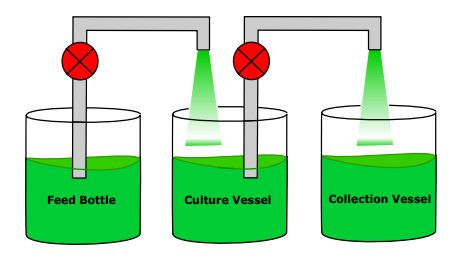
Patients with inflammatory bowel disease (IBD) often suffer from mood disorders and cognitive decline, which has prompted research into abnormalities in emotional brain regions and their functional analysis. However, most IBD studies only focus on single-modality neuroimaging technologies. Due to a limited spatiotemporal resolution, it is unfeasible to fully explore deep brain source activities and accurately evaluate the brain functional connectivity. Therefore, we propose an electroencephalography (EEG)-functional magnetic resonance imaging (fMRI)source imaging method based on an empirical mode diagram decomposition (EMDD) and performed a synchronous EEG-fMRI source imaging analysis on 21 IBD patients and 11 healthy subjects. The high-frequency spatial components of the fMRI were extracted through EMDD as prior constraints and compared with the EEG source imaging based on the entire fMRI spatial prior. Then, the cortical source time series were reconstructed according to the Desikan-Killiany atlas for an effective connectivity analysis. The results showed that the EEG-fMRI source imaging based on EMDD had a better performance, with the average log model evidence increased by 29.60% and the average explained variance increased by 19.12%. There were significant differences in the activation intensity of a series of abnormal brain regions between IBD patients and healthy controls, some of which were newly discovered: the uncus, claustrum, lentiform nucleus, and lingual gyrus. Moreover, the findings from the effective connectivity analysis of cortical source signals revealed that IBD patients had information flow loss in the frontal lobes, central areas, left parietal lobe, and right temporal lobe, and the information flow intensity of the right lingual gyrus was enhanced.
Citation: Yujie Kang, Wenjie Li, Jidong Lv, Ling Zou, Haifeng Shi, Wenjia Liu. Exploring brain dysfunction in IBD: A study of EEG-fMRI source imaging based on empirical mode diagram decomposition[J]. Mathematical Biosciences and Engineering, 2025, 22(4): 962-987. doi: 10.3934/mbe.2025035
[1] | Carlo Bianca . Differential equations frameworks and models for the physics of biological systems. AIMS Biophysics, 2024, 11(2): 234-238. doi: 10.3934/biophy.2024013 |
[2] | Carlo Bianca . Interplay and multiscale modeling of complex biological systems. AIMS Biophysics, 2022, 9(1): 56-60. doi: 10.3934/biophy.2022005 |
[3] | Carlo Bianca . Theoretical frameworks and models for biological systems. AIMS Biophysics, 2020, 7(3): 167-168. doi: 10.3934/biophy.2020013 |
[4] | Carlo Bianca . Mathematical and computational modeling of biological systems: advances and perspectives. AIMS Biophysics, 2021, 8(4): 318-321. doi: 10.3934/biophy.2021025 |
[5] | Bradley Vis, Jonathan J. Powell, Rachel E. Hewitt . Imaging flow cytometry methods for quantitative analysis of label-free crystalline silica particle interactions with immune cells. AIMS Biophysics, 2020, 7(3): 144-166. doi: 10.3934/biophy.2020012 |
[6] | Mario P. Carante, Francesca Ballarini . Modelling cell death for cancer hadrontherapy. AIMS Biophysics, 2017, 4(3): 465-490. doi: 10.3934/biophy.2017.3.465 |
[7] | Mehmet Yavuz, Fuat Usta . Importance of modelling and simulation in biophysical applications. AIMS Biophysics, 2023, 10(3): 258-262. doi: 10.3934/biophy.2023017 |
[8] | Domenico Lombardo, Pietro Calandra, Maria Teresa Caccamo, Salvatore Magazù, Luigi Pasqua, Mikhail A. Kiselev . Interdisciplinary approaches to the study of biological membranes. AIMS Biophysics, 2020, 7(4): 267-290. doi: 10.3934/biophy.2020020 |
[9] | Nor Afiqah Mohd Aris, Siti Suhana Jamaian . Dynamical analysis of fractional-order chemostat model. AIMS Biophysics, 2021, 8(2): 182-197. doi: 10.3934/biophy.2021014 |
[10] | Raghvendra P Singh, Guillaume Brysbaert, Marc F Lensink, Fabrizio Cleri, Ralf Blossey . Kinetic proofreading of chromatin remodeling: from gene activation to gene repression and back. AIMS Biophysics, 2015, 2(4): 398-411. doi: 10.3934/biophy.2015.4.398 |
Patients with inflammatory bowel disease (IBD) often suffer from mood disorders and cognitive decline, which has prompted research into abnormalities in emotional brain regions and their functional analysis. However, most IBD studies only focus on single-modality neuroimaging technologies. Due to a limited spatiotemporal resolution, it is unfeasible to fully explore deep brain source activities and accurately evaluate the brain functional connectivity. Therefore, we propose an electroencephalography (EEG)-functional magnetic resonance imaging (fMRI)source imaging method based on an empirical mode diagram decomposition (EMDD) and performed a synchronous EEG-fMRI source imaging analysis on 21 IBD patients and 11 healthy subjects. The high-frequency spatial components of the fMRI were extracted through EMDD as prior constraints and compared with the EEG source imaging based on the entire fMRI spatial prior. Then, the cortical source time series were reconstructed according to the Desikan-Killiany atlas for an effective connectivity analysis. The results showed that the EEG-fMRI source imaging based on EMDD had a better performance, with the average log model evidence increased by 29.60% and the average explained variance increased by 19.12%. There were significant differences in the activation intensity of a series of abnormal brain regions between IBD patients and healthy controls, some of which were newly discovered: the uncus, claustrum, lentiform nucleus, and lingual gyrus. Moreover, the findings from the effective connectivity analysis of cortical source signals revealed that IBD patients had information flow loss in the frontal lobes, central areas, left parietal lobe, and right temporal lobe, and the information flow intensity of the right lingual gyrus was enhanced.
The chemostat refers to a laboratory device used for the growth of micro-organisms in a culture environment [1,2], that has been regarded as an idealization of the nature to study microbial ecosystems in stationary stage [3]. It turned out to be an important investigation field due to a large number of applications, especially in waste water treatment [4,5] but also in ecological and environmental sciences (see [6,7,8,9,10]).
It is worth mentioning that the chemostat has been subject to a large number of scientific publications and books, not only in biology and ecology but also in mathematics. Indeed there exists a specific research area about the so-called "theory of the chemostat" [11,12] where many researchers have been involved in the last years. This interest has been strengthened by the fact that the chemostat device can be mathematically modeled in a simple way which reproduces quite faithfully real bio-processes.
Let us recall quickly in what consists the original chemostat device. It is composed of three tanks, the feed bottle, the culture vessel and the collection vessel, which are interconnected by pumps (see Figure 1). The substrate is stored in the feed bottle and provided with a given flow rate to the culture vessel, where interactions between nutrient and microbial biomass take place. The media from the culture vessel is also withdrawn towards the collection vessel with the same flow rate, to keep the volume in the culture vessel constant.
The mathematical model of the chemostat is given by the following dynamical system
dsdt=D(sin−s)−μ(s)x, | (1.1) |
dxdt=−Dx+μ(s)x, | (1.2) |
where s=s(t) and x=x(t) denote the concentration of substrate and species, respectively, sin is the input concentration of nutrient, D is the removal rate (also called input flow rate), and μ(⋅) is the specific growth function describing the kinetics of the nutrient consumption by the bacterial species. Here, we assume that the yield coefficient of the conversion of the substrate into biomass is equal to 1 (that is always possible to impose by a change of the unit of the biomass concentration). More specifically, we consider in the present work the Haldane growth function
μ(s)=μ0sks+s+s2ki,s≥0, | (1.3) |
where ks is the affinity constant and ki a parameter modeling the growth inhibition under large concentrations of substrate. Since it will be useful later, let us define the number
sm:=argmaxμ(s)=√kiks. | (1.4) |
Many works have been dedicated to this classical deterministic model of the chemostat (see for instance [11,12]) but most of time the removal rate D is kept constant, although it is well-known that in practice it is frequently subject to disturbances (see for instance [13] where some chronicles of time varying removal rates are depicted).
Motivated by this fact, it has been proposed to model the perturbations on the input flow rate in the chemostat model (1.1)–(1.2) by D+Φ(z∗(θtω)), where z∗(θtω) denotes the Ornstein-Uhlenbeck process (introduced in more detail in Section 2) and Φ is a bounded function defined as
Φ(z)=2dπarctan(z), | (1.5) |
where d>0 (see, for instance, [14,15] for other possible functions). Indeed, any odd function Φ which is increasing and such that limz→+∞Φ(z)=d can be considered.
In this way, once practitioners provide an interval [Dl,Dr]⊂R, 0<Dl<D<Dr<∞, (typically obtained from observations) we can define d=Dr−D=D−Dl and then the perturbed input flow is bounded for every time and any realization of the noise, i.e.,
Dl≤D+Φ(z∗(θtω))≤Dr∀t∈R. | (1.6) |
Then, the random chemostat model becomes
dsdt=(D+Φ(z∗(θtω)))(sin−s)−μ(s)x, | (1.7) |
dxdt=(D+Φ(z∗(θtω)))x+μ(s)x. | (1.8) |
One may wonder the reason why we consider this way of modeling bounded random fluctuations to perturb the input flow D in the model (1.1)–(1.2), instead of considering other stochastic process, such as for instance the well-known standard Wiener process. Indeed, this way has been typically used to model real perturbations and provides several advantages from both mathematical and biological points of view, see for instance [15].
On the one hand, this way of modeling noise fits in a loyal way the bounded variations of the input flow rate observed in real life. On the contrary, the Wiener process is unbounded with probability one which leads to arbitrary large (possibly negative) values of the corresponding perturbed input flow rate, which is not realistic at all from the biological point of view. We refer readers to [15,16] where the authors explain the relevant drawbacks found when perturbing the input flow in the classical deterministic chemostat (1.1)–(1.2) with a Wiener process (where μ is a Monod growth function).
Moreover, the approach proposed in this paper allows to prove the persistence of the bacterial species (under some conditions on the growth function), as it is observed by practitioners on very long time periods despite variations of the input flow rate. This is not the case when considering the Wiener process where persistence cannot be ensured (see [16] and [17] where the Wiener process is used to model disturbances on the input flow and environmental perturbations in the classical deterministic chemostat).
In industrial setup, large concentrations of the input substrate sin can be observed and it is also well-known that bacterial species may suffer from growth inhibition under very large concentrations s. The non-monotonic growth function (1.3) precisely models this fact (see [18]). Differently to the classical case, where the growth function μ is assumed to be increasing (as this is the case for the Monod function), the dynamics of the deterministic chemostat model with such non-monotonic growth function may exhibit a bi-stability for certain values of the dilution rate D (see for instance [11]). Depending on the initial condition, the state of the system converges asymptotically to the wash-out of the biomass (which is not a desirable state) or to a positive equilibrium. This kind of instability is observed in practice and present an issue in industrial applications because it requires a good monitoring of the system to detect if the state belongs the attraction basin of the wash-out equilibrium [19,20,21,22]. Most of the time, practitioners prefer to size the process to avoid such a behavior, i.e. such that the system admits an unique globally stable equilibrium (see also [23,24]). This mathematically amounts to have the following condition
D<min(μ(sin),μ(sm)) | (1.9) |
(this result is recalled later). The purpose of the present work is to study the behavior of the random dynamics when the constant removal rate is replaced by D+Φ(z∗(θtω)) where D satisfies condition (1.9).
The realizations of this variable may satisfy or not condition (1.9) at some times t. If not, one may wonder if this could lead the biomass to extinction. This question is of primer importance for the practitioners for the good health of the bio-process. In other words, for a nominal removal rate that satisfies condition (1.9), is the persistence of the biomass always guaranteed, even when the realizations of the noise provide effective values of the removal rate that do not satisfy this condition? Precise definitions of persistence in the framework of the chemostat will be given later.
Let us underline that stochastic modeling of the chemostat has received a great attention in the literature, considering different kinds of demographic noise [25,26,27,28,29,30], but few works have dealt with noise on the input, and much less in the case of considering Haldane consumption function, whereas this is quite natural for an open system as the chemostat, which is often the main source of fluctuations.
The paper is organized in the following way: in Section 2 we provide preliminaries and classical results about the deterministic chemostat model (1.1)–(1.2). In Section 3 we study the properties of the solutions of the random chemostat model with Haldane consumption kinetics (1.7)–(1.8). Then, in Section 4 we give conditions to ensure both uniform weak and strong persistence of the species. In Section 5, several simulations are presented to support the theoretical study. Finally, we present some conclusions in Section 6.
In this section we recall briefly some results that are useful in this paper. For the sake of clarity, we split this preliminary section in three different parts: the first one concerns classical results of the deterministic chemostat model (1.1)–(1.2), the second one is about the Ornstein-Uhlenbeck process and the third one recalls the definitions of persistence.
The next proposition recalls the classical results about the chemostat model (1.1)–(1.2) when μ is a non-monotonic function. We refer readers to [11,12] for proofs and more details.
Proposition 2.1. Assume that there exists ˆs∈(0,sin) such that the function μ is increasing on (0,ˆs) and decreasing on (ˆs,sin). Define the break-even concentrations λ−(D), λ+(D) as follows
λ−(D)=min{s∈[0,ˆs];μ(s)≥D},D∈[0,μ(ˆs)]λ+(D)=max{s∈[ˆs,sin];μ(s)≥D},D∈[μ(sin),μ(ˆs)] |
1. If D>μ(ˆs), the system (1.1)–(1.2) possesses a unique equilibrium E0:=(0,sin), which is globally asymptotically stable on R2+.
2. If D<μ(sin), the system (1.1)–(1.2) admits a unique positive equilibrium E−:=(sin−λ−(D),λ−(D)) which is globally asymptotically stable on R⋆+×R+.
3. If D∈[μ(sin),μ(ˆs)], the system (1.1)–(1.2) presents a bi-stability between E− and E0. From any initial condition in R⋆+×R+ excepted on a set of null measure, the solution converges asymptotically to E− or E0.
Remark 1. In practice, only the second case is desirable because it guarantees that in any situation the wash-out of the biomass is avoided.
Remark 2. For the Haldane expression (1.3), one has explicit expressions of the functions λ±
λ±(D)=(μ0−D)ki±√(μ0−D)2k2i−4D2kski2D. |
Let us recall that the concept of break-even concentrations has been revisited in the context of stochastic models of the chemostat [31,32] but we will not need it here. We keep the classical deterministic definition.
In the rest of the paper, we shall consider that we are in conditions of Proposition 2.1, that is μ non-monotonic on the interval [0,sin] (otherwise the analysis is similar to monotonic growth function and cannot present bi-stability). Throughout the paper we shall consider the following hypothesis
Assumption 1. There exists sm∈(0,sin) such that the function μ is increasing on (0,sm) and decreasing on (sm,sin).
We present here briefly the Ornstein-Uhlenbeck (OU) process. For more details we refer readers to [13,33,34].
The Ornstein-Uhlenbeck (OU) process is a stationary mean-reverting Gaussian stochastic process defined as
(t,ω)↦z(t,ω):=z∗(θtω)=−βν0∫−∞eβsθtω(s)ds,for allt∈R,ω∈Ω,β,ν>0, | (2.1) |
where ω denotes a standard Wiener process in a probability space (Ω,F,P), β is the mean reversion constant representing the strength with which the process is attracted by the mean, ν>0 is the volatility constant describing the variation or the size of the noise and θt denotes the usual Wiener shift flow given by
θtω(⋅)=ω(⋅+t)−ω(t),t∈R. |
We note that the OU process (2.1) can be obtained as the stationary solution of the Langevin equation
dz+βzdt=νdω. | (2.2) |
Typically, the OU process (2.1) can model the position of a particle by taking into account its friction in a fluid (which is the main difference with the typical standard Wiener process). Indeed, it can be considered as a generalization of the standard Wiener process and provides a link between the standard Wiener process (β=0, ν=1) and no noise at all (β=1, ν=0).
From now on we consider β and ν fixed and z∗(θtω) the OU process defined above.
We recall in the next proposition some of its properties.
Proposition 2.2 (See [35,36]). There exists a θt-invariant set ˜Ω∈F of Ω of full measure such that for ω∈˜Ω and β,ν>0, we have
(i) the random variable |z∗(ω)| is tempered with respect to {θt}t∈R, i.e., for a.e. ω∈˜Ω,
limt→∞e−ηtsupt∈R|z∗(θ−tω)|=0,for all η>0. |
(ii) this mapping is a stationary solution of (2.2) with continuous trajectories
(t,ω)→z∗(θtω)=−βν0∫−∞eβs(θtω)(s)ds; |
(iii) for any ω∈˜Ω one has:
limt→±∞|z∗(θtω)|t=0;limt→±∞1t∫t0z∗(θsω)ds=0; |
limt→±∞1t∫t0|z∗(θsω)|ds=E[|z∗|]<∞. |
We recall here the definitions of (uniform) persistence (see for instance [37]) that we consider in the present work.
Definition 2.1. The dynamics of (1.7)–(1.8) is strongly persistent if there exists ε>0 such that for any initial condition (s(0),x(0)) in R+×R⋆+ and any realization of the D+Φ(z∗(θ(⋅)ω)), the solution verifies
lim inft→+∞x(t)>ε. |
Definition 2.2. The dynamics of (1.7)–(1.8) is weakly persistent if there exists ε>0 such that for any initial condition (s(0),x(0)) in R+×R⋆+ and any realization of D+Φ(z∗(θ(⋅)ω)), the solution verifies
lim supt→+∞x(t)>ε. |
In this section we study the random chemostat model (1.7)–(1.8) presented in the introduction. We prove the existence and uniqueness of a global positive solution and provide results about the existence of absorbing and attracting sets which, in addition, are deterministic (i.e. that do not depend on the realization of the noise). In addition, we derive first conditions under which extinction of species cannot be avoided.
In the sequel, we denote X:={(s,x)∈R2:s,x≥0} for the positive cone.
Theorem 3.1. For any initial condition u(0)∈X, system (1.7)–(1.8) possesses a unique global solution u(t;0,ω,u(0))=(s(t;0,ω,u(0)),x(t;0,ω,u(0)))∈C1([0,+∞);X). In addition, it remains in X for every t>0.
Proof. Let us first write system (1.7)–(1.8) as
dudt=L(θtω)u+F(u,θtω), |
where
u=(sx),L(θtω)=(−(D+Φ(z∗(θtω)))00−(D+Φ(z∗(θtω)))) |
and
F(u,θtω)=((D+Φ(z∗(θtω)))sin−μ0sks+s+s2kixμ0sks+s+s2kix). |
F(⋅,θtω)∈C1(X×[0,+∞);X) whence F is locally Lipschitz respect to u∈X. Therefore, for each realization of the noise, du/dt=L(θtω)u+F(u,θtω) is an non-autonomous differential equation with a right member Lipschitz with respect to u and continuous with respect to t (recall that z∗(θtω) is continuous respect to t and Φ is also continuous). Therefore, the solution of the Cauchy problem admits an unique local solution of system (1.7)–(1.8) from the theory of ordinary differential equations.
Let us first show that both x and s remain in X for any u0∈X. To this end, we notice first that x(t)=0 solves (1.7). By uniqueness of the Cauchy problem, we deduce that any other solution is such that x(t)≠0 for any t. Notice also that one has, thanks to (1.6),
dsdt|s=0=(D+Φ(z∗(θtω)))sin>0, |
which proves that the axis s=0 is repulsive in X. This demonstrates the positiveness of the solution of system (1.7)–(1.8).
Now we prove that both the substrate and the microorganisms concentrations remain bounded for every time. To this end, define v(t):=s(t)+x(t). Then the variable v is solution of the following differential equation
dvdt=(D+Φ(z∗(θtω)))(sin−v) |
and, thanks to (1.6),
dvdt≤Drsin−Dlv, |
By comparison of solutions of scalar ODEs (see [38]) we obtain
v(t;0,ω,v(0))≤v(0)e−Dlt+DrDlsin(1−e−Dlt),t≥0. |
Then, v is forward bounded and since v=s+x≥0, we deduce that both the solution s and x are also bounded for positive time. The unique solution of system (1.7)–(1.8) is thus defined for any t≥0.
Now, we prove the existence of deterministic attracting and absorbing sets for the solutions of the random chemostat model (1.7)–(1.8).
Theorem 3.2. The system (1.7)–(1.8) possesses a deterministic (forward) attracting set
A:={(s,x)∈X:s+x=sin}. | (3.1) |
Proof. Define the variable q(t):=s(t)−sin+x(t). Then q satisfies the following differential equation
dqdt=−(D+Φ(z∗(θtω)))q |
whose solution is given by
q(t;0,ω,q(0))=q(0)e−∫t0(D+Φ(z∗(θsω)))ds. | (3.2) |
From (1.6), we have
q(0)e−Drt≤q(t;0,ω,q(0))≤q(0)e−Dlt |
and taking limit when t goes to infinity we have
limt→+∞q(t;0,ω,q(0))=0, |
which means that, for any ε>0, there exists T(ω,ε)>0 such that
q(t;0,ω,q(0))∈[−ε,ε],t≥T(ω,ε). |
This proves that
Bε:={(s,x)∈X:sin−ε≤s+x≤sin+ε}. |
is a (forward) deterministic absorbing set for system (1.7)–(1.8). As ε>0 is arbitrary, the proof is done.
Remark 3. Let us underline that the attracting set (3.1) obtained in Theorem 3.2 does not depend on the event ω∈Ω. This is another particularity that we obtain when considering this way of modeling random bounded realizations.
Our aim now is to provide first conditions on the parameters of system (1.7)–(1.8) under which extinction of the species occurs.
Theorem 3.3. Assume that the inequality
Dl>μ(sm) | (3.3) |
is fulfilled, where sm is defined in (1.4). Then, the singleton
Ae:={(sin,0)}⊂A |
is a (forward) attracting set.
Proof.
From the equation describing the dynamics of the species (1.8) and (1.6) one has
dxdt=−(D+Φ(z∗(θtω)))x+μ(s)x≤−(Dl−μ(sm))x. |
and by comparison of solutions of scalar ordinary differential equations [38], we have
x(t;0,ω,x(0))≤x(0)e−(Dl−μ(sm))t. | (3.4) |
Taking limit when t goes to infinity in (3.4), we obtain from (3.3) and Proposition 2.2 (ⅲ),
limt→+∞x(t;0,ω,x(0))=0. |
In this section our aim is to provide conditions to ensure persistence of the species in the sense defined in Section 2.3.
We shall distinguish in the following weak and strong persistence, as defined for instance in [37]. Before stating a result concerning the weak persistence of species, let us first provide a property linked to the choice of the function Φ.
Proposition 4.1. Let be Φ(z)=2dπarctan(z) defined as in (1.5). Then
limt→+∞1t∫t0Φ(z∗(θsω))ds=0,a.s. inΩ. | (4.1) |
Proof. Since Φ(z)=2dπarctan(z), then we have
∫Ω|2dπarctan(z∗(ω))|dP(ω)≤d|Ω|=d |
whence Φ(z∗(⋅))∈L1(Ω,F,P). Then, since P is invariant by θ (see [34,35]), from the Birkhoff ergodic theorem it yields that
limt→+∞1t∫t0Φ(z∗(θsω))ds=E[Φ(z∗(ω))],a.s. inΩ. |
Hence, it is enough to show that E[Φ(z∗(ω))]=0. In fact, we have
E[Φ(z∗(ω))]=∫RΦ(x)fOU(x)dx=0, |
where fOU denotes the density function of the random variable z∗(⋅), which is an even function since it is gaussian, and Φ is an odd function.
Remark 4. From Proposition 4.1 we notice that we could model random perturbations as in this paper, i.e., by means of Φ(z∗(θtω)), and the ergodic property (4.1) remains true as long as Φ is an odd measurable function.
Theorem 4.1. Assume that the inequality
μ(sin)>D. | (4.2) |
is fulfilled. Then, the random chemostat model (1.7)–(1.8) is weakly persistent, that is there exits ε>0 such that for any initial condition x(0)∈X, any realization satisfies
lim supt→+∞x(t;0,ω,x(0))≥ε. |
Proof. Let ε>0 be such that μ(s)>D for all s∈[sin−ε,sin+ε] and define
η:=min{μ(s)−D:s∈[sin−ε,sin+ε]}>0. |
From Theorem 3.2, we know that s(t;0,ω,s(0)) converges asymptotically to the set [0,sin]. Therefore, there exists T(ω,ε)>0 such that s(t;0,ω,s(0))<sin+ε for any t>T(ω,ε).
Consider now, for t>T(ω,ε), the sets
U(t):={τ∈[T(ω,ε),t]:s(τ;0,ω,s(0))<sin−ε},V(t):={τ∈[T(ω,ε),t]:s(τ;0,ω,s(0))∈[sin−ε,sin+ε]} |
and the functions in [0,1]
u(t):=measU(t)t−T(ω,ε),v(t):=measV(t)t−T(ω,ε)=1−u(t). |
Then, from (1.8), one can write the following inequality
˙x(t)≥{−(D+Φ(z∗(θtω)))x(t),t∈U(t)(η−Φ(z∗(θtω)))x(t),t∈V(t) |
and by integration between T(ω,ε) and t>T(ω,ε) one obtain
x(t)≥x(T(ω,ε))e(t−T(ω,ε))[u(t)(−D)+(1−u(t))η−1t−T(ω,ε)∫tT(ω,ε)Φ(z∗(θrω))dr],t>T(ω,ε). | (4.3) |
Assume that one has
limt→+∞u(t)=0. |
From Proposition 4.1, one has
limt→+∞e−1t−T(ω,ε)∫tT(ω,ε)Φ(z∗(θrω))dr=1 |
and then (4.3) gives
limt→+∞x(t)=+∞ |
which is a contradiction since x is bounded. We deduce thus that the sets U(⋅) are necessarily such that
measU(t)→+∞ when t→+∞ |
that is
meas({t:s(t)<sin−ε})=+∞ |
which shows that one has
lim inft→+∞s(t)≤sin−ε |
or equivalently
lim supt→+∞x(t)≥ε>0 |
since s(t)+x(t) converges to sin for any realization (see the proof of Theorem 3.2).
Remark 5. Theorem 4.1 proves the weak (uniform) persistence of species as long as D<μ(sin) is fulfilled, the same condition that guarantees the persistence in the deterministic case (see Proposition 2.1). However, let us underline that we do not impose the upper bound Dr of the variations of the removal rate to fulfill this inequality. This means that one could have realizations of the disturbances such that the effective value of the removal rate is above μ(sin) on large periods of time making the species to be arbitrary closed to the extinction but it will always persist.
In the next result we consider a stronger condition on the upper bound Dr of the removal rate which ensures the strong persistence of the species.
Theorem 4.2. Assume that the inequality
Dr<μ(sin) | (4.4) |
is fulfilled. Then, the random chemostat model (1.7)–(1.8) is strongly persistent and the set
Ap:={(x,y)∈X:s+x=sin,x≥sin−λ−(Dr)}⊂A |
is (forward) attracting, where the function λ− is defined in Proposition 2.1.
Proof. Take ε>0 such that μ(s)>Dr for any s∈[sin−ε,sin+ε] and posit
η:=min{μ(s)−Dr:s∈[sin−ε,sin+ε]}>0. |
From now on, we will s(t), x(t) and q(t) instead of s(t;0,ω,s(0)), x(t;0,ω,x(0)) and q(t;0,ω,q(0)) to make the readability easier even though we recall that every state variable depends on the noise.
From Theorem 3.2, we know that the variable q(t)=s(t)−sin+x(t) converges asymptotically to zero for any realization, and thus any solution s(t) of the random model (1.7)–(1.8) converges to the set [0,sin]. Consequently, there exists T(ω,ε)>0 such that
s(t)<sin+ε,q(t)>−κ,∀t>T(ω,ε), |
where
κ:=μ(sin−ε/2)−Drμ(sin−ε/2)(ε/2)>0. |
If s(t)∈[sin−ε,sin+ε] for any t>T(ω,ε), then one has, from (1.8), ˙x(t)>ηx(t) for any t>T(ω,ε), which implies that x is unbounded, thus a contradiction. We deduce that there exists a finite time ˉT(ω)≥T(ω,ε) such that s(ˉT(ω))≤sin−ε/2. On another hand, from (1.7), s(t) can be written as the solution of the non-autonomous dynamics
dσdt=F(t,σ):=(D+Φ(z∗(θtω))−μ(σ))(sin−σ)−μ(σ)q(t). | (4.5) |
Note that one has
F(t,sin−ε/2)≤(Dr−μ(sin−ε/2))(ε/2)−μ(sin−ε/2)q(t) |
and then
F(t,sin−ε/2)≤(Dr−μ(sin−ε/2))(ε/2)+μ(sin−ε/2)κ=0,t>T(ω,ε) |
(from the definition of κ). We deduce that the set [0,sin−ε/2] is forward invariant for the semi-flow {˙σ=F(t,σ),t>T(ω,ε)}. Therefore, one has
s(t)≤sin−ε/2,t>ˉT(ω). |
Then, once identified σ with s, from (4.5)
dsdt≤(Dr−μ(s))(sin−s)−μ(s)q(t),t>ˉT(ω) |
and from the comparison of solutions of scalar ordinary differential equations [38], one has the inequality s(t)≤s+(t) for any t>ˉT(ω), where s+(t) is solution of the Cauchy problem
ds+dt=(Dr−μ(s+))(sin−s+)−μ(s+)q(t),s+(ˉT(ω))=s(ˉT(ω)). |
Note that the solution s+(t) belongs to the interval [0,sin] for any t≥ˉT(ω) (and is thus bounded) and that its dynamics is asymptotic autonomous with limiting dynamics
ds†dt=(Dr−μ(s†))(sin−s†) | (4.6) |
Under assumption Dr<μ(sin), one has necessarily λ−(Dr)<sin and the property
(Dr−μ(σ))(λ−(Dr)−σ)>0,∀σ∈[0,sin]∖{λ−(Dr)} |
is fulfilled. One finally obtains that any solution of (4.6) in [0,sin] is such that s†(t)→λ−(Dr) when t→+∞. From the theory of asymptotically autonomous dynamical systems [39], one concludes that s+(t) converges also to λ−(Dr) when t→+∞, which proves that one has
lim supt→+∞s(t)≤λ−(Dr) |
or equivalently
lim inft→+∞x(t)≥sin−λ−(Dr)>0 |
since s(t)+x(t) converges to sin for any realization. This demonstrates the strong persistence of the random dynamics (1.7)–(1.8) with the explicit lower bound sin−λ−(Dr).
Now we consider the last situation when μ(sin)<D<μ(sm) which corresponds to the bi-stability in the deterministic case. In the random framework, one cannot guarantee persistence nor wash-out of the biomass. As we shall see later on simulations, the asymptotic behavior of the solutions depends on the initial condition and the realization of the noise. However we show that an upper bound on the biomass can be provided.
Theorem 4.3. Assume x(0)>0. Then, the upper bound
lim supt→+∞x(t;0,ω,x(0))≤sin−λ−(Dl) |
for any solution of the random dynamics (1.7)–(1.8) as long as Dl<μ(sm).
Proof. Let us remark that in this proof we will write x(t), s(t) and q(t), or simply x, s and q, instead of x(t;0,ω,x(0)), s(t;0,ω,s(0)) and q(t;0,ω,q(0)) for the sake of simplicity.
Consider x(0)>0. Then, from (1.8) one has
dxdt=(μ(s)−(D+Φ(z∗(θtω))))x=(μ(sin−x−q)−(D+Φ(z∗(θtω))))x:=F(t,x), | (4.7) |
where q=sin−x−s.
We know that limt→+∞q(t)=0 then, for every ε>0 there exists T(ω,ε)>0 such that |q(t)|<ε for every t>T(ω,ε). Thus x(t)<sin+ε for every t>T(ω,ε).
Moreover, for all x∈(sin−λ−(Dl)+ε,sin+ε), one has sin−x−q∈(−q−ε,λ−(Dl)−q−ε). In fact, since sin−x−q=s≥0 (see Theorem 3.1), then we have sin−x−q∈(0,λ−(Dl)−q−ε)⊂(0,λ−(Dl)) whence F(t,x)<0 since μ(0,λ−(Dl))<Dl and D+Φ(z∗(θtω))≥Dl.
Thus,
lim supt→+∞x(t)≤sin−λ−(Dl)+ε |
for every ε>0.
In this section we present several numerical simulations to support our theoretical results in three different cases: extinction, strong persistence and weak persistence of species.
Two different figures are presented in each case. The first figure (with two panels) concerns the dynamics of the substrate (top) and the species (bottom) in the random chemostat model (1.7)–(1.8) for different realizations of the noise (in colored continuous lines) along with the case without noise i.e. the deterministic chemostat model (in blue dashed lines). In addition, in each panel we display a little box with a zoom to illustrate better the dynamics around the attracting set. The second figure offers information about the consumption function of the consumer species and those parameters involved in conditions to have extinction, weak persistence and strong persistence of species.
We first present the case of extinction in Figure 2. We consider s(0)=14, x(0)=5 as initial conditions and set sin=14, μ0=4, ks=7.5, ki=4, D=1.4 and the interval given by practitioners from observations is [Dl,Dr]=[1.15,1.65], then d=0.25. In addition, ν=1 and β=1. We can observe in Figure 3 that Dl>μ(sm) holds true and then, as proved in Theorem 3.3, the species extinguishes (the attracting set in this case is {(0,sin)}).
This is indeed quite intuitive as condition (3.3) basically means that the nominal value D of the removal rate is too large large compared to the growth kinetics (recall that Dl>μ(sm) implies D>μ(sm)), even though we start far away from the washout (sin,0).
Now we present situations where weak and strong persistence is ensured. To compare, we start with the case of weak persistence by considering s(0)=16.7, x(0)=0.01 as initial conditions and set sin=16.7, μ0=7, ks=7, ki=7. In addition, β=1 and ν=1. Once fixed these parameters, the removal rate D will be the set to allow conditions (4.4) and (4.2) be true or false.
In Figure 4 we set D=1.7 and the interval [Dl,Dr]=[1.45,1.95] then d=0.25. In this case D<μ(sin) is fulfilled (observe Figure 5) then we have weak persistence of species, see Theorem 4.1. Although we start very closed to the washout (sin,0), we observe persistence of species.
In Figure 6 we set D=1.4 and the interval [Dl,Dr]=[1.15,1.65] then d=0.25. In this case Dr<μ(sin) holds true (see Figure 10) and then strong persistence of species is obtained from Theorem 4.1.
Moreover, we would like to notice that OU process proves again to be a powerful tool when modeling real (bounded) noises. In addition, it allows us to observe clearly the difference between weak and strong persistence.
Finally we present some numerical simulations to observe that both extinction and persistence can be obtained when Dl<μ(sin)<D is fulfilled, as in the deterministic case. However, the issue here depends also on the realization of the noise.
We have considered the chemostat model (1.1)–(1.2) with Haldane consumption kinetics, under bounded perturbations on the input flow rate, motivated by real cases in industrial setup and biotechnology. To this end, we use a bounded saturated function of the Ornstein-Uhlenbeck process that allows us to ensure the noise to be bounded in realistic intervals.
We prove in Theorem 3.1 existence and uniqueness of global positive solution of the corresponding random chemostat (1.7)–(1.8) by means of standard results from the theory of ODEs and thanks to properties of the Ornstein-Uhlenbeck process. Then, in Theorem 3.2 we establish the existence of an absorbing and attracting set which has the nice property to be deterministic, i.e. that does not depend on the realization of the noise.
We then focused on the long-time behavior of the random dynamics inside this attracting set. To this end, we first proved in Theorem 3.3 that extinction of species cannot be avoided as long as Dl>μ(sm) whatever is the input concentration sin. On the opposite, we proved in Theorem 4.1 the weakly uniform persistence of species when D<μ(sin), which means that species can be temporarily arbitrary closed to the extinction but still persist even when having random disturbances in the input flow. The condition D<μ(sin) ensures persistence in the deterministic case but the effective removal rate does not necessarily fulfill this condition depending on the realizations. Finally, we prove the strong persistence of the species in Theorem 4.2 under the stronger condition Dr<μ(sin). In this case, we provide an explicit lower bound for the asymptotic concentration of the species, a useful information for practitioners.
In addition, we support the theoretical results with several numerical simulations which depict the possible behaviors of the random dynamics. Moreover, this allows us to illustrate the difference between weak and strong persistence: once fixed every parameter we change the removal rate D to let conditions μ(sin)>D and μ(sin)>Dr be true or not. Condition to have weak persistence (μ(sin)>D) does not involve the noise and then the random realizations of the species can fluctuate closed to zero and we still ensure persistence whereas condition to have strong persistence (μ(sin)>Dr) only fulfills for realizations of the perturbed removal rate inside a small enough interval.
To conclude, we would like to stress that the Ornstein-Uhlenbeck process has proved once again to be relevant tool to model random disturbances in a biological framework. This stochastic process fits in a quite loyal way the bounded fluctuations that are observed in practice, and justify the (weak or strong) persistence of the biomass despite the possible realizations of noise, as also observed in practice.
This work has been partially supported by Proyecto de Excelencia P12-FQM-1492 from Junta de Andalucía, Project PGC2018-096540-B-I00 from Ministerio de Ciencia, Innovación y Universidades (Spanish government) and Project US-1254251 from Consejería de Economía y Conocimiento (Junta de Andalucía).
The authors declare there is no conflict of interests.
[1] |
T. H. Taft, A. Bedell, M. R. Craven, L. Guadagnoli, S. Quinton, S. B. Hanauer, Initial assessment of post-traumatic stress in a US cohort of inflammatory bowel disease patients, Inflammatory Bowel Dis., 25 (2019), 1577–1585. https://doi.org/10.1093/ibd/izz032 doi: 10.1093/ibd/izz032
![]() |
[2] |
T. H. Bisgaard, K. H. Allin, L. Keefer, A. N. Ananthakrishnan, T. Jess, Depression and anxiety in inflammatory bowel disease: epidemiology, mechanisms and treatment, Nat. Rev. Gastroenterol. Hepatol., 19 (2022), 717–726. https://doi.org/10.1038/s41575-022-00634-6 doi: 10.1038/s41575-022-00634-6
![]() |
[3] |
A. K. Thomann, M. M. Schmitgen, D. Kmuche, M. P. Ebert, P. A. Thomann, K. Szabo, et al., Exploring joint patterns of brain structure and function in inflammatory bowel diseases using multimodal data fusion, Neurogastroenterol. Motil., 33 (2021), e14078. https://doi.org/10.1111/nmo.14078 doi: 10.1111/nmo.14078
![]() |
[4] |
G. Thapaliya, S. Eldeghaidy, S. J. Radford, S. T. Francis, G. W. Moran, An examination of resting-state functional connectivity in patients with active Crohn's disease, Front. Neurosci., 17 (2023), 1265815. https://doi.org/10.3389/fnins.2023.1265815 doi: 10.3389/fnins.2023.1265815
![]() |
[5] |
U. A. Kelleci, T. Calhan, A. Sahin, Z. A. Ozemir, R. Kahraman, K. Ozdil, et al., Electroencephalography findings in Crohn's disease, Clin. EEG Neurosci., 50 (2019), 129–133. https://doi.org/10.1177/1550059418767589 doi: 10.1177/1550059418767589
![]() |
[6] |
A. Kibleur, S. Pellissier, V. Sinniger, J. Robert, E. Gronlier, D. Clarencon, et al., Electroencephalographic correlates of low-frequency vagus nerve stimulation therapy for Crohn's disease, Clin. Neurophysiol., 129 (2018), 1041–1046. https://doi.org/10.1016/j.clinph.2018.02.127 doi: 10.1016/j.clinph.2018.02.127
![]() |
[7] |
Z. Fang, E. Lynn, M. Huc, S. Fogel, V. J. Knott, N. Jaworska, Simultaneous EEG+fMRI study of brain activity during an emotional Stroop task in individuals in remission from depression, Cortex, 155 (2022), 237–250. https://doi.org/10.1016/j.cortex.2022.07.010 doi: 10.1016/j.cortex.2022.07.010
![]() |
[8] |
Y. Yang, S. Luo, W. Wang, X. Gao, X. Yao, T. Wu, From bench to bedside: Overview of magnetoencephalography in basic principle, signal processing, source localization and clinical applications, NeuroImage Clin., 42 (2024), 103608. https://doi.org/10.1016/j.nicl.2024.103608 doi: 10.1016/j.nicl.2024.103608
![]() |
[9] |
K. Yu, X. Niu, B. He, Neuromodulation management of chronic neuropathic pain in the central nervous system, Adv. Funct. Mater., 30 (2020), 1908999. https://doi.org/10.1002/adfm.201908999 doi: 10.1002/adfm.201908999
![]() |
[10] |
A. Seeland, M. M. Krell, S. Straube, E. A. Kirchner, Empirical comparison of distributed source localization methods for single-trial detection of movement preparation, Front. Hum. Neurosci., 12 (2018), 340. https://doi.org/10.3389/fnhum.2018.00340 doi: 10.3389/fnhum.2018.00340
![]() |
[11] |
R. Coben, D. C. Hammond, M. Arns, 19 channel z-score and LORETA neurofeedback: Does the evidence support the hype, Appl. Psychophysiol. Biofeedback, 44 (2019), 1–8. https://doi.org/10.1007/s10484-018-9420-6 doi: 10.1007/s10484-018-9420-6
![]() |
[12] | C. Wei, K. Lou, Z. Wang, M. Zhao, D. Mantini, Q. Liu, Edge sparse basis network: A deep learning framework for EEG source localization, in 2021 International Joint Conference on Neural Networks (IJCNN), (2021), 1–8. https://doi.org/10.1109/IJCNN52387.2021.9533968 |
[13] |
Z. Jiang, Y. Liu, W. Li, Y. Dai, L. Zou, Integration of simultaneous fMRI and EEG source localization in emotional decision problems, Behav. Brain Res., 448 (2023), 114445. https://doi.org/10.1016/j.bbr.2023.114445 doi: 10.1016/j.bbr.2023.114445
![]() |
[14] |
S. M. Sadjadi, E. Ebrahimzadeh, M. Shams, M. Seraji, H. S. Zadeh, Localization of epileptic foci based on simultaneous EEG–fMRI data, Front. Neurol., 12 (2021), 645594. https://doi.org/10.3389/fneur.2021.645594 doi: 10.3389/fneur.2021.645594
![]() |
[15] |
N. Moradi, B. G. Goodyear, R. C. Sotero, Deep EEG source localization via EMD-based fMRI high spatial frequency, Plos One, 19 (2024), e0299284. https://doi.org/10.137/journal.pone.0299284 doi: 10.137/journal.pone.0299284
![]() |
[16] |
J. Riffi, A. M. Mahraz, A. Abbad, H. Tairi, 3D extension of the fast and adaptive bidimensional empirical mode decomposition, Multidimens. Syst. Signal Process., 26 (2015), 823–834. https://doi.org/10.1007/s11045-014-0283-6 doi: 10.1007/s11045-014-0283-6
![]() |
[17] |
T. Nguyen, T. Zhou, T. Potter, L. Zou, Y. Zhang, The cortical network of emotion regulation: Insights from advanced EEG-fMRI integration analysis, IEEE Trans. Med. Imaging, 38 (2019), 2423–2433. https://doi.org/10.1109/TML.2019.2900978 doi: 10.1109/TML.2019.2900978
![]() |
[18] |
B. Wang, A. D. N. Initiative, Enhanced brain efficiency network by integrating the new causality with fMRI and its application for Alzheimer's disease study, Biomed. Signal Process. Control, 86 (2023), 105364. https://doi.org/10.1016/j.bspc.2023.105364 doi: 10.1016/j.bspc.2023.105364
![]() |
[19] |
S. N. Kalburgi, T. Kleinert, D. Aryan, K. Nash, B. Schiller, T. Koenig, MICROSTATELAB: The EEGLAB Toolbox for resting-state Microstate Analysis, Brain Topogr., 37 (2023), 621–645. https://doi.org/10.1007/s10548-023-01003-5 doi: 10.1007/s10548-023-01003-5
![]() |
[20] |
M. Amann, M. Andelova, A. Pfister, N. M. Lenke, S. Traud, J. Reinhardt, et al., Subcortical brain segmentation of two dimensional T1-weighted data sets with FMRIB's Integrated Registration and Segmentation Tool (FIRST), NeuroImage Clin., 7 (2015), 43–52. https://doi.org/10.1016/j.nicl.2014.11.010 doi: 10.1016/j.nicl.2014.11.010
![]() |
[21] | R. K. Niazy, G. Iannetti, C. F. Beckmann, M. Brady, S. M. Smith, Improved FMRI artifact reduction from simultaneously acquired EEG data using slice dependant template matching, NeuroReport, 80 (1999). |
[22] |
C. Yan, X. Wang, X. Zuo, Y. Zang, DPABI: Data processing & analysis for (resting-state) brain imaging, Neuroinformatics, 14 (2016), 339–351. https://doi.org/10.1007/s12021-016-9299-4 doi: 10.1007/s12021-016-9299-4
![]() |
[23] |
A. Agosstini, F. Benuzzi, D. Ballotta, F. Rizzello, P. Gionchetti, N. Filippini, Differential brain structural and functional patterns in Crohn's disease patients are associated with different disease stages, Inflammatory Bowel Dis., 29 (2023), 1297–1305. https://doi.org/10.1093/ibd/izad029 doi: 10.1093/ibd/izad029
![]() |
[24] |
J. Deng, J. Sun, S. Lu, K. Yue, W. Li, H. Shi, et al., Exploring neural activity in inflammatory bowel diseases using functional connectivity and DKI-fMRI fusion, Behav. Brain Res., 443 (2023), 114325. https://doi.org/10.1016/j.bbr.2023.114325 doi: 10.1016/j.bbr.2023.114325
![]() |
[25] |
K. Friston, L. Harrison, J. Daunizeau, S. Kiebel, C. Phillips, N. T. Barreto, et al., Multiple sparse priors for the M/EEG inverse problem, NeuroImage, 39 (2008), 1104–1120. https://doi.org/10.1016/j.neuroimage.2007.09.048 doi: 10.1016/j.neuroimage.2007.09.048
![]() |
[26] | X. Lei, Electromagnetic brain imaging based on standardized resting-state networks, in 2012 5th International Conference on BioMedical Engineering and Informatics, (2012), 40–44. https://doi.org/10.1109.BMEI.2012.6512901 |
[27] |
K. Friston, J. Mattout, N. T. Barreto, J. Ashhburner, W. Penny, Variational free energy and the Laplace approximation, NeuroImage, 34 (2007), 220–234. https://doi.org/10.1016/j.neuroimage.2006.08.035 doi: 10.1016/j.neuroimage.2006.08.035
![]() |
[28] |
N. J. T. Barreto, E. A. Vazquez, W. D. Penny, Bayesian M/EEG source reconstruction with spatio-temporal priors, NeuroImage, 39 (2008), 318–335. https://doi.org/10.1016/j.neuroimage.2007.07.062 doi: 10.1016/j.neuroimage.2007.07.062
![]() |
[29] |
R. N. Henson, D. G. Wakeman, V. Litvak, K. J. Friston, A parametric empirical Bayesian framework for the EEG/MEG inverse problem: generative models for multi-subject and multi-modal integration, Front. Hum Neurosci., 5 (2011), 76. https://doi.org/10.3389/fnhum.2011.00076 doi: 10.3389/fnhum.2011.00076
![]() |
[30] |
X. Geng, X. Fan, Y. Zhong, M. F. Casanova, E. M. Sokhadze, X. Li, et al., Abnormalities of EEG functional connectivity and effective connectivity in children with autism spectrum disorder, Brain Sci., 13 (2023), 130. https://doi.org/10.3390/brainsci13010130 doi: 10.3390/brainsci13010130
![]() |
[31] |
J. Vorwerk, C. H. Wolters, D. Baumgarten, Global sensitivity of EEG source analysis to tissue conductivity uncertainties, Front. Hum. Neurosci., 18 (2024), 1335212. https://doi.org/10.3389/fnhum.2024.1335212 doi: 10.3389/fnhum.2024.1335212
![]() |
[32] |
S. J. Vogrin, C. Plummer, EEG source imaging—clinical considerations for EEG acquisition and signal processing for improved temporo-spatial resolution, J. Clin. Neurophysiol., 41 (2024), 8–18. https://doi.org/10.1097/WNP.0000000000001023 doi: 10.1097/WNP.0000000000001023
![]() |
[33] |
G. Lin, A. Lin, Y. Mi, D. Gu, Measurement of information transfer based on phase increment transfer entropy, Chaos Soliton. Fract., 174 (2023), 113864. https://doi.org/10.1016/j.chaos.2023.113864 doi: 10.1016/j.chaos.2023.113864
![]() |
[34] |
G. Chiarion, L. Sparacino, Y. Antonacci, L. Faes, L. Mesin, Connectivity analysis in EEG data: A tutorial review of the state of the art and emerging trends, Bioengineering, 10 (2023), 372. https://doi.org/10.3390/bioengineering10030372 doi: 10.3390/bioengineering10030372
![]() |
[35] |
A. Ekhlasi, A. M. Nasrabadi, M. Mohammadi, Classification of the children with ADHD and healthy children based on the directed phase transfer entropy of EEG signals, Front. Biomed. Technol., 8 (2021). https://doi.org/10.18502/fbt.v8i2.6515 doi: 10.18502/fbt.v8i2.6515
![]() |
[36] |
C. Jao, C. I. Lau, L. Lien, Y. Tsai, K. Chu, C. Hsiao, et al., Using fractal dimension analysis with the Desikan–Killiany atlas to assess the effects of normal aging on subregional cortex alterations in adulthood, Brain Sci., 11 (2021), 107. https://doi.org/10.3390/brainsci11010107 doi: 10.3390/brainsci11010107
![]() |
[37] |
A. Z. Snyder, Intrinsic brain activity and resting state networks, Neurosci. in the 21st century, 2022 (2022), 1939–1990. https://doi.org/10.1007/978-3-030-88832-9_133 doi: 10.1007/978-3-030-88832-9_133
![]() |
[38] |
R. Abreu, J. F. Soares, A. C. Lima, L. Sousa, S. Batista, M. C. Branco, et al., Optimizing EEG source reconstruction with concurrent fMRI-derived spatial priors, Brain Topogr., 35 (2022), 282–301. https://doi.org/10.1007/s10548-022-00891-3 doi: 10.1007/s10548-022-00891-3
![]() |
[39] |
A. Ojeda, K. K. Delgado, T. Mullen, Fast and robust block-sparse Bayesian learning for EEG source imaging, NeuroImage, 174 (2018), 449–462. https://doi.org/10.1016/j.neuroimage.2018.03.048 doi: 10.1016/j.neuroimage.2018.03.048
![]() |
[40] |
X. Lei, P. Xu, C. Luo, J. Zhao, D. Zhou, D. Yao, fMRI functional networks for EEG source imaging, Hum. Brain Mapp., 32 (2011), 1141–1160. https://doi.org/10.1002/hbm.21098 doi: 10.1002/hbm.21098
![]() |
[41] |
S. Yang, M. B. Reveret, Y. J. Choo, M. C. Chang, Association between chronic pain and alterations in the mesolimbic dopaminergic system, Brain Sci., 10 (2020), 701. https://doi.org/10.3390/brainsci10100701 doi: 10.3390/brainsci10100701
![]() |
[42] |
Y. S. Liaw, G. J. Augustine, The claustrum and consciousness: An update, Int. J. Clin. Health Psychol., 23 (2023), 100405. https://doi.org/10.1016/j.ijchp.2023.100405 doi: 10.1016/j.ijchp.2023.100405
![]() |
[43] |
D. Zhong, K. Jin, R. Wang, B. Chen, J. Zhang, C. Ren, et al., Microalgae‐based hydrogel for inflammatory bowel disease and its associated anxiety and depression, Adv. Mater., 36 (2024), 2312275. https://doi.org/10.1002/adma.202312275 doi: 10.1002/adma.202312275
![]() |
[44] |
Y. Yang, T. Wei, W. Yang, S. Hu, H. Jiang, W. Dong, et al., Dysfunction of the lenticular nucleus is associated with dystonia in Wilson's disease, Brain Sci., 13 (2022), 7. https://doi.org/10.3390/brainsci13010007 doi: 10.3390/brainsci13010007
![]() |
[45] |
B. C. Duchesne, L. T. Stike, G. I. Zubicaray, K. L. McMahon, P. M. Thompson, I. B. Hickie, et al., Lingual gyrus surface area is associated with anxiety-depression severity in young adults: A genetic clustering approach, Eneuro, 5 (2018). https://doi.org/10.1523/ENEURO.0153-17.2017 doi: 10.1523/ENEURO.0153-17.2017
![]() |
[46] |
C. Koutsarnakis, S. Komaitis, E. Drosos, A. V. Kalyvas, G. P. Skandalakis, F. Liakos, et al., Mapping the superficial morphology of the occipital lobe: Proposal of a universal nomenclature for clinical and anatomical use, Neurosurg. Rev., 44 (2021), 335–350. https://doi.org/10.1007/s10143-019-01212-2 doi: 10.1007/s10143-019-01212-2
![]() |
[47] |
S. Smerconish, J. E. Schmitt, Neuroanatomical correlates of cognitive dysfunction in 22q11.2 deletion syndrome, Genes, 15 (2024), 440. https://doi.org/10.3390/genes15040440 doi: 10.3390/genes15040440
![]() |
[48] |
A. Ogura, H. Watanabe, K. Kawabata, R. Ohdake, Y. Tanaka, M. Masuda, et al., Semantic deficits in ALS related to right lingual/fusiform gyrus network involvement, EBioMedicine, 47 (2019), 506–517. https://doi.org/10.1016/j.ebiom.2019.08.022 doi: 10.1016/j.ebiom.2019.08.022
![]() |
[49] |
N. Aldusary, G. L. Taber, P. Freund, F. C. Fierz, K. P. Weber, A. Baeshhen, et al., Abnormal connectivity and brain structure in patients with visual snow, Front. Hum. Neurosci., 14 (2020), 582031. https://doi.org/10.3389/fnhum.2020.582031 doi: 10.3389/fnhum.2020.582031
![]() |
1. | Sana Abdulkream Alharbi, Azmin Sham Rambely, A New ODE-Based Model for Tumor Cells and Immune System Competition, 2020, 8, 2227-7390, 1285, 10.3390/math8081285 | |
2. | Bruno Carbonaro, Marco Menale, Towards the Dependence on Parameters for the Solution of the Thermostatted Kinetic Framework, 2021, 10, 2075-1680, 59, 10.3390/axioms10020059 | |
3. | Carlo Bianca, On the Modeling of Energy-Multisource Networks by the Thermostatted Kinetic Theory Approach: A Review with Research Perspectives, 2022, 15, 1996-1073, 7825, 10.3390/en15217825 | |
4. | Carlo Bianca, Thermostatted Kinetic Theory Structures in Biophysics: Generalizations and Perspectives, 2024, 4, 2673-9909, 1278, 10.3390/appliedmath4040069 | |
5. | Carlo Bianca, A decade of thermostatted kinetic theory models for complex active matter living systems, 2024, 50, 15710645, 72, 10.1016/j.plrev.2024.06.015 | |
6. | Carlo Bianca, The Role of the Table of Games in the Discrete Thermostatted Kinetic Theory, 2024, 12, 2227-7390, 2356, 10.3390/math12152356 | |
7. | Bruno Carbonaro, Marco Menale, A nonconservative kinetic framework under the action of an external force field: Theoretical results with application inspired to ecology, 2023, 34, 0956-7925, 1170, 10.1017/S0956792523000232 | |
8. | Fengling Jia, Peiyan He, Lixin Yang, A Novel Coupled Memristive Izhikevich Neuron Model and Its Complex Dynamics, 2024, 12, 2227-7390, 2244, 10.3390/math12142244 | |
9. | Bruno Carbonaro, Marco Menale, Markov Chains and Kinetic Theory: A Possible Application to Socio-Economic Problems, 2024, 12, 2227-7390, 1571, 10.3390/math12101571 | |
10. | Marco Menale, Ezio Venturino, A kinetic theory approach to modeling prey–predator ecosystems with expertise levels: analysis, simulations and stability considerations, 2024, 43, 2238-3603, 10.1007/s40314-024-02726-2 | |
11. | Muhammad Naeem Aslam, Nadeem Shaukat, Muhammad Sarmad Arshad, Muhammad Waheed Aslam, Javed Hussain, An intelligent approach for analyzing the effects of normal tumor immune unhealthy diet model through unsupervised physics informed neural-networks integrated with meta-heuristic algorithms, 2025, 18, 1864-5909, 10.1007/s12065-024-01007-5 |