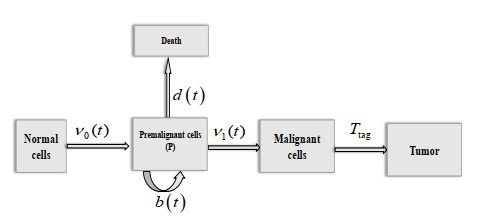
Lung cancer is a cancer with the fastest growth in the incidence and mortality all over the world, which is an extremely serious threat to human's life and health. Evidences reveal that external environmental factors are the key drivers of lung cancer, such as smoking, radiation exposure and so on. Therefore, it is urgent to explain the mechanism of lung cancer risk due to external environmental factors experimentally and theoretically. However, it is still an open issue regarding how external environment factors affect lung cancer risk. In this paper, we summarize the main mathematical models involved the gene mutations for cancers, and review the application of the models to analyze the mechanism of lung cancer and the risk of lung cancer due to external environmental exposure. In addition, we apply the model described and the epidemiological data to analyze the influence of external environmental factors on lung cancer risk. The result indicates that radiation can cause significantly an increase in the mutation rate of cells, in particular the mutation in stability gene that leads to genomic instability. These studies not only can offer insights into the relationship between external environmental factors and human lung cancer risk, but also can provide theoretical guidance for the prevention and control of lung cancer.
Citation: Lingling Li, Mengyao Shao, Xingshi He, Shanjing Ren, Tianhai Tian. Risk of lung cancer due to external environmental factor and epidemiological data analysis[J]. Mathematical Biosciences and Engineering, 2021, 18(5): 6079-6094. doi: 10.3934/mbe.2021304
[1] | Na Li, Peng Luo, Chunyang Li, Yanyan Hong, Mingjun Zhang, Zhendong Chen . Analysis of related factors of radiation pneumonia caused by precise radiotherapy of esophageal cancer based on random forest algorithm. Mathematical Biosciences and Engineering, 2021, 18(4): 4477-4490. doi: 10.3934/mbe.2021227 |
[2] | Yong Ding, Jian-Hong Liu . The signature lncRNAs associated with the lung adenocarcinoma patients prognosis. Mathematical Biosciences and Engineering, 2020, 17(2): 1593-1603. doi: 10.3934/mbe.2020083 |
[3] | Lingling Li, Yulu Hu, Xin Li, Tianhai Tian . Mathematical modeling the gene mechanism of colorectal cancer and the effect of radiation exposure. Mathematical Biosciences and Engineering, 2024, 21(1): 1186-1202. doi: 10.3934/mbe.2024050 |
[4] | Mengyang Han, Xiaoli Wang, Yaqi Li, Jianjun Tan, Chunhua Li, Wang Sheng . Identification of coagulation-associated subtypes of lung adenocarcinoma and establishment of prognostic models. Mathematical Biosciences and Engineering, 2023, 20(6): 10626-10658. doi: 10.3934/mbe.2023470 |
[5] | Ming Zhang, Yingying Zhou, Yanli Zhang . High Expression of TLR2 in the serum of patients with tuberculosis and lung cancer, and can promote the progression of lung cancer. Mathematical Biosciences and Engineering, 2020, 17(3): 1959-1972. doi: 10.3934/mbe.2020104 |
[6] | S. Dinesh Krishnan, Danilo Pelusi, A. Daniel, V. Suresh, Balamurugan Balusamy . Improved graph neural network-based green anaconda optimization for segmenting and classifying the lung cancer. Mathematical Biosciences and Engineering, 2023, 20(9): 17138-17157. doi: 10.3934/mbe.2023764 |
[7] | Michael James Horry, Subrata Chakraborty, Biswajeet Pradhan, Maryam Fallahpoor, Hossein Chegeni, Manoranjan Paul . Factors determining generalization in deep learning models for scoring COVID-CT images. Mathematical Biosciences and Engineering, 2021, 18(6): 9264-9293. doi: 10.3934/mbe.2021456 |
[8] | Linbo Zhang, Mei Xin, Peng Wang . Identification of a novel snoRNA expression signature associated with overall survival in patients with lung adenocarcinoma: A comprehensive analysis based on RNA sequencing dataset. Mathematical Biosciences and Engineering, 2021, 18(6): 7837-7860. doi: 10.3934/mbe.2021389 |
[9] | Yifan Wang, Lu Zhang, Yan Li, Fei Wu, Shiyu Cao, Feng Ye . Predicting the prognosis of HER2-positive breast cancer patients by fusing pathological whole slide images and clinical features using multiple instance learning. Mathematical Biosciences and Engineering, 2023, 20(6): 11196-11211. doi: 10.3934/mbe.2023496 |
[10] | Donggu Lee, Sunju Oh, Sean Lawler, Yangjin Kim . Bistable dynamics of TAN-NK cells in tumor growth and control of radiotherapy-induced neutropenia in lung cancer treatment. Mathematical Biosciences and Engineering, 2025, 22(4): 744-809. doi: 10.3934/mbe.2025028 |
Lung cancer is a cancer with the fastest growth in the incidence and mortality all over the world, which is an extremely serious threat to human's life and health. Evidences reveal that external environmental factors are the key drivers of lung cancer, such as smoking, radiation exposure and so on. Therefore, it is urgent to explain the mechanism of lung cancer risk due to external environmental factors experimentally and theoretically. However, it is still an open issue regarding how external environment factors affect lung cancer risk. In this paper, we summarize the main mathematical models involved the gene mutations for cancers, and review the application of the models to analyze the mechanism of lung cancer and the risk of lung cancer due to external environmental exposure. In addition, we apply the model described and the epidemiological data to analyze the influence of external environmental factors on lung cancer risk. The result indicates that radiation can cause significantly an increase in the mutation rate of cells, in particular the mutation in stability gene that leads to genomic instability. These studies not only can offer insights into the relationship between external environmental factors and human lung cancer risk, but also can provide theoretical guidance for the prevention and control of lung cancer.
As we all know cancer is caused by the cumulative mutations in multiple genes, accompanied by selective advantages [1]. Some hallmarks are acquired through the mutation and selection of cells in the development of tumor. They include but are not limited to the following signs: tissue invasion and metastasis, limitless replicative potential, resistance of programmed cell apoptosis, tumor promotion inflammation, sustained angiogenesis, insensitivity to growth-inhibitory signals, self-sufficiency in growth signals, reprogramming of energy metabolism, mutation immune destruction and, evading genetic instability [2,3,4]. With advances in DNA technology, high-throughput DNA sequencing has revolutionized the understanding of cancer, and previously undetected mutational signatures are discovered in the evolution of cancer. The evidence suggests that tumor contains abundant gene mutations, such as p53, KRAS, TP53, APC, chromosomal aberrations and so on [5,6,7]. However, most of them are passenger mutations that are not selectively advantageous to the cell clone [8,9]. The passenger mutation is not the reason why the tumor exists, and the onset of neoplasia is driven by the driver gene mutations that confer selective advantage to the cell clone [10]. Historically, many cancer researches were focused on the driver gene mutation with selective advantage that drives the initiation and progression of tumor. Comprehensive sequencing efforts revealed that two to eight mutations in the normal cells were involved for a solid tumor [11]. These significant discoveries motivate researchers to investigate how many driver genes are mutated for human certain cancer.
Although the outcomes from biological research are eye-opening, the reconciliation of these biological knowledge and epidemiologic or clinical observations still poses a significant challenge. To address this issue, biologically-based mathematical model has been presented to study the fundamental processes from healthy cells to a tumor, which is an efficient method for studying the mechanism of some cancers. As a pioneering study, Armitage and Doll proposed the multistage model to describe the development of tumor, who discovered that there was a linear relationship between logarithm of age and the risk of many cancers [12]. However, the model ignored the fact that the cells with gene mutations involved the clonal expansion of cells. In response to this issue, Armitage and Doll presented the model including two gene mutations with selective advantage to cells [13]. In their model, the normal cells mutated into the premalignant cells with clonal expansion, and then the mutated cells gave rise to an exponentially increasing proliferation and further mutated into a malignant tumor cell. Nevertheless, they did not give the exact biological meaning of the model. Later, Knudson examined 48 cases of retinoblastoma and proposed the hypothesis that retinoblastoma was the result of two gene mutations in the normal stem cells [14]. They discovered the gene RB, which is the earliest tumor suppressor gene. In addition, Moolgavkar et al. developed the model involving two mutation events to simulate the incidence rate or mortality data of most cancers at different ages [15,16,17,18,19]. In recent years, this model was widely applied to analyze the risk of various cancers due to external environmental factors.
Many models incorporated more biological knowledge were readily developed based on the model with two gene mutations, such as the model with multiple pathways with gene regulation and the model with more than two events [20,21,22,23]. Genetic instability caused by mutation in stability gene is an important mark in the development of cancer. Then, the model with genetic instability has been proposed to account for the mechanism of genetic instability in tumorigenesis [24,25,26,27,28,29,30]. Hazelton et al. presented a longitudinal biologically-based model to study lung cancer, which considered individual smoking histories, the probability of tumor in lung tissue, the mortality of lung cancer, CT screen detection and other factors [31]. Zhang and Simon described the model with the number of hits between two and six and considered clonal expansion of all mutated cells [32,33]. Their result showed that the fitting effect of the model with three hits to the female breast cancer incidence data was superior to that of the two-stage model. Moreover, the number of mutations in gene between two and fourteen was required for female breast cancer [34]. With the development of DNA sequencing technology, a large number of gene alternations were identified at base-pair resolution. These information on carcinogenesis pathway should be considered in the cancer model. Tomasetti et al. presented an approach to estimate the number of mutation in driver genes in lung and colon adenocarcinomas, which combined epidemiological data with genome sequencing information [35]. The result suggested the model involving three mutations in driver gene was the optimal model for lung cancer. Recently, the mechanistic model with molecular driver pathway was developed to predict the risk of lung adenocarcinoma, which indicated that radiation mainly affects the pathway involving transmembrane receptor-mutant whereas smoking primary influences the pathway with transducer-mutant in the development of lung adenocarcinoma [36].
In this paper, we mainly discuss the application of the cancer model with gene mutations on the analysis of cancer risk. For example, how to measure the risk of lung cancer due to external interventions (such as smoking and radiation exposure) through the mathematical framework and epidemiological data. Some important extensions of the cancer model and relevant references are reviewed firstly. In Section 2, we describe the clonal expansion model with two mutations in driver gene, more than two driver gene mutations and genomic instability and the application of these models on cancer studies. In Section 3, the effect of external environmental exposure on lung cancer risk are analyzed by the models mentioned in Section 2. In Section 4, we summary some relevant conclusions, and point out the shortcomings of the study as well as some perspectives to cancer research.
Evidence suggested that it required at least two driver gene mutations to develop malignant cells for most human cancer [1]. Thus, the model with two mutations in driver genes is widely used to study the pathogenesis of various cancers, which takes tumorigenesis as the final result of two driver gene mutations with rate-limiting in the healthy cells. The detailed model can be seen in Figure 1. The meanings of parameters in the model are as follows,
ν0(t)– the mutation rate from a healthy cell to the cells with gene mutation at time t;
b(t)– the birth rate at which a cell with gene mutation becomes into two same daughter cells at time t;
d(t)– the death rate at which a cell with gene mutation dies at time t;
ν1(t)– the mutation rate from a premalignant cell with gene mutation to a malignant cell that multiply indefinitely at time t.
The model assumes that once malignant cell is generated in the tissue, malignant tumor is detected with probability one after a suitable incubation period. The incubation time from the malignant cell to the tumor detected clinically, Ttag, is often considered as a constant [21,33,37].
What we are pay attention to are the probability of malignant cells by time t, p(t), and h(t) that denotes the hazard function by time t. The relationship between h(t) and p(t) is as follows [38]
p(t)=1−exp{−∫t0h(u)du}. | (2.1) |
In this model, let X0(t), X1(t) and X2(t) signify the number of healthy cells, premalignant cells, and malignant cells at time t, respectively. Healthy cells, X0(t) usually has three main growth patterns: gompertz curve, logistic curve and constant. Therefore, the number of healthy cells, X0(t), is usually considered as deterministic growth curve in the assessment of cancer risk [39].
For e<t, the following probability generating functions are defined to seek the solution of hazard function,
Φ(x1,x2;e,t)=∑j,kP{X1(t)=j,X2(t)=k|X1(e)=0,X2(e)=0}xj1xk2. | (2.2) |
According to Theorem 5A in [40] and the Kolmogorov forward equation, the derivative of the probability generating function Φ with respect to t is written as
dΦ(x1,x2;e,t)dt=(x1−1)ν0(t)X0(t)Φ(x1,x2;e,t)+{ν1(t)x1x2+b(t)x21+d(t)−[b(t)+d(t)+ν1(t)]x1}dΦ(x1,x2;e,t)dx1 | (2.3) |
with initial condition Φ(x1,x2;0,0)=1 by the definition of Φ.
By Eq (2.3), we have
dΦ(1,0;0,t)dt=−ν1(t)dΦdx1(1,0;0,t). | (2.4) |
Further,
dΦdx1(1,0;0,t)Φ(1,0;0,t)=E[X1(t)|X2(t)=0], | (2.5) |
and thus
h(t)=−dΦ(1,0;0,t)dtΦ(1,0;0,t)=ν1(t)E[X1(t)|X2(t)=0]. | (2.6) |
The conditional expectation in Eq (2.6) can be written as E[X1(t)] for certain cancers that are a rare disease, that is, P(t)≈0. Then, the hazard function can be given by h(t)=ν1(t)E[X1(t)].
From Eq (2.2), we can obtain that
dΦdx1(1,1;0,t)=E[X1(t)]. | (2.7) |
By differentiating the Eq (2.3), E[X1(t)] can be given by
E[X1(t)]dt=ν0(t)X0(t)+[b(t)−d(t)]E[X1(t)]. | (2.8) |
Thus,
h(t)≈ν1(t)∫t0{ν0(s)X0(s)exp∫ts[b(u)−d(u)]du}ds. | (2.9) |
However, the approximation value of h(t) mentioned above is poor when the probability of tumor is high. Hence, the exact solution of h(t) should be explored when a cancer is not a rare disease. By differentiating Eq (2.3) with respect to x1 and Eq (2.5), the conditional expectation, E[X1(t)|X2(t)=0], is derived as
E[X1(t)|X2(t)=0]dt=ν0(t)X0(t)+[b(t)−d(t)]E[X1(t)|X2(t)=0]−ν1(t)Var[X1(t)|X2(t)=0]. | (2.10) |
The solution of conditional expectation in Eq (2.10) is not easy to implement. To address this issue, some papers discussed the solution of the conditional expectation, E(⋅|⋅). Clewell et al. given the mathematical foundation for the approximation of Var[X1(t)|X2(t)=0], which was easy to implement for the model parameters with time-varying [41,42]. Besides, they found that the exact solution of hazard function, h(t), could be obtained when the parameters were time-constant after a slight modification. Crump et al. given the numerical solution of hazard function in the model with two mutations in genes by the Kolmogorov backward equations [43].
When the number of healthy cells, X0(t)=N, and the model parameters are time-constant, hazard function of the model can be written as
h(t)=ν0Nb(pq(exp{−qt}−exp{−pt})q(exp{−pt})−p(exp{qt})), | (2.11) |
where p=−(b−d−ν1)−√(b−d−ν1)2+4bν12 and q=−(b−d−ν1)+√(b−d−ν1)2+4bν12. For time-varying parameters, the time is usually divided into several subintervals with steady parameters to solve the function hazard.
The maximum number of driver gene mutations in certain tumor is very significant for the diagnosis and treatment of some cancers [5,11]. For this purpose, the model with more than two mutations in driver genes was built to estimate the number of driver gene mutations in the process from healthy cells to the malignant tumor. The model is shown in Figure 2.
Similar to the model with two mutations, let X0(t), Xi(t) i=1,2,⋯,k−1 and Xk(t) signify the numbers of healthy cells, premalignant cells with i gene mutations, and malignant cells at time t, respectively. For e≤t, the following probability generating functions are considered,
Ψ(x1,x2,⋯,xk;e,t)=∑i1,⋯,ikp{X1(t)=i1,⋯,Xk(t)=ik|X1(e)=0,X2(e)=0,⋯,Xk(e)=0}xi11xi22⋯xikk, | (2.12) |
and
Φi(xi,xi+1,⋯,xk;e,t)=∑i1,⋯,ikp{Xi(t)=ii,⋯,Xk(t)=ik|Xi(e)=1,Xi+1(e)=0,⋯,Xk(e)=0}xiiixii+1i+1⋯xikk. | (2.13) |
Then, h(t) yields
h(t)=νk−1(t)E[Xk−1(t)|Xk(t)=0]. | (2.14) |
For the number of healthy cells with X0(t)=N and the model parameters with time-constant, h(t) is also given by,
h(t)=−ν0N[Φ1(1,⋯,1,0;0,t)−1]. | (2.15) |
The detailed derivation can be seen in references [30,38].
Evidence suggested that less than 15 driver gene mutations might be responsible to drive and maintain the initiation and progression of the tumor [5,34]. Comprehensive sequencing efforts revealed that two to eight driver mutations in healthy cells are required in a tumor[11]. Thus, the model with more than two mutations was presented to estimate the maximum gene mutation number of lung cancer. From the US registry of Surveillance, Epidemiology, and End Results (SEER), the lung cancer incidence rate is adopted into a testing system, the fitting results shown that two to eight mutations in driver genes were required to drive the incidence of lung cancer [38]. Moreover, the result suggested that three mutations in driver genes occur to cause lung cancer with the highest probability [35,44]. Hence, the model with three gene mutations was usually utilized to analyze the risk of lung cancer due to some external factors [21,28].
Genomic instability plays a vital role in the development of almost all human cancers, which drives the occurrence and development of tumor [3]. Little et al. described the multistage model with genomic instability for carcinogenesis based on the model that Nowak et al. presented [24,25,26,27]. The model with genomic instability is displayed in Figure 3, which contains the cancer-stage (horizontal direction) and genomic instability stage (vertical direction). In the model, cancer-stage involves the mutations of oncogenes or tumor suppressor genes, and genomic instability caused by the mutation in stability genes.
This model with genomic instability was often used to analyze the mechanism of chromosome instability or microsatellite instability theoretically [24,45,46,47]. Little et al. gave the detailed derivation of the model with genomic instability, and applied this model to match the incidence rate of colon cancer in whites from the SEER registry during the year 1973–2002 [25]. They turned out that the model with five mutations of tumor suppressor genes or oncogenes and two instability mutations was better than other models for colon cancer. For lung cancer, the main type of genomic instability is chromosomal instability, which is caused by an abnormality in the number of chromosomes [48,49,50]. Thus, we are focus on the model with one genomic instability and two mutations in oncogenes or tumor suppressor genes. Z¨ollner et al. used the model with one genomic instability and two mutations in oncogenes or tumor suppressor genes to study lung cancer carcinogenesis in the Mayak Workers [28]. However, they did not analyze the mechanism of genomic instability and only made a comparison between the model with genomic instability and the two-three stages model without genomic instability.
Lung cancer has a high mortality in malignant tumors throughout the world [51,52,53], which hits a top incidence rate. Studies indicate that more than 90% of lung cancer is closely related to external factors such as cigarette smoking, radiation, air pollution, dietary habit and so on [44]. Among them, cigarette smoking and radiation are two major inducements to increase the risk of lung cancer. Moolgavkar et al.[19] studied the influence of smoking on the mortality of lung cancer by analyzing the intensity and duration of the smoking in the US over the year 1975–2000. Their result suggested a great influence of changing smoking behaviors on lung cancer mortality.
Lung cancer incidence or mortality from Nagasaki and Hiroshima in Japan is strongly dependent on radiation exposure, which is often used to evaluate the lung cancer risk due to the environment with high concentrations of radiation [30,38,54]. Here, we mainly discuss the impact of single environmental factor on lung cancer risk by the fitting of the model described above to epidemiological data. We adopt the incidence rate data of lung cancer from Nagasaki and Hiroshima and the Osaka Cancer Registry in Japan into a testing system. The data from Nagasaki and Hiroshima and the Osaka cancer registry can be downloaded in the URL http://www.rerf.jp and http://www.iph.pref.osaka.jp/omc/ocr/, and the patients from the Osaka cancer registry do not involve the radiation. The models described in the above are used to simulate the data from Nagasaki and Hiroshima during the year 1958–1987 and the Osaka cancer registry during the year 1974–1997. Maximum likelihood is employed to estimate the optimal parameter values of the model [30]. The lag time from a malignant cell into clinically detectable tumor is assumed to be 5 years [21,28]. Wilcoxon rank sum test for the real data and the simulated data shows that the smallest value of P (two-tailed) is greater than 0.2. Thus, the models fit the incidence rate data of lung cancer well.
For the model with two mutations in genes, the following formulas are obtained by the expressions of p and q,
{γ=b−d−ν1=−(p+q)≈b−dbν1=−pq. | (3.1) |
The optimal parameter values of the model is given in Table 1 for male patients and female patients. It reveals that radiation may induce the division of cells (b) and cause or increase cell death.
Parameters | The data with radiation | The data without radiation | |||
Male | Female | Male | Female | ||
ν0Nb | 0.003 | 0.002 | 0.015 | 0.005 | |
γ | 0.158 | 0.123 | 0.219 | 0.171 | |
bν1 | 1.524×10−6 | 6.597×10−6 | 1.440×10−7 | 5.52×10−7 |
The model with three mutations in driver genes can better fit the data than that with two mutations in driver genes. However, not all parameters can be identified form the data alone for this model. To address this issue, the most commonly used method is to fix the values of some parameters by biological results. The result of the model with three mutations in driver genes can be seen in reference [30] for the effect of radiation on lung cancer risk, which suggests that radiation increases the risk of lung cancer mainly by inducing the mutation of genes.
In the model with genomic instability, we set the mutation rate of cells including genomic instability and that of cells without genomic instability to be the same, respectively. In addition, γ1,GI=γ1 since genomic instability do not affect the net growth rate of cell[30]. The optimal parameter values of the model in Table 2 suggest that radiation mainly increases the mutation rates of genes, especially the mutation rate of stability genes for lung cancer. For the rates of cell reproduction, however, radiation doesn't cause the increase of them. It could be because radiation results in high death rates among cells.
Parameters | The data with radiation | The data without radiation | |||
Male | Female | Male | Female | ||
γ1,GI, γ1(≈b1−d1) | 0.160 | 0.128 | 0.219 | 0.209 | |
μ0, μ1 | 3.012×10−8 | 10−8 | 4.041×10−9 | 7.087×10−9 | |
μ0,GI, μ1,GI | 1.402×10−4 | 5.634×10−1 | 6.025×10−6 | 9.536×10−6 | |
υ0N | 1.147×10−3 | 1.466×10−6 | 1.456×10−7 | 6.729×10−8 | |
υ1 | 9.965×10−6 | 1.081×10−6 | 8.732×10−10 | 1.571×10−14 |
The functional relationship between radiation intensity and the parameters in the model is an open issue in the field of cancer. Zaballa and his co-workers indicated that the clonal expansion of cells has a nonlinear response mechanism with radon exposure rate by analyzing the mortality of lung cancer from the Wismut cohort [55]. Z¨ollner and his co-workers applied the model with the number of mutations between two and three to analyze the impact of Plutonium exposure on lung cancer risk, which indicated that the radiation effect shown a delayed response at an early stage and drop significantly with age [28]. In addition, studies suggested that the relationship between the radiation dose and the net proliferation rate of cells was nonlinear [28,55,56,57,58].
Cancer risk is affected by various carcinogens. However, the analysis to the joint effect of various carcinogens exposure on the risk of cancer is hard implement because of lacking full information of various factors. Therefore, the study for the cancer risk due to various carcinogens is still relatively lacking. For lung cancer, the radiation exposure and cigarette smoking are two major reasons to cause the increase in the incidence or mortality of lung cancer. Researches illustrated that more than 33% of the lung cancer cases were closely associated with smoking while almost 7% were relevant to radiation exposure[59]. The joint influence of smoking and exposure to radiation on lung cancer risk is noteworthy for the smokers who smoke less than a pack of cigarettes per day, while for heavy smokers who smoke greater than or equal to a pack per day, that appears to be additive or even sub-additive.
The two-mutation model is often used to study the risk of cancer due to environmental factors, since the parameters of the model can be identified by simulating epidemiological data. For example, the net proliferation rate and the mutation rate per cell division that we are interested in can be studied to analyze the effect of radiation and smoking intensity on lung cancer risk. The relationship between these model parameters and the radiation and smoking intensity can be described by the function depends on the dose of radiation, smoking index, and the time at exposure. Many studies suggested that the relationship between the dose rate of radiation and the net proliferation rate of cells is nonlinear, which revealed that the net growth rate of cells has a marked increase when the dose rate is larger than the critical value [28,55,56,57,58]. Recently, Castelletti et al.[36] proposed the mechanistic model with molecular pathways based on the two-mutation model to study the risk of lung adenocarcinoma due to smoking and exposure to radiation. Using molecular data from Caucasian and Asian patients [60,61], they found that radiation mainly plays a role in the pathway involving transmembrane receptor–mutant while smoking affects the pathway with transducer-mutant. In addition, the mechanisms of smoking and radiation is no interaction for lung adenocarcinoma, and the relationship between smoking intensity and the net growth rate of cells is a exponential function.
We mainly describe the applications of some mechanistic models based on biological knowledge on the study of lung cancer in this paper. The model with two hits is the most primitive model, which views complex carcinogenesis as two rate-limiting genomic events. There are plenty of work to study this model such as the solutions, properties and applications of the model. As the development of next-generation DNA sequencing techniques, more and more biological information are known for cancer. More complex and specific models are required for studying cancer. Therefore, some extended models based on the model with two hits are developed. These models set up the bridge between mathematics and biology.
The mechanistic models based on biological discoveries can well explain the impact of external environmental exposure on lung cancer risk. Although there are many models that involve some additional pathways such as genomic instability, these mathematical models may not be broad enough to declare the complicated carcinogenesis. In addition, not all biological parameters of the model can be obtained by fitting the data alone, which is a challenge to estimate model parameters. The commonly approaches to solve this issue are to assume suitable values for some parameters by known information or estimate the set of parameters instead of single parameterm[62]. The fitting results so far are obtained by the simplifying hypotheses such as parameter with time-constant and neglecting the growth of malignant cells. Nevertheless, the information in malignant cells may be very valuable for the study of lung cancer risk due to external environmental exposure. Therefore, the more detailed biological processes should be included in the cancer model. Besides, some papers provided other models to analyze the dynamics of cell population and key processes of tumorigenesis, such as multi-scale model, the model considering age-structured, stochastic reaction-diffusion model and so on [63,64,65,66,67,68,69].
There are a lot of work in mathematical modeling of cancer, however, the work on the variable parameters model of cancer is still lacking. In addition, the types of lung cancer and other external factors other than smoking and radiation should be considered for studying the risk of lung cancer. With the development of technology in DNA sequencing, information from TCGA analyses is commonly used to study the sequence of gene mutations in the certain tumor [70,71,72,73,74,75]. Thus, the specific model with these information should be developed to deepen the understanding of the mechanisms of carcinogenesis. The future studies for lung cancer should incorporated the information of gene regulatory pathways and several external factors.
This work was funded by the Natural Science Foundation of China (NO.12001417), the Science and Technology Key Project of Henan Province (No.212102310464), Key Scientific Research Project of Higher Education Institutions of Henan Province (NO.21A110015), and Guizhou Science and Technology Planning Project grant number [2020]4Y167. We would like to thank the anonymous reviewers for their constructive comments on the manuscript.
All authors declare no conflicts of interest in this paper.
[1] |
B. Vogelstein, K. W. Kinzler, Cancer genes and the pathways they control, Nat. Med., 10 (2004), 789–799. doi: 10.1038/nm1087
![]() |
[2] | D. Hanahan, R. A. Weinberg, The hallmarks of cancer, Cell, 100 (2000), 57–70. |
[3] |
D. Hanahan, R. A. Weinberg, The hallmarks of cancer: The next generation, Cell, 144 (2011), 646–674. doi: 10.1016/j.cell.2011.02.013
![]() |
[4] |
A. Amer, A. Nagah, T. T. Tian, X. A. Zhang, Mutation mechanisms of breast cancer among the female population in china, Curr. Bioinform., 15 (2020), 253–259. doi: 10.2174/1574893615666191220141548
![]() |
[5] |
L. D. Wood, D. W. Parson, S. Jones, J. Lin, T. Sj¨oblom, R. J. Leary, et al., The genomic landscapes of human breast and colorectal cancers, Science, 318 (2007), 1108–1113. doi: 10.1126/science.1145720
![]() |
[6] | S. Basu, S. Lee, J. Salotti, S. Basu, K. Sakchaisri, X. Xiao, et al., Oncogenic RAS-induced perinuclear signaling complexes requiring KSR1 regulate signal transmission to downstream targets, Cancer Res., 78 (2017), 891–908. |
[7] |
S. Y. Hong, Y. R. Kao, T. C. Lee, C. W. Wu, Upregulation of e3 ubiquitin ligase cblc enhances egfr dysregulation and signaling in lung adenocarcinoma, Cancer Res., 78 (2018), 4984–4996. doi: 10.1158/0008-5472.CAN-17-3858
![]() |
[8] |
I. Bozic, T. Antal, H. Ohtsuki, H. Carter, M. A. Nowak, Accumulation of driver and passenger mutations during tumor progression, Proc. Natl. Acad. Sci. U.S.A., 107 (2010), 18545–18550. doi: 10.1073/pnas.1010978107
![]() |
[9] |
C. Tomasetti, B. Vogelstein, G. Parmigiani, Half or more of the somatic mutations in cancers of self-renewing tissues originate prior to tumor, Proc. Natl. Acad. Sci. U.S.A., 110 (2013), 1999–2004. doi: 10.1073/pnas.1221068110
![]() |
[10] |
S. Jones, W. D. Chen, G. Parmigiani, F. Diehl, S. D. Markowitz, Comparative lesion sequencing provides insights into tumor evolution, Proc. Natl. Acad. Sci. U.S.A., 105 (2008), 4283–4288. doi: 10.1073/pnas.0712345105
![]() |
[11] | B. Vogelstein, N. Papadopoulos, V. E. Velculescu, S. Zhou, L. A. Diaz, K. W. Kinzler, Cancer genome landscapes, Science, 339 (2013), 1546–1558. |
[12] |
P. Armitage, R. Doll, The age distribution of cancer and multi-stage theory of carcinogenesis, Br. J. Cancer, 8 (1954), 1–12. doi: 10.1038/bjc.1954.1
![]() |
[13] | P. Armitage, R. Doll, A two-stage theory of carcinogenesis in relation to the age distribution of human cancer, Br. J. Cancer, 9 (1957), 161–169. |
[14] |
A. G. Knudson, Mutation and cancer: statistical study of retinoblastoma, Proc. Natl. Acad. Sci. U.S.A., 68 (1971), 820–823. doi: 10.1073/pnas.68.4.820
![]() |
[15] |
S. H. Moolgavkar, A. G. Knudson, Mutation and cancer: a model for human carcinogenesis, J. Natl. Cancer. Inst., 66 (1981), 1037–1052. doi: 10.1093/jnci/66.6.1037
![]() |
[16] | S. H. Moolgavkar, N. E. Day, R. G. Stevens, Two-stage model for carcinogenesis: epidemiology of breast cancer in females, J. Natl. Cancer Inst., 65 (1980), 559–569. |
[17] |
S. H. Moolgavkar, A. Dewanji, D. J. Venzon, A stochastic two-stage model for cancer risk assessment I: The hazard function and the probability of tumor, Risk Anal., 8 (1988), 383–392. doi: 10.1111/j.1539-6924.1988.tb00502.x
![]() |
[18] |
R. Meza, J. Jeon, S. H. Moolgavkar, E. G. Luebeck, Age-specific incidence of cancer: phases, transitions, and biological implications, Proc. Natl. Acad. Sci. U.S.A., 105 (2008), 16284–16289. doi: 10.1073/pnas.0801151105
![]() |
[19] | S. H. Moolgavkar, T. R. Holford, D. T. Levy, C. Y. Kong, M. Foy, L. Clarke, et al., Impact of reduced tobacco smoking on lung cancer mortality in the United States during 1975–2000, J. Natl. Cancer Inst., 104 (2012), 1–7. |
[20] |
W. R¨uhm, M. Eidemller, J. C. Kaiser, Biologically-based mechanistic models of radiation-related carcinogenesis applied to epidemiological data, Int. J. Radiat. Biol., 93 (2017), 1093–1117. doi: 10.1080/09553002.2017.1310405
![]() |
[21] |
L. Li, T. Tian, X. Zhang, The impact of radiation on the development of lung cancer, J. Theor. Biol., 428 (2017), 147–152. doi: 10.1016/j.jtbi.2017.06.020
![]() |
[22] |
B. M. Lang, J. Kuipers, B. Misselwitz, N. Beerenwinkel, Predicting colorectal cancer risk from adenoma detection via a two-type branching process model, PLOS Comput. Biol., 16 (2020), e1007552. doi: 10.1371/journal.pcbi.1007552
![]() |
[23] |
C. Paterson, H. Clevers, I. Bozic, Mathematical model of colorectal cancer initiation, Proc. Natl. Acad. Sci. U.S.A., 117 (2020), 20681–20688. doi: 10.1073/pnas.2003771117
![]() |
[24] |
M. A. Nowak, N. L. Komarova, A. Sengupta, P. V. Jallepalli, I. M. Shih, B. Vogelstein, et al., The role of chromosomal instability in tumor initiation, Proc. Natl. Acad. Sci. U.S.A., 99 (2002), 16226–16231. doi: 10.1073/pnas.202617399
![]() |
[25] |
M. P. Little, E. G. Wright, A stochastic carcinogenesis model incorporating genemic instability fitted to colon cancer data, Math. Biosci., 183 (2003), 111–134. doi: 10.1016/S0025-5564(03)00040-3
![]() |
[26] | M. P. Little, P. Vineis, G. Li, A stochastic carcinogenesis model incorporating multiple types of genomic instability fitted to colon cancer data, J. Theor. Biol., 254 (2017), 229–238. |
[27] |
M. P. Little, Cancer models, genomic instability and somatic cellular darwinian evolution, Biol. Direct., 5 (2010), 1–19. doi: 10.1186/1745-6150-5-1
![]() |
[28] |
S. Z¨ollner, M. E. Sokolnikov, M. Eidem¨oller, Beyond two-stage models for lung carcinogenesis in the Mayak Workers: implications for plutonium risk, PLoS ONE, 10 (2015), 1–20. doi: 10.1371/journal.pone.0116884
![]() |
[29] |
M. Eidem¨uller, E. Holmberg, P. Jacob, M. Lundell, P. Karlsson, Breast cancer risk and possible mechanisms of radiation-induced genomic instability in the Swedish hemangioma cohort after reanalyzed dosimetry, Mutat. Res., 775 (2015), 1–9. doi: 10.1016/j.mrfmmm.2015.03.002
![]() |
[30] |
L. L. Li, T. H. Tian, X. A. Zhang, L. Pang, Mathematical modeling the pathway of genomic stability in lung cancer, Sci. Rep., 9 (2019), 14136–14144. doi: 10.1038/s41598-019-50500-w
![]() |
[31] |
W. D. Hazelton, G. Goodman, W. N. Rom, M. Tockman, M. Thornquist, S. H. Moolgavkar, et al., Longitudinal multistage model for lung cancer incidence, mortality, and CT detected indolent and aggressive cancers, Math. Biosci., 240 (2012), 20–34. doi: 10.1016/j.mbs.2012.05.008
![]() |
[32] |
X. Zhang, R. Simon, Estimating the number of rate limiting genomic changes for human breast cancer, Breast Cancer Res. Treat., 91 (2005), 121–124. doi: 10.1007/s10549-004-5782-y
![]() |
[33] |
X. Zhang, Y. Fang, Y. Zhao, W. Zheng, Mathematical modeling the pathway of human breast cancer, Math. Biosci., 253 (2014), 25–29. doi: 10.1016/j.mbs.2014.03.011
![]() |
[34] |
L. Li, T. Tian, X. Zhang, Mutation mechanisms of human breast cancer, J. Comput. Biol., 25 (2018), 1–9. doi: 10.1089/cmb.2018.29010.jh
![]() |
[35] |
C. Tomasetti, L. Marchionni, M. A. Nowak, G. Parmigiani, B. Vogelstein, Only three driver gene mutations are required for the development of lung and colorectal cancers, Proc. Natl. Acad. Sci. U.S.A., 112 (2015), 118–123. doi: 10.1073/pnas.1421839112
![]() |
[36] |
N. Castelletti, J. C. Kaiser, C. Simonetto, K. Furukawa, H. Küchenhoff, G.T. Stathopoulos, Risk of lung adenocarcinoma from smoking and radiation arises in distinct molecular pathways, Carcinogenesis, 40 (2019), 1240–1250. doi: 10.1093/carcin/bgz036
![]() |
[37] |
M. D. Radmacher, R. Simon, Estimation of Tamoxifen's efficiency for preventing the formation and growth of breast tumors, J. Natl. Cancer Inst., 92 (2000), 48–53. doi: 10.1093/jnci/92.1.48
![]() |
[38] |
L. L. Li, T. T. Tian, X. A. Zhang, Stochastic modelling of multistage carcinogenesis and progression of human lung cancer, J. Theor. Biol., 479 (2019), 81–89. doi: 10.1016/j.jtbi.2019.07.006
![]() |
[39] |
S. H. Moolgavkar, G. Luebeck, Two-event model for carcinogenesis: biological, mathematical, and statistical considerations, Risk Anal., 10 (1990), 323–341. doi: 10.1111/j.1539-6924.1990.tb01053.x
![]() |
[40] | E. Parzen, Stochastic processes, in Applied Mathematics, San Francisco: Holden-Day, (1962), 188–299. |
[41] |
H. J. Clewell, D. W. Quinn, M. E. Andersen, R. B. Conolly, An improved approximation to the exact solution of the two-stage clonal growth model of cancer, Risk Anal., 15 (1995), 467–473. doi: 10.1111/j.1539-6924.1995.tb00339.x
![]() |
[42] | R. T. Hoogenveen, H. J. Clewell, M. E. Andersen, W. Slob, An alternative exact solution of the two- stage clonal growth model of cancer, Risk Anal., 19 (1999), 9–14. |
[43] |
K. S. Crump, R. P. Subramaniam, C. B. van Landingham, A numerical solution to the nonhomogeneous two-stage MVK model of cancer, Risk Anal., 25 (2005), 921–926. doi: 10.1111/j.1539-6924.2005.00651.x
![]() |
[44] | W. Song, S. Powers, Z. Wei, A. H. Yusuf, Substantial contribution of extrinsic risk factors to cancer development, Nature, 529 (2015), 43–47. |
[45] |
N. L. Komarovaa, A. Senguptac, M. A. Nowak, Mutation cselection networks of cancer initiation: tumor suppressor genes and chromosomal instability, J. Theor. Biol., 223 (2003), 433–450. doi: 10.1016/S0022-5193(03)00120-6
![]() |
[46] |
M. A. Nowak, F. Michor, Y. Iwasa, Genetic instability and clonal expansion, J. Theor. Biol., 241 (2006), 26–32. doi: 10.1016/j.jtbi.2005.11.012
![]() |
[47] |
A. D. Asatryan, N. L. Komarova, Evolution of genetic instability in heterogeneous tumors, J. Theor. Biol., 396 (2016), 1–12. doi: 10.1016/j.jtbi.2015.11.028
![]() |
[48] |
C. M. Choi, K. W. Seo, S. J. Jang, Y. M. Oh, T. S. Shim, W. S. Kim, et al., Chromosomal instability is a risk factor for poor prognosis of adenocarcinoma of the lung: Fluorescence in situ hybridization analysis of paraffin-embedded tissue from Korean patients, Lung Cancer, 64 (2009), 66–70. doi: 10.1016/j.lungcan.2008.07.016
![]() |
[49] | V. I. Minina, M. Y. Sinitsky, V. G. Druzhinin, A. Fucic, M. L. Bakanova, A. V. Ryzhkova, et al., Chromosome aberrations in peripheral blood lymphocytes of lung cancer patients exposed to radon and air pollution, Eur. J. Cancer Prev., 27 (2016), 6–12. |
[50] | C. J. Gomes, S. Centuori, J. D. Martinez, Abstract 3509: Overexpression of 14-3-3g contributes to chromosomal instability in human lung cancer, Cancer Res., 74 (2014), 3509–3509. |
[51] | D. M. Parkin, F. Bray, J. Ferlay, P. Pisani, Global cancer statistics, 2002, CA Cancer J. Clin., 55 (2005), 74–108. |
[52] |
J. Ferlay, H. R. Shin, F. Bray, D. Forman, C. Mathers, D. M. Parkin, Estimates of worldwide burden of cancer in 2008: globocan 2008, Int. J. Cancer, 127 (2010), 2893–2917. doi: 10.1002/ijc.25516
![]() |
[53] |
J. C. Martin, N. Lunet, A. G. Marrˊon, C. L. Moyano, N. M. Santander, R. Cleries, et al., Projections in breast and lung cancer mortality among women: a bayesian analysis of 52 countries worldwide, Cancer Res., 78 (2018), 4436–4442. doi: 10.1158/0008-5472.CAN-18-0187
![]() |
[54] |
L. Walsh, F. Dufey, A. Tschense, M. Schnelzer, B. Grosche, M. Kreuzer, Radon and the risk of cancer mortality-internal Poisson models for the German uranium miners cohort, Health Phys., 99 (2010), 292–300. doi: 10.1097/HP.0b013e3181cd669d
![]() |
[55] |
I. Zaballa, M. Eidem¨uller, Mechanistic study on lung cancer mortality after radon exposure in the wismut cohort supports important role of clonal expansion in lung carcinogenesis, Radiat. Environ. Biophys., 55 (2016), 299–315. doi: 10.1007/s00411-016-0659-0
![]() |
[56] | P. Jacob, R. Meckbach, M. Sokolnikov, V. V. Khokhryakov, E. Vasilenko, Lung cancer risk of Mayak workers: modelling of carcinogenesis and bystander effect, Radiat. Environ. Biophys., 46, (2007), 383–394. |
[57] |
W. Heidenreich, L. Tomasek, B. Grosche, K. Leuraud, D. Laurier, Lung cancer mortality in the European uranium miners cohort analysed with a biologically based model taking into account radon measurement error, Radiat. Environ. Biophys., 51 (2012), 263–275. doi: 10.1007/s00411-012-0423-z
![]() |
[58] |
M. Eidem¨uller, P. Jacob, R. S. D. Lane, S. E. Frost, L. B. Zablotska, Lung cancer mortality (1950–1999) among Eldorado uranium workers: a comparison of models of carcinogenesis and empirical excess risk models, PloS ONE, 7 (2012), e41431. doi: 10.1371/journal.pone.0041431
![]() |
[59] |
K. Furukawa, D. L. Preston, S. L¨onn, S. Funamoto, K. Mabuchi, Radiation and smoking effects on lung cancer incidence among atomic bomb survivors, Radiat. Res., 174 (2010), 72–82. doi: 10.1667/RR2083.1
![]() |
[60] |
J. D. Campbell, A. Alexandrov, J. Kim, J. Wala, A. H. Berger, C. Sekhar, et al., Distinct patterns of somatic genome alterations in lung adenocarcinomas and squamous cell carcinomas, Nat. Genet., 48 (2016), 607–616. doi: 10.1038/ng.3564
![]() |
[61] |
K. Wu, X. Zhang, F. Li, D. Xiao, Y. Hou, S. Zhu, et al., Frequent alterations in cytoskeleton remodelling genes in primary and metastatic lung adenocarcinomas, Nat. Commun., 6 (2015), 10131. doi: 10.1038/ncomms10131
![]() |
[62] | A. F. Brouwer, R. Meza, M. C. Eisenberg, A systematic approach to determining the identifiability of multistage carcinogenesis models, Risk Anal., 37 (2016), 1375–1387. |
[63] |
T. Alarcn, H. M. Byrne, P. K. Maini, A multiple scale model for tumor growth, Multiscale Model. Sim., 3 (2005), 440–475. doi: 10.1137/040603760
![]() |
[64] |
M. D. Johnston, C. M. Edwards, W. F. Bodmer, P. K. Maini, S. J. Chapman, Mathematical modeling of cell population dynamics in the colonic crypt and in colorectal cancer, Proc. Natl. Acad. Sci. U.S.A., 104 (2007), 4008–4013. doi: 10.1073/pnas.0611179104
![]() |
[65] |
A. G. Fletcher, P. J. Murray, P. K. Maini, Multiscale modelling of intestinal crypt organization and carcinogenesis, Math. Models Methods Appl. Sci., 25 (2015), 2563–2585. doi: 10.1142/S0218202515400187
![]() |
[66] |
R. D. L. Cruz, P. Guerrero, J. Calvo, T. Alarcón, Coarse-graining and hybrid methods for efficient simulation of stochastic multi-scale models of tumour growth, J. Comput. Physics, 350 (2017), 974–991. doi: 10.1016/j.jcp.2017.09.019
![]() |
[67] | A. R. A. Anderson, P. K. Maini, Mathematical oncology, Bull. Math. Biol., 80 (2018), 945–953. |
[68] | C. Surulescu, J. Kelkel, On some models for cancer cell migration through tissue networks, Math. Biosci. Eng., 8 (2012), 575–589. |
[69] |
H. L. Yang, J. Z. Lei, A mathematical model of chromosome recombination-induced drug resistance in cancer therapy, Math. Biosci. Eng., 16 (2019), 7098–7111. doi: 10.3934/mbe.2019356
![]() |
[70] |
The Cancer Genome Atlas Research Network, Comprehensive genomic characterization of squamous cell lung cancers, Nature, 489 (2012), 519–525. doi: 10.1038/nature11404
![]() |
[71] |
The Cancer Genome Atlas Research Network, Comprehensive molecular profiling of lung adenocarcinoma, Nature, 511 (2014), 543–550. doi: 10.1038/nature13385
![]() |
[72] |
G. J. Thomas, The cancer genome atlas research network: A sight to behold, Endocr. Pathol., 25 (2014), 362–365. doi: 10.1007/s12022-014-9345-4
![]() |
[73] |
N. Misra, E. Szczurek, M. Vingron, Inferring the paths of somatic evolution in cancer, Bioinformatics, 30 (2014), 2456–2463. doi: 10.1093/bioinformatics/btu319
![]() |
[74] |
D. Ramazzotti, G. Caravagna, L. O. Loohuis, A. Graudenzi, I. Kosunsky, A. Paroni, et al., CAPRI: efficient inference of cancer progression models from cross-sectional data, Bioinformatics, 31 (2015), 3016–3026. doi: 10.1093/bioinformatics/btv296
![]() |
[75] | G. Caravagna, A. Graudenzi, D. Ramazzotti, L. D. Sano, G. Mauri, V. Moreno, et al., Algorithmic methods to infer the evolutionary trajectories in cancer progression, Proc. Natl. Acad. Sci. U.S.A., 113 (2015), E4025. |
1. | Ge Song, Guizhen Liang, Tianhai Tian, Xinan Zhang, Mathematical Modeling and Analysis of Tumor Chemotherapy, 2022, 14, 2073-8994, 704, 10.3390/sym14040704 | |
2. | Anna Romaszko-Wojtowicz, Andżelika Lorenc, Adam Buciński, Anna Doboszyńska, Effects of Tobacco Smoking on the Survivability of Patients with Multiple Cancers and Single Lung Cancer, 2022, 19, 1660-4601, 9179, 10.3390/ijerph19159179 | |
3. | Zhenxing Li, Yu Shi, Zimeng Liu, Tiemao Shi, Identification of Urban Respiratory Health Risk Distribution Patterns and Spatial Environmental Impact Factors, 2022, 1556-5068, 10.2139/ssrn.4096146 | |
4. | Yanfei Zhang, Wei Feng, Zhiyuan Wu, Weiming Li, Lixin Tao, Xiangtong Liu, Feng Zhang, Yan Gao, Jian Huang, Xiuhua Guo, Deep-Learning Model of ResNet Combined with CBAM for Malignant–Benign Pulmonary Nodules Classification on Computed Tomography Images, 2023, 59, 1648-9144, 1088, 10.3390/medicina59061088 | |
5. | Yian Wang, Qijia Yan, Chunmei Fan, Yongzhen Mo, Yumin Wang, Xiayu Li, Qianjin Liao, Can Guo, Guiyuan Li, Zhaoyang Zeng, Wei Xiong, He Huang, Overview and countermeasures of cancer burden in China, 2023, 66, 1674-7305, 2515, 10.1007/s11427-022-2240-6 | |
6. | Qiong Ma, Xiao Zeng, Xueke Li, Liting You, Xi Fu, Fengming You, Yifeng Ren, Current status and prospects of the relationship between oral microbiome and the occurrence and development of lung cancer, 2024, 69, 0023-074X, 381, 10.1360/TB-2023-0474 | |
7. | Ronghao Zhu, Jing Huang, Fenhong Qian, The role of tumor-associated macrophages in lung cancer, 2025, 16, 1664-3224, 10.3389/fimmu.2025.1556209 | |
8. | Enas S. Alsatari, Kelly R. Smith, Sapthala P. Loku Galappaththi, Elba A. Turbat-Herrera, Santanu Dasgupta, The Current Roadmap of Lung Cancer Biology, Genomics and Racial Disparity, 2025, 26, 1422-0067, 3818, 10.3390/ijms26083818 |
Parameters | The data with radiation | The data without radiation | |||
Male | Female | Male | Female | ||
ν0Nb | 0.003 | 0.002 | 0.015 | 0.005 | |
γ | 0.158 | 0.123 | 0.219 | 0.171 | |
bν1 | 1.524×10−6 | 6.597×10−6 | 1.440×10−7 | 5.52×10−7 |
Parameters | The data with radiation | The data without radiation | |||
Male | Female | Male | Female | ||
γ1,GI, γ1(≈b1−d1) | 0.160 | 0.128 | 0.219 | 0.209 | |
μ0, μ1 | 3.012×10−8 | 10−8 | 4.041×10−9 | 7.087×10−9 | |
μ0,GI, μ1,GI | 1.402×10−4 | 5.634×10−1 | 6.025×10−6 | 9.536×10−6 | |
υ0N | 1.147×10−3 | 1.466×10−6 | 1.456×10−7 | 6.729×10−8 | |
υ1 | 9.965×10−6 | 1.081×10−6 | 8.732×10−10 | 1.571×10−14 |
Parameters | The data with radiation | The data without radiation | |||
Male | Female | Male | Female | ||
ν0Nb | 0.003 | 0.002 | 0.015 | 0.005 | |
γ | 0.158 | 0.123 | 0.219 | 0.171 | |
bν1 | 1.524×10−6 | 6.597×10−6 | 1.440×10−7 | 5.52×10−7 |
Parameters | The data with radiation | The data without radiation | |||
Male | Female | Male | Female | ||
γ1,GI, γ1(≈b1−d1) | 0.160 | 0.128 | 0.219 | 0.209 | |
μ0, μ1 | 3.012×10−8 | 10−8 | 4.041×10−9 | 7.087×10−9 | |
μ0,GI, μ1,GI | 1.402×10−4 | 5.634×10−1 | 6.025×10−6 | 9.536×10−6 | |
υ0N | 1.147×10−3 | 1.466×10−6 | 1.456×10−7 | 6.729×10−8 | |
υ1 | 9.965×10−6 | 1.081×10−6 | 8.732×10−10 | 1.571×10−14 |