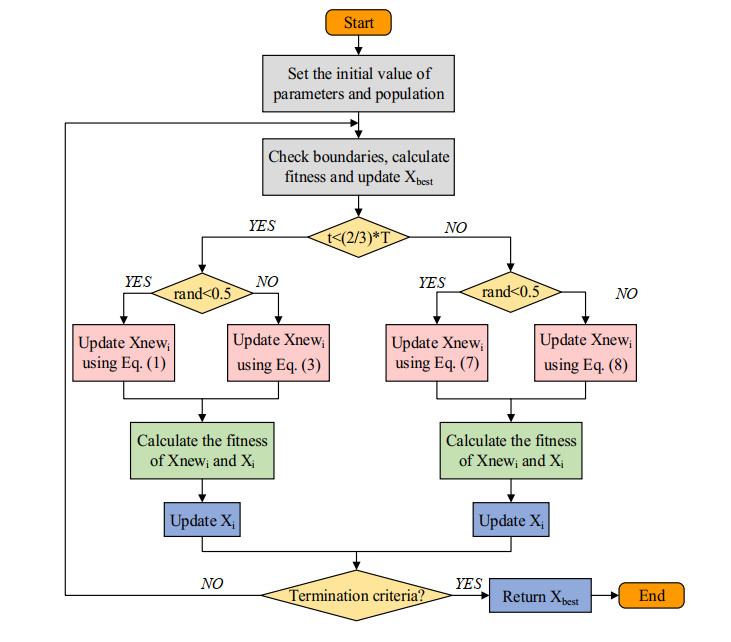
Citation: Rui Zhang, Shuicai Wu, Weiwei Wu, Hongjian Gao, Zhuhuang Zhou. Computer-assisted needle trajectory planning and mathematical modeling for liver tumor thermal ablation: A review[J]. Mathematical Biosciences and Engineering, 2019, 16(5): 4846-4872. doi: 10.3934/mbe.2019244
[1] | Giuseppe D'Onofrio, Enrica Pirozzi . Successive spike times predicted by a stochastic neuronal model with a variable input signal. Mathematical Biosciences and Engineering, 2016, 13(3): 495-507. doi: 10.3934/mbe.2016003 |
[2] | Aniello Buonocore, Luigia Caputo, Enrica Pirozzi, Maria Francesca Carfora . A simple algorithm to generate firing times for leaky integrate-and-fire neuronal model. Mathematical Biosciences and Engineering, 2014, 11(1): 1-10. doi: 10.3934/mbe.2014.11.1 |
[3] | Aniello Buonocore, Luigia Caputo, Enrica Pirozzi, Maria Francesca Carfora . Gauss-diffusion processes for modeling the dynamics of a couple of interacting neurons. Mathematical Biosciences and Engineering, 2014, 11(2): 189-201. doi: 10.3934/mbe.2014.11.189 |
[4] | Tianyuan Xu, Shanming Ji, Chunhua Jin, Ming Mei, Jingxue Yin . EARLY AND LATE STAGE PROFILES FOR A CHEMOTAXIS MODEL WITH DENSITY-DEPENDENT JUMP PROBABILITY. Mathematical Biosciences and Engineering, 2018, 15(6): 1345-1385. doi: 10.3934/mbe.2018062 |
[5] | Massimiliano Tamborrino . Approximation of the first passage time density of a Wiener process to an exponentially decaying boundary by two-piecewise linear threshold. Application to neuronal spiking activity. Mathematical Biosciences and Engineering, 2016, 13(3): 613-629. doi: 10.3934/mbe.2016011 |
[6] | Huili Wei, Wenhe Li . Dynamical behaviors of a Lotka-Volterra competition system with the Ornstein-Uhlenbeck process. Mathematical Biosciences and Engineering, 2023, 20(5): 7882-7904. doi: 10.3934/mbe.2023341 |
[7] | Buyu Wen, Bing Liu, Qianqian Cui . Analysis of a stochastic SIB cholera model with saturation recovery rate and Ornstein-Uhlenbeck process. Mathematical Biosciences and Engineering, 2023, 20(7): 11644-11655. doi: 10.3934/mbe.2023517 |
[8] | Virginia Giorno, Serena Spina . On the return process with refractoriness for a non-homogeneous Ornstein-Uhlenbeck neuronal model. Mathematical Biosciences and Engineering, 2014, 11(2): 285-302. doi: 10.3934/mbe.2014.11.285 |
[9] | Antonio Di Crescenzo, Fabio Travaglino . Probabilistic analysis of systems alternating for state-dependent dichotomous noise. Mathematical Biosciences and Engineering, 2019, 16(6): 6386-6405. doi: 10.3934/mbe.2019319 |
[10] | Xichao Duan, Sanling Yuan, Kaifa Wang . Dynamics of a diffusive age-structured HBV model with saturating incidence. Mathematical Biosciences and Engineering, 2016, 13(5): 935-968. doi: 10.3934/mbe.2016024 |
Meta-heuristic optimization algorithms develop rapidly [1,2,3] because of its simple concept, flexibility and ability to avoid local optima, and have been widely used in solving various complex optimization problems in the real world [4,5]. According to different inspiration of the algorithms, meta-heuristics can be divided into three main categories: evolutionary, physics-based and swarm intelligence based techniques. The inspirations of evolutionary algorithms are the laws of evolution in nature. There are some representative evolutionary algorithms such as Genetic Algorithm (GA) [6], Differential Evolution Algorithm (DE) [7], Evolution Strategy (ES) [8], Biogeography-Based Optimizer (BBO) [9] and Probability-Based Incremental Learning (PBIL) [10]. Inspired by the physical rules of the universe, physics-based techniques include Simulated Annealing (SA) [11], Gravity Search Algorithm (GSA) [12], Black Hole Algorithm (BH) [13], Multi-Verse Optimizer (MVO) [14], Sine Cosine Algorithm (SCA) [15], Arithmetic Optimization Algorithm (AOA) [16], Heat Transfer Relation-based Optimization Algorithm (HTOA) [17] and so forth. Swarm intelligence (SI) based methods belong to the most popular category, which are inspired by swarm behaviors of creatures in nature. The representative SI algorithms include Particle Swarm Optimization (PSO) [18], Ant Colony Optimization Algorithm (ACO) [19], Firefly Algorithm (FA) [20], Grey Wolf Optimizer (GWO) [21], Cuckoo Search Algorithm (CS) [22], Whale Optimization Algorithm (WOA) [23], Salp Swarm Algorithm (SSA) [24], Remora Optimization Algorithm [25], Slime Mould Algorithm (SMA) [26], and Horse herd Optimization Algorithm (HOA) [27].
The Aquila Optimizer (AO) [28] and Harris Hawks Optimization (HHO) [29] are both latest SI algorithms that simulate hunting behaviors of Aquila and Harris' hawks respectively. Due to the short time for AO to be proposed, there is no research on the improvement of AO yet, but AO has been used to solve the real-world optimization problems. AlRassas et al. [30] applied AO to optimize parameters of Adaptive Neuro-Fuzzy Inference System (ANFIS) model to boost the prediction accuracy of oil production forecasting. This research revels the good practicable performance of AO. For another thing, once the HHO was proposed, it attracted a large number of researchers to improve or apply it to solve optimization problems in many fields. Chen et al. [31] proposed the first powerful variant of HHO by integrating chaos, topological multi-population, and differential evolution (DE) strategies. Chaos mechanism is for exploitation, multi-population strategy is for global search ability, and the DE mechanism is for increasing the accuracy of the solutions. Inspired by the survival-of-the-fittest principle of evolutionary algorithms, Al-Betar et al. [32] proposed three new versions of HHO incorporated tournament, proportional and linear rank-based strategies respectively to accelerate convergence. The proposed new versions show a better balance between the exploration and exploitation and enhance local optima avoidance as well. Song et al. [33] utilized dimension decision strategy in CS to improve the convergence speed, and Gaussian mutation to increase the convergence accuracy and premature convergence avoidance. Yousri et al. [34] improved the exploration performance of HHO using the fractional calculus (FOC) memory concept. The hawks move with a fractional-order velocity, and the escaping energy of prey is adaptively adjusted based on FOC parameters to avoid local optima stagnation. Gupta et al. [35] enhanced the search-efficiency and premature convergence avoidance of HHO by adding a nonlinear energy parameter, different settings for rapid dives, opposition-based learning strategy and a greedy selection mechanism. Akdag et al. [36] introduced seven types of random distribution functions to increase the performance of HHO, and then applied the modified HHO to solve optimum power flow (OPF) problem. Yousri et al. [37] applied HHO to optimize parameters of the Proportional-Integral controller for designing load frequency control (LFC). Jia et al. [38] proposed a dynamic HHO using a mutation mechanism to avoid local optima and enhance the search capability. This improved HHO was applied for satellite image segmentation as well.
Otherwise, there are also attempts of hybrid algorithm of HHO. Hussain et al. [39] integrated sine-cosine algorithm (SCA) in HHO for numerical optimization and feature selection. The SCA integration is used to cater ineffective exploration in HHO, moreover exploitation is enhanced by dynamically adjusting candidate solutions for avoiding solution stagnancy in HHO. Bao et al. [40] proposed HHO-DE by hybridizing HHO and DE algorithms. The convergence accuracy, ability to avoid local optima and stability are greatly improved compared to HHO and DE. Houssein et al. [41] proposed a hybrid algorithm called CHHO-CS by combining HHO with CS and chaotic maps. The CHHO-CS achieves a better balance between exploration and exploitation phases, and effectively avoids premature convergence. Kaveh et al. [42] hybridized HHO with Imperialist Competitive Algorithm (ICA). Combination of the exploration strategy of ICA and exploitation technique of HHO helps to achieve a better search performance. The satisfactory outcomes of several HHO-based hybrid algorithms proposed in the literature show potential research direction.
Thus, in view of defects in the slow convergence and local optima stagnation of HHO and inspired by the above researches, we try a hybridization to enhance the performance of HHO and AO. An improved hybrid Aquila Optimizer and Harris Hawks Optimization namely IHAOHHO is proposed. First of all, we combine the exploration phase of AO with the exploitation phase of HHO together. This operation extracts and retains the strong exploration and exploitation capabilities of basic AO and HHO. Then, in order to further improve the performance of IHAOHHO, the representative-based hunting (RH) and opposition-based learning (OBL) strategies are introduced into IHAOHHO. RH is mixed into the exploration phase to increase the diversification and OBL is added into the exploitation phase to avoid local optima stagnation, respectively. Thus, the capabilities of exploration, exploitation and local optima avoidance are effectively enhanced in the proposed algorithm. The standard and CEC2017 benchmark functions and three engineering design problems are utilized to test the exploration and exploitation capabilities of IHAOHHO. The proposed algorithm is compared with basic AO, HHO, and several well-known meta-heuristic algorithms, including HOA, SSA, WOA, GWO, MVO, IPOP-CMA-ES [43], LSHADE [44], Sine-cosine and Spotted Hyena-based Chimp Optimization Algorithm (SSC) [45] and RUNge Kutta Optimizer (RUN) [46]. The experimental results show that the proposed IHAOHHO algorithm outperforms other state-of-the-art algorithms.
The rest of this paper is organized as follows: The Section 2, provides a brief overview of the related work: basic AO and HHO algorithms, as well as the RH and OBL strategies. The Section 3, describes in detail the proposed hybrid algorithm. The Section 4, conducts simulation experiments and results analysis. Finally, Section 5, concludes the paper.
AO is a latest novel swarm intelligence algorithm proposed by Abualigah et al. in 2021. There are four hunting behaviors of Aquila for different kinds of prey. Aquila can switch hunting strategies flexibly for different prey, and then uses its fast speed united with sturdy feet and claws to attack prey. The brief description of mathematical model can be described as follows.
Step 1: Expanded exploration: high soar with a vertical stoop
In this method, the Aquila flies high over the ground and explorers the search space widely, and then a vertical dive will be taken once the Aquila determines the area of the prey. The mathematical representation of this behavior is written as:
X(t+1)=Xbest(t)×(1−tT)+(XM(t)−Xbest(t)×rand) | (1) |
XM(t)=1NN∑i=1Xi(t) | (2) |
where Xbest(t) represents the best position obtained so far, and XM(t) denotes to the average position of all Aqulias in current iteration. t and T are the current iteration and the maximum number of iterations, respectively. N is the population size and rand is a random number between 0 and 1.
Step 2: Narrowed exploration: contour flight with short glide attack
This is the most commonly used hunting method for Aquila. It uses short gliding to attack the prey after descending within the selected area and flying around the prey. The position update formula is represented as:
X(t+1)=Xbest(t)×LF(D)+XR(t)+(y−x)×rand | (3) |
where, XR(t) represents a random position of the hawk, and D is the dimension size. LF(D) represents Levy flight function, which is presented as follows:
LF(D)=s×u×σ|v|1β | (4) |
σ=(Γ(1+β)×sin(πβ2)Γ(1+β2)×β×2(β−12)) | (5) |
where, s and β are constant values equal to 0.01 and 1.5 respectively, u and v are random numbers between 0 and 1. y and x are used to present the spiral shape in the search, which are calculated as follows:
{x=r×sin(θ)y=r×cos(θ)r=r1+0.00565×D1θ=−ω×D1+3×π2 | (6) |
where, r1 means the number of search cycles between 1 and 20, D1 is composed of integer numbers from 1 to the dimension size (D), and ω is equal to 0.005.
Step 3: Expanded exploitation: low flight with a slow descent attack
In the third method, when the area of prey is roughly determined, the Aquila descends vertically to do a preliminary attack. AO exploits the selected area to get close to and attack the prey. This behavior is presented as follows:
X(t+1)=(Xbest(t)−XM(t))×α−rand+((UB−LB)×rand+LB)×δ | (7) |
where α and δ are the exploitation adjustment parameters fixed to 0.1, UB and LB are the upper and lower bounds of the problem.
Step 4: Narrowed exploitation: walking and grab prey
In this method, the Aquila chases the prey in the light of its escape trajectory, and then attacks the prey on the ground. The mathematical representation of this behavior is as follows:
{X(t+1)=QF×Xbest(t)−(G1×X(t)×rand)−G2×LF(D)+rand×G1QF(t)=t2×rand−1(1−T)2G1=2×rand−1G2=2×(1−tT) | (8) |
where X(t) is the current position, and QF(t) represents the quality function value which used to balance the search strategy. G1 denotes the movement parameter of Aquila during tracking prey, which is a random number between [-1, 1]. G2 denotes the flight slope when chasing prey, which decreases linearly from 2 to 0.
The flowchart of AO is shown in Figure 1.
HHO is a new meta-heuristic optimization algorithm proposed by Heidari et al. in 2019. It is inspired by the unique cooperative foraging activities of Harris' hawk. Harris' hawk can show a variety of chasing patterns according to the dynamic nature of the environment and the escaping patterns of the prey. These switching activities are conducive to confuse the running prey, and the cooperative strategies can help Harris' hawk chase the detected prey to exhaustion, which increases its vulnerability. The brief description of mathematical model is as follows.
The Harris' hawks usually perch on some random locations, wait and monitor the desert to detect the prey. There are two perching strategies based on the positions of other family members and the prey, which are selected in accordance with the random q value.
X(t+1)={XR(t)−rand|Xr(t)−2rand×X(t)|q⩾0.5(Xbest(t)−XM(t))−rand(LB+rand(UB−LB))q < 0.5 | (9) |
where q is random number between 0 and 1.
The HHO algorithm has a transition mechanism from exploration to exploitation phase based on the escaping energy of the prey, and then changes the different exploitative behaviors. The energy of the prey is modeled as follows, which decreases during the escaping behavior.
E=2E0(1−tT) | (10) |
where E represents the escaping energy of the prey, E0 is the initial state of the energy. When |E|⩾1, the algorithm performs the exploration stage, and when |E|<1, the algorithm performs the exploitation phase.
In this phase, four different chasing and attacking strategies are proposed on the basis of the escaping energy of the prey and chasing styles of the Harris' hawks. Except for the escaping energy, parameter r is also utilized to choose the chasing strategy, which indicates the chance of the prey in successfully escaping (r<0.5) or not (r⩾0.5) before attack.
i.Soft besiege
When r⩾0.5 and |E|⩾0.5, the prey still has enough energy and tries to escape, so the Harris' hawks encircle it softly to make the prey more exhausted and then attack it. This behavior is modeled as follows:
X(t+1)=ΔX(t)−E|JXbest(t)−X(t)| | (11) |
ΔX(t)=Xbest(t)−X(t) | (12) |
J=2(1−rand) | (13) |
where ΔX(t) indicates the difference between the position of prey and the current position, J represents the random jump strength of prey.
ii.Hard besiege
When r⩾0.5 and |E|<0.5, the prey has a low escaping energy, and the Harris' hawks encircle the prey readily and finally attack it. In this situation, the positions are updated as follows:
X(t+1)=Xbest(t)−E|ΔX(t)| | (14) |
iii.Soft besiege with progressive rapid dives
When |E|⩾0.5 and r<0.5, the prey has enough energy to successfully escape, so the Harris' hawks perform soft besiege with several rapid dives around the prey and try to progressively correct its position and direction. This behavior is modeled as follows:
Y=Xbest(t)−E|JXbest(t)−X(t)| | (15) |
Z=Y+S×LF(D) | (16) |
X(t+1)={YifF(Y)<F(X(t))ZifF(Z)<F(X(t)) | (17) |
where S is a random vector. Note that, only the better position between Y and Z is selected as the next position.
iv.Hard besiege with progressive rapid dives
When |E|<0.5 and r<0.5, the prey has no enough energy to escape, so the hawks perform a hard besiege to decrease the distance between their average position and the prey, and finally attack and kill the prey. The mathematical representation of this behavior is as follows:
Y=Xbest(t)−E|JXbest(t)−XM(t)| | (18) |
Z=Y+S×LF(D) | (19) |
X(t+1)={YifF(Y)<F(X(t))ZifF(Z)<F(X(t)) | (20) |
Note that only the better position between Y and Z will be the next position for the new iteration.
The flowchart of HHO is displayed in Figure 2.
The strategy of representative-based hunting was first proposed to improve the exploration and diversification of GWO algorithm in 2021 [47]. To achieve this, an archive called representative archive (RA) is constructed to maintain the representative solutions. A random representative search agent is selected from the five-best search agents archived by the RA, and a random search agent is selected from the RA. Meanwhile, two random search agents are selected from the population. These four selections efficiently improve the diversity, exploration capability and premature convergence avoidance. The mathematical model of RH is as follows:
X(t + 1) = XR_best+cd×(X(t)−XR_archive)+σ×(Xrand1−Xrand2) | (21) |
where XR_best and XR_archive are randomly selected from the five-best representative search agents and the whole archive, respectively. Xrand1 and Xrand2 are randomly selected from the whole population. σ and the Cauchy distribution cd are calculated by:
σ = (T−tT−1)Exponent×(σinitial−σfinal)+σfinal | (22) |
cd = z0+0.1×tan(π(rand−0.5)) | (23) |
σ is a nonlinearly decreasing parameter form 1 to 0, which leads to decrease the exploration to exploitation over the course of iterations, forming a transition from exploration to exploitation. The cd coefficient assists to enhance the random behavior, preferring exploration and escaping from the local optima.
Opposition-based learning (OBL) is a powerful optimization tool proposed by Tizhoosh in 2005 [48]. The main idea of OBL is simultaneously considering the fitness of an estimate and its corresponding counter estimate to obtain a better candidate solution (Figure 3). An optimization process usually starts at a random initial solution. If the random solution is near the optimal solution, the algorithm converges fast. However, it's possible that the initial solution is far away from the optimum or just at exact opposite position. In this case, it might take quite long time to converge or not converge at all. Thus, considering the opposite direction of the candidate solution in each step increases the probability of finding a better solution. We can choose the opposite point as the candidate solution once the opposite solution is beneficial and then proceed to the next iteration. The OBL concept has successfully been used in varieties of meta-heuristics algorithms [49,50,51,52,53] to improve the convergence speed. OBL is defined by:
xjOBL=lj+uj−xj,j=1,2,…,n | (24) |
where xjOBL represents the opposite solution, ljand ujare the lower and upper bounds of the problem in jth dimension. The opposite solution described by Eq (24) can effectively help the population jump out of the local optima.
The AO simulates hunting behaviors for fast-moving prey within a wide flying area in exploration phase. The characteristics of these behaviors make AO have a strong global search ability and fast convergence speed. However, the selected search space is not exhaustively searched during the exploitation phase. The role of Levy flight is weak in the late iterations, which tends to result in premature convergence. Thus, the AO algorithm possesses good exploration capability and fast convergence speed, but it is hard to escape from local optima in the exploitation stage. For the HHO algorithm, the experimental results show that deficiencies of insufficient diversification of the population and low convergence speed exist in the exploration phase. On the basis of the energy and escape probability of the prey, four different hunting strategies are used to implement various position updating methods in the exploitation phase. In addition, the transition mechanism from exploration to exploitation is a good way to adapt to animal characteristics. As a whole, the energy of prey decreases with the increase of iterations, making the algorithm enter the exploitation stage.
In this work, we retain the exploration phase of AO and the exploitation phase of HHO, and combine them together through the transition mechanism. The exploration phase of HHO is highly dominant with randomization that seems clueless search mechanism. In contrast, the position updating in exploration phase of AO is based on the best solution and average position with some randomness, which is more reasonable. And the four exploitation strategies based on the different values of E and r help the algorithm fully exploit the search space. This hybridization is beneficial to give full play to the advantages of these two basic algorithms. The global search capability, faster convergence speed, and detailed exploitation of the algorithms are all reserved. However, the diversity of the population in the exploration phase is insufficient due to the lack of randomness. As described in Section 2.3, RH is designed for improving the exploration and diversification of an optimization algorithm. Selections from different sub-population can efficiently improve the diversity and exploration capability. Thus, RH strategy is utilized to further improve the diversification of the population in exploration phase, which is conducive to find the most promising region quickly. Besides, AO and HHO possess a common defect of local optima stagnation. The OBL strategy can utilize the opposite solution to make the population jump out of the local optima. Therefore, OBL strategy is added to the exploitation phase to enhance the ability to jump out of the local optima as well. All these strategies effectively improve the convergence speed, convergence accuracy and the overall optimization performance of the hybrid algorithm. This improved hybrid Aquila Optimizer and Harris Hawks Optimization algorithm is named IHAOHHO. Different phases of IHAOHHO are illustrated in Figure 4. The pseudo-code of IHAOHHO is given in Algorithm 1, and the summarized flowchart is shown in Figure 5.
Algorithm 1 Pseudo-code of IHAOHHO |
1: Set the initial values of the population size N and the maximum number of iterations T |
2: Initialize positions of the population X |
3: While t < T |
4: Update x, y, cd |
5: For i = 1 to N |
6: If t < T/2 % Exploration part of AO |
7: Archiving |
8: If rand < 0.5 |
9: Update the position of Xnewi and X_Ri using Eqs (1) and (21), respectively |
10: Calculate the fitness of Xnewi and X_Ri |
11: Update the position of Xi |
12: Else |
13: Update the position of Xnewi and X_Ri using Eqs (3) and (21), respectively |
14: Calculate the fitness of Xnewi and X_Ri |
15: Update the position of Xi |
16: End if |
17: Else % Exploitation part of HHO |
18: If r ≥ 0.5 and |E| ≥ 0.5 |
19: Update the position of Xi using Eq (11) |
20: End if |
21: If r ≥ 0.5 and |E| < 0.5 |
22: Update the position of Xi using Eq (14) |
23: End if |
24: If r < 0.5 and |E| ≥ 0.5 |
25: Update the position of Xi using Eq (15) |
26: End if |
27: If r < 0.5 and |E| < 0.5 |
28: Update the position of Xi using Eq (18) |
29: End if |
30: Update the position of Xnewi using Eq (24) % Opposition-based learning (OBL) |
31: If f(Xnewi) < f(Xi) |
32: Xi = Xnewi |
33: End if |
34: End if |
35: t = t + 1 |
36: End for |
37: For i = 1 to N |
38: Check if the position goes out of the search space boundary and bring it back. |
39: Calculate the fitness of Xi |
40: Update Xbest |
41: End for |
42: End while |
43: Return Xbest |
The computational complexity is an important indicator for an algorithm, which is used to evaluate its time consumption during operating. The computational complexity of the IHAOHHO depends on three rules: initialization, evaluation of fitness, and updating of hawks. In the initialization stage, the computational complexity of positions generated of N hawks is O(N). Then, the computational complexity of fitness evaluation for the best solution is O(N) during the iteration process. Finally, the computational complexities of position updating of hawks and fitness comparison in one iteration are O(2 × N × D) and O(N) respectively, where D is dimension size of the problem. Therefore, the total computational complexity of the proposed IHAOHHO algorithm is O(N × (1 + 2 × D × T + 2 × T)). As described in the literature, the computational complexity of AO and HHO are both O(N × (1 + D × T + T)). Compared to the basic AO and HHO, the computational complexity of IHAOHHO is slightly increased due to the RH and OBL strategies, which is acceptable for improving the convergence accuracy and speed of the algorithm.
In this section, we implement four main experiments to evaluate the performance of the proposed IHAOHHO algorithm. Standard benchmark function experiment is carried out firstly, which is used to evaluate the performance of the algorithm in solving 23 simple numerical optimization problems. Secondly, the CEC2017 benchmark functions are tested to assess the performance of the algorithm in solving complex numerical optimization problems. Then, sensitivity analysis is performed to investigate the effect of the control parameters. The last one is engineering design problems, which aims to assess the performance of IHAOHHO in solving real-world problems. All experiments are implemented in MATLAB R2016a on a PC with Intel (R) core (TM) i5-9500 CPU @ 3.00GHz and RAM 16GB memory on OS windows 10.
We utilize 23 standard benchmark functions to test the performance of the IHAOHHO algorithm, which are divided into three types including unimodal, multimodal and fixed-dimension multimodal benchmark functions. The main characteristic of unimodal functions is that there is only one global optimum but no local optima. This kind of functions can be used to evaluate the exploitation capability and convergence rate of an algorithm. Unlike unimodal functions, multimodal and fixed-dimension multimodal functions have one global optimum and multiple local optima. These types of functions are utilized to evaluate the exploration and local optima avoidance capabilities. The benchmark function details are listed in Tables 1-3.
Function | Dim | Range | fmin |
F1(x)=∑ni=1x2i | 30 | [-100, 100] | 0 |
F2(x)=∑ni=1|xi|+∏ni=1|xi| | 30 | [-10, 10] | 0 |
F3(x)=∑ni=1(∑ij−1xj)2 | 30 | [-100, 100] | 0 |
F4(x)=maxi{|xi|,1⩽i⩽n} | 30 | [-100, 100] | 0 |
F5(x)=∑n−1i=1[100(xi+1−x2i)2+(xi−1)2] | 30 | [-30, 30] | 0 |
F6(x)=∑ni=1(xi+5)2 | 30 | [-100, 100] | 0 |
F7(x)=∑ni=1ix4i+random[0,1) | 30 | [-1.28, 1.28] | 0 |
Function | Dim | Range | fmin |
F8(x)=∑ni=1−xisin(√|xi|) | 30 | [-500, 500] | -418.9829 × 30 |
F9(x)=∑ni=1[x2i−10cos(2πxi)+10] | 30 | [-5.12, 5.12] | 0 |
F10(x)=−20exp(−0.2√1n∑ni=1x2i)−exp(1n∑ni=1cos(2πxi))+20+e | 30 | [-32, 32] | 0 |
F11(x)=14000∑ni=1x2i−∏ni=1cos(xi√i)+1 | 30 | [-600, 600] | 0 |
F12(x)=πn{10sin(πy1)+∑n−1i=1(yi−1)2[1+10sin2(πyi+1)]+(yn}+∑ni=1u(xi,10,100,4), where yi=1+xi+14,u(xi,a,k,m)={k(xi−a)mxi>a0−a<xi<ak(−xi−a)mxi<−a | 30 | [-50, 50] | 0 |
F13(x)=0.1(sin2(3πx1)+∑ni=1(xi−1)2[1+sin2(3πxi+1)]+(xn−1)2[1+sin2(2πxn)])+∑ni=1u(xi,5,100,4) | 30 | [-50, 50] | 0 |
Function | Dim | Range | fmin |
F14(x)=(1500+∑25j=11j+∑2i=1(xi−aij)6)−1 | 2 | [-65, 65] | 1 |
F15(x)=∑11i=1[ai−x1(b2i+bix2)b2i+bix3+x4]2 | 4 | [-5, 5] | 0.00030 |
F16(x)=4x21−2.1x41+13x61+x1x2−4x22+x42 | 2 | [-5, 5] | -1.0316 |
F17(x)=(x2−5.14π2x21+5πx1−6)2+10(1−18π)cosx1+10 | 2 | [-5, 5] | 0.398 |
F18(x)=[1+(x1+x2+1)2(19−14x1+3x21−14x2+6x1x2+3x22)]×[30+(2x1−3x2)2×(18−32x2+12x21+48x2−36x1x2+27x22)] | 2 | [-2, 2] | 3 |
F19(x)=−∑4i=1ciexp(−∑3j=1aij(xj−pij)2) | 3 | [-1, 2] | -3.86 |
F20(x)=−∑4i=1ciexp(−∑6j=1aij(xj−pij)2) | 6 | [0, 1] | -3.32 |
F21(x)=−∑5i=1[(X−ai)(X−ai)T+ci]−1 | 4 | [0, 10] | -10.1532 |
F22(x)=−∑7i=1[(X−ai)(X−ai)T+ci]−1 | 4 | [0, 10] | -10.4028 |
F23(x)=−∑10i=1[(X−ai)(X−ai)T+ci]−1 | 4 | [0, 10] | -10.5363 |
For verification of the results, IHAOHHO is compared with the basic AO, HHO, and HOA, SSA, WOA, GWO, MVO as several well-known meta-heuristic algorithms. For all tests, we set the population size N = 30, dimension size D = 30, maximum number of iterations T = 500, and run 30 times independently. The parameter settings of each algorithm are shown in Table 4. After all, the average and standard deviation results of these test functions are exhibited in Table 5. Figure 6 shows the convergence curves of 23 test functions. The partial search history, trajectory and average fitness maps are shown in Figure 7. The Wilcoxon signed-rank test results are also listed in Table 6.
Algorithm | Parameters |
IHAOHHO | σinitial = 1; σfinal = 0; Exponent = 2 |
AO | U = 0.00565; r1 = 10; ω = 0.005; α = 0.1; δ = 0.1; G1∈[-1, 1]; G2 = [2,0] |
HHO | q∈[0, 1]; r∈[0, 1]; E0∈[-1, 1]; E1 = [2,0]; E∈ [-2, 2]; |
HOA | hβ = 0.9; hγ = 0.5; sβ = 0.2; sγ = 0.1; iγ = 0.3; dα = 0.5; dβ = 0.2; dγ = 0.1; rδ = 0.1; rγ = 0.05 |
SSA | c1 = [1,0]; c2∈[0, 1]; c3∈[0, 1] |
WOA | a1 = [2,0]; a2 = [-1, -2]; b = 1 |
GWO | a = [2,0] |
MVO | WEP ∈ [0.2, 1]; TDR ∈ [0, 1]; r1, r2, r3 ∈ [0, 1] |
F | IHAOHHO | AO | HHO | HOA | SSA | WOA | MVO | GWO | |
F1 | Avg | 3.3660E-253 | 7.9345E-97 | 3.3401E-96 | 1.7886E-130 | 2.1594E-07 | 1.8120E-73 | 1.2681 | 1.0585E-27 |
Std | 0 | 4.3457E-96 | 1.7664E-95 | 8.6475E-130 | 3.8328E-07 | 8.0771E-73 | 3.8945E-01 | 9.7097E-28 | |
F2 | Avg | 1.5599E-127 | 1.7619E-60 | 1.9990E-49 | 2.1595E-66 | 1.7056 | 8.8551E-51 | 3.7930 | 7.8893E-17 |
Std | 8.5347E-127 | 9.6504E-60 | 9.5039E-49 | 1.1828E-65 | 1.3736 | 3.2476E-50 | 1.6432E+01 | 5.7891E-17 | |
F3 | Avg | 2.7431E-199 | 2.2314E-104 | 8.4798E-72 | 1.7856E+02 | 1.3173E+03 | 4.4381E+04 | 2.2448E+02 | 2.6928E-05 |
Std | 0 | 1.2222E-103 | 4.4387E-71 | 5.2479E+02 | 1.1198E+03 | 1.1940E+04 | 1.2075E+03 | 6.0686E-05 | |
F4 | Avg | 2.2207E-129 | 1.6827E-69 | 1.1057E-49 | 4.5440E-65 | 1.2612E+01 | 5.6993E+01 | 2.1139 | 8.0152E-07 |
Std | 1.1068E-128 | 7.6613E-69 | 3.0441E-49 | 2.4668E-64 | 3.8228 | 2.5529E+01 | 1.0284 | 8.3055E-07 | |
F5 | Avg | 5.3852E-04 | 5.4421E-03 | 2.1438E-02 | 2.8964E+01 | 2.2071E+02 | 2.7871E+01 | 3.8794E+02 | 2.7221E+01 |
Std | 2.2657E-03 | 1.2008E-02 | 2.4291E-02 | 3.5795E-02 | 3.4556E+02 | 4.6073E-01 | 7.0618E-02 | 7.9162E-01 | |
F6 | Avg | 3.5863E-06 | 1.2028E-04 | 1.4716E-04 | 6.5645 | 2.5056E-07 | 3.4988E-01 | 1.1414 | 7.2412E-01 |
Std | 7.7280E-06 | 1.7490E-04 | 2.0847E-04 | 3.4100E-01 | 3.8211E-07 | 1.7102E-01 | 4.4812E-01 | 3.9184E-01 | |
F7 | Avg | 9.5324E-05 | 1.0504E-04 | 1.3211E-04 | 6.7657E-02 | 1.6706E-01 | 2.7105E-03 | 3.4554E-02 | 2.1436E-03 |
Std | 7.6717E-05 | 8.6163E-05 | 1.4567E-04 | 4.0688E-02 | 7.8018E-02 | 3.2053E-03 | 1.5231E-02 | 1.3151E-03 | |
F8 | Avg | -12569.3858 | -8010.0774 | -12569.0257 | -4106.2204 | -7362.4999 | -10632.1912 | -7648.8103 | -6101.7628 |
Std | 1.8228E-01 | 4.0684E+03 | 7.5912E-01 | 7.7530E+02 | 8.6690E+02 | 0 | 5.2123E+02 | 8.9531E+02 | |
F9 | Avg | 0 | 0 | 0 | 8.0284E+01 | 5.3197E+01 | 0 | 1.2456E+02 | 3.3205 |
Std | 0 | 0 | 0 | 1.0686E+02 | 2.0211E+01 | 4.6777E-15 | 3.4040E+01 | 4.302 | |
F10 | Avg | 8.8818E-16 | 8.8818E-16 | 8.8818E-16 | 5.7436E-15 | 2.6880 | 2.7886E-15 | 1.7605 | 9.8230E-14 |
Std | 0 | 0 | 0 | 1.7413E-15 | 6.5906E-01 | 2.421E-15 | 6.6839E-01 | 1.7092E-14 | |
F11 | Avg | 0 | 0 | 0 | 2.0978E-01 | 2.3006E-02 | 1.1525E-02 | 8.6051E-01 | 5.3578E-03 |
Std | 0 | 0 | 0 | 3.7796E-01 | 1.5437E-02 | 4.4029E-02 | 8.3088E-02 | 7.3633E-03 | |
F12 | Avg | 2.6974E-07 | 2.8068E-06 | 1.9506E-05 | 1.1656 | 7.8473 | 2.1363E-02 | 1.8746 | 5.7227E-02 |
Std | 4.4163E-07 | 5.7142E-06 | 3.8352E-05 | 2.1289E-01 | 2.7926 | 1.2107E-02 | 1.1276 | 9.6891E-02 | |
F13 | Avg | 3.0227E-06 | 4.9439E-05 | 9.7772E-05 | 3.0571 | 1.8860E+01 | 5.3918E-01 | 1.7158E-01 | 6.2217E-01 |
Std | 5.0785E-06 | 9.2988E-05 | 9.5928E-05 | 1.8645E-01 | 1.6484E+01 | 2.9800E-01 | 1.0772E-01 | 2.5487E-01 | |
F14 | Avg | 1.5932 | 2.0487 | 1.2629 | 2.9322 | 1.3943 | 2.8615 | 0.998 | 4.6223 |
Std | 9.2477E-01 | 2.1859 | 5.1727E-01 | 2.1542 | 9.5834E-01 | 3.3284 | 2.7158E-11 | 4.1450 | |
F15 | Avg | 4.4227E-04 | 4.9359E-04 | 3.9582E-04 | 7.1269E-03 | 2.2249E-03 | 7.5110E-04 | 7.8593E-03 | 5.7748E-03 |
Std | 3.4587E-04 | 1.1786E-04 | 2.3573E-04 | 7.5345E-03 | 4.9361E-03 | 4.9981E-04 | 1.3437E-02 | 8.9518E-03 | |
F16 | Avg | -1.0316 | -1.0312 | -1.0316 | -0.99752 | -1.0316 | -1.0316 | -1.0316 | -1.0316 |
Std | 3.1088E-08 | 4.0636E-04 | 7.1130E-09 | 3.1957E-02 | 1.8547E-14 | 7.6118E-10 | 4.5176E-07 | 3.6867E-08 | |
F17 | Avg | 3.9789E-01 | 3.9813E-01 | 3.9789E-01 | 3.9790E-01 | 3.9789E-01 | 3.9790E-01 | 3.9789E-01 | 3.9789E-01 |
Std | 4.0428E-05 | 3.0290E-04 | 4.5913E-05 | 1.1204E-03 | 9.2471E-15 | 1.5755E-05 | 7.7424E-08 | 7.6565E-07 | |
F18 | Avg | 3 | 3.0243 | 3 | 7.7479 | 3 | 3.0001 | 3 | 3 |
Std | 3.3248E-06 | 5.5712E-02 | 6.1620E-07 | 1.6153E+01 | 1.9031E-13 | 3.1781E-04 | 2.5080E-06 | 4.7166E-05 | |
F19 | Avg | -3.8258 | -3.8535 | -3.8597 | -3.8619 | -3.8628 | -3.8551 | -3.8628 | -3.8598 |
Std | 7.1972E-02 | 8.7650E-03 | 3.8900E-03 | 6.2715E-04 | 1.9037E-12 | 8.6573E-03 | 3.7043E-06 | 3.9554E-03 | |
F20 | Avg | -3.0792 | -3.1678 | -3.0813 | -3.2580 | -3.2335 | -3.2080 | -3.2448 | -3.2721 |
Std | 1.1913E-01 | 7.3334E-02 | 9.9164E-02 | 7.5769E-02 | 6.4415E-02 | 1.1882E-01 | 5.9771E-02 | 7.6156E-02 | |
F21 | Avg | -10.1525 | -10.1422 | -5.2145 | -9.3937 | -6.6406 | -9.2821 | -6.9627 | -9.6452 |
Std | 1.4463E-03 | 1.8290E-02 | 8.8739E-01 | 1.2967 | 3.4554 | 1.9234 | 3.1618 | 1.5462 | |
F22 | Avg | -10.4026 | -10.3914 | -5.0820 | -9.5706 | -9.7954 | -7.6313 | -8.6587 | -10.4009 |
Std | 6.6551E-04 | 2.1551E-02 | 8.7380E-03 | 1.5379 | 1.8875 | 3.0683 | 2.7631 | 1.2358E-03 | |
F23 | Avg | -10.5359 | -10.5292 | -5.1234 | -9.8166 | -8.7191 | -7.0303 | -7.5296 | -10.5348 |
Std | 6.6691E-04 | 1.2664E-02 | 52035E-03 | 1.0080 | 3.1206 | 3.4442 | 3.5941 | 8.4873E-04 |
F | IHAOHHO vs AO | IHAOHHO vs HHO | IHAOHHO vs HOA | IHAOHHO vs SSA | IHAOHHO vs WOA | IHAOHHO vs MVO | IHAOHHO vs GWO |
F1 | 6.1035E-05 | 6.1035E-05 | 6.1035E-05 | 6.1035E-05 | 6.1035E-05 | 6.1035E-05 | 6.1035E-05 |
F2 | 6.1035E-05 | 6.1035E-05 | 6.1035E-05 | 6.1035E-05 | 6.1035E-05 | 6.1035E-05 | 6.1035E-05 |
F3 | 6.1035E-05 | 6.1035E-05 | 6.1035E-05 | 6.1035E-05 | 6.1035E-05 | 6.1035E-05 | 6.1035E-05 |
F4 | 6.1035E-05 | 6.1035E-05 | 6.1035E-05 | 6.1035E-05 | 6.1035E-05 | 6.1035E-05 | 6.1035E-05 |
F5 | 0.10699 | 1.2207E-04 | 6.1035E-05 | 6.1035E-05 | 6.1035E-05 | 6.1035E-05 | 6.1035E-05 |
F6 | 1.8311E-04 | 1.8311E-04 | 6.1035E-05 | 1.1597E-03 | 6.1035E-05 | 6.1035E-05 | 6.1035E-05 |
F7 | 6.3721E-02 | 0.10699 | 6.1035E-05 | 6.1035E-05 | 6.1035E-05 | 6.1035E-05 | 6.1035E-05 |
F8 | 6.1035E-05 | 0.63867 | 6.1035E-05 | 6.1035E-05 | 6.1035E-05 | 6.1035E-05 | 6.1035E-05 |
F9 | NaN | NaN | 6.1035E-05 | 6.1035E-05 | NaN | 6.1035E-05 | 6.1035E-05 |
F10 | NaN | NaN | 6.1035E-05 | 6.1035E-05 | 1.9531E-03 | 6.1035E-05 | 6.1035E-05 |
F11 | NaN | NaN | 0.1250 | 6.1035E-05 | NaN | 6.1035E-05 | NaN |
F12 | 2.6245E-03 | 1.2207E-04 | 6.1035E-05 | 6.1035E-05 | 6.1035E-05 | 6.1035E-05 | 6.1035E-05 |
F13 | 2.1545E-02 | 1.2207E-04 | 6.1035E-05 | 6.1035E-05 | 6.1035E-05 | 6.1035E-05 | 6.1035E-05 |
F14 | 0.97797 | 0.45428 | 1.8066E-02 | 2.6245E-03 | 0.18762 | 6.1035E-05 | 3.5339E-02 |
F15 | 6.1035E-05 | 0.45428 | 6.1035E-05 | 6.1035E-05 | 6.1035E-05 | 6.1035E-05 | 0.3028 |
F16 | 6.1035E-05 | 8.3618E-03 | 6.1035E-05 | 6.1035E-05 | 9.4604E-02 | 6.1035E-05 | 5.5359E-02 |
F17 | 6.1035E-04 | 0.45428 | 6.1035E-05 | 6.1035E-05 | 0.27686 | 3.0518E-04 | 1.0254E-02 |
F18 | 8.3252E-02 | 1.2207E-04 | 8.3252E-02 | 6.1035E-05 | 0.48871 | 0.67877 | 0.13538 |
F19 | 7.2998E-02 | 1.8066E-02 | 7.2998E-02 | 6.1035E-05 | 1.8066E-02 | 6.1035E-05 | 3.0151E-02 |
F20 | 6.1035E-04 | 0.93408 | 8.5449E-04 | 1.5259E-03 | 2.0142E-03 | 6.1035E-05 | 8.5449E-04 |
F21 | 3.3569E-03 | 6.1035E-05 | 6.1035E-05 | 4.2120E-02 | 1.2207E-04 | 2.0142E-03 | 1.8311E-04 |
F22 | 1.2207E-04 | 6.1035E-05 | 6.1035E-05 | 8.3252E-02 | 1.8311E-04 | 4.126E-02 | 8.3618E-03 |
F23 | 1.1597E-03 | 6.1035E-05 | 6.1035E-05 | 3.3026E-02 | 6.1035E-05 | 1.6882E-02 | 6.7139E-03 |
Unimodal test functions are usually used to investigate the exploitation capability of the algorithm since they have only one global optimum and no local optima. As seen from Table 5, the IHAOHHO performs much better than other selected algorithms exclude F6. For all unimodal functions exclude F6, IHAOHHO obtains the smallest average values and standard deviations compared to other algorithms, which indicate the best accuracy and stability among all these algorithms. Hence, the exploitation capability of the proposed IHAOHHO algorithm is competitive with all the selected meta-heuristic algorithms.
Multimodal test functions F8-F23 contain plentiful local optima whose number increases exponentially with the dimension size of the problem. These functions are very useful to evaluate the exploration ability and local optima avoidance of the algorithm. It can be seen from Table 5 that IHAOHHO outperforms other algorithms in most of the multimodal and fixed-dimension multimodal functions. For multimodal functions F8-F13, IHAOHHO shows completely superiority than other selected algorithms with the best average values and standard deviations. For ten fixed-dimensions multimodal functions F14-F23, IHAOHHO performs barely satisfactory. The IHAOHHO outperforms others in terms of both average values and standard deviations in F21-F23, and achieves the best accuracy for F16-F18. These results reveal that IHAOHHO can also provide superior exploration capability.
Search agents tend to change drastically to investigate promising regions of the search space in early iterations, and then exploit the region in detail and converge gradually as the number of iterations increases. Convergence curves of the IHAOHHO, AO, HHO, HOA, SSA, WOA, GWO, and MVO for 23 standard benchmark functions are given in Figure 6, which show the convergence rate of algorithms. As we can see, IHAOHHO shows competitive performance compared to other state-of-the-art algorithms. The IHAOHHO algorithm presents faster convergence speed than all other algorithms in F1-F4 and F8-F11. For else test functions, IHAOHHO may not have much advantages than other algorithms in terms of convergence speed with the reason that some algorithms are excellent as well, but the convergence accuracy of IHAOHHO is better than other algorithms in most of the test functions.
The superiority of IHAOHHO in terms of convergence speed is likely to come from the RH strategy in exploration phase. To be specific, the RH strategy provides better randomness and diversity for the search agents, making search agents explore the search space widely and randomly. The improvement of randomness and diversity increases the probability of finding the most promising region quickly. The advantage of convergence accuracy is likely to be derived from the OBL strategy, which improves randomness of search agents. The search agents can choose the better one to jump out of the local optima in each iteration. These two strategies help the hybrid algorithm outperforms the basic AO and HHO. Overall, IHAOHHO can efficiently achieve great solutions for all 23 standard benchmark functions.
Furthermore, Figure 7 shows us the results of several representative test functions on search history, trajectory, average fitness and convergence curve. From search history maps, we can see the search agents' distribution of the IHAOHHO while exploring and exploiting the search space. Because of the fast convergence, the vast majority of search agents are concentrated near the global optimum. Inspecting trajectory figures in Figure 7, the first search agent constantly oscillates in the first dimension of the search space, which suggests that the search agent investigates the most promising areas and better solutions widely. This powerful search capability is likely to come from the RH and OBL strategies. The average fitness presents if exploration and exploitation are conducive to improve the first random population and an accurate approximation of the global optimum can be found in the end. Similarly, it can be noticed that the average fitness oscillates like trajectories in the early iterations, and then decreases abruptly and levels off accordingly. The average fitness maps show the great improvement of the first random population and the acquisition of the final global optimal approximation as well. At last, the convergence curves reveal the best fitness values found by search agents after each of iteration. By observing this, the IHAOHHO shows very fast convergence speed.
The Wilcoxon signed-rank test is a non-parametric statistical test and useful to evaluate the statistical performance differences between the proposed IHAOHHO algorithm and other algorithms. As is well-known, p-values less than 0.05 indicate that there is a significant difference between the two compared algorithms. The calculated results of Wilcoxon signed-rank test between IHAOHHO and other seven algorithms for each benchmark functions are listed in Table 6. According to the criterion of 0.05, IHAOHHO outperforms all other algorithms in varying degrees. This superiority is statistically significant on unimodal functions F1-F6, which strongly indicates that IHAOHHO possesses high exploitation. IHAOHHO also shows better results on multimodal function F8-F23, which may suggest that IHAOHHO has a high capability of exploration. To sum up, the IHAOHHO algorithm can provide better results on almost all benchmark functions than other comparative algorithms.
Standard benchmark function experiments demonstrate the superior performance on simple problems of the proposed IHAOHHO algorithm. The complex functions can help to investigate the performance on intricate problems. One of the most challenging test function suites called CEC2017 [54] is selected to further test the performance of IHAOHHO, which contains 30 functions with more than half of the challenging hybrid and composition functions as shown in Table 7. The test results are compared to some well-known and latest algorithms proposed recently, in which IPOP-CMA-ES and LSHADE are the best behaved on CEC2017 in the literature. As the previous section described, each algorithm is ran 30 times with 500 iterations, and average and standard deviation results are presented in Table 8. From the comparison results, the proposed IHAOHHO obtains the 3rd rank following IPOP-CMA-ES and LSHADE, and exceeds SSC, RUN and HOA methods completely. It reveals that IHAOHHO can also achieve better results on complex functions.
Function | Name | Dim | Range | fmin |
Unimodal functions | ||||
F24 | Shifted and Rotated Bent Cigar Function | 10 | [-100, 100] | 100 |
F25 | Shifted and Rotated Zakharov Function | 10 | [-100, 100] | 300 |
Multimodal functions | ||||
F26 | Shifted and Rotated Rosenbrock's Function | 10 | [-100, 100] | 400 |
F27 | Shifted and Rotated Rastrigin's Function | 10 | [-100, 100] | 500 |
F28 | Shifted and Rotated Expanded Scaffer's F6 Function | 10 | [-100, 100] | 600 |
F29 | Shifted and Rotated Lunacek Bi-RastriginFunction | 10 | [-100, 100] | 700 |
F30 | Shifted and Rotated Non-Continuous Rastrigin's Function | 10 | [-100, 100] | 800 |
F31 | Shifted and Rotated Levy Function | 10 | [-100, 100] | 900 |
F32 | Shifted and Rotated Schwefel's Function | 10 | [-100, 100] | 1000 |
Hybrid functions (N is basic number of functions) | ||||
F33 | Hybrid Function 1 (N = 3) | 10 | [-100, 100] | 1100 |
F34 | Hybrid Function 2 (N = 3) | 10 | [-100, 100] | 1200 |
F35 | Hybrid Function 3 (N = 3) | 10 | [-100, 100] | 1300 |
F36 | Hybrid Function 4 (N = 4) | 10 | [-100, 100] | 1400 |
F37 | Hybrid Function 5 (N = 4) | 10 | [-100, 100] | 1500 |
F38 | Hybrid Function 6 (N = 4) | 10 | [-100, 100] | 1600 |
F39 | Hybrid Function 6 (N = 5) | 10 | [-100, 100] | 1700 |
F40 | Hybrid Function 6 (N = 5) | 10 | [-100, 100] | 1800 |
F41 | Hybrid Function 6 (N = 5) | 10 | [-100, 100] | 1900 |
F42 | Hybrid Function 6 (N = 6) | 10 | [-100, 100] | 2000 |
Composite functions (N is basic number of functions) | ||||
F43 | Composite Function 1 (N = 3) | 10 | [-100, 100] | 2100 |
F44 | Composite Function 2 (N = 3) | 10 | [-100, 100] | 2200 |
F45 | Composite Function 3 (N = 4) | 10 | [-100, 100] | 2300 |
F46 | Composite Function 4 (N = 4) | 10 | [-100, 100] | 2400 |
F47 | Composite Function 5 (N = 5) | 10 | [-100, 100] | 2500 |
F48 | Composite Function 6 (N = 5) | 10 | [-100, 100] | 2600 |
F49 | Composite Function 7 (N = 6) | 10 | [-100, 100] | 2700 |
F50 | Composite Function 8 (N = 6) | 10 | [-100, 100] | 2800 |
F51 | Composite Function 9 (N = 6) | 10 | [-100, 100] | 2900 |
F52 | Composite Function 10 (N = 3) | 10 | [-100, 100] | 3000 |
F | IHAOHHO | IPOP-CMAES | LSHADE | SSC | RUN | HOA | |
F24 | Avg | 1.77467E + 09 | 1.00000E + 02 | 1.00000E + 02 | 2.50699E + 09 | 3.75483E + 04 | 3.50163E + 08 |
Std | 1.11254E + 09 | 0.00000E + 00 | 0.00000E + 00 | 1.72594E + 09 | 1.40126E + 04 | 1.57335E + 08 | |
F25 | Avg | 4.69684E + 03 | 3.00000E + 02 | 3.00000E + 02 | 4.84926E + 03 | 5.05458E + 04 | 6.03840E + 03 |
Std | 1.99656E + 03 | 0.00000E + 00 | 0.00000E + 00 | 2.04210E + 03 | 8.29687E + 03 | 2.15957E + 03 | |
F26 | Avg | 4.15353E + 02 | 4.00091E + 02 | 4.00000E + 02 | 6.35108E + 02 | 5.13264E + 02 | 4.53729E + 02 |
Std | 1.69972E + 01 | 4.43520E-02 | 0.00000E + 00 | 1.98490E + 02 | 1.81438E + 01 | 3.02199E + 01 | |
F27 | Avg | 5.57466E + 02 | 5.19197E + 02 | 5.02340E + 02 | 5.60349E + 02 | 6.53772E + 02 | 5.74943E + 02 |
Std | 1.01328E + 01 | 8.41520E + 00 | 8.75000E-01 | 1.84807E + 01 | 2.91163E + 01 | 1.10416E + 01 | |
F28 | Avg | 6.29286E + 02 | 6.00000E + 02 | 6.00000E + 02 | 6.36104E + 02 | 6.40548E + 02 | 6.37022E + 02 |
Std | 7.67590E + 00 | 0.00000E + 00 | 2.59000E-07 | 1.01438E + 01 | 8.25192E + 00 | 8.80160E + 00 | |
F29 | Avg | 7.69414E + 02 | 7.32212E + 02 | 7.12230E + 02 | 8.13508E + 02 | 9.36961E + 02 | 7.79986E + 02 |
Std | 1.20032E + 01 | 3.93460E + 00 | 6.05000E-01 | 1.64856E + 01 | 5.73895E + 01 | 1.27443E + 01 | |
F30 | Avg | 8.40446E + 02 | 8.14912E + 02 | 8.02140E + 02 | 8.46992E + 02 | 9.21845E + 02 | 8.55157E + 02 |
Std | 7.39460E + 00 | 8.47500E + 00 | 1.03100E + 00 | 1.04671E + 01 | 2.62983E + 01 | 8.87940E + 00 | |
F31 | Avg | 1.36798E + 03 | 9.00000E + 02 | 9.00000E + 02 | 1.44797E + 03 | 3.52693E + 03 | 1.09708E + 03 |
Std | 2.12360E + 02 | 0.00000E + 00 | 0.00000E + 00 | 2.89598E + 02 | 8.96934E + 02 | 1.40757E + 02 | |
F32 | Avg | 2.20829E + 03 | 2.29077E + 03 | 1.07003E + 03 | 2.89454E + 03 | 5.14578E + 03 | 2.87917E + 03 |
Std | 1.90189E + 02 | 2.37652E + 02 | 5.65600E + 01 | 2.06134E + 02 | 7.73937E + 02 | 2.06107E + 02 | |
F33 | Avg | 1.20861E + 03 | 1.16709E + 03 | 1.10002E + 03 | 1.38357E + 03 | 1.26564E + 03 | 1.30871E + 03 |
Std | 2.31887E + 01 | 1.35981E + 02 | 1.20000E-01 | 1.02038E + 02 | 3.23837E + 01 | 9.44024E + 01 | |
F34 | Avg | 9.65212E + 06 | 1.61308E + 03 | 1.33295E + 03 | 2.29908E + 06 | 1.38367E + 07 | 1.75532E + 07 |
Std | 6.54481E + 06 | 1.73789E + 02 | 8.62600E + 01 | 6.06195E + 06 | 9.36578E + 06 | 1.17226E + 07 | |
F35 | Avg | 1.73810E + 04 | 1.35805E + 03 | 1.30374E + 03 | 4.09334E + 04 | 2.63421E + 04 | 1.34045E + 06 |
Std | 1.00028E + 04 | 6.27520E + 01 | 3.26000E + 00 | 2.07982E + 04 | 1.45826E + 04 | 1.11750E + 06 | |
F36 | Avg | 2.51467E + 03 | 1.47391E + 03 | 1.40019E + 03 | 6.63735E + 03 | 2.27458E + 05 | 2.80598E + 03 |
Std | 1.21526E + 03 | 4.18650E + 01 | 4.50000E-01 | 1.23909E + 03 | 1.87179E + 05 | 1.25523E + 03 | |
F37 | Avg | 8.52054E + 03 | 1.51384E + 03 | 1.50033E + 03 | 1.77014E + 04 | 1.42723E + 04 | 3.15987E + 04 |
Std | 3.16501E + 03 | 2.10632E + 01 | 2.00000E-01 E + 0 |
7.89396E + 03 | 3.59942E + 03 | 3.55718E + 04 | |
F38 | Avg | 1.90369E + 03 | 1.66968E + 03 | 1.60087E + 03 | 1.92836E + 03 | 2.84572E + 03 | 2.00480E + 03 |
Std | 1.00750E + 02 | 3.53711E + 01 | 3.60000E-01 | 1.12527E + 02 | 3.28867E + 02 | 1.58304E + 02 | |
F39 | Avg | 1.78843E + 03 | 1.77638E + 03 | 1.70137E + 03 | 1.79644E + 03 | 2.24703E + 03 | 1.90857E + 03 |
Std | 3.41834E + 01 | 1.23878E + 01 | 3.84000E + 00 | 1.87221E + 01 | 2.22813E + 02 | 8.69727E + 01 | |
F40 | Avg | 1.71900E + 04 | 1.90952E + 03 | 1.80359E + 03 | 1.27448E + 05 | 6.11908E + 05 | 3.62014E + 05 |
Std | 1.05037E + 04 | 2.39660E + 01 | 7.60000E + 00 | 1.40079E + 05 | 7.60372E + 05 | 2.40106E + 05 | |
F41 | Avg | 1.58292E + 05 | 1.91811E + 03 | 1.90026E + 03 | 2.81911E + 04 | 4.43675E + 05 | 1.66843E + 04 |
Std | 4.02208E + 05 | 2.12890E + 01 | 3.00000E-02 | 6.61395E + 03 | 3.45206E + 05 | 1.56893E + 04 | |
F42 | Avg | 2.17157E + 03 | 2.05983E + 03 | 2.00023E + 03 | 2.28443E + 03 | 2.56095E + 03 | 2.26788E + 03 |
Std | 6.49892E + 01 | 1.85766E + 01 | 4.30000E-01 | 6.52079E + 01 | 1.70413E + 02 | 8.48882E + 01 | |
F43 | Avg | 2.30614E + 03 | 2.31934E + 03 | 2.25542E + 03 | 2.32175E + 03 | 2.44601E + 03 | 2.35311E + 03 |
Std | 2.47564E + 01 | 8.46660E + 00 | 5.21600E + 01 | 6.07100E + 01 | 2.52926E + 01 | 4.32033E + 01 | |
F44 | Avg | 2.41213E + 03 | 2.73101E + 03 | 2.30010E + 03 | 3.59786E + 03 | 3.31843E + 03 | 2.35109E + 03 |
Std | 8.37419E + 01 | 6.26970E + 01 | 1.70000E-01 | 7.07466E + 02 | 1.86094E + 03 | 3.37269E + 01 | |
F45 | Avg | 2.62413E + 03 | 2.63000E + 03 | 2.60230E + 03 | 2.65854E + 03 | 2.80612E + 03 | 2.72046E + 03 |
Std | 2.12841E + 01 | 5.55150E + 00 | 1.42000E + 00 | 2.74840E + 01 | 2.95349E + 01 | 2.25245E + 01 | |
F46 | Avg | 2.78904E + 03 | 2.70961E + 03 | 2.68830E + 03 | 2.80479E + 03 | 2.98605E + 03 | 2.82014E + 03 |
Std | 2.75184E + 01 | 9.78340E + 00 | 9.16500E + 01 | 2.35894E + 01 | 4.61846E + 01 | 5.20187E + 01 | |
F47 | Avg | 2.93523E + 03 | 2.93212E + 03 | 2.92377E + 03 | 3.02323E + 03 | 2.93603E + 03 | 2.96740E + 03 |
Std | 1.96255E + 01 | 8.33760E + 00 | 2.13000E + 01 | 5.86012E + 01 | 2.67693E + 01 | 1.82177E + 01 | |
F48 | Avg | 3.28386E + 03 | 3.21722E + 03 | 2.90000E + 03 | 4.05668E + 03 | 4.50153E + 03 | 3.33849E + 03 |
Std | 2.31698E + 02 | 2.03330E + 02 | 0.00000E + 00 | 2.87720E + 02 | 1.27120E + 03 | 4.73594E + 02 | |
F49 | Avg | 3.11226E + 03 | 3.08917E + 03 | 3.08903E + 03 | 3.11634E + 03 | 3.31206E + 03 | 3.23484E + 03 |
Std | 1.80612E + 01 | 4.24482E + 01 | 1.05000E + 00 | 2.68443E + 01 | 3.57031E + 01 | 5.28018E + 01 | |
F50 | Avg | 3.21692E + 03 | 3.27673E + 03 | 3.15435E + 03 | 3.25502E + 03 | 3.28169E + 03 | 3.49994E + 03 |
Std | 1.07032E + 02 | 9.66220E + 00 | 1.10920E + 01 | 3.19693E + 01 | 2.06487E + 01 | 1.36289E + 03 | |
F51 | Avg | 3.35870E + 03 | 3.27842E + 03 | 3.13492E + 03 | 3.36830E + 03 | 4.24526E + 03 | 3.37358E + 03 |
Std | 6.51044E + 01 | 5.74741E + 01 | 3.87000E + 00 | 9.54409E + 01 | 2.74106E + 02 | 7.28448E + 01 | |
F52 | Avg | 3.29001E + 06 | 3.28462E + 04 | 3.41838E + 03 | 7.61412E + 06 | 3.99731E + 06 | 4.12253E + 06 |
Std | 2.57991E + 06 | 2.60735E + 04 | 2.28600E + 01 | 4.84549E + 06 | 2.71864E + 06 | 4.95066E + 06 |
The performance of an optimization algorithm is affected by the values of the control parameters. For the sake of better performance, the influence of the parameters should be investigated to select the appropriate values. The IHAOHHO algorithm owns three parameters σinitial, σfinal and Exponent in Eq (22). At the end of the iteration, the algorithm needs to search in detail and minimize randomness as much as possible. Thus, σfinal should be equal to 0 to get rid of the random term in Eq (21). Next, the left two parametersσinitial and Exponent are assessed by the representative standard and CEC2017 benchmark functions in Table 9. The mean-square error values are obtained using benchmark functions from different categories including unimodal, multimodal and fixed-multimodal of standard benchmark functions, and unimodal, multimodal, hybrid and composite of CEC2017 with different parameters. The best performance bolded is obtained by values 1 and 2 for parameters σinitial and Exponent.
F | σinitial = 1, E = 1 |
σinitial = 1, E = 1.5 |
σinitial = 1, E = 2 |
σinitial = 1.5, E = 1 |
σinitial = 1.5, E = 1.5 |
σinitial = 1.5, E = 2 |
σinitial = 2, E = 1 |
σinitial = 2, E = 1.5 |
σinitial = 2, E = 2 |
F5 | 2.62E - 06 | 3.89E - 06 | 3.80E - 08 | 1.90E - 06 | 2.44E - 06 | 5.84E - 08 | 4.24E - 06 | 8.99E - 07 | 3.79E - 06 |
F7 | 4.04E - 08 | 6.20E - 08 | 1.47E - 08 | 5.79E - 08 | 1.32E - 07 | 6.93E - 08 | 3.18E - 08 | 1.60E - 08 | 2.08E - 08 |
F9 | 0 | 0 | 0 | 0 | 0 | 0 | 0 | 0 | 0 |
F13 | 1.16E - 10 | 1.04E - 11 | 3.20E - 12 | 3.29E - 11 | 3.81E - 11 | 2.52E - 11 | 5.34E - 12 | 8.14E - 11 | 7.61E - 11 |
F14 | 5.4418 | 0.43653 | 0.10935 | 0.54588 | 0.87306 | 0.87306 | 0.54591 | 1.7461 | 5.1709 |
F18 | 1.18E - 09 | 3.02E - 09 | 7.59E - 11 | 8.23E - 11 | 25.1496 | 25.1379 | 1.96E - 08 | 2.73E - 06 | 25.1593 |
F25 | 6.50E + 07 | 6.63E + 07 | 6.55E + 07 | 5.50E + 07 | 4.99E + 07 | 4.84E + 07 | 6.22E + 07 | 6.56E + 07 | 7.25E + 07 |
F26 | 2.94E + 04 | 2.14E + 04 | 1.51E + 04 | 1.26E + 04 | 1.87E + 04 | 1.86E + 04 | 1.47E + 04 | 2.76E + 04 | 2.70E + 04 |
F30 | 2.43E + 035 | 1.73E + 03 | 1.78E + 03 | 2.18E + 03 | 2.12E + 03 | 2.10E + 03 | 1.74E + 03 | 2.04E + 03 | 2.13E + 03 |
F33 | 6.61E + 04 | 5.12E + 04 | 2.92E + 04 | 8.56E + 04 | 4.04E + 04 | 6.08E + 04 | 5.48E + 04 | 4.45E + 04 | 6.75E + 04 |
F39 | 2.43E + 04 | 1.94E + 04 | 1.73E + 04 | 1.54E + 04 | 1.68E + 04 | 1.26E + 04 | 1.21E + 04 | 1.46E + 04 | 1.22E + 04 |
F44 | 4.31E + 04 | 5.06E + 04 | 4.78E + 04 | 5.88E + 04 | 4.08E + 04 | 9.79E + 04 | 4.93E + 04 | 6.43E + 04 | 7.79E + 04 |
F49 | 1.91E + 05 | 1.95E + 05 | 1.85E + 05 | 1.93E + 05 | 1.87E + 05 | 2.01E + 05 | 1.90E + 05 | 1.95E + 05 | 1.88E + 05 |
Considering equality and inequality constraints is a necessary process for optimization because most optimization problems have constraints in the real world. In this subsection, three well-known constrained engineering design problems, which include speed reducer design problem, tension/compression spring design problem and three-bar truss design problem, are solved to further verify the performance of IHAOHHO. The results of IHAOHHO are compared to the basic AO, HHO, and HOA, SSA, WOA, GWO, MVO as well. The parameter settings are the same as the previous numerical experiments. For all tests, each algorithm is ran 15 times independently. The best result among 15 times for each algorithm and the Wilcoxon signed-rank test results between IHAOHHO and other algorithms are shown in Tables 10-12.
Algorithm | Optimum variables | Optimum weight | P-value | ||||||
x1 | x2 | x3 | x4 | x5 | x6 | x7 | |||
IHAOHHO | 3.49683 | 0.7 | 17 | 7.33302 | 7.8 | 3.35006 | 5.28575 | 2995.816 | NaN |
AO | 3.49688 | 0.7 | 17 | 8.10828 | 7.8 | 3.37081 | 5.28578 | 3008.168 | 0.025574 |
HHO | 3.49731 | 0.7 | 17 | 7.3 | 7.8 | 3.47527 | 5.28482 | 3028.6976 | 0.035339 |
HOA | 3.56008 | 0.7 | 17 | 7.34912 | 7.8 | 3.49325 | 5.28415 | 3058.577 | 6.1035e-05 |
SSA | 3.49732 | 0.7 | 17 | 8.03843 | 7.80061 | 3.52296 | 5.28577 | 3049.1538 | 0.012451 |
WOA | 3.4976 | 0.7 | 17 | 7.3 | 7.8 | 3.44134 | 5.28525 | 3019.3398 | 0.043721 |
MVO | 3.52164 | 0.7 | 17 | 7.44477 | 8.29729 | 3.43143 | 5.2842 | 3038.4984 | 0.018066 |
GWO | 3.49231 | 0.7 | 17.0038 | 8.1759 | 8.04815 | 3.35214 | 5.28783 | 3013.2315 | 0.0026245 |
Algorithm | Optimum variables | Optimum weight | P-value | ||
d | D | N | |||
IHAOHHO | 0.054826 | 0.49772 | 5.273 | 0.010881 | NaN |
AO | 0.051647 | 0.38603 | 9.3553 | 0.011692 | 6.1035e-05 |
HHO | 0.059559 | 0.64197 | 3.4141 | 0.012329 | 0.047913 |
HOA | 0.054031 | 0.47388 | 6.0876 | 0.011188 | 0.63867 |
SSA | 0.05 | 0.326589 | 12.8798 | 0.012149 | 0.00030518 |
WOA | 0.059166 | 0.62905 | 3.534 | 0.012186 | 0.047913 |
MVO | 0.059421 | 0.63742 | 3.4573 | 0.012282 | 0.025574 |
GWO | 0.057335 | 0.57116 | 4.1668 | 0.011579 | 6.1035e-05 |
Algorithm | Optimum variables | Optimum weight | P-value | |
x1 | x2 | |||
IHAOHHO | 0.79182 | 0.39856 | 263.8608 | NaN |
AO | 0.7932 | 0.39476 | 263.8689 | 0.04126 |
HHO | 0.7824 | 0.42541 | 263.8795 | 0.010254 |
HOA | 0.78192 | 0.4268 | 263.8841 | 0.030151 |
SSA | 0.78139 | 0.42834 | 263.8895 | 6.1035e-05 |
WOA | 0.77541 | 0.44621 | 263.9826 | 0.00012207 |
MVO | 0.78196 | 0.42668 | 263.8837 | 6.1035e-05 |
GWO | 0.79341 | 0.39418 | 263.8703 | 0.00018311 |
This problem aims to optimize seven variables to minimize the speed reducer's total weights, which include the face width (x1), module of teeth (x2), a discrete design variable on behalf of the teeth in the pinion (x3), length of the first shaft between bearings (x4), length of the second shaft between bearings (x5), diameters of the first shaft (x6) and diameters of the second shaft (x7). Four constraints: covering stress, bending stress of the gear teeth, stresses in the shafts and transverse deflections of the shafts as shown in Figure 8 should be satisfied. The mathematical formulation is represented as follows:
Minimize
f(→x)=0.7854x1x22(3.3333x23+14.9334x3−43.0934)−1.508x1(x26+x27)+7.4777(x36+x37), |
Subject to
g1(→x)=27x1x22x3−1⩽0,g2(→x)=397.5x1x22x23−1⩽0,g3(→x)=1.93x34x2x3x46−1⩽0,g4(→x)=1.93x35x2x3x47−1⩽0,g5(→x)=√(745x4x2x3)2+16.9×106110.0x36−1⩽0,g6(→x)=√(745x4x2x3)2+157.5×10685.0x36−1⩽0,g7(→x)=x2x340−1⩽0,g8(→x)=5x2x1−1⩽0,g9(→x)=x112x2−1⩽0,g10(→x)=1.5x6+1.9x4−1⩽0,g11(→x)=1.1x7+1.9x5−1⩽0, |
Variable range
2.6⩽x1⩽3.6,0.7⩽x2⩽0.8,17⩽x3⩽28,7.3⩽x4⩽8.3,7.8⩽x5⩽8.3,2.9⩽x6⩽3.9,5.0⩽x7⩽5.5, |
Compared to other algorithms, IHAOHHO can obviously achieve better results in the speed reducer design problem, as shown in Table 10. P-values in Table 10 show us the significant difference between IHAOHHO and other algorithms, proving the statistical superiority of the proposed algorithm.
In this case, the intention is to minimize the weight of the tension/compression spring shown in Figure 9. Constraints on surge frequency, shear stress and deflection must be satisfied during optimum design. There are three parameters need to be minimized, including the wire diameter(d), mean coil diameter(D) and the number of active coils (N). The mathematical form of this problem can be written as follows:
Consider
→x=[x1 x2 x3 x4]=[d D N],. |
Minimize
f(→x)=(x3+2)x2x21, |
Subject to
g1(→x)=1−x32x371785x41⩽0,g2(→x)=4x22−x1x212566(x2x31−x41)+15108x21⩽0,g3(→x)=1−140.45x1x22x3⩽0,g4(→x)=x1+x21.5−1⩽0, |
Variable range
0.05⩽x1⩽2.00,0.25⩽x2⩽1.30,2.00⩽x3⩽15.00, |
The experiment results are listed in Table 11 and show that the IHAOHHO can attain the best weight values compared to all other algorithms. IHAOHHO obtains the significant different results compared to others exclude HOA.
The three-bar truss design problem is a classical optimization application in civil engineering field. The main intention of this case is to minimize the weight of a truss with three bars by considering two structural parameters as illustrated in Figure 10. Deflection, stress and buckling are the three main constrains. The mathematical formulation of this problem is given:
Consider
→x=[x1 x2]=[A1 A2], |
Minimize
f(→x)=(2√2x1+x2)∗l, |
Subject to
g1(→x)=√2x1+x2√2x21+2x1x2P−σ⩽0,g2(→x)=x2√2x21+2x1x2P−σ⩽0,g3(→x)=1√2x2+x1P−σ⩽0, |
Consider
0⩽x1,x2⩽1, |
where
l=100cm,P=2KN/cm2,σ=2KN/cm2. |
Results of IHAOHHO for solving three-bar truss design problem are listed in Table 12. It can be observed that IHAOHHO outperforms other comparative optimization algorithms. Also, it is clear that the p-values between IHAOHHO and other methods are all smaller than 0.05.
As a summary, the experiments in this section prove the superiority of the proposed IHAOHHO algorithm in different characteristics and applications in the real world. IHAOHHO performs better than the basic AO and HHO, and selected well-known algorithms in various degrees, which demonstrates the exploration and exploitation capabilities improvement of IHAOHHO. Excellent performance in solving engineering design problems suggests that IHAOHHO can be widely used in real-world optimization problems.
In this paper, an improved hybrid Aquila Optimizer and Harris Hawks Optimization algorithm is proposed by combining the exploration part of AO with the exploitation part of HHO. The advantageous parts of basic AO and HHO are combined to keep the well-behaved exploration and exploitation capabilities. Two strategies including representative-based hunting and opposition-based learning are incorporated into the proposed IHAOHHO to further improve the optimization performance. The representative-based hunting strategy can effectively enhance the diversity of the population and fully explore the search space. The opposition-based learning strategy contributes to keep the algorithm from trapping in local optima. This algorithm is evaluated by standard benchmark functions and CEC2017 test functions to analyze its exploration, exploitation and local optima avoidance capabilities. The experiments show competitive results compared to other state-of-the-art meta-heuristic algorithms, which prove that IHAOHHO has better optimization performance than others. Three engineering design problems are solved as well to further verify the superiority of the algorithm, and the results are also competitive with other meta-heuristic algorithms.
The performance of the proposed algorithm on CEC2017 benchmark functions still needs to be improved. The exploration and exploitation capabilities need to be further investigated to break the limitations on CEC2017 test suit. And the transition from exploration to exploitation phase of IHAOHHO is simple. For further work, the transition mechanism can be improved to provide a better balance between the exploration and exploitation phases of this algorithm. Besides, the IHAOHHO algorithm can only solve single-objective optimization problems. Multi-objective version of IHAOHHO may be developed to solve multi-objective problems in the future.
This work was partially supported by Sanming University introduces high-level talents to start scientifc research funding support Project (20YG01, 20YG14), Guiding science and technology projects in Sanming City (2020-S-39, 2021-S-8), Educational research projects of young and middle-aged teachers in Fujian Province (JAT200638, JAT200618), Scientifc research and development fund of Sanming University (B202029, B202009), Collaborative education project of industry university cooperation of the Ministry of Education (202002064014), School level education and teaching reform project of Sanming University (J2010306, J2010305), Higher education research project of Sanming University (SHE2102, SHE2013).
On behalf of all authors, the corresponding author states that there is no conflict of interest.
[1] | R. L. Siegel, K. D. Miller and A. Jemal, Cancer statistics, 2019, CA Cancer J. Clin., 69 (2019), 7–34. |
[2] | L. S. Poulou, E. Botsa and I. Thanou, et al., Percutaneous microwave ablation vs radiofrequency ablation in the treatment of hepatocellular carcinoma, World J. Hepatol., 7 (2015), 1054–1063. |
[3] | K. A. Cronin, A. J. Lake and S. Scott, et al., Annual report to the nation on the status of cancer, part I: National cancer statistics, Cancer, 124 (2018), 2785–2800. |
[4] | J. Tejeda-Maldonado, I. García-Juárez and J. Aguirre-Valadez, et al., Diagnosis and treatment of hepatocellular carcinoma: An update, World J. Hepatol., 7 (2015), 362–376. |
[5] | N. Bhardwaj, A. D. Strickland and F. Ahmad, et al., A comparative histological evaluation of the ablations produced by microwave, cryotherapy and radiofrequency in the liver, Pathology, 41 (2009), 168–172. |
[6] | C. Schumann, Visualization and heuristic modeling for planning of minimally-and non-invasive tissue ablation, Ph.D.Dissertation, Jacobs University Bremen, Bremen, Germany, (2017). |
[7] | M. W. Lee, S. S. Raman and N. H. Asvadi, et al., Radiofrequency ablation of hepatocellular carcinoma as bridge therapy to liver transplantation: A 10-year intention-to-treat analysis, Hepatology, 65 (2017), 1979–1990. |
[8] | M. Ahmed, L. Solbiati and C. L. Brace, et al., Image-guided tumor ablation: standardization of terminology and reporting criteria-a 10-year update, Radiology, 273 (2014), 241–260. |
[9] | W. Wu, Z. Zhou and S. Wu, et al., Automatic liver segmentation on volumetric CT images using supervoxel-based graph cuts, Comput. Math. Methods Med., 2016 (2016), 9093721. |
[10] | W. Wu, S. Wu and Z Zhou, et al., 3D liver tumor segmentation in CT images using improved fuzzy C-means and graph cuts, BioMed. Res. Int., 2017 (2017), 5207685. |
[11] | R. Zhang, Z. Zhou and W. Wu, et al., An improved fuzzy connectedness method for automatic three-dimensional liver vessel segmentation in CT images, J. Healthc. Eng., 2018 (2018), 2376317. |
[12] | K. F. Chu and D. E. Dupuy, Thermal ablation of tumours: biological mechanisms and advances in therapy, Nat. Rev. Cancer, 14 (2014), 199–208. |
[13] | M. Mendiratta-Lala, O. R. Brook and B. D. Midkiff, et al., Quality initiatives: strategies for anticipating and reducing complications and treatment failures in hepatic radiofrequency ablation, Radiographics, 30 (2010), 1107–1122. |
[14] | A. Z. Fonseca, S. Santin and L. G. L. Gomes, et al., Complications of radiofrequency ablation of hepatic tumors: Frequency and risk factors, World J. Hepatol., 6 (2014), 107–113. |
[15] | S. N. Goldberg, C. J. Grassi and J. F. Cardella, et al., Image-guided tumor ablation: standardization of terminology and reporting criteria, Radiology, 235 (2005), 728–739. |
[16] | Schumann C, Rieder C and Preusser T, et al., State of the art in computer-assisted planning, intervention, and assessment of liver-tumor ablation, Crit. Rev. Biomed. Eng., 38 (2010), 31–52. |
[17] | C. Schumann, J. Bieberstein and C. Trumm, et al., Fast automatic path proposal computation for hepatic needle placement, Medical Imaging 2010: Visualization, Image-Guided Procedures, and Modeling, (2010), 76251J. |
[18] | C. Baegert, C. Villard and P. Schreck, et al., Multi-criteria trajectory planning for hepatic radiofrequency ablation, International Conference on Medical Image Computing and Computer-Assisted Intervention, (2007), 676–684. |
[19] | C. Baegert, C. Villard and P. Schreck, et al., Precise determination of regions of interest for hepatic RFA planning, Medical Imaging 2007: Visualization and Image-Guided Procedures, (2007), 650923. |
[20] | A. Seitel, M. Engel and C. M. Sommer, et al., Computer-assisted trajectory planning for percutaneous needle insertions, Med. Phys., 38 (2011), 3246–3259. |
[21] | J. Nocedal, and S. Wright, Numerical optimization, 2nd edition, New York: Springer, (2006), 29–32. |
[22] | E. Triantaphyllou, B. Shu and S. N. Sanchez, et al., Multi-criteria decision making: an operations research approach, Encyclopedia of Electrical and Electronics Engineering, 15 (1998), 175–186. |
[23] | A. L. Jaimes, S. Z. Martınez and C. A. C. Coello, An introduction to multiobjective optimization techniques, Optim. Polymer Processing, (2009), 29–57. |
[24] | R. T. Marler and J. S. Arora, Survey of multi-objective optimization methods for engineering, Struct. Multidiscipl. Optim., 26 (2004), 369–395. |
[25] | M. J. D. Powell, An efficient method for finding the minimum of a function of several variables without calculating derivatives, Comput. J., 7 (1964), 155–162. |
[26] | J. A. Nelder and R. Mead, A simplex method for function minimization, Comput. J., 7 (1965), 308–313. |
[27] | A. Khachaturyan, S. Semenovsovskaya and B. Vainshtein, The thermodynamic approach to the structure analysis of crystals, Acta Crystallogr. A, 37 (1981), 742–754. |
[28] | C. Villard, L. Soler and N. Papier, et al., RF-Sim: a treatment planning tool for radiofrequency ablation of hepatic tumors, Seventh International Conference on Information Visualization, (2003), 561–566. |
[29] | C. Villard, C. Baegert and P. Schreck, et al., Optimal trajectories computation within regions of interest for hepatic RFA planning, International Conference on Medical Image Computing and Computer-Assisted Intervention, (2005), 49–56. |
[30] | C. Baegert, C. Villard and P. Schreck, et al., Trajectory optimization for the planning of percutaneous radiofrequency ablation of hepatic tumors, Comput. Aided Surg., 12 (2007), 82–90. |
[31] | D. Craft, Multi-criteria optimization methods in radiation therapy planning: a review of technologies and directions, arXiv preprint arXiv:1305.1546, (2013). |
[32] | N. Hamzé, J. Voirin and P. Collet, et al., Pareto front vs. weighted sum for automatic trajectory planning of deep brain stimulation, International Conference on Medical Image Computing and Computer-Assisted Intervention, (2016), 534–541. |
[33] | W. Stadler, A survey of multicriteria optimization or the vector maximum problem, part I: 1776–1960, J. Optim. Theory Appl., 29 (1979), 1–52. |
[34] | O. Castillo, L. Trujillo and P. Melin, Multiple objective genetic algorithms for path-planning optimization in autonomous mobile robots, Soft Comput., 11 (2007), 269–279. |
[35] | K. Teichert, A hyperboxing Pareto approximation method applied to radiofrequency ablation treatment planning, Fraunhofer-Verlag, (2014). |
[36] | C. Schumann, J. Bieberstein and C. Trumm, et al., Fast automatic path proposal computation for hepatic needle placement, Medical Imaging 2010: Visualization, Image-Guided Procedures, and Modeling, (2010), 76251J. |
[37] | J. P. Snyder, Album of Map Projections, United States Geological Survey Professional Paper, United States Government Printing Office, (1989), 1453. |
[38] | J. P. Snyder, Map projections--A working manual, US Government Printing Office, (1987). |
[39] | C. Schumann, J. Bieberstein and S. Braunewell, et al., Visualization support for the planning of hepatic needle placement, Int. J. Comput. Assist. Radiol. Surg., 7 (2012), 191–197. |
[40] | N. Greene, Environment mapping and other applications of world projections, IEEE Comput. Graph. Appl., 6 (1986), 21–29. |
[41] | R. Khlebnikov, B. Kainz and J. Muehl, et al., Crepuscular rays for tumor accessibility planning, IEEE Trans. Vis. Comput. Gr., 17 (2011), 2163–2172. |
[42] | C. Schumann, C. Rieder and S. Haase, et al., Interactive access path exploration for planning of needle-based interventions, Roboter-Assistenten Werden Sensitiv., (2013), 103–106. |
[43] | B. Laugwitz, T. Held and M. Schrepp, Construction and evaluation of a user experience questionnaire, Symposium of the Austrian HCI and Usability Engineering Group, (2008), 63–76. |
[44] | C. Schumann, C. Rieder and S. Haase, et al., Interactive multi-criteria planning for radiofrequency ablation, Int. J. Comput. Assist. Radiol. Surg., 10 (2015), 879–889. |
[45] | A. Helck, C. Schumann and J. Aumann, et al., Automatic path proposal computation for CT-guided percutaneous liver biopsy, Int. J. Comput. Assist. Radiol. Surg., 11 (2016), 2199–2205. |
[46] | S. Liu, J. Liu and J. Xu, et al., Preoperative surgical planning for robot-assisted liver tumour ablation therapy based on collision-free reachable workspaces, Int. J. Robot. Autom., 32 (2017). |
[47] | L. Mundeleer, D. Wikler and T. Leloup, et al., Computer-assisted needle positioning for liver tumour radiofrequency ablation (RFA), Int. J. Med. Robot., 5 (2009), 458–464. |
[48] | C. Villard, L. Soler and A. Gangi, Radiofrequency ablation of hepatic tumors: simulation, planning, and contribution of virtual reality and haptics, Comput. Methods Biomech. Biomed. Eng., 8 (2005), 215–227. |
[49] | T. Livraghi, S. N. Goldberg and S. Lazzaroni, et al., Hepatocellular carcinoma: radio-frequency ablation of medium and large lesions, Radiology, 214 (2000), 761–768. |
[50] | L. Crocetti, T. De Baere and R. Lencioni, Quality improvement guidelines for radiofrequency ablation of liver tumours, Cardiovasc. Intervent. Radiol., 33 (2010), 11–17. |
[51] | S. K. Y. Chang, W. W. Hlaing and L. Yang, et al., Current technology in navigation and robotics for liver tumours ablation, Ann. Acad. Med. Singapore, 40 (2011), 231–236. |
[52] | H. Ren, K. Wu and X. Kang, Surgical navigation and planning with minimum radiation in orthopaedic interventions, Orthop. Proc., 95 (2013), 53. |
[53] | N. Toshikuni, Y. Takuma and T. Goto, et al., Prognostic factors in hepatitis C patients with a single small hepatocellular carcinoma after radiofrequency ablation, Hepatogastroenterology, 59 (2012), 2361–2366. |
[54] | T. Butz, S. K. Warfield and K. Tuncali, et al., Pre-and intra-operative planning and simulation of percutaneous tumor ablation, International Conference on Medical Image Computing and Computer-Assisted Intervention, (2000), 317–326. |
[55] | G. D. Dodd III, M. S. Frank and M. Aribandi, et al., Radiofrequency thermal ablation: computer analysis of the size of the thermal injury created by overlapping ablations, AJR Am. J. Roentgenol., 177 (2001), 777–782. |
[56] | Y. S. Khajanchee, D. Streeter and L. L. Swanstrom, et al., A mathematical model for preoperative planning of radiofrequency ablation of hepatic tumors, Surg. Endosc., 18 (2004), 696–701. |
[57] | M. H. Chen, W. Yang and K. Yan, et al., Large liver tumors: protocol for radiofrequency ablation and its clinical application in 110 patients-mathematic model, overlapping mode, and electrode placement process, Radiology, 232 (2004), 260–271. |
[58] | A. Meijster, J. B. T. M. Roerdink and W. H. Hesselink, A general algorithm for computing distance transforms in linear time, In: Goutsias J., Vincent L., Bloomberg D.S. (eds), Mathematical Morphology and Its Applications to Image and Signal Processing, Boston: Springer, (2002), 331–340. |
[59] | K. Trovato, S. Dalal and J. Krücker, et al., Automated RFA planning for complete coverage of large tumors, Medical Imaging 2009: Visualization, Image-Guided Procedures, and Modeling, (2009), 72610D. |
[60] | L. Yang, R. Wen and J. Qin, et al., A robotic system for overlapping radiofrequency ablation in large tumor treatment, IEEE/ASME Trans. Mech., 15 (2010), 887–897. |
[61] | B. Duan, R. Wen and C. B. Chng, et al., Image-guided robotic system for radiofrequency ablation of large liver tumor with single incision, 2015 12th International Conference on Ubiquitous Robots and Ambient Intelligence (URAI), (2015), 284–289. |
[62] | P. Liu, J. Qin and B. Duan, et al., Overlapping radiofrequency ablation planning and robot-assisted needle insertion for large liver tumors, Int. J. Med. Robot., 15 (2019), e1952. |
[63] | P. G. Bolhuis, D. Frenkel and S. C. Mau, et al., Entropy difference between crystal phases, Nature, 388 (1997), 235–236. |
[64] | S. X. Liu, S. Dalal and J. Kruecker, Automated microwave ablation therapy planning with single and multiple entry points, Medical Imaging 2012: Image-Guided Procedures, Robotic Interventions, and Modeling, (2012), 831635. |
[65] | R. H. Byrd, P. Lu and J. Nocedal, et al., A limited memory algorithm for bound constrained optimization, SIAM J. Sci. Comput., 16 (1995), 1190–1208. |
[66] | C. Zhu, R. H. Byrd and P. Lu, et al., Algorithm 778: L-BFGS-B: Fortran subroutines for large-scale bound-constrained optimization, ACM T. Math. Software, 23 (1997), 550–560. |
[67] | K. F. Wang, W. Pan and F. Wang, et al., Geometric optimization of a mathematical model of radiofrequency ablation in hepatic carcinoma, Asian Pac. J. Cancer Prev., 14 (2013), 6151–6158. |
[68] | H. Ren, E. Campos-Nanez and Z, Yaniv, et al., Treatment planning and image guidance for radiofrequency ablation of large tumors, IEEE J. Biomed. Health Inform., 18 (2014), 920–928. |
[69] | Z. Yaniv, P. Cheng and E. Wilson, et al., Needle-based interventions with the image-guided surgery toolkit (IGSTK): From phantoms to clinical trials, IEEE Trans. Biomed. Eng., 57 (2010), 922–933. |
[70] | H. Zhang, F. Banovac and S. Munuo, et al., Treatment planning and image guidance for radiofrequency ablation of liver tumors, Medical Imaging 2007: Visualization and Image-Guided Procedures, (2007), 650922. |
[71] | H. Ren, W. Guo and S. S. Ge, et al., Coverage planning in computer-assisted ablation based on genetic algorithm, Comput. Biol. Med., 49 (2014), 36–45. |
[72] | R. Chen, F. Lu and K. Wang, et al., Semiautomatic radiofrequency ablation planning based on constrained clustering process for hepatic tumors, IEEE Trans. Biomed. Eng., 65 (2018), 645–657. |
[73] | H. H. Pennes, Analysis of tissue and arterial blood temperatures in the resting human forearm, J. Appl. Physiol., 1 (1948), 93–122. |
[74] | C. Cattaneo, Surune forme de l'equation de la chaleur eliminant la paradoxe d'une propagation instantantee, Compt. Rendu., 247 (1958), 431–433. |
[75] | P. Vernotte, Les paradoxes de la theorie continue de l'equation de la chaleur, Compt. Rendu, 246 (1958), 3154–3155. |
[76] | J. A. L. Molina, M. J. Rivera and M. Trujillo, et al., Effect of the thermal wave in radiofrequency ablation modeling: an analytical study, Phys. Med. Biol., 53 (2008), 1447. |
[77] | P. Liang, B. Dong and X. Yu, et al., Computer-assisted dynamic simulation of microwave-induced thermal distribution in coagulation of liver cancer, IEEE Trans. Biomed. Eng., 48 (2001), 821–829. |
[78] | W. Zhai, J, Xu and Y, Zhao, et al., Preoperative surgery planning for percutaneous hepatic microwave ablation, International Conference on Medical Image Computing and Computer-Assisted Intervention, (2008), 569–577. |
[79] | Q. Nan, X. M. Guo and F. Zhai, The contrast of two kinds of microwave antenna SAR simulation, Appl. Mech. Mat., 553 (2014), 379–383. |
[80] | F. Hübner, R. Schreiner and C. Reimann, et al., Ex vivo validation of microwave thermal ablation simulation using different flow coefficients in the porcine liver, Med. Eng. Phys., 66 (2019), 56–64. |
[81] | J. Chiang, K. Hynes and C. L. Brace, Flow-dependent vascular heat transfer during microwave thermal ablation. 2012 Annual International Conference of the IEEE Engineering in Medicine and Biology Society, (2012), 5582–5585. |
[82] | U. Zurbuchen, C. Holmer and K. S. Lehmann, et al., Determination of the temperature-dependent electric conductivity of liver tissue ex vivo and in vivo: Importance for therapy planning for the radiofrequency ablation of liver tumours, Int. J. Hyperthermia, 26 (2010), 26–33. |
[83] | J. A. López-Molina, M. J. Rivera and M. Trujillo, et al., Assessment of hyperbolic heat transfer equation in theoretical modeling for radiofrequency heating techniques, Open Biomed. Eng. J., 2 (2008), 22–27. |
[84] | M. Zhang, Z. Zhou and S. Wu, et al., Simulation of temperature field for temperature-controlled radio frequency ablation using a hyperbolic bioheat equation and temperature-varied voltage calibration: a liver-mimicking phantom study, Phys. Med. Biol., 60 (2015), 9455–9471. |
[85] | R. Chen, F. Lu and F. Wu, et al., An analytical solution for temperature distributions in hepatic radiofrequency ablation incorporating the heat-sink effect of large vessels, Phys. Med. Biol., 63 (2018), 235026. |
[86] | E. H. Ooi, K. W. Lee and S. Yap, et al., The effects of electrical and thermal boundary condition on the simulation of radiofrequency ablation of liver cancer for tumours located near to the liver boundary, Comput. Biol. Med., 106 (2019), 12–23. |
[87] | C. Rieder, T. Kroeger and C. Schumann, et al., GPU-based real-time approximation of the ablation zone for radiofrequency ablation, IEEE Trans. Vis. Comput. Gr., 17 (2011), 1812–1821. |
[88] | C. Schumann, C. Rieder and J. Bieberstein, et al., State of the art in computer-assisted planning, intervention, and assessment of liver-tumor ablation, Crit. Rev. Biomed. Eng., 38 (2010), 31–52. |
[89] | P. Mariappan, P. Weir and R. Flanagan, et al., GPU-based RFA simulation for minimally invasive cancer treatment of liver tumours, Int. J. Comput. Assist. Radiol. Surg., 12 (2017), 59–68. |
[90] | D. R. Di, Z. Z. He and Z. Q. Sun, et al., A new nano-cryosurgical modality for tumor treatment using biodegradable MgO nanoparticles, Nanomedicine., 8 (2012), 1233–1241. |
[91] | Q. Wang, Z. S. Deng and J, Liu, Theoretical evaluations of magnetic nanoparticle-enhanced heating on tumor embedded with large blood vessels during hyperthermia, J. Nanopart. Res., 14 (2012), 974–984. |
[92] | Z. Q. Sun, Y. Yang and J. Liu, In vivo experiments and numerical investigations on nanocryosurgical freezing of target tissues with large blood vessels, J. Biomed. Nanotechnol., 8 (2012), 10–18. |
[93] | N. Hamzé, I. Peterlík and S. Cotin, et al., Preoperative trajectory planning for percutaneous procedures in deformable environments, Comput. Med. Imaging Graph., 47 (2016), 16–28. |
[94] | X. Tan, P. Yu and K. B. Lim, et al., Robust needle trajectory planning for flexible needle insertion using Markov decision processes, Int. J. Comput. Assist. Radiol. Surg., 13 (2018), 1439–1451. |
[95] | P. Li, Z. Yang and S. Jiang, Needle-tissue interactive mechanism and steering control in image-guided robot-assisted minimally invasive surgery: a review, Med. Biol. Eng. Comput., 56 (2018), 931–949. |
[96] | H. M. Luu, W. Niessen and T. V. Walsum, et al., An automatic registration method for pre-and post-interventional CT images for assessing treatment success in liver RFA treatment, Med. Phys., 42 (2015), 5559–5567. |
[97] | W. H. Greene, S. Chelikani and K. Purushothaman, et al., Constrained non-rigid registration for use in image-guided adaptive radiotherapy, Med. Image Anal., 13 (2009), 809–817. |
[98] | J. Zhang, M. Ding and F. Meng, et al., Respiratory motion correction in free-breathing ultrasound image sequence for quantification of hepatic perfusion, Med. Phys., 38 (2011), 4737–4748. |
[99] | T. P. O'shea, J. C. Bamber and E. J. Harris, Temporal regularization of ultrasound-based liver motion estimation for image-guided radiation therapy, Med Phys, 43 (2016), 455–464. |
[100] | Y. H. Noorda, L. W. Bartels and M. A. Viergever, et al., Subject-specific four-dimensional liver motion modeling based on registration of dynamic MRI, J. Med. Imaging, 3 (2016), 015002. |
[101] | A. Mastmeyer, M. Wilms and H. Handels, Population-based respiratory 4D motion atlas construction and its application for VR simulations of liver punctures, Medical Imaging 2018: Image Processing, (2018), 1057417. |
[102] | N. Mohammed, S. Currie and M. Glegg, et al., Analysis of heart substructures motion and changes using 4DCT dataset and heart atlas in lung cancer patients, Int. J. Radiat. Oncol. Biol. Phys., 102 (2018), e557. |
[103] | M. Abayazid, T. Kato and S. G. Silverman, et al., Using needle orientation sensing as surrogate signal for respiratory motion estimation in percutaneous interventions, Int. J. Comput. Assist. Radiol. Surg., 13 (2018), 125–133. |
[104] | J. Borgert, S. Krüger and H. Timinger, et al., Respiratory motion compensation with tracked internal and external sensors during CT-guided procedures, Comput. Aided Surg., 11 (2006), 119–125. |
[105] | P. Lei, F. Moeslein and B. J. Wood, et al., Real-time tracking of liver motion and deformation using a flexible needle, Int. J. Comput. Assist. Radiol. Surg., 6 (2011), 435–446. |
[106] | C. S. Park, S. K. Hall and C. Liu, et al., A model of tissue contraction during thermal ablation. Physiol. Meas., 37 (2016), 1474–1484. |
[107] | D. Liu and C. L Brace, Numerical simulation of microwave ablation incorporating tissue contraction based on thermal dose, Phys. Med. Biol., 62 (2017), 2070–2086. |
[108] | V. Lopresto, L. Strigari and L. Farina, et al., CT-based investigation of the contraction of ex vivo tissue undergoing microwave thermal ablation, Phys. Med. Biol., 63 (2018), 055019. |
[109] | C. S. Park, C. Liu and S. K. Hall, et al., A thermoelastic deformation model of tissue contraction during thermal ablation, Int. J. Hyperthermia, 34 (2018), 221–228. |
[110] | L. Farina, Y. Nissenbaum and M. Cavagnaro, et al., Tissue shrinkage in microwave thermal ablation: comparison of three commercial devices, Int. J. Hyperthermia, 34 (2018), 382–391. |
1. | Virginia Giorno, Amelia G. Nobile, Some time-inhomogeneous diffusion models for population growth in random environments, 2024, 10075704, 108502, 10.1016/j.cnsns.2024.108502 |
Algorithm 1 Pseudo-code of IHAOHHO |
1: Set the initial values of the population size N and the maximum number of iterations T |
2: Initialize positions of the population X |
3: While t < T |
4: Update x, y, cd |
5: For i = 1 to N |
6: If t < T/2 % Exploration part of AO |
7: Archiving |
8: If rand < 0.5 |
9: Update the position of Xnewi and X_Ri using Eqs (1) and (21), respectively |
10: Calculate the fitness of Xnewi and X_Ri |
11: Update the position of Xi |
12: Else |
13: Update the position of Xnewi and X_Ri using Eqs (3) and (21), respectively |
14: Calculate the fitness of Xnewi and X_Ri |
15: Update the position of Xi |
16: End if |
17: Else % Exploitation part of HHO |
18: If r ≥ 0.5 and |E| ≥ 0.5 |
19: Update the position of Xi using Eq (11) |
20: End if |
21: If r ≥ 0.5 and |E| < 0.5 |
22: Update the position of Xi using Eq (14) |
23: End if |
24: If r < 0.5 and |E| ≥ 0.5 |
25: Update the position of Xi using Eq (15) |
26: End if |
27: If r < 0.5 and |E| < 0.5 |
28: Update the position of Xi using Eq (18) |
29: End if |
30: Update the position of Xnewi using Eq (24) % Opposition-based learning (OBL) |
31: If f(Xnewi) < f(Xi) |
32: Xi = Xnewi |
33: End if |
34: End if |
35: t = t + 1 |
36: End for |
37: For i = 1 to N |
38: Check if the position goes out of the search space boundary and bring it back. |
39: Calculate the fitness of Xi |
40: Update Xbest |
41: End for |
42: End while |
43: Return Xbest |
Function | Dim | Range | fmin |
F1(x)=∑ni=1x2i | 30 | [-100, 100] | 0 |
F2(x)=∑ni=1|xi|+∏ni=1|xi| | 30 | [-10, 10] | 0 |
F3(x)=∑ni=1(∑ij−1xj)2 | 30 | [-100, 100] | 0 |
F4(x)=maxi{|xi|,1⩽i⩽n} | 30 | [-100, 100] | 0 |
F5(x)=∑n−1i=1[100(xi+1−x2i)2+(xi−1)2] | 30 | [-30, 30] | 0 |
F6(x)=∑ni=1(xi+5)2 | 30 | [-100, 100] | 0 |
F7(x)=∑ni=1ix4i+random[0,1) | 30 | [-1.28, 1.28] | 0 |
Function | Dim | Range | fmin |
F8(x)=∑ni=1−xisin(√|xi|) | 30 | [-500, 500] | -418.9829 × 30 |
F9(x)=∑ni=1[x2i−10cos(2πxi)+10] | 30 | [-5.12, 5.12] | 0 |
F10(x)=−20exp(−0.2√1n∑ni=1x2i)−exp(1n∑ni=1cos(2πxi))+20+e | 30 | [-32, 32] | 0 |
F11(x)=14000∑ni=1x2i−∏ni=1cos(xi√i)+1 | 30 | [-600, 600] | 0 |
F12(x)=πn{10sin(πy1)+∑n−1i=1(yi−1)2[1+10sin2(πyi+1)]+(yn}+∑ni=1u(xi,10,100,4), where yi=1+xi+14,u(xi,a,k,m)={k(xi−a)mxi>a0−a<xi<ak(−xi−a)mxi<−a | 30 | [-50, 50] | 0 |
F13(x)=0.1(sin2(3πx1)+∑ni=1(xi−1)2[1+sin2(3πxi+1)]+(xn−1)2[1+sin2(2πxn)])+∑ni=1u(xi,5,100,4) | 30 | [-50, 50] | 0 |
Function | Dim | Range | fmin |
F14(x)=(1500+∑25j=11j+∑2i=1(xi−aij)6)−1 | 2 | [-65, 65] | 1 |
F15(x)=∑11i=1[ai−x1(b2i+bix2)b2i+bix3+x4]2 | 4 | [-5, 5] | 0.00030 |
F16(x)=4x21−2.1x41+13x61+x1x2−4x22+x42 | 2 | [-5, 5] | -1.0316 |
F17(x)=(x2−5.14π2x21+5πx1−6)2+10(1−18π)cosx1+10 | 2 | [-5, 5] | 0.398 |
F18(x)=[1+(x1+x2+1)2(19−14x1+3x21−14x2+6x1x2+3x22)]×[30+(2x1−3x2)2×(18−32x2+12x21+48x2−36x1x2+27x22)] | 2 | [-2, 2] | 3 |
F19(x)=−∑4i=1ciexp(−∑3j=1aij(xj−pij)2) | 3 | [-1, 2] | -3.86 |
F20(x)=−∑4i=1ciexp(−∑6j=1aij(xj−pij)2) | 6 | [0, 1] | -3.32 |
F21(x)=−∑5i=1[(X−ai)(X−ai)T+ci]−1 | 4 | [0, 10] | -10.1532 |
F22(x)=−∑7i=1[(X−ai)(X−ai)T+ci]−1 | 4 | [0, 10] | -10.4028 |
F23(x)=−∑10i=1[(X−ai)(X−ai)T+ci]−1 | 4 | [0, 10] | -10.5363 |
Algorithm | Parameters |
IHAOHHO | σinitial = 1; σfinal = 0; Exponent = 2 |
AO | U = 0.00565; r1 = 10; ω = 0.005; α = 0.1; δ = 0.1; G1∈[-1, 1]; G2 = [2,0] |
HHO | q∈[0, 1]; r∈[0, 1]; E0∈[-1, 1]; E1 = [2,0]; E∈ [-2, 2]; |
HOA | hβ = 0.9; hγ = 0.5; sβ = 0.2; sγ = 0.1; iγ = 0.3; dα = 0.5; dβ = 0.2; dγ = 0.1; rδ = 0.1; rγ = 0.05 |
SSA | c1 = [1,0]; c2∈[0, 1]; c3∈[0, 1] |
WOA | a1 = [2,0]; a2 = [-1, -2]; b = 1 |
GWO | a = [2,0] |
MVO | WEP ∈ [0.2, 1]; TDR ∈ [0, 1]; r1, r2, r3 ∈ [0, 1] |
F | IHAOHHO | AO | HHO | HOA | SSA | WOA | MVO | GWO | |
F1 | Avg | 3.3660E-253 | 7.9345E-97 | 3.3401E-96 | 1.7886E-130 | 2.1594E-07 | 1.8120E-73 | 1.2681 | 1.0585E-27 |
Std | 0 | 4.3457E-96 | 1.7664E-95 | 8.6475E-130 | 3.8328E-07 | 8.0771E-73 | 3.8945E-01 | 9.7097E-28 | |
F2 | Avg | 1.5599E-127 | 1.7619E-60 | 1.9990E-49 | 2.1595E-66 | 1.7056 | 8.8551E-51 | 3.7930 | 7.8893E-17 |
Std | 8.5347E-127 | 9.6504E-60 | 9.5039E-49 | 1.1828E-65 | 1.3736 | 3.2476E-50 | 1.6432E+01 | 5.7891E-17 | |
F3 | Avg | 2.7431E-199 | 2.2314E-104 | 8.4798E-72 | 1.7856E+02 | 1.3173E+03 | 4.4381E+04 | 2.2448E+02 | 2.6928E-05 |
Std | 0 | 1.2222E-103 | 4.4387E-71 | 5.2479E+02 | 1.1198E+03 | 1.1940E+04 | 1.2075E+03 | 6.0686E-05 | |
F4 | Avg | 2.2207E-129 | 1.6827E-69 | 1.1057E-49 | 4.5440E-65 | 1.2612E+01 | 5.6993E+01 | 2.1139 | 8.0152E-07 |
Std | 1.1068E-128 | 7.6613E-69 | 3.0441E-49 | 2.4668E-64 | 3.8228 | 2.5529E+01 | 1.0284 | 8.3055E-07 | |
F5 | Avg | 5.3852E-04 | 5.4421E-03 | 2.1438E-02 | 2.8964E+01 | 2.2071E+02 | 2.7871E+01 | 3.8794E+02 | 2.7221E+01 |
Std | 2.2657E-03 | 1.2008E-02 | 2.4291E-02 | 3.5795E-02 | 3.4556E+02 | 4.6073E-01 | 7.0618E-02 | 7.9162E-01 | |
F6 | Avg | 3.5863E-06 | 1.2028E-04 | 1.4716E-04 | 6.5645 | 2.5056E-07 | 3.4988E-01 | 1.1414 | 7.2412E-01 |
Std | 7.7280E-06 | 1.7490E-04 | 2.0847E-04 | 3.4100E-01 | 3.8211E-07 | 1.7102E-01 | 4.4812E-01 | 3.9184E-01 | |
F7 | Avg | 9.5324E-05 | 1.0504E-04 | 1.3211E-04 | 6.7657E-02 | 1.6706E-01 | 2.7105E-03 | 3.4554E-02 | 2.1436E-03 |
Std | 7.6717E-05 | 8.6163E-05 | 1.4567E-04 | 4.0688E-02 | 7.8018E-02 | 3.2053E-03 | 1.5231E-02 | 1.3151E-03 | |
F8 | Avg | -12569.3858 | -8010.0774 | -12569.0257 | -4106.2204 | -7362.4999 | -10632.1912 | -7648.8103 | -6101.7628 |
Std | 1.8228E-01 | 4.0684E+03 | 7.5912E-01 | 7.7530E+02 | 8.6690E+02 | 0 | 5.2123E+02 | 8.9531E+02 | |
F9 | Avg | 0 | 0 | 0 | 8.0284E+01 | 5.3197E+01 | 0 | 1.2456E+02 | 3.3205 |
Std | 0 | 0 | 0 | 1.0686E+02 | 2.0211E+01 | 4.6777E-15 | 3.4040E+01 | 4.302 | |
F10 | Avg | 8.8818E-16 | 8.8818E-16 | 8.8818E-16 | 5.7436E-15 | 2.6880 | 2.7886E-15 | 1.7605 | 9.8230E-14 |
Std | 0 | 0 | 0 | 1.7413E-15 | 6.5906E-01 | 2.421E-15 | 6.6839E-01 | 1.7092E-14 | |
F11 | Avg | 0 | 0 | 0 | 2.0978E-01 | 2.3006E-02 | 1.1525E-02 | 8.6051E-01 | 5.3578E-03 |
Std | 0 | 0 | 0 | 3.7796E-01 | 1.5437E-02 | 4.4029E-02 | 8.3088E-02 | 7.3633E-03 | |
F12 | Avg | 2.6974E-07 | 2.8068E-06 | 1.9506E-05 | 1.1656 | 7.8473 | 2.1363E-02 | 1.8746 | 5.7227E-02 |
Std | 4.4163E-07 | 5.7142E-06 | 3.8352E-05 | 2.1289E-01 | 2.7926 | 1.2107E-02 | 1.1276 | 9.6891E-02 | |
F13 | Avg | 3.0227E-06 | 4.9439E-05 | 9.7772E-05 | 3.0571 | 1.8860E+01 | 5.3918E-01 | 1.7158E-01 | 6.2217E-01 |
Std | 5.0785E-06 | 9.2988E-05 | 9.5928E-05 | 1.8645E-01 | 1.6484E+01 | 2.9800E-01 | 1.0772E-01 | 2.5487E-01 | |
F14 | Avg | 1.5932 | 2.0487 | 1.2629 | 2.9322 | 1.3943 | 2.8615 | 0.998 | 4.6223 |
Std | 9.2477E-01 | 2.1859 | 5.1727E-01 | 2.1542 | 9.5834E-01 | 3.3284 | 2.7158E-11 | 4.1450 | |
F15 | Avg | 4.4227E-04 | 4.9359E-04 | 3.9582E-04 | 7.1269E-03 | 2.2249E-03 | 7.5110E-04 | 7.8593E-03 | 5.7748E-03 |
Std | 3.4587E-04 | 1.1786E-04 | 2.3573E-04 | 7.5345E-03 | 4.9361E-03 | 4.9981E-04 | 1.3437E-02 | 8.9518E-03 | |
F16 | Avg | -1.0316 | -1.0312 | -1.0316 | -0.99752 | -1.0316 | -1.0316 | -1.0316 | -1.0316 |
Std | 3.1088E-08 | 4.0636E-04 | 7.1130E-09 | 3.1957E-02 | 1.8547E-14 | 7.6118E-10 | 4.5176E-07 | 3.6867E-08 | |
F17 | Avg | 3.9789E-01 | 3.9813E-01 | 3.9789E-01 | 3.9790E-01 | 3.9789E-01 | 3.9790E-01 | 3.9789E-01 | 3.9789E-01 |
Std | 4.0428E-05 | 3.0290E-04 | 4.5913E-05 | 1.1204E-03 | 9.2471E-15 | 1.5755E-05 | 7.7424E-08 | 7.6565E-07 | |
F18 | Avg | 3 | 3.0243 | 3 | 7.7479 | 3 | 3.0001 | 3 | 3 |
Std | 3.3248E-06 | 5.5712E-02 | 6.1620E-07 | 1.6153E+01 | 1.9031E-13 | 3.1781E-04 | 2.5080E-06 | 4.7166E-05 | |
F19 | Avg | -3.8258 | -3.8535 | -3.8597 | -3.8619 | -3.8628 | -3.8551 | -3.8628 | -3.8598 |
Std | 7.1972E-02 | 8.7650E-03 | 3.8900E-03 | 6.2715E-04 | 1.9037E-12 | 8.6573E-03 | 3.7043E-06 | 3.9554E-03 | |
F20 | Avg | -3.0792 | -3.1678 | -3.0813 | -3.2580 | -3.2335 | -3.2080 | -3.2448 | -3.2721 |
Std | 1.1913E-01 | 7.3334E-02 | 9.9164E-02 | 7.5769E-02 | 6.4415E-02 | 1.1882E-01 | 5.9771E-02 | 7.6156E-02 | |
F21 | Avg | -10.1525 | -10.1422 | -5.2145 | -9.3937 | -6.6406 | -9.2821 | -6.9627 | -9.6452 |
Std | 1.4463E-03 | 1.8290E-02 | 8.8739E-01 | 1.2967 | 3.4554 | 1.9234 | 3.1618 | 1.5462 | |
F22 | Avg | -10.4026 | -10.3914 | -5.0820 | -9.5706 | -9.7954 | -7.6313 | -8.6587 | -10.4009 |
Std | 6.6551E-04 | 2.1551E-02 | 8.7380E-03 | 1.5379 | 1.8875 | 3.0683 | 2.7631 | 1.2358E-03 | |
F23 | Avg | -10.5359 | -10.5292 | -5.1234 | -9.8166 | -8.7191 | -7.0303 | -7.5296 | -10.5348 |
Std | 6.6691E-04 | 1.2664E-02 | 52035E-03 | 1.0080 | 3.1206 | 3.4442 | 3.5941 | 8.4873E-04 |
F | IHAOHHO vs AO | IHAOHHO vs HHO | IHAOHHO vs HOA | IHAOHHO vs SSA | IHAOHHO vs WOA | IHAOHHO vs MVO | IHAOHHO vs GWO |
F1 | 6.1035E-05 | 6.1035E-05 | 6.1035E-05 | 6.1035E-05 | 6.1035E-05 | 6.1035E-05 | 6.1035E-05 |
F2 | 6.1035E-05 | 6.1035E-05 | 6.1035E-05 | 6.1035E-05 | 6.1035E-05 | 6.1035E-05 | 6.1035E-05 |
F3 | 6.1035E-05 | 6.1035E-05 | 6.1035E-05 | 6.1035E-05 | 6.1035E-05 | 6.1035E-05 | 6.1035E-05 |
F4 | 6.1035E-05 | 6.1035E-05 | 6.1035E-05 | 6.1035E-05 | 6.1035E-05 | 6.1035E-05 | 6.1035E-05 |
F5 | 0.10699 | 1.2207E-04 | 6.1035E-05 | 6.1035E-05 | 6.1035E-05 | 6.1035E-05 | 6.1035E-05 |
F6 | 1.8311E-04 | 1.8311E-04 | 6.1035E-05 | 1.1597E-03 | 6.1035E-05 | 6.1035E-05 | 6.1035E-05 |
F7 | 6.3721E-02 | 0.10699 | 6.1035E-05 | 6.1035E-05 | 6.1035E-05 | 6.1035E-05 | 6.1035E-05 |
F8 | 6.1035E-05 | 0.63867 | 6.1035E-05 | 6.1035E-05 | 6.1035E-05 | 6.1035E-05 | 6.1035E-05 |
F9 | NaN | NaN | 6.1035E-05 | 6.1035E-05 | NaN | 6.1035E-05 | 6.1035E-05 |
F10 | NaN | NaN | 6.1035E-05 | 6.1035E-05 | 1.9531E-03 | 6.1035E-05 | 6.1035E-05 |
F11 | NaN | NaN | 0.1250 | 6.1035E-05 | NaN | 6.1035E-05 | NaN |
F12 | 2.6245E-03 | 1.2207E-04 | 6.1035E-05 | 6.1035E-05 | 6.1035E-05 | 6.1035E-05 | 6.1035E-05 |
F13 | 2.1545E-02 | 1.2207E-04 | 6.1035E-05 | 6.1035E-05 | 6.1035E-05 | 6.1035E-05 | 6.1035E-05 |
F14 | 0.97797 | 0.45428 | 1.8066E-02 | 2.6245E-03 | 0.18762 | 6.1035E-05 | 3.5339E-02 |
F15 | 6.1035E-05 | 0.45428 | 6.1035E-05 | 6.1035E-05 | 6.1035E-05 | 6.1035E-05 | 0.3028 |
F16 | 6.1035E-05 | 8.3618E-03 | 6.1035E-05 | 6.1035E-05 | 9.4604E-02 | 6.1035E-05 | 5.5359E-02 |
F17 | 6.1035E-04 | 0.45428 | 6.1035E-05 | 6.1035E-05 | 0.27686 | 3.0518E-04 | 1.0254E-02 |
F18 | 8.3252E-02 | 1.2207E-04 | 8.3252E-02 | 6.1035E-05 | 0.48871 | 0.67877 | 0.13538 |
F19 | 7.2998E-02 | 1.8066E-02 | 7.2998E-02 | 6.1035E-05 | 1.8066E-02 | 6.1035E-05 | 3.0151E-02 |
F20 | 6.1035E-04 | 0.93408 | 8.5449E-04 | 1.5259E-03 | 2.0142E-03 | 6.1035E-05 | 8.5449E-04 |
F21 | 3.3569E-03 | 6.1035E-05 | 6.1035E-05 | 4.2120E-02 | 1.2207E-04 | 2.0142E-03 | 1.8311E-04 |
F22 | 1.2207E-04 | 6.1035E-05 | 6.1035E-05 | 8.3252E-02 | 1.8311E-04 | 4.126E-02 | 8.3618E-03 |
F23 | 1.1597E-03 | 6.1035E-05 | 6.1035E-05 | 3.3026E-02 | 6.1035E-05 | 1.6882E-02 | 6.7139E-03 |
Function | Name | Dim | Range | fmin |
Unimodal functions | ||||
F24 | Shifted and Rotated Bent Cigar Function | 10 | [-100, 100] | 100 |
F25 | Shifted and Rotated Zakharov Function | 10 | [-100, 100] | 300 |
Multimodal functions | ||||
F26 | Shifted and Rotated Rosenbrock's Function | 10 | [-100, 100] | 400 |
F27 | Shifted and Rotated Rastrigin's Function | 10 | [-100, 100] | 500 |
F28 | Shifted and Rotated Expanded Scaffer's F6 Function | 10 | [-100, 100] | 600 |
F29 | Shifted and Rotated Lunacek Bi-RastriginFunction | 10 | [-100, 100] | 700 |
F30 | Shifted and Rotated Non-Continuous Rastrigin's Function | 10 | [-100, 100] | 800 |
F31 | Shifted and Rotated Levy Function | 10 | [-100, 100] | 900 |
F32 | Shifted and Rotated Schwefel's Function | 10 | [-100, 100] | 1000 |
Hybrid functions (N is basic number of functions) | ||||
F33 | Hybrid Function 1 (N = 3) | 10 | [-100, 100] | 1100 |
F34 | Hybrid Function 2 (N = 3) | 10 | [-100, 100] | 1200 |
F35 | Hybrid Function 3 (N = 3) | 10 | [-100, 100] | 1300 |
F36 | Hybrid Function 4 (N = 4) | 10 | [-100, 100] | 1400 |
F37 | Hybrid Function 5 (N = 4) | 10 | [-100, 100] | 1500 |
F38 | Hybrid Function 6 (N = 4) | 10 | [-100, 100] | 1600 |
F39 | Hybrid Function 6 (N = 5) | 10 | [-100, 100] | 1700 |
F40 | Hybrid Function 6 (N = 5) | 10 | [-100, 100] | 1800 |
F41 | Hybrid Function 6 (N = 5) | 10 | [-100, 100] | 1900 |
F42 | Hybrid Function 6 (N = 6) | 10 | [-100, 100] | 2000 |
Composite functions (N is basic number of functions) | ||||
F43 | Composite Function 1 (N = 3) | 10 | [-100, 100] | 2100 |
F44 | Composite Function 2 (N = 3) | 10 | [-100, 100] | 2200 |
F45 | Composite Function 3 (N = 4) | 10 | [-100, 100] | 2300 |
F46 | Composite Function 4 (N = 4) | 10 | [-100, 100] | 2400 |
F47 | Composite Function 5 (N = 5) | 10 | [-100, 100] | 2500 |
F48 | Composite Function 6 (N = 5) | 10 | [-100, 100] | 2600 |
F49 | Composite Function 7 (N = 6) | 10 | [-100, 100] | 2700 |
F50 | Composite Function 8 (N = 6) | 10 | [-100, 100] | 2800 |
F51 | Composite Function 9 (N = 6) | 10 | [-100, 100] | 2900 |
F52 | Composite Function 10 (N = 3) | 10 | [-100, 100] | 3000 |
F | IHAOHHO | IPOP-CMAES | LSHADE | SSC | RUN | HOA | |
F24 | Avg | 1.77467E + 09 | 1.00000E + 02 | 1.00000E + 02 | 2.50699E + 09 | 3.75483E + 04 | 3.50163E + 08 |
Std | 1.11254E + 09 | 0.00000E + 00 | 0.00000E + 00 | 1.72594E + 09 | 1.40126E + 04 | 1.57335E + 08 | |
F25 | Avg | 4.69684E + 03 | 3.00000E + 02 | 3.00000E + 02 | 4.84926E + 03 | 5.05458E + 04 | 6.03840E + 03 |
Std | 1.99656E + 03 | 0.00000E + 00 | 0.00000E + 00 | 2.04210E + 03 | 8.29687E + 03 | 2.15957E + 03 | |
F26 | Avg | 4.15353E + 02 | 4.00091E + 02 | 4.00000E + 02 | 6.35108E + 02 | 5.13264E + 02 | 4.53729E + 02 |
Std | 1.69972E + 01 | 4.43520E-02 | 0.00000E + 00 | 1.98490E + 02 | 1.81438E + 01 | 3.02199E + 01 | |
F27 | Avg | 5.57466E + 02 | 5.19197E + 02 | 5.02340E + 02 | 5.60349E + 02 | 6.53772E + 02 | 5.74943E + 02 |
Std | 1.01328E + 01 | 8.41520E + 00 | 8.75000E-01 | 1.84807E + 01 | 2.91163E + 01 | 1.10416E + 01 | |
F28 | Avg | 6.29286E + 02 | 6.00000E + 02 | 6.00000E + 02 | 6.36104E + 02 | 6.40548E + 02 | 6.37022E + 02 |
Std | 7.67590E + 00 | 0.00000E + 00 | 2.59000E-07 | 1.01438E + 01 | 8.25192E + 00 | 8.80160E + 00 | |
F29 | Avg | 7.69414E + 02 | 7.32212E + 02 | 7.12230E + 02 | 8.13508E + 02 | 9.36961E + 02 | 7.79986E + 02 |
Std | 1.20032E + 01 | 3.93460E + 00 | 6.05000E-01 | 1.64856E + 01 | 5.73895E + 01 | 1.27443E + 01 | |
F30 | Avg | 8.40446E + 02 | 8.14912E + 02 | 8.02140E + 02 | 8.46992E + 02 | 9.21845E + 02 | 8.55157E + 02 |
Std | 7.39460E + 00 | 8.47500E + 00 | 1.03100E + 00 | 1.04671E + 01 | 2.62983E + 01 | 8.87940E + 00 | |
F31 | Avg | 1.36798E + 03 | 9.00000E + 02 | 9.00000E + 02 | 1.44797E + 03 | 3.52693E + 03 | 1.09708E + 03 |
Std | 2.12360E + 02 | 0.00000E + 00 | 0.00000E + 00 | 2.89598E + 02 | 8.96934E + 02 | 1.40757E + 02 | |
F32 | Avg | 2.20829E + 03 | 2.29077E + 03 | 1.07003E + 03 | 2.89454E + 03 | 5.14578E + 03 | 2.87917E + 03 |
Std | 1.90189E + 02 | 2.37652E + 02 | 5.65600E + 01 | 2.06134E + 02 | 7.73937E + 02 | 2.06107E + 02 | |
F33 | Avg | 1.20861E + 03 | 1.16709E + 03 | 1.10002E + 03 | 1.38357E + 03 | 1.26564E + 03 | 1.30871E + 03 |
Std | 2.31887E + 01 | 1.35981E + 02 | 1.20000E-01 | 1.02038E + 02 | 3.23837E + 01 | 9.44024E + 01 | |
F34 | Avg | 9.65212E + 06 | 1.61308E + 03 | 1.33295E + 03 | 2.29908E + 06 | 1.38367E + 07 | 1.75532E + 07 |
Std | 6.54481E + 06 | 1.73789E + 02 | 8.62600E + 01 | 6.06195E + 06 | 9.36578E + 06 | 1.17226E + 07 | |
F35 | Avg | 1.73810E + 04 | 1.35805E + 03 | 1.30374E + 03 | 4.09334E + 04 | 2.63421E + 04 | 1.34045E + 06 |
Std | 1.00028E + 04 | 6.27520E + 01 | 3.26000E + 00 | 2.07982E + 04 | 1.45826E + 04 | 1.11750E + 06 | |
F36 | Avg | 2.51467E + 03 | 1.47391E + 03 | 1.40019E + 03 | 6.63735E + 03 | 2.27458E + 05 | 2.80598E + 03 |
Std | 1.21526E + 03 | 4.18650E + 01 | 4.50000E-01 | 1.23909E + 03 | 1.87179E + 05 | 1.25523E + 03 | |
F37 | Avg | 8.52054E + 03 | 1.51384E + 03 | 1.50033E + 03 | 1.77014E + 04 | 1.42723E + 04 | 3.15987E + 04 |
Std | 3.16501E + 03 | 2.10632E + 01 | 2.00000E-01 E + 0 |
7.89396E + 03 | 3.59942E + 03 | 3.55718E + 04 | |
F38 | Avg | 1.90369E + 03 | 1.66968E + 03 | 1.60087E + 03 | 1.92836E + 03 | 2.84572E + 03 | 2.00480E + 03 |
Std | 1.00750E + 02 | 3.53711E + 01 | 3.60000E-01 | 1.12527E + 02 | 3.28867E + 02 | 1.58304E + 02 | |
F39 | Avg | 1.78843E + 03 | 1.77638E + 03 | 1.70137E + 03 | 1.79644E + 03 | 2.24703E + 03 | 1.90857E + 03 |
Std | 3.41834E + 01 | 1.23878E + 01 | 3.84000E + 00 | 1.87221E + 01 | 2.22813E + 02 | 8.69727E + 01 | |
F40 | Avg | 1.71900E + 04 | 1.90952E + 03 | 1.80359E + 03 | 1.27448E + 05 | 6.11908E + 05 | 3.62014E + 05 |
Std | 1.05037E + 04 | 2.39660E + 01 | 7.60000E + 00 | 1.40079E + 05 | 7.60372E + 05 | 2.40106E + 05 | |
F41 | Avg | 1.58292E + 05 | 1.91811E + 03 | 1.90026E + 03 | 2.81911E + 04 | 4.43675E + 05 | 1.66843E + 04 |
Std | 4.02208E + 05 | 2.12890E + 01 | 3.00000E-02 | 6.61395E + 03 | 3.45206E + 05 | 1.56893E + 04 | |
F42 | Avg | 2.17157E + 03 | 2.05983E + 03 | 2.00023E + 03 | 2.28443E + 03 | 2.56095E + 03 | 2.26788E + 03 |
Std | 6.49892E + 01 | 1.85766E + 01 | 4.30000E-01 | 6.52079E + 01 | 1.70413E + 02 | 8.48882E + 01 | |
F43 | Avg | 2.30614E + 03 | 2.31934E + 03 | 2.25542E + 03 | 2.32175E + 03 | 2.44601E + 03 | 2.35311E + 03 |
Std | 2.47564E + 01 | 8.46660E + 00 | 5.21600E + 01 | 6.07100E + 01 | 2.52926E + 01 | 4.32033E + 01 | |
F44 | Avg | 2.41213E + 03 | 2.73101E + 03 | 2.30010E + 03 | 3.59786E + 03 | 3.31843E + 03 | 2.35109E + 03 |
Std | 8.37419E + 01 | 6.26970E + 01 | 1.70000E-01 | 7.07466E + 02 | 1.86094E + 03 | 3.37269E + 01 | |
F45 | Avg | 2.62413E + 03 | 2.63000E + 03 | 2.60230E + 03 | 2.65854E + 03 | 2.80612E + 03 | 2.72046E + 03 |
Std | 2.12841E + 01 | 5.55150E + 00 | 1.42000E + 00 | 2.74840E + 01 | 2.95349E + 01 | 2.25245E + 01 | |
F46 | Avg | 2.78904E + 03 | 2.70961E + 03 | 2.68830E + 03 | 2.80479E + 03 | 2.98605E + 03 | 2.82014E + 03 |
Std | 2.75184E + 01 | 9.78340E + 00 | 9.16500E + 01 | 2.35894E + 01 | 4.61846E + 01 | 5.20187E + 01 | |
F47 | Avg | 2.93523E + 03 | 2.93212E + 03 | 2.92377E + 03 | 3.02323E + 03 | 2.93603E + 03 | 2.96740E + 03 |
Std | 1.96255E + 01 | 8.33760E + 00 | 2.13000E + 01 | 5.86012E + 01 | 2.67693E + 01 | 1.82177E + 01 | |
F48 | Avg | 3.28386E + 03 | 3.21722E + 03 | 2.90000E + 03 | 4.05668E + 03 | 4.50153E + 03 | 3.33849E + 03 |
Std | 2.31698E + 02 | 2.03330E + 02 | 0.00000E + 00 | 2.87720E + 02 | 1.27120E + 03 | 4.73594E + 02 | |
F49 | Avg | 3.11226E + 03 | 3.08917E + 03 | 3.08903E + 03 | 3.11634E + 03 | 3.31206E + 03 | 3.23484E + 03 |
Std | 1.80612E + 01 | 4.24482E + 01 | 1.05000E + 00 | 2.68443E + 01 | 3.57031E + 01 | 5.28018E + 01 | |
F50 | Avg | 3.21692E + 03 | 3.27673E + 03 | 3.15435E + 03 | 3.25502E + 03 | 3.28169E + 03 | 3.49994E + 03 |
Std | 1.07032E + 02 | 9.66220E + 00 | 1.10920E + 01 | 3.19693E + 01 | 2.06487E + 01 | 1.36289E + 03 | |
F51 | Avg | 3.35870E + 03 | 3.27842E + 03 | 3.13492E + 03 | 3.36830E + 03 | 4.24526E + 03 | 3.37358E + 03 |
Std | 6.51044E + 01 | 5.74741E + 01 | 3.87000E + 00 | 9.54409E + 01 | 2.74106E + 02 | 7.28448E + 01 | |
F52 | Avg | 3.29001E + 06 | 3.28462E + 04 | 3.41838E + 03 | 7.61412E + 06 | 3.99731E + 06 | 4.12253E + 06 |
Std | 2.57991E + 06 | 2.60735E + 04 | 2.28600E + 01 | 4.84549E + 06 | 2.71864E + 06 | 4.95066E + 06 |
F | σinitial = 1, E = 1 |
σinitial = 1, E = 1.5 |
σinitial = 1, E = 2 |
σinitial = 1.5, E = 1 |
σinitial = 1.5, E = 1.5 |
σinitial = 1.5, E = 2 |
σinitial = 2, E = 1 |
σinitial = 2, E = 1.5 |
σinitial = 2, E = 2 |
F5 | 2.62E - 06 | 3.89E - 06 | 3.80E - 08 | 1.90E - 06 | 2.44E - 06 | 5.84E - 08 | 4.24E - 06 | 8.99E - 07 | 3.79E - 06 |
F7 | 4.04E - 08 | 6.20E - 08 | 1.47E - 08 | 5.79E - 08 | 1.32E - 07 | 6.93E - 08 | 3.18E - 08 | 1.60E - 08 | 2.08E - 08 |
F9 | 0 | 0 | 0 | 0 | 0 | 0 | 0 | 0 | 0 |
F13 | 1.16E - 10 | 1.04E - 11 | 3.20E - 12 | 3.29E - 11 | 3.81E - 11 | 2.52E - 11 | 5.34E - 12 | 8.14E - 11 | 7.61E - 11 |
F14 | 5.4418 | 0.43653 | 0.10935 | 0.54588 | 0.87306 | 0.87306 | 0.54591 | 1.7461 | 5.1709 |
F18 | 1.18E - 09 | 3.02E - 09 | 7.59E - 11 | 8.23E - 11 | 25.1496 | 25.1379 | 1.96E - 08 | 2.73E - 06 | 25.1593 |
F25 | 6.50E + 07 | 6.63E + 07 | 6.55E + 07 | 5.50E + 07 | 4.99E + 07 | 4.84E + 07 | 6.22E + 07 | 6.56E + 07 | 7.25E + 07 |
F26 | 2.94E + 04 | 2.14E + 04 | 1.51E + 04 | 1.26E + 04 | 1.87E + 04 | 1.86E + 04 | 1.47E + 04 | 2.76E + 04 | 2.70E + 04 |
F30 | 2.43E + 035 | 1.73E + 03 | 1.78E + 03 | 2.18E + 03 | 2.12E + 03 | 2.10E + 03 | 1.74E + 03 | 2.04E + 03 | 2.13E + 03 |
F33 | 6.61E + 04 | 5.12E + 04 | 2.92E + 04 | 8.56E + 04 | 4.04E + 04 | 6.08E + 04 | 5.48E + 04 | 4.45E + 04 | 6.75E + 04 |
F39 | 2.43E + 04 | 1.94E + 04 | 1.73E + 04 | 1.54E + 04 | 1.68E + 04 | 1.26E + 04 | 1.21E + 04 | 1.46E + 04 | 1.22E + 04 |
F44 | 4.31E + 04 | 5.06E + 04 | 4.78E + 04 | 5.88E + 04 | 4.08E + 04 | 9.79E + 04 | 4.93E + 04 | 6.43E + 04 | 7.79E + 04 |
F49 | 1.91E + 05 | 1.95E + 05 | 1.85E + 05 | 1.93E + 05 | 1.87E + 05 | 2.01E + 05 | 1.90E + 05 | 1.95E + 05 | 1.88E + 05 |
Algorithm | Optimum variables | Optimum weight | P-value | ||||||
x1 | x2 | x3 | x4 | x5 | x6 | x7 | |||
IHAOHHO | 3.49683 | 0.7 | 17 | 7.33302 | 7.8 | 3.35006 | 5.28575 | 2995.816 | NaN |
AO | 3.49688 | 0.7 | 17 | 8.10828 | 7.8 | 3.37081 | 5.28578 | 3008.168 | 0.025574 |
HHO | 3.49731 | 0.7 | 17 | 7.3 | 7.8 | 3.47527 | 5.28482 | 3028.6976 | 0.035339 |
HOA | 3.56008 | 0.7 | 17 | 7.34912 | 7.8 | 3.49325 | 5.28415 | 3058.577 | 6.1035e-05 |
SSA | 3.49732 | 0.7 | 17 | 8.03843 | 7.80061 | 3.52296 | 5.28577 | 3049.1538 | 0.012451 |
WOA | 3.4976 | 0.7 | 17 | 7.3 | 7.8 | 3.44134 | 5.28525 | 3019.3398 | 0.043721 |
MVO | 3.52164 | 0.7 | 17 | 7.44477 | 8.29729 | 3.43143 | 5.2842 | 3038.4984 | 0.018066 |
GWO | 3.49231 | 0.7 | 17.0038 | 8.1759 | 8.04815 | 3.35214 | 5.28783 | 3013.2315 | 0.0026245 |
Algorithm | Optimum variables | Optimum weight | P-value | ||
d | D | N | |||
IHAOHHO | 0.054826 | 0.49772 | 5.273 | 0.010881 | NaN |
AO | 0.051647 | 0.38603 | 9.3553 | 0.011692 | 6.1035e-05 |
HHO | 0.059559 | 0.64197 | 3.4141 | 0.012329 | 0.047913 |
HOA | 0.054031 | 0.47388 | 6.0876 | 0.011188 | 0.63867 |
SSA | 0.05 | 0.326589 | 12.8798 | 0.012149 | 0.00030518 |
WOA | 0.059166 | 0.62905 | 3.534 | 0.012186 | 0.047913 |
MVO | 0.059421 | 0.63742 | 3.4573 | 0.012282 | 0.025574 |
GWO | 0.057335 | 0.57116 | 4.1668 | 0.011579 | 6.1035e-05 |
Algorithm | Optimum variables | Optimum weight | P-value | |
x1 | x2 | |||
IHAOHHO | 0.79182 | 0.39856 | 263.8608 | NaN |
AO | 0.7932 | 0.39476 | 263.8689 | 0.04126 |
HHO | 0.7824 | 0.42541 | 263.8795 | 0.010254 |
HOA | 0.78192 | 0.4268 | 263.8841 | 0.030151 |
SSA | 0.78139 | 0.42834 | 263.8895 | 6.1035e-05 |
WOA | 0.77541 | 0.44621 | 263.9826 | 0.00012207 |
MVO | 0.78196 | 0.42668 | 263.8837 | 6.1035e-05 |
GWO | 0.79341 | 0.39418 | 263.8703 | 0.00018311 |
Algorithm 1 Pseudo-code of IHAOHHO |
1: Set the initial values of the population size N and the maximum number of iterations T |
2: Initialize positions of the population X |
3: While t < T |
4: Update x, y, cd |
5: For i = 1 to N |
6: If t < T/2 % Exploration part of AO |
7: Archiving |
8: If rand < 0.5 |
9: Update the position of Xnewi and X_Ri using Eqs (1) and (21), respectively |
10: Calculate the fitness of Xnewi and X_Ri |
11: Update the position of Xi |
12: Else |
13: Update the position of Xnewi and X_Ri using Eqs (3) and (21), respectively |
14: Calculate the fitness of Xnewi and X_Ri |
15: Update the position of Xi |
16: End if |
17: Else % Exploitation part of HHO |
18: If r ≥ 0.5 and |E| ≥ 0.5 |
19: Update the position of Xi using Eq (11) |
20: End if |
21: If r ≥ 0.5 and |E| < 0.5 |
22: Update the position of Xi using Eq (14) |
23: End if |
24: If r < 0.5 and |E| ≥ 0.5 |
25: Update the position of Xi using Eq (15) |
26: End if |
27: If r < 0.5 and |E| < 0.5 |
28: Update the position of Xi using Eq (18) |
29: End if |
30: Update the position of Xnewi using Eq (24) % Opposition-based learning (OBL) |
31: If f(Xnewi) < f(Xi) |
32: Xi = Xnewi |
33: End if |
34: End if |
35: t = t + 1 |
36: End for |
37: For i = 1 to N |
38: Check if the position goes out of the search space boundary and bring it back. |
39: Calculate the fitness of Xi |
40: Update Xbest |
41: End for |
42: End while |
43: Return Xbest |
Function | Dim | Range | fmin |
F1(x)=∑ni=1x2i | 30 | [-100, 100] | 0 |
F2(x)=∑ni=1|xi|+∏ni=1|xi| | 30 | [-10, 10] | 0 |
F3(x)=∑ni=1(∑ij−1xj)2 | 30 | [-100, 100] | 0 |
F4(x)=maxi{|xi|,1⩽i⩽n} | 30 | [-100, 100] | 0 |
F5(x)=∑n−1i=1[100(xi+1−x2i)2+(xi−1)2] | 30 | [-30, 30] | 0 |
F6(x)=∑ni=1(xi+5)2 | 30 | [-100, 100] | 0 |
F7(x)=∑ni=1ix4i+random[0,1) | 30 | [-1.28, 1.28] | 0 |
Function | Dim | Range | fmin |
F8(x)=∑ni=1−xisin(√|xi|) | 30 | [-500, 500] | -418.9829 × 30 |
F9(x)=∑ni=1[x2i−10cos(2πxi)+10] | 30 | [-5.12, 5.12] | 0 |
F10(x)=−20exp(−0.2√1n∑ni=1x2i)−exp(1n∑ni=1cos(2πxi))+20+e | 30 | [-32, 32] | 0 |
F11(x)=14000∑ni=1x2i−∏ni=1cos(xi√i)+1 | 30 | [-600, 600] | 0 |
F12(x)=πn{10sin(πy1)+∑n−1i=1(yi−1)2[1+10sin2(πyi+1)]+(yn}+∑ni=1u(xi,10,100,4), where yi=1+xi+14,u(xi,a,k,m)={k(xi−a)mxi>a0−a<xi<ak(−xi−a)mxi<−a | 30 | [-50, 50] | 0 |
F13(x)=0.1(sin2(3πx1)+∑ni=1(xi−1)2[1+sin2(3πxi+1)]+(xn−1)2[1+sin2(2πxn)])+∑ni=1u(xi,5,100,4) | 30 | [-50, 50] | 0 |
Function | Dim | Range | fmin |
F14(x)=(1500+∑25j=11j+∑2i=1(xi−aij)6)−1 | 2 | [-65, 65] | 1 |
F15(x)=∑11i=1[ai−x1(b2i+bix2)b2i+bix3+x4]2 | 4 | [-5, 5] | 0.00030 |
F16(x)=4x21−2.1x41+13x61+x1x2−4x22+x42 | 2 | [-5, 5] | -1.0316 |
F17(x)=(x2−5.14π2x21+5πx1−6)2+10(1−18π)cosx1+10 | 2 | [-5, 5] | 0.398 |
F18(x)=[1+(x1+x2+1)2(19−14x1+3x21−14x2+6x1x2+3x22)]×[30+(2x1−3x2)2×(18−32x2+12x21+48x2−36x1x2+27x22)] | 2 | [-2, 2] | 3 |
F19(x)=−∑4i=1ciexp(−∑3j=1aij(xj−pij)2) | 3 | [-1, 2] | -3.86 |
F20(x)=−∑4i=1ciexp(−∑6j=1aij(xj−pij)2) | 6 | [0, 1] | -3.32 |
F21(x)=−∑5i=1[(X−ai)(X−ai)T+ci]−1 | 4 | [0, 10] | -10.1532 |
F22(x)=−∑7i=1[(X−ai)(X−ai)T+ci]−1 | 4 | [0, 10] | -10.4028 |
F23(x)=−∑10i=1[(X−ai)(X−ai)T+ci]−1 | 4 | [0, 10] | -10.5363 |
Algorithm | Parameters |
IHAOHHO | σinitial = 1; σfinal = 0; Exponent = 2 |
AO | U = 0.00565; r1 = 10; ω = 0.005; α = 0.1; δ = 0.1; G1∈[-1, 1]; G2 = [2,0] |
HHO | q∈[0, 1]; r∈[0, 1]; E0∈[-1, 1]; E1 = [2,0]; E∈ [-2, 2]; |
HOA | hβ = 0.9; hγ = 0.5; sβ = 0.2; sγ = 0.1; iγ = 0.3; dα = 0.5; dβ = 0.2; dγ = 0.1; rδ = 0.1; rγ = 0.05 |
SSA | c1 = [1,0]; c2∈[0, 1]; c3∈[0, 1] |
WOA | a1 = [2,0]; a2 = [-1, -2]; b = 1 |
GWO | a = [2,0] |
MVO | WEP ∈ [0.2, 1]; TDR ∈ [0, 1]; r1, r2, r3 ∈ [0, 1] |
F | IHAOHHO | AO | HHO | HOA | SSA | WOA | MVO | GWO | |
F1 | Avg | 3.3660E-253 | 7.9345E-97 | 3.3401E-96 | 1.7886E-130 | 2.1594E-07 | 1.8120E-73 | 1.2681 | 1.0585E-27 |
Std | 0 | 4.3457E-96 | 1.7664E-95 | 8.6475E-130 | 3.8328E-07 | 8.0771E-73 | 3.8945E-01 | 9.7097E-28 | |
F2 | Avg | 1.5599E-127 | 1.7619E-60 | 1.9990E-49 | 2.1595E-66 | 1.7056 | 8.8551E-51 | 3.7930 | 7.8893E-17 |
Std | 8.5347E-127 | 9.6504E-60 | 9.5039E-49 | 1.1828E-65 | 1.3736 | 3.2476E-50 | 1.6432E+01 | 5.7891E-17 | |
F3 | Avg | 2.7431E-199 | 2.2314E-104 | 8.4798E-72 | 1.7856E+02 | 1.3173E+03 | 4.4381E+04 | 2.2448E+02 | 2.6928E-05 |
Std | 0 | 1.2222E-103 | 4.4387E-71 | 5.2479E+02 | 1.1198E+03 | 1.1940E+04 | 1.2075E+03 | 6.0686E-05 | |
F4 | Avg | 2.2207E-129 | 1.6827E-69 | 1.1057E-49 | 4.5440E-65 | 1.2612E+01 | 5.6993E+01 | 2.1139 | 8.0152E-07 |
Std | 1.1068E-128 | 7.6613E-69 | 3.0441E-49 | 2.4668E-64 | 3.8228 | 2.5529E+01 | 1.0284 | 8.3055E-07 | |
F5 | Avg | 5.3852E-04 | 5.4421E-03 | 2.1438E-02 | 2.8964E+01 | 2.2071E+02 | 2.7871E+01 | 3.8794E+02 | 2.7221E+01 |
Std | 2.2657E-03 | 1.2008E-02 | 2.4291E-02 | 3.5795E-02 | 3.4556E+02 | 4.6073E-01 | 7.0618E-02 | 7.9162E-01 | |
F6 | Avg | 3.5863E-06 | 1.2028E-04 | 1.4716E-04 | 6.5645 | 2.5056E-07 | 3.4988E-01 | 1.1414 | 7.2412E-01 |
Std | 7.7280E-06 | 1.7490E-04 | 2.0847E-04 | 3.4100E-01 | 3.8211E-07 | 1.7102E-01 | 4.4812E-01 | 3.9184E-01 | |
F7 | Avg | 9.5324E-05 | 1.0504E-04 | 1.3211E-04 | 6.7657E-02 | 1.6706E-01 | 2.7105E-03 | 3.4554E-02 | 2.1436E-03 |
Std | 7.6717E-05 | 8.6163E-05 | 1.4567E-04 | 4.0688E-02 | 7.8018E-02 | 3.2053E-03 | 1.5231E-02 | 1.3151E-03 | |
F8 | Avg | -12569.3858 | -8010.0774 | -12569.0257 | -4106.2204 | -7362.4999 | -10632.1912 | -7648.8103 | -6101.7628 |
Std | 1.8228E-01 | 4.0684E+03 | 7.5912E-01 | 7.7530E+02 | 8.6690E+02 | 0 | 5.2123E+02 | 8.9531E+02 | |
F9 | Avg | 0 | 0 | 0 | 8.0284E+01 | 5.3197E+01 | 0 | 1.2456E+02 | 3.3205 |
Std | 0 | 0 | 0 | 1.0686E+02 | 2.0211E+01 | 4.6777E-15 | 3.4040E+01 | 4.302 | |
F10 | Avg | 8.8818E-16 | 8.8818E-16 | 8.8818E-16 | 5.7436E-15 | 2.6880 | 2.7886E-15 | 1.7605 | 9.8230E-14 |
Std | 0 | 0 | 0 | 1.7413E-15 | 6.5906E-01 | 2.421E-15 | 6.6839E-01 | 1.7092E-14 | |
F11 | Avg | 0 | 0 | 0 | 2.0978E-01 | 2.3006E-02 | 1.1525E-02 | 8.6051E-01 | 5.3578E-03 |
Std | 0 | 0 | 0 | 3.7796E-01 | 1.5437E-02 | 4.4029E-02 | 8.3088E-02 | 7.3633E-03 | |
F12 | Avg | 2.6974E-07 | 2.8068E-06 | 1.9506E-05 | 1.1656 | 7.8473 | 2.1363E-02 | 1.8746 | 5.7227E-02 |
Std | 4.4163E-07 | 5.7142E-06 | 3.8352E-05 | 2.1289E-01 | 2.7926 | 1.2107E-02 | 1.1276 | 9.6891E-02 | |
F13 | Avg | 3.0227E-06 | 4.9439E-05 | 9.7772E-05 | 3.0571 | 1.8860E+01 | 5.3918E-01 | 1.7158E-01 | 6.2217E-01 |
Std | 5.0785E-06 | 9.2988E-05 | 9.5928E-05 | 1.8645E-01 | 1.6484E+01 | 2.9800E-01 | 1.0772E-01 | 2.5487E-01 | |
F14 | Avg | 1.5932 | 2.0487 | 1.2629 | 2.9322 | 1.3943 | 2.8615 | 0.998 | 4.6223 |
Std | 9.2477E-01 | 2.1859 | 5.1727E-01 | 2.1542 | 9.5834E-01 | 3.3284 | 2.7158E-11 | 4.1450 | |
F15 | Avg | 4.4227E-04 | 4.9359E-04 | 3.9582E-04 | 7.1269E-03 | 2.2249E-03 | 7.5110E-04 | 7.8593E-03 | 5.7748E-03 |
Std | 3.4587E-04 | 1.1786E-04 | 2.3573E-04 | 7.5345E-03 | 4.9361E-03 | 4.9981E-04 | 1.3437E-02 | 8.9518E-03 | |
F16 | Avg | -1.0316 | -1.0312 | -1.0316 | -0.99752 | -1.0316 | -1.0316 | -1.0316 | -1.0316 |
Std | 3.1088E-08 | 4.0636E-04 | 7.1130E-09 | 3.1957E-02 | 1.8547E-14 | 7.6118E-10 | 4.5176E-07 | 3.6867E-08 | |
F17 | Avg | 3.9789E-01 | 3.9813E-01 | 3.9789E-01 | 3.9790E-01 | 3.9789E-01 | 3.9790E-01 | 3.9789E-01 | 3.9789E-01 |
Std | 4.0428E-05 | 3.0290E-04 | 4.5913E-05 | 1.1204E-03 | 9.2471E-15 | 1.5755E-05 | 7.7424E-08 | 7.6565E-07 | |
F18 | Avg | 3 | 3.0243 | 3 | 7.7479 | 3 | 3.0001 | 3 | 3 |
Std | 3.3248E-06 | 5.5712E-02 | 6.1620E-07 | 1.6153E+01 | 1.9031E-13 | 3.1781E-04 | 2.5080E-06 | 4.7166E-05 | |
F19 | Avg | -3.8258 | -3.8535 | -3.8597 | -3.8619 | -3.8628 | -3.8551 | -3.8628 | -3.8598 |
Std | 7.1972E-02 | 8.7650E-03 | 3.8900E-03 | 6.2715E-04 | 1.9037E-12 | 8.6573E-03 | 3.7043E-06 | 3.9554E-03 | |
F20 | Avg | -3.0792 | -3.1678 | -3.0813 | -3.2580 | -3.2335 | -3.2080 | -3.2448 | -3.2721 |
Std | 1.1913E-01 | 7.3334E-02 | 9.9164E-02 | 7.5769E-02 | 6.4415E-02 | 1.1882E-01 | 5.9771E-02 | 7.6156E-02 | |
F21 | Avg | -10.1525 | -10.1422 | -5.2145 | -9.3937 | -6.6406 | -9.2821 | -6.9627 | -9.6452 |
Std | 1.4463E-03 | 1.8290E-02 | 8.8739E-01 | 1.2967 | 3.4554 | 1.9234 | 3.1618 | 1.5462 | |
F22 | Avg | -10.4026 | -10.3914 | -5.0820 | -9.5706 | -9.7954 | -7.6313 | -8.6587 | -10.4009 |
Std | 6.6551E-04 | 2.1551E-02 | 8.7380E-03 | 1.5379 | 1.8875 | 3.0683 | 2.7631 | 1.2358E-03 | |
F23 | Avg | -10.5359 | -10.5292 | -5.1234 | -9.8166 | -8.7191 | -7.0303 | -7.5296 | -10.5348 |
Std | 6.6691E-04 | 1.2664E-02 | 52035E-03 | 1.0080 | 3.1206 | 3.4442 | 3.5941 | 8.4873E-04 |
F | IHAOHHO vs AO | IHAOHHO vs HHO | IHAOHHO vs HOA | IHAOHHO vs SSA | IHAOHHO vs WOA | IHAOHHO vs MVO | IHAOHHO vs GWO |
F1 | 6.1035E-05 | 6.1035E-05 | 6.1035E-05 | 6.1035E-05 | 6.1035E-05 | 6.1035E-05 | 6.1035E-05 |
F2 | 6.1035E-05 | 6.1035E-05 | 6.1035E-05 | 6.1035E-05 | 6.1035E-05 | 6.1035E-05 | 6.1035E-05 |
F3 | 6.1035E-05 | 6.1035E-05 | 6.1035E-05 | 6.1035E-05 | 6.1035E-05 | 6.1035E-05 | 6.1035E-05 |
F4 | 6.1035E-05 | 6.1035E-05 | 6.1035E-05 | 6.1035E-05 | 6.1035E-05 | 6.1035E-05 | 6.1035E-05 |
F5 | 0.10699 | 1.2207E-04 | 6.1035E-05 | 6.1035E-05 | 6.1035E-05 | 6.1035E-05 | 6.1035E-05 |
F6 | 1.8311E-04 | 1.8311E-04 | 6.1035E-05 | 1.1597E-03 | 6.1035E-05 | 6.1035E-05 | 6.1035E-05 |
F7 | 6.3721E-02 | 0.10699 | 6.1035E-05 | 6.1035E-05 | 6.1035E-05 | 6.1035E-05 | 6.1035E-05 |
F8 | 6.1035E-05 | 0.63867 | 6.1035E-05 | 6.1035E-05 | 6.1035E-05 | 6.1035E-05 | 6.1035E-05 |
F9 | NaN | NaN | 6.1035E-05 | 6.1035E-05 | NaN | 6.1035E-05 | 6.1035E-05 |
F10 | NaN | NaN | 6.1035E-05 | 6.1035E-05 | 1.9531E-03 | 6.1035E-05 | 6.1035E-05 |
F11 | NaN | NaN | 0.1250 | 6.1035E-05 | NaN | 6.1035E-05 | NaN |
F12 | 2.6245E-03 | 1.2207E-04 | 6.1035E-05 | 6.1035E-05 | 6.1035E-05 | 6.1035E-05 | 6.1035E-05 |
F13 | 2.1545E-02 | 1.2207E-04 | 6.1035E-05 | 6.1035E-05 | 6.1035E-05 | 6.1035E-05 | 6.1035E-05 |
F14 | 0.97797 | 0.45428 | 1.8066E-02 | 2.6245E-03 | 0.18762 | 6.1035E-05 | 3.5339E-02 |
F15 | 6.1035E-05 | 0.45428 | 6.1035E-05 | 6.1035E-05 | 6.1035E-05 | 6.1035E-05 | 0.3028 |
F16 | 6.1035E-05 | 8.3618E-03 | 6.1035E-05 | 6.1035E-05 | 9.4604E-02 | 6.1035E-05 | 5.5359E-02 |
F17 | 6.1035E-04 | 0.45428 | 6.1035E-05 | 6.1035E-05 | 0.27686 | 3.0518E-04 | 1.0254E-02 |
F18 | 8.3252E-02 | 1.2207E-04 | 8.3252E-02 | 6.1035E-05 | 0.48871 | 0.67877 | 0.13538 |
F19 | 7.2998E-02 | 1.8066E-02 | 7.2998E-02 | 6.1035E-05 | 1.8066E-02 | 6.1035E-05 | 3.0151E-02 |
F20 | 6.1035E-04 | 0.93408 | 8.5449E-04 | 1.5259E-03 | 2.0142E-03 | 6.1035E-05 | 8.5449E-04 |
F21 | 3.3569E-03 | 6.1035E-05 | 6.1035E-05 | 4.2120E-02 | 1.2207E-04 | 2.0142E-03 | 1.8311E-04 |
F22 | 1.2207E-04 | 6.1035E-05 | 6.1035E-05 | 8.3252E-02 | 1.8311E-04 | 4.126E-02 | 8.3618E-03 |
F23 | 1.1597E-03 | 6.1035E-05 | 6.1035E-05 | 3.3026E-02 | 6.1035E-05 | 1.6882E-02 | 6.7139E-03 |
Function | Name | Dim | Range | fmin |
Unimodal functions | ||||
F24 | Shifted and Rotated Bent Cigar Function | 10 | [-100, 100] | 100 |
F25 | Shifted and Rotated Zakharov Function | 10 | [-100, 100] | 300 |
Multimodal functions | ||||
F26 | Shifted and Rotated Rosenbrock's Function | 10 | [-100, 100] | 400 |
F27 | Shifted and Rotated Rastrigin's Function | 10 | [-100, 100] | 500 |
F28 | Shifted and Rotated Expanded Scaffer's F6 Function | 10 | [-100, 100] | 600 |
F29 | Shifted and Rotated Lunacek Bi-RastriginFunction | 10 | [-100, 100] | 700 |
F30 | Shifted and Rotated Non-Continuous Rastrigin's Function | 10 | [-100, 100] | 800 |
F31 | Shifted and Rotated Levy Function | 10 | [-100, 100] | 900 |
F32 | Shifted and Rotated Schwefel's Function | 10 | [-100, 100] | 1000 |
Hybrid functions (N is basic number of functions) | ||||
F33 | Hybrid Function 1 (N = 3) | 10 | [-100, 100] | 1100 |
F34 | Hybrid Function 2 (N = 3) | 10 | [-100, 100] | 1200 |
F35 | Hybrid Function 3 (N = 3) | 10 | [-100, 100] | 1300 |
F36 | Hybrid Function 4 (N = 4) | 10 | [-100, 100] | 1400 |
F37 | Hybrid Function 5 (N = 4) | 10 | [-100, 100] | 1500 |
F38 | Hybrid Function 6 (N = 4) | 10 | [-100, 100] | 1600 |
F39 | Hybrid Function 6 (N = 5) | 10 | [-100, 100] | 1700 |
F40 | Hybrid Function 6 (N = 5) | 10 | [-100, 100] | 1800 |
F41 | Hybrid Function 6 (N = 5) | 10 | [-100, 100] | 1900 |
F42 | Hybrid Function 6 (N = 6) | 10 | [-100, 100] | 2000 |
Composite functions (N is basic number of functions) | ||||
F43 | Composite Function 1 (N = 3) | 10 | [-100, 100] | 2100 |
F44 | Composite Function 2 (N = 3) | 10 | [-100, 100] | 2200 |
F45 | Composite Function 3 (N = 4) | 10 | [-100, 100] | 2300 |
F46 | Composite Function 4 (N = 4) | 10 | [-100, 100] | 2400 |
F47 | Composite Function 5 (N = 5) | 10 | [-100, 100] | 2500 |
F48 | Composite Function 6 (N = 5) | 10 | [-100, 100] | 2600 |
F49 | Composite Function 7 (N = 6) | 10 | [-100, 100] | 2700 |
F50 | Composite Function 8 (N = 6) | 10 | [-100, 100] | 2800 |
F51 | Composite Function 9 (N = 6) | 10 | [-100, 100] | 2900 |
F52 | Composite Function 10 (N = 3) | 10 | [-100, 100] | 3000 |
F | IHAOHHO | IPOP-CMAES | LSHADE | SSC | RUN | HOA | |
F24 | Avg | 1.77467E + 09 | 1.00000E + 02 | 1.00000E + 02 | 2.50699E + 09 | 3.75483E + 04 | 3.50163E + 08 |
Std | 1.11254E + 09 | 0.00000E + 00 | 0.00000E + 00 | 1.72594E + 09 | 1.40126E + 04 | 1.57335E + 08 | |
F25 | Avg | 4.69684E + 03 | 3.00000E + 02 | 3.00000E + 02 | 4.84926E + 03 | 5.05458E + 04 | 6.03840E + 03 |
Std | 1.99656E + 03 | 0.00000E + 00 | 0.00000E + 00 | 2.04210E + 03 | 8.29687E + 03 | 2.15957E + 03 | |
F26 | Avg | 4.15353E + 02 | 4.00091E + 02 | 4.00000E + 02 | 6.35108E + 02 | 5.13264E + 02 | 4.53729E + 02 |
Std | 1.69972E + 01 | 4.43520E-02 | 0.00000E + 00 | 1.98490E + 02 | 1.81438E + 01 | 3.02199E + 01 | |
F27 | Avg | 5.57466E + 02 | 5.19197E + 02 | 5.02340E + 02 | 5.60349E + 02 | 6.53772E + 02 | 5.74943E + 02 |
Std | 1.01328E + 01 | 8.41520E + 00 | 8.75000E-01 | 1.84807E + 01 | 2.91163E + 01 | 1.10416E + 01 | |
F28 | Avg | 6.29286E + 02 | 6.00000E + 02 | 6.00000E + 02 | 6.36104E + 02 | 6.40548E + 02 | 6.37022E + 02 |
Std | 7.67590E + 00 | 0.00000E + 00 | 2.59000E-07 | 1.01438E + 01 | 8.25192E + 00 | 8.80160E + 00 | |
F29 | Avg | 7.69414E + 02 | 7.32212E + 02 | 7.12230E + 02 | 8.13508E + 02 | 9.36961E + 02 | 7.79986E + 02 |
Std | 1.20032E + 01 | 3.93460E + 00 | 6.05000E-01 | 1.64856E + 01 | 5.73895E + 01 | 1.27443E + 01 | |
F30 | Avg | 8.40446E + 02 | 8.14912E + 02 | 8.02140E + 02 | 8.46992E + 02 | 9.21845E + 02 | 8.55157E + 02 |
Std | 7.39460E + 00 | 8.47500E + 00 | 1.03100E + 00 | 1.04671E + 01 | 2.62983E + 01 | 8.87940E + 00 | |
F31 | Avg | 1.36798E + 03 | 9.00000E + 02 | 9.00000E + 02 | 1.44797E + 03 | 3.52693E + 03 | 1.09708E + 03 |
Std | 2.12360E + 02 | 0.00000E + 00 | 0.00000E + 00 | 2.89598E + 02 | 8.96934E + 02 | 1.40757E + 02 | |
F32 | Avg | 2.20829E + 03 | 2.29077E + 03 | 1.07003E + 03 | 2.89454E + 03 | 5.14578E + 03 | 2.87917E + 03 |
Std | 1.90189E + 02 | 2.37652E + 02 | 5.65600E + 01 | 2.06134E + 02 | 7.73937E + 02 | 2.06107E + 02 | |
F33 | Avg | 1.20861E + 03 | 1.16709E + 03 | 1.10002E + 03 | 1.38357E + 03 | 1.26564E + 03 | 1.30871E + 03 |
Std | 2.31887E + 01 | 1.35981E + 02 | 1.20000E-01 | 1.02038E + 02 | 3.23837E + 01 | 9.44024E + 01 | |
F34 | Avg | 9.65212E + 06 | 1.61308E + 03 | 1.33295E + 03 | 2.29908E + 06 | 1.38367E + 07 | 1.75532E + 07 |
Std | 6.54481E + 06 | 1.73789E + 02 | 8.62600E + 01 | 6.06195E + 06 | 9.36578E + 06 | 1.17226E + 07 | |
F35 | Avg | 1.73810E + 04 | 1.35805E + 03 | 1.30374E + 03 | 4.09334E + 04 | 2.63421E + 04 | 1.34045E + 06 |
Std | 1.00028E + 04 | 6.27520E + 01 | 3.26000E + 00 | 2.07982E + 04 | 1.45826E + 04 | 1.11750E + 06 | |
F36 | Avg | 2.51467E + 03 | 1.47391E + 03 | 1.40019E + 03 | 6.63735E + 03 | 2.27458E + 05 | 2.80598E + 03 |
Std | 1.21526E + 03 | 4.18650E + 01 | 4.50000E-01 | 1.23909E + 03 | 1.87179E + 05 | 1.25523E + 03 | |
F37 | Avg | 8.52054E + 03 | 1.51384E + 03 | 1.50033E + 03 | 1.77014E + 04 | 1.42723E + 04 | 3.15987E + 04 |
Std | 3.16501E + 03 | 2.10632E + 01 | 2.00000E-01 E + 0 |
7.89396E + 03 | 3.59942E + 03 | 3.55718E + 04 | |
F38 | Avg | 1.90369E + 03 | 1.66968E + 03 | 1.60087E + 03 | 1.92836E + 03 | 2.84572E + 03 | 2.00480E + 03 |
Std | 1.00750E + 02 | 3.53711E + 01 | 3.60000E-01 | 1.12527E + 02 | 3.28867E + 02 | 1.58304E + 02 | |
F39 | Avg | 1.78843E + 03 | 1.77638E + 03 | 1.70137E + 03 | 1.79644E + 03 | 2.24703E + 03 | 1.90857E + 03 |
Std | 3.41834E + 01 | 1.23878E + 01 | 3.84000E + 00 | 1.87221E + 01 | 2.22813E + 02 | 8.69727E + 01 | |
F40 | Avg | 1.71900E + 04 | 1.90952E + 03 | 1.80359E + 03 | 1.27448E + 05 | 6.11908E + 05 | 3.62014E + 05 |
Std | 1.05037E + 04 | 2.39660E + 01 | 7.60000E + 00 | 1.40079E + 05 | 7.60372E + 05 | 2.40106E + 05 | |
F41 | Avg | 1.58292E + 05 | 1.91811E + 03 | 1.90026E + 03 | 2.81911E + 04 | 4.43675E + 05 | 1.66843E + 04 |
Std | 4.02208E + 05 | 2.12890E + 01 | 3.00000E-02 | 6.61395E + 03 | 3.45206E + 05 | 1.56893E + 04 | |
F42 | Avg | 2.17157E + 03 | 2.05983E + 03 | 2.00023E + 03 | 2.28443E + 03 | 2.56095E + 03 | 2.26788E + 03 |
Std | 6.49892E + 01 | 1.85766E + 01 | 4.30000E-01 | 6.52079E + 01 | 1.70413E + 02 | 8.48882E + 01 | |
F43 | Avg | 2.30614E + 03 | 2.31934E + 03 | 2.25542E + 03 | 2.32175E + 03 | 2.44601E + 03 | 2.35311E + 03 |
Std | 2.47564E + 01 | 8.46660E + 00 | 5.21600E + 01 | 6.07100E + 01 | 2.52926E + 01 | 4.32033E + 01 | |
F44 | Avg | 2.41213E + 03 | 2.73101E + 03 | 2.30010E + 03 | 3.59786E + 03 | 3.31843E + 03 | 2.35109E + 03 |
Std | 8.37419E + 01 | 6.26970E + 01 | 1.70000E-01 | 7.07466E + 02 | 1.86094E + 03 | 3.37269E + 01 | |
F45 | Avg | 2.62413E + 03 | 2.63000E + 03 | 2.60230E + 03 | 2.65854E + 03 | 2.80612E + 03 | 2.72046E + 03 |
Std | 2.12841E + 01 | 5.55150E + 00 | 1.42000E + 00 | 2.74840E + 01 | 2.95349E + 01 | 2.25245E + 01 | |
F46 | Avg | 2.78904E + 03 | 2.70961E + 03 | 2.68830E + 03 | 2.80479E + 03 | 2.98605E + 03 | 2.82014E + 03 |
Std | 2.75184E + 01 | 9.78340E + 00 | 9.16500E + 01 | 2.35894E + 01 | 4.61846E + 01 | 5.20187E + 01 | |
F47 | Avg | 2.93523E + 03 | 2.93212E + 03 | 2.92377E + 03 | 3.02323E + 03 | 2.93603E + 03 | 2.96740E + 03 |
Std | 1.96255E + 01 | 8.33760E + 00 | 2.13000E + 01 | 5.86012E + 01 | 2.67693E + 01 | 1.82177E + 01 | |
F48 | Avg | 3.28386E + 03 | 3.21722E + 03 | 2.90000E + 03 | 4.05668E + 03 | 4.50153E + 03 | 3.33849E + 03 |
Std | 2.31698E + 02 | 2.03330E + 02 | 0.00000E + 00 | 2.87720E + 02 | 1.27120E + 03 | 4.73594E + 02 | |
F49 | Avg | 3.11226E + 03 | 3.08917E + 03 | 3.08903E + 03 | 3.11634E + 03 | 3.31206E + 03 | 3.23484E + 03 |
Std | 1.80612E + 01 | 4.24482E + 01 | 1.05000E + 00 | 2.68443E + 01 | 3.57031E + 01 | 5.28018E + 01 | |
F50 | Avg | 3.21692E + 03 | 3.27673E + 03 | 3.15435E + 03 | 3.25502E + 03 | 3.28169E + 03 | 3.49994E + 03 |
Std | 1.07032E + 02 | 9.66220E + 00 | 1.10920E + 01 | 3.19693E + 01 | 2.06487E + 01 | 1.36289E + 03 | |
F51 | Avg | 3.35870E + 03 | 3.27842E + 03 | 3.13492E + 03 | 3.36830E + 03 | 4.24526E + 03 | 3.37358E + 03 |
Std | 6.51044E + 01 | 5.74741E + 01 | 3.87000E + 00 | 9.54409E + 01 | 2.74106E + 02 | 7.28448E + 01 | |
F52 | Avg | 3.29001E + 06 | 3.28462E + 04 | 3.41838E + 03 | 7.61412E + 06 | 3.99731E + 06 | 4.12253E + 06 |
Std | 2.57991E + 06 | 2.60735E + 04 | 2.28600E + 01 | 4.84549E + 06 | 2.71864E + 06 | 4.95066E + 06 |
F | σinitial = 1, E = 1 |
σinitial = 1, E = 1.5 |
σinitial = 1, E = 2 |
σinitial = 1.5, E = 1 |
σinitial = 1.5, E = 1.5 |
σinitial = 1.5, E = 2 |
σinitial = 2, E = 1 |
σinitial = 2, E = 1.5 |
σinitial = 2, E = 2 |
F5 | 2.62E - 06 | 3.89E - 06 | 3.80E - 08 | 1.90E - 06 | 2.44E - 06 | 5.84E - 08 | 4.24E - 06 | 8.99E - 07 | 3.79E - 06 |
F7 | 4.04E - 08 | 6.20E - 08 | 1.47E - 08 | 5.79E - 08 | 1.32E - 07 | 6.93E - 08 | 3.18E - 08 | 1.60E - 08 | 2.08E - 08 |
F9 | 0 | 0 | 0 | 0 | 0 | 0 | 0 | 0 | 0 |
F13 | 1.16E - 10 | 1.04E - 11 | 3.20E - 12 | 3.29E - 11 | 3.81E - 11 | 2.52E - 11 | 5.34E - 12 | 8.14E - 11 | 7.61E - 11 |
F14 | 5.4418 | 0.43653 | 0.10935 | 0.54588 | 0.87306 | 0.87306 | 0.54591 | 1.7461 | 5.1709 |
F18 | 1.18E - 09 | 3.02E - 09 | 7.59E - 11 | 8.23E - 11 | 25.1496 | 25.1379 | 1.96E - 08 | 2.73E - 06 | 25.1593 |
F25 | 6.50E + 07 | 6.63E + 07 | 6.55E + 07 | 5.50E + 07 | 4.99E + 07 | 4.84E + 07 | 6.22E + 07 | 6.56E + 07 | 7.25E + 07 |
F26 | 2.94E + 04 | 2.14E + 04 | 1.51E + 04 | 1.26E + 04 | 1.87E + 04 | 1.86E + 04 | 1.47E + 04 | 2.76E + 04 | 2.70E + 04 |
F30 | 2.43E + 035 | 1.73E + 03 | 1.78E + 03 | 2.18E + 03 | 2.12E + 03 | 2.10E + 03 | 1.74E + 03 | 2.04E + 03 | 2.13E + 03 |
F33 | 6.61E + 04 | 5.12E + 04 | 2.92E + 04 | 8.56E + 04 | 4.04E + 04 | 6.08E + 04 | 5.48E + 04 | 4.45E + 04 | 6.75E + 04 |
F39 | 2.43E + 04 | 1.94E + 04 | 1.73E + 04 | 1.54E + 04 | 1.68E + 04 | 1.26E + 04 | 1.21E + 04 | 1.46E + 04 | 1.22E + 04 |
F44 | 4.31E + 04 | 5.06E + 04 | 4.78E + 04 | 5.88E + 04 | 4.08E + 04 | 9.79E + 04 | 4.93E + 04 | 6.43E + 04 | 7.79E + 04 |
F49 | 1.91E + 05 | 1.95E + 05 | 1.85E + 05 | 1.93E + 05 | 1.87E + 05 | 2.01E + 05 | 1.90E + 05 | 1.95E + 05 | 1.88E + 05 |
Algorithm | Optimum variables | Optimum weight | P-value | ||||||
x1 | x2 | x3 | x4 | x5 | x6 | x7 | |||
IHAOHHO | 3.49683 | 0.7 | 17 | 7.33302 | 7.8 | 3.35006 | 5.28575 | 2995.816 | NaN |
AO | 3.49688 | 0.7 | 17 | 8.10828 | 7.8 | 3.37081 | 5.28578 | 3008.168 | 0.025574 |
HHO | 3.49731 | 0.7 | 17 | 7.3 | 7.8 | 3.47527 | 5.28482 | 3028.6976 | 0.035339 |
HOA | 3.56008 | 0.7 | 17 | 7.34912 | 7.8 | 3.49325 | 5.28415 | 3058.577 | 6.1035e-05 |
SSA | 3.49732 | 0.7 | 17 | 8.03843 | 7.80061 | 3.52296 | 5.28577 | 3049.1538 | 0.012451 |
WOA | 3.4976 | 0.7 | 17 | 7.3 | 7.8 | 3.44134 | 5.28525 | 3019.3398 | 0.043721 |
MVO | 3.52164 | 0.7 | 17 | 7.44477 | 8.29729 | 3.43143 | 5.2842 | 3038.4984 | 0.018066 |
GWO | 3.49231 | 0.7 | 17.0038 | 8.1759 | 8.04815 | 3.35214 | 5.28783 | 3013.2315 | 0.0026245 |
Algorithm | Optimum variables | Optimum weight | P-value | ||
d | D | N | |||
IHAOHHO | 0.054826 | 0.49772 | 5.273 | 0.010881 | NaN |
AO | 0.051647 | 0.38603 | 9.3553 | 0.011692 | 6.1035e-05 |
HHO | 0.059559 | 0.64197 | 3.4141 | 0.012329 | 0.047913 |
HOA | 0.054031 | 0.47388 | 6.0876 | 0.011188 | 0.63867 |
SSA | 0.05 | 0.326589 | 12.8798 | 0.012149 | 0.00030518 |
WOA | 0.059166 | 0.62905 | 3.534 | 0.012186 | 0.047913 |
MVO | 0.059421 | 0.63742 | 3.4573 | 0.012282 | 0.025574 |
GWO | 0.057335 | 0.57116 | 4.1668 | 0.011579 | 6.1035e-05 |
Algorithm | Optimum variables | Optimum weight | P-value | |
x1 | x2 | |||
IHAOHHO | 0.79182 | 0.39856 | 263.8608 | NaN |
AO | 0.7932 | 0.39476 | 263.8689 | 0.04126 |
HHO | 0.7824 | 0.42541 | 263.8795 | 0.010254 |
HOA | 0.78192 | 0.4268 | 263.8841 | 0.030151 |
SSA | 0.78139 | 0.42834 | 263.8895 | 6.1035e-05 |
WOA | 0.77541 | 0.44621 | 263.9826 | 0.00012207 |
MVO | 0.78196 | 0.42668 | 263.8837 | 6.1035e-05 |
GWO | 0.79341 | 0.39418 | 263.8703 | 0.00018311 |