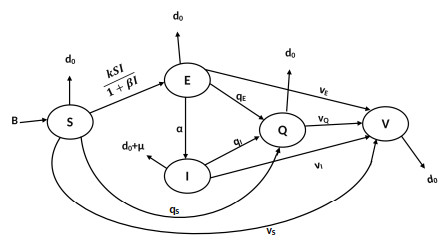
This paper investigates the long-time behavior for a Navier–Stokes–Allen–Cahn system, a diffuse interface model for two-phase incompressible flows with unmatched densities, non-constant viscosities, and a singular Flory–Huggins potential. First, we establish the dissipativity of strong solutions via some a priori estimates. Then, we demonstrate the regular-continuity of the semigroup, which allows us to prove the existence of the global attractor in the strong solutions space.
Citation: Chunyou Sun, Junyan Tan. Attractors for a Navier–Stokes–Allen–Cahn system with unmatched densities[J]. Communications in Analysis and Mechanics, 2025, 17(1): 237-262. doi: 10.3934/cam.2025010
[1] | C. W. Chukwu, Fatmawati . Modelling fractional-order dynamics of COVID-19 with environmental transmission and vaccination: A case study of Indonesia. AIMS Mathematics, 2022, 7(3): 4416-4438. doi: 10.3934/math.2022246 |
[2] | K. A. Aldwoah, Mohammed A. Almalahi, Mansour A. Abdulwasaa, Kamal Shah, Sunil V. Kawale, Muath Awadalla, Jihan Alahmadi . Mathematical analysis and numerical simulations of the piecewise dynamics model of Malaria transmission: A case study in Yemen. AIMS Mathematics, 2024, 9(2): 4376-4408. doi: 10.3934/math.2024216 |
[3] | Rahat Zarin, Amir Khan, Aurangzeb, Ali Akgül, Esra Karatas Akgül, Usa Wannasingha Humphries . Fractional modeling of COVID-19 pandemic model with real data from Pakistan under the ABC operator. AIMS Mathematics, 2022, 7(9): 15939-15964. doi: 10.3934/math.2022872 |
[4] | Oluwaseun F. Egbelowo, Justin B. Munyakazi, Manh Tuan Hoang . Mathematical study of transmission dynamics of SARS-CoV-2 with waning immunity. AIMS Mathematics, 2022, 7(9): 15917-15938. doi: 10.3934/math.2022871 |
[5] | Sara Salem Alzaid, Badr Saad T. Alkahtani . Real-world validation of fractional-order model for COVID-19 vaccination impact. AIMS Mathematics, 2024, 9(2): 3685-3706. doi: 10.3934/math.2024181 |
[6] | Moh. Mashum Mujur Ihsanjaya, Nanang Susyanto . A mathematical model for policy of vaccinating recovered people in controlling the spread of COVID-19 outbreak. AIMS Mathematics, 2023, 8(6): 14508-14521. doi: 10.3934/math.2023741 |
[7] | Ishtiaq Ali, Sami Ullah Khan . Dynamics and simulations of stochastic COVID-19 epidemic model using Legendre spectral collocation method. AIMS Mathematics, 2023, 8(2): 4220-4236. doi: 10.3934/math.2023210 |
[8] | Nadiyah Hussain Alharthi, Mdi Begum Jeelani . Analyzing a SEIR-Type mathematical model of SARS-COVID-19 using piecewise fractional order operators. AIMS Mathematics, 2023, 8(11): 27009-27032. doi: 10.3934/math.20231382 |
[9] | Chontita Rattanakul, Inthira Chaiya . A mathematical model for predicting and controlling COVID-19 transmission with impulsive vaccination. AIMS Mathematics, 2024, 9(3): 6281-6304. doi: 10.3934/math.2024306 |
[10] | Salah Boulaaras, Ziad Ur Rehman, Farah Aini Abdullah, Rashid Jan, Mohamed Abdalla, Asif Jan . Coronavirus dynamics, infections and preventive interventions using fractional-calculus analysis. AIMS Mathematics, 2023, 8(4): 8680-8701. doi: 10.3934/math.2023436 |
This paper investigates the long-time behavior for a Navier–Stokes–Allen–Cahn system, a diffuse interface model for two-phase incompressible flows with unmatched densities, non-constant viscosities, and a singular Flory–Huggins potential. First, we establish the dissipativity of strong solutions via some a priori estimates. Then, we demonstrate the regular-continuity of the semigroup, which allows us to prove the existence of the global attractor in the strong solutions space.
COVID-19 is a contagious disease that can be easily transmitted from one person to another. At the end of 2019, Wuhan, China, was the site of this terrible infection for the first time. Later, this disease gave rise to a worldwide outbreak, which the World Health Organization (WHO) notified in the first quarter of 2020 [1]. Almost all of the countries of the world have experienced widespread transmission of this virus. The aforementioned sickness has currently claimed the lives of almost 50 billion people. COVID-19 has affected more than 500 million people worldwide. We cite [2,3] for a detailed explanation of how diseases are contracted and spread. Because the pandemic has affected the entire world, every nation has implemented its own policies. Some nations have implemented lockdowns, while others have issued laws requiring strict safety measures like wearing face masks and avoiding large gatherings, etc. The countries most impacted by the disease are Brazil, Italy, India, Iran, Spain, USA and the UK. Earlier research suggested that the virus originated in animals, but later research showed that it may also transfer from person to person [4,5].
Numerous infectious diseases are being investigated for potential treatments, controls, cures, etc. Since epidemiology is an important field of medical science, one of the key subfields from this viewpoint is mathematical biology. In contrast to earlier times, researchers are now becoming increasingly interested in biomathematics and bio-math engineering. In order to give a framework for comprehending the dynamics of diverse infectious diseases, mathematical models have been utilized. The most effective tools for studying different diseases are mathematical models [6,7,8]. We can create numerous predictions, regulating strategies, etc [9,10,11], for a disease in a community by using mathematical models. Considering these factors, researchers have shown a strong interest in the aforementioned subject, we refer the reader to [12,13,14,15]. Many mathematical models have recently been developed by researchers for COVID-19 as well; we provide e.g., see [16,17,18,19]. Major public health difficulties and an economic catastrophe are being faced internationally as a result of the aforementioned disease's recent pandemic status and quick global spread.
In order to theoretically improve existing mathematical models for the aforementioned disease, it is necessary to evaluate the influence of recently developed vaccinations with known high efficacy that have weak transmission-controlling measures. For example, Pearson et al. [20] studied COVID-19 immunization in low as well as middle-income countries. they found it to be very cost-effective, according to recent research [21], if a vaccination with high efficacy and low cost is available. This will have a big impact on disease eradication. Prior to pharmaceutical interventions like treatment and immunization, non-pharmaceutical precautionary measures like the self-quarantining of confirmed cases, isolation, using of face masks, hand washing, social distancing, lockdowns, avoiding gatherings, and closing schools have been employed. This, however, is not a permanent solution. The best strategy will thus be to develop a suitable immunization and make it broadly accessible in every civilization at a fair price. Investigation of COVID-19, various researchers have developed many models. In order to do this, we have aimed to develop a mathematical model with a class of people who have received vaccinations and qualitatively analyze it while considering the therapeutic choices, immunization of those who are susceptible, and healthcare for infected/hospitalized people. Our model takes into account certain important biological and epidemiological elements of the disease under study, such as the disease's inhibitory effect, mortality rates from infections and natural causes, birth rate, and various vaccination rates.
Here, we build the dynamical model for COVID-19. Additionally, we depict the interactions between several model compartments in flow charts in Figure 1. Consequently, we first explain our model as follows:
{dSdt=B−kSI1+βI−(VS+d0+qS)SdEdt=kSI1+βI−(α+d0+qE+vE)EdIdt=αE−(d0+μ+vI+qI)IdQdt=qSS+qEE+qII−(d0+vQ)QdVdt=vSS+vEE+vII+vQQ−d0V. | (1.1) |
Parameters | Physical meaning and representation |
Susceptible compartment | |
Exposed compartment | |
Infected compartment | |
Quarantine compartment | |
Vaccinated compartment | |
Birth rate | |
COVID death rate | |
Natural death rate | |
inhibitory effect rate | |
Saturation constant | |
Infection rate from exposed class | |
Quarantine from susceptible class | |
Quarantine from exposed class | |
Quarantine from infected class | |
Vaccination rate of susceptible | |
Vaccination rate of exposed | |
Vaccination rate of infected | |
Vaccination rate of quarantine |
In Figure 1, the model is presented as a flowchart. Epidemiological models have been examined using difference equations or classical order derivatives. Some references are cited as [22,23]. The aforementioned operators cannot generate the phenomenon's global dynamical behavior because of their local nature [24,25,26,27]. As a result, academics have now taken the majority of models into account when considering fractional order derivatives [28,29,30,31]. The growth of the aforementioned field is attributable to its numerous applications to practical issues, particularly in the fields of mathematical biology and dynamical analysis [32,33,34,35,36]. As far as we are aware, fractional calculus is being used to describe an increasing number of real-world scenarios; we refer the reader to [37,38,39,40,41,42,43]. Additionally, the idea of fractional calculus has been applied in a number of scientific and technological fields [44,45,46]. Because of its global nature and memory-preserving methodology, the theory of fractional calculus has been widely used in the mathematical modeling of numerous diseases for further information, see [47,48,49]. The conventional analytical and numerical approaches have been upgraded to handle situations with fractional order derivatives (see references [50,51]). To examine diverse dynamical issues involving fractional order derivatives, the classical decomposition, perturbation, and transformation methods, for instance, have been expanded (some references are cited as [52,53]). Traditional numerical techniques, such as RKM tools, Euler and Taylor methods, the Adams-Bashforth method, etc., have been upgraded more and more to cope with fractional order problems (see [54,55]). Recently, a novel idea has been identified that describes the crossover behavior of the dynamics, because the states of multiple evolution processes frequently change suddenly. Due to these effects, typical derivatives, whether classical or fractional, cannot be used to appropriately address the relevant multi-phase behaviors. Therefore, scholars [56,57,58,59,60,61] have suggested this idea, which has the potential to appropriately address the aforementioned behavior to a certain extent. As a result, we also attempt at numerical analysis for our studied mathematical model by looking at multi-phase behaviors under various fractional orders by utilizing some numerical technique based on Newton interpolation polynomials. Discussion and a graphic presentation versus some actual facts are offered.
The provided model (1.1) can be stated as follows in the sense of the new derivative:
PWCDφ+0(S)(t)=B−kSI1+βI−(VS+d0+qS)SPWCDφ+0(E)(t)=kSI1+βI−(α+d0+qE+vE)EPWCDφ+0(I)(t)=αE−(d0+μ+vI+qI)IPWCDφ+0(Q)(t)=qSS+qEE+qII−(d0+vQ)QPWCDφ+0(V)(t)=vSS+vEE+vII+vQQ−d0V, | (1.2) |
where PWCD+0 denotes piecewise classical or Caputo derivative with two sub-intervals in [0,T].
For further clarification, we respectively express the left hand sides of (1.2) as follows:
PWCDφ+0(S)(t)={D+0[S(t)], 0<t≤t1,CDφ+0(S)(t)=1Γ(1−φ)∫t2t1(t−η)−φdS(η)dηdη, t1<t≤T,,PWCDφ+0(E)(t)={D+0[E(t)], 0<t≤t1,CDφ+0(E)(t)=1Γ(1−φ)∫t2t1(t−η)−φdE(η)dηdη, t1<t≤T,,PWCDφ+0(I)(t)={D+0[I(t)], 0<t≤t1,CDφ+0(I)(t)=1Γ(1−δ)∫t2t1(t−η)−δdI(η)dηdη, t1<t≤T,,PWCDφ+0(Q)(t)={D+0[Q(t)], 0<t≤t1,CDφ+0(Q)(t)=1Γ(1−δ)∫t2t1(t−η)−δdQ(η)dηdη, t1<t≤T,,PWCDφ+0(V)(t)={D+0[V(t)], 0<t≤t1,CDφ+0(V)(t)=1Γ(1−δ)∫t2t1(t−η)−δdV(η)dη(η)dη, t1<t≤T,, | (1.3) |
where D+0 and CDφ+0 are classical and Caputo derivatives, respectively.
Our manuscript is structured as follows: In the first section, we give introduction. In the Section 2, we give basic results. Stability results are given in the Section 3. Numerical results are given in the Section 4. In the Section 5, the fractional order form of the proposed model is investigated. In the Section 6, we given conclusion.
We recollect some fundamental results of fractional calculus.
Definition 2.1. [43] Let ϖ>0, then, the non-integer order integral of a function S:[0,∞)→R is defined as
Iφ+0S(t)=1Γ(φ)∫t0S(η)(t−η)1−φdη, |
provided that right-hand side exists. Also, derivative in the Caputo sense is defined as
Dφ0+S(t)={1Γ(1−φ)∫t0(t−η)−φS′(η)dη, 0<φ<1,dSdt, φ=1. |
Lemma 2.2. [43] Let h∈L[0,∞), then, the solution of
Dφ0+S(t)=h(t), φ∈(0,1],S(0)=S0 |
is given by
S(t)=S0+1Γ(φ)∫t0h(η)(t−η)1−φdη. |
Here, we recollect the piecewise definitions of the fractional order derivative and integral.
Definition 2.3. [61] If h(t) is a differentiable function, then the definition of the classical, and fractional piecewise derivative is
PWC0Dφth(t)={h′(t), 0<t≤t1,Dφ0+h(t) t1<t≤t2, |
such that PWC0Dφt is the classical derivative for 0<t≤t1 and fractional derivative for t1<t≤t2.
Definition 2.4. [61] Let h be a differentiable function, then, the classical, and fractional order piecewise integration is given as
PWIφ0+h(t)={∫t0h(η)dη, 0<t≤t1,1Γ(φ)∫tt1(t−η)φ−1h(η)d(η) t1<t≤t2, |
such that PFI+0φ(t) denotes the classical integration for 0<t≤t1, and Reimann-Liouville integration for t1<t≤t2.
Lemma 2.5. [61] Consider the problem with the piecewise fractional order derivative
PFC0Dφth(t)=F(t,h(t)), 0<φ≤1, |
whose solution is given by
h(t)={h0+∫t0h(η)dη, 0<t≤t1;h(t1)+1Γ(φ)∫tt1(t−η)φ−1h(η)d(η) t1<t≤t2. |
Here, we define the Taylor series from [59]; consider
Dφtx(t)=F(t,x(t)),x(0)=x0, | (2.1) |
where F:[0,∞)×R→R. The generalized Taylor series is thus given by
x(t+h)=x(t)+hφγ(φ+1)Dφtx(t)+h2φγ(2φ+1)D2φtx(t)+... | (2.2) |
The outcomes of fractional calculus are used here. D2φtx=DφtF(t,x(t))+F(t,x(t))DφxF(t,x(t)). By using (2.2), after rearranging the terms, we obtain the general formula shown below.
x(t+h)=x(t)+hφγ(φ+1)F(t,x(t))+hφ2Γ(φ+1)[K1+K2], | (2.3) |
where
K1=F(ti,xi(ti)), K2=F(ti+2hφΓ(φ+1)Γ(2φ+1), x(ti)+2hφΓ(φ+1)Γ(2φ+1)F(ti,xi(ti))). | (2.4) |
Assume that φ=1; the standard method described by (4.2).
We initially examine our model's feasibility in this part. In order to achieve this, we add each equation in the model (1.1) under the condition that
N(t)=S(t)+E(t)+I(t)+Q(t)+V(t), |
one has
dNdt=B−d0N−μI. | (3.1) |
From (3.1), we have
limt→∞supN≤N0. |
Then, limt→∞supN=N0 if and only if limt→∞supI=0.
In the system (1.1), the first equation yields
0≤limt→∞supS≤S0. |
Similarly, the second equation of the model (1.1) yields
0≤limt→∞supE≤E0. |
From above, we conclude that dNdt<0 if N>N0. Additionally, we have
χ={(S,E,I,Q,V)∈R5+:N≤N0≤Bd0}. | (3.2) |
Disease free equilibrium points: We analyze whether the system (1.1) has an equilibrium point. E0=(S0,0,0,0)=(Bdo,0,0,0) is a representation of the model (1.1) disease-free equilibrium.
S0=BvS+d0+qSQ0=S0d0+vQV0=vSS0+vQQ0d0. |
Endemic equilibrium: From (1.1), lets assume that dSdt=0,dEdt=0,dIdt=0,dQdt=0 and dVdt=0; then, by simple calculation one can find the endemic equilibrium to be as follows:
S∗(t)=(α+d0+qE+vE)(d0+μ+vI+qI)(1+βI∗)αkE∗(t)=(d0+μ+vI+qI)I∗αI∗(t)=αkβ−(vS+d0+qS)(α+d0+qE+vE)(d0+μ+vI+qI)(α+d0+qE+vE)(d0+μ+vI+qI)(k+β(vS+d0+qS))Q∗(t)=qSS∗+qEE∗+qII∗d0+vQV∗(t)=vSS∗+vQQ∗+vII∗d0. |
In epidemiology, the idea of the fundamental reproduction number, or R0, outlines how illnesses spread and are treated. Both the frequency of the illness in the populace and the best countermeasures to protect the neighborhood's residents from the deadly virus are disclosed by R0. The most recent method for determining R0 is as follows. If we set χ=(E,I), we have from the system (1.1) the following
dχdt=H−F, |
where
H=(kSI1+βI0) |
and
F=((α+d0+qE+vE)E−αE(t)+(d0+μ+vI+qI)I). |
The Jacobian of H for the disease-free equilibrium is
H=(0kS000), |
and for the disease-free equilibrium, the Jacobian of F is given by
F=(α+d0+qE+vE0−αd0+μ+qI+vI). |
Hence
F−1=1(α+d0+qE+vE)(d0+μ+vI+qI)(d0+μ+vI+qI0αα+d0+qE+vE.). |
We have
HF−1=1(α+d0+qE+vE)(d0+μ+vI+qI)(αkS0000). |
Hence, the required R0 is given by
R0=kαB(α+d0+vE+qE)(vS+d0+qS)(d0+μ+qI+vI). | (3.3) |
If and only if the reproduction number meets the requirement that R0<1, then we may state that the disease-free equilibrium is stable and the population is disease-free. You can halt the spread of an epidemic by exercising prudence. By sitting the numerical values of the variables above, R0=0.98347. This number shows that the spread of COVID-19 in the locality is well managed.
On the basis of R0, we established the following theorem.
Theorem 3.1. (ⅰ) If R0≤1, then positive equilibria for system (1.1) are not possible.
(ⅱ) If R0>1, then there is an endemic equilibrium, often known as a distinct positive (unique) equilibrium E∗=(S∗,I∗,Q∗,V∗).
Some further results on equilibrium point with global and local stability are presented here for system (1.1).
Theorem 3.2. The disease-free equilibrium E0 is locally asymptotically stable, if R0≤1, and unstable if R0>1.
Proof. The Jacobian matrix at E0 is given below:
M0=[−(vS+d0+qS)0−kBvS+d0+qS000−(α+d0+qE+vE)−kBvS+d0+qS000α−(d0+μ+vI+qI)00qSqEqI−(d0+vQ)00vSvEvIvQ−d0]. |
From a simple straightforward calculation M0 has three eigenvalues which are negative; Λ1=−(vS+d0+qS), Λ2=−(d0+vQ) and Λ3=−d0. The next eigenvalues of M0 are derived from
Λ2+(2d0+α+μ+vI+qI+qE+vE)Λ+(α+d0+qE+vE)(d0+μ+vI+qI)−kαBvS+d0+qS=0. | (3.4) |
If R0>1, then,
(α+d0+qE+vE)(d0+μ+vI+qI)−kαBvS+d0+qS<0, |
which means that (3.4) has two roots, i.e., one positive root and one negative root. Therefore, the disease-free equilibrium E0 has an unstable saddle point. When R0=1, to get the result for the disease-free equilibrium E0 that is globally asymptotically stable, we consider the function called the Lyapunov function.
L(E,I)=αE+(α+d0+qE+vE)I. |
Taking the derivative of L(E,I) with respect to time t gives
dL(E,I)dt=[kαS1+αI−(α+d0+qE+vE)(d0+μ+vI+qI)]I≤[kαS−(α+d0+qE+vE)(d0+μ+vI+qI)]I≤αkS0R0(R0SS0−1)≤0. | (3.5) |
Suppose that I=0 implies that dL(E,I)dt=0. Furthermore, when R0=1, which implies that E0 is globally asymptotically stable in Φ.
In the next theorem, we study the stability at point E∗.
Theorem 3.3. If R0>1, the endemic equilibrium E∗ is globally asymptotically stable.
Proof. For the system (1.1), the Jacobian matrix is
M=[−kI∗1+βI∗−(vS+d0+vS)0kS∗(1+βI∗)200kI∗1+βI∗−(α+d0+qE+vE)kS∗(1+βI∗)2000α(d0+μ+vI+qI)00qSqEqI−(d0+vQ)0vSvEvIvQ−d0]. |
We see that the eigen values of M' i.e., Λ1=−d0 and Λ2=−(d0+vQ) are negative. Also, we have
Λ3+A1Λ2+A2Λ+A3=0, |
where
A1=kI∗1+βI∗+3d0+vS+vE+μ+vI+qI+qE+qS>0A2=(kI∗1+βI∗+vS+d0+qS)(2d0+α+μ+vI+qI+vE+qE)+(α+d0+qE+vE)(d0+μ+vI+qI)−αkS∗(1+βI∗)2>0>(kI∗1+βI∗+vS+d0+qS)(2d0+α+μ+vI+qI+vE+qE)>0A3=(kI∗1+βI∗+vS+d0+qS)[(α+d0+vE+qE)(d0+μ+vI+qI)−αkS∗(1+βI∗)2]+αkI∗(1+βI∗).αkS∗(1+βI∗)2>0. |
Consider the following equation
αkS∗(1+βI∗)=(α+d0+qE+vI)(d0+μ+qI). |
Using the Routh-Hurwitz theorem, we have that A1.A2−A3>0 by a simple direct calculation which shows that E∗ is locally asymptotically stable. This completes the proof.
Using the parameters from Table 2, we conducted the numerical simulations for model (1.1), we describe them in this section. Here, we describe how we simulate our system (1.1) by using the second-order Runge-Kutta (RK2) method as implemented in [64]. For this, we took into consideration
dx(t)dt=Ψ(t,x(t)). | (4.1) |
Parameters | Physical meaning and representation | Numerical value |
S | Susceptible compartment | 222.6 million[65] |
E | Exposed compartment | 120million [65,66,67] |
I | Infected compartment | 1.30[65] |
Q | Quarantine compartment | 1.30 [65] |
V | Vaccinated compartment | 100 millions [66] |
B | Birth rate | 1000059×365 [67] |
μ | COVID death rate | 0.018 [67] |
d0 | Natural death rate | 159×365 [67] |
β | inhibitory effect rate | 0.0701 [65,67] |
k | Saturation constant | 0.00019 [66,67] |
α | Infection rate from exposed class | 0.0833 [65,67] |
qS | Quarantine from susceptible class | 0.0701 [65,66,67] |
qE | Quarantine from exposed class | 0.13 [65,67,68] |
qI | Quarantine from infected class | 0.0701 [67] |
vS | Vaccination rate of susceptible | 0.0001 [65,67] |
vE | Vaccination rate of exposed | 0.4 [65,67] |
vI | Vaccination rate of infected | 0.00001 [65,67] |
vQ | Vaccination rate of quarantine | 0.0999 [65,67] |
Here x=(S,E,I,Q,V). The suggested approach allows us to express the relevant iterative formula as
xi+1=xi+h2Ψ(ti+h2,xi(ti)+K12). | (4.2) |
Here, K1=hF(ti,xi(ti)), also, h=ti+1−ti. We simulated our results by using the numerical approach described in (4.2) and use the numbers from Table 2 in order to evaluate with our built-in model (1.1). We use the results of the numerical simulations to demonstrate our theoretical results. The parameter values and their descriptions for the numerical simulations are listed in Table 2. However, some parameter values that are unknown have been assumed for the sake of illustration.
We applied 300 days of the actual data from Pakistan. The overall population of the nation is approximately equal to 222.60 million, according to the data in [65,67]. Nearly 47 of the nation's population was fully vaccinated during the past nine months, while 57 was partially vaccinated. In order to analyze the dynamics of disease propagation and the impact of the vaccination on its cure, we simulated the outcomes for 300 days as shown in Figures 2–6, respectively by using the numerical approach described by (4.2) of RK2 type. Here, we describe the model (1.1).
Figures 2 through 6 depict the spread of the disease over a 300-day period at the specified speeds. The exposed class and the susceptible class as shown by the actual data that were employed. The infection spread relatively quickly in less than 50 days. As a result, there were also more persons under quarantine. More people had full or partial vaccinations during this time. We can notice that there were more persons who had received vaccinations.
Here, we develop a numerical scheme based on RK2 method for the model (1.2) as expressed in terms of (1.3). We have developed a numerical method for the two sub-intervals of [0,T]. We express the system (1.2) in terms of 1.3 by using (x)=(S,E,I,Q,V) as follows:
PWCDφ+0(S)(t)={D+0[S(t)]=Ψ1(t,(x)), 0<t≤t1,CDφ+0(S)(t)=Ψ1(t,(x)), t1<t≤T,,PWCDφ+0(E)(t)={D+0[E(t)]=Ψ2(t,(x)),, 0<t≤t1,CDφ+0(E)(t)=Ψ2(t,(x)),, t1<t≤T,,PWCDφ+0(I)(t)={D+0[I(t)]=Ψ3(t,(x)),, 0<t≤t1,CDφ+0(I)(t)=Ψ3(t,(x)),, t1<t≤T,,PWCDφ+0(Q)(t)={D+0[Q(t)]=Ψ4(t,(x)),, 0<t≤t1,CDφ+0(Q)(t)=Ψ4(t,(x)),, t1<t≤T,,PWCDφ+0(V)(t)={D+0[V(t)]=Ψ5(t,(x)),, 0<t≤t1,CDφ+0(V)(t)=Ψ5(t,(x)), t1<t≤T. | (5.1) |
We may write the aforesaid system (5.1) by using (4.2) for classical order derivative when 0<t<t1 and (2.3) with the (2.4) for the fractional order Caputo derivative with t1<t<T. Hence, we can write the first equation of the system (5.1) as follows:
S(ti+1))={Si−1(ti−1)+h2Ψ1[ti−1+h2,xi−1(ti−1)+K12], 0<t≤t1Si(ti)+hφγ(φ+1)Ψ1(ti,xi(ti))+hφ2Γ(φ+1)[K2+K3], t1<t≤T, | (5.2) |
where h=ti+1−ti, and
K1=Ψ1(ti−1,xi−1(ti−1)), K2=Ψ1(ti,xi(ti)),K3=Ψ1(ti+2hφΓ(φ+1)Γ(2φ+1),x(ti)+2hφΓ(φ+1)Γ(2φ+1)Ψ1(ti,xi(ti))). | (5.3) |
Similarly, the equations for the other compartments can be written as follows:
E(ti+1))={Ei−1(ti−1)+h2Ψ2[ti−1+h2,xi−1(ti−1)+K12], 0<t≤t1Ei(ti)+hφγ(φ+1)Ψ2(ti,xi(ti))+hφ2Γ(φ+1)[K2+K3], t1<t≤T, | (5.4) |
I(ti+1))={Ii−1(ti−1)+h2Ψ3[ti−1+h2,xi−1(ti−1)+K12], 0<t≤t1Ii(ti)+hφγ(φ+1)Ψ3(ti,xi(ti))+hφ2Γ(φ+1)[K2+K3], t1<t≤T, | (5.5) |
Q(ti+1))={Qi−1(ti−1)+h2Ψ4[ti−1+h2,xi−1(ti−1)+K12], 0<t≤t1Qi(ti)+hφγ(φ+1)Ψ4(ti,xi(ti))+hφ2Γ(φ+1)[K2+K3], t1<t≤T, | (5.6) |
and
V(ti+1))={Vi−1(ti−1)+h2Ψ5[ti−1+h2,xi−1(ti−1)+K12], 0<t≤t1Vi(ti)+hφγ(φ+1)Ψ5(ti,xi(ti))+hφ2Γ(φ+1)[K2+K3], t1<t≤T. | (5.7) |
Here, we plot the approximated results for two sets of fractional orders in two cases.
Case Ⅰ: When 0<φ≤0.6, the corresponding solutions exhibiting crossover behavior were obtained as in Figures 7–11 for different fractional orders.
Case Ⅱ: Now we present the numerical results corresponding to different fractional orders such that 0.6<φ<1 in Figures 12–16, respectively which show the crossover behaviors of different compartments.
Here, we discuss the results of modeling of the piecewise derivative shown in Figures 7–16. We observed a the sudden change after 150 days. So, for various fractional orders in [0,50) and [50,300], we have plotted the numerical findings for systems (1.3) and (5.1). It is possible to see the declines and rises in several compartments with obvious crossover behavior. This novel idea makes a significant contribution to the understanding of how a system that experiences sudden changes in its dynamics behaves. Additionally, the fractional order derivative is a global operator that furthers our comprehension of the phenomenon. In Figure 17, we have compared some real data of infected cases with the simulated results at given fractional orders. Data applied here were taken from Pakistan from January 1, 2021 to January 30, 2021 (see [65]). We see that the simulated results were very similar to the real data which confirm the authentication of the fractional order model that we have considered.
Also, we have compared the simulated data for the vaccinated class for the period of 11 days i.e., March 1, 2022 to March 11, 2022 (see [67]) in Figure 18.
Here, a dynamical system for the COVID-19 disease has been examined. The traditional method for determining the conditions for both local and global stability, as well as for computing R0 at equilibrium locations, is to employ classical analysis. Additionally, the classical model scenario was numerically simulated. We then developed a strong algorithm to carry out the numerical analysis of the suggested model under the conditions of the new concept, extending the RK2 approach to piece-wise derivative applications. Using actual data from Pakistan for the first 300 days by splitting into two sub intervals, we have carried out a number of numerical simulations for various fractional orders. We have found that fractional calculus that involves applying in terms of piecewise derivative is more effective at clearly indicating the rapid changes in various model compartments. Crossover behavior is what we call such behavior. In the aforementioned process, a system experiences a rapid change in its state of dynamics and runs its crossover behavior is terminated. The aforementioned idea can be further refined to encompass more complex dynamical systems with various types of fractional differential operators. Also, in the future, we will combine stochastic type and fractals-fractional differential type operators in piecewise form for various infectious disease models.
The authors declare they have not used artificial intelligence (AI) tools in the creation of this article.
This work was supported and funded by the Deanship of Scientific Research at Imam Mohammad Ibn Saud Islamic University (IMSIU) (Grant Number IMSIU- RG23124).
The authors declare that they have no competing interest.
[1] |
S. M. Allen, J. W. Cahn, A microscopic theory for antiphase boundary motion and its application to antiphase domain coarsening, Acta Metall., 27 (1979), 1085–1095. https://doi.org/10.1016/0001-6160(79)90196-2 doi: 10.1016/0001-6160(79)90196-2
![]() |
[2] | D. T. Wasan, Interfacial transport processes and rheology: structure and dynamics of thin liquid films, Chem. Eng. Educ., 26 (1992), 104–112. |
[3] |
H. Abels, On a diffuse interface model for two-phase flows of viscous, incompressible fluids with matched densities, Arch. Ration. Mech. Anal., 194 (2009), 463–506. https://doi.org/10.1007/s00205-008-0160-2 doi: 10.1007/s00205-008-0160-2
![]() |
[4] |
D. M. Anderson, G. B. McFadden, A. A. Wheeler, Diffuse-interface methods in fluid mechanics, Annu. Rev. Fluid Mech., 30 (1998), 139–165. https://doi.org/10.1146/annurev.fluid.30.1.139 doi: 10.1146/annurev.fluid.30.1.139
![]() |
[5] | J. J. Feng, C. Liu, J. Shen, P. Yue, An energetic variational formulation with phase field methods for interfacial dynamics of complex fluids: advantages and challenges, In: Modeling of Soft Matter, New York: Springer, 2005, 1–26. https://doi.org/10.1007/0-387-32153-5_1 |
[6] |
J. Shen, X. Yang, A phase-field model and its numerical approximation for two-phase incompressible flows with different densities and viscosities, SIAM J. Sci. Comput., 32 (2010), 1159–1179. https://doi.org/10.1137/09075860X doi: 10.1137/09075860X
![]() |
[7] |
J. Lowengrub, L. Truskinovsky, Quasi-incompressible Cahn-Hilliard fluids and topological transitions, Proc. R. Soc. Lond. A, 454 (1998), 2617–2654. https://doi.org/10.1098/rspa.1998.0273 doi: 10.1098/rspa.1998.0273
![]() |
[8] |
X. Chen, D. Hilhorst, E. Logak, Mass conserving Allen-Cahn equation and volume preserving mean curvature flow, Interfaces and Free Bound., 12 (2010), 527–549. https://doi.org/10.4171/IFB/244 doi: 10.4171/IFB/244
![]() |
[9] |
J. W. Cahn, On spinodal decomposition, Acta Metall., 9 (1961), 795–801. https://doi.org/10.1016/0001-6160(61)90182-1 doi: 10.1016/0001-6160(61)90182-1
![]() |
[10] |
Z. Han, R. Xu, Improved growth estimate for one-dimensional sixth-order Boussinesq equation with logarithmic nonlinearity, Appl. Math. Lett., 160 (2025), 109290. https://doi.org/10.1016/j.aml.2024.109290 doi: 10.1016/j.aml.2024.109290
![]() |
[11] |
R. Xu, Y. Yang, B. Liu, J. Shen, S. Huang, Global existence and blowup of solutions for the multidimensional sixth-order "good" Boussinesq equation, Z. Angew. Math. Phys., 66 (2015), 955–976. https://doi.org/10.1007/s00033-014-0459-9 doi: 10.1007/s00033-014-0459-9
![]() |
[12] |
Z. Han, R. Xu, Y. Yang, The qualitative behavior for one-dimensional sixth-order Boussinesq equation with logarithmic nonlinearity, Discrete Contin. Dyn. Syst. -S, 16 (2023), 3131–3145. https://doi.org/10.3934/dcdss.2023141 doi: 10.3934/dcdss.2023141
![]() |
[13] |
R. Xu, Y. Luo, J. Shen, S. Huang, Global existence and blow up for damped generalized Boussinesq equation, Acta Math. Appl. Sin. Engl. Ser., 33 (2017), 251–262. https://doi.org/10.1007/s10255-017-0655-4 doi: 10.1007/s10255-017-0655-4
![]() |
[14] |
R. Chen, Z. Liang, D. Wang, R. Xu, On the inviscid limit of the compressible Navier-Stokes equations near Onsager's regularity in bounded domains, Sci. China Math., 67 (2024), 1–22. https://doi.org/10.1007/s11425-022-2085-3 doi: 10.1007/s11425-022-2085-3
![]() |
[15] |
R. Hosek, V. Macha, Weak-strong uniqueness for Navier-Stokes/Allen-Cahn system, Czechoslovak Math. J., 69 (2019), 837–851. https://doi.org/10.21136/CMJ.2019.0520-17 doi: 10.21136/CMJ.2019.0520-17
![]() |
[16] |
H. Wu, Well-posedness of a diffuse-interface model for two-phase incompressible flows with thermo-induced Marangoni effect, Eur. J. Appl. Math., 28 (2017), 380–434. https://doi.org/10.1017/S0956792516000322 doi: 10.1017/S0956792516000322
![]() |
[17] |
X. Xu, L. Zhao, C. Liu, Axisymmetric solutions to coupled Navier-Stokes/Allen-Cahn equations, SIAM J. Math. Anal., 41 (2010), 2246–2282. https://doi.org/10.1137/090754698 doi: 10.1137/090754698
![]() |
[18] |
Y. Chen, V. D. Radulescu, R. Xu, High energy blowup and blowup time for a class of semilinear parabolic equations with singular potential on manifolds with conical singularities, Commun. Math. Sci., 21 (2023), 25–63. https://doi.org/10.4310/CMS.2023.v21.n1.a2 doi: 10.4310/CMS.2023.v21.n1.a2
![]() |
[19] |
Q. Lin, R. Xu, Global well-posedness of the variable-order fractional wave equation with variable exponent nonlinearity, J. Lond. Math. Soc., 111 (2025), e70091. https://doi.org/10.1112/jlms.70091 doi: 10.1112/jlms.70091
![]() |
[20] | M. Giga, A. Kirshtein, C. Liu, Variational modeling and complex fluids, In: Handbook of Mathematical Analysis in Mechanics of Viscous Fluids, Cham: Springer, 2018, 73–113. https://doi.org/10.1007/978-3-319-13344-7_2 |
[21] |
J. Jiang, Y. Li, C. Liu, Two-phase incompressible flows with variable density: an energetic variational approach, Discrete Contin. Dyn. Syst., 37 (2017), 3243–3284. https://doi.org/10.3934/dcds.2017138 doi: 10.3934/dcds.2017138
![]() |
[22] |
C. Liu, J. Shen, X. Yang, Decoupled energy stable schemes for a phase-field model of two-phase incompressible flows with variable density, J. Sci. Comput., 62 (2015), 601–622. https://doi.org/10.1007/s10915-014-9867-4 doi: 10.1007/s10915-014-9867-4
![]() |
[23] |
S. Chen, Y. Salmaniw, R. Xu, Global existence for a singular Gierer-Meinhardt system, J. Differ. Equ., 262 (2017), 2940–2960. https://doi.org/10.1016/j.jde.2016.11.022 doi: 10.1016/j.jde.2016.11.022
![]() |
[24] |
S. Chen, Y. Salmaniw, R. Xu, Bounded solutions to a singular parabolic system, J. Math. Anal. Appl., 455 (2017), 936–978. https://doi.org/10.1016/j.jmaa.2017.06.012 doi: 10.1016/j.jmaa.2017.06.012
![]() |
[25] |
R. Xu, W. Lian, Y. Niu, Global well-posedness of coupled parabolic systems, Sci. China Math., 63 (2020), 321–356. https://doi.org/10.1007/s11425-017-9280-x doi: 10.1007/s11425-017-9280-x
![]() |
[26] |
R. Xu, J. Liu, Y. Niu, S. Chen, Asymptotic behaviour of solution for multidimensional viscoelasticity equation with nonlinear source term, Bound. Value Probl., 2013 (2013), 1–13. https://doi.org/10.1186/1687-2770-2013-42 doi: 10.1186/1687-2770-2013-42
![]() |
[27] |
R. Xu, J. Su, Global existence and finite time blow-up for a class of semilinear pseudo-parabolic equations, J. Funct. Anal., 264 (2013), 2732–2763. https://doi.org/10.1016/j.jfa.2013.03.010 doi: 10.1016/j.jfa.2013.03.010
![]() |
[28] |
R. Xu, Y. Niu, Addendum to "Global existence and finite time blow-up for a class of semilinear pseudo-parabolic equations'' [J. Func. Anal. 264 (2013) 2732–2763], J. Funct. Anal., 270 (2016), 4039–4041. https://doi.org/10.1016/j.jfa.2016.02.026 doi: 10.1016/j.jfa.2016.02.026
![]() |
[29] |
A. Giorgini, A. Miranville, R. Temam, Uniqueness and regularity for the Navier-Stokes-Cahn-Hilliard system, SIAM J. Math. Anal., 51 (2019), 2535–2574. https://doi.org/10.1137/18M1223459 doi: 10.1137/18M1223459
![]() |
[30] |
A. Giorgini, R. Temam, Attractors for the Navier-Stokes-Cahn-Hilliard system, Discrete Contin. Dyn. Syst. -S, 15 (2022), 2249–2274. https://doi.org/10.3934/dcdss.2022118 doi: 10.3934/dcdss.2022118
![]() |
[31] |
A. Giorgini, M. Grasselli, H. Wu, On the mass-conserving Allen-Cahn approximation for incompressible binary fluids, J. Funct. Anal., 283 (2022), 109631. https://doi.org/10.1016/j.jfa.2022.109631 doi: 10.1016/j.jfa.2022.109631
![]() |
[32] | R. Temam, Infinite-dimensional dynamical systems in mechanics and physics, New York: Springer-Verlag, 1997. https://doi.org/10.1007/978-1-4612-0645-3 |
[33] | A. Miranville, The Cahn-Hilliard Equation: Recent Advances and Applications, CBMS-NSF Regional Conference Series in Applied Mathematics, vol. 95, Society for Industrial and Applied Mathematics (SIAM), Philadelphia, PA, 2019. https://doi.org/10.1137/1.9781611975925 |
Parameters | Physical meaning and representation |
Susceptible compartment | |
Exposed compartment | |
Infected compartment | |
Quarantine compartment | |
Vaccinated compartment | |
Birth rate | |
COVID death rate | |
Natural death rate | |
inhibitory effect rate | |
Saturation constant | |
Infection rate from exposed class | |
Quarantine from susceptible class | |
Quarantine from exposed class | |
Quarantine from infected class | |
Vaccination rate of susceptible | |
Vaccination rate of exposed | |
Vaccination rate of infected | |
Vaccination rate of quarantine |
Parameters | Physical meaning and representation | Numerical value |
S | Susceptible compartment | 222.6 million[65] |
E | Exposed compartment | 120million [65,66,67] |
I | Infected compartment | 1.30[65] |
Q | Quarantine compartment | 1.30 [65] |
V | Vaccinated compartment | 100 millions [66] |
B | Birth rate | 1000059×365 [67] |
μ | COVID death rate | 0.018 [67] |
d0 | Natural death rate | 159×365 [67] |
β | inhibitory effect rate | 0.0701 [65,67] |
k | Saturation constant | 0.00019 [66,67] |
α | Infection rate from exposed class | 0.0833 [65,67] |
qS | Quarantine from susceptible class | 0.0701 [65,66,67] |
qE | Quarantine from exposed class | 0.13 [65,67,68] |
qI | Quarantine from infected class | 0.0701 [67] |
vS | Vaccination rate of susceptible | 0.0001 [65,67] |
vE | Vaccination rate of exposed | 0.4 [65,67] |
vI | Vaccination rate of infected | 0.00001 [65,67] |
vQ | Vaccination rate of quarantine | 0.0999 [65,67] |
Parameters | Physical meaning and representation |
Susceptible compartment | |
Exposed compartment | |
Infected compartment | |
Quarantine compartment | |
Vaccinated compartment | |
Birth rate | |
COVID death rate | |
Natural death rate | |
inhibitory effect rate | |
Saturation constant | |
Infection rate from exposed class | |
Quarantine from susceptible class | |
Quarantine from exposed class | |
Quarantine from infected class | |
Vaccination rate of susceptible | |
Vaccination rate of exposed | |
Vaccination rate of infected | |
Vaccination rate of quarantine |
Parameters | Physical meaning and representation | Numerical value |
S | Susceptible compartment | 222.6 million[65] |
E | Exposed compartment | 120million [65,66,67] |
I | Infected compartment | 1.30[65] |
Q | Quarantine compartment | 1.30 [65] |
V | Vaccinated compartment | 100 millions [66] |
B | Birth rate | 1000059×365 [67] |
μ | COVID death rate | 0.018 [67] |
d0 | Natural death rate | 159×365 [67] |
β | inhibitory effect rate | 0.0701 [65,67] |
k | Saturation constant | 0.00019 [66,67] |
α | Infection rate from exposed class | 0.0833 [65,67] |
qS | Quarantine from susceptible class | 0.0701 [65,66,67] |
qE | Quarantine from exposed class | 0.13 [65,67,68] |
qI | Quarantine from infected class | 0.0701 [67] |
vS | Vaccination rate of susceptible | 0.0001 [65,67] |
vE | Vaccination rate of exposed | 0.4 [65,67] |
vI | Vaccination rate of infected | 0.00001 [65,67] |
vQ | Vaccination rate of quarantine | 0.0999 [65,67] |