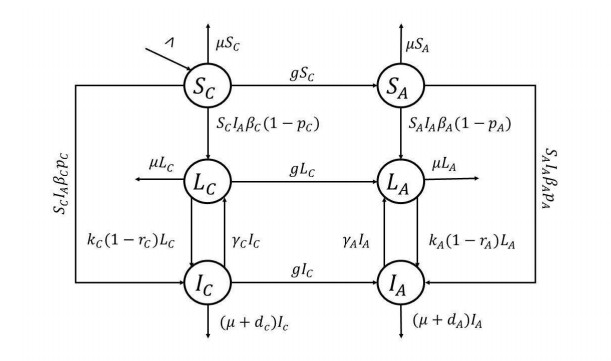
Hepatitis B is a major global challenge, but there is a lack of epidemiological research on hepatitis B incidence from a change point perspective. This study aimed to fill this gap by identifying significant change points and trends in hepatitis time series in Xinjiang, China. The datasets were obtained from the Xinjiang Information System for Disease Control and Prevention. The Mann-Kendall-Sneyers (MKS) test was used to detect change points and trend changes on the hepatitis B time series of 14 regions in Xinjiang, and the effectiveness of this method was validated by comparing it with the binary segmentation (BS) and segment regression (SR) methods. Based on the results of change point analysis, the prevention and control policies and measures of hepatitis in Xinjiang were discussed. The results showed that 8 regions (57.1%) with at least one change fell within the 95% confidence interval (CI) in all 14 regions by the MKS test, where five regions (Turpan (TP), Hami (HM), Bayingolin (BG), Kyzylsu Kirgiz (KK), Altai (AT)) were identified at one change point, two change points existed for two regions (Aksu (AK), Hotan (HT)) and three change points was detected in 1 region (Bortala (BT)). Most of the change points occurred at both ends of the sequence. More change points indicated an upward trend in the front half of the sequence, while in the latter half, many change points indicated a downward trend prominently. Finally, in comparing the results of the three change point tests, the MKS test showed a 61.5% agreement (8/13) with the BS and SR.
Citation: Liping Yang, Na Xie, Yanru Yao, Chunxia Wang, Maozai Tian, Kai Wang. Hepatitis B time series in Xinjiang, China (2006–2021): change point detection based on the Mann-Kendall-Sneyers test[J]. Mathematical Biosciences and Engineering, 2024, 21(2): 2458-2469. doi: 10.3934/mbe.2024108
[1] | Hongyan Wang, Shaoping Jiang, Yudie Hu, Supaporn Lonapalawong . Analysis of drug-resistant tuberculosis in a two-patch environment using Caputo fractional-order modeling. AIMS Mathematics, 2024, 9(11): 32696-32733. doi: 10.3934/math.20241565 |
[2] | Saima Rashid, Yolanda Guerrero Sánchez, Jagdev Singh, Khadijah M Abualnaja . Novel analysis of nonlinear dynamics of a fractional model for tuberculosis disease via the generalized Caputo fractional derivative operator (case study of Nigeria). AIMS Mathematics, 2022, 7(6): 10096-10121. doi: 10.3934/math.2022562 |
[3] | Hasib Khan, Jehad Alzabut, J.F. Gómez-Aguilar, Praveen Agarwal . Piecewise mABC fractional derivative with an application. AIMS Mathematics, 2023, 8(10): 24345-24366. doi: 10.3934/math.20231241 |
[4] | Qun Dai, Longkun Zhang . A dual delays epidemic model for TB with adaptive mobility behavior. AIMS Mathematics, 2025, 10(7): 15231-15263. doi: 10.3934/math.2025683 |
[5] | Cicik Alfiniyah, Wanwha Sonia Putri Artha Soetjianto, Ahmadin, Muhamad Hifzhudin Noor Aziz, Siti Maisharah Sheikh Ghadzi . Mathematical modeling and optimal control of tuberculosis spread among smokers with case detection. AIMS Mathematics, 2024, 9(11): 30472-30492. doi: 10.3934/math.20241471 |
[6] | Manvendra Narayan Mishra, Faten Aldosari . Comparative study of tuberculosis infection by using general fractional derivative. AIMS Mathematics, 2025, 10(1): 1224-1247. doi: 10.3934/math.2025058 |
[7] | Kamel Guedri, Rahat Zarin, Mowffaq Oreijah . Evaluating the impact of vaccination and progression delays on tuberculosis dynamics with disability outcomes: A case study in Saudi Arabia. AIMS Mathematics, 2025, 10(4): 7970-8001. doi: 10.3934/math.2025366 |
[8] | Alessandra Jannelli, Maria Paola Speciale . On the numerical solutions of coupled nonlinear time-fractional reaction-diffusion equations. AIMS Mathematics, 2021, 6(8): 9109-9125. doi: 10.3934/math.2021529 |
[9] | J. Vanterler da C. Sousa, E. Capelas de Oliveira, L. A. Magna . Fractional calculus and the ESR test. AIMS Mathematics, 2017, 2(4): 692-705. doi: 10.3934/Math.2017.4.692 |
[10] | Fawaz K. Alalhareth, Seham M. Al-Mekhlafi, Ahmed Boudaoui, Noura Laksaci, Mohammed H. Alharbi . Numerical treatment for a novel crossover mathematical model of the COVID-19 epidemic. AIMS Mathematics, 2024, 9(3): 5376-5393. doi: 10.3934/math.2024259 |
Hepatitis B is a major global challenge, but there is a lack of epidemiological research on hepatitis B incidence from a change point perspective. This study aimed to fill this gap by identifying significant change points and trends in hepatitis time series in Xinjiang, China. The datasets were obtained from the Xinjiang Information System for Disease Control and Prevention. The Mann-Kendall-Sneyers (MKS) test was used to detect change points and trend changes on the hepatitis B time series of 14 regions in Xinjiang, and the effectiveness of this method was validated by comparing it with the binary segmentation (BS) and segment regression (SR) methods. Based on the results of change point analysis, the prevention and control policies and measures of hepatitis in Xinjiang were discussed. The results showed that 8 regions (57.1%) with at least one change fell within the 95% confidence interval (CI) in all 14 regions by the MKS test, where five regions (Turpan (TP), Hami (HM), Bayingolin (BG), Kyzylsu Kirgiz (KK), Altai (AT)) were identified at one change point, two change points existed for two regions (Aksu (AK), Hotan (HT)) and three change points was detected in 1 region (Bortala (BT)). Most of the change points occurred at both ends of the sequence. More change points indicated an upward trend in the front half of the sequence, while in the latter half, many change points indicated a downward trend prominently. Finally, in comparing the results of the three change point tests, the MKS test showed a 61.5% agreement (8/13) with the BS and SR.
The human population has not been spared by infectious diseases irrespective of the medical facilities. In spite of great achievement in the advancement of medicine, the world is still struggling to combat infectious diseases [1]. Mycobacterium tuberculosis (MTB) is responsible for Tuberculosis (TB) infection which primarily affects the lungs (pulmonary TB). However, the disease can also affect some other systems in the human body such as the circulatory system, the central nervous system, the genital-urinary system. TB can be transmitted through a medium including the kiss, speak, cough, sneeze [1,2]. It can even affect individuals through the utilization of infected persons unsterilized eating utensils. In some instance, pregnant women who have an active TB can affect the baby in the womb. The actively infected individuals can cause infection but not the latent TB patient. The transmission of TB dependents on the number of infectious droplets expelled by a carrier, the quality of ventilation available and finally, the duration of the individuals exposed to virulence of the MTB strain. The spread of TB can be reduced by isolating the active infectious individual and immediately introducing viable anti-tuberculosis therapy [3,4,5]. At the moment, 95% of the world total TB patients of 8 million each year are found in the developing countries. The age bracket that most affected by TB is 15–59. In the developing countries, TB has been identified as the leading death causes in the majority of the Sub-Sahara counties [6]. TB has been established as the leading cause of death in sub-Saharan Africa which account for at least 2 million deaths [7]. TB in children under the age of fifteen (15) is very critical public health problem since it account for most of the current TB transmission [8].
Adaption of modern practice help improves TB control because of factors such as endogenous reactivation, the presence of multi-drug resistant TB, the high rate of HIV incidence in the last two decades. Exogenous re-infection of TB is another concern and very common in Africa. A comprehensive understanding of treatment with respect to the regional transmission of TB is crucial in the fighting of this disease [9].
Mathematical modeling in recent times has been identified as an essential tool that provides qualitative information about the epidemiology of many diseases and strategies in controlling them. Several theoretical studies have been undertaking with regard to TB infection. Several integer-order TB models have been constructed and investigated by some authors [10,11,12,13,14]. The mentioned integer TB models do not predict accurately because they do not have memory effect in order to provide an accurate prediction. However, non-integer models possess memory effect and most of the operators have crossover properties that enhance accurate predictions.
Fractional calculus (FC) is a branch of applied mathematics that generalizes the integer calculus to fractional order [15,16]. In recent times, the use of fractional calculus in the scientific community has gained enough attention because of its numerous applications including epidemiology, engineering, water resource management [17,18,19,20,21,22,23,24]. The use of fractional derivative in modeling epidemiology has become important because the result takes into consideration the memory effect which naturally occurs in several biological models. The reason is that fractional derivative models give the real phenomena associated with the given problem. The fractional of derivatives and integrals give a vivid description of the memory and hereditary characteristics inherent in many materials and other processes [25]. Usually, the integer order can provide information about two points which realistically may not be true. The concept of classical fractional-order derivative was proposed by Riemann and Liouville. It was well known that Caputo and Riemann-Liouville have singular kernels. Next, Caputo and Fabrizio in [26] presented a new definition of fractional derivative without singular kernel which proved to be good and many researchers are applying. Caputo and Fabrizio [27] also investigated the notion of fractional derivative and applications to the hysteresis phenomena. The fractional calculus is now used in mathematical modeling of impulsive fractional functional differential equations. A more interesting study regarding the impulsive fractional functional differential equations and their applications is studied in [28].
Recently, Atangana and Baleanu developed a new operator which is based on generalized Mittag-Leffler function where the kernel is non-singular and non-local kernel [29]. Numerous studies on non-integer order models in the sense Atangana and Baleanu have been carried out by researchers. For instance, Atangana and Koca in [30] studied chaos in a simple nonlinear system using Atangana-Baleanu derivatives in Caputo sense. Atangana and Owolabi in [31] developed a new numerical approach for fractional differential equations for solving the system of fractional derivative equations which proved to be efficient. Atangana and Gomez [32] presented a detailed analysis of the decolonization of fractional calculus rules: breaking commutativity and associativity to capture more natural phenomena. Khan et al. [33] studied the TB dynamics under the framework of the work of Atangana-Baleanu derivative with relapse cases. Bonyah in [34] studied a new 5-D hyperchaotic system with four wings by Atangana-Baleanu operator. Khan and Atangana [35] studied the Ebola disease with different fractional operators. The comparison of Atangana-Baleanu derivative with Caputo-Fabrizio for the dengue model are studied in [36]. Bank data through fractional modeling and their analysis is studied in [37]. The dynamics of the chaotic system through Caputo-Fabrizio and the Atangana-Baleanu derivative is studied in [38]. The authors studied in [39] through different fractional operators of fractional calculus for bank data. Using the Atangana-Baleanu derivative the authors studied the TB dynamics [40]. The previously defined fractional operators have some definiens which may or may not correctly investigate the dynamics of such mathematical models that come from science and engineering fields. The Caputo derivative has a singular and local kernel which may not exactly studied the dynamics of a particular problem but these operators are still well known to the researchers and uses for the modeling dynamics. The Caputo-Fabrizio operator was defined without singular kernel. Many researchers used this operator for modeling purposes and still popular among researchers. To have a more precise definition of fractional operator a new operator called Atangana-Baleanu derivative was defined which has a non-singular and non-local kernel and has been used widely for many problems of science and engineering and found it interesting. This new definition is now much famous among researchers around the world. Here, in this work, we consider this newly operator to formulate a new mathematical model for TB dynamics in which we consider the adults and the children as a two groups and present to discuss deeply its mathematical as well as numerical results. This is the first attempt of the authors to have a new mathematical that exploring the children and adults TB dynamics.
It is well known that integer order model does not describes well the dynamics of the disease because there involve history of the disease and their memory. The fractional order models well address the memory effect involve in the disease. At each value of the fractional order parameters we can have a solution but in integer order model we don't have except at the integer case. The difference between two points can be effectively estimated through fractional order models. It is also well known that fractional order models are good for data fitting, where we have variety of choice's for the fractional order parameter. The Caputo operator is singular and local while the AB operator is non-singular and nonlocal. The cross over behavior in the TB model can be addressed well through the AB derivative. Therefore, we considered both the operators and its comparison through graphical results.
To date, there has not been anyone who has investigated the dynamics of TB model with children and adults population using Caputo and Atangana-Baleanu derivative. So, this study investigates a fractional order model in the Atangana-Baleanu sense to explore the spread of TB diseases with in children and adults population. We classify the population into children and adult classes. Based on the risk of transmission, most TB in children is usually not contagious compared to TB in adults [8,41]. Hence, the TB model that involves two-age-classes that distinguishes children and adults can indicate a new understanding of TB transmission.
This paper begins by mathematical preliminaries of the fractional derivative. The description of the model with the two-age-class transmission is given in Section 3. The TB model in Caputo derivative with basic properties, calculation of the basic reproduction number, and the numerical result are done in Section 4. The expression of the TB model in Atangana–Baleanu derivative with the existence and uniqueness of the solution, the numerical iterative, and also the numerical simulation are presented in Section 5. The comparison results of both derivatives with various fractional order parameters are shown in Section 6. Finally, the conclusion is shown in Section 7.
We present the details of the fractional operators in the following sections that should be used later in the proposed study.
Definition 2.1. (see [16]) For α>0, with n−1<α<n,n∈N, the fractional derivative in the sense of Caputo is defined to be:
CDαtf(t)=dαf(t)dtα:=1Γ(n−α)∫t0(t−s)(n−α−1)f(n)(s)ds, | (2.1) |
where the symbol Γ(⋅) denotes the gamma function.
The Riemann-Liouville fractional integral is defined by
Iαtf(t)=1Γ(α)∫t0(t−s)(α−1)f(s)ds. | (2.2) |
Definition 2.2. (see [29]) Suppose f∈H1(0,b), b>0, α∈[0,1], then the Atangana-Baleanu fractional operator in Caputo sense (ABC) can be written as follows:
ABC0Dαt(f(t))=B(α)1−α∫t0f′(s)Eα[−α(t−s)α1−α]ds, | (2.3) |
where B(α)=1−α+αΓ(α) is a normalized function with B(0)=B(1)=1 and Eα is Mittag-Leffler function
Eα(z)=∞∑k=0(z)kΓ(αk+1),α>0. |
The integral associated to the ABC derivative in Riemann-Liouville sense is given by,
Iαt(f(t))=1−αB(α)f(t)+αB(α)Γ(α)∫t0f(s)(t−s)α−1ds. | (2.4) |
The present section describes the formulation of the mathematical model of TB transmission with two groups of human that is, the children and the adults. We design the model by splitting the population into children (C) and the adults class (A). Furthermore, each of the classes is divided into three classes, namely, the susceptible classes (SC, SA), the latent TB classes (LC, LA), and the active TB classes (IC, IA), where SC, LC and IC represent the susceptible, latent and the active population at any time t respectively, whereas the adults sub-population are respectively shown by SA, LA and IA. Hence, the total population is N=SC+LC+IC+SA+LA+IA. Individuals who are in the latent stage are not infectious and are thus unable to transmit bacteria. We also assumed that the natural death rate of the children and adults population is equal as we consider the average natural death rate of the total population.
The majority of the children with tuberculosis are not infectious to others [8,41]. Hence, we assume that only the active TB adults could spread TB with in the population. In this model, it is assumed that children got TB infection by doing contacts with active TB adults. Therefore, the latent TB and active TB children do not spread TB within the population. A small percentage of newly infected individuals from the sub-population is assumed to undergo fast progress directly into the class of active TB, while the rest are latently infected and enter the latent class [42]. Furthermore, the TB patients both children and adults cannot recover completely but will enter the latent stage again. Considering these assumptions and facts the following system of evolutionary dynamics of the TB individuals is described through the following systems where the description of the parameters flows and the variables are given in Figure 1.
dSCdt=Λ−βCIASC−(g+μ)SC,dLCdt=βC(1−pC)IASC+γCIC−kC(1−rC)LC−(g+μ)LC,dICdt=βCpCIASC+kC(1−rC)LC−γCIC−(g+μ+dC)IC,dSAdt=gSC−βAIASA−μSA,dLAdt=(1−pA)βAIASA+gLC+γAIA−kA(1−rA)LA−μLA,dIAdt=pAβAIASA+kA(1−rA)LA+gIC−(γA+μ+dA)IA, | (3.1) |
where the initial values for the model variables are given by
SC(0)=SC0,LC(0)=LC0,IC(0)=IC0,SA(0)=SA0,LA(0)=LA0,andIA(0)=IA0. |
In the above model (3.1), the population of healthy people is generated by the birth rate Λ and the growing up rate of children is shown by g. The natural death rate of children and adults is denoted by μ. The effective contact rate for both the children and adults is shown by βC and βA. The proportion of fast progression of latent class children to infected class children is given by pC while for adults from latent adult class to infected adult class is by pA. The rate of progression from latent to infected class for the children compartment is shown by kC while for the adults infection progression from latent to infected class is given by kA. The rate of effective therapy for children and adults are shown respectively by γC and γA. The rate of effective chemoprophylaxis for children and adults are shown by rC and rA respectively. Natural death from TB disease in children infected class is given by dC while for the adults with TB infection by dA. The description of the parameters for the TB model is set out in Table 1.
Description | Parameter | |
Populations growth rate | Λ | |
Growing-up rate for children | g | |
Natural death rate | μ | |
Children | Adult | |
population | population | |
Infection rate | βC | βA |
Proportion of fast progression | pC | pA |
Rate of progression from latent to the infectious | kC | kA |
Rate of effective therapy | γC | γA |
Rate of effective chemoprophylaxis | rC | rA |
TB death rate | dC | dA |
The model (3.1) has the biologically feasible region on Ω1 with
Ω1={(SC,LC,IC,SA,LA,IA)∈R6+:0≤N≤Λμ}, |
which is feasible biologically and all the results associated holds for it. It should be noted that the parameters involved in the model (3.1) are associated to humans so it is non-negative and the region shown by Ω1 is positive invariant for the described model (3.1) above. The region shown for the model (3.1) below is well-posed and the entire solutions for the initial values belonging to Ω1, remains in Ω1 for every time t≥0.
Next, in the following section, we explore the TB model in Caputo sense. We apply the definitions of Caputo operator described in above section, and apply it on our model (3.1) and present the results associated to it in the following section in details.
This section present a TB model with two groups of humans which is shown in (3.1), by applying the Caputo derivative on it. The fractional order model of TB transmission with two-age-class in the Caputo sense is given by:
CDαtSC=Λ−βCIASC−(g+μ)SC,CDαtLC=βC(1−pC)IASC+γCIC−kC(1−rC)LC−(g+μ)LC,CDαtIC=βCpCIASC+kC(1−rC)LC−γCIC−(g+μ+dC)IC,CDαtSA=gSC−βAIASA−μSA,CDαtLA=(1−pA)βAIASA+gLC+γAIA−kA(1−rA)LA−μLA,CDαtIA=pAβAIASA+kA(1−rA)LA+gIC−(γA+μ+dA)IA, | (4.1) |
where α represents the fractional order and 0<α≤1. The initial values for the model variables in fractional order model (4.1) are given by,
SC(0)=SC0,LC(0)=LC0,IC(0)=IC0,SA(0)=SA0,LA(0)=LA0,andIA(0)=IA0. |
This section deals with the positivity of the model solution in the Caputo derivative. We assume that the initial condition of the model (4.1) is non-negative. Let us consider
Ω2={x=(SC,LC,IC,SA,LA,IA)∈R6+:SC,LC,IC,SA,LA,IA≥0}. |
Here, recalling the important concept given in [43] to prove our result.
Lemma 4.1. (see [43]) Suppose that f(t)∈C[a,b] and CDαtf(t)∈C[a,b], for 0<α≤1, then we have
f(t)=f(a)+1Γ(α)CDαtf(ξ)(t−a)α |
with a≤ξ≤t, ∀t∈(a,b].
Corollary 4.2. Consider that f(t)∈C[a,b] and CDαtf(t)∈C[a,b], for 0<α≤1. Then if
(1) CDαtf(t)≥0,∀t∈(a,b), then f(t) is non-decreasing.
(2) CDαtf(t)≤0,∀t∈(a,b), then f(t) is non-increasing.
Now, we state the main theorem.
Theorem 4.3. A unique solution exists for x(t)=(SC,LC,IC,SA,LA,IA)T of the model (4.1) and the solution will be in Ω2. Additionally, the solution is non-negative.
Proof. Using Theorem 3.1. and Remark 3.2. of [44], it is easily to prove the solution existence and also the uniqueness of the solution of the model (4.1) for all t>0. Next, we will prove the positivity of the solution of the model (4.1). In order to do, we have to prove for every hyperplane bounding the nonnegative orthant, of the field points of the vector Ω2. It follows from the model given by (4.1), we have
CDαtSC∣SC=0=Λ≥0,CDαtLC∣LC=0=βC(1−pC)IASC+γCIC≥0,CDαtIC∣IC=0=βCpCIASC+kC(1−rC)LC≥0,CDαtSA∣SA=0=gSC≥0,CDαtLA∣LA=0=(1−pA)βAIASA+gLC+γAIA≥0,CDαtIA∣IA=0=kA(1−rA)LA+gIC≥0. |
By using Corollary 1, the model solution remains in Ω2.
Thus, it follows from the results described above the feasible given by Ω2 is positively invariant for model (4.1).
We present here the possible fixed points of the fractional model given by (4.1) and then, we obtain at these possible equilibrium points the stability analysis of the model. For the given model (4.1), there exists two possible equilibrium points that is, the disease free and the endemic equilibrium. We can obtain these equisetum points by setting the time rate of change equal to zero of the model (4.1) and is given by:
CDαtSC∣SC=0=0,CDαtLC∣LC=0=0,CDαtIC∣IC=0=0,CDαtSA∣SA=0=0,CDαtLA∣LA=0=0,CDαtIA∣IA=0=0. |
We have the following expression,
Λ−βCIASC−(g+μ)SC=0,βC(1−pC)IASC+γCIC−kC(1−rC)LC−(g+μ)LC=0,βCpCIASC+kC(1−rC)LC−γCIC−(g+μ+dC)IC=0,gSC−βAIASA−μSA=0,(1−pA)βAIASA+gLC+γAIA−kA(1−rA)LA−μLA=0,pAβAIASA+kA(1−rA)LA+gIC−(γA+μ+dA)IA=0. | (4.2) |
At the disease free equilibrium, denoted by E01, we have from (4.2),
E01=(Λg+μ,0,0,gΛμ(g+μ),0,0). |
The endemic equilibrium of the model (4.1) is given by E22=(S∗C,L∗C,I∗C,S∗A,L∗A,I∗A) where
S∗C=ΛI∗AβC+g+μL∗C=βC(1−pC)I∗AS∗C+γCI∗Cμ+g+kC(1−rC)I∗C=βCpCI∗AS∗C+kC(1−rC)L∗CγC+g+μ+dCS∗A=gS∗CβAI∗A+μL∗A=(1−pA)βAI∗AS∗A+gL∗C+γAI∗AkA(1−rA)+μI∗A=kA(1−rA)L∗A+gI∗CγA+μ+dA−βApAS∗A. |
The endemic equilibrium E22 exists if γA+μ+dA>βApAS∗A.
In order to find the basic reproduction number R0, we consider the method in [45] and the matrices are given by
F=(000P9000P10000P12000P13),V=(P2−γC00−P5P300−g0P4−rA0−g−P6P7). | (4.3) |
The basic reproduction number is the spectral radius of ρ(FV−1) and is given by
R0=R1+R2+R3+R4, | (4.4) |
where
R1=P6P3(gP9+P2P12)(P4P7−P6rA)(P2P3−P5γC),R2=P4P2(gP10+P3P13)(P4P7−P6rA)(P2P3−P5γC),R3=P6γC(gP10−P5P12)(P4P7−P6rA)(P2P3−P5γC),R4=P4P5(gP9−P13γC)(P4P7−P6rA)(P2P3−P5γC), | (4.5) |
where
P1=g+μ,P2=kC(1−rC)+g+μ,P3=γC+dC+g+μ,P4=kA(1−rA)+μ,P5=kC(1−rC),P6=kA(1−rA),P7=γA+dA+μ,P8=βCS0C,P9=βC(1−pC)S0c,P10=βCpCS0C,P11=βAS0A,P12=βA(1−pA)S0A,P13=βApAS0A, | (4.6) |
where S0C and S0A represent the disease-free equilibrium (DFE).
Next, we show the stability of the system at the disease-free case, we follow the following theorem.
Theorem 4.4. For any p1,p2∈Z, such that gcd(p1,p2)=1. Let α=(p1p2) and consider M=p2, then the DFE of the system (4.1) is locally asymptotically stable (LAS) if |arg(λ)|>π2M, for all roots λ of the characteristic Eq. (4.7) of the matrix JE01.
det(diag[λp1λp1λp1λp1λp1λp1]−JE01)=0. | (4.7) |
Proof. At the disease free equilibrium E01, the Jacobian matrix is given by
J(E01)=(−P10000−P80−P2γC00P90P5−P300P10g00−μ0−P110g00−P4P12+γA00g0P6P13−P7). | (4.8) |
We obtain the characteristics equation associated to J(E01), given by
(λp1+μ)(λp1+μ+g)[λ4p1+b1λ3p1+b2λ2p1+b3λp1+b4]=0. | (4.9) |
The arguments of the roots of the equation λp1+μ=0, λp1+μ+g=0 are as follow:
arg(Πk)=πp1+k2πp1>πM>π2M,wherek=0,1⋯,(p1−1). | (4.10) |
In Eq. (4.9), the roots are negative, i.e., −μ,−(μ+g), while the rest are computed from
Q(λ)=[λ4p1+b1λ3p1+b2λ2p1+b3λp1+b4], | (4.11) |
where
b1=γA+dA+kA(1−rA)−βApAS0A+γC+dC+kC(1−rC)+2g+4μ,b2=(γC+dC+g+μ)(γA+dA+kA(1−rA)+2μ)−βApAS0AP2P4⏟+(kA(1−rA)+μ)(kC(1−rC)+g+μ)+(dA+μ)(kA(1−rA)+μ)+μγA+P7P2−βAkA(1−pA)(1−rA)S0A⏟+((dC+g+μ)(kC(1−rC)+g+μ)+γC(g+μ))×(1−βApAS0A(γC+dC+g+μ)+gβCpCS0C(dC+g+μ)(kC(1−rC)+g+μ)+γC(g+μ))⏟,b3=(P4+P7−P13)(dC(kC(1−rC)+g+μ)+(g+μ)(γC+kC(1−rC)+g+μ))(P3+P2)(dA(kA(1−rA)+μ)+kA(1−rA)(μ−βAS0A)+μ(γA−βApAS0A+μ))−gβCS0C(kA(1−rA)+pC(g+2μ)+kC(1−rC)),b4=C1C2(1−R0), | (4.12) |
where C1=(dA(kA(1−rA)+μ)+μ(γA+kA(1−rA)+μ)),
C2=(dC(kC(1−rC)+g+μ)+(g+μ)(γC+kC(1−rC)+g+μ)).
The function Q(λ) will give eigenvalues with negative real part if bi>0 for i=1,2,3,4 and b1b2b3>b21b4+b23. All bi>0 when R0<1 and the conditions b1b2b3>b21b4+b23 ensure the stability of the disease free case when R0<1. If R0<1, then the necessary condition fulfil for all the roots of characteristics equation i.e., |arg(λ)|>π2M. Thus the DFE is LAS for R0<1.
The following subsection explore the numerical simulation of the Caputo model.
The aim of this section is to discuss a numerical simulation of the model (4.1). The simulation is done with several fractional order values to determine population dynamics in the model. The numerical results of the Caputo derivative are obtained by the Predictor-Corrector method [46,47]. Parameters values used in these simulations are set out in Table 2. We take the initial condition SC(0)=1500,LC(0)=135,IC(0)=75,SA(0)=1000,LA(0)=103 and IA(0)=60. Here, we use 50 years for the time horizon. The simulations are conducted with different values of the order of the fractional derivative α=1,0.9,0.8,0.7,0.6 and 0.5. We have the simulation results of the TB model (4.1) in Figure 2. In this case the value of R0 is R0=91.5021>1, which indicates the disease will persist in the population. We can observe in Figure 2 that as the values of α decrease, the individuals infected with TB decreases whereas the population of susceptible individuals increases.
Parameter | Value | Ref. |
Λ | 1000 | Assumed |
βA | 0.02 | [48] |
g | 114 | [50] |
γA | 0.5 | Assumed |
pA | 0.3 | Assumed |
kA | 0.005 | [49] |
rA | 0.5 | Assumed |
dA | 0.05751 | [51] |
p | 0.3 | Assumed |
γ | 0.5 | Assumed |
r | 0.5 | Assumed |
βC | 0.01 | [48] |
μ | 0.0143 | [49] |
γC | 0.5 | Assumed |
pC | 0.4 | Assumed |
kC | 0.005 | [49] |
rC | 0.5 | Assumed |
dC | 0.0575 | [51] |
d | 0.05751 | [51] |
β | 0.02 | [48] |
k | 0.005 | [49] |
Next, we will see the effect of chemoprophylaxis and treatment on the active TB populations in both children and adults. The numerical simulation is deployed by varying the effectiveness of chemoprophylaxis (rC,rA) and treatment (γC,γA). In Figures 3, 4, we display the dynamic of the active TB populations for different values of the efficacy of chemoprophylaxis and treatment. For α=0.9, it is shown that the population of the active TB both children and adults have decreased when the efficacy of chemoprophylaxis and treatment have increased. Conversely, when the efficacy of chemoprophylaxis and treatment falls, the population of the active TB both children and adults will rise. The likewise behavior is seen for α=0.7. This shows that chemoprophylaxis and treatment were given to patients with latent stage and active TB respectively can reduce the number of TB infection populations of both children and adults.
Next, we express the model of TB in Atangana-Baleanu derivative where their kernel is non-singular and non-local which best describes the dynamics of real world problem.
The newly derivative called the Atangana-Baleanu derivative where their kernel is non-local and non singular is considered here to apply on our model presented in (3.1). We follow the definition described above and have the following representation:
ABC0DαtSC=Λ−βCIASC−(g+μ)SC,ABC0DαtLC=βC(1−pC)IASC+γCIC−kC(1−rC)LC−(g+μ)LC,ABC0DαtIC=βCpCIASC+kC(1−rC)LC−γCIC−(g+μ+dC)IC,ABC0DαtSA=gSC−βAIASA−μSA,ABC0DαtLA=(1−pA)βAIASA+gLC+γAIA−kA(1−rA)LA−μLA,ABC0DαtIA=pAβAIASA+kA(1−rA)LA+gIC−(γA+μ+dA)IA, | (5.1) |
with some appropriate initial conditions. For the TB fractional model in Atangana-Baleanu form is studied further to obtain their existence and uniqueness. We have the following:
Here, we display the uniqueness and existence results associated to the AB model (5.1). In order to show the results for the model we follow the results of the fixed-point theory. We first write the (5.1) in the form shown below,
{ABC0Dαtu(t)=G(t,u(t))u(0)=u0,0<t<T<∞. | (5.2) |
In system (5.2), u(t)=(SC,LC,IC,SA,LA,IA) is simply a vector having the state variable and G is therefore a continuous vector function given as
G=(G1G2G3G4G5G6)=(Λ−βCIASC−(g+μ)SCβC(1−pC)IASC+γCIC−kC(1−rC)LC−(g+μ)LCβCpCIASC+kC(1−rC)LC−γCIC−(g+μ+dC)ICgSC−βAIASA−μSA(1−pA)βAIASA+gLC+γAIA−kA(1−rA)LA−μLApAβAIASA+kA(1−rA)LA+gIC−(γA+μ+dA)IA) |
and u0(t)=(SC(0),LC(0),IC(0),SA(0),LA(0),IA(0)) representing the state variables initial conditions in vector form. The above function namely, G satisfies the condition of Lipschitz continuity and can be described as below:
‖G(t,u1(t))−G(t,u2(t))‖≤M‖u1(t)−u2(t)‖. | (5.3) |
The following result is provided in order to show the model existence and their solution as well as uniqueness for the model (5.1).
Theorem 5.1. (Existence and uniqueness) The model given by (5.2) has the unique solution provided that the following condition satisfy,
(1−α)ABC(α)M+αABC(α)Γ(α)TαmaxM<1. | (5.4) |
Proof. To prove the above statement, we need to use the fractional integral associated to the Atangana-Baleanu model given by (2.4). The application of this integral on (5.2), leads to the following non-linear voltera integral equation:
u(t)=u0+(1−α)ABC(α)G(t,u(t))+αABC(α)Γ(α)∫t0(t−ε)α−1G(ε,u(ε))dε. | (5.5) |
We assure that J=(0,T) and take into consideration the operator ϕ:C(J,R6)→C(J,R6) defined by
ϕ[u(t)]=u0+(1−α)ABC(α)G(t,u(t))+αABC(α)Γ(α)∫t0(t−ε)α−1G(ε,u(ε))dε. | (5.6) |
Equation (5.5) turns to
u(t)=ϕ[u(t)]. | (5.7) |
The supremum norm on J, ‖.‖J is ‖u(t)‖J=supt∈J‖u(t)‖,u(t)∈C.
Obviously, C(J,R6) along the norm ‖.‖J present a Banach space. Additionally, we can demonstrate simply the following inequality
‖∫t0D(t,ε)u(ε)dε‖≤T‖D(t,ε)‖J‖u(t)‖J. | (5.8) |
with u(t)∈C(J,R6),D(t,ε)∈C(J2,R).
In a way that
‖D(t,ε)‖J=supt,ε∈J|D(t,ε)|. |
Applying the definition of ϕ stated in (5.7), we derive
‖ϕ[u1(t)]−ϕ[u2(t)]‖J≤‖(1−α)ABC(α)G(t,u1(t))−G(t,u2(t))+αABC(α)T(α)×∫t0(t−ε)α−1(G(ε,u1(ε))−G(ε,u2(ε)))dε‖. |
Furthermore, using the principles of triangular inequality and Lipschitz condition stated in (5.3) couple with the results in (5.8) we get the following after some algebraic simplification.
‖ϕ[u1(t)]−ϕ[u2(t)]‖J≤((1−α)ABC(α)M+αABC(α)Γ(α)MTαmax)‖u1(t)−u2(t)‖J. |
Thus, we eventually have
‖ϕ[u1(t)]−ϕ[u2(t)]‖J≤β‖u1(t)−u2(t)‖J, |
where
β=(1−α)ABC(α)M+αABC(α)Γ(α)MTαmax. |
Therefore, the operator ϕ will become a contraction if condition (5.4) holds on C(J,R6). As a result of Banach fixed point theorem, system (5.2) possesses a unique solution.
This section derive the numerical scheme of the TB model in the ABC form which given by Eq. (5.1) using the method in [52] by using Adams-Bashforth method. By using the same procedure in [52], we write (5.1) to the following
ABC0DαtSC=G1(t,SC,LC,IC,SA,LA,IA)ABC0DαtLC=G2(t,SC,LC,IC,SA,LA,IA),ABC0DαtIC=G3(t,SC,LC,IC,SA,LA,IA),ABC0DαtSA=G4(t,SC,LC,IC,SA,LA,IA),ABC0DαtLA=G5(t,SC,LC,IC,SA,LA,IA),ABC0DαtIA=G6(t,SC,LC,IC,SA,LA,IA). | (5.9) |
By utilizing the fundamental theorem of fractional calculus, we convert (5.9) to fractional
SC(t)−SC(0)=(1−α)ABC(α)G1(t,SC)+αABC(α)Γ(α)∫t0(t−ε)α−1G1(ε,SC)dε,LC(t)−LC(0)=(1−α)ABC(α)G2(t,LC)+αABC(α)Γ(α)∫t0(t−ε)α−1G2(ε,LC)dε,IC(t)−IC(0)=(1−α)ABC(α)G3(t,IC)+αABC(α)Γ(α)∫t0(t−ε)α−1G3(ε,IC)dε,SA(t)−SA(0)=(1−α)ABC(α)G4(t,SA)+αABC(α)Γ(α)∫t0(t−ε)α−1G4(ε,SA)dε,LA(t)−LA(0)=(1−α)ABC(α)G5(t,LC)+αABC(α)Γ(α)∫t0(t−ε)α−1G5(ε,LA)dε,IA(t)−IA(0)=(1−α)ABC(α)G6(t,IA)+αABC(α)Γ(α)∫t0(t−ε)α−1G6(ε,IA)dε. | (5.10) |
At t=tn+1,n=0,1,2,..., we have
SC(tn+1)−SC(0)=(1−α)ABC(α)G1(tn,SC)+αABC(α)Γ(α)n∑j=0∫tj+1tj(tn+1−ε)α−1G1(ε,SC)dε,LC(tn+1)−LC(0)=(1−α)ABC(α)G2(tn,LC)+αABC(α)Γ(α)n∑j=0∫tj+1tj(tn+1−ε)α−1G2(ε,LC)dε,IC(tn+1)−IC(0)=(1−α)ABC(α)G3(tn,IC)+αABC(α)Γ(α)n∑j=0∫tj+1tj(tn+1−ε)α−1G3(ε,IC)dε,SA(tn+1)−SA(0)=(1−α)ABC(α)G4(tn,SA)+αABC(α)Γ(α)n∑j=0∫tj+1tj(tn+1−ε)α−1G4(ε,SA)dε,LA(tn+1)−LA(0)=(1−α)ABC(α)G5(tn,LA)+αABC(α)Γ(α)n∑j=0∫tj+1tj(tn+1−ε)α−1G5(ε,LA)dε,IA(tn+1)−IA(0)=(1−α)ABC(α)G6(tn,IA)+αABC(α)Γ(α)n∑j=0∫tj+1tj(tn+1−ε)α−1G6(ε,IA)dε. | (5.11) |
The integral in Eq. (5.11) is approximated through the two-point interpolation polynomial. Hence, we have the iterative scheme for the TB model (5.1). After some calculations, we finally obtain the approximation solution as
SC(tn+1)=SC(0)+(1−α)ABC(α)G1(tn,SC)+αABC(α)n∑j=0(hαG1(tj,SC)Γ(α+2)((n+1−j)α(n−j+2+α)−(n−j)α(n−j+2+2α))−hαG1(tj−1,SC)Γ(α+2)((n+1−j)α+1−(n−j)α(n−j+1+α))), |
LC(tn+1)=LC(0)+(1−α)ABC(α)G2(tn,LC)+αABC(α)n∑j=0(hαG2(tj,LC)Γ(α+2)((n+1−j)α(n−j+2+α)−(n−j)α(n−j+2+2α))−hαG2(tj−1,LC)Γ(α+2)((n+1−j)α+1−(n−j)α(n−j+1+α))), |
IC(tn+1)=IC(0)+(1−α)ABC(α)G3(tn,IC)+αABC(α)n∑j=0(hαG3(tj,IC)Γ(α+2)((n+1−j)α(n−j+2+α)−(n−j)α(n−j+2+2α))−hαG3(tj−1,IC)Γ(α+2)((n+1−j)α+1−(n−j)α(n−j+1+α))), |
SA(tn+1)=SA(0)+(1−α)ABC(α)G4(tn,SA)+αABC(α)n∑j=0(hαG4(tj,SA)Γ(α+2)((n+1−j)α(n−j+2+α)−(n−j)α(n−j+2+2α))−hαG4(tj−1,SA)Γ(α+2)((n+1−j)α+1−(n−j)α(n−j+1+α))), |
LA(tn+1)=LA(0)+(1−α)ABC(α)G5(tn,LA)+αABC(α)n∑j=0(hαG5(tj,LA)Γ(α+2)((n+1−j)α(n−j+2+α)−(n−j)α(n−j+2+2α))−hαG5(tj−1,LA)Γ(α+2)((n+1−j)α+1−(n−j)α(n−j+1+α))), |
IA(tn+1)=IA(0)+(1−α)ABC(α)G6(tn,IA)+αABC(α)n∑j=0(hαG6(tj,IA)Γ(α+2)((n+1−j)α(n−j+2+α)−(n−j)α(n−j+2+2α))−hαG6(tj−1,IA)Γ(α+2)((n+1−j)α+1−(n−j)α(n−j+1+α))). |
Next, we present the simulation result of the TB model (5.1) using the numerical scheme.
This section provides the simulation results of Atangana-Baleanu model (5.1) using the iterative scheme obtained in (5.12). The parameter values listed in Table 2 are for obtaining the numerical results 2. The simulation results are shown in Figure 5 by considering different values of α=1,0.9,0.8,0.7 and 0.6. In Figure 5, we can see that by decreasing the values of α, the population of infected compartments decreases, while the susceptible population increase.
In Figures 6 and 7, we present the effect of the efficacy of chemoprophylaxis (rC and rA) and treatment (γC,γA) on the TB transmission for different values of α. From Figure 6, it can be seen that as the chemoprophylaxis strategy increase, the infected TB in both children and adults population decrease. From Figure 7, it is shown that as the treatment strategy increase, the infected TB in both children and adults population decrease significantly.
This present section demonstrates the comparison of the Caputo and Atangana-Baleanu operators graphically. The parameter values used in these simulations are given in Table 2. In order to have a comparison of these two operators we considered many values of α=1,0.7,0.5,0.3 and the results were displayed Figures 8–11. It can be seen in Figures 8–11 that there exists a significant difference in the dynamical behavior of the TB infected population when the values of α decrease within both fractional operators. From these figures, we see that Atangana-Baleanu derivative give faster decreases in the results compare to the Caputo derivative when the fractional order parameter decrease for the reduction of TB infection. This comparison section demonstrate the effectiveness of the Atangana-Baleanu derivative over the Caputo derivative.
The findings reported here shed new light on the fractional-order model with Caputo and Atangana-Baleanu derivative to investigate the spread of TB with children and adults population. Brief mathematical results for the TB model are investigated. The basic reproduction number of the Caputo model and its equilibrium are obtained and presented the results. Each model is studied independently in the fractional operators of Caputo and Atangana-Baleanu. We then performed the numerical simulations of the Caputo model type for different values of the fractional-order using the predictor-corrector method. Then, we apply the fractional Atangana-Baleanu operator to the model and analyzed and obtained the associated results. We proved the uniqueness and existence of the Atangana-Baleanu model. The numerical simulation of the Atangana-Baleanu model was conducted using the Adams–Bashforth scheme. We further presented the graphical results of Atangana-Baleanu model for various fractional order parameters. A comparison of the proposed operators graphically presented. These graphical results for comparison show that the Atangana-Baleanu results are more appropriate for the better decrease in infection while the Caputo is less. We conclude from graphical results that increasing chemoprophylaxis and treatment rates then the TB infective both children and adult cases can be reduced. The graphical results show that a decrease in fractional order parameter causes a significant decrease in the infective TB population. Therefore, the present work is a novel analysis on the TB dynamics and will be more useful for the readers and public health authorities. In future work the TB age fractional model can be considered in the light of fractional impulse model and the results can be compared with non-impulse TB model, stated in [53,54,55]. This is important because the impulse effect is attracting the receiving considerable attention and eliciting widespread interest in epidemiological models.
Part of this research is financially supported by Unversitas Airlangga 2018.
All authors declare no conflict of interest.
[1] |
D. Razavi-Shearer, I. Gamkrelidze, M. H. Nguyen, D. S. Chen, P. Van Damme, Z. Abbas, et al., Global prevalence, treatment, and prevention of hepatitis B virus infection in 2016: a modelling study, Lancet Gastroenterol., 3 (2018), 383–403. https://doi.org/10.1016/S2468-1253(18)30056-6 doi: 10.1016/S2468-1253(18)30056-6
![]() |
[2] |
N. Nelson, P. Easterbrook, B. Mcmahon, Epidemiology of hepatitis b virus infection and impact of vaccination on disease, Clin. Liver Dis., 20 (2016), 607–628. https://doi.org/10.1016/j.cld.2016.06.006 doi: 10.1016/j.cld.2016.06.006
![]() |
[3] | WHO, Hepatitis B fact sheets, Available from: http://www.who.int/news-room/fact-sheets/detail/hepatitis-b. (accessed July 4, 2023) |
[4] |
J. Shen, N. Zhang, Change-point model on nonhomogeneous poisson processes with application in copy number profiling by next-generation DNA sequencing, Ann. Appl. Stat., 6 (2012), 476–496. https://doi.org/10.1214/11-AOAS517 doi: 10.1214/11-AOAS517
![]() |
[5] |
V. Muggeo, G. Adelfio, Efficient change point detection for genomic sequences of continuous measurements, Bioinformatics, 2 (2011), 27. https://doi.org/10.1093/bioinformatics/btq647 doi: 10.1093/bioinformatics/btq647
![]() |
[6] | G. Teyssire, A. Kirman, Long memory in economics, Springer Berlin Heidelberg, 2007. https://doi.org/10.1007/978-3-540-34625-8 |
[7] |
X. Cao, J. Wang, J. Liao, Z. Gao, D. Jiang, J. Sun, et al., EBacterioplankton community responses to key environmental variables in plateau freshwater lake ecosystems: A structural equation modeling and change point analysis, Sci. Total Environ., 580 (2017), 457–467. https://doi.org/10.1016/j.scitotenv.2016.11.143 doi: 10.1016/j.scitotenv.2016.11.143
![]() |
[8] | K. Shi, Y. Touge, Identifying the shift in global wildfire weather conditions over the past four decades: An analysis based on change-points and long-term trends, Geosci. Lett., 10 (2023). https://doi.org/10.1186/s40562-022-00255-6 |
[9] | G. Texier, M. Farouh, L. Pellegrin, M. Jackson, J. Meynard, X. Deparis, et al., Outbreak definition by change point analysis: A tool for public health decision?, BMC Med. Inform. Decis. Mak., 16 (2016). https://doi.org/10.1186/s12911-016-0271-x |
[10] |
X. Chen, H. Wang, W. Lyu, R. Xu, The mann-kendall-sneyers test to identify the change points of covid-19 time series in the united states, BMC Med. Res. Methodol., 22 (2022), 1–9. https://doi.org/10.1186/s12874-022-01714-6 doi: 10.1186/s12874-022-01714-6
![]() |
[11] |
V. Punyapornwithaya, Lumpy skin disease outbreaks in africa, europe, and asia (2005–2022): Multiple change point analysis and time series forecast, Viruses, 14 (2022), 2203. https://doi.org/10.3390/v14102203 doi: 10.3390/v14102203
![]() |
[12] |
H. Mann, Nonparametric tests against trend, Econometrica, 13 (1945), 245–259. https://doi.org/10.2307/1907187 doi: 10.2307/1907187
![]() |
[13] | M. Kendall, Rank Correlation Methods, Br. J. Psychol., 25 (1990), 86–91. |
[14] | R. Sneyers, On the Statistical Analysis of Series of Observations, J. Biol. Chem., 258 (1990), 13680–13684. |
[15] |
Q. Zhang, C. Xu, S. Becker, T. Jiang, Sediment and runoff changes in the Yangtze River basin during past 50 years, J. Hydrol., 331 (2006), 511–523. https://doi.org/10.1016/j.jhydrol.2006.05.036 doi: 10.1016/j.jhydrol.2006.05.036
![]() |
[16] | M. Kendall M, A. Stuart, The advanced theory of statistics. Vol.3: Design and analysis, and time-series, J. R. Stat. Soc., 147 (1983). https://doi.org/10.2307/2988093 |
[17] | D. R. Helsel, R. M. Hirsch, K. R. Ryberg, S. A. Archfield, E. J. Gilroy, Statistical Methods in Water Resources, U.S. Geological Survey Techniques and Methods, book 4, chap. A3,458p, 2020. https://doi.org/10.3133/tm4a3 |
[18] | C. Fu, Q. Wang, The Definition and Detection of the Abrupt Climatic Change, Chin. J. Atmos. Sci., 04 (1992), 482–493. |
[19] | J. Searcy, C. Hardison, Double mass curves. Geological Survey Water Supply Paper 1541-B, U.S. Geological Survey, Washington., 1960. |
[20] | S. Wglarczyk, On the stationarity of extreme levels of some Polish lakes. I. Preliminary results from statistical test, Limnol. Rev., 9 (2009), 129–138. |
[21] |
H. Cho, P. Fryzlewicz, Multiple-change-point detection for high dimensional time series via sparsified binary segmentation, J. R. Stat. Soc., 77 (2015), 475–507. https://doi.org/10.1111/rssb.12079 doi: 10.1111/rssb.12079
![]() |
[22] | V. Muggeo, G. Sottile, M. Porcu, Modelling COVID-19 outbreak: Segmented regression to assess lockdown effectiveness, Research Gate, 10 (2020). |
[23] |
R. Killick R, P. Fearnhead, I. Eckley, Optimal detection of changepoints with a linear computational cost, J. Am. Stat. Assoc., 107 (2012), 1590–1598. https://doi.org/10.1080/01621459.2012.737745 doi: 10.1080/01621459.2012.737745
![]() |
[24] |
R. Killick, I. Eckley, Changepoint: An r package for changepoint analysis, J. Stat. Softw., 58 (2015), 1–19. https://doi.org/10.18637/jss.v058.i03 doi: 10.18637/jss.v058.i03
![]() |
[25] | V. Muggeo, Segmented: An R Package to Fit Regression Models With Broken-Line Relationships, Rnews., 8 (2008), 20–25. |
[26] | V. Muggeo, Regression Models with Breakpoints/Changepoints Estimation, 2015. |
[27] |
A. Hardi, K. Kawai, S. Lee, K. Maekawa, Change Point Analysis of Exchange Rates Using Bootstrapping Methods: An Application to the Indonesian Rupiah 2000–2008, Asia-Pacific Financ. Mark., 22 (2015), 429–444. https://doi.org/10.1007/s10690-015-9206-2 doi: 10.1007/s10690-015-9206-2
![]() |
[28] |
J. Lin, J. Chen, Y. Li, Bayesian Analysis of Student t Linear Regression with Unknown Change-Point and Application to Stock Data Analysis, Comput. Econ., 40 (2012), 203–217. https://doi.org/10.1007/s10614-011-9305-8 doi: 10.1007/s10614-011-9305-8
![]() |
[29] |
O. Seidou, J. Asselin, T. Ouarda, Bayesian multivariate linear regression with application to change point models in hydrometeorological variables, Water Resour. Res., 43 (2007), 387–390. https://doi.org/10.1029/2005WR004835 doi: 10.1029/2005WR004835
![]() |
[30] |
H. Zhang, J. Stephen, J. Carter, Change Point Detection and Trend Analysis for Time Series, Chin. J. Chem. Phys., 35 (2022), 399–406. https://doi.org/10.1063/1674-0068/cjcp2112266 doi: 10.1063/1674-0068/cjcp2112266
![]() |
[31] |
X. Wu, X. Zhou, Y. Chen, K. Zhai, R. Sun, G. Luo, et al., The Impact of COVID-19 Lockdown on Cases of and Deaths From AIDS, Gonorrhea, Syphilis, Hepatitis B, and Hepatitis C: Interrupted Time Series Analysis, JMIR Public Health Surveill., 9 (2023), 399–406. https://doi.org/10.2196/40591 doi: 10.2196/40591
![]() |
[32] |
M. Xiridou, P. Adam, A. Meiberg, M. Visser, A. Matser, J.de Wit, et al., The impact of the COVID-19 pandemic on hepatitis B virus vaccination and transmission among men who have sex with men: A mathematical modelling study, Vaccine., 40 (2022), 4889–4896. https://doi.org/10.1016/j.vaccine.2022.06.075 doi: 10.1016/j.vaccine.2022.06.075
![]() |
1. | Lili Han, Qiuhui Pan, Baolin Kang, Mingfeng He, Effects of masks on the transmission of infectious diseases, 2021, 2021, 1687-1847, 10.1186/s13662-021-03321-z | |
2. | Ibrahim M. Hezam, Abdelaziz Foul, Adel Alrasheedi, A dynamic optimal control model for COVID-19 and cholera co-infection in Yemen, 2021, 2021, 1687-1847, 10.1186/s13662-021-03271-6 | |
3. | Sunil Kumar, R.P. Chauhan, Abdel-Haleem Abdel-Aty, M.R. Alharthi, A study on transmission dynamics of HIV/AIDS model through fractional operators, 2021, 22, 22113797, 103855, 10.1016/j.rinp.2021.103855 | |
4. | A. Babaei, M. Ahmadi, H. Jafari, A. Liya, A mathematical model to examine the effect of quarantine on the spread of coronavirus, 2021, 142, 09600779, 110418, 10.1016/j.chaos.2020.110418 | |
5. | A Sunarto, J Sulaiman, J V L Chew, Performance of FSPAOR iteration for solving one-dimensional space-fractional diffusion equation, 2021, 1803, 1742-6588, 012004, 10.1088/1742-6596/1803/1/012004 | |
6. | Fehaid Salem Alshammari, Muhammad Altaf Khan, Dynamic behaviors of a modified SIR model with nonlinear incidence and recovery rates, 2021, 60, 11100168, 2997, 10.1016/j.aej.2021.01.023 | |
7. | Nadia Gul, Rubi Bilal, Ebrahem A. Algehyne, Maryam G. Alshehri, Muhammad Altaf Khan, Yu-Ming Chu, Saeed Islam, The dynamics of fractional order Hepatitis B virus model with asymptomatic carriers, 2021, 60, 11100168, 3945, 10.1016/j.aej.2021.02.057 | |
8. | Ernestine Atangana, Paul J. Oberholster, Anthony R. Turton, Will the extraction of COVID-19 from wastewater help flatten the curve?, 2021, 271, 00456535, 129429, 10.1016/j.chemosphere.2020.129429 | |
9. | Fatma Bozkurt, Ali Yousef, Thabet Abdeljawad, Analysis of the outbreak of the novel coronavirus COVID-19 dynamic model with control mechanisms, 2020, 19, 22113797, 103586, 10.1016/j.rinp.2020.103586 | |
10. | Yacine El hadj Moussa, Ahmed Boudaoui, Saif Ullah, Fatma Bozkurt, Thabet Abdeljawad, Manar A. Alqudah, Stability analysis and simulation of the novel Corornavirus mathematical model via the Caputo fractional-order derivative: A case study of Algeria, 2021, 26, 22113797, 104324, 10.1016/j.rinp.2021.104324 | |
11. | Xiao-Hong Zhang, Aatif Ali, Muhammad Altaf Khan, Mohammad Y. Alshahrani, Taseer Muhammad, Saeed Islam, Juan Luis García Guirao, Mathematical Analysis of the TB Model with Treatment via Caputo-Type Fractional Derivative, 2021, 2021, 1607-887X, 1, 10.1155/2021/9512371 | |
12. | Mati Ur Rahman, Muhammad Arfan, Zahir Shah, Poom Kumam, Meshal Shutaywi, Nonlinear fractional mathematical model of tuberculosis (TB) disease with incomplete treatment under Atangana-Baleanu derivative, 2021, 60, 11100168, 2845, 10.1016/j.aej.2021.01.015 | |
13. | Tanvi A, Rajiv Aggarwal, Yashi A. Raj, A fractional order HIV-TB co-infection model in the presence of exogenous reinfection and recurrent TB, 2021, 104, 0924-090X, 4701, 10.1007/s11071-021-06518-9 | |
14. | Mohammad Partohaghighi, Ali Akgül, Rubayyi T. Alqahtani, New Type Modelling of the Circumscribed Self-Excited Spherical Attractor, 2022, 10, 2227-7390, 732, 10.3390/math10050732 | |
15. | Liheng Xie, Yongping Zhang, Estimations and Control of Julia Sets of the SIS Model Perturbed by Noise, 2023, 111, 0924-090X, 4931, 10.1007/s11071-022-08048-4 | |
16. | Mouhcine Naim, Fouad Lahmidi, Abdelwahed Namir, Abdelfatah Kouidere, Dynamics of an fractional SEIR epidemic model with infectivity in latent period and general nonlinear incidence rate, 2021, 152, 09600779, 111456, 10.1016/j.chaos.2021.111456 | |
17. | Peter Witbooi, Gbenga Abiodun, Mozart Nsuami, A model of malaria population dynamics with migrants, 2021, 18, 1551-0018, 7301, 10.3934/mbe.2021361 | |
18. | Nabeela Anwar, Shafaq Naz, Muhammad Shoaib, Reliable numerical treatment with Adams and BDF methods for plant virus propagation model by vector with impact of time lag and density, 2022, 8, 2297-4687, 10.3389/fams.2022.1001392 | |
19. | Kolade M. Owolabi, Edson Pindza, A nonlinear epidemic model for tuberculosis with Caputo operator and fixed point theory, 2022, 2, 27724425, 100111, 10.1016/j.health.2022.100111 | |
20. | Qiuping Chen, Shanshan Yu, Jia Rui, Yichao Guo, Shiting Yang, Guzainuer Abudurusuli, Zimei Yang, Chan Liu, Li Luo, Mingzhai Wang, Zhao Lei, Qinglong Zhao, Laurent Gavotte, Yan Niu, Roger Frutos, Tianmu Chen, Transmissibility of tuberculosis among students and non-students: an occupational-specific mathematical modelling, 2022, 11, 2049-9957, 10.1186/s40249-022-01046-z | |
21. | Mouhcine Naim, Yassine Sabbar, Mostafa Zahri, Behzad Ghanbari, Anwar Zeb, Nadia Gul, Salih Djilali, Fouad Lahmidi, The impact of dual time delay and Caputo fractional derivative on the long-run behavior of a viral system with the non-cytolytic immune hypothesis, 2022, 97, 0031-8949, 124002, 10.1088/1402-4896/ac9e7a | |
22. | Yan Cao, A.S. El-Shafay, Kamal Sharma, Ali A. Rajhi, Amin Salih Mohammed, Muhammad Bilal Riaz, Ali Althobaiti, S.A. Najati, Investigating the spread of a disease on the prey and predator interactions through a nonsingular fractional model, 2022, 32, 22113797, 105084, 10.1016/j.rinp.2021.105084 | |
23. | Puntani Pongsumpun, 2021, Local stability analysis of mathematical model of Tuberculosis disease in Thailand, 9781450388450, 99, 10.1145/3456172.3456206 | |
24. | Andang Sunarto, Praveen Agarwal, Jackel Vui Lung Chew, Jumat Sulaiman, Approximation Solution of the Fractional Parabolic Partial Differential Equation by the Half-Sweep and Preconditioned Relaxation, 2021, 13, 2073-8994, 1005, 10.3390/sym13061005 | |
25. | Ertugrul Karacuha, Esra Ergun, Nisa Ozge Onal Tugrul, Kamil Karacuha, Vasil Tabatadze, Analyzing Response Efficiency to COVID-19 and Underlying Factors of the Outbreak With Deep Assessment Methodology and Fractional Calculus, 2021, 9, 2169-3536, 157812, 10.1109/ACCESS.2021.3129904 | |
26. | E. Bonyah, C. W. Chukwu, M. L. Juga, , Modeling fractional-order dynamics of Syphilis via Mittag-Leffler law, 2021, 6, 2473-6988, 8367, 10.3934/math.2021485 | |
27. | Kunquan Lan, Linear first order Riemann-Liouville fractional differential and perturbed Abel's integral equations, 2022, 306, 00220396, 28, 10.1016/j.jde.2021.10.025 | |
28. | M.M. El-Dessoky, Muhammad Altaf Khan, Modeling and analysis of an epidemic model with fractal-fractional Atangana-Baleanu derivative, 2022, 61, 11100168, 729, 10.1016/j.aej.2021.04.103 | |
29. | Taye Faniran, Aatif Ali, Matthew O. Adewole, Babatunde Adebo, Olukunmi Olatunji Akanni, Asymptotic behavior of Tuberculosis between smokers and non-smokers, 2022, 5, 26668181, 100244, 10.1016/j.padiff.2021.100244 | |
30. | Taye Samuel Faniran, Matthew O. Adewole, Hijaz Ahmad, Farah A. Abdullah, Dynamics of tuberculosis in HIV–HCV co-infected cases, 2023, 16, 1793-5245, 10.1142/S1793524522500917 | |
31. | Erick Manuel Delgado Moya, Diego Samuel Rodrigues, Fractional order modeling for injectable and oral HIV pre-exposure prophylaxis, 2023, 3, 2767-8946, 139, 10.3934/mmc.2023013 | |
32. | Erick Manuel DELGADO MOYA, Alain PİETRUS, S´everine BERNARD, Silvere PAUL NUİRO, A mathematical model with fractional order for obesity with positive and negative interactions and its impact on the diagnosis of diabetes, 2023, 6, 2636-8692, 133, 10.33187/jmsm.1339842 | |
33. | Yudi Ari Adi, , An investigation of Susceptible–Exposed–Infectious–Recovered (SEIR) tuberculosis model dynamics with pseudo-recovery and psychological effect, 2024, 6, 27724425, 100361, 10.1016/j.health.2024.100361 | |
34. | Esam Y. Salah, Bhausaheb Sontakke, Mohammed S. Abdo, Wasfi Shatanawi, Kamaleldin Abodayeh, M. Daher Albalwi, Md. Kamrujjaman, Conformable Fractional-Order Modeling and Analysis of HIV/AIDS Transmission Dynamics, 2024, 2024, 1687-9651, 1, 10.1155/2024/1958622 | |
35. | Nabeela Anwar, Iftikhar Ahmad, Adiqa Kausar Kiani, Muhammad Shoaib, Muhammad Asif Zahoor Raja, Numerical treatment for mathematical model of farming awareness in crop pest management, 2023, 9, 2297-4687, 10.3389/fams.2023.1208774 | |
36. | Cicik Alfiniyah, Wanwha Sonia Putri Artha Soetjianto, Muhamad Hifzhudin Noor Aziz, Siti Maisharah Sheikh Ghadzi, Mathematical modeling and optimal control of tuberculosis spread among smokers with case detection, 2024, 9, 2473-6988, 30472, 10.3934/math.20241471 | |
37. | Slavi Georgiev, Mathematical Identification Analysis of a Fractional-Order Delayed Model for Tuberculosis, 2023, 7, 2504-3110, 538, 10.3390/fractalfract7070538 | |
38. | Morufu Oyedunsi Olayiwola, Kamilu Adewale Adedokun, A novel tuberculosis model incorporating a Caputo fractional derivative and treatment effect via the homotopy perturbation method, 2023, 47, 2522-8307, 10.1186/s42269-023-01091-0 | |
39. | Tasmia Roshan, Surath Ghosh, Sunil Kumar, Dynamical Behaviour of a Fractional-order SEIB Model, 2024, 63, 1572-9575, 10.1007/s10773-024-05724-6 | |
40. | Abdulsamad Engida Sado, Belela Samuel Kotola, A mathematical model based on ABC fractional order for TB transmission with treatment interruptions in case of Bule Hora town, Ethiopia, 2024, 47, 23529148, 101498, 10.1016/j.imu.2024.101498 | |
41. | K. A. Aldwoah, Mohammed A. Almalahi, Mansour A. Abdulwasaa, Kamal Shah, Sunil V. Kawale, Muath Awadalla, Jihan Alahmadi, Mathematical analysis and numerical simulations of the piecewise dynamics model of Malaria transmission: A case study in Yemen, 2024, 9, 2473-6988, 4376, 10.3934/math.2024216 | |
42. | Afeez Abidemi, Kolade M. Owolabi, Unravelling the dynamics of Lassa fever transmission with nosocomial infections via non-fractional and fractional mathematical models, 2024, 139, 2190-5444, 10.1140/epjp/s13360-024-04910-z | |
43. | Iffatricia Haura Febiriana, Dipo Aldila, Bevina Desjwiandra Handari, Puji Budi Setia Asih, Muhamad Hifzhudin Noor Aziz, Exploring the Interplay Between Social Awareness and the Use of Bed Nets in a Malaria Control Program, 2024, 6, 25889338, 196, 10.1016/j.jobb.2024.06.005 | |
44. | Wadhah Al‐sadi, Zhouchao Wei, Tariq Q. S. Abdullah, Abdulwasea Alkhazzan, J. F. Gómez‐Aguilar, Dynamical and numerical analysis of the hepatitis B virus treatment model through fractal–fractional derivative, 2024, 0170-4214, 10.1002/mma.10348 | |
45. | Erick M. D. Moya, Diego Samuel Rodrigues, A mathematical model for the study of latent tuberculosis under 3HP and 1HP regimens, 2024, 4, 2767-8946, 400, 10.3934/mmc.2024032 | |
46. | Tanni Rani Nandi, Amit Kumar Saha, Sudharonjon Roy, Analysis of a fractional order epidemiological model for tuberculosis transmission with vaccination and reinfection, 2024, 14, 2045-2322, 10.1038/s41598-024-73392-x | |
47. | Abdurkadir Edeo Gemeda, Legesse Lemecha Obsu, Eshetu Dadi Gurmu, Shambel Tadesse Degefa, Mathematical modeling of tuberculosis transmission dynamics with vaccination and two lines of treatments: a caputo fractional approach, 2024, 1598-5865, 10.1007/s12190-024-02308-9 | |
48. | Sibaliwe Maku Vyambwera, Peter Witbooi, Victor Kovtunenko, Optimal Intervention Strategies on TB Epidemiology in a Prison Population, 2024, 2024, 1085-3375, 10.1155/aaa/6482099 | |
49. | Ihtisham ul Haq, Nigar Ali, Hijaz Ahmad, Ramadan Sabra, M. Daher Albalwi, Imtiaz Ahmad, Mathematical analysis of a coronavirus model with Caputo, Caputo–Fabrizio–Caputo fractional and Atangana–Baleanu–Caputo differential operators, 2025, 18, 1793-5245, 10.1142/S1793524523500857 | |
50. | Manvendra Narayan Mishra, Faten Aldosari, Comparative study of tuberculosis infection by using general fractional derivative, 2025, 10, 2473-6988, 1224, 10.3934/math.2025058 | |
51. | Kuldeep Malik, Saad Althobaiti, Impact of the infected population and nonlinear incidence rate on the dynamics of the SIR model, 2025, 2025, 2731-4235, 10.1186/s13662-025-03897-w | |
52. | Qursam Fatima, Mubashir Qayyum, Murad Khan Hassani, Ali Akgül, Dynamical analysis of fractional hepatitis B model with Gaussian uncertainties using extended residual power series algorithm, 2025, 15, 2045-2322, 10.1038/s41598-025-88310-y | |
53. | Samson Linus Manu, Shikaa Samuel, Taparki Richard, Eshi Priebe Dovi, Mathematical Model for Prediction of Tuberculosis in Nigeria Using Hybrid Fractional Differential Equations and Artificial Neural Network Methods, 2025, 27731863, 100248, 10.1016/j.fraope.2025.100248 | |
54. | Nauman Raza, Shaiza Irum, Shafiullah Niazai, Muhammad Asad Ullah, Mohammad Y. Alshahrani, Andrew Omame, A mathematical framework of HIV and TB co-infection dynamics, 2025, 15, 2045-2322, 10.1038/s41598-025-91871-7 | |
55. | Chaimae El Mourabit, Nadia Idrissi Fatmi, A new model of the impact of chronic hepatitis C and its treatment on the development of tuberculosis: An optimal control and sensitivity analysis, 2025, 19, 26667207, 100574, 10.1016/j.rico.2025.100574 | |
56. | Morufu Oyedunsi Olayiwola, Ezekiel Abiodun Oluwafemi, Modeling Tuberculosis Dynamics with Awareness and Vaccination Using Laplace-Adomian and Fractional Calculus, 2025, 2731-8095, 10.1007/s40995-025-01830-0 | |
57. | Muath Awadalla, Fatmawati Fatmawati, Hajer Zaway, Kumama Regassa Cheneke, Computational methods for fractional order HIV model: a predictor-corrector approach, 2025, 1598-5865, 10.1007/s12190-025-02591-0 |
Description | Parameter | |
Populations growth rate | Λ | |
Growing-up rate for children | g | |
Natural death rate | μ | |
Children | Adult | |
population | population | |
Infection rate | βC | βA |
Proportion of fast progression | pC | pA |
Rate of progression from latent to the infectious | kC | kA |
Rate of effective therapy | γC | γA |
Rate of effective chemoprophylaxis | rC | rA |
TB death rate | dC | dA |
Parameter | Value | Ref. |
Λ | 1000 | Assumed |
βA | 0.02 | [48] |
g | 114 | [50] |
γA | 0.5 | Assumed |
pA | 0.3 | Assumed |
kA | 0.005 | [49] |
rA | 0.5 | Assumed |
dA | 0.05751 | [51] |
p | 0.3 | Assumed |
γ | 0.5 | Assumed |
r | 0.5 | Assumed |
βC | 0.01 | [48] |
μ | 0.0143 | [49] |
γC | 0.5 | Assumed |
pC | 0.4 | Assumed |
kC | 0.005 | [49] |
rC | 0.5 | Assumed |
dC | 0.0575 | [51] |
d | 0.05751 | [51] |
β | 0.02 | [48] |
k | 0.005 | [49] |
Description | Parameter | |
Populations growth rate | Λ | |
Growing-up rate for children | g | |
Natural death rate | μ | |
Children | Adult | |
population | population | |
Infection rate | βC | βA |
Proportion of fast progression | pC | pA |
Rate of progression from latent to the infectious | kC | kA |
Rate of effective therapy | γC | γA |
Rate of effective chemoprophylaxis | rC | rA |
TB death rate | dC | dA |
Parameter | Value | Ref. |
Λ | 1000 | Assumed |
βA | 0.02 | [48] |
g | 114 | [50] |
γA | 0.5 | Assumed |
pA | 0.3 | Assumed |
kA | 0.005 | [49] |
rA | 0.5 | Assumed |
dA | 0.05751 | [51] |
p | 0.3 | Assumed |
γ | 0.5 | Assumed |
r | 0.5 | Assumed |
βC | 0.01 | [48] |
μ | 0.0143 | [49] |
γC | 0.5 | Assumed |
pC | 0.4 | Assumed |
kC | 0.005 | [49] |
rC | 0.5 | Assumed |
dC | 0.0575 | [51] |
d | 0.05751 | [51] |
β | 0.02 | [48] |
k | 0.005 | [49] |