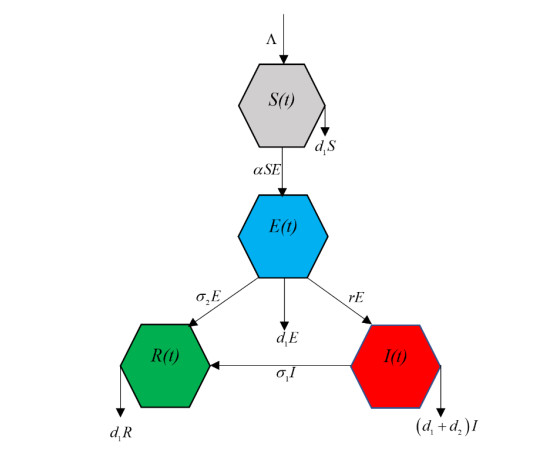
Citation: Daniela De Silva, Giorgio Tortone. Improvement of flatness for vector valued free boundary problems[J]. Mathematics in Engineering, 2020, 2(4): 598-613. doi: 10.3934/mine.2020027
[1] | Adil Yousif, Awad Ali . The impact of intervention strategies and prevention measurements for controlling COVID-19 outbreak in Saudi Arabia. Mathematical Biosciences and Engineering, 2020, 17(6): 8123-8137. doi: 10.3934/mbe.2020412 |
[2] | Tao Chen, Zhiming Li, Ge Zhang . Analysis of a COVID-19 model with media coverage and limited resources. Mathematical Biosciences and Engineering, 2024, 21(4): 5283-5307. doi: 10.3934/mbe.2024233 |
[3] | Salma M. Al-Tuwairqi, Sara K. Al-Harbi . Modeling the effect of random diagnoses on the spread of COVID-19 in Saudi Arabia. Mathematical Biosciences and Engineering, 2022, 19(10): 9792-9824. doi: 10.3934/mbe.2022456 |
[4] | Lin Feng, Ziren Chen, Harold A. Lay Jr., Khaled Furati, Abdul Khaliq . Data driven time-varying SEIR-LSTM/GRU algorithms to track the spread of COVID-19. Mathematical Biosciences and Engineering, 2022, 19(9): 8935-8962. doi: 10.3934/mbe.2022415 |
[5] | Sarah R. Al-Dawsari, Khalaf S. Sultan . Modeling of daily confirmed Saudi COVID-19 cases using inverted exponential regression. Mathematical Biosciences and Engineering, 2021, 18(3): 2303-2330. doi: 10.3934/mbe.2021117 |
[6] | Weike Zhou, Aili Wang, Fan Xia, Yanni Xiao, Sanyi Tang . Effects of media reporting on mitigating spread of COVID-19 in the early phase of the outbreak. Mathematical Biosciences and Engineering, 2020, 17(3): 2693-2707. doi: 10.3934/mbe.2020147 |
[7] | Sarafa A. Iyaniwura, Musa Rabiu, Jummy F. David, Jude D. Kong . Assessing the impact of adherence to Non-pharmaceutical interventions and indirect transmission on the dynamics of COVID-19: a mathematical modelling study. Mathematical Biosciences and Engineering, 2021, 18(6): 8905-8932. doi: 10.3934/mbe.2021439 |
[8] | Yue Deng, Siming Xing, Meixia Zhu, Jinzhi Lei . Impact of insufficient detection in COVID-19 outbreaks. Mathematical Biosciences and Engineering, 2021, 18(6): 9727-9742. doi: 10.3934/mbe.2021476 |
[9] | Damilola Olabode, Jordan Culp, Allison Fisher, Angela Tower, Dylan Hull-Nye, Xueying Wang . Deterministic and stochastic models for the epidemic dynamics of COVID-19 in Wuhan, China. Mathematical Biosciences and Engineering, 2021, 18(1): 950-967. doi: 10.3934/mbe.2021050 |
[10] | Saima Akter, Zhen Jin . A fractional order model of the COVID-19 outbreak in Bangladesh. Mathematical Biosciences and Engineering, 2023, 20(2): 2544-2565. doi: 10.3934/mbe.2023119 |
One of the most global deathly diseases is HIV (Human Immunodeficiency Virus), which has its genesis from crossed kinds from chimpanzees to humans. No one knew this disease before 1980, and its transmission was not coupled with visible signs or symptoms. Almost, by 1980, HIV has spread to the five continents, also within this interval of time, more than 300,000 persons were infected by HIV. Another well-known deathly infectious disease is called Ebola. It killed many people around the world in the past years. Some scientists believed that its transmission comes from an infected animal such as non-human mammals or bats. Many other deathly diseases are existing due to the interaction between different living beings and humans. So far, humans have developed several medical protocols and techniques to protect themselves [1].
Now, all the world suffering from the COVID-19 outbreak, which is currently continuing, and the number of infections is rising steadily. This is due to the presence of many factors that increase the complexities of COVID-19 infection and create barriers to disease management. Among the most important of these barriers is the origin of the infection is still unknown, and the incubation period of the virus is entirely unlimited. Moreover, there is yet no direct treatment for this virus, or anti-vaccine. Therefore, disease control largely depends on timely diagnosis and isolation. One essential factor in the spread of this disease is the transition of infected people from one place to another, which affects more people and therefore causes the spread of this disease. As a result, many countries have prohibited air traffic for a long time, and they stopped all kinds of sports and competitions. Besides, every country around the globe is trying to reduce unnecessary travel and to reduce cases of infection in their countries [2].
Thus, scientists and researchers all over the world are trying to find out and improve vaccine or cure for the outbreak. So that in the future, such as pandemic may be controlled, from a medical engineering point framework, an infectious disease can be well known and understood by using the mathematical models. This idea was started in 1927. After that, many different mathematical models have been constructed for various diseases and infections [3,4,5,6,7,8]. Wu et al. [9] introduced the well-known SEIR model (Susceptible-Exposed-Infectious-Recovered) model to describe the transmission dynamics and estimated the national and global spread of the disease, depending on reported data from December 31, 2019, to January 28, 2020. They also estimated that the basic reproductive number for COVID-19 was about 2.68. Read et al. [10] reported a value of 3.1 for the basic reproductive number based on data fitting of the SEIR model, using an assumption of Poisson-distributed daily time increments. Tang et al. [11] proposed a deterministic compartmental model incorporating the clinical progression of the disease, the individual epidemiological status, and the involvement measures. They found that the reproductive control number could be as high as 6.47, and that involvement strategies such as condensed contact tracing followed by isolation and quarantine can effectively reduce the control reproduction number and the transmission risk. Imai et al. [12] conducted computational modeling of potential epidemic tracks to estimate the size of the disease outbreak in Wuhan, with a focus on the human to other human transmissions. Their results imply that control measures need to block well over 60% of transmission to be effective in containing the outbreak. Also, Gao et al. [13] developed a deep learning algorithm to analyze the infectivity of the novel coronavirus and predict its potential hosts. Their results indicate that bats and minks maybe two animal hosts of this virus. Most of these models have emphasized the significant role of the direct, human-to-human transmission pathway in this epidemic, as highlighted by the facts that the majority of the infected individuals did not have any contact with the marketplaces in Wuhan, that the number of infections has been rapidly increasing, and that the disease has spread to all provinces in China as well as more than 20 other countries. Many infected individuals exhibit a relatively long incubation period so that they do not show any symptoms and are unaware of their infection for as long as 10–14 days. During this time, they can easily transmit the disease to other people through direct contact. On the other hand, the models published thus far have not considered the role of the environment in the transmission of COVID-19. Several other modeling studies have already been performed for the COVID-19 epidemic [9,10,14,15,16,17,18,19,20,21,22,23].
Because the mathematical model can reach understood and definite conclusions about the COVID's outbreak, a cascade of SEIR models has been constructed and developed to describe the mechanisms of its transmission from the source of infection, reservoir, hosts to humans [22]. De la Sen, M., et al. discussed a generalized time-varying SEIR propagation disease model subject to delays which potentially involves mixed regular and impulsive vaccination rules [24]. Song et al. studied the SVEIRS infectious disease model with pulse and two-time delays. The pulse vaccination strategy is used as an effective strategy for the elimination of infectious disease [25].
Using a modified version of similar models, this paper is devoted to predicting the new COVID-19 model to be more suitable for more cases in any country by mathematical analysis of the considered model. Within this work, a proposed SEIR model has been constructed with global analysis. We aim to discover the dynamics of the transmission of the virus through humans. Another target is to study and find out the ideal protocols, control, and strategies that can reduce the outbreak significantly based on data on spreading the COVID-19 in Saudi Arabia.
During spreading COVID-19 in any country, the population could be divided into four dynamic sub-populations (see figure 1) which are described with the parameters of transmission rates as follows [1,7,17,24,26]:
●
●
●
●
●
●
●
●
●
●
●
We define the total population size by
N(t)=S(t)+E(t)+I(t)+R(t) | (1) |
According to the inflows and outflows in Figure 1, we can convert them into first-order ordinary non-linear differential equations as follows [1,7,26]:
dS(t)dt=Λ−αS(t)E(t)−d1S(t) | (2) |
dE(t)dt=αS(t)E(t)−(d1+σ2+r)E(t) | (3) |
dI(t)dt=rE(t)−(d1+d2+σ1)I(t) | (4) |
dR(t)dt=σ1I(t)+σ2E(t)−d1R(t) | (5) |
By simplify the above equations, we have
dS(t)dt=Λ−(αE(t)+d1)S(t) | (6) |
dE(t)dt=αS(t)E(t)−ε1E(t) | (7) |
dI(t)dt=rE(t)−ε2I(t) | (8) |
dR(t)dt=σ1I(t)+σ2E(t)−d1R(t) | (9) |
where
All the solutions of the proposed SEIR model with its initial condition is a subset in the interval
Proof. All the right-hand sides of the proposed SEIR model is completely continuous and locally Lipschitzian on
From the Eq (6) where
dS(t)dt⩾−MS(t) | (10) |
By solving the above differential inequality, we get
S(t)⩾S(0)e−Mt⩾0 | (11) |
Hence,
From the Eq (7), we have
dE(t)dt⩾−ε1E(t) | (12) |
which gives
E(t)⩾E(0)e−ε1t⩾0 | (13) |
Hence,
In similar manners for the rest equations, we have
dI(t)dt⩾−ε2I(t)→I(t)⩾I(0)e−ε2t⩾0 | (14) |
and
dR(t)dt⩾−d1R(t)→R(t)⩾R(0)e−d1t⩾0 | (15) |
Hence,
All the solutions of the proposed SEIR model structure that initiate in
Proof. By differentiating both sides of the Eq (1), we get
N′(t)=S′(t)+E′(t)+I′(t)+R′(t) | (16) |
Substituting from the proposed SEIR model (6)–(9), we get
N′Λ+d1N=Λ−d2I | (17) |
From theorem 1, we have
N′(t)+d1N(t)⩽Λ | (18) |
Then, we obtain
N(t)⩽(N(0)−Λd1)e−d1t+Λd1 | (19) |
Thus, when
To determine the epidemic equilibrium of this model, we set all the derivatives equal to zero and solve the system as follows [7]:
S′(t)=E′(t)=I′(t)=R′(t)=0 | (20) |
which gives
0=Λ−αSE−d1S | (21) |
0=αSE−ε1E | (22) |
0=rE−ε2I | (23) |
0=σ1I+σ2E−d1R | (24) |
From the Eq (22), we have
S=ε1α | (25) |
From the Eq (23), we have
E=ε2rI | (26) |
Substitute from Eqs (25) and (26) into the Eq (21), we get
I=rd1αε2(αΛd1ε1−1)=rd1αε2(ℜ0−1) | (27) |
where
ℜ0=αΛd1ε1=αΛd1(d1+σ2+r) | (28) |
The number
The reproduction number is positive, and it is zero if there is no transmission, where
Substitute from Eq (27) into Eq (26), we obtain
E=d1α(ℜ0−1) | (29) |
Substitute from Eqs (25), (27), and (29) into Eq (24), we get
R=(rσ1+ε2σ2αε2)(ℜ0−1) | (30) |
Thus, at disease-free equilibrium (DFE)
Then, if
E∗0=(S∗,E∗,I∗,R∗) | (31) |
where
Thus, the system has a unique disease-free equilibrium
To get the reproduction number
The Jacobian matrix of the proposed SEIR model takes the following form:
JE0=[−αE−d1−αS00αEαS−ε1000r−ε200σ2σ1−d1] | (32) |
By using the Jacobian's linearization method, we will linearize the first two equations considering the equilibrium situation of the system
Hence, we consider the following functions
F(S,E)=Λ−αS(t)E(t)−d1S(t) | (33) |
G(S,E)=αS(t)E(t)−ε1E(t) | (34) |
Then, we have
[FSFEGSGE][S(t)−S(0)E(t)−E(0)]=[−αE(0)−d1−αS(0)αE(0)αS(0)−ε1][S(t)−S(0)E(t)−E(0)] | (35) |
By substituting from the equilibrium position, we obtain
[S′(t)E′(t)]=[−d1−αΛd10αΛd1−ε1][S(t)−Λd1E(t)] | (36) |
Hence, the coupled non-linear Eqs (6) and (7) has been linearized to the forms:
dS(t)dt=Λ−d1S(t)−αΛd1E(t) | (37) |
and
dE(t)dt=(αΛ−d1ε1d1)E(t) | (38) |
Hence, the Jacobian matrix of the proposed SEIR model after linearization at equilibrium is given by [1,7]:
JE0=[−d1−αΛd1000αΛd1−ε1000r−ε200σ2σ1−d1] | (39) |
By calculating the characteristic equation given by
[λ1λ2λ3λ4]=[−ε2−d1−d1αΛ−d1ε1d1] | (40) |
Hartman–Grobman theorem state that the solutions of a square system of non-linear ordinary differential Eqs (6)–(9) in a neighborhood of a steady-state look "qualitatively" just like the solutions of the linearized system near the point
Thus, from (40) we obtain the following condition of equilibrium
αΛ−d1ε1<0 | (41) |
Thus,
If the matrix
Hence, from (39), we have
det(JE0)=det[−d1−αΛd1000αΛd1−ε1000r−ε200σ2σ1−d1]=−ε2d1(αΛ−d1ε1)≠0 | (42) |
Thus, the condition (41) is the only condition of the equilibrium of the proposed SEIR model.
Therefore, the unique equilibrium condition of the proposed SEIR model is
αΛd1ε1−1≠0 | (43) |
and the reproduction number (RBN)
The proposed SEIR model
Proof. From the Jacobian matrix of the system (39) which is defined at
λ1=−ε2<0,λ2=−d1<0,andλ3=−d1<0 | (44) |
Thus, the system is locally stable when
ℜ0=αΛd1ε1<1 | (45) |
and the instability condition is
ℜ0=αΛd1ε1>1 | (46) |
Thus, we have
ℜ0→{ℜ0>1Unstable situationℜ0=0Notransmissionℜ0<1Stable situation} | (47) |
Local sensitivity analysis is a sensitivity analysis that examines the change in the output values that result from a change in one input value (one-parameter). The sensitivity or elasticity of the reproduction number
℘pℜ0=∂ℜ0∂p/∂ℜ0∂pℜ0pℜ0p=±%Δℜ0%Δp | (48) |
The sensitivity of
Applying the formula (48) into the reproduction number
ℜ0=αΛd1ε1=αΛd1(d1+σ2+r) | (49) |
Then,
℘αℜ0=∂ℜ0∂α/∂ℜ0∂α(ℜ0α)(ℜ0α)=1>0 | (50) |
℘rℜ0=∂ℜ0∂r/∂ℜ0∂r(ℜ0r)(ℜ0r)=−rε1<0 | (51) |
℘σ2ℜ0=∂ℜ0∂σ2/∂ℜ0∂σ2(ℜ0σ2)(ℜ0σ2)=−σ2ε1<0 | (52) |
℘d1ℜ0=∂ℜ0∂d1/∂ℜ0∂d1(ℜ0d1)(ℜ0d1)=−(d1+ε1)ε1<0 | (53) |
The fact that
From relation (50)
Lyapunov functions are scalar functions that may be used to prove the global stability of equilibrium. Lyapunov states that if a function
The SEIR model
Proof. We will consider the proposed SEIR model on the space of the first three variables only
We construct the Lyapunov function on
V=κ(S−S∗−S∗ln(SS∗))+Eε1+Ir | (54) |
where
The Eq (54) shows that, at the disease-free equilibrium
Now, we have to show that
The Eq (54) can be re-written as follows
V=κS∗(SS∗−1−ln(SS∗))+Eε1+Ir | (55) |
The first term is positive for any value of
Now, we take the derivative of the Eq (54), we obtain
V′=κ(1−S∗S)S′+E′ε1+I′r | (56) |
Substitute from the first three equation of the SEIR model and using the Eq (26), we obtain
V′=2Λκ−καε2rSI−d1κS−Λ2κSd1+καΛε2d1rI+αε2ε1rSI−ε2rI | (57) |
We choose
V′=−Λε1(d1SΛ+Λd1S−2)+ε2rI(ℜ0−1) | (58) |
Since
For the first term, consider
The second possibility is
Therefore,
According to Lyapunov's theorem, the disease-free equilibrium is globally asymptotically stable for the system of the proposed SEIR model in all [7].
After linearization of the system of the SEIR model with the initial values, the system takes the form
dS(t)dt=Λ−d1S(t)−αΛd1E(t) | (59) |
dE(t)dt=(αΛ−d1ε1d1)E(t) | (60) |
dI(t)dt=rE(t)−ε2I(t) | (61) |
dR(t)dt=σ1I(t)+σ2E(t)−d1R(t) | (62) |
We assume the initial conditions of the above system take the form
{S(t),E(t),I(t),R(t)}|t=0={S(0),E(0),I(0),R(0)} | (63) |
To solve the above system, we start to solve the equation (60) as following
E(t)=E(0)eγ1t | (64) |
where
Then, by substitute from Eq (64) into the Eq (61), we get
dI(t)dt+ε2I(t)=rE(0)eγ1t | (65) |
By solving the above equation, we get the infection function in the form
I(t)=(I(0)−γ2)e−ε2t+γ2eγ1t | (66) |
where
We can solve the first equation of the system by using the Eq (64)
dS(t)dt−d1S(t)=Λ−αΛE(0)d1eγ1t | (67) |
After solving the Eq (67), we get
S(t)=(S(0)+γ3−Λd1)e−d1t+(Λd1−γ3eγ1t) | (68) |
where
By inserting the Eqs (64), (66), and (68) into the Eq (62), we can solve the ordinary differential equation
To verify the proposed SEIR model, we will use the real data of spreading COVID-19 in Saudi Arabia. The spreading of COVID-19 has been stared in Saudi Arabia on March 3, 2020. The discovered cases continued with small numbers until the beginning of April, then the number of cases detected in infection increased. So, we decided to consider April 1, 2020, which is the real beginning of the spread of the COVID-19 epidemic in Saudi Arabia.
We used the statistics tables which issued from the Saudi Ministry of Health [27]and the daily official statement issued by the ministry as well as from the Wikipedia website [28], which also depends on the ministry's website and some other sites that would announce these statistics.
Another source of these data is the Saudi Center for Diseases Prevention and Control [29]. To obtain some information about the kingdom's population, mortality rate, and population growth rate, the official website of the General Statistics Authority of Saudi Arabia has been used.
To study the situation of spreading COVID-19 in Saudi Arabia until June 15, 2020, we will represent the curve of the daily number of infections and the time series curve of the total number of infections as in the Figures 2 and 3.
Figure 2 shows that the number of cases on April 1, 2020, was 157 infections, and it reaches 4507 infections on June 15, 2020, and between the two numbers, the curves passed through many variations up and down. Figure 3 shows that the total number of cases at the same interval started with 157 infections and reaches an accumulated amount of 132,098 infections on June 15, 2020. So, we will use this data through the proposed SEIR model to stand on whether there is a convergence between the model results and the real data [27,28,29].
According to the official data of Saudi Arabia, we have the following initial data, which is considered as the initial conditions of the system based on the SEIR model, as in Table 1 [27,28,29]:
|
|
|
|
34,218,169 | |
157 | 99 |
where
The total number of a new birth of Saudi children and new residents
Parameter | Value | Background |
|
2300 | Calculated |
|
|
Calculated |
|
|
Estimated |
|
|
Calculated |
|
|
Calculated |
|
|
Calculated |
After using the above values of the parameter and by using MAPLE software, we get the results that indicate the number of daily infections as outcomes of the proposed SEIR model. The following figure shows the numerical results of the proposed SEIR model against the real data with different values of the parameter
Moreover, the reproduction number RBN
To illustrate the convergence between the results of the proposed SEIR model and the real results, we will display Figure 5, which shows the cumulatively infected numbers within the same interval referred to earlier. It is noted that the curve of the real data is setting between the three cases of the proposed SEIR model with the mention values of
Now, we will predict the situation of spreading COVID-19 in Saudi Arabia based on the current data and parameters with the same rates without any change in the procedures. We illustrate the results of the total number of infections by applying the proposed SEIR model for the next four hundred days, starting from April 1, 2020, and end on May 5, 2021. In Figure 6, the curves and results show whether the number of infections will not breakdown, and the spreading of the COVID-19 will continue with an unstable situation or not. The curves have been done by using the same three values of the parameters
We will show what we should do against this situation in the section of the best protocol to breakdown spreading the COVID-19.
To study the sensitivity of the critical parameters against the reproduction number RBN
α⩽1.8×10−9,σ2⩾0.004,r⩾0.005 | (69) |
Now, we are in the most critical section, which tells us what we should do to breakdown the spreading of COVID-19 in Saudi Arabia. Therefore, in this section, we will study the change of each parameter for the next 400 days of spreading the virus to know which one and which value will make the curve of the number of daily infections will reach the maximum value and then converts to go down.
Figure 10 shows that a reduction of the parameter
According to the results in Figures 10–12, we can get the ideal situation of breakdown the spreading of COVID-19 in Saudi Arabia when we merge all the changes of the parameter at the same time to get the ideal case. So, we will calculate the number of daily infections based on the new values of the parameters, as in Table 3 as follows:
Parameter | Value | Background |
|
2300 | Calculated |
|
|
Calculated |
|
|
Estimated |
|
|
Calculated |
|
|
Calculated |
|
|
Calculated |
Figure 13 shows the number of daily infections when we apply the values of the parameters, as in Table 3, which gives us the best situation to breakdown the spreading of the COVID-19 in Saudi Arabia in the next stage. The figure represents that the daily number of infections will go down faster when we change all the parameters as in Table 3 more than changing each parameter separately. Moreover, it is clear to see that the spreading will breakdown immediately after reaching those values of the parameters, and the breakdown of spreading COVID-19 could be on July 31, 2020. Thus, by going back to the definition of each parameter, we know now what we should do to breakdown the spreading of COVID-19 in Saudi Arabia. So, we can write the best protocol, which must the population in Saudi Arabia follow it to breakdown the spread of COVID-19 in Saudi Arabia and go back to their natural life.
To get the ideal situation which can help us to the breakdown of spreading COVID-19 in Saudi Arabia, we must start implementing the following protocol and procedures (see Figure 14).
(1) They are decreasing the value of the transmission rate from the susceptible population to infected but not detected by testing the population to be
(2) They are increasing the value of the transmission rate from infected and detected by testing the population to the recovery zone to be
(3) They are increasing the value of the transmission rate population
This paper proposed a new statistical SEIR epidemic for the outbreak of new COVID-19 coronaviruses. This proposed pandemic model offers a new method for evaluating and handling the COVID-19 epidemic. The real data of spreading COVID-19 in Saudi Arabia has been used to verify the results of that proposed model. The results show that the proposed SEIR model is a successful model to analyze the spreading of epidemics like COVID-19 in Saudi Arabia. The ideal protocol consists some steps, and advices have been introduced in detail to help the Saudi Arabia population to speed the breakdown spreading of COVID-19. One of the main subjects in that protocol is prevention is better than treatment. The other essential issue which helps to breakdown the spread of COVID-19 is to stay away and be at home as you can and make the infected people in an isolated zone or secured area.
Lastly, we need to increase the number of tests that confirm the infection and include effective and sufficient medication and vitamins, tonics, and supplements to protect non-infected individuals.
This work was funded by Research and Development Grants Program for National Research Institutions and Centers (GRANTS), Target Research Program, Infectious Diseases Research Grant Program, King Abdulaziz City for Science and Technology (KACST), Kingdom of Saudi Arabia, [grant number (5-20-01-007-0002)]. The authors are very grateful and thank the Research and Development Grants Program for National Research Institutions and Centers (GRANTS), Target Research Program, Infectious Diseases Research Grant Program, King Abdulaziz City for Science and Technology (KACST), Kingdom of Saudi Arabia, to fund this project and this work.
The Authors declare that this work has no conflict of interest.
[1] | Caffarelli LA, Alt HW (1981) Existence and regularity for a minimum problem with free boundary. J Reine Angew Math 325: 105-144. |
[2] | Caffarelli LA (1987) A Harnack inequality approach to the regularity of free boundaries. Part I. Lipschitz free boundaries are C1,α. Rev Mat Iberoamericana 3: 139-162. |
[3] | Caffarelli LA (1988) A Harnack inequality approach to the regularity of free boundaries. Part III. Existence theory, compactness, and dependence on x. Ann Scuola Norm Sci 15: 583-602. |
[4] | Caffarelli LA (1989) A Harnack inequality approach to the regularity of free boundaries. Part II. Flat free boundaries are Lipschitz. Commun Pure Appl Math 42: 55-78. |
[5] | Caffarelli LA, Roquejoffre JM, Sire Y (2010) Variational problems for free boundaries for the fractional Laplacian. J Eur Math Soc 12: 1151-1179. |
[6] |
Caffarelli LA, Shahgholian H, Yeressian K (2018) A minimization problem with free boundary related to a cooperative system. Duke Math J 167: 1825-1882. doi: 10.1215/00127094-2018-0007
![]() |
[7] | De Philippis G, Spolaor L, Velichkov B (2019) Regularity of the free boundary for the two-phase Bernoulli problem. arXiv:1911.02165. |
[8] | De Silva D (2011) Free boundary regularity for a problem with right hand side. Interface Free Bound 13: 223-238. |
[9] | De Silva D, Ferrari F, Salsa S (2014) On two phase free boundary problems governed by elliptic equations with distributed sources. Discrete Contin Dyn Syst Ser S 7: 673-693. |
[10] |
De Silva D, Roquejoffre JM (2012) Regularity in a one-phase free boundary problem for the fractional Laplacian. Ann I H Poincare An 29: 335-367. doi: 10.1016/j.anihpc.2011.11.003
![]() |
[11] | De Silva D, Savin O, Sire Y (2014) A one-phase problem for the fractional Laplacian: Regularity of flat free boundaries. Bull Inst Math Acad Sin 9: 111-145. |
[12] |
Kriventsov D, Lin FH (2018) Regularity for shape optimizers: The nondegenerate case. Commun Pure Appl Math 71: 1535-1596. doi: 10.1002/cpa.21743
![]() |
[13] |
Kriventsov D, Lin FH (2019) Regularity for shape optimizers: The degenerate case. Commun Pure Appl Math 72: 1678-1721. doi: 10.1002/cpa.21810
![]() |
[14] |
Mazzoleni D, Terracini S, Velichkov B (2017) Regularity of the optimal sets for some spectral functionals. Geom Funct Anal 27: 373-426. doi: 10.1007/s00039-017-0402-2
![]() |
[15] |
Mazzoleni D, Terracini S, Velichkov B (2020) Regularity of the free boundary for the vectorial Bernoulli problem. Anal PDE 13: 741-763. doi: 10.2140/apde.2020.13.741
![]() |
1. | Manuel De la Sen, Santiago Alonso-Quesada, Asier Ibeas, On a Discrete SEIR Epidemic Model with Exposed Infectivity, Feedback Vaccination and Partial Delayed Re-Susceptibility, 2021, 9, 2227-7390, 520, 10.3390/math9050520 | |
2. | Hongfan Lu, Yuting Ding, Silin Gong, Shishi Wang, Mathematical modeling and dynamic analysis of SIQR model with delay for pandemic COVID-19, 2021, 18, 1551-0018, 3197, 10.3934/mbe.2021159 | |
3. | Hamdy Youssef, Najat Alghamdi, Magdy A. Ezzat, Alaa A. El-Bary, Ahmed M. Shawky, Study on the SEIQR model and applying the epidemiological rates of COVID-19 epidemic spread in Saudi Arabia, 2021, 6, 24680427, 678, 10.1016/j.idm.2021.04.005 | |
4. | Manuel De la Sen, Santiago Alonso-Quesada, Asier Ibeas, Raul Nistal, On a Discrete SEIR Epidemic Model with Two-Doses Delayed Feedback Vaccination Control on the Susceptible, 2021, 9, 2076-393X, 398, 10.3390/vaccines9040398 | |
5. | Xinyu Liu, Zimeng Lv, Yuting Ding, Mathematical modeling and stability analysis of the time-delayed SAIM model for COVID-19 vaccination and media coverage, 2022, 19, 1551-0018, 6296, 10.3934/mbe.2022294 | |
6. | Muhammad Abdurrahman Rois, Cicik Alfiniyah, Chidozie W. Chukwu, Dynamic analysis and optimal control of COVID-19 with comorbidity: A modeling study of Indonesia, 2023, 8, 2297-4687, 10.3389/fams.2022.1096141 | |
7. | Shabana Bibi, Muhammad Saad Khan, Sherif A. El-Kafrawy, Thamir A. Alandijany, Mai M. El-Daly, Qudsia Yousafi, Dua Fatima, Arwa A. Faizo, Leena H. Bajrai, Esam I. Azhar, Virtual screening and molecular dynamics simulation analysis of Forsythoside A as a plant-derived inhibitor of SARS-CoV-2 3CLpro, 2022, 30, 13190164, 979, 10.1016/j.jsps.2022.05.003 | |
8. | Mengying Wang, Cuixia Lee, Wei Wang, Yingyun Yang, Cheng Yang, Yi-Zhang Jiang, Early Warning of Infectious Diseases in Hospitals Based on Multi-Self-Regression Deep Neural Network, 2022, 2022, 2040-2309, 1, 10.1155/2022/8990907 | |
9. | R. Prem Kumar, P.K. Santra, G.S. Mahapatra, Global stability and analysing the sensitivity of parameters of a multiple-susceptible population model of SARS-CoV-2 emphasising vaccination drive, 2023, 203, 03784754, 741, 10.1016/j.matcom.2022.07.012 | |
10. | Ali Yousef, Fatma Bozkurt, Thabet Abdeljawad, Emad Emreizeeq, A mathematical model of COVID-19 and the multi fears of the community during the epidemiological stage, 2023, 419, 03770427, 114624, 10.1016/j.cam.2022.114624 | |
11. | Jiaji Pan, Zhongxiang Chen, Yixuan He, Tongliang Liu, Xi Cheng, Jun Xiao, Hao Feng, Why Controlling the Asymptomatic Infection Is Important: A Modelling Study with Stability and Sensitivity Analysis, 2022, 6, 2504-3110, 197, 10.3390/fractalfract6040197 | |
12. | Othman A.M. Omar, Yousef Alnafisah, Reda A. Elbarkouky, Hamdy M. Ahmed, COVID-19 deterministic and stochastic modelling with optimized daily vaccinations in Saudi Arabia, 2021, 28, 22113797, 104629, 10.1016/j.rinp.2021.104629 | |
13. | Moirangthem Bidyaluxmi Devi, Arpita Devi, Praveen Kumar Gupta, Dharmendra Tripathi, Response of vaccination on community transmission of COVID-19: a dynamical approach, 2022, 231, 1951-6355, 3749, 10.1140/epjs/s11734-022-00652-0 | |
14. | Abdullah Ahmadini, Ahmed Msmali, Zico Mutum, Yashpal Singh Raghav, Anibal Coronel, The Mathematical Modeling Approach for the Wastewater Treatment Process in Saudi Arabia during COVID-19 Pandemic, 2022, 2022, 1607-887X, 1, 10.1155/2022/1061179 | |
15. | Zhaobin Xu, Dongqing Wei, Qiangcheng Zeng, Hongmei Zhang, Yinghui Sun, Jacques Demongeot, More or less deadly? A mathematical model that predicts SARS-CoV-2 evolutionary direction, 2023, 153, 00104825, 106510, 10.1016/j.compbiomed.2022.106510 | |
16. | Ali AlArjani, Md Taufiq Nasseef, Sanaa M. Kamal, B. V. Subba Rao, Mufti Mahmud, Md Sharif Uddin, Application of Mathematical Modeling in Prediction of COVID-19 Transmission Dynamics, 2022, 47, 2193-567X, 10163, 10.1007/s13369-021-06419-4 | |
17. | Ziren Chen, Lin Feng, Harold A. Lay, Khaled Furati, Abdul Khaliq, SEIR model with unreported infected population and dynamic parameters for the spread of COVID-19, 2022, 198, 03784754, 31, 10.1016/j.matcom.2022.02.025 | |
18. | Abdullah A. Al-Shammari, Hamad Ali, Barrak Alahmad, Faisal H. Al-Refaei, Salman Al-Sabah, Mohammad H. Jamal, Abdullah Alshukry, Qais Al-Duwairi, Fahd Al-Mulla, The Impact of Strict Public Health Measures on COVID-19 Transmission in Developing Countries: The Case of Kuwait, 2021, 9, 2296-2565, 10.3389/fpubh.2021.757419 | |
19. | Haile Habenom, Mulualem Aychluh, D.L. Suthar, Qasem Al-Mdallal, S.D. Purohit, Modeling and analysis on the transmission of covid-19 Pandemic in Ethiopia, 2022, 61, 11100168, 5323, 10.1016/j.aej.2021.10.054 | |
20. | Kamal Shah, Thabet Abdeljawad, Rahim Ud Din, To study the transmission dynamic of SARS-CoV-2 using nonlinear saturated incidence rate, 2022, 604, 03784371, 127915, 10.1016/j.physa.2022.127915 | |
21. | Raffaele D’Ambrosio, Patricia Díaz de Alba, Giuseppe Giordano, Beatrice Paternoster, 2022, Chapter 7, 978-3-031-10521-0, 90, 10.1007/978-3-031-10522-7_7 | |
22. | Fahad M. Alswaidi, Abdullah M. Assiri, Haya H. Alhaqbani, Mohrah M. Alalawi, Characteristics and outcome of COVID-19 cases in Saudi Arabia: Review of six-months of data (March–August 2020), 2021, 29, 13190164, 682, 10.1016/j.jsps.2021.04.030 | |
23. | Sadiqah Al Marzooq, COVID-19 OVERVIEW IN SAUDI ARABIA USING THE SIRV MODEL, 2023, 11, 2350-0530, 10.29121/granthaalayah.v11.i3.2023.5079 | |
24. | Lijuan Niu, Qiaoling Chen, Zhidong Teng, Ramziya Rifhat, Ge Zhang, Stationary distribution and probability density function of a stochastic COVID-19 infections model with general incidence, 2024, 361, 00160032, 106963, 10.1016/j.jfranklin.2024.106963 | |
25. | Faten S. Alamri, Edward L. Boone, Ryad Ghanam, Fahad Alswaidi, Monitoring COVID-19 pandemic in Saudi Arabia using SEIRD model parameters with MEWMA, 2023, 16, 18760341, 2038, 10.1016/j.jiph.2023.09.009 | |
26. | Jianlan Zhou, Guozhong Huang, Shenyuan Gao, Zhijin Chen, Xuehong Gao, Study on the influencing factors of piecewise multi-strain crossover epidemic spread under data contamination, 2023, 4, 26664496, 305, 10.1016/j.jnlssr.2023.07.002 | |
27. | Yaroslav Vyklyuk, Denys Nevinskyi, Kateryna Hazdiuk, Continuous-discrete GeoSEIR(D) model for modelling and analysis of geo spread COVID-19, 2024, 10, 26665212, 100155, 10.1016/j.ibmed.2024.100155 | |
28. | Mohamad Tafrikan, Yolanda Norasia, Aly Syafrudin, Muhammad Abdurrahman Rois, 2024, 3104, 0094-243X, 020009, 10.1063/5.0194546 | |
29. | Poosan Moopanar Muthu, Anagandula Praveen Kumar, Optimal control and bifurcation analysis of SEIHR model for COVID-19 with vaccination strategies and mask efficiency, 2024, 12, 2544-7297, 10.1515/cmb-2023-0113 | |
30. | Nafe Moradkhani, Frederick Benaben, Benoit Montreuil, Matthieu Lauras, Thibaut Cerabona, Clara Le Duff, Louis Faugere, Julien Jeany, A force-inspired paradigm for performance-based decision support—Physics of Decision application in nonlinear dynamical systems, 2024, 41, 2452414X, 100656, 10.1016/j.jii.2024.100656 | |
31. | Amr Elsonbaty, Waleed Adel, A. Aldurayhim, A. El-Mesady, Mathematical modeling and analysis of a novel monkeypox virus spread integrating imperfect vaccination and nonlinear incidence rates, 2024, 15, 20904479, 102451, 10.1016/j.asej.2023.102451 | |
32. | Muhammad Abdurrahman Rois, Cicik Alfiniyah, Santi Martini, Dipo Aldila, Farai Nyabadza, Modeling and optimal control of COVID-19 with comorbidity and three-dose vaccination in Indonesia, 2024, 6, 25889338, 181, 10.1016/j.jobb.2024.06.004 | |
33. | Shui-Lin Peng, Hong-Jue Wang, Hao Peng, Xiang-Bin Zhu, Xiang Li, Jianmin Han, Dandan Zhao, Zhao-Long Hu, NLSI: An innovative method to locate epidemic sources on the SEIR propagation model, 2023, 33, 1054-1500, 10.1063/5.0152859 | |
34. | Yubo Fan, Lixin Yan, Cheng Zhang, Yating Chen, Danjie Wu, 2023, Predicting Material Demand During Sudden Health Emergencies Based on Enhanced SEIR-D Model, 9798400708138, 1358, 10.1145/3644116.3644361 | |
35. | Sanad Al-Khawaja, Omar S. Asfour, The impact of COVID-19 on the importance and use of public parks in Saudi Arabia, 2024, 15, 20904479, 102286, 10.1016/j.asej.2023.102286 | |
36. | Olena Kiseleva, Sergiy Yakovlev, Dmytro Chumachenko, Oleksandr Kuzenkov, Exploring Bifurcation in the Compartmental Mathematical Model of COVID-19 Transmission, 2024, 12, 2079-3197, 186, 10.3390/computation12090186 | |
37. | Bolun Chen, Shuai Han, Xiaoluan Liu, Zhe Li, Ting Chen, Min Ji, Prediction of an epidemic spread based on the adaptive genetic algorithm, 2023, 11, 2296-424X, 10.3389/fphy.2023.1195087 | |
38. | Zenebe Shiferaw Kifle, Legesse Lemecha Obsu, Mathematical modeling and analysis of COVID-19 and TB co-dynamics, 2023, 9, 24058440, e18726, 10.1016/j.heliyon.2023.e18726 |
|
|
|
|
34,218,169 | |
157 | 99 |
Parameter | Value | Background |
|
2300 | Calculated |
|
|
Calculated |
|
|
Estimated |
|
|
Calculated |
|
|
Calculated |
|
|
Calculated |
|
|
|
|
34,218,169 | |
157 | 99 |
Parameter | Value | Background |
|
2300 | Calculated |
|
|
Calculated |
|
|
Estimated |
|
|
Calculated |
|
|
Calculated |
|
|
Calculated |
Parameter | Value | Background |
|
2300 | Calculated |
|
|
Calculated |
|
|
Estimated |
|
|
Calculated |
|
|
Calculated |
|
|
Calculated |