)dt−exp(−rτI)KI−exp(−rτO)(l1P(τO)+l0)]=supτIEp[exp(−rτI)(G(P(τI))−KI)]=:H(p), | (3.3) |
where
G(p):=supτOEp[∫τO0exp(−rt)(P(t)−C)dt−exp(−rτO)(l1P(τO)+l0)]. | (3.4) |
(2) Assume
(1−α)C+(αC−rKO)exp(−rδ)≤0. |
We first solve problem (3.4) and then problem (3.3).
For problem (3.4), noting
Ep[∫∞0exp(−rt)(P(t)−C)dt]=pr−μ−Cr, |
we see that
Ep[∫∞0exp(−rt)(P(t)−C)dt]≥−l1p−l0, |
which implies
τ∗O=+∞ |
and
G(p)=pr−μ−Cr. |
Set
h(p):=pr−μ−Cr−KI. |
Since
{p:p>0andrh(p)−μph′(p)≥0}={p:p≥C+rKI}, |
the exercise region of problem (3.3) takes the form [pI,+∞) for some
pI≥C+rKI. |
The function H satisfies
rH−μpH′−12σ2p2H″=0 |
on the continuation region (0,pI) and is Lipschitz continuous on (0,+∞) and C1 continuous at pI. Thus, we get
H(p)=Bpλ2 |
and B and pI solve
{Bpλ2I=pIr−μ−Cr−KI,λ2Bpλ2−1I=1r−μ, |
by which we finish the proof of (ⅰ).
(3) Assume
(1−α)C+(αC−rKO)exp(−rδ)>0 |
and (3.1) hold. We again first solve problem (3.4) and then problem (3.3).
Set
g(p):=−l1p−l0. |
A straight calculation shows that
{p:p>0andrg−μpg′(p)−p+C≥0}=(0,(1−α)C+(αC−rKO)exp(−rδ)1−α(1−exp((μ−r)δ))), |
which means the exercise region of problem (3.4) takes the form (0,pO] for some
pO≤(1−α)C+(αC−rKO)exp(−rδ)1−α(1−exp((μ−r)δ)). |
The function G satisfies
rG−μpG′−12σ2p2G″−p+C=0 |
on the continuation region (pO,+∞) and is Lipschitz continuous on (0,+∞) and C1 continuous at pO. Thus, we get
G(p)=Apλ1+pr−μ−Cr, |
and A and pO solve
{Apλ1O+pOr−μ−Cr=−l1pO−l0,λ1Apλ1−1O+1r−μ=−l1, |
which implies coefficient A and the optimal exit trigger pricer of (ⅱ).
To solve problem (3.4), we define
h(p):=G(p)−KI. |
In light of (3.1),
{p:p>0andrh(p)−μph′(p)≥0}={p:p≥C+rKI}, |
thus, the exercise region of problem (3.3) takes the form [pI,+∞) for some
pI≥C+rKI. |
The function H satisfies
rH−μpH′−12σ2p2H″=0 |
on the continuation region (0,pI) and is Lipschitz continuous on (0,+∞) and C1 continuous at pI. Thus, we get
H(p)=Bpλ2, |
and B and pI solve
{Bpλ2I=Apλ1I+pIr−μ−Cr−KI,λ2Bpλ2−1I=λ1Apλ1−1I+1r−μ, |
by which we finish the proof of (ⅱ).
We analyze the effects of output reduction during the exit period on entry-exit decisions.
If r≤μ, the firm has an optimal time τ∗I=0 to activate the project and should never exit the project. Thus, the reduction does not affect entry-exit decisions.
If r>μ and
(1−α)C+(αC−rKO)exp(−rδ)≤0, |
the optimal time to activate the project is given by
τ∗I=inf{t:t>0,P(t)≥pI}, |
where
pI=λ2λ2−1(r−μ)(Cr+KI). |
Thus, the reduction does not affect the optimal activating time. As same as the case of r≤μ, the firm should never exit the project and the reduction does not affect exit decisions.
Assume that r>μ and
(1−α)C+(αC−rKO)exp(−rδ)>0. |
We first analyze the effects of reduction on the optimal start time of the exit. By (ⅱ) of Theorem 3.2, the optimal trigger price is an increasing function of α if
exp(−μδ)(C−rKO)>C−exp(−rδ)rKO, |
a decreasing function if
exp(−μδ)(C−rKO)<C−exp(−rδ)rKO, |
and a constant function if
exp(−μδ)(C−rKO)=C−exp(−rδ)rKO. |
We list some examples to illustrate the analysis. Taking
r=0.2,μ=−0.1,σ=0.3,δ=2,C=5,KI=20,andKO=−10, |
we have
exp(−μδ)(C−rKO)>C−exp(−rδ)rKO, |
then the optimal trigger price is an increasing function of α. See Figure 1. If we replace μ=−0.1 with μ=0.1, we have a decreasing function. See Figure 2.
We conclude that:
(1) If
exp(−μδ)(C−rKO)>C−exp(−rδ)rKO, |
as the reduction increases (i.e., α decreases), the firm will postpone the exit.
(2) If
exp(−μδ)(C−rKO)<C−exp(−rδ)rKO, |
as the reduction increases, the firm will advance the exit.
(3) If
exp(−μδ)(C−rKO)=C−exp(−rδ)rKO, |
the reduction does not affect the exit.
To analyze the effects of reduction on the optimal time to activate the project, we define two functions as follows:
f(p):=A(λ2−λ1)pλ1 |
and
g(p):=−(λ2−1)r−μp+λ2(Cr+KI) |
according to (ⅱ) of Theorem 3.2. Thus, the functions f and g intersect at two points, say, (p1,f(p1)) and (p2,f(p2)) with p1<p2, and the optimal trigger price pI of activating the project is given by pI=p2.
To capture the behavior of pI as α varies, we only need to examine the behavior of the coefficient A as α varies. Setting
M:=(C−exp(−rδ)rKO)(1−exp((μ−r)δ))+(1−λ1)exp(−rδ)(C−rKO−Cexp(μδ)+exp((μ−r)δ)rKO) |
and
N:=−C(1−exp(−rδ))(1−exp((μ−r)δ)), |
after some calculations, we find that:
(1) If M/N≤0, A is increasing on [0,1] and pI is decreasing on [0,1].
(2) If M/N≥1, A is decreasing on [0,1] and pI is increasing on [0,1].
(3) If 0<M/N<1, A is decreasing on [0,M/N] and increasing on [M/N,1] and pI is increasing on [0,M/N] and decreasing on [M/N,1].
Numerical examples help us understand the analysis above.
Figure 3 demonstrates the decreasing of pI (r=0.2, μ=−0.1, σ=0.3, δ=0.6, C=5, KI=20, and KO=−10). Figure 4 demonstrates the increasing of pI (r=0.2, μ=0.1, σ=0.3, δ=2, C=5, KI=20, and KO=−10), and Figure 5 demonstrates the non-monotonicity of pI (r=0.2, μ=−0.1, σ=0.3, δ=0.6, C=5, KI=20, and KO=−10).
In other words, here are the effects of the reduction on the optimal time of activating the project:
(1) If M/N≤0, as the reduction increases (i.e., α decreases), the firm will postpone activating the project.
(2) If M/N≥0, as the reduction increases, the firm will advance activating the project.
(3) If 0<M/N<1, as the reduction increases, the firm will postpone activating the project then advance activating the project.
There is an apparent phenomenon that firms usually reduce their output rate during stopping the regular production of a project. We intend to analyze the effects of reduction on the optimal entry-exit decision. Since the papers [3,15] have investigated the effects of the construction period on entry-exit decisions, we assume that the project has been constructed and the production immediately starts for concentrating on the study of the effects of reduction.
We introduce the output rate into the models [14,15] and describe the problem using the optimal stopping theory, obtaining explicit solutions in Theorems 3.1 and 3.2. These explicit solutions help us in discovering the effects of reduction on the optimal entry-exit decision.
We carefully examine the effects of reduction. According the analysis listed in Section 4, we come to the effects of reduction as follows. If the firm should never exit the project to obtain the maximal profit, the reduction does not affect the optimal entry-exit decision. However, if the firm exits the project in finite time, the effects show in a complicated manner. We study the effects analytically and numerically, and provide the relations of the parameters involved in the model that can determine the effects.
The author declares he has not used Artificial Intelligence (AI) tools in the creation of this article.
Many thanks are due to the editors and reviewers for their constructive suggestions and valuable comments. This work is partially supported by the Fundamental Research Funds for the Central Universities (Grant No. N2023034).
No potential conflict of interest exists regarding the publication of this paper.
[1] |
Nikolic, S., Daniel, S., Haque, R., Belkina, M., Hassan, G.M., Grundy, S., et al., ChatGPT versus Engineering Education Assessment: A Multidisciplinary and Multi-institutional Benchmarking and Analysis of this Generative Artificial Intelligence Tool to Investigate Assessment Integrity. European Journal of Engineering Education, 2023, 48(4): 559‒614. https://doi.org/10.1080/03043797.2023.2213169 doi: 10.1080/03043797.2023.2213169
![]() |
[2] | Mollick, E., Co-intelligence: Living and Working with AI. London. WH Allen. 2024. |
[3] |
Nikolic, S., Sandison, C., Haque, R., Daniel, S., Grundy, S., Belkina, M., et al., ChatGPT, Copilot, Gemini, SciSpace and Wolfram versus Higher Education Assessments: An Updated Multi-Institutional Study of the Academic Integrity Impacts of Generative Artificial Intelligence (GenAI) on Assessment, Teaching and Learning in Engineering. Australasian Journal of Engineering Education, 2024, 29(2): 126‒153. https://doi.org/10.1080/22054952.2024.2372154 doi: 10.1080/22054952.2024.2372154
![]() |
[4] |
Bearman, M., Tai, J., Dawson, P., Boud, D. and Ajjawi, R., Developing evaluative judgement for a time of generative artificial intelligence. Assessment & Evaluation in Higher Education, 2024, 49(6): 893‒905. https://doi.org/10.1080/02602938.2024.2335321 doi: 10.1080/02602938.2024.2335321
![]() |
[5] |
Kizilcec, R.F., Huber, E., Papanastasiou, E.C., Cram, A., Makridis, C.A., Smolansky, A., et al., Perceived impact of generative AI on assessments: Comparing educator and student perspectives in Australia, Cyprus, and the United States. Computers and Education: Artificial Intelligence, 2024, 7: 100269. https://doi.org/10.1016/j.caeai.2024.100269 doi: 10.1016/j.caeai.2024.100269
![]() |
[6] | Quince, Z., Petkoff, K., Michael, R.N., Daniel, S. and Nikolic, S., The current ethical considerations of using GenAI in engineering education and practice: A systematic literature review. 35th Annual Conference of the Australasian Association for Engineering Education, 2024, Christchurch, New Zealand. |
[7] | Hysaj, A., Farouqa, G., Khan, S.A. and Hiasat, L., A Tale of Academic Writing Using AI Tools: Lessons Learned from Multicultural Undergraduate Students. Social Computing and Social Media, Cham. 2024. https://doi.otg/10.1007/978-3-031-61305-0_3 |
[8] | Fatahi, B., Nguyen, L.D., Khabbaz, H. and Hadgraft, R., Virtual Teammates: Transforming Engineering Learning through Generative AI Integration. 35th Australasian Association of Engineering Education Conference, Christchurch, New Zealand. 2024. |
[9] |
Honig, C., Rios, S. and Desu, A., Generative AI in engineering education: understanding acceptance and use of new GPT teaching tools within a UTAUT framework. Australasian Journal of Engineering Education, 2025, 1‒13. https://doi.org/10.1080/22054952.2025.2467500 doi: 10.1080/22054952.2025.2467500
![]() |
[10] |
Ajjawi, R., Tai, J., Dollinger, M., Dawson, P., Boud, D. and Bearman, M., From authentic assessment to authenticity in assessment: broadening perspectives. Assessment & Evaluation in Higher Education, 2023, 49(4): 499‒510. https://doi.org/10.1080/02602938.2023.2271193 doi: 10.1080/02602938.2023.2271193
![]() |
[11] |
Miao, G., Ranaraja, I., Grundy, S., Brown, N., Belkina, M. and Goldfinch, T., Project-based learning in Australian & New Zealand universities: current practice and challenges. Australasian Journal of Engineering Education, 2024, 1‒13. https://doi.org/10.1080/22054952.2024.2358576 doi: 10.1080/22054952.2024.2358576
![]() |
[12] | Mills, J.E. and Treagust, D.F., Engineering education—Is problem-based or project-based learning the answer. Australasian Journal of Engineering Education, 2003, 3(2): 2‒16. |
[13] |
Baig, M.I. and Yadegaridehkordi, E., ChatGPT in the higher education: A systematic literature review and research challenges. International journal of educational research, 2024,127: 102411. https://doi.org/10.1016/j.ijer.2024.102411 doi: 10.1016/j.ijer.2024.102411
![]() |
[14] | Nikolic, S. and Beckman, K., Supporting Engineering Project-Based Learning through the Use of ChatGPT and Generative AI: A Case Study. In H. Crompton & D. Burke (Eds.), Artificial Intelligence Applications in Higher Education: Theories, Ethics, and Case Studies for Universities, 2025,215‒232. Routledge. https://doi.org/10.4324/9781003440178 |
[15] |
Grilli, L. and Pedota, M., Creativity and artificial intelligence: A multilevel perspective. Creativity and Innovation Management, 2024, 33(2): 234‒247. https://doi.org/10.1111/caim.12580 doi: 10.1111/caim.12580
![]() |
[16] | Crawley, E.F., Malmqvist, J., Lucas, W.A. and Brodeur, D.R., The CDIO syllabus v2. 0. An updated statement of goals for engineering education. Proceedings of the 7th International CDIO Conference, 2011. |
[17] |
Lee, D., Arnold, M., Srivastava, A., Plastow, K., Strelan, P., Ploeckl, F., et al., The impact of generative AI on higher education learning and teaching: A study of educators' perspectives. Computers and Education: Artificial Intelligence, 2024, 6: 100221. https://doi.org/10.1016/j.caeai.2024.100221 doi: 10.1016/j.caeai.2024.100221
![]() |
[18] |
Lim, W.M., Gunasekara, A., Pallant, J.L., Pallant, J.I. and Pechenkina, E., Generative AI and the future of education: Ragnarök or reformation? A paradoxical perspective from management educators. The International Journal of Management Education, 2023, 21(2): 100790. https://doi.org/10.1016/j.ijme.2023.100790 doi: 10.1016/j.ijme.2023.100790
![]() |
[19] |
Nikolic, S., Wentworth, I., Sheridan, L., Moss, S., Duursma, E., Jones, R.A., et al., A systematic literature review of attitudes, intentions and behaviours of teaching academics pertaining to AI and generative AI (GenAI) in higher education: An analysis of GenAI adoption using the UTAUT framework. Australasian Journal of Educational Technology, 2024, 40(6): 56‒75. https://doi.org/10.14742/ajet.9643 doi: 10.14742/ajet.9643
![]() |
[20] |
Ahmed, Z., Shanto, S.S. and Jony, A.I., Potentiality of generative AI tools in higher education: Evaluating ChatGPT's viability as a teaching assistant for introductory programming courses. STEM Education, 2024, 4(3): 165‒182. https://doi.org/10.3934/steme.2024011 doi: 10.3934/steme.2024011
![]() |
[21] |
Kim, D., Majdara, A. and Olson, W., A Pilot Study Inquiring into the Impact of ChatGPT on Lab Report Writing in Introductory Engineering Labs. International Journal of Technology in Education, 2024, 7(2): 259‒289. https://doi.org/10.46328/ijte.691 doi: 10.46328/ijte.691
![]() |
[22] | Nikolic, S., Heath, A., Vu, B.A., Daniel, S., Alimardani, A., Sandison, C., et al., Prompt Potential: A Pilot Assessment of Using Generative Artificial Intelligence (ChatGPT-4) as a Tutor for Engineering and Maths. 52nd Annual Conference of the European Society for Engineering Education (SEFI), 2024, Lausanne, Switzerland. |
[23] |
Liu, J., Wang, C., Liu, Z., Gao, M., Xu, Y., Chen, J., et al., A bibliometric analysis of generative AI in education: current status and development. Asia Pacific Journal of Education, 2024, 44(1): 156‒175. https://doi.org/10.1080/02188791.2024.2305170 doi: 10.1080/02188791.2024.2305170
![]() |
[24] |
Bobrytska, V.I., Krasylnykova, H.V., Beseda, N.А., Krasylnykov, S.R. and Skyrda, T.S., Artificial intelligence (AI) in Ukrainian Higher Education: A Comprehensive Study of Stakeholder Attitudes, Expectations and Concerns. International Journal of Learning, Teaching and Educational Research, 2024, 23(1): 400‒426. https://doi.org/10.26803/ijlter.23.1.20 doi: 10.26803/ijlter.23.1.20
![]() |
[25] |
Luo, J., A critical review of GenAI policies in higher education assessment: a call to reconsider the "originality" of students' work. Assessment & Evaluation in Higher Education, 2024, 49(5): 651‒664. https://doi.org/10.1080/02602938.2024.2309963 doi: 10.1080/02602938.2024.2309963
![]() |
[26] |
Southworth, J., Migliaccio, K., Glover, J., Reed, D., McCarty, C., Brendemuhl, J., et al, Developing a model for AI Across the curriculum: Transforming the higher education landscape via innovation in AI literacy. Computers and Education: Artificial Intelligence, 2023, 4: 100127. https://doi.org/10.1016/j.caeai.2023.100127 doi: 10.1016/j.caeai.2023.100127
![]() |
[27] |
Shailendra, S., Kadel, R., and Sharma, A., Framework for Adoption of Generative Artificial Intelligence (GenAI) in Education. IEEE Transactions on Education, 2024, 67(5): 777‒785. https://doi.org/10.1109/TE.2024.3432101 doi: 10.1109/TE.2024.3432101
![]() |
[28] |
Shanto, S.S., Ahmed, Z. and Jony, A.I., PAIGE: A generative AI-based framework for promoting assignment integrity in higher education. STEM Education, 2023, 3(4), 288‒305. https://doi.org/10.3934/steme.2023018 doi: 10.3934/steme.2023018
![]() |
[29] | Ambikairajah, E., Sirojan, T., Thiruvaran, T. and Sethu, V., ChatGPT in the Classroom: A Shift in Engineering Design Education. 2024 IEEE Global Engineering Education Conference (EDUCON), 2024, Kos, Greece. |
[30] |
Salinas-Navarro, D.E., Vilalta-Perdomo, E., Michel-Villarreal, R. and Montesinos, L., Designing experiential learning activities with generative artificial intelligence tools for authentic assessment. Interactive Technology and Smart Education, 2024, 21(4): 708‒734. https://doi.org/10.1108/ITSE-12-2023-0236 doi: 10.1108/ITSE-12-2023-0236
![]() |
[31] |
Prieto, S.A., Mengiste, E.T. and García de Soto, B., Investigating the Use of ChatGPT for the Scheduling of Construction Projects. Buildings, 2023, 13(4): 1‒16. https://doi.org/10.3390/buildings13040857 doi: 10.3390/buildings13040857
![]() |
[32] |
Fosso Wamba, S., Queiroz, M.M., Chiappetta Jabbour, C.J. and Shi, C., Are both generative AI and ChatGPT game changers for 21st-Century operations and supply chain excellence? International journal of production economics, 2023,265: 109015. https://doi.org/10.1016/j.ijpe.2023.109015 doi: 10.1016/j.ijpe.2023.109015
![]() |
[33] | Manresa, A., Sammour, A., Mas-Machuca, M., Chen, W. and Botchie, D., Humanizing GenAI at work: bridging the gap between technological innovation and employee engagement. Journal of Managerial Psychology, 2024, ahead-of-print. https://doi.org/10.1108/JMP-05-2024-0356 |
[34] | Humlum, A. and Vestergaard, E., The Adoption of ChatGPT. University of Chicago, Becker Friedman Institute for Economics Working Paper, 2024(2024-50). |
[35] |
Guo, P., Saab, N., Post, L.S. and Admiraal, W., A review of project-based learning in higher education: Student outcomes and measures. International journal of educational research, 2020,102: 101586. https://doi.org/https://doi.org/10.1016/j.ijer.2020.101586 doi: 10.1016/j.ijer.2020.101586
![]() |
[36] |
Gomez-del Rio, T. and Rodriguez, J., Design and assessment of a project-based learning in a laboratory for integrating knowledge and improving engineering design skills. Education for Chemical Engineers, 2022, 40: 17‒28. https://doi.org/https://doi.org/10.1016/j.ece.2022.04.002 doi: 10.1016/j.ece.2022.04.002
![]() |
[37] | Gregory, S., O'Connell, J., Butler, D., McDonald, M., Kerr, T., Schutt, S., et al., New applications, new global audiences: Educators repurposing and reusing 3D virtual and immersive learning resources. 2015 ASCILITE Annual Conference, 2015, Perth, Australia. |
[38] |
Nikolic, S., Suesse, T.F., Grundy, S., Haque, R., Lyden, S., Lal, S., et al., Assessment integrity and validity in the teaching laboratory: adapting to GenAI by developing an understanding of the verifiable learning objectives behind laboratory assessment selection. European Journal of Engineering Education, 2025, 1‒28. https://doi.org/10.1080/03043797.2025.2456944 doi: 10.1080/03043797.2025.2456944
![]() |
[39] |
Lee, M.J.W., Nikolic, S., Vial, P.J., Ritz, C., Li, W. and Goldfinch, T., Enhancing project-based learning through student and industry engagement in a video-augmented 3-D virtual trade fair. IEEE Transactions on Education, 2016, 59(4): 290‒298. https://doi.org/10.1109/TE.2016.2546230 doi: 10.1109/TE.2016.2546230
![]() |
[40] |
Witarsa, and Muhammad, S., Critical thinking as a necessity for social science students capacity development: How it can be strengthened through project based learning at university. Frontiers in Education, 2023, 7(1). https://doi.org/10.3389/feduc.2022.983292 doi: 10.3389/feduc.2022.983292
![]() |
[41] |
Markula, A. and Aksela, M., The key characteristics of project-based learning: how teachers implement projects in K-12 science education. Disciplinary and Interdisciplinary Science Education Research, 2022, 4(1): 2. https://doi.org/10.1186/s43031-021-00042-x doi: 10.1186/s43031-021-00042-x
![]() |
[42] |
Beneroso, D. and Robinson, J., Online project-based learning in engineering design: Supporting the acquisition of design skills. Education for Chemical Engineers, 2022, 38: 38‒47. https://doi.org/https://doi.org/10.1016/j.ece.2021.09.002 doi: 10.1016/j.ece.2021.09.002
![]() |
[43] |
Edström, K. and Kolmos, A., PBL and CDIO: complementary models for engineering education development. European Journal of Engineering Education, 2014, 39(5): 539‒555. https://doi.org/10.1080/03043797.2014.895703 doi: 10.1080/03043797.2014.895703
![]() |
[44] | Crawley, E.F., The CDIO Syllabus: A statement of goals for undergraduate engineering education. Massachusetts Institute of Technology Cambridge. 2001. |
[45] | Ramírez de Dampierre, M., Gaya-López, M.C. and Lara-Bercial, P.J., Evaluation of the Implementation of Project-Based-Learning in Engineering Programs: A Review of the Literature. Education Sciences, 2024, 14(10): 1107. https://www.mdpi.com/2227-7102/14/10/1107 |
[46] |
Tanveer, B. and Usman, M., An Empirical Study on the Use of CDIO in Software Engineering Education. IEEE Transactions on Education, 2022, 65(4): 684‒694. https://doi.org/10.1109/TE.2022.3163911 doi: 10.1109/TE.2022.3163911
![]() |
[47] |
O'Connor, S., Power, J. and Blom, N., A systematic review of CDIO knowledge library publications (2010–2020): An Overview of trends and recommendations for future research. Australasian Journal of Engineering Education, 2023, 28(2): 166‒180. https://doi.org/10.1080/22054952.2023.2220265 doi: 10.1080/22054952.2023.2220265
![]() |
[48] | Malmqvist, J., Lundqvist, U., Rosén, A. and Edström, K., The CDIO syllabus 3.0-an updated statement of goals. 18th International CDIO Conference, June 13-15 2022, Reykjavik. 2022. |
[49] |
Sablatzky, T., The Delphi method. Hypothesis: Research Journal for Health Information Professionals, 2022, 34(1). https://doi.org/10.18060/26224 doi: 10.18060/26224
![]() |
[50] | Linstone, H.A. and Turoff, M., The delphi method, Addison-Wesley Reading, MA, 1975. |
[51] |
Kokotsaki, D., Menzies, V. and Wiggins, A., Project-based learning: A review of the literature. Improving Schools, 2016, 19(3): 267‒277. https://doi.org/10.1177/1365480216659733 doi: 10.1177/1365480216659733
![]() |
[52] |
Francisco, M.G., Canciglieri Junior, O. and Sant'Anna, Â.M.O., Design for six sigma integrated product development reference model through systematic review. International Journal of Lean Six Sigma, 2020, 11(4): 767‒795. https://doi.org/10.1108/IJLSS-05-2019-0052 doi: 10.1108/IJLSS-05-2019-0052
![]() |
[53] | Dowling, D., Hadgraft, R., Carew, A., McCarthy, T., Hargreaves, D., Baillie, C., et al., Engineering Your Future, Melbourne. Wiley. 2019. |
[54] |
Bukar, U.A., Sayeed, M.S., Razak, S.F.A., Yogarayan, S., and Sneesl, R., Decision-Making Framework for the Utilization of Generative Artificial Intelligence in Education: A Case Study of ChatGPT. IEEE Access, 2024. https://doi.org/10.1109/ACCESS.2024.3425172 doi: 10.1109/ACCESS.2024.3425172
![]() |
[55] |
Guo, W., Li, W. and Tisdell, C.C., Effective pedagogy of guiding undergraduate engineering students solving first-order ordinary differential equations. Mathematics, 2021, 9(14): 1623. https://doi.org/10.3390/math9141623. doi: 10.3390/math9141623
![]() |