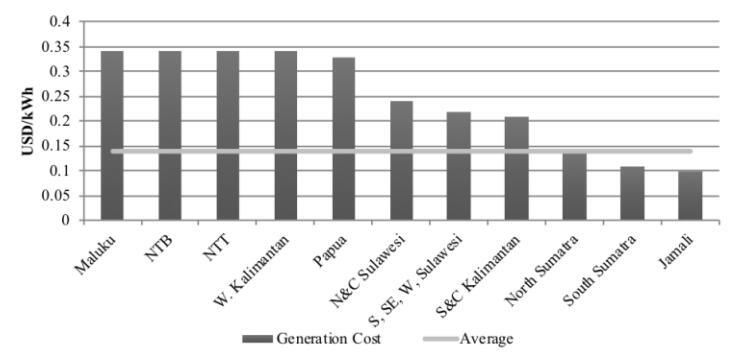
Citation: Lukáš Pichl, Taisei Kaizoji. Volatility Analysis of Bitcoin Price Time Series[J]. Quantitative Finance and Economics, 2017, 1(4): 474-485. doi: 10.3934/QFE.2017.4.474
[1] | Ali Omidkar, Razieh Es'haghian, Hua Song . Developing a machine learning model for fast economic optimization of solar power plants using the hybrid method of firefly and genetic algorithms, case study: optimizing solar thermal collector in Calgary, Alberta. Green Finance, 2024, 6(4): 698-727. doi: 10.3934/GF.2024027 |
[2] | Yoram Krozer . Financing of the global shift to renewable energy and energy efficiency. Green Finance, 2019, 1(3): 264-278. doi: 10.3934/GF.2019.3.264 |
[3] | Laura Grumann, Mara Madaleno, Elisabete Vieira . The green finance dilemma: No impact without risk – a multiple case study on renewable energy investments. Green Finance, 2024, 6(3): 457-483. doi: 10.3934/GF.2024018 |
[4] | Manuel Padeira Navarro, Margarida Catalão-Lopes . A structured financial product applied to renewable energies. Green Finance, 2019, 1(1): 82-93. doi: 10.3934/GF.2019.1.82 |
[5] | Biljana Ilić, Dragica Stojanovic, Gordana Djukic . Green economy: mobilization of international capital for financing projects of renewable energy sources. Green Finance, 2019, 1(2): 94-109. doi: 10.3934/GF.2019.2.94 |
[6] | Samuel Asante Gyamerah, Bright Emmanuel Owusu, Ellis Kofi Akwaa-Sekyi . Modelling the mean and volatility spillover between green bond market and renewable energy stock market. Green Finance, 2022, 4(3): 310-328. doi: 10.3934/GF.2022015 |
[7] | Rui M. Pereira, Alfredo M. Pereira . Financing a renewable energy feed-in tariff with a tax on carbon dioxide emissions: A dynamic multi-sector general equilibrium analysis for Portugal. Green Finance, 2019, 1(3): 279-296. doi: 10.3934/GF.2019.3.279 |
[8] | Clement Allan Tisdell . Renewable energy use and the renewable energy sector’s development: public finance, environmental externalities and sustainability. Green Finance, 2019, 1(2): 156-173. doi: 10.3934/GF.2019.2.156 |
[9] | Gireesh Shrimali . Do clean energy (equity) investments add value to a portfolio?. Green Finance, 2019, 1(2): 188-204. doi: 10.3934/GF.2019.2.188 |
[10] | Wen-Tien Tsai . Green finance for mitigating greenhouse gases and promoting renewable energy development: Case study in Taiwan. Green Finance, 2024, 6(2): 249-264. doi: 10.3934/GF.2024010 |
As an emerging economy, Indonesia faces multi-faceted challenges in its rapidly growing energy sector, which includes providing modern energy services to the poor, reducing oil dependency, and decarbonization (Kusdiana, 2008). Indonesia's electrification ratio in 2015 was 88.3%. The government of Indonesia aims to increase the electrification ratio to 99.7% by 2025 and also meet the rapidly increasing electricity demand, which is projected to increase on average by 8.6% per year for the period 2016–2025 (PLN, 2016). Indonesia has a lot of rural and remote areas, and a majority of people without access to electricity lives either in the countryside or on small islands (UNDP Indonesia, 2009). In most of the cases in the past, diesel generators have been used for the electrification of remote areas and islands that are too remote for grid extension (Bhattacharyya, 2013). However, as it can be observed in Figure 1, transportation of fuel to remote areas and islands creates great differences in the generation costs. On the other hand, as renewable energy technologies have been increasingly deployed worldwide, the costs of renewable energy technologies have significantly decreased in recent years, and renewable hybrid mini-grid systems have been perceived as one of the best choices for electrification and replacement of traditional diesel-based systems in more and more places. A hybrid mini-grid system is a system combing two or more energy sources, operated jointly, including a storage unit and connection to a local AC distribution network. The Indonesian government perceives renewable hybrid mini-grid systems as one of the key solutions to achieve the targeted electrification ratio, and also to decarbonize and lower generation cost of existing traditional diesel-based systems (PLN, 2016).
In this paper, local data are used to evaluate the economic viability of renewable hybrid mini-grid systems in Indonesia. In some studies, hybrid mini-grid systems with mini-hydro energy and wind energy are shown to have cost competitiveness against traditional diesel-based systems in places with rich resources (Nerini et al., 2016). However, this study looks into economic viability of hybrid mini-grid systems with solar energy, since solar energy is more widely available compared to hydro energy and wind energy, thus the results of the analyses could be applied to different communities, too. The economic viability assessment is performed first by comparing the levelized cost of energy (LCOE) of the hybrid systems with that of conventional diesel systems. LCOE is often used when evaluating national energy policies to assess the cost competitiveness of various generation sources. However, there are limitations to LCOE in assessing commercial project viability. Therefore, in order to accurately portray private sector decision-making, profitability indices, such as Net Present Values (NPV) and Internal Rate of Returns (IRR) are deployed to assess the economic viability of renewable hybrid mini-grid systems in Indonesia. Through the LOCE analysis, this paper shows how renewable hybrid mini-grid systems are becoming more economically viable option compared to traditional diesel-based systems in certain areas, and also to highlight how financial structure affects the economic viability of renewable hybrid mini-grid systems when compared to traditional diesel-based systems, which is the first contribution of this paper. The second contribution of this paper is to present the impact of financial structure on the economic viability of renewable hybrid mini-grid systems through the NPV and IRR analyses, which portray private sector decision-making more accurately. Lastly, as the final contribution of this paper, another original perspective is presented through further looking into how international aid (grant funding) could improve the economic viability of renewable hybrid mini-grid systems under different financial scenarios. Policymakers, funding agencies, and private investors seeking to invest in renewable hybrid mini-grid systems in Indonesia should find this information relevant.
In the remaining of the paper, section 2 introduces literature review on economic viability studies of renewable hybrid mini-grid systems, section 3 explains the analysis methods and the data and assumption used for the analysis, and section 4 presents results of the analysis. In section 4, first results of the optimal mini-grid systems are shown. Next, results of LCOE comparison between the hybrid system and the diesel system in three financing scenarios are presented, and lastly results of NPV and IRR analyses for the hybrid system are described, which show what kind of financing scenario would bring profit to private investors. Section 5 provides discussion and policy recommendations based on the results of this study, followed by conclusion.
The benefits of renewable hybrid mini-grid systems in providing access to electricity in a sustainable manner have been shown in various studies, such as in the study conducted by Chowdhury et al. (2015), focusing on solar energy based hybrid mini-grid systems in Bangladesh. As the prices of renewable energy technologies have decrease substantially in the recent period, the numbers of studies that look into the economic viability of renewable hybrid mini-grid systems as options for electrification purpose have also increased greatly. Adaramola et al. (2014) investigate the LCOE of a renewable hybrid mini-grid system with solar PV, wind, battery and diesel for a small community in Ghana that consists of 400 households, and further conduct sensitivity analysis for various inputs for the model so that the findings of the study can be applied to other locations in Ghana. Study conducted by Tsuanyo et al. (2015) models optimal renewable hybrid mini-grid system with solar PV and diesel generator to be used at the international institute of engineering in Burkina Faso. They show that LCOE of the renewable mini-grid system is lower than that of the diesel mini-grid system, especially because the project site has high electricity demand during daytime. These increasing numbers of studies for different locations are practically meaningful, which can help facilitating installation of renewable hybrid mini-grid systems for the purpose of electrification and decreasing dependence on conventional diesel systems. However, some papers quote LCOE for mini-grids or potential range for LCOE with minimal reference to the assumptions underlying the analyses (Tsuanyo et al., 2015; Li and Yu, 2016), additionally a lot of the papers' assumptions behind the analyses do not integrate local data. Because diesel generators and lead acid batteries have been in the market for a long time, their price have been remained rather steady, while the price of solar PV module and converter, two of the main components of renewable hybrid mini-grid systems have significantly decreased in recent years, as shown in Figure 2. And considering the price difference among countries as depicted in Figure 3, it is vitally important to employ local data for these economic viability assessments. Furthermore, some studies such as the one by Tao and Finenko (2016) point out the limitations of LCOE analysis in assessing commercial project viability, and conduct NPV, IRR, and payback period analyses to assess the economic viability of renewable energy technologies. The study conducted by Phuangpornpitak and Kumar (2007) also provides in-depth analysis of the renewable hybrid mini-grid systems with solar PV, wind, battery, and diesel that have been actually installed in the national parks in Thailand. They present the cost structure and the results of NPV, IRR, and payback period analyses of the systems, and show that one of the systems has positive NPV, while the other ones have negative NPV and IRR lower than the interest of the loan used for the investment (Phuangpornpitak and Kumar, 2007). These studies that conduct economic viability assessment of renewable hybrid mini-grid systems by deploying profitability indices to accurately portray private sector decision-making can provide great information to practitioners and policy makers, but the numbers of such studies are still not large. Furthermore, there are much less studies that examine how different financial scenarios with different debt/equity ratios could change the economic viability of renewable hybrid mini-grid systems in comparison with that of diesel mini-grid systems. Also, considering that a lot of the mini-grid systems have been installed utilizing international aid and government fund (discussed in detail in section 5), it would be valuable to investigate how different amount of grant aid affects the economic viability of the mini-grid systems under different financial scenarios. This paper addresses these points, and conducts the assessment of the economic viability of the renewable hybrid mini-grid systems in Indonesia with accurately portraying private sector decision-making process with reliable local data, and investigating how different financial scenarios affect the viability of the renewable hybrid mini-grid systems.
For the economic viability assessment, first LCOE of the optimally modeled renewable hybrid mini-grid system and diesel-based mini-grid system are calculated and compared to each other. Then, further economic viability analyses with NPV and IRR are conducted for the renewable hybrid mini-grid system. In this paper, renewable hybrid mini-grid systems with solar PV, batteries, and diesel generators are considered. When designing the optimal mini-grid systems, in order to increase the efficiency of the diesel generator and to prevent the common problem in engines called cylinder glazing, the operational range for diesel generator was set between 20–100%, and the lower limit on the state of charge (25%) was set for battery.
The project site affects the economic viability of the project by changing the cost of fuel, components, and availability of renewable resources. In this analysis, given its relatively low electrification ratio among islands in Indonesia, a generic village with 1, 475 people in 350 households in Nusa Tenggara Timur (NTT), which is located at latitude 10°47.7′ south and longitude 122°51.4′ east, is set as the assumed project site. However, sensitivity analysis is conducted for prices of components and fuel accounting for transportation costs, thus the outcome of the analysis could be applied to other locations with similar renewable resources. The optimal renewable hybrid mini-grid systems are designed utilizing HOMER simulation techniques based on the electricity demand profile, renewable resources and technical details, as well as the costs of components. Due to the lack of the actual ground measured solar irradiance data in the region, daily average solar irradiance data is used, which is obtained from NASA Surface meteorology and Solar Energy database based on the latitude and the longitude of the assumed project site (Figure 4). Since the solar irradiance data is daily average data, the hourly basis effect of clouds and rain are not taken in to account in this study. Assumption of the electricity demand profile shown in the Figure 5 is created based on the typical electrical appliances for remote villages in Indonesia (Blum, 2013) with day-to-day random variability of 10% and time-step variability (60 minutes) of 20%, and without any seasonal variability (details for the electricity demand profile are presented in Appendix). In this assumed electricity demand profile, the total average daily electricity demand at the village is 586.65 kWh, with a peak load of 124.39 kW.
The examined renewable hybrid mini-grid system consists of solar PV, batteries, converters, diesel generators, and grid networks. The latest data on average component prices and their operation and maintenance costs (O & M) for solar PV, batteries, converters, and grid networks in Indonesia were provided by the Ministry of Energy and Mineral Resources of the Republic of Indonesia in June, 2016 (MEMR, 2016). The given data did not include transportation costs for the components, which could drive up the prices to a great extent when projects are conducted in remote areas, thus sensitivity analysis with multipliers up to 1.5 are taken for the costs of solar PV, batteries, and converters. Multiplier 1.25 would be considered as a reference case for the assumed project site in the analysis, and multiplier 1.5 could be considered as a conservative view. The cost of diesel generators and their average O & M costs are assumed based on the data published by Lazard (2016). Residential fuel price in Indonesia in December 2015 (IEA, 2016) is used as the fuel price in the analysis, and again to take transportation cost into account, as a proxy to reflect the location-dependence differences, multipliers up to 2.73 are considered. The multipliers are calculated based on the analysis of PLN's official cost of electricity in the entire networks they serve, which provides: low cost (equivalent to the lowest official diesel price), medium (two times higher than the lowest cost) and high (2.73 times higher than the lowest cost). Medium (2) would be considered as a reference case for the assumed project. The costs of components and their replacement costs, O & M costs and fuel cost are summarized in Table 1.
Components | System Cost | O & M Cost | Replacement Cost |
Solar PV | US$2, 100/kW to US$3, 150/kW | US$19/yr/kW | - |
Batteries | US$180/unit to US$270/unit (1 kWh generic Lead Acid Battery) | US$10/yr/unit | US$150/unit to US$225/unit |
Converters | US$900/kW to US$1, 350/kW | US$7.8/yr/kW | US$800/kW to US$1, 200/kW |
Diesel Generators | US$650/kW | US$0.05/hr/kW | US$650/kW |
Transmission Infrastructure | US$2, 000/km | US$160/yr/km | - |
Fuel Cost | $0.5007/litter to $1.367/litter | - | - |
LCOE of the renewable hybrid mini-grid system and the diesel-based mini-grid system are calculated using:
LCOE=PkWh=∑25t=1(Capitalt+O&Mt+Fuelt)∗(1+r)−t∑25t=1kWh(1+r)−t | (1) |
where: PkWh = the constant lifetime remuneration to the supplier for electricity; kWh = the amount of electricity produced in kWh; (1+r)−t = the discount factor for year t (reflecting payments to capital); Capitalt = total capital construction costs in year t; O&Mt = operation and maintenance costs in year t; Fuelt = Fuel costs in year t.
The discount rate used in the analysis is based on weighted average cost of capital (WACC), which is calculated for the following three cases using cost of equity, cost of debt, and corporate tax in Indonesia: debt/equity ratio (D/E) of 4, 1, and 100% equity. WACC is calculated using:
WACC=EV×Re+DV×Rd(1−Cx) | (2) |
where: Re = cost of equity; Rd = cost of debt; E = market value of the firm's equity; D = market value of the firm's debt; V = E + D; Cx = corporate tax rate.
Cost of equity is calculated using:
Re=Rf+β×Rp | (3) |
where: Rf = risk free rate; β = beta (a statistical measure of the volatility); Rp = market risk premium.
The numbers used for the calculation of WACC is summarized in Table 2.
Risk Free Rate | 7.62% |
Market Risk Premium | 8.0% |
Beta | 0.84 |
Cost of Equity | 14.34% |
Cost of Debt | 12.27% |
Corporate Tax Rate | 25% |
Source: Created based on (Fernandez, 2016) and (Trading Economics, 2017). |
The NPV and IRR of the renewable hybrid mini-grid system in three financing scenarios will be calculated with the aforementioned D/E ratios and grant finance, in order to examine what kind of financial structure would make the renewable hybrid mini-grid systems projects economically viable from private investors' perspectives. NPV is the difference between the initial capital cost and the present value of all net cash outflows and inflows of a particular project. The following equation represents the NPV formula:
NPV=∑Tt=1Ct(1+r)t−Co | (4) |
where: Ct = net cash inflow for year t; Co = initial investment costs; r = discount rate.
A project with a positive NPV indicates that the project is economically viable, which brings net profit to the organization.
In order to judge economic viability of potential investments, comparison of IRR and cost of capital is also conducted. For the purpose of IRR and NPV, the revenue here is assumed to be solely from the sale of electricity without any subsidy at the price equivalent to the generation cost at the assumed project site, which is US$0.34/kWh (Rauch, 2014). In order to reflect the expected increase in global fuel prices in the future, which is indicated by the IEA (IEA, 2015b), the authors assume an annual increase of the generation cost by 3%. Considering that additional revenue from sources such as carbon market mechanisms and subsidies are not considered, the analysis of the economic viability could be perceived as a conservative view, because additional sources of revenue would only improve the project's financial position.
The WACC for the three different D/E ratios under the aforementioned cost of debt, cost of equity, and corporate tax rate in Indonesia are provided in Table 3.
D/E Ratio | WACC |
4 | 10.23% |
1 | 11.77% |
100% Equity | 14.34% |
Based on the assumed electricity demand profile, renewable resources, and technical details, costs of components and discount rates (WACC), the optimal mini-grid systems are designed for a generic village with 1, 475 people in 350 households in NTT, Indonesia, using HOMER simulation techniques.
Table 4 summarizes the components and LCOE of the optimal renewable hybrid mini-grid system and diesel mini-grid system in the reference case with WACC of 11.77%.
System | Composition | LCOE | |
Renewable Hybrid Mini-grid system | Solar PV | 60 kW | $0.57 |
Diesel Generator | 140 kW | ||
1 kwh Lead Acid Battery | 200 strings | ||
System Converter | 30 kW | ||
Transmission Infrastructure | 3.5 km | ||
Diesel Mini-grid system | Diesel Generator | 140 kW | $0.60 |
Diesel Generator | 30 kW | ||
Transmission Infrastructure | 3.5 km |
The result presented in Table 4 show that LCOE of the renewable hybrid mini-grid system is lower than that of the diesel mini-grid system. In this case, 60kw of Solar PV provides over 40% of the total generation to meet the total electricity demand, which cuts CO2 emission by approximately 66% over the 25-year lifetime of the system. Figure 6 shows the system diagrams of both systems. Figure 7 provides the cost breakdown of the renewable hybrid mini-grid system. Figure 8 shows an example of how the PV, the diesel generator and batteries could interact with each other to serve the village load in one day in June in the reference case. Since the electricity demand is given with day-to-day random variability of 10% and time-step variability (60 minutes) of 20%, the demand varies in different days, so as how the electricity is served.
The result of sensitivity analysis for LCOE of the renewable hybrid mini-grid system and the diesel system with different discount rate (WACC) is presented in Figure 9. The LCOE is presented in ranges in Figure 9: the lower bound is with components prices and fuel prices equivalent to that of mainland Indonesia. The reference case is with components prices and fuel prices of mainland multiplied by 1.25 and 2, respectively. The higher bound is with components prices and fuel prices of mainland multiplied by 1.5 and 2.73, respectively. As shown in Figure 9, since renewable hybrid mini-grid systems are more capital intensive, their LCOE increases at a higher rate compared to that of diesel mini-grid systems as the discount rate increases. The analysis shows that with high discount rates, LCOE of diesel systems can be lower than that of hybrid systems at the lower bond (the lowest component and fuel prices). However, in the reference cases, high operation cost of diesel mini-grid systems makes the LCOE of the renewable hybrid mini-grid system lower than that of the diesel system even under the most conservative case with 100% equity financing (WACC: 14.34%). These results clearly show that when project site is remote enough from the mainland, renewable hybrid mini-grid systems cost less than diesel mini-grid systems for electrification purposes providing 24-hour reliable electricity under the assumptions in this paper. However, the LCOE of the renewable hybrid mini-grid system is still above the generation cost in the assumed project site, which is US$0.34/kWh. In order to further examine the economic viability of renewable hybrid mini-grid systems, NPV and IRR analyses in three financing scenarios are conducted.
The analyses of NPV and IRR are conducted for the following three financing scenarios: D/E of 4, 1, and 100% equity, with grant finance ranging from 0% to 50% of the total cost of the project.
Figure 10 articulates the result of NPV analysis. It shows that the project is not economically viable when no grant finance is put in. However, if 35% of the total cost of the project is covered through grant finance, then all of the three scenarios become economically viable. The cost of capital under different financing scenarios has great influence on the NPV: with grant financing of 35%, the difference of NPV between the 100% equity scenario and the D/E of 4 scenario is around $90, 000.
Figure 11 presents the result of IRR analysis for the three financing scenarios. As shown in the Figure 11, the IRR of the project will be above the cost of capital under all three scenarios with grant financing of around 35%.
The NPV and IRR in the case with 35% grant financing is summarized in Table 5.
NPV | WACC | IRR | |
D/E: 4 | $131, 737 | 14.34% | 18% |
D/E: 1 | $92, 391 | 11.77% | |
100 Equity | $42, 827 | 10.23% |
The results have shown that although renewable hybrid mini-grid systems are less costly than diesel mini-grid systems in the remote areas, such as the assumed project site in Indonesia, 35% of the total cost of the project needs to be covered by other sources (such as grant finance) in order to make the hybrid systems attractive to private investors.
During fiscal years 2011–2015, renewable hybrid mini-grid systems have been installed in 22 sites in Indonesia utilizing international aid and government funds (MEMR, 2016). In order to achieve targeted electrification ratio in Indonesia, mobilizing private investment would be crucial. Although it is clear that renewable hybrid mini-grid systems not only reduce generation cost compared to prevailing diesel mini-grid systems, but also reduce environmental impact and provide electricity in a more sustainable manner, the LCOE, NPV and IRR analyses have shown that access to low cost financing could be one of the critical barriers to attracting private investment into renewable hybrid mini-grid projects. As clearly shown in the analysis, pure equity financing greatly reduces the economic viability of the investment compared to that of D/E of 4 or with some grant finance. Hence, enhancing access to low cost financing and reducing cost of capital are critical for further facilitating implementation of renewable hybrid mini-grid projects. For this purpose, establishing international aid funds that are specifically designated for facilitating deployment of renewable hybrid mini-grid systems would greatly improve access to finance and help build a proper enabling environment. Also, international aid and government funds can be marshaled to enhance the enabling environment and reduce the costs of capital by addressing key risks through means such as technical assistance that addresses non-economic barriers related to project transaction costs or the lack of local expertise. Another notable policy recommendation is utilization of green bonds. Noted for their risk-alleviating features and appeal to institutional and socially responsible investors, green bonds are gaining prominence in climate change and sustainable development finance frameworks around the globe. In the same manner that corporations, municipal governments, and supranational institutions provided nearly $27 billion to renewable energy projects in both developed and developing countries (Tolliver et al., 2019), national and municipal governments of Indonesia may benefit from the flexible payment schedules, credit enhancement techniques, leveraging options, and broad investor appeal by including green bonds in their finance schemes for renewable hybrid mini-grid projects.
In order to reduce the cost of capital for renewable hybrid mini-grid projects, key risks of the projects, such as revenue uncertainty, needs to be addressed. Revenues through sale of electricity would be the primary source of revenue for the renewable hybrid mini-grid projects. Uncertainty in expected revenue may further increase the cost of capital by increasing risk. Reducing risk by making use of a combination of public and private investments, including grants, economic development funds, as well as private sector and utility investment would be very important for both facilitating the deployment of renewable hybrid mini-grid systems and enhancing the enabling environment for private investments. For instance, private-public partnerships in the forms of cost sharing arrangements, pay-for-service contracts, and other forms may have great potential to cost-effectively leverage public investments and reduce risk for private investments.
This study assessed the economic viability of renewable hybrid mini-grid systems in Indonesia. By designing optimal mini-grid systems for the assumed project site and comparing the LCOE of the optimal renewable hybrid mini-grid system and diesel hybrid mini-grid system, this study found that the LCOE of renewable hybrid mini-grid systems are lower than that of diesel mini-grid systems in the assumed project site. In order to accurately portray private sector decision-making, adding to the LCOE analysis, NPV and IRR analyses have been conducted for the optimal renewable hybrid mini-grid system. The LOCE, NPV, and IRR analyses have shown that debt and equity ratio greatly affect the profitability of the renewable hybrid mini-grid projects, which means enhancing access to low cost financing is one of the critical factors for facilitating implementation of the renewable hybrid mini-grid projects. The analyses have also shown that grant finance that covers 35% of the total project cost would make the project economically viable even in the most conservative case with purely equity finance.
The results of this paper may also be applicable to other places that share similar characteristics such as remoteness and high fuel prices. The limitation of this study lies in the use of daily average solar irradiance data instead of the actual ground measured data. When the hourly-basis effects of rain and clouds are considered, the output of solar PV changes so as the optimal mini-grid system. Future studies on the economic viability assessment of renewable hybrid systems could make the assessment more accurate by employing the ground-measured data for solar irradiance.
Finally, based on the results of this study, the authors want to highlight the following point: creating proper enabling environment by improving access to low cost financing, placing proper regulations that minimize the risk related to the projects, and creating collaborative partnership by making use of a combination of public and private investments should be a major policy objective in order to facilitate deployment of renewable hybrid mini-grid systems and to achieve targeted electrification ratio in a sustainable manner.
This research was made possible by the kind support for data collection by Indonesia's Ministry of Energy and Mineral Resources. The authors would like to thank the anonymous peer reviewers for their helpful comments.
The authors declare no conflict of interest.
[1] |
Andersen TG, Bollerslev T, Diebold FX, et al. (2000) Exchange Rate Returns Standardized by Realized Volatility are (Nearly) Gaussian. Multinatl Financ J 4: 159–179. doi: 10.17578/4-3/4-2
![]() |
[2] |
Andersen TG, Bollerslev T, Diebold FX, et al. (2001) The distribution of realized stock return volatility. J Financ Econ 61: 43–76. doi: 10.1016/S0304-405X(01)00055-1
![]() |
[3] |
Andersen TG, Bollerslev T, Diebold FX (2007) Roughing it up: including jump components in measuring, modeling and forecasting asset return volatility. Rev Econ Stat 89: 701–720. doi: 10.1162/rest.89.4.701
![]() |
[4] | Balcilar M, Bouri E, Gupta R, et al. (2017) Can volume predict Bitcoin returns and volatility? A quantiles-based approach. Econ Model 64: 74–81. |
[5] | Bariviera AF, Basgall MJ, Hasperué W, et al. (2017) Some stylized facts of the Bitcoin market, Physica A: Stat Mechanics Appl 484: 82–90. |
[6] |
Barndorff-Nielsen OE, Shephard N (2004) Power and Bipower Variation with Stochastic Volatility and Jumps. J Financ Econom 2: 1–37. doi: 10.1093/jjfinec/nbh001
![]() |
[7] |
Blau BM (2017) Price dynamics and speculative trading in bitcoin. Res Int Bus Financ 41: 493–499. doi: 10.1016/j.ribaf.2017.05.010
![]() |
[8] | Boudt K, Cornelissen J, Payseur S, et al. (2017) high frequency: Tools for High frequency Data Analysis. R package version 0.5. Available from: https://CRAN.R-project.org/package=highfrequency. |
[9] | Bouri E, Azzi G, Dyhrberg AH (2017a) On the return-volatility relationship in the Bitcoin market around the price crash of 2013. Econ 11: 1–16. |
[10] | Bouri E, Molnár P, Azzi G, et al. (2017b) On the hedge and safe haven properties of Bitcoin: Is it really more than a diversifier? Financ Res Letters 20: 192–198. |
[11] | Bradbury D (2013) The problem with Bitcoin. Comput Fraud Security 2013: 5–8. |
[12] |
Brandvold M, Molnár P, Vagstad K, et al. (2015) Price discovery on Bitcoin exchanges. J Int Financ Mark, Inst Money 36: 18–35. doi: 10.1016/j.intfin.2015.02.010
![]() |
[13] | Cheah ET, Fry J (2015) Speculative bubbles in Bitcoin markets? An empirical investigation into the fundamental value of Bitcoin. Econ Lett 130: 32–36. |
[14] | Dwyer GP (2015) The economics of Bitcoin and similar private digital currencies. J Financ Stab 17: 81–91. |
[15] | Dyhrberg AH (2016) Hedging capabilities of Bitcoin. Is it the virtual gold? Financ Res Lett 16: 139–144. |
[16] |
Extance A (2015) Bitcoin and beyond. Nature 526: 21–23. doi: 10.1038/526021a
![]() |
[17] | Franzke C (2012) Predictability of extreme events in a nonlinear stochastic-dynamical model. Physical Rev E 85. |
[18] | Fritsch S, Guenther F (2016) neuralnet: Training of Neural Networks. R package version 1.33. Available online: https://CRAN.R-project.org/package=neuralnet. |
[19] | Hawkes AG, Oakes D (1974) A Cluster Process Representation of a Self-Exciting Process. J Appl Prob 11: 493–503. |
[20] | Hsieh WW (2009) Machine Learning Methods in the Environmental Sciences, Cambridge University Press, Cambridge, UK. |
[21] | Katsiampa P (2017) Volatility estimation for Bitcoin: A comparison of GARCH models. Econ Lett 158: 3–6. |
[22] | Kim YB, Kim JG, Kim W, et al. (2016) Predicting Fluctuations in Cryptocurrency Transactions Based on User Comments and Replies. PLoS ONE 11. |
[23] | Kristoufek L (2013) BitCoin meets Google Trends and Wikipedia: Quantifying the relationship between phenomena of the Internet era. Scientific rep 3. |
[24] | Kristoufek L (2015) What Are the Main Drivers of the Bitcoin Price? Evidence from Wavelet Coherence Analysis. PLoS One 10. |
[25] |
Urquhart A (2016) The inefficiency of Bitcoin. Econ Lett 148: 80–82. doi: 10.1016/j.econlet.2016.09.019
![]() |
[26] |
Urquhart A (2017) Price clustering in Bitcoin. Econ Lett 159: 145–148. doi: 10.1016/j.econlet.2017.07.035
![]() |
1. | Hassan Ali, Han Phoumin, Steven R. Weller, Beni Suryadi, Cost–Benefit Analysis of HELE and Subcritical Coal-Fired Electricity Generation Technologies in Southeast Asia, 2021, 13, 2071-1050, 1591, 10.3390/su13031591 | |
2. | Andrea Cabanero, Lars Nolting, Aaron Praktiknjo, Mini-Grids for the Sustainable Electrification of Rural Areas in Sub-Saharan Africa: Assessing the Potential of KeyMaker Models, 2020, 13, 1996-1073, 6350, 10.3390/en13236350 | |
3. | Hassan Ali, Han Phoumin, Steven R. Weller, Beni Suryadi, 2021, Chapter 6, 978-981-16-1999-1, 147, 10.1007/978-981-16-2000-3_6 | |
4. | Bin Mo, Zhenghui Li, Juan Meng, The dynamics of carbon on green energy equity investment: quantile-on-quantile and quantile coherency approaches, 2022, 29, 0944-1344, 5912, 10.1007/s11356-021-15647-y | |
5. | Susann Stritzke, Prem Jain, The Sustainability of Decentralised Renewable Energy Projects in Developing Countries: Learning Lessons from Zambia, 2021, 14, 1996-1073, 3757, 10.3390/en14133757 | |
6. | Rohit Pawar, Kelvy P. Dalsania, Anirbid Sircar, Kriti Yadav, Namrata Bist, Renewable energy hybridization: a comprehensive review of integration strategies for efficient and sustainable power generation, 2024, 1618-954X, 10.1007/s10098-024-02951-7 | |
7. | Alya Nurul Shafira, Subhan Petrana, Rahma Muthia, Widodo Wahyu Purwanto, Techno-economic analysis of a hybrid renewable energy system integrated with productive activities in an underdeveloped rural region of eastern Indonesia, 2023, 7, 2515-4230, 1247, 10.1093/ce/zkad068 | |
8. | Raynaldi P. Aulia, Evi Steelyana W, T.N. Mursitama, E. Sitepu, F.T. Basaria, A Study in Government Procurement System: Public-Private Partnership for Infrastructure in Energy Sector, 2023, 388, 2267-1242, 01014, 10.1051/e3sconf/202338801014 | |
9. | Théo Chamarande, Sandrine Mathy, Benoit Hingray, Designing policies to reduce the carbon footprint of mini-grids in Africa, 2025, 396, 03062619, 126296, 10.1016/j.apenergy.2025.126296 |
Components | System Cost | O & M Cost | Replacement Cost |
Solar PV | US$2, 100/kW to US$3, 150/kW | US$19/yr/kW | - |
Batteries | US$180/unit to US$270/unit (1 kWh generic Lead Acid Battery) | US$10/yr/unit | US$150/unit to US$225/unit |
Converters | US$900/kW to US$1, 350/kW | US$7.8/yr/kW | US$800/kW to US$1, 200/kW |
Diesel Generators | US$650/kW | US$0.05/hr/kW | US$650/kW |
Transmission Infrastructure | US$2, 000/km | US$160/yr/km | - |
Fuel Cost | $0.5007/litter to $1.367/litter | - | - |
Risk Free Rate | 7.62% |
Market Risk Premium | 8.0% |
Beta | 0.84 |
Cost of Equity | 14.34% |
Cost of Debt | 12.27% |
Corporate Tax Rate | 25% |
Source: Created based on (Fernandez, 2016) and (Trading Economics, 2017). |
D/E Ratio | WACC |
4 | 10.23% |
1 | 11.77% |
100% Equity | 14.34% |
System | Composition | LCOE | |
Renewable Hybrid Mini-grid system | Solar PV | 60 kW | $0.57 |
Diesel Generator | 140 kW | ||
1 kwh Lead Acid Battery | 200 strings | ||
System Converter | 30 kW | ||
Transmission Infrastructure | 3.5 km | ||
Diesel Mini-grid system | Diesel Generator | 140 kW | $0.60 |
Diesel Generator | 30 kW | ||
Transmission Infrastructure | 3.5 km |
NPV | WACC | IRR | |
D/E: 4 | $131, 737 | 14.34% | 18% |
D/E: 1 | $92, 391 | 11.77% | |
100 Equity | $42, 827 | 10.23% |
Components | System Cost | O & M Cost | Replacement Cost |
Solar PV | US$2, 100/kW to US$3, 150/kW | US$19/yr/kW | - |
Batteries | US$180/unit to US$270/unit (1 kWh generic Lead Acid Battery) | US$10/yr/unit | US$150/unit to US$225/unit |
Converters | US$900/kW to US$1, 350/kW | US$7.8/yr/kW | US$800/kW to US$1, 200/kW |
Diesel Generators | US$650/kW | US$0.05/hr/kW | US$650/kW |
Transmission Infrastructure | US$2, 000/km | US$160/yr/km | - |
Fuel Cost | $0.5007/litter to $1.367/litter | - | - |
Risk Free Rate | 7.62% |
Market Risk Premium | 8.0% |
Beta | 0.84 |
Cost of Equity | 14.34% |
Cost of Debt | 12.27% |
Corporate Tax Rate | 25% |
Source: Created based on (Fernandez, 2016) and (Trading Economics, 2017). |
D/E Ratio | WACC |
4 | 10.23% |
1 | 11.77% |
100% Equity | 14.34% |
System | Composition | LCOE | |
Renewable Hybrid Mini-grid system | Solar PV | 60 kW | $0.57 |
Diesel Generator | 140 kW | ||
1 kwh Lead Acid Battery | 200 strings | ||
System Converter | 30 kW | ||
Transmission Infrastructure | 3.5 km | ||
Diesel Mini-grid system | Diesel Generator | 140 kW | $0.60 |
Diesel Generator | 30 kW | ||
Transmission Infrastructure | 3.5 km |
NPV | WACC | IRR | |
D/E: 4 | $131, 737 | 14.34% | 18% |
D/E: 1 | $92, 391 | 11.77% | |
100 Equity | $42, 827 | 10.23% |