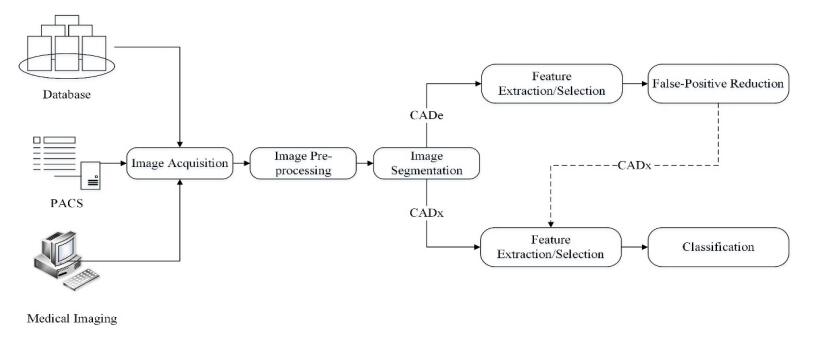
Citation: Muntaser Safan. Mathematical analysis of an SIR respiratory infection model with sex and gender disparity: special reference to influenza A[J]. Mathematical Biosciences and Engineering, 2019, 16(4): 2613-2649. doi: 10.3934/mbe.2019131
[1] | Haixia Hou, Yankun Cao, Xiaoxiao Cui, Zhi Liu, Hongji Xu, Cheng Wang, Wensheng Zhang, Yang Zhang, Yadong Fang, Yu Geng, Wei Liang, Tie Cai, Hong Lai . Medical image management and analysis system based on web for fungal keratitis images. Mathematical Biosciences and Engineering, 2021, 18(4): 3667-3679. doi: 10.3934/mbe.2021183 |
[2] | Tingxi Wen, Hanxiao Wu, Yu Du, Chuanbo Huang . Faster R-CNN with improved anchor box for cell recognition. Mathematical Biosciences and Engineering, 2020, 17(6): 7772-7786. doi: 10.3934/mbe.2020395 |
[3] | Eric Ke Wang, liu Xi, Ruipei Sun, Fan Wang, Leyun Pan, Caixia Cheng, Antonia Dimitrakopoulou-Srauss, Nie Zhe, Yueping Li . A new deep learning model for assisted diagnosis on electrocardiogram. Mathematical Biosciences and Engineering, 2019, 16(4): 2481-2491. doi: 10.3934/mbe.2019124 |
[4] | Binju Saju, Neethu Tressa, Rajesh Kumar Dhanaraj, Sumegh Tharewal, Jincy Chundamannil Mathew, Danilo Pelusi . Effective multi-class lungdisease classification using the hybridfeature engineering mechanism. Mathematical Biosciences and Engineering, 2023, 20(11): 20245-20273. doi: 10.3934/mbe.2023896 |
[5] | Zijian Wang, Yaqin Zhu, Haibo Shi, Yanting Zhang, Cairong Yan . A 3D multiscale view convolutional neural network with attention for mental disease diagnosis on MRI images. Mathematical Biosciences and Engineering, 2021, 18(5): 6978-6994. doi: 10.3934/mbe.2021347 |
[6] | Haiyan Song, Cuihong Liu, Shengnan Li, Peixiao Zhang . TS-GCN: A novel tumor segmentation method integrating transformer and GCN. Mathematical Biosciences and Engineering, 2023, 20(10): 18173-18190. doi: 10.3934/mbe.2023807 |
[7] | Xi Lu, Xuedong Zhu . Automatic segmentation of breast cancer histological images based on dual-path feature extraction network. Mathematical Biosciences and Engineering, 2022, 19(11): 11137-11153. doi: 10.3934/mbe.2022519 |
[8] | Eric Ke Wang, Nie Zhe, Yueping Li, Zuodong Liang, Xun Zhang, Juntao Yu, Yunming Ye . A sparse deep learning model for privacy attack on remote sensing images. Mathematical Biosciences and Engineering, 2019, 16(3): 1300-1312. doi: 10.3934/mbe.2019063 |
[9] | Vasileios E. Papageorgiou, Georgios Petmezas, Pantelis Dogoulis, Maxime Cordy, Nicos Maglaveras . Uncertainty CNNs: A path to enhanced medical image classification performance. Mathematical Biosciences and Engineering, 2025, 22(3): 528-553. doi: 10.3934/mbe.2025020 |
[10] | Bin Zhang, Linkun Sun, Yingjie Song, Weiping Shao, Yan Guo, Fang Yuan . DeepFireNet: A real-time video fire detection method based on multi-feature fusion. Mathematical Biosciences and Engineering, 2020, 17(6): 7804-7818. doi: 10.3934/mbe.2020397 |
Abbreviations: AUC: Area under the curve; BCDR: Breast Cancer Digital Repository; CAD: Computer-aided detection/diagnosis; CADe: Computer-aided detection; CADx: Computer-aided diagnosis; CNN(s): Convolutional neural network(s); CT: Computed tomography; DCNN(s): Deep convolutional neural network(s); DDSM: Digital Database for Screening Mammography; ILD: Interstitial lung disease; LIDC: Lung Image Database Consortium; LIDC-IDRI: Lung Image Database Consortium image collection; LUNA16: Lung Nodule Analysis 2016; MRI: Magnetic resonance imaging; MIAS: Mammography Image Analysis Society; NELSON: Nederlands-LeuvensLongkanker Screenings Onderzoek; ODNN: Optimal deep neural network; PROMISE12: Prostate MRI Image Segmentation 2012; PFMP: Prostate Fused-MRI-Pathology; ROC: Receiver operating characteristic; T2W: T2-weighted; WBCD: Wisconsin Breast Cancer Dataset
Medical imaging has become indispensable for the detection or diagnosis of diseases, especially for the diagnosis of cancers combined with a biopsy, and gradually become an important basis for precision medicine [1,2]. Currently, imaging techniques for medical applications are mainly based on X-rays, computed tomography (CT), magnetic resonance imaging (MRI), positron emission tomography (PET) and ultrasound [3].
However, with the development of science and technology and the promotion of medical imaging applications, data interpretation and analysis manually has gradually become a challenging task [4,5]. Radiologists may misinterpret diseases because of inexperience or fatigue, leading to missed diagnosis, that is, false negative results, non-lesions may be interpreted as lesions, or benign lesions may be misinterpreted as malignant, that is, false positive results [6,7,8,9,10,11,12,13]. According to statistics, the misdiagnosis rate caused by human in medical image analysis can reach 10–30% [14]. In this background, the CAD system can be a great helpful tool for radiologists in medical image analysis.
The CAD system was originally developed for breast cancer screening from mammograms in the 1960s [15,16]. Nowadays, it is one of the most important areas of research in the field of medical image analysis and radiomics. There are two important aspects in current CAD research: Computer-aided detection (CADe) and computer-aided diagnosis (CADx), respectively [17]. CADe takes advantage of the computer output to determine the location of suspicious lesions. CADx, on the other hand, gives an output that determines the characterization of lesions. The workflow of a typical CAD system (shown in Figure 1) in medical image analysis can be divided into four steps: Image pre-processing, segmentation, feature extraction and selection, lesion classification.
CAD systems are widely used for the detection and diagnosis of diseases in medical image analysis, such as breast cancer, lung cancer, prostate cancer, bone suppression, skin lesions, and Alzheimer's disease. The application of CAD systems can improve the accuracy of diagnosis, reduce time consumption, and optimize the radiologists' workloads [18,19,20,21,22,23,24].
Deep learning is a new technique that is overtaking the traditional machine learning techniques and is increasingly being used in CAD systems [25]. Generally, features are extracted manually in machine learning, while in deep learning, it is a completely automatic process. In addition, simple features such as colors, edges, and textures can be obtained in machine learning, while in deep learning, some hierarchical or compositional features are accessible during the training process.
Typically, deep learning methods can be divided into four categories: CNN-based methods, restricted Boltzmann machines (RBMs), autoencoders, and sparse coding. Recently, the CNN-based methods have attracted more and more attention around the world, which have achieved promising results in literature. A typical CNN framework (shown in Figure 2) is composed of one or more convolution layers and pooling layers (optional), followed by one or more fully connected layers [26].
Many CNN-based models have been proposed since LeNet-5 [27], such as AlexNet [28], VGG-Net (VGG-16 and VGG-19) [29], GoogLeNet [30], ResNet [31], and SPP-Net [32], which focus on increasing the network depths and designing more flexible structures. Deep convolutional neural network (DCNN), as a newly emerging form of medical image analysis, allows the automatic extraction of features and supervised learning of large scale datasets, leading to quantitative clinical decisions.
The application of CNN-based methods for medical images is quite different from those for natural images [33]. On the one hand, a large scale labeled dataset, for example, the ImageNet, is required for the training and testing of CNNs. On the other hand, medical images are usually grayscale instead of containing RGB channels. However, large scale medical image datasets are not always available due to the intensive labeling work and expert experience requirement.
In this paper, the current state-of-the-art deep learning techniques used in CAD research are presented in section 2, which focus on CNN-based methods. A summary of open available medical image databases and the most commonly used evaluation metrics in literature is listed in section 3. Challenges and future perspectives of CAD systems using CNN-based methods are summarized in section 4, followed by a brief conclusion.
Briefly speaking, conventional CAD systems consist of two different parts: Lesion detection and false-positive reduction. Lesion detection is primarily based on algorithms specific to the detection task, resulting in many candidate lesions. False-positive reduction is commonly based on traditional machine learning methods to reduce the false positive rate. However, even with these complicated and sophisticated programs, the general performance of conventional CAD systems is not good, thus hampering their widespread usage in clinical practice.
In contrast, deep learning techniques, particularly CNN-base methods, may provide us a single step solution of CAD. Additionally, the unique nature of transfer learning may accelerate the development of CAD systems for various diseases and different imaging modalities.
Early reports of CNN-based CAD systems for breast cancer [34], lung cancer [35] and Alzheimer's disease [36,37,38] have shown promising results regarding the performance in detecting and diagnosing diseases [39,40,41,42,43,44,45,46,47,48,49,50,51,52,53,54,55,56,57,58,59,60].
The primary goal of CADe is to increase the detection rate of diseases while reducing the false negative rate possibly due to the observers' mistakes or fatigue. In this overview, medical image analysis tasks such as segmentation, identification, localization and detection are considered as CADe [39,40,41,42,43,49,50,54,55].
In 2019, Fujita et al. designed a novel deep neural network architecture for the detection of fibrillations and flutters [39]. As the most common Arrhythmia clinically, fibrillations and flutters will increase the risk of heart failure, dementia, and stroke. In their work, the proposed CNN could effectively detect Arrhythmias without preprocessing on raw data.
With the purpose of identifying Parkinson's disease (PD) automatically, Luis et al. applied the recurrence plots to map the motor signals onto the image domain, which were further used to feed a CNN [40]. Experimental results showed significant improvement compared to their previous works with an average accuracy of over 87%.
Li et al. proposed an effective knowledge transfer method based on a small dataset from a local hospital and a large shared dataset from the Alzheimer's disease neuroimaging initiative, that is, transfer learning [41]. The detection accuracy of Alzheimer's disease increased by approximately 20% compared with that of a model simply based on the original small dataset. Since it is a common challenge in medical image analysis, the authors have provided a practical solution to the limited training data.
In 2018, Martin et al. developed a CADe system for the ureteral stones identification in CT slice volumes [42]. By using a CNN that worked directly on the high resolution CT volumes, the proposed method was evaluated on a large dataset from 465 patients with annotations performed by an expert radiologist. Finally, they achieved a sensitivity of 100% and an average of 2.68 false positives per patient.
Sajid et al. presented a DCNN architecture for the brain tumors segmentation in MRI images [43]. The proposed network consisted of multiple neural networks which were connected in sequential order with the feeding of convolutional feature maps at the peer level. Experimental results on BRATS 2015 benchmark data showed the effectiveness and superiority of their method.
In 2016, Shin et al. conducted experiments for thoraco-abdominal lymph node detection and to explore how the CNN performance changed according to factors of CNN architectures, dataset characteristics, and transfer learning [49]. They considered five different CNN architectures, which achieved state-of-the-art performances in various computer vision applications.
In 2015, Ronneberger et al. presented a network and training strategy for the segmentation of neuronal structures in electron microscopic stacks, which strongly depended on the data augmentation [50]. The proposed network was trained with very few images and superior to the previously developed methods in terms of the segmentation performance.
CADx not only involves the detection of suspicious lesions, but also the characterization and classification of the detected lesions. In this overview, medical image analysis tasks such as classification, characterization, recognition and diagnosis are considered as CADx [44,47,48,51,52,53,56,57,58,59].
In 2019, Ahmad et al. summarized the evidence for clinical applications of CADx and artificial intelligence in colonoscopy [51]. Artificial intelligence-based software could analyze video colonoscopy in the future only to support lesion detection and characterization, but also to assess technical quality.
Gonzxalez-Dxıaz et al. presented a CAD system called as DermaKNet for skin lesion diagnosis [52]. By incorporating the expert knowledge provided by dermatologists into the decision process, the authors aimed to overcome the traditional limitation of deep learning regarding the interpretability of the results. This work indicated that multi-task losses will allow for fusing segmentation and diagnosis networks into end-to-end trainable architectures.
Jeyaraj et al. developed an automated and computer-aided oral cancer diagnosis system by investigating patient's hyperspectral images [53]. With the application of regression-based partitioned algorithm, an accuracy of 94.5%, a sensitivity of 94.0%, and a specificity of 98.0% was obtained in their work. Since there was no a necessity of expert knowledge, the proposed system was easily developed on simple workbench in practice.
In 2018, Raghavendra et al. trained an eighteen-layer CNN to extract robust features from the digital fundus images for the accurate diagnosis of glaucoma [54]. With a relatively small data set, they obtained an accuracy of 98.13%, which demonstrated the robustness of the proposed CAD system. Similar to Fujita's work in [39], the authors also presented a novel CAD system for the automatic characterization of heart diseases [55]. The main difference between their works lied in the specific approaches that they used for feature extraction or classification.
Hosseini-Asl et al.proposed a three dimensional CNN (3D-CNN) to improve the prediction of Alzheimer's disease, which could show generic features extracted from brain images, adapt to different domain datasets, and accurately classify subjects with improved fine-tuning method [56]. Experimental results on the ADNI dataset demonstrated the superior performance compared to other CNN-based methods and conventional classifiers.
Similarly, Farooq et al. used a DCNN-based pipeline for the diagnosis of Alzheimer's disease from MRI scans [57]. Since it was quite difficult to diagnose Alzheimer's disease in elderly people and required a highly discriminative feature representation for classification, deep learning techniques were capable of this work. Experimental results were performed on the ADNI dataset with an accuracy of 98.8%.
In 2017, two optimized massive-training artificial neural network (MTANN) architectures and four distinct CNN architectures with different depths were used in [60] for lung nodules detection and identification. The results with the sensitivity of 100% and 22.7 false positives per patient showed the superior performance of MTANN architectures than compared to CNN architectures.
In 2016, Anthimopoulos et al. adopted and evaluated a DCNN designed for the classification of interstitial lung disease (ILD) patterns [59]. To train and evaluate the scheme, they used a dataset of 14, 696 image patches, derived by 120 CT scans from different scanners and hospitals. In addition, their proposed method was the first DCNN designed for a specific medical problem. The classification performance with an accuracy of 85.5% indicated that CNNs can be effectively used for analyzing lung patterns.
Some recent applications of CNN-based methods for CAD research are summarized in Table 1.
Reference | Year | Application | Method | Dataset | Result |
Fujita et al. [39] | 2019 | Fibrillations and flutters detection | DCNN | Physiobank(PTB) dataset | Accuracy: 98.45% Sensitvity: 99.87% Specificity: 99.27% |
Luis et al. [40] | 2019 | Parkinson's disease identification | CNN | HandPD dataset | Accuracy: 87% |
Gonzxalez-Dxıaz et al. [52] | 2019 | Skin lesion diagnosis | DermaKNet | 2017 ISBI Challenge | Specificity: 95% AUC: 0.917 |
Jeyaraj et al. [53] | 2019 | Oral cancer CAD system | DCNN | TCIA, GDC | Accuracy: 94.5% Sensitivity: 94.0% Specificity: 98.0% |
Martin et al. [42] | 2018 | Ureteral stone identification | CNN | Clinical | Sensitivity: 100% FP/scan: 2.68 |
Sajid et al. [43] | 2018 | Brain tumors segmentation | DCNN | BRATS 2015 | — |
Hosseini-Asl et al. [56] | 2018 | Alzheimer's disease diagnosis | 3D-CNN | ADNI dataset | Sensitivity: 76% F1-score: 0.75 |
Farooq et al. [57] | 2017 | Multi-class classification of Alzheimer's disease | CNN | ADNI dataset | Accuracy: 98.88% |
Liao et al. [44] | 2017 | Lung nodules diagnosis | A modified U-Net | Kaggle Data Science Bowl of 2017 | Accuracy: 81.42% Recall: 85.62% |
Tulder et al. [58] | 2016 | Lung CT images classification | Convolutional RBM | ILD CT scans | Accuracy: 89.0% |
Anthimopoulos et al. [59] | 2016 | Lung pattern classification | DCNN | ILD CT scans | Accuracy: 85.5% |
Pratt et al. [47] | 2016 | Diabetic retinopathy diagnosis | CNN | Kaggle Data Science Bowl of 2016 | Accuracy: 75.0% Sensitivity: 95.0% |
Gao et al. [48] | 2016 | Lung CT attenuation patterns classification | CNN | ILD CT scans | Accuracy: 87.9% |
Shin et al. [49] | 2016 | Thoraco-abdominal lymph node(LN) detection | DCNN | CT scans | AUC: 0.93–0.95 |
Ronneberger et al. [50] | 2015 | Biomedical image segmentation | CNN | ISBI challenge | Accuracy: 92.03% |
To the researchers, CNN-based methods are actively used for tasks such as classification, localization, segmentation and registration in medical image analysis. To the clinicians and radiologists, it is not the separation or combination of these tasks but the incorporation of them with a unified system that plays a significant role in clinical applications, known as the CAD systems.
According to a recent survey with bibliometric analysis, current CAD researches covered a wide range of diseases [16]. In this section, the latest clinical applications of CNN-based methods for CAD research are introduced, including breast cancer diagnosis, lung nodule detection and prostate cancer localization.
Breast cancer is one of the most common cancers for women. Thousands of women suffer from breast cancer around the world every year [61]. It was also predicted that there would be 19.3 million new cancer cases worldwide by 2025 [62]. The early detection and diagnosis can decrease the death rate of breast cancer significantly.
There have been numerous studies investigating the application of CAD systems for breast cancer detection and diagnosis, which used various medical imaging modalities and CNN-based methods [63,64,65,66,67,68,69,70,71,72,73,74,75,76].
In 2019, Chiang et al. proposed a fast and effective breast cancer CAD system based on 3D-CNN [63]. On evaluation with a test set of 171 tumors, the authors achieved sensitivities of 95%, 90%, 85% and 80% at 14.03, 6.92, 4.91 and 3.62 false positives per patient (with six passes), respectively. The results indicated the feasibility of their methods, however, the number of false positives at 100% sensitivity should be further reduced.
Samala et al. developed a DCNN for the classification of malignant and benign masses in digital breast tomosynthesis (DBT) [64]. This work demonstrated that multi-stage transfer learning could take advantage of the knowledge gained through source tasks from unrelated and related domains. Also, when the training sample size was limited, an additional stage of transfer learning was advantageous.
In 2018, Zhou et al. presented a segmentation-free method to classify benign and malignant breast tumors using CNNs [72]. With the proposed model trained on 540 images, an accuracy of 95.8%, a sensitivity of 96.2%, and a specificity of 95.7% was obtained, which was a promising result. Besides, it was the first attempt that made use of radiomics based on CNN to automatically extract high-throughput features from shear-wave elastography (SWE) data to classify breast tumors.
Gao et al. compared one hand-crafted feature extractor and five transfer learning feature extractors based on deep learning for breast cancer histology images classification [73]. The average accuracy was improved to 82.90% when using the five transfer learning feature groups.
In 2017, Li et al. established a 3D-CNN to discriminate between benign and malignant breast tumors [74]. The results with an accuracy of 78.1%, a sensitivity of 74.4% and a specificity of 82.3% demonstrated that 3D-CNN methods could be a promising technology for breast cancer classification without manual feature extraction.
Kooi et al. provided a head-to-head comparison between the state-of-the-art algorithms in mammography CAD systems [75]. A reader study was also performed, indicating that there was no significant difference between the proposed network and radiologists in terms of the detection and diagnosis accuracy.
In 2016, Samala et al. designed a DCNN to differentiate microcalcification candidates detected during the prescreening stage in a CAD system for clustered microcalcification [76]. As a validation, the selected DCNN was compared with their previously designed CNN architectures. The AUC of CNN and DCNN was 0.89 and 0.93, respectively (p < 0.05, which was statistically significant).
Some recent applications of CNN-based methods for breast cancer diagnosis are summarized in Table 2.
Reference | Year | Application | Method | Dataset | Result |
Chiang et al. [63] | 2019 | Breast ultrasound CAD system | 3D-CNN | Clinical | Sensitivity: 95.0% FP/scan: 14.03 |
Samala et al. [64] | 2019 | Breast masses classification | DCNN, transfer learning | DDSM | AUC: 0.85 ± 0.05 for single-stage transfer learning AUC: 0.91 ± 0.03 for multi-stage transfer learning |
Gao et al. [73] | 2018 | Breast cancer diagnosis | Shallow-Deep CNN(SD-CNN) | BCDR | Accuracy: 82.9% |
Zhou et al. [72] | 2018 | Breast tumors classification | CNN | Clinical | Accuracy: 95.8% Sensitivity: 96.2% Specificity: 95.7% |
Kooi et al. [75] | 2017 | Breast mammography CAD system | CNN | Clinical | AUC: 0.875–0.941 |
Becker et al. [65] | 2017 | Breast cancer detection | ANN | Clinical | AUC: 0.82 |
Li et al. [74] | 2017 | Breast tumors classification | 3D-CNN | Clinical | Accuracy: 78.1% Sensitivity: 74.4% Specificity: 82.3% AUC: 0.801 |
Zhou et al. [66] | 2017 | Breast tissue density classification | AlexNet | Clinical | Accuracy: 76% |
Kooi et al. [67] | 2017 | Masses discrimination | DCNNs | WBCD | AUC: 0.80 |
Ayelet et al. [68] | 2016 | Breast tumors detection and classification | Faster R-CNN | Clinical | Accuracy: 77% AUC: 0.72 |
Posada et al. [69] | 2016 | Breast cancer detection and diagnosis | AlexNet, VGGNet | MIAS | Accuracy: 60.01% and 64.52%, respectively |
Samala et al. [70] | 2016 | Digital breast tomosynthesis(DBT) CAD system | DCNN | Clinical | AUC: 0.90 |
Dhungel et al. [71] | 2016 | Masses classification | CNN | DDSM | Accuracy: 84.00% ± 4.00% |
Samala et al. [76] | 2016 | Breast CAD system | CNN, DCNN | Clinical | AUC: 0.89 and 0.93, respectively |
Lung cancer is one of the most frequent and leading causes to death all over the world. It was reported that there were approximately 1.8×106 new cases of lung cancer globally in 2012 [77,78]. Early detection of lung cancer, which is typically viewed in the form of lung nodules, is an efficient way to improve the survival rate.
The objectives in literature focusing on lung CAD systems can be divided into two categories: Lung nodules detection and classification [79,80,81,82,83,84,85,86,87,88,89,90,91]. The Lung Image Database Consortium (LIDC) and Lung Image Database Consortium image collection (LIDC-IDRI) are the most commonly used databases for the validation of experimental results.
In 2019, Shi et al. proposed a DCNN-based transfer learning method for the false-positive reduction of lung nodules detection [80]. The VGG-16 was adopted to extract discriminative features and the SVM was used to classify lung nodules. A sensitivity of 87.2% with 0.39 false positives per scan was reached, which was higher than other methods.
Savitha et al. analyzed the lung CT scan images using the optimal deep neural network (ODNN) and linear discriminate analysis (LDA) [83]. To detect and identify the lung cancer, the authors used a combination of ODNN and modified gravitational search algorithm (MGSA). An accuracy of 94.56%, a sensitivity of 96.2% and a specificity of 94.2% was given in the comparative results.
In 2018, Nishio et al. developed a CADx system to classify lung cancers between benign nodule, primary lung cancer, and metastatic lung cancer [84]. The proposed system was validated using different combinations of methods: Conventional machine learning classifiers, DCNN-based method with transfer learning, and DCNN-based method without transfer learning. In addition, they found that larger image size as input to train a DCNN improved the performance of lung nodules classification.
Dey et al. introduced a lung nodules classification method with high performance, which combined a three dimensional DCNN (3D-DCNN) and an ensemble method [89]. Compared to the shallow 3D-CNN architectures used in previous studies, the proposed 3D-DCNN could capture the features of spherical-shaped nodules more effectively.
In 2017, Anton et al. evaluated the effectiveness of a novel DCNN architecture for lung nodule malignancy classification [90]. The evaluation was based on the state-of-the-art ResNet architecture in their work. Further, the authors explored how the curriculum learning, transfer learning and varying network depth influenced the accuracy of malignancy classification.
In 2016, Li et al. designed a DCNN-based method for lung nodules classification, which had an advantage of automatic representation learning and strong generalization ability [91]. The DCNNs were trained by 62, 492 regions of interests (ROIs) samples including 40, 772 nodules and 21, 720 non-nodules from the LIDC database.
Some recent applications of CNN-based methods for lung nodules detection are summarized in Table 3.
Reference | Year | Application | Method | Dataset | Result |
Shi et al. [80] | 2019 | Lung nodules detection | VGG-16 | CT scans | Sensitivity: 87.2% FP/scan: 0.39 |
Savitha et al. [83] | 2019 | Lung cancers classification | Optimal deep neural network(ODNN) | Clinical | Accuracy: 94.56% Sensitivity: 96.20% Specificity: 94.20% |
Zhao et al. [84] | 2018 | Lung nodules classification | LeNet, AlexNet | LIDC | Accuracy: 82.20% AUC: 0.877 |
Dey et al. [89] | 2018 | Lung nodules classification | 3D-DCNN | LUNA16 | Competition performance metric(CPM): 0.910 |
Nishio et al. [82] | 2018 | Lung cancer CAD system | DCNN | Clinical | Accuracy: 68% |
Anton et al. [90] | 2017 | Lung CT CAD system | ResNet | LIDC-IDRI | Sensitivity: 91.07% Accuracy: 89.90% |
Ding et al. [85] | 2017 | Lung CAD system | DCNNs | LUNA16 | Sensitivity: 94.60% FROC: 0.893 |
Dou et al. [86] | 2017 | Lung nodules detection | 3D-CNN | LUNA16 | Sensitivity: 90.50% FP/scan: 1.0 |
Cheng et al. [87] | 2016 | Lung lesions classification | OverFeat | LIDC | Sensitivity: 90.80% ± 5.30 |
Li et al. [91] | 2016 | Lung nodules classification | DCNN | LIDC | Sensitivity: 87.10% FP/scan: 4.62 |
Liu et al. [88] | 2016 | Lung nodules classification | Multi-view CNN(MV-CNN) | LIDC-IDRI | Error rate: 5.41% Sensitivity: 90.49% Specificity: 99.91% |
Hua et al. [79] | 2015 | Lung nodules classification | CNN | LIDC | Sensitivity: 73.30% Specificity: 78.7% |
Prostate cancer is the most common malignancies among men and remains a second leading cause to deaths in men globally [92,93]. It was predicted that there would be 1.7 million new cancer cases by 2030. The early detection and diagnosis of prostate cancer can help to survive nine out of 10 men for the last five years.
Since it is a newly emerging area of research, more and more researchers pay attention to the prostate cancer detection, localization and diagnosis using CNN-based methods in CAD systems [94,95,96,97,98,99,100,101,102,103,104,105,106,107].
In 2019, Li et al. showed a new region-based CNN (R-CNN) framework for multi-task prediction of prostate cancer using an Epithelial Network Head and a Grading Network Head [95]. As a result, they achieved an accuracy of 99.07% and an average AUC of 0.998, which was the state-of-the-art performance in epithelial cells detection and Gleason grading tasks simultaneously. This work would help the pathologists to make the diagnosis more efficiently in the near future.
Leng et al. designed a framework for automatic identification of prostate cancer from colorimetric analysis of H & E and IHC-stained histopathological specimens [96]. The methods introduced in their work could be modularly integrated into digital pathology frameworks for detection of prostate cancer on whole slide images of histopathology slides. In addition, the proposed methods could be extended naturally to other related cancers as well.
In 2018, Chen et al. demonstrated that the state-of-the-art deep neural network could be retrained quickly with limited data provided by the PROSTATEx challenge [97]. They used inception V3 and VGG-16 which were pre-trained on the ImageNet, and obtained an AUC of 0.81 and 0.83, respectively. Also, compounding results from models trained with different image combination could improve the classification performance.
Song et al. proposed an automatic approach based on DCNN, inspired from VGG-Net, to differentiate prostate cancer and noncancerous tissues in multi-parametric MRI images using the PROSTATEx database [98].Further, Wang et al. improved this network by modifying a term in the loss function during the back-propagation process [99].
In 2017, Rampun et al. proposed a prostate cancer CAD system and suggested a set of discriminative texture descriptors extracted from T2-weighted (T2W) MRI images [94]. To test and evaluate their method, the authors collected 418 samples from 45 patients using 9-fold cross validation approach. Experimental results indicated that it was comparable among existing CAD systems using multimodality MRI.
Le et al. presented an automated method based on multimodal CNNs for two prostate cancer diagnostic tasks [106]. In the first phase, the proposed network aimed to classify cancerous and noncancerous tissues, while in the second phase, it was used for the differentiation between clinically significant prostate cancer and indolent prostate cancer. Finally, the authors obtained the promising results with a sensitivity of 89.85% and a specificity of 95.83% for prostate tissues classification, as well as a sensitivity of 100% and a specificity of 76.92% for prostate cancer characterization, respectively.
Yang et al. introduced an automated method for the prostate cancer localization in multi-parametric MRI images and assessed the agammaessiveness of detected lesions using multimodal multi-label CNNs [107]. Comprehensive evaluation demonstrated that the proposed method was superior to other networks in terms of the representative features extraction.
Some recent applications of CNN-based methods for prostate cancer localization are summarized in Table 4.
Reference | Year | Application | Method | Dataset | Result |
Li et al. [95] | 2019 | Prostate cancer grading | R-CNN | Clinical | Accuracy: 89.4% |
Wang et al. [99] | 2018 | Clinically significant prostate cancer CAD system | Dual-path CNN | Clinical | Sensitivity: 89.78% FP/scan: 1 |
Ishioka et al. [100] | 2018 | Prostate cancer CAD system | DCNN | Clinical (two training datasets: = 301, = 34) | AUC: 0.645 and 0.636, respectively |
Song et al. [98] | 2018 | Prostate cancer CADx | DCNN | PROSTATEx | Sensitivity: 87% Specificity: 90.6% AUC: 0.944 |
Chen et al. [97] | 2018 | Clinically significant prostate cancer classification | Inception V3, VGG-16 | PROSTATEx | AUC: 0.81 and 0.83 |
Kohl et al. [101] | 2017 | Prostate cancer detection | Fully convolutional networks (FCNs) | Clinical | Sensitivity: 55% Specificity: 98% |
Yang et al. [102] | 2017 | Prostate cancer detection | Co-trained CNNs | Clinical | Sensitivity: 46.00%, 92.00% and 97.00% FP/scan: 0.1, 1 and 10 |
Yang et al. [107] | 2017 | Prostate cancer localization and characterization | Multimodal multi-label CNNs | Clinical | Sensitivity: 98.6% Specificity: 98.3% Accuracy: 98.5% AUC: 0.998 |
Jin et al. [103] | 2017 | Prostate cancer detection | CNN | PROMISE12 | AUC: 0.974 |
Wang et al. [104] | 2017 | Prostate cancer detection | DCNN | PFMP | AUC: 0.84 |
Le et al. [106] | 2017 | Prostate cancer diagnosis | Multimodal CNNs | Clinical | Sensitivity: 89.85% Specificity: 95.83% |
Liu et al. [105] | 2017 | Prostate cancer lesions classification | XmasNet | PROSTATEx | AUC: 0.84 |
A well-characterized repository plays an important role in the performance evaluation of a CAD system [108]. Most of the researchers collect clinical data from different hospitals, which is a time-consuming and intensive process. Besides, extra work is required to normalize these images. Therefore, it is necessary to develop a standard database for the effective and objective performance evaluation among CAD systems [109].
In this section, some open available medical image databases are introduced, which are commonly used for breast cancer diagnosis, lung nodule detection and prostate cancer localization in literature, respectively.
The Mammography Image Analysis Society (MIAS) database contains left and right breast images from 161 patients with a total of 322 images, including 208 normal, 63 benign and 51 malignant (abnormal) cases [110,111]. For each X-ray film, it is associated with some medical information like lesion location, image scale, and malignancy annotated by experienced radiologists.
The Digital Database for Screening Mammography (DDSM) is the largest public breast image database, which consists of 2, 620 cases with a total of 10, 480 images including two images from each breast [112,113]. Each case is associated with some patient information, like age at the time of examination, subtlety rating for abnormalities and so on. Researchers have got satisfactory results using this database [114,115].
The Wisconsin Breast Cancer Dataset (WBCD) is publically available from the UCI Machine Learning Repository used for the validation of various classification algorithms [116]. There are 569 instances associated with 32 attributes in this database, which are collected from the Fine Needle Aspirates (FNA) of human breast tissues.
The Breast Cancer Digital Repository (BCDR) is the first Portuguese digital mammogram database [117]. It has at present a total of 1, 010 cases including digital content (3, 703 digitized film mammography images) and associated metadata. Precise segmentations of identified lesions (manual contours made by medical specialists) are also provided. Currently, two repositories are available for public domain: One containing digitalized film mammography, known as the BCDR-FM, and the other containing full field digital mammography, known as the BCDR-DM. Also, four benchmarking datasets representatives of benign and malignant lesions are available for free download to registered users.
A brief summary of these databases is shown in Table 5.
Database | Image modality | No. of patients | No. of benign samples | No. of malignant samples | No. of normal samples | Link |
MIAS | X-rays | 161 | 63 | 51 | 208 | http://www.wiau.man.ac.uk/services/MIAS/MIASweb.html |
WBCD | Mammography | 569 | 357 | 212 | - | http://marathon.csee.usf.edu/ Mammography/Database.html |
DDSM | Mammography | 2, 620 | 4, 044 | 3, 656 | 2, 780 | https://archive.ics.uci.edu/ml/datasets/Breast+Cancer+Wisconsin+%28Diagnostic%29 |
BCDR | Ultrasound | 1, 010 | - | - | 3, 703 | http://bcdr.inegi.up.pt/ |
The LIDC-IDRI database contains 1, 018 CT scans from 1, 010 patients with a total of 244, 527 images, which includes various imaging modalities like CT, digital radiography (DX), and computed radiography (CR) [118,119]. Each scan is associated with a XML file that records the annotations such as nodule ID, non-nodule ID, and reading sessions, performed by four expert radiologists.
The Lung Nodule Analysis 2016 (LUNA16) is one of the most commonly used database for lung cancer detection or diagnosis [120]. There are 888 CT scans with a total of 272 lung images in this database, and each scan is associated with the location of lesions as well as the image size. It is worth mentioning that the CT scans in this database are taken from the LIDC-IDRI database with the tumors smaller than 3mm are removed.
The Nederlands-LeuvensLongkanker Screenings Onderzoek (NELSON) database is usually employed to investigate lung nodule measurement, automatic detection and segmentation [121,122]. The ANODE09 database, which originates from the NELSON database, contains 55 anonymized thoracic CT scans [123].
The Japanese Society of Radiological Technology (JSRT) database has been used for various medical applications such as image pre-processing, image compression CAD system as well as picture archiving and communication system (PACS). Each sample in this database is associated with some clinical information such as patient age, gender, benign or malignant, and degree of subtlety in visual detection of nodules.
A brief summary of these databases is shown in Table 6.
Database | Image modality | No. of scans | No. of slices | No. of images | Link |
LIDC-IDRI | CT, DX, CR | 1, 018 | ─ | 244, 527 | https://wiki.cancerimagingarchive.net/display/Public/LIDC-IDRI |
LUNA16 | CT | 888 | 1, 084 | 272 | https://luna16.grand-challenge.org/download/ |
ANODE09 | CT | 55 | 451.5 | ─ | https://www.rug.nl/research/portal/datasets/nederlandsleuvens-longkanker-screenings-onderzoek-nelson.html |
JSRT | X-rays | ─ | ─ | 247 | http://db.jsrt.or.jp/eng.php |
The PROSTATEx Challenge focuses on the diagnostic classification of clinically significant prostate cancer in a quantitative way [124]. This collection is a retrospective set of prostate MRI studies, which include T2W, dynamic contrast enhanced (DCE), and diffusion-weighted (DW) imaging. It contains 349 studies from 346 patients with a total of 309, 251 images acquired without an endorectal coil.
The Prostate MRI Image Segmentation 2012 (PROMISE12) challenge aims to compare interactive and (semi)-automatic segmentation algorithms of the prostate in MRI images. Patients with benign diseases, for example, benign prostatic hyperplasia, and prostate cancer are both covered in this database. Additionally, data is collected from multiple medical centers with multimodality MRI images for the purpose of robustness and generalization testing.
The Cancer Imaging Archive Prostate Fused-MRI-Pathology (PFMP) dataset comprises a total of 28 T1-weighted (T1W), T2W, DW, and DCE prostate MRI along with digitized histopathology images of corresponding radical prostatectomy specimens, which are acquired on a 3.0T Siemens TrioTim [125]. The MRI scans also have a mapping of extent of prostate cancer on them.
The Prostate-3T dataset provides prostate transversal T2W MRI images acquired on a 3.0T Siemens TrioTim using only a pelvic phased-array coil, which is commonly used for prostate cancer detection [126]. It was used for TCIA as part of an ISBI challenge competition in 2013. There are 64 cases with a total of 1, 258 images in this dataset.
A brief summary of these databases is shown in Table 7.
Database | Image modality | No. of cases | No. of images | Link |
PROSTATEx | MRI | 346 | 309, 251 | https://wiki.cancerimagingarchive.net/display/Public/SPIE-AAPM-NCI+PROSTATEx+Challenges |
PFMP | MRI | 28 | 32, 508 | https://pathology.cancerimagingarchive.net/pathdata/ |
PROMISE12 | MRI | 50 | ─ | https://promise12.grand-challenge.org/Download/ |
Prostate-3T | MRI | 64 | 1, 258 | https://wiki.cancerimagingarchive.net/display/Public/Prostate-3T |
The CAD system performance is evaluated by various metrics such as accuracy, precision, sensitivity, specificity, F1-score, recall, true positive rate (TPR), false positive rate (FPR), dice-coefficient, receiver operating characteristic (ROC) curve and area under the curve (AUC), etc.. The calculation formula of the most commonly used evaluation metrics in literature are summarized in Table 8.
Metric | Calculation Formula | |
accuracy | accuracy=(TP+TN)(TP+TN+FP+FN) | Eq 1 |
precision | precision=TP(TP+FP) | Eq 2 |
sensitivity | sensitivity=TP(TP+FN) | Eq 3 |
specificity | specificity=TN(TN+FP) | Eq 4 |
F1−score | F1−score=2×(precision×recall)(precision+recall) | Eq 5 |
TPR | TPR=TP(TP+FN) | Eq 6 |
FPR | FPR=FP(TN+FP) | Eq 7 |
dice−coefficient | dice−coefficient=2×|P∩GT||P|+|GT| | Eq 8 |
Recently, more and more CAD systems have been developed for various diseases in medical image analysis. However, due to the complex structure of medical images and difficulty in establishing a standard library of biomedical signs, there are challenges in CAD research.
Firstly, the sample size with valid annotation is too small. On the one hand, it is of high cost and time consuming for radiologists or clinicians to label the medical images. On the other hand, the coverage of existed image libraries is not comprehensive. Secondly, the standardization of datasets and evaluation metrics is needed. Currently, most CAD systems are proposed based on various open available medical image databases, and there is no a standard for the performance evaluation. Obviously, it is very difficult to make a correct and reliable comparison among these systems. Thirdly, there are many difficulties in applying CAD systems to clinical use. Since the daily tasks of radiologists or clinicians are heavy, and the interface of the medical imaging system is not open to the public, it seems unpractical for the developed CAD systems to integrate seamlessly with other systems used in hospitals.
Many different CNN architectures have been proposed or adopted for medical image analysis, typically for image segmentation and classification tasks [127,128].
In [129], Chen et al. proposed a 2D bridged U-Net for the prostate segmentation. As a modified U-Net, the exponential ReLU, as an alternative of ReLU, and the Dice loss, as one of the pervasive loss functions, was adopted. In [130], Milletari et al. developed a V-Net for volumetric medical image segmentation. Despite the popularity of CNN-based methods, they could only be used for 2D images processing while most medical image data used in clinical practice consisted of 3D volumes.
In [131], Hussain et al. implemented an automated brain tumor segmentation algorithm using DCNN. The state-of-the-art neural network optimization strategies, such as dropout and batch normalization, are used in their work. Besides, the authors adopted the non-linear activation and inception module to build a new ILinear nexus architecture.
Generally, these architectures mainly focus on reducing the parameter space, saving computational time and dealing with 3D modalities. How to choose a more suited network architecture according to various medical image analysis tasks needs further research.
As it is known to all, deep learning architectures require a large amount of training data. Besides, most deep learning techniques, for example, CNN-based methods, require labeled data for supervised learning, which is difficult and time-consuming clinically. How to take the best advantage of limited data for training and how to train deeper networks effectively remains to be addressed.
There are two widely used solutions in literature that can partially deal with the mentioned problem. The first one is data augmentation, which uses the affine transformations such as translation, rotation, and scaling to generate more data from the existed data. The other is transfer learning, which has achieved promising results in medical image analysis [132,133]. The workflow of transfer learning is composed of two parts: Pre-trained on a large labeled dataset (such as ImageNet) and fine-tuning on the target dataset.
Despite the challenges associated with the introduction of deep learning methods and CAD systems in clinical settings, the promising results that are too valuable to discard.
Deep learning techniques extract knowledge from big data and produce an output that can be used for personalized treatment, which promotes the development of precision medicine. Unlike the conventional medical treatment, in precision medicine, the examination will go deep into the smallest molecular and genomic information, and medical staff will make the diagnostic decision according to the subtle differences among patients.
With the development of big data and medical imaging, radiomics comes into being [134]. By using a large number of medical images and feature-related algorithms, it aims to transform the region of interests into feature maps with high resolution. The standardized image acquisition, automated image analysis, radiomics of molecular images, and prognostic response evaluation are the key points in radiomics. At present, radiomics has been applied to the diagnosis, treatment, and prognosis of cancers, such as breast cancer and lung cancer, and has achieved promising results in literature.
In the future, medical image data will be linked more readily to non-imaging data in electronic medical records, such as gender, age, medical history and so on, known as imaging grouping. Deep learning techniques, when applied to electronic medical records, can help derive patient representations that may lead to predictions and augmentations of clinical decision support systems [135].
With the current rapid development of deep learning techniques, and CNN-based methods in particular, there are prospects for a more widespread application of CNN-based CAD systems in clinical practice. These techniques are not expected to replace the radiologists in the foreseeable future, but potentially facilitate the routine workflow, improve detection and diagnosis accuracy, reduce the probability of mistake and error, and enhance patients' satisfaction.
In this paper, an overview on CNN-based methods and their application in the field of CAD research is presented. It is concluded that CNN-based methods are increasingly being used in all sub-fields of medical image analysis, such as lesion segmentation, detection and classification. Despite the restrictions associated with data augmentation and transfer learning, they can be effectively used to deal with limited training data. Recent studies demonstrate that CNN-based methods in CAD research would greatly benefit the development of medical image analysis, and the future directions may towards radiomics, precision medicine and imaging grouping. This paper can provide researchers in medical image analysis with a systematic picture of the CNN-based methods used in CAD research.
This work was supported in part by grants from National Natural Science Foundation of China (Grant No. 61303099).
All authors declare no conflict of interest in this paper.
[1] | B. Hancioglu, D. Swigon and G. Clermont, A dynamical model of human immune response to influenza A virus infection, J. Theor. Biol., 246 (2007), 70–86. |
[2] | L. Mohler, D. Flockerzi, H. Sann, et al., Mathematical model of influenza A virus production in large-scale microcarrier culture, Biotechnol. Bioeng., 90 (2005), 46–58. |
[3] | C. J. Luke and K. Subbarao, Vaccines for pandemic influenza, Emerg. Infect. Dis., 12 (2006), 66–72. |
[4] | M. Erdem, M. Safan, C. Castillo-Chavez, Mathematical Analysis of an SIQR Influenza model with Imperfect quarantine, B. Math. Biol., 79 (2017), 1612–1636. |
[5] | A. Flahault, E. Vergu, L. Coudeville, et al., Strategies for containing a global influenza pandemic, Vaccine, 24 (2006), 6751–6755. |
[6] | B. J. Coburn, B. G.Wagner and S. Blower, Modeling influenza epidemics and pandemics: insights into the future of swine flu (H1N1), BMC Med., 7 (2009), 30–37. |
[7] | P. Y. Boelle, P. Bermillon, J. C. Desenclos, A preliminary estimation of the reproduction ratio for new influenza A(H1N1) from the outbreak in Mexico, March-April 2009, Eurosurveillance, 14 (2009), pii=19205. |
[8] | H. Nishiura, C. Castillo-Chavez, M. Safan, et al., Transmission potential of the new influenza A (H1N1) virus and its age-specificity in Japan, Eurosurveillance, 14 (2009), pii=19227. |
[9] | H. Nishiura H, G. Chowell, M. Safan, et al., Pros and cons of estimating the reproduction number from early epidemic growth rate of influenza A (H1N1) 2009, Theor. Biol. Med. Model., 7 (2010), 1–9. |
[10] | M. Nuno, Z. Feng, M. Martcheva, et al., Dynamics of two-strain influenza with isolation and partial cross-immunity, SIAM J. Appl. Math., 65 (2005), 964–982. |
[11] | A. L. Vivas-Barber, C. Castillo-Chavez and E. Barany, Dynamics of an "SAIQR" Influenza Model, Biomath., 3 (2014), 1–13. |
[12] | H. Hethcote, M. Zhien and L. Shengbing, Effects of quarantine in six endemic models for infectious diseases, Math. Biosci., 180 (2002), 141–160. |
[13] | A. Ruggieri, W. Malorni and W. Ricciardi, Gender disparity in response to anti-viral vaccines: new clues toward personalized vaccinology, Ital. J. Gender-Specific Med., 2 (2016), 93–98. |
[14] | S. L. Klein, A. Pekosz, C. Passaretti, et al., Sex, Gender and Influenza, World Health Organization, Geneva, (2010), 1–58. |
[15] | S. L. Klein and K. L. Flanagan, Sex differences in immune responses, Nat. Rev. Immunol., 16 (2016), 626–638. |
[16] | D. Furman, B. P. Hejblum, N. Simon, et al., Systems analysis of sex differences reveals an immunosuppressive role for testosterone in the response to influenza vaccination, P. Natl. Acad. Sci. USA, 111 (2014), 869–874. |
[17] | S. L. Klein, A. Hodgson and D. P. Robinson, Mechanisms of sex disparities in influenza pathogenesis, J. Leukoc. Biol., 92 (2012), 67–73. |
[18] | S. L. Klein, I. Marriott and E. N. Fish, Sex-based differences in immune function and responses to vaccination, Trans. R. Soc. Trop. Med. Hyg., 109 (2015), 9–15. |
[19] | A. Ruggieri, S. Anticoli, A. D'Ambrosio, et al., The influence of sex and gender on immunity, infection and vaccination, Ann. Ist. Super Sanità, 52 (2016), 198–204. |
[20] | A. L. Fink and S. Klein, Sex and Gender Impact Immune Responses to Vaccines Among the Elderly, Physiology, 30 (2015), 408–416. |
[21] | J. Fisher, N. Jung, N. Robinson, et al., Sex differences in immune responses to infectious diseases, Infection, 43 (2015), 399–403. |
[22] | S. L. Klein, Sex influences immune responses to viruses, and efficacy of prophylaxis and therapeutic treatments for viral diseases, Bioessays, 34 (2012), 1050–1059. |
[23] | J. V. Lunzen and M. Altfeld, Sex Differences in Infectious Diseases? Common but Neglected, J. Infect. Dis., 209(S3) (2014), S79–80. |
[24] | X. Tan, L. Yuan, J. Zhou, et al., Modelling the initial transmission dynamics of influenza A H1N1 in Guangdong Province, China, Int. J. Infect. Dis., 17 (2017), e479–e484. |
[25] | N. S. Cardell and D. E. Kanouse, Modeling heterogeneity in susceptibility and infectivity for HIV infection, in Mathematical and Statistical Approaches to AIDS Epidemiology, Lecture notes in biomathematics, 88 (eds. C. Castillo-Chavez), Springer-Verlag, Berlin Heidelberg New York London Paris Tokyo Hong Kong, (1989), 138–156. |
[26] | M. Safan and K. Dietz, On the eradicability of infections with partially protective vaccination in models with backward bifurcation, Math. Biosci. Eng., 6 (2009), 395–407. |
[27] | M. Safan, M Kretzschmar and K. P. Hadeler, Vaccination based control of infections in SIRS models with reinfection: special reference to pertussis, J. Math. Biol., 67 (2013), 1083–1110. |
[28] | O. Neyrolles and L. Quintana-Murci, Sexual Inequality in Tuberculosis, PLoS Med., 6 (2009), e1000199. https://doi.org/10.1371/journal.pmed.1000199. |
[29] | World Health Organization, Global tuberculosis control 2009: epidemiology, strategy, financing, Geneva: WHO, 2009. Available from: http://www.who.int/tb/country/en/index.html. |
[30] | European Centre for Disease Prevention and Control, Pertussis. In: ECDC. Annual epidemiological report for 2015. Stockholm: ECDC; 2017. |
[31] | World Health Organization (2018), Global Health Observatory (GHO) data: Number of women living with HIV, accessed 29 November 2018, http://www.who.int/gho/hiv/epidemic_status/cases_adults_women_children_text/en/. |
[32] | U.S. Department of Health & Human Services, Office on Women's Health (last updated 21 November 2018), Women and HIV, accessed 29 November 2018, https://www. womenshealth.gov/hiv-and-aids/women-and-hiv. |
[33] | Avert (last updated 21 August 2018), Global information and education on HIV and AIDS: Women and girls (HIV and AIDS), accessed 29 November 2018, https://www.avert.org/ professionals/hiv-social-issues/key-affected-populations/women. |
[34] | O. Diekmann, J. A. P. Heesterbeek and M. G. Roberts, The Construction of next-Generation Matrices for Compartmental Epidemic Models, J. R. Soc. Interface, 47 (2010), 873–885. |
[35] | H. Thieme, Mathematics in Population Biology, Princeton university press, Princeton, New Jersey, 2003. |
[36] | C. Castillo-Chavez, Z. Feng and W. Huang, On the computation of R0 and its role on global stability, in Mathematical Approaches for Emerging and Reemerging Infectious Diseases: An Introduction (eds. C. Castillo-Chavez, S. Blower, P. van den Driessche, D. Krirschner and A. A. Yakubu), The IMA Volumes in Mathematics and its Applications 125, Springer-Verlag, New York, (2002), 229–250. |
[37] | O. Patterson-Lomba, M. Safan, S. Towers, et al., Modeling the Role of Healthcare Access Inequalities in Epidemic Outcomes, Math. Biosci. Eng., 13 (2016), 1011–1041. |
[38] | United States Centers for Disease Control and Prevention (2011) A CDC framework for preventing infectious diseases: Sustaining the Essentials and Innovating for the Future, October 2011. |
[39] | World Health Organization. Evaluation of influenza vaccine effectiveness: a guide to the design and interpretation of observational studies, Geneva: World Health Organization, 2017. |
[40] | H. L. Smith and H. Thieme, Dynamical Systems and Population Persistence, AMS, 2011. |
[41] | M. Eichner, M. Schwehm, L. Eichner, et al., Direct and indirect effects of influenza vaccination, BMC Infect. Dis., 17 (2017), 308–315. |
1. | Rakesh Ranjan, Anupam Singh, Aliea Rizvi, Tejasvi Srivastava, 2020, Chapter 18, 978-981-15-3368-6, 235, 10.1007/978-981-15-3369-3_18 | |
2. | Kotaro Ito, Hirotaka Muraoka, Naohisa Hirahara, Eri Sawada, Shunya Okada, Takashi Kaneda, Quantitative assessment of normal submandibular glands and submandibular sialadenitis using CT texture analysis: A retrospective study, 2020, 22124403, 10.1016/j.oooo.2020.10.007 | |
3. | Roberto Arias, Fabián Narváez, Hugo Franco, 2020, Chapter 22, 978-3-030-46784-5, 273, 10.1007/978-3-030-46785-2_22 | |
4. | Giuseppe Cuccu, Johan Jobin, Julien Clement, Akansha Bhardwaj, Carolin Reischauer, Harriet Thony, Philippe Cudre-Mauroux, 2020, Hydra: Cancer Detection Leveraging Multiple Heads and Heterogeneous Datasets, 978-1-7281-6251-5, 4842, 10.1109/BigData50022.2020.9378042 | |
5. | Kotaro Ito, Hirotaka Muraoka, Naohisa Hirahara, Eri Sawada, Shunya Okada, Takashi Kaneda, Computed tomography texture analysis of mandibular condylar bone marrow in diabetes mellitus patients, 2021, 0911-6028, 10.1007/s11282-021-00517-7 | |
6. | Francesco De Logu, Filippo Ugolini, Vincenza Maio, Sara Simi, Antonio Cossu, Daniela Massi, Romina Nassini, Marco Laurino, Recognition of Cutaneous Melanoma on Digitized Histopathological Slides via Artificial Intelligence Algorithm, 2020, 10, 2234-943X, 10.3389/fonc.2020.01559 | |
7. | Simone Giovanni Gugliandolo, Matteo Pepa, Lars Johannes Isaksson, Giulia Marvaso, Sara Raimondi, Francesca Botta, Sara Gandini, Delia Ciardo, Stefania Volpe, Giulia Riva, Damari Patricia Rojas, Dario Zerini, Paola Pricolo, Sarah Alessi, Giuseppe Petralia, Paul Eugene Summers, Frnacesco Alessandro Mistretta, Stefano Luzzago, Federica Cattani, Ottavio De Cobelli, Enrico Cassano, Marta Cremonesi, Massimo Bellomi, Roberto Orecchia, Barbara Alicja Jereczek-Fossa, MRI-based radiomics signature for localized prostate cancer: a new clinical tool for cancer aggressiveness prediction? Sub-study of prospective phase II trial on ultra-hypofractionated radiotherapy (AIRC IG-13218), 2021, 31, 0938-7994, 716, 10.1007/s00330-020-07105-z | |
8. | Nikolaos Papandrianos, Elpiniki Papageorgiou, Athanasios Anagnostis, Anna Feleki, A Deep-Learning Approach for Diagnosis of Metastatic Breast Cancer in Bones from Whole-Body Scans, 2020, 10, 2076-3417, 997, 10.3390/app10030997 | |
9. | Lin Shui, Haoyu Ren, Xi Yang, Jian Li, Ziwei Chen, Cheng Yi, Hong Zhu, Pixian Shui, The Era of Radiogenomics in Precision Medicine: An Emerging Approach to Support Diagnosis, Treatment Decisions, and Prognostication in Oncology, 2021, 10, 2234-943X, 10.3389/fonc.2020.570465 | |
10. | Fan Fu, Jianyong Wei, Miao Zhang, Fan Yu, Yueting Xiao, Dongdong Rong, Yi Shan, Yan Li, Cheng Zhao, Fangzhou Liao, Zhenghan Yang, Yuehua Li, Yingmin Chen, Ximing Wang, Jie Lu, Rapid vessel segmentation and reconstruction of head and neck angiograms using 3D convolutional neural network, 2020, 11, 2041-1723, 10.1038/s41467-020-18606-2 | |
11. | Paarth Bir, Valentina E. Balas, 2020, A Review on Medical Image Analysis with Convolutional Neural Networks, 978-1-7281-5070-3, 870, 10.1109/GUCON48875.2020.9231203 | |
12. | Babu P. Mohan, Antonio Facciorusso, Shahab R. Khan, Saurabh Chandan, Lena L. Kassab, Paraskevas Gkolfakis, Georgios Tziatzios, Konstantinos Triantafyllou, Douglas G. Adler, Real-time computer aided colonoscopy versus standard colonoscopy for improving adenoma detection rate: A meta-analysis of randomized-controlled trials, 2020, 29-30, 25895370, 100622, 10.1016/j.eclinm.2020.100622 | |
13. | Hakan Özcan, Bülent Gürsel Emiroğlu, Hakan Sabuncuoğlu, Selçuk Özdoğan, Ahmet Soyer, Tahsin Saygı, A comparative study for glioma classification using deep convolutional neural networks, 2021, 18, 1551-0018, 1550, 10.3934/mbe.2021080 | |
14. | Utku Kose, Omer Deperlioglu, Jafar Alzubi, Bogdan Patrut, 2021, Chapter 2, 978-981-15-6324-9, 15, 10.1007/978-981-15-6325-6_2 | |
15. | Laith Alzubaidi, Jinglan Zhang, Amjad J. Humaidi, Ayad Al-Dujaili, Ye Duan, Omran Al-Shamma, J. Santamaría, Mohammed A. Fadhel, Muthana Al-Amidie, Laith Farhan, Review of deep learning: concepts, CNN architectures, challenges, applications, future directions, 2021, 8, 2196-1115, 10.1186/s40537-021-00444-8 | |
16. | Vegi Jeevana, B. Sai Ram Charan, Lung Cancer Prediction Using Chest X-Rays, 2021, 2395-602X, 236, 10.32628/IJSRST218236 | |
17. | Himanshu Pant, Manoj Chandra Lohani, Ashutosh Kumar Bhatt, Janmejay Pant, Rakesh Kumar Sharma, 2021, Thoracic Disease Detection using Deep Learning, 978-1-6654-0360-3, 1197, 10.1109/ICCMC51019.2021.9418343 | |
18. | Philipp Jansen, Adelaida Creosteanu, Viktor Matyas, Amrei Dilling, Ana Pina, Andrea Saggini, Tobias Schimming, Jennifer Landsberg, Birte Burgdorf, Sylvia Giaquinta, Hansgeorg Müller, Michael Emberger, Christian Rose, Lutz Schmitz, Cyrill Geraud, Dirk Schadendorf, Jörg Schaller, Maximilian Alber, Frederick Klauschen, Klaus G. Griewank, Deep Learning Assisted Diagnosis of Onychomycosis on Whole-Slide Images, 2022, 8, 2309-608X, 912, 10.3390/jof8090912 | |
19. | Shtwai Alsubai, A Critical Review on the 3D Cephalometric Analysis Using Machine Learning, 2022, 11, 2073-431X, 154, 10.3390/computers11110154 | |
20. | Zahid Ullah, Mona Jamjoom, A Deep Learning for Alzheimer’s Stages Detection Using Brain Images, 2023, 74, 1546-2226, 1457, 10.32604/cmc.2023.032752 | |
21. | Hanan Sharif, Faisal Rehman, Amina Rida, 2022, Deep Learning: Convolutional Neural Networks for Medical Image Analysis - A Quick Review, 978-1-6654-9819-7, 1, 10.1109/ICoDT255437.2022.9787469 | |
22. | Kumiko Tanaka, Taka-aki Nakada, Nozomi Takahashi, Takahiro Dozono, Yuichiro Yoshimura, Hajime Yokota, Takuro Horikoshi, Toshiya Nakaguchi, Koichiro Shinozaki, Superiority of Supervised Machine Learning on Reading Chest X-Rays in Intensive Care Units, 2021, 8, 2296-858X, 10.3389/fmed.2021.676277 | |
23. | Xiaonan Shi, Yong Deng, Yige Fang, Yajuan Chen, Ni Zeng, Limei Fu, Luminita Moraru, A Hemolysis Image Detection Method Based on GAN-CNN-ELM, 2022, 2022, 1748-6718, 1, 10.1155/2022/1558607 | |
24. | Thejus Shaji, K. Ravi, E. Vignesh, A. Sinduja, 2023, 124, 978-3-0364-1151-4, 111, 10.4028/p-52096g | |
25. | Junxiu Wang, Jianchao Zeng, Hongwei Li, Xiaoqing Yu, Enas Abdulhay, A Deep Learning Radiomics Analysis for Survival Prediction in Esophageal Cancer, 2022, 2022, 2040-2309, 1, 10.1155/2022/4034404 | |
26. | Yue Zhao, Jie Zhang, Dayu Hu, Hui Qu, Ye Tian, Xiaoyu Cui, Application of Deep Learning in Histopathology Images of Breast Cancer: A Review, 2022, 13, 2072-666X, 2197, 10.3390/mi13122197 | |
27. | Wang, MD Yaoting, Chai, MD Huihui, Ye, MD Ruizhong, Li, MD, PhD Jingzhi, Liu, MD Ji-Bin, Lin Chen, Peng, MD Chengzhong, Point-of-Care Ultrasound: New Concepts and Future Trends, 2021, 5, 2576-2516, 268, 10.37015/AUDT.2021.210023 | |
28. | Yan Zhang, Cuilan Gong, Ling Zheng, Xiaoyan Li, Xiaomei Yang, Enas Abdulhay, Deep Learning for Intelligent Recognition and Prediction of Endometrial Cancer, 2021, 2021, 2040-2309, 1, 10.1155/2021/1148309 | |
29. | Jahanzaib Latif, Shanshan Tu, Chuangbai Xiao, Sadaqat Ur Rehman, Mazhar Sadiq, Muhammad Farhan, Farhan Ullah, Digital Forensics Use Case for Glaucoma Detection Using Transfer Learning Based on Deep Convolutional Neural Networks, 2021, 2021, 1939-0122, 1, 10.1155/2021/4494447 | |
30. | Jakub Kluk, Marek R. Ogiela, AI Approaches in Computer-Aided Diagnosis and Recognition of Neoplastic Changes in MRI Brain Images, 2022, 12, 2076-3417, 11880, 10.3390/app122311880 | |
31. | Xiaochuan Li, Yuan Ke, 2022, Chapter 49, 978-3-031-12052-7, 663, 10.1007/978-3-031-12053-4_49 | |
32. | Kotaro Ito, Takumi Kondo, V. Carlota Andreu-Arasa, Baojun Li, Naohisa Hirahara, Hirotaka Muraoka, Osamu Sakai, Takashi Kaneda, Quantitative assessment of the maxillary sinusitis using computed tomography texture analysis: odontogenic vs non-odontogenic etiology, 2022, 38, 0911-6028, 315, 10.1007/s11282-021-00558-y | |
33. | Antonio Ferrer-Sánchez, Jose Bagan, Joan Vila-Francés, Rafael Magdalena-Benedito, Leticia Bagan-Debon, Prediction of the risk of cancer and the grade of dysplasia in leukoplakia lesions using deep learning, 2022, 132, 13688375, 105967, 10.1016/j.oraloncology.2022.105967 | |
34. | Mehmet A. Gulum, Christopher M. Trombley, Mehmed Kantardzic, A Review of Explainable Deep Learning Cancer Detection Models in Medical Imaging, 2021, 11, 2076-3417, 4573, 10.3390/app11104573 | |
35. | Yusik Cho, Alena Jalics, Ding Lv, Marissa Gilbert, Kathy Dickson, Dawei Chen, Thomas Nguyen, Hannah Joines, Brandon Kakos, Chaoyang Chen, Stephen Lemos, 2021, Predicting Rotator Cuff Tear Severity Using Radiographic Images and Machine Learning Techniques, 9781450390439, 237, 10.1145/3497623.3497661 | |
36. | Islam R. Abdelmaksoud, Ahmed Shalaby, Ali Mahmoud, Mohammed Elmogy, Ahmed Aboelfetouh, Mohamed Abou El-Ghar, Moumen El-Melegy, Norah Saleh Alghamdi, Ayman El-Baz, Precise Identification of Prostate Cancer from DWI Using Transfer Learning, 2021, 21, 1424-8220, 3664, 10.3390/s21113664 | |
37. | Babatunde S. Emmanuel, 2021, Improved Approach to Feature Dimension Reduction for Efficient Diagnostic Classification of Breast Cancer, 978-1-6654-3493-5, 1, 10.1109/ICMEAS52683.2021.9692388 | |
38. | Aneeqa Ijaz, Muhammad Nabeel, Usama Masood, Tahir Mahmood, Mydah Sajid Hashmi, Iryna Posokhova, Ali Rizwan, Ali Imran, Towards using cough for respiratory disease diagnosis by leveraging Artificial Intelligence: A survey, 2022, 29, 23529148, 100832, 10.1016/j.imu.2021.100832 | |
39. | Kotaro Ito, Mayu Kurasawa, Tadasu Sugimori, Hirotaka Muraoka, Naohisa Hirahara, Eri Sawada, Shinichi Negishi, Kazutaka Kasai, Takashi Kaneda, Risk assessment of external apical root resorption associated with orthodontic treatment using computed tomography texture analysis, 2023, 39, 0911-6028, 75, 10.1007/s11282-022-00604-3 | |
40. | Ange Lou, Shuyue Guan, Murray Loew, CFPNet-M: A light-weight encoder-decoder based network for multimodal biomedical image real-time segmentation, 2023, 154, 00104825, 106579, 10.1016/j.compbiomed.2023.106579 | |
41. | Chetan Gedam, Design and Development of AI based Approach for Histopathology Cancer Screening and Identification, 2022, 2581-9429, 104, 10.48175/IJARSCT-2241 | |
42. | Nagaraj Yamanakkanavar, Jae Young Choi, Bumshik Lee, Multiscale and Hierarchical Feature-Aggregation Network for Segmenting Medical Images, 2022, 22, 1424-8220, 3440, 10.3390/s22093440 | |
43. | Xinhui Wang, Long Zhou, Yaofa Wang, Haochuan Jiang, Hongwei Ye, Improved low-dose positron emission tomography image reconstruction using deep learned prior, 2021, 66, 0031-9155, 115001, 10.1088/1361-6560/abfa36 | |
44. | Louis Jaffeux, Alfons Schwarzenböck, Pierre Coutris, Christophe Duroure, Ice crystal images from optical array probes: classification with convolutional neural networks, 2022, 15, 1867-8548, 5141, 10.5194/amt-15-5141-2022 | |
45. | Asma Baccouche, Begonya Garcia-Zapirain, Cristian Castillo Olea, Adel S. Elmaghraby, Breast Lesions Detection and Classification via YOLO-Based Fusion Models, 2021, 69, 1546-2226, 1407, 10.32604/cmc.2021.018461 | |
46. | Hao Wen, Chang Huang, Shengmin Guo, The Application of Convolutional Neural Networks (CNNs) to Recognize Defects in 3D-Printed Parts, 2021, 14, 1996-1944, 2575, 10.3390/ma14102575 | |
47. | Ihsan Ullah, Farman Ali, Babar Shah, Shaker El-Sappagh, Tamer Abuhmed, Sang Hyun Park, A deep learning based dual encoder–decoder framework for anatomical structure segmentation in chest X-ray images, 2023, 13, 2045-2322, 10.1038/s41598-023-27815-w | |
48. | Qi Mao, Shuguang Zhao, Lijia Ren, Zhiwei Li, Dongbing Tong, Xing Yuan, Haibo Li, Intelligent immune clonal optimization algorithm for pulmonary nodule classification, 2021, 18, 1551-0018, 4146, 10.3934/mbe.2021208 | |
49. | Kaylie Cullison, Danilo Maziero, Benjamin Spieler, Eric A. Mellon, 2022, 8, 9780323916899, 211, 10.1016/B978-0-323-91689-9.00011-X | |
50. | Ruifang Qi, Kun Yang, Rongmin Li, Gustavo Ramirez, Deep Learning-Based Ultrasound Imaging Diagnosis for Gonadotropin-Releasing Hormone Agonists Treatment of Central Precocious Puberty, 2021, 2021, 1875-919X, 1, 10.1155/2021/4512506 | |
51. | Hong’an Li, Min Zhang, Dufeng Chen, Jing Zhang, Meng Yang, Zhanli Li, Image Color Rendering Based on Hinge-Cross-Entropy GAN in Internet of Medical Things, 2023, 135, 1526-1506, 779, 10.32604/cmes.2022.022369 | |
52. | Ruiyang Ren, Haozhe Luo, Chongying Su, Yang Yao, Wen Liao, Machine learning in dental, oral and craniofacial imaging: a review of recent progress, 2021, 9, 2167-8359, e11451, 10.7717/peerj.11451 | |
53. | Cheryl Angelica, Hendrik Purnama, Fredy Purnomo, 2021, Impact of Computer Vision With Deep Learning Approach in Medical Imaging Diagnosis, 978-1-6654-4002-8, 37, 10.1109/ICCSAI53272.2021.9609708 | |
54. | Anudeep Katrevula, Goutham Reddy Katukuri, Aniruddha Pratap Singh, Pradev Inavolu, Hardik Rughwani, Siddhartha Reddy Alla, Mohan Ramchandani, Nageshwar Reddy Duvvur, Real-World Experience of AI-Assisted Endocytoscopy Using EndoBRAIN—An Observational Study from a Tertiary Care Center, 2022, 0976-5042, 10.1055/s-0042-1758535 | |
55. | Daniele Corradini, Leonardo Brizi, Caterina Gaudiano, Lorenzo Bianchi, Emanuela Marcelli, Rita Golfieri, Riccardo Schiavina, Claudia Testa, Daniel Remondini, Challenges in the Use of Artificial Intelligence for Prostate Cancer Diagnosis from Multiparametric Imaging Data, 2021, 13, 2072-6694, 3944, 10.3390/cancers13163944 | |
56. | Huakun Yang, Qian Chen, Keren Fu, Lei Zhu, Lujia Jin, Bensheng Qiu, Qiushi Ren, Hongwei Du, Yanye Lu, Boosting medical image segmentation via conditional-synergistic convolution and lesion decoupling, 2022, 101, 08956111, 102110, 10.1016/j.compmedimag.2022.102110 | |
57. | Yu Xia, Fumei Xu, Naeem Jan, Design and Application of Machine Learning-Based Evaluation for University Music Teaching, 2022, 2022, 1563-5147, 1, 10.1155/2022/4081478 | |
58. | Dhasny Lydia M, Prakash M, 2022, Performance Evaluation of Convolutional Neural Network for Lung Cancer Detection, 978-1-6654-8385-8, 293, 10.1109/ICESIC53714.2022.9783533 | |
59. | Jiamin Liang, Xin Yang, Yuhao Huang, Haoming Li, Shuangchi He, Xindi Hu, Zejian Chen, Wufeng Xue, Jun Cheng, Dong Ni, Sketch guided and progressive growing GAN for realistic and editable ultrasound image synthesis, 2022, 79, 13618415, 102461, 10.1016/j.media.2022.102461 | |
60. | Laura Kocet, Katja Romarič, Janez Žibert, Automatic detection of Gibbs artefact in MR images with transfer learning approach, 2023, 31, 09287329, 239, 10.3233/THC-220234 | |
61. | Anil K. Philip, Betty Annie Samuel, Saurabh Bhatia, Shaden A. M. Khalifa, Hesham R. El-Seedi, Artificial Intelligence and Precision Medicine: A New Frontier for the Treatment of Brain Tumors, 2022, 13, 2075-1729, 24, 10.3390/life13010024 | |
62. | Maria Baldeon-Calisto, Zhouping Wei, Shatha Abudalou, Yasin Yilmaz, Kenneth Gage, Julio Pow-Sang, Yoganand Balagurunathan, A multi-object deep neural network architecture to detect prostate anatomy in T2-weighted MRI: Performance evaluation, 2023, 2, 2673-8880, 10.3389/fnume.2022.1083245 | |
63. | James Requa, Tuatini Godard, Rajni Mandal, Bonnie Balzer, Darren Whittemore, Eva George, Frenalyn Barcelona, Chalette Lambert, Jonathan Lee, Allison Lambert, April Larson, Gregory Osmond, High-fidelity detection, subtyping, and localization of five skin neoplasms using supervised and semi-supervised learning, 2023, 14, 21533539, 100159, 10.1016/j.jpi.2022.100159 | |
64. | Demetra Demetriou, Rodney Hull, Mmamoletla Kgoebane-Maseko, Zarina Lockhat, Zodwa Dlamini, 2023, Chapter 5, 978-3-031-21505-6, 93, 10.1007/978-3-031-21506-3_5 | |
65. | Kun Lan, Gloria Li, Yang Jie, Rui Tang, Liansheng Liu, Simon Fong, Convolutional neural network with group theory and random selection particle swarm optimizer for enhancing cancer image classification, 2021, 18, 1551-0018, 5573, 10.3934/mbe.2021281 | |
66. | Xuewei Mao, Wei Shan, Wilson Fox, Jinpeng Yu, Subtraction technique on 18F-fluoro-2-deoxy-d-glucose positron emission tomography (18F-FDG-PET) images, 2023, 1368-2199, 1, 10.1080/13682199.2023.2169989 | |
67. | Doniyorjon Mukhtorov, Madinakhon Rakhmonova, Shakhnoza Muksimova, Young-Im Cho, Endoscopic Image Classification Based on Explainable Deep Learning, 2023, 23, 1424-8220, 3176, 10.3390/s23063176 | |
68. | Rupsa Rani Sahu, Anjana Raut, Swati Samantaray, 2022, Technological Empowerment: Applications of Machine Learning in Oral Healthcare, 978-1-6654-6109-2, 1, 10.1109/ASSIC55218.2022.10088392 | |
69. | Njud S. Alharbi, Stelios Bekiros, Hadi Jahanshahi, Jun Mou, Qijia Yao, Spatiotemporal wavelet-domain neuroimaging of chaotic EEG seizure signals in epilepsy diagnosis and prognosis with the use of graph convolutional LSTM networks, 2024, 181, 09600779, 114675, 10.1016/j.chaos.2024.114675 | |
70. | Ibtihaj Ahmad, Mian Aitazaz Ahmad, Sadia Jabbar Anwar, 2023, Transfer Learning and Dual Attention Network Based Nuclei Segmentation in Head and Neck Digital Cancer Histology Images, 979-8-3503-2138-8, 01, 10.1109/ECAI58194.2023.10193937 | |
71. | Yang Liu, Chen Chen, Enguang Zuo, Ziwei Yan, Chenjie Chang, Zhiyuan Cheng, Xiaoyi Lv, Cheng Chen, MURDA: Multisource Unsupervised Raman Spectroscopy Domain Adaptation Model with Reconstructed Target Domains for Medical Diagnosis Assistance, 2024, 96, 0003-2700, 15540, 10.1021/acs.analchem.4c01581 | |
72. | Valentine Wargnier-Dauchelle, Thomas Grenier, Françoise Durand-Dubief, François Cotton, Michaël Sdika, A Weakly Supervised Gradient Attribution Constraint for Interpretable Classification and Anomaly Detection, 2023, 42, 0278-0062, 3336, 10.1109/TMI.2023.3282789 | |
73. | Hexuan Hu, Jianyu Zhang, Tianjin Yang, Qiang Hu, Yufeng Yu, Qian Huang, PATrans: Pixel-Adaptive Transformer for edge segmentation of cervical nuclei on small-scale datasets, 2024, 168, 00104825, 107823, 10.1016/j.compbiomed.2023.107823 | |
74. | О.Є. Дудін, ЦИФРОВА ПАТОЛОГІЯ ПРИ МЕЛАНОМІ: ДОСЯГНЕННЯ, БАР’ЄРИ ТА ПЕРСПЕКТИВИ, 2023, 1997-7468, 9, 10.11603/mie.1996-1960.2022.4.13411 | |
75. | Hongbing Wu, Zhuo Zhang, Yuchen Zhang, Baoshan Sun, Xiaochen Zhang, ACX-UNet: a multi-scale lung parenchyma segmentation study with improved fusion of skip connection and circular cross-features extraction, 2024, 18, 1863-1703, 525, 10.1007/s11760-023-02770-1 | |
76. | Huei-Yung Lin, Chun-Ke Chang, Van Luan Tran, Lane detection networks based on deep neural networks and temporal information, 2024, 98, 11100168, 10, 10.1016/j.aej.2024.04.027 | |
77. | Agnieszka Pregowska, Agata Roszkiewicz, Magdalena Osial, Michael Giersig, How scanning probe microscopy can be supported by artificial intelligence and quantum computing?, 2024, 87, 1059-910X, 2515, 10.1002/jemt.24629 | |
78. | Ugur Kilic, Isil Karabey Aksakalli, Gulsah Tumuklu Ozyer, Tugay Aksakalli, Baris Ozyer, Senol Adanur, Riccardo Ortale, Exploring the Effect of Image Enhancement Techniques with Deep Neural Networks on Direct Urinary System X‐Ray (DUSX) Images for Automated Kidney Stone Detection, 2023, 2023, 0884-8173, 10.1155/2023/3801485 | |
79. | Momina Liaqat Ali, Zunaira Rauf, Asifullah Khan, Anabia Sohail, Rafi Ullah, Jeonghwan Gwak, CB-HVT Net: A Channel-Boosted Hybrid Vision Transformer Network for Lymphocyte Detection in Histopathological Images, 2023, 11, 2169-3536, 115740, 10.1109/ACCESS.2023.3324383 | |
80. | Wafae Abbaoui, Sara Retal, Brahim El Bhiri, Nassim Kharmoum, Soumia Ziti, Towards revolutionizing precision healthcare: A systematic literature review of artificial intelligence methods in precision medicine, 2024, 46, 23529148, 101475, 10.1016/j.imu.2024.101475 | |
81. | Jin L Tan, Dileepa Pitawela, Mohamed A Chinnaratha, Andrawus Beany, Enrik J Aguila, Hsiang‐Ting Chen, Gustavo Carneiro, Rajvinder Singh, Exploring vision transformers for classifying early Barrett's dysplasia in endoscopic images: A pilot study on white‐light and narrow‐band imaging, 2024, 8, 2397-9070, 10.1002/jgh3.70030 | |
82. | Qiwen Zhang, Yichao Wang, Research on mechanical property prediction of hot rolled steel based on lightweight multi-branch convolutional neural network, 2023, 37, 23524928, 107445, 10.1016/j.mtcomm.2023.107445 | |
83. | Haiyan Song, Cuihong Liu, Shengnan Li, Peixiao Zhang, TS-GCN: A novel tumor segmentation method integrating transformer and GCN, 2023, 20, 1551-0018, 18173, 10.3934/mbe.2023807 | |
84. | An-Yu Su, Ming-Long Wu, Yu-Hsueh Wu, Deep learning system for the differential diagnosis of oral mucosal lesions through clinical photographic imaging, 2024, 19917902, 10.1016/j.jds.2024.10.019 | |
85. | Ryan Fogarty, Dmitry Goldgof, Lawrence Hall, Alex Lopez, Joseph Johnson, Manoj Gadara, Radka Stoyanova, Sanoj Punnen, Alan Pollack, Julio Pow-Sang, Yoganand Balagurunathan, Classifying Malignancy in Prostate Glandular Structures from Biopsy Scans with Deep Learning, 2023, 15, 2072-6694, 2335, 10.3390/cancers15082335 | |
86. | Krishna Román, José Llumiquinga, Stalyn Chancay, Manuel Eugenio Morocho-Cayamcela, 2023, Chapter 23, 978-3-031-45437-0, 337, 10.1007/978-3-031-45438-7_23 | |
87. | M. Guo, W. Shen, M. Zhou, Y. Song, J. Liu, W. Xiong, Y. Gao, Safety and efficacy of carbamazepine in the treatment of trigeminal neuralgia: A metanalysis in biomedicine, 2024, 21, 1551-0018, 5335, 10.3934/mbe.2024235 | |
88. | Panagiotis Papachristou, My Söderholm, Jon Pallon, Marina Taloyan, Sam Polesie, John Paoli, Chris D Anderson, Magnus Falk, Evaluation of an artificial intelligence-based decision support for the detection of cutaneous melanoma in primary care: a prospective real-life clinical trial, 2024, 191, 0007-0963, 125, 10.1093/bjd/ljae021 | |
89. | Yanzhen Liu, Xinbao Wu, Yudi Sang, Chunpeng Zhao, Yu Wang, Bojing Shi, Yubo Fan, Evolution of Surgical Robot Systems Enhanced by Artificial Intelligence: A Review, 2024, 6, 2640-4567, 10.1002/aisy.202300268 | |
90. | Alessia Artesani, Alessandro Bruno, Fabrizia Gelardi, Arturo Chiti, Empowering PET: harnessing deep learning for improved clinical insight, 2024, 8, 2509-9280, 10.1186/s41747-023-00413-1 | |
91. | Chao-Hung Kuo, Guan-Tze Liu, Chi-En Lee, Jing Wu, Kaitlyn Casimo, Kurt E. Weaver, Yu-Chun Lo, You-Yin Chen, Wen-Cheng Huang, Jeffrey G. Ojemann, Decoding micro-electrocorticographic signals by using explainable 3D convolutional neural network to predict finger movements, 2024, 411, 01650270, 110251, 10.1016/j.jneumeth.2024.110251 | |
92. | Demetra Demetriou, Zarina Lockhat, Luke Brzozowski, Kamal S. Saini, Zodwa Dlamini, Rodney Hull, The Convergence of Radiology and Genomics: Advancing Breast Cancer Diagnosis with Radiogenomics, 2024, 16, 2072-6694, 1076, 10.3390/cancers16051076 | |
93. | Nanda Deepa Thimmappa, MRA for Preoperative Planning and Postoperative Management of Perforator Flap Surgeries: A Review, 2024, 59, 1053-1807, 797, 10.1002/jmri.28946 | |
94. | K. Anbumani, R. Balamanigandan, T. Rajesh Kumar, A. Sasi Kumar, Mahaveerakannan. R, 2023, Identification of Breast Cancer using Jelly fish Based Deep Wavelet Autoencoder, 979-8-3503-9737-6, 2189, 10.1109/ICACCS57279.2023.10112750 | |
95. | Kotaro Ito, Naohisa Hirahara, Hirotaka Muraoka, Eri Sawada, Satoshi Tokunaga, Takashi Kaneda, Texture analysis using short-tau inversion recovery magnetic resonance images to differentiate squamous cell carcinoma of the gingiva from medication-related osteonecrosis of the jaw, 2024, 40, 0911-6028, 219, 10.1007/s11282-023-00725-3 | |
96. | Angeline Pearl G P, J. Anitha, 2024, Classification of Alzheimer’s Disease with Generated MRI Images using Randomized CNN, 979-8-3503-9156-5, 1, 10.1109/ICSTSN61422.2024.10670775 | |
97. | Xian-Ya Zhang, Qi Wei, Ge-Ge Wu, Qi Tang, Xiao-Fang Pan, Gong-Quan Chen, Di Zhang, Christoph F. Dietrich, Xin-Wu Cui, Artificial intelligence - based ultrasound elastography for disease evaluation - a narrative review, 2023, 13, 2234-943X, 10.3389/fonc.2023.1197447 | |
98. | Raju S. Maher, Shobha K. Bhawiskar, 2023, Chapter 39, 978-94-6463-135-7, 456, 10.2991/978-94-6463-136-4_39 | |
99. | Samira Sajed, Amir Sanati, Jorge Esparteiro Garcia, Habib Rostami, Ahmad Keshavarz, Andreia Teixeira, The effectiveness of deep learning vs. traditional methods for lung disease diagnosis using chest X-ray images: A systematic review, 2023, 147, 15684946, 110817, 10.1016/j.asoc.2023.110817 | |
100. | Dayangku Nur Faizah Pengiran Mohamad, Syamsiah Mashohor, Rozi Mahmud, Marsyita Hanafi, Norafida Bahari, Transition of traditional method to deep learning based computer-aided system for breast cancer using Automated Breast Ultrasound System (ABUS) images: a review, 2023, 56, 0269-2821, 15271, 10.1007/s10462-023-10511-6 | |
101. | Maya A. Joshi, Sean D. Tallman, Three-dimensional convolutional neural network for age-at-death estimation of deceased individuals through cranial computed tomography scans, 2023, 34, 26662256, 200557, 10.1016/j.fri.2023.200557 | |
102. | Taiga Nakajima, Shinichi Yoshida, 2024, Chapter 16, 978-981-99-7592-1, 167, 10.1007/978-981-99-7593-8_16 | |
103. | Mark Movh, Isah A. Lawal, 2023, Chapter 6, 978-3-031-44083-0, 54, 10.1007/978-3-031-44084-7_6 | |
104. | Coşku Öksüz, Oğuzhan Urhan, Mehmet Kemal Güllü, An integrated convolutional neural network with attention guidance for improved performance of medical image classification, 2024, 36, 0941-0643, 2067, 10.1007/s00521-023-09164-x | |
105. | Ayse Betul Cengiz, A. Stephen McGough, 2023, How much data do I need? A case study on medical data, 979-8-3503-2445-7, 3688, 10.1109/BigData59044.2023.10386440 | |
106. | Yonghan Lu, Chengjian Qiu, Qiaoying Teng, Jun Chen, Robert Free, Lu Liu, Yuqing Song, Zhe Liu, 2023, LC-SegDiff: Label-Constraint Diffusion Model for Medical Image Segmentation, 979-8-3503-3748-8, 3305, 10.1109/BIBM58861.2023.10385655 | |
107. | Ramin Yousefpour Shahrivar, Fatemeh Karami, Ebrahim Karami, Enhancing Fetal Anomaly Detection in Ultrasonography Images: A Review of Machine Learning-Based Approaches, 2023, 8, 2313-7673, 519, 10.3390/biomimetics8070519 | |
108. | Bader Aldughayfiq, Farzeen Ashfaq, N. Z. Jhanjhi, Mamoona Humayun, YOLO-Based Deep Learning Model for Pressure Ulcer Detection and Classification, 2023, 11, 2227-9032, 1222, 10.3390/healthcare11091222 | |
109. | Rômulo Sérgio Araújo Gomes, Guilherme Henrique Peixoto de Oliveira, Diogo Turiani Hourneaux de Moura, Ana Paula Samy Tanaka Kotinda, Carolina Ogawa Matsubayashi, Bruno Salomão Hirsch, Matheus Oliveira Veras, João Guilherme Ribeiro Jordão Sasso, Roberto Paolo Trasolini, Wanderley Marques Bernardo, Eduardo Guimarães Hourneaux de Moura, Endoscopic ultrasound artificial intelligence-assisted for prediction of gastrointestinal stromal tumors diagnosis: A systematic review and meta-analysis, 2023, 15, 1948-5190, 528, 10.4253/wjge.v15.i8.528 | |
110. | Zengxin Qi, Wenwen Zeng, Di Zang, Zhe Wang, Lanqin Luo, Xuehai Wu, Jinhua Yu, Ying Mao, Classifying disorders of consciousness using a novel dual-level and dual-modal graph learning model, 2024, 22, 1479-5876, 10.1186/s12967-024-05729-z | |
111. | Bogdan Ionut Anghel, Radu Lupu, Understanding Regulatory Changes: Deep Learning in Sustainable Finance and Banking, 2024, 17, 1911-8074, 295, 10.3390/jrfm17070295 | |
112. | Huihui Jia, Songqiao Tang, Wanliang Guo, Peng Pan, Yufeng Qian, Dongliang Hu, Yakang Dai, Yang Yang, Chen Geng, Haitao Lv, Differential diagnosis of congenital ventricular septal defect and atrial septal defect in children using deep learning–based analysis of chest radiographs, 2024, 24, 1471-2431, 10.1186/s12887-024-05141-y | |
113. | Tsi-Shu Huang, Kevin Wang, Xiu-Yuan Ye, Chii-Shiang Chen, Fu-Chuen Chang, Paschalis Vergidis, Yang Zhang, Attention-Guided Transfer Learning for Identification of Filamentous Fungi Encountered in the Clinical Laboratory, 2023, 11, 2165-0497, 10.1128/spectrum.04611-22 | |
114. | Heng-Le Wei, Cunsheng Wei, Yibo Feng, Wanying Yan, Yu-Sheng Yu, Yu-Chen Chen, Xindao Yin, Junrong Li, Hong Zhang, Predicting the efficacy of non-steroidal anti-inflammatory drugs in migraine using deep learning and three-dimensional T1-weighted images, 2023, 26, 25890042, 108107, 10.1016/j.isci.2023.108107 | |
115. | Meijing Wu, Guangxia Cui, Shuchang Lv, Lijiang Chen, Zongmei Tian, Min Yang, Wenpei Bai, Deep convolutional neural networks for multiple histologic types of ovarian tumors classification in ultrasound images, 2023, 13, 2234-943X, 10.3389/fonc.2023.1154200 | |
116. | Josh Frederich, Julieta Himawan, Mia Rizkinia, 2024, 3080, 0094-243X, 110002, 10.1063/5.0200741 | |
117. | Abolfazl Mehbodniya, Satheesh Narayanasami, Julian L. Webber, Amarendra Kothalanka, Sudhakar Sengan, Rajasekar Rangasamy, D. Stalin David, 2023, Chapter 39, 978-981-19-7454-0, 525, 10.1007/978-981-19-7455-7_39 | |
118. | Dan Tang, Jinjing Chen, Lijuan Ren, Xie Wang, Daiwei Li, Haiqing Zhang, Reviewing CAM-Based Deep Explainable Methods in Healthcare, 2024, 14, 2076-3417, 4124, 10.3390/app14104124 | |
119. | Shimeng Shi, Hongru Li, Yifu Zhang, Xinzhuo Wang, Semantic information-guided attentional GAN-based ultrasound image synthesis method, 2025, 102, 17468094, 107273, 10.1016/j.bspc.2024.107273 | |
120. | Baocan Zhang, Xiaolu Jiang, Wei Zhao, An Enhanced Mask Transformer for Overlapping Cervical Cell Segmentation Based on DETR, 2024, 12, 2169-3536, 176586, 10.1109/ACCESS.2024.3505616 | |
121. | Kun Wang, Yong Han, Yuguang Ye, Yusi Chen, Daxin Zhu, Yifeng Huang, Ying Huang, Yijie Chen, Jianshe Shi, Bijiao Ding, Jianlong Huang, Mixed reality infrastructure based on deep learning medical image segmentation and 3D visualization for bone tumors using DCU-Net, 2024, 22121374, 100654, 10.1016/j.jbo.2024.100654 | |
122. | Abolfazl Pordeli Shahreki, Fatemeh Sadat Hosseini-Baharanchi, Masoud Roudbari, Diagnosis of Tuberculosis Using Medical Images by Convolutional Neural Networks, 2024, 31, 2008-2843, 180, 10.34172/jkmu.2024.29 | |
123. | Wensheng Li, Jie Zhang, Jianlin Guo, Xiaoxu Wang, Guangyuan Xu, Yun Peng, Liyun Tu, 2024, Automated Detection and Classification of Pediatric Middle Ear Diseases from CT using Entropy Projection and Feature Interaction, 979-8-3503-8622-6, 2156, 10.1109/BIBM62325.2024.10822350 | |
124. | Rupali A. Patil, V. V. Dixit, Detection and classification of mammogram using ResNet-50, 2025, 1573-7721, 10.1007/s11042-025-20679-4 | |
125. | Nasar Alwahaibi, Maryam Alwahaibi, Mini review on skin biopsy: traditional and modern techniques, 2025, 12, 2296-858X, 10.3389/fmed.2025.1476685 | |
126. | Takao Tomono, Kazuya Tsujimura, The discriminative ability on anomaly detection using quantum kernels for shipping inspection, 2025, 12, 2662-4400, 10.1140/epjqt/s40507-025-00335-4 | |
127. | D.V. Robota, B.S. Burlaka , SELECTING AN EFFECTIVE METHOD OF COLOR NORMALIZATION FOR HISTOLOGICAL IMAGES OF INTESTINAL TISSUES IN DEEP LEARNING MODEL DEVELOPMENT, 2025, 25, 2077-1126, 203, 10.31718/2077-1096.25.1.203 | |
128. | Taiga Nakajima, Yoshua Kazukuni Nomura, Narufumi Suganuma, Shinichi Yoshida, 2025, Chapter 16, 978-981-96-4755-2, 210, 10.1007/978-981-96-4756-9_16 |
Reference | Year | Application | Method | Dataset | Result |
Fujita et al. [39] | 2019 | Fibrillations and flutters detection | DCNN | Physiobank(PTB) dataset | Accuracy: 98.45% Sensitvity: 99.87% Specificity: 99.27% |
Luis et al. [40] | 2019 | Parkinson's disease identification | CNN | HandPD dataset | Accuracy: 87% |
Gonzxalez-Dxıaz et al. [52] | 2019 | Skin lesion diagnosis | DermaKNet | 2017 ISBI Challenge | Specificity: 95% AUC: 0.917 |
Jeyaraj et al. [53] | 2019 | Oral cancer CAD system | DCNN | TCIA, GDC | Accuracy: 94.5% Sensitivity: 94.0% Specificity: 98.0% |
Martin et al. [42] | 2018 | Ureteral stone identification | CNN | Clinical | Sensitivity: 100% FP/scan: 2.68 |
Sajid et al. [43] | 2018 | Brain tumors segmentation | DCNN | BRATS 2015 | — |
Hosseini-Asl et al. [56] | 2018 | Alzheimer's disease diagnosis | 3D-CNN | ADNI dataset | Sensitivity: 76% F1-score: 0.75 |
Farooq et al. [57] | 2017 | Multi-class classification of Alzheimer's disease | CNN | ADNI dataset | Accuracy: 98.88% |
Liao et al. [44] | 2017 | Lung nodules diagnosis | A modified U-Net | Kaggle Data Science Bowl of 2017 | Accuracy: 81.42% Recall: 85.62% |
Tulder et al. [58] | 2016 | Lung CT images classification | Convolutional RBM | ILD CT scans | Accuracy: 89.0% |
Anthimopoulos et al. [59] | 2016 | Lung pattern classification | DCNN | ILD CT scans | Accuracy: 85.5% |
Pratt et al. [47] | 2016 | Diabetic retinopathy diagnosis | CNN | Kaggle Data Science Bowl of 2016 | Accuracy: 75.0% Sensitivity: 95.0% |
Gao et al. [48] | 2016 | Lung CT attenuation patterns classification | CNN | ILD CT scans | Accuracy: 87.9% |
Shin et al. [49] | 2016 | Thoraco-abdominal lymph node(LN) detection | DCNN | CT scans | AUC: 0.93–0.95 |
Ronneberger et al. [50] | 2015 | Biomedical image segmentation | CNN | ISBI challenge | Accuracy: 92.03% |
Reference | Year | Application | Method | Dataset | Result |
Chiang et al. [63] | 2019 | Breast ultrasound CAD system | 3D-CNN | Clinical | Sensitivity: 95.0% FP/scan: 14.03 |
Samala et al. [64] | 2019 | Breast masses classification | DCNN, transfer learning | DDSM | AUC: 0.85 ± 0.05 for single-stage transfer learning AUC: 0.91 ± 0.03 for multi-stage transfer learning |
Gao et al. [73] | 2018 | Breast cancer diagnosis | Shallow-Deep CNN(SD-CNN) | BCDR | Accuracy: 82.9% |
Zhou et al. [72] | 2018 | Breast tumors classification | CNN | Clinical | Accuracy: 95.8% Sensitivity: 96.2% Specificity: 95.7% |
Kooi et al. [75] | 2017 | Breast mammography CAD system | CNN | Clinical | AUC: 0.875–0.941 |
Becker et al. [65] | 2017 | Breast cancer detection | ANN | Clinical | AUC: 0.82 |
Li et al. [74] | 2017 | Breast tumors classification | 3D-CNN | Clinical | Accuracy: 78.1% Sensitivity: 74.4% Specificity: 82.3% AUC: 0.801 |
Zhou et al. [66] | 2017 | Breast tissue density classification | AlexNet | Clinical | Accuracy: 76% |
Kooi et al. [67] | 2017 | Masses discrimination | DCNNs | WBCD | AUC: 0.80 |
Ayelet et al. [68] | 2016 | Breast tumors detection and classification | Faster R-CNN | Clinical | Accuracy: 77% AUC: 0.72 |
Posada et al. [69] | 2016 | Breast cancer detection and diagnosis | AlexNet, VGGNet | MIAS | Accuracy: 60.01% and 64.52%, respectively |
Samala et al. [70] | 2016 | Digital breast tomosynthesis(DBT) CAD system | DCNN | Clinical | AUC: 0.90 |
Dhungel et al. [71] | 2016 | Masses classification | CNN | DDSM | Accuracy: 84.00% ± 4.00% |
Samala et al. [76] | 2016 | Breast CAD system | CNN, DCNN | Clinical | AUC: 0.89 and 0.93, respectively |
Reference | Year | Application | Method | Dataset | Result |
Shi et al. [80] | 2019 | Lung nodules detection | VGG-16 | CT scans | Sensitivity: 87.2% FP/scan: 0.39 |
Savitha et al. [83] | 2019 | Lung cancers classification | Optimal deep neural network(ODNN) | Clinical | Accuracy: 94.56% Sensitivity: 96.20% Specificity: 94.20% |
Zhao et al. [84] | 2018 | Lung nodules classification | LeNet, AlexNet | LIDC | Accuracy: 82.20% AUC: 0.877 |
Dey et al. [89] | 2018 | Lung nodules classification | 3D-DCNN | LUNA16 | Competition performance metric(CPM): 0.910 |
Nishio et al. [82] | 2018 | Lung cancer CAD system | DCNN | Clinical | Accuracy: 68% |
Anton et al. [90] | 2017 | Lung CT CAD system | ResNet | LIDC-IDRI | Sensitivity: 91.07% Accuracy: 89.90% |
Ding et al. [85] | 2017 | Lung CAD system | DCNNs | LUNA16 | Sensitivity: 94.60% FROC: 0.893 |
Dou et al. [86] | 2017 | Lung nodules detection | 3D-CNN | LUNA16 | Sensitivity: 90.50% FP/scan: 1.0 |
Cheng et al. [87] | 2016 | Lung lesions classification | OverFeat | LIDC | Sensitivity: 90.80% ± 5.30 |
Li et al. [91] | 2016 | Lung nodules classification | DCNN | LIDC | Sensitivity: 87.10% FP/scan: 4.62 |
Liu et al. [88] | 2016 | Lung nodules classification | Multi-view CNN(MV-CNN) | LIDC-IDRI | Error rate: 5.41% Sensitivity: 90.49% Specificity: 99.91% |
Hua et al. [79] | 2015 | Lung nodules classification | CNN | LIDC | Sensitivity: 73.30% Specificity: 78.7% |
Reference | Year | Application | Method | Dataset | Result |
Li et al. [95] | 2019 | Prostate cancer grading | R-CNN | Clinical | Accuracy: 89.4% |
Wang et al. [99] | 2018 | Clinically significant prostate cancer CAD system | Dual-path CNN | Clinical | Sensitivity: 89.78% FP/scan: 1 |
Ishioka et al. [100] | 2018 | Prostate cancer CAD system | DCNN | Clinical (two training datasets: = 301, = 34) | AUC: 0.645 and 0.636, respectively |
Song et al. [98] | 2018 | Prostate cancer CADx | DCNN | PROSTATEx | Sensitivity: 87% Specificity: 90.6% AUC: 0.944 |
Chen et al. [97] | 2018 | Clinically significant prostate cancer classification | Inception V3, VGG-16 | PROSTATEx | AUC: 0.81 and 0.83 |
Kohl et al. [101] | 2017 | Prostate cancer detection | Fully convolutional networks (FCNs) | Clinical | Sensitivity: 55% Specificity: 98% |
Yang et al. [102] | 2017 | Prostate cancer detection | Co-trained CNNs | Clinical | Sensitivity: 46.00%, 92.00% and 97.00% FP/scan: 0.1, 1 and 10 |
Yang et al. [107] | 2017 | Prostate cancer localization and characterization | Multimodal multi-label CNNs | Clinical | Sensitivity: 98.6% Specificity: 98.3% Accuracy: 98.5% AUC: 0.998 |
Jin et al. [103] | 2017 | Prostate cancer detection | CNN | PROMISE12 | AUC: 0.974 |
Wang et al. [104] | 2017 | Prostate cancer detection | DCNN | PFMP | AUC: 0.84 |
Le et al. [106] | 2017 | Prostate cancer diagnosis | Multimodal CNNs | Clinical | Sensitivity: 89.85% Specificity: 95.83% |
Liu et al. [105] | 2017 | Prostate cancer lesions classification | XmasNet | PROSTATEx | AUC: 0.84 |
Database | Image modality | No. of patients | No. of benign samples | No. of malignant samples | No. of normal samples | Link |
MIAS | X-rays | 161 | 63 | 51 | 208 | http://www.wiau.man.ac.uk/services/MIAS/MIASweb.html |
WBCD | Mammography | 569 | 357 | 212 | - | http://marathon.csee.usf.edu/ Mammography/Database.html |
DDSM | Mammography | 2, 620 | 4, 044 | 3, 656 | 2, 780 | https://archive.ics.uci.edu/ml/datasets/Breast+Cancer+Wisconsin+%28Diagnostic%29 |
BCDR | Ultrasound | 1, 010 | - | - | 3, 703 | http://bcdr.inegi.up.pt/ |
Database | Image modality | No. of scans | No. of slices | No. of images | Link |
LIDC-IDRI | CT, DX, CR | 1, 018 | ─ | 244, 527 | https://wiki.cancerimagingarchive.net/display/Public/LIDC-IDRI |
LUNA16 | CT | 888 | 1, 084 | 272 | https://luna16.grand-challenge.org/download/ |
ANODE09 | CT | 55 | 451.5 | ─ | https://www.rug.nl/research/portal/datasets/nederlandsleuvens-longkanker-screenings-onderzoek-nelson.html |
JSRT | X-rays | ─ | ─ | 247 | http://db.jsrt.or.jp/eng.php |
Database | Image modality | No. of cases | No. of images | Link |
PROSTATEx | MRI | 346 | 309, 251 | https://wiki.cancerimagingarchive.net/display/Public/SPIE-AAPM-NCI+PROSTATEx+Challenges |
PFMP | MRI | 28 | 32, 508 | https://pathology.cancerimagingarchive.net/pathdata/ |
PROMISE12 | MRI | 50 | ─ | https://promise12.grand-challenge.org/Download/ |
Prostate-3T | MRI | 64 | 1, 258 | https://wiki.cancerimagingarchive.net/display/Public/Prostate-3T |
Metric | Calculation Formula | |
accuracy | accuracy=(TP+TN)(TP+TN+FP+FN) | Eq 1 |
precision | precision=TP(TP+FP) | Eq 2 |
sensitivity | sensitivity=TP(TP+FN) | Eq 3 |
specificity | specificity=TN(TN+FP) | Eq 4 |
F1−score | F1−score=2×(precision×recall)(precision+recall) | Eq 5 |
TPR | TPR=TP(TP+FN) | Eq 6 |
FPR | FPR=FP(TN+FP) | Eq 7 |
dice−coefficient | dice−coefficient=2×|P∩GT||P|+|GT| | Eq 8 |
Reference | Year | Application | Method | Dataset | Result |
Fujita et al. [39] | 2019 | Fibrillations and flutters detection | DCNN | Physiobank(PTB) dataset | Accuracy: 98.45% Sensitvity: 99.87% Specificity: 99.27% |
Luis et al. [40] | 2019 | Parkinson's disease identification | CNN | HandPD dataset | Accuracy: 87% |
Gonzxalez-Dxıaz et al. [52] | 2019 | Skin lesion diagnosis | DermaKNet | 2017 ISBI Challenge | Specificity: 95% AUC: 0.917 |
Jeyaraj et al. [53] | 2019 | Oral cancer CAD system | DCNN | TCIA, GDC | Accuracy: 94.5% Sensitivity: 94.0% Specificity: 98.0% |
Martin et al. [42] | 2018 | Ureteral stone identification | CNN | Clinical | Sensitivity: 100% FP/scan: 2.68 |
Sajid et al. [43] | 2018 | Brain tumors segmentation | DCNN | BRATS 2015 | — |
Hosseini-Asl et al. [56] | 2018 | Alzheimer's disease diagnosis | 3D-CNN | ADNI dataset | Sensitivity: 76% F1-score: 0.75 |
Farooq et al. [57] | 2017 | Multi-class classification of Alzheimer's disease | CNN | ADNI dataset | Accuracy: 98.88% |
Liao et al. [44] | 2017 | Lung nodules diagnosis | A modified U-Net | Kaggle Data Science Bowl of 2017 | Accuracy: 81.42% Recall: 85.62% |
Tulder et al. [58] | 2016 | Lung CT images classification | Convolutional RBM | ILD CT scans | Accuracy: 89.0% |
Anthimopoulos et al. [59] | 2016 | Lung pattern classification | DCNN | ILD CT scans | Accuracy: 85.5% |
Pratt et al. [47] | 2016 | Diabetic retinopathy diagnosis | CNN | Kaggle Data Science Bowl of 2016 | Accuracy: 75.0% Sensitivity: 95.0% |
Gao et al. [48] | 2016 | Lung CT attenuation patterns classification | CNN | ILD CT scans | Accuracy: 87.9% |
Shin et al. [49] | 2016 | Thoraco-abdominal lymph node(LN) detection | DCNN | CT scans | AUC: 0.93–0.95 |
Ronneberger et al. [50] | 2015 | Biomedical image segmentation | CNN | ISBI challenge | Accuracy: 92.03% |
Reference | Year | Application | Method | Dataset | Result |
Chiang et al. [63] | 2019 | Breast ultrasound CAD system | 3D-CNN | Clinical | Sensitivity: 95.0% FP/scan: 14.03 |
Samala et al. [64] | 2019 | Breast masses classification | DCNN, transfer learning | DDSM | AUC: 0.85 ± 0.05 for single-stage transfer learning AUC: 0.91 ± 0.03 for multi-stage transfer learning |
Gao et al. [73] | 2018 | Breast cancer diagnosis | Shallow-Deep CNN(SD-CNN) | BCDR | Accuracy: 82.9% |
Zhou et al. [72] | 2018 | Breast tumors classification | CNN | Clinical | Accuracy: 95.8% Sensitivity: 96.2% Specificity: 95.7% |
Kooi et al. [75] | 2017 | Breast mammography CAD system | CNN | Clinical | AUC: 0.875–0.941 |
Becker et al. [65] | 2017 | Breast cancer detection | ANN | Clinical | AUC: 0.82 |
Li et al. [74] | 2017 | Breast tumors classification | 3D-CNN | Clinical | Accuracy: 78.1% Sensitivity: 74.4% Specificity: 82.3% AUC: 0.801 |
Zhou et al. [66] | 2017 | Breast tissue density classification | AlexNet | Clinical | Accuracy: 76% |
Kooi et al. [67] | 2017 | Masses discrimination | DCNNs | WBCD | AUC: 0.80 |
Ayelet et al. [68] | 2016 | Breast tumors detection and classification | Faster R-CNN | Clinical | Accuracy: 77% AUC: 0.72 |
Posada et al. [69] | 2016 | Breast cancer detection and diagnosis | AlexNet, VGGNet | MIAS | Accuracy: 60.01% and 64.52%, respectively |
Samala et al. [70] | 2016 | Digital breast tomosynthesis(DBT) CAD system | DCNN | Clinical | AUC: 0.90 |
Dhungel et al. [71] | 2016 | Masses classification | CNN | DDSM | Accuracy: 84.00% ± 4.00% |
Samala et al. [76] | 2016 | Breast CAD system | CNN, DCNN | Clinical | AUC: 0.89 and 0.93, respectively |
Reference | Year | Application | Method | Dataset | Result |
Shi et al. [80] | 2019 | Lung nodules detection | VGG-16 | CT scans | Sensitivity: 87.2% FP/scan: 0.39 |
Savitha et al. [83] | 2019 | Lung cancers classification | Optimal deep neural network(ODNN) | Clinical | Accuracy: 94.56% Sensitivity: 96.20% Specificity: 94.20% |
Zhao et al. [84] | 2018 | Lung nodules classification | LeNet, AlexNet | LIDC | Accuracy: 82.20% AUC: 0.877 |
Dey et al. [89] | 2018 | Lung nodules classification | 3D-DCNN | LUNA16 | Competition performance metric(CPM): 0.910 |
Nishio et al. [82] | 2018 | Lung cancer CAD system | DCNN | Clinical | Accuracy: 68% |
Anton et al. [90] | 2017 | Lung CT CAD system | ResNet | LIDC-IDRI | Sensitivity: 91.07% Accuracy: 89.90% |
Ding et al. [85] | 2017 | Lung CAD system | DCNNs | LUNA16 | Sensitivity: 94.60% FROC: 0.893 |
Dou et al. [86] | 2017 | Lung nodules detection | 3D-CNN | LUNA16 | Sensitivity: 90.50% FP/scan: 1.0 |
Cheng et al. [87] | 2016 | Lung lesions classification | OverFeat | LIDC | Sensitivity: 90.80% ± 5.30 |
Li et al. [91] | 2016 | Lung nodules classification | DCNN | LIDC | Sensitivity: 87.10% FP/scan: 4.62 |
Liu et al. [88] | 2016 | Lung nodules classification | Multi-view CNN(MV-CNN) | LIDC-IDRI | Error rate: 5.41% Sensitivity: 90.49% Specificity: 99.91% |
Hua et al. [79] | 2015 | Lung nodules classification | CNN | LIDC | Sensitivity: 73.30% Specificity: 78.7% |
Reference | Year | Application | Method | Dataset | Result |
Li et al. [95] | 2019 | Prostate cancer grading | R-CNN | Clinical | Accuracy: 89.4% |
Wang et al. [99] | 2018 | Clinically significant prostate cancer CAD system | Dual-path CNN | Clinical | Sensitivity: 89.78% FP/scan: 1 |
Ishioka et al. [100] | 2018 | Prostate cancer CAD system | DCNN | Clinical (two training datasets: = 301, = 34) | AUC: 0.645 and 0.636, respectively |
Song et al. [98] | 2018 | Prostate cancer CADx | DCNN | PROSTATEx | Sensitivity: 87% Specificity: 90.6% AUC: 0.944 |
Chen et al. [97] | 2018 | Clinically significant prostate cancer classification | Inception V3, VGG-16 | PROSTATEx | AUC: 0.81 and 0.83 |
Kohl et al. [101] | 2017 | Prostate cancer detection | Fully convolutional networks (FCNs) | Clinical | Sensitivity: 55% Specificity: 98% |
Yang et al. [102] | 2017 | Prostate cancer detection | Co-trained CNNs | Clinical | Sensitivity: 46.00%, 92.00% and 97.00% FP/scan: 0.1, 1 and 10 |
Yang et al. [107] | 2017 | Prostate cancer localization and characterization | Multimodal multi-label CNNs | Clinical | Sensitivity: 98.6% Specificity: 98.3% Accuracy: 98.5% AUC: 0.998 |
Jin et al. [103] | 2017 | Prostate cancer detection | CNN | PROMISE12 | AUC: 0.974 |
Wang et al. [104] | 2017 | Prostate cancer detection | DCNN | PFMP | AUC: 0.84 |
Le et al. [106] | 2017 | Prostate cancer diagnosis | Multimodal CNNs | Clinical | Sensitivity: 89.85% Specificity: 95.83% |
Liu et al. [105] | 2017 | Prostate cancer lesions classification | XmasNet | PROSTATEx | AUC: 0.84 |
Database | Image modality | No. of patients | No. of benign samples | No. of malignant samples | No. of normal samples | Link |
MIAS | X-rays | 161 | 63 | 51 | 208 | http://www.wiau.man.ac.uk/services/MIAS/MIASweb.html |
WBCD | Mammography | 569 | 357 | 212 | - | http://marathon.csee.usf.edu/ Mammography/Database.html |
DDSM | Mammography | 2, 620 | 4, 044 | 3, 656 | 2, 780 | https://archive.ics.uci.edu/ml/datasets/Breast+Cancer+Wisconsin+%28Diagnostic%29 |
BCDR | Ultrasound | 1, 010 | - | - | 3, 703 | http://bcdr.inegi.up.pt/ |
Database | Image modality | No. of scans | No. of slices | No. of images | Link |
LIDC-IDRI | CT, DX, CR | 1, 018 | ─ | 244, 527 | https://wiki.cancerimagingarchive.net/display/Public/LIDC-IDRI |
LUNA16 | CT | 888 | 1, 084 | 272 | https://luna16.grand-challenge.org/download/ |
ANODE09 | CT | 55 | 451.5 | ─ | https://www.rug.nl/research/portal/datasets/nederlandsleuvens-longkanker-screenings-onderzoek-nelson.html |
JSRT | X-rays | ─ | ─ | 247 | http://db.jsrt.or.jp/eng.php |
Database | Image modality | No. of cases | No. of images | Link |
PROSTATEx | MRI | 346 | 309, 251 | https://wiki.cancerimagingarchive.net/display/Public/SPIE-AAPM-NCI+PROSTATEx+Challenges |
PFMP | MRI | 28 | 32, 508 | https://pathology.cancerimagingarchive.net/pathdata/ |
PROMISE12 | MRI | 50 | ─ | https://promise12.grand-challenge.org/Download/ |
Prostate-3T | MRI | 64 | 1, 258 | https://wiki.cancerimagingarchive.net/display/Public/Prostate-3T |
Metric | Calculation Formula | |
accuracy | accuracy=(TP+TN)(TP+TN+FP+FN) | Eq 1 |
precision | precision=TP(TP+FP) | Eq 2 |
sensitivity | sensitivity=TP(TP+FN) | Eq 3 |
specificity | specificity=TN(TN+FP) | Eq 4 |
F1−score | F1−score=2×(precision×recall)(precision+recall) | Eq 5 |
TPR | TPR=TP(TP+FN) | Eq 6 |
FPR | FPR=FP(TN+FP) | Eq 7 |
dice−coefficient | dice−coefficient=2×|P∩GT||P|+|GT| | Eq 8 |