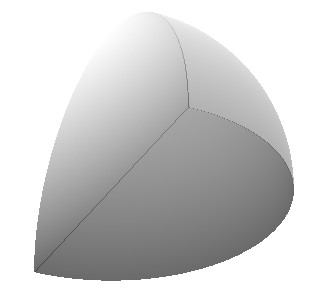
Odor is central to food quality. Still, a major challenge is to understand how the odorants present in a given food contribute to its specific odor profile, and how to predict this olfactory outcome from the chemical composition. In this proof-of-concept study, we seek to develop an integrative model that combines expert knowledge, fuzzy logic, and machine learning to predict the quantitative odor description of complex mixtures of odorants. The model output is the intensity of relevant odor sensory attributes calculated on the basis of the content in odor-active comounds. The core of the model is the mathematically formalized knowledge of four senior flavorists, which provided a set of optimized rules describing the sensory-relevant combinations of odor qualities the experts have in mind to elaborate the target odor sensory attributes. The model first queries analytical and sensory databases in order to standardize, homogenize, and quantitatively code the odor descriptors of the odorants. Then the standardized odor descriptors are translated into a limited number of odor qualities used by the experts thanks to an ontology. A third step consists of aggregating all the information in terms of odor qualities across all the odorants found in a given product. The final step is a set of knowledge-based fuzzy membership functions representing the flavorist expertise and ensuring the prediction of the intensity of the target odor sensory descriptors on the basis of the products' aggregated odor qualities; several methods of optimization of the fuzzy membership functions have been tested. Finally, the model was applied to predict the odor profile of 16 red wines from two grape varieties for which the content in odorants was available. The results showed that the model can predict the perceptual outcome of food odor with a certain level of accuracy, and may also provide insights into combinations of odorants not mentioned by the experts.
Citation: N. Mejean Perrot, Alice Roche, Alberto Tonda, Evelyne Lutton, Thierry Thomas-Danguin. Predicting odor profile of food from its chemical composition: Towards an approach based on artificial intelligence and flavorists expertise[J]. Mathematical Biosciences and Engineering, 2023, 20(12): 20528-20552. doi: 10.3934/mbe.2023908
[1] | Yongxiu Shi, Haitao Wan . Refined asymptotic behavior and uniqueness of large solutions to a quasilinear elliptic equation in a borderline case. Electronic Research Archive, 2021, 29(3): 2359-2373. doi: 10.3934/era.2020119 |
[2] | Shu Wang, Mengmeng Si, Rong Yang . Dynamics of stochastic 3D Brinkman-Forchheimer equations on unbounded domains. Electronic Research Archive, 2023, 31(2): 904-927. doi: 10.3934/era.2023045 |
[3] | Xiaoxia Wang, Jinping Jiang . The uniform asymptotic behavior of solutions for 2D g-Navier-Stokes equations with nonlinear dampness and its dimensions. Electronic Research Archive, 2023, 31(7): 3963-3979. doi: 10.3934/era.2023201 |
[4] |
Yichen Zhang, Meiqiang Feng .
A coupled |
[5] | Meng-Xue Chang, Bang-Sheng Han, Xiao-Ming Fan . Global dynamics of the solution for a bistable reaction diffusion equation with nonlocal effect. Electronic Research Archive, 2021, 29(5): 3017-3030. doi: 10.3934/era.2021024 |
[6] | Özlem Batıt Özen, Erbil Çetin, Öyküm Ülke, Aynur Şahin, Fatma Serap Topal . Existence and uniqueness of solutions for (p, q)-difference equations with integral boundary conditions. Electronic Research Archive, 2025, 33(5): 3225-3245. doi: 10.3934/era.2025142 |
[7] | José Luiz Boldrini, Jonathan Bravo-Olivares, Eduardo Notte-Cuello, Marko A. Rojas-Medar . Asymptotic behavior of weak and strong solutions of the magnetohydrodynamic equations. Electronic Research Archive, 2021, 29(1): 1783-1801. doi: 10.3934/era.2020091 |
[8] | Asma Al-Jaser, Osama Moaaz . Second-order general Emden-Fowler differential equations of neutral type: Improved Kamenev-type oscillation criteria. Electronic Research Archive, 2024, 32(9): 5231-5248. doi: 10.3934/era.2024241 |
[9] | Haibo Cui, Junpei Gao, Lei Yao . Asymptotic behavior of the one-dimensional compressible micropolar fluid model. Electronic Research Archive, 2021, 29(2): 2063-2075. doi: 10.3934/era.2020105 |
[10] | Zongming Guo, Fangshu Wan . Weighted fourth order elliptic problems in the unit ball. Electronic Research Archive, 2021, 29(6): 3775-3803. doi: 10.3934/era.2021061 |
Odor is central to food quality. Still, a major challenge is to understand how the odorants present in a given food contribute to its specific odor profile, and how to predict this olfactory outcome from the chemical composition. In this proof-of-concept study, we seek to develop an integrative model that combines expert knowledge, fuzzy logic, and machine learning to predict the quantitative odor description of complex mixtures of odorants. The model output is the intensity of relevant odor sensory attributes calculated on the basis of the content in odor-active comounds. The core of the model is the mathematically formalized knowledge of four senior flavorists, which provided a set of optimized rules describing the sensory-relevant combinations of odor qualities the experts have in mind to elaborate the target odor sensory attributes. The model first queries analytical and sensory databases in order to standardize, homogenize, and quantitatively code the odor descriptors of the odorants. Then the standardized odor descriptors are translated into a limited number of odor qualities used by the experts thanks to an ontology. A third step consists of aggregating all the information in terms of odor qualities across all the odorants found in a given product. The final step is a set of knowledge-based fuzzy membership functions representing the flavorist expertise and ensuring the prediction of the intensity of the target odor sensory descriptors on the basis of the products' aggregated odor qualities; several methods of optimization of the fuzzy membership functions have been tested. Finally, the model was applied to predict the odor profile of 16 red wines from two grape varieties for which the content in odorants was available. The results showed that the model can predict the perceptual outcome of food odor with a certain level of accuracy, and may also provide insights into combinations of odorants not mentioned by the experts.
We consider a mixture of mesoscale size particles within an ambient fluid that contains locally aligned microscopic scale rod-like molecules, that is a nematic liquid crystals. This type of mixture, which is usually referred to as a nematic colloid material, has emerged in the recent years as the material of choice for testing a number of exciting hypothesis in the design of new materials. An overview of the field, and its applications, from the physical point of view is available in the reviews [17,27].
The mathematical studies of such systems are still relatively few and focus on two extreme situations:
● the effect produced by one colloidal particle, particularly related to the so-called 'defect patterns' that is the strong distortions produced at the interface between the particle and the ambient nematic fluid;
● the collective effects produced by the presence of many particle, fairly uniformly distributed, with a focus on the homogenised material.
In the first direction one should note that defects appear because of the anchoring conditions at the boundary of the particles, which generate topological obstructions [1,2,8,9,10,11,28]. Indeed, there have been a number of works, identifying several physically relevant regimes [1] and the influence of external fields [2].
Our work focuses on the second direction, namely on long-scale effects produced by the effects of a large number of particles, namely on the homogenisation regime. There have been a couple of works in this direction, on which our builds, namely [5,6,7]. The main novelty of our approach, compared to those in [5,6,7], is that we allow for a much larger class of surface energy densities. We do not assume that the surface energy density is bounded from below and we do consider surface energy densities of quartic growth, which is the maximal growth compatible with the Sobolev embeddings. Surface energy densities of quartic growth have been proposed in the physical literature [3,15,24,26].
We focus on a regime in which the total volume of the particles is much smaller than that of the ambient nematic environment, known as the dilute regime. Our aim is to provide a mathematical understanding of statements from the physical literature e.g., [19,25] showing that in such a regime the colloidal nematics behave like a homogenised, standard nematic material, but with different (better) properties than those of the original nematic material.
In our previous work [12], we provided a first approach to these issues and we showed that using periodically distributed identical particles, one can design a suitable surface energy to obtain an apriori designed potential, that models the main physical properties of the material (in particular the nematic-isotropic transition temperature).
The purpose of these notes is two-fold: on the one hand, to extend the main results of [12] to polydisperse and inhomogenoeus nematic colloids; on the other hand, to explore a regime of parameters that differs from the one considered in [12].
Realistically, a set of colloidal inclusions will hardly be identical: The particles will differ in their size, shape, or charge. In order to account for polydispersity, we will consider several populations of colloidal inclusions, which may differ in their shape and properties. Moreover, we will not require the centres of mass of the inclusions to be homogeneously distributed in space. In mathematical terms, let P1, P2, …, PJ be subsets of R3 (the reference shapes of the inclusions), and let Ω⊆R3 be a bounded, smooth domain (the container). We define
Pjε:=Njε⋃i=1(xi,jε+εαRi,jεPj)forj∈{1,…,J}, | (1.1) |
where α is a positive number, the xi,jε's are points in Ω and the Ri,jε are rotation matrices that satisfy suitable assumptions (see Section 2.1). As in [7,12], we work in the dilute regime, namely we assume that α>1 so that the total volume occupied by the inclusions, |Pε|≈ε3α−3, tends to zero as ε→0. However, we also assume that α<3/2 so that the total surface area of the inclusions, σ(∂Pε)≈ε2α−3, diverges as ε→0. We define Pε:=∪jPjε and Ωε:=Ω∖Pε (the space that is effectively occupied by the nematic liquid crystal). In accordance with the Landau-de Gennes theory, the nematic liquid crystal is described by a tensorial order parameter, that is, a symmetric, trace-free (3×3)-matrix field Q. We consider the free energy functional
Fε[Q]:=∫Ωε(fe(∇Q)+fb(Q))dx+ε3−2αJ∑j=1∫∂Pjεfjs(Q,ν)dσ. | (1.2) |
Here, fe, fb are suitable elastic and bulk energy densities (in the Landau-de Gennes theory, fe is typically a positive definite, quadratic form in ∇Q and fb is a quartic polynomial in Q; see Section 2.1), fjs is a surface anchoring energy densities (which may vary for different species of inclusions), and ν denotes the exterior unit normal to Ω. We prove a convergence result for local minimisers of Fε to local minimsers of the effective free energy functional:
F0[Q]:=∫Ω(fe(∇Q)+fb(Q)+fhom(Q,x))dx. |
The "homogenised potential" fhom, which keeps memory of the surface integral, is explicitly computable in terms of the fjs's, the distribution of the centres of mass xi,jε and the rotations Ri,jε. As an application of this result, we show that polydisperse inclusions may be used to mimic the effects of an applied electric field. More precisely, for a pre-assigned parameter W∈R and a pre-assigned symmetric matrix P, we may tune the shape Pjε of the inclusions and the surface energy densities, so to have in the limit
fhom(Q)=Wtr(QP). |
When P has the form P=E⊗E for some E∈R3, this expression may be interpreted as an electrostatic energy density induced by the "effective field" E, up to terms that do not depend on Q.
Moving beyond the issue of polydispersity we consider another physically restrictive assumption we made in [12], namely concerning the anchoring strength. In (1.2), the scaling of parameters is chosen so to have a factor of ε3−2α in front of the surface integral, which compensates exactly the growth of the surface area, σ(∂Pε)≈ε2α−3. However, other choices of the scaling are possible, corresponding to different choices of the anchoring strength at the boundary of the inclusions. One can easily check that having a weaker anchoring, say of the type ε2α−3+δ with δ>0 will lead to a vanishing of the homogenized term, so the main interest is to understand what happens for stronger anchoring.To illustrate this possibility, we study the asymptotic behaviour, as ε→0, of minimisers of
Fε,γ[Q]:=∫Ωε(fe(∇Q)+fb(Q))dx+ε3−2α−γJ∑j=1∫∂Pjεfjs(Q,ν)dσ, |
where γ is a positive parameter. This scaling corresponds to a much stronger surface anchoring and we expect the behaviour of minimisers to be dominated by the surface energy, as ε→0. Indeed, we will show that for γ small enough the functionals Fε,γ Γ-converge to the constrained functional
˜F(Q):={∫Ω(fe(∇Q)+fb(Q))dxiffhom(Q(x),x)=0fora.e.x∈Ω+∞otherwise, |
as ε→0.
This result leaves a number of interesting of open problems, the most immediate ones being what is the optimal range of γ for which this holds and, directly related to this, if one gets a different limit for large values of γ.
The paper is organized as follows: in the following, in Section 2.1 we consider the polydisperse setting and the general homogenisation result. The main results of this section, namely Theorem 2.1 and Proposition 2.2 are presented after the introduction of the mathematical setting, in Subsection 2.1. The proof of the results is provided in Subsection 2.3 after a number of preliminary results, need in the proof, provided in Subsection 2.2.
In Section 3 we provide an application of the results in Section 2.1, namely showing that, in a polydispersive regime, one can obtain a linear term in the homogenised potential (Proposition 3.2).
Finally, in Section 4 we study the case when the scaling of the anchoring strength is ε3−2α−γ with γ suitably small, and provide in Theorem 4.3 the Γ-converegence result mentioned above. Its proof is done at the end of the section after a number of preliminary results.
The Landau-de Gennes Q-tensor. In the Landau-de Gennes theory, the local configuration of a nematic liquid crystal is represented by a symmetric, symmetric, trace-free, real (3×3)-matrix, known as the Q-tensor, which describes the anisotropic optical properties of the medium [13,21]. We denote by S0 the set of matrix as above. For Q, P∈S0, we denote Q⋅P:=tr(QP). This defines a scalar product on S0, and the corresponding norm will be denoted by |Q|:=(tr(Q2))1/2=(∑i,jQij)1/2.
The domain. let P1, P2, …, PJ be subsets of R3 (the reference shapes of the inclusions), and let Ω⊆R3 be a bounded, smooth domain (the container). We define Pjε as in (1.1), where α, xi,jε, Ri,jε satisfy the following assumptions:
H1. 1<α<3/2.
H2. There exists a constant λΩ>0 such that
dist(xi,jε,∂Ω)+12inf(h,k)≠(i,j)|xh,kε−xi,jε|≥λΩε |
for any ε>0, any j∈{1,…,J} and any i∈{1,…,Njε}.
H3. For any j∈{1,…,J}, there exists a non-negative function ξj∈L∞(Ω), such that
μjε:=ε3Njε∑i=1δxi,jε⇀∗ξjdxasmeasuresinR3,asε→0. |
H4. For any j∈{1,…,J}, there exists a Lipschitz-continuous map Rj∗:¯Ω→SO(3) such that Ri,jε=Rj∗(xi,jε) for any ε>0 and any i∈{1,…,Njε}.
H5. For any j∈{1,…,J}, Pj⊆R3 is a compact, convex set whose interior contains the origin.
The assumption (H2) is a separation condition on the inclusions. As a consequence of (H2), the number of the inclusions, for each population j, is Njε≲ε−3. Therefore, the total volume of the inclusions in each population is bounded by Njεε3≲ε3α−3→0, because of (H1). Thus, we are in the diluted regime, as in [7,12]. We define
Pε:=J⋃j=1Pjε,Ωε:=Ω∖Pε. |
The assumption H5 guarantees that Ωε is a Lipschitz domain.
The free energy functional. For Q∈H1(Ωε,S0), we consider the free energy functional
Fε[Q]:=∫Ωε(fe(∇Q)+fb(Q))dx+ε3−2αJ∑j=1∫∂Pjεfjs(Q,ν)dσ. | (2.1) |
The surface anchoring energy densities depend on j, as colloids that belong to different populations may have different surface properties. For the rest, our assumptions for the elastic energy density fe, bulk energy density fb, and surface energy densities fjs are the same as in [12]. We say that a function f:S0⊗R3→R is strongly convex if there exists θ>0 such that the function S0⊗R3∋D↦f(D)−θ|D|2 is convex.
H6. fe:S0⊗R3→[0,+∞) is differentiable and strongly convex. Moreover, there exists a constant λe>0 such that
λ−1e|D|2≤fe(D)≤λe|D|2,|(∇fe)(D)|≤λe(|D|+1) |
for any D∈S0⊗R3.
H7. fb:S0→R is continuous, non-negative and there exists λb>0 such that 0≤fb(Q)≤λb(|Q|6+1) for any Q∈S0.
H8. For any j∈{1,…,J}, the function fjs:S0×S2→R is locally Lipschitz-continuous. Moreover, there exists a constant λs>0 such that
|fjs(Q1,ν1)−fjs(Q2,ν2)|≤λs(|Q1|3+|Q2|3+1)(|Q1−Q2|+|ν1−ν2|) |
for any j∈{1,…,J} and any (Q1,ν1), (Q2,ν2) in S0×S2.
A physically relevant example of elastic energy density fe that satisfies (H6) is given by
fLdGe(∇Q):=L1∂kQij∂kQij+L2∂jQij∂kQik+L3∂jQik∂kQij | (2.2) |
(Einstein's summation convention is assumed), so long as the coefficients L1, L2, L3 satisfy
L1>0,−L1<L3<2L1,−35L1−110L3<L2 | (2.3) |
(see e.g., [13,20]). The assumption (H7) is satisfied by the quartic Landau-de Gennes bulk potential, given by
fLdGb(Q):=atr(Q2)−btr(Q3)+c(tr(Q2))2+κ(a,b,c) |
where a∈R, b>0, c>0 are coefficients depending on the material and the temperature and κ(a,b,c)∈R is a constant, chosen in such a way that inffLdGb=0. An example of surface energy density that satisfies (H8) is the Rapini-Papoular type energy density:
fs(Q,ν):=Wtr(Q−Qν)2withQν:=ν⊗ν−Id3 |
and W a (typically positive) parameter. However, (H8) allows for much more general surface energy densities, which may not be positive and may have up to quartic growth in Q (for examples, see e.g., [3,15,24,26] and the references therein). In addition to (H8), physically relevant surface energy densities must satisfy symmetry properties (frame-indifference, invariance with respect to the sign of ν) but these will play no rôle in our analysis.
The homogenised potential. For any j∈{1,…,J}, let us define fjhom:S0ׯΩ→R as
fjhom(Q,x):=∫∂Pjfjs(Q,Rj∗(x)νPj)dσfor(Q,x)∈S0ׯΩ, | (2.4) |
where νPj denotes the inward-pointing unit normal to ∂Pj, and Rj∗:¯Ω→SO(3) is the map given by (H4). Finally, let
fhom(Q,x):=J∑j=1ξj(x)fjhom(Q,x)for(Q,x)∈S0ׯΩ, | (2.5) |
where ξj∈L∞(Ω) is the function given by (H3). Our candidate homogenised functional is defined for any Q∈H1(Ω,S0) as
F0[Q]:=∫Ω(fe(∇Q)+fb(Q)+fhom(Q,x))dx. | (2.6) |
The convergence result. The assumptions (H1)–(H8) are not enough to guarantee that global minimisers of Fε exist and actually, it may happen that Fε is unbounded from below [12, Lemma 3.6]. Instead, our main result focus on the asymptotic behaviour of local minimisers. Given g∈H1/2(∂Ω,S0), we let H1g(Ωε,S0) — respectively, H1g(Ω,S0) — be the set of maps Q∈H1(Ωε,S0) — respectively, Q∈H1(Ω,S0) — that satisfy Q=g on ∂Ω, in the sense of traces. For each Q∈H1g(Ωε,S0), we define the map EεQ∈H1g(Ω,S0) by EεQ:=Q on Ωε and EεQ:=Qi,jε on Pi,jε, where Qi,jε is the unique solution of Laplace's problem
{−ΔQi,jε=0inPi,jεQi,jε=Qon∂Piε. | (2.7) |
Theorem 2.1. Suppose that the assumptions (H1)–(H8) are satisfied. Suppose, moreover, that Q0∈H1g(Ω,S0) is an isolated H1-local minimiser for F0 — that is, there exists δ0>0 such that
F0[Q0]<F0[Q] |
for any Q∈H1g(Ω,S0) such that Q≠Q0 and ‖Q−Q0‖H1(Ω)≤δ0. Then, for any ε small enough, there exists an H1-local minimiser Qε for Fε such that EεQε→Q0 strongly in H1(Ω) as ε→0.
The proof of Theorem 2.1 follows a variational approach, and is based on the following fact:
Proposition 2.2. Let Qε∈H1g(Ωε,S0) be such that EεQε⇀Q weakly in H1(Ω) as ε→0. Then, there holds
∫Ω(fe(∇Q)+fb(Q))dx≤lim infε→0∫Ωε(fe(∇Qε)+fb(Qε))dx | (2.8) |
∫Ωfhom(Q,x)dx=limε→0ε3−2αJ∑j=1∫∂Pjεfjs(Qε,ν)dσ. | (2.9) |
Proposition 2.2 can be reformulated as a Γ-convergence result. Indeed, from Proposition 2.2 we immediately have F0≤Γ−lim infε→0Fε (with respect to a suitable topology, induced by the operator Eε). A trivial recovery sequence suffices to obtain the opposite Γ-lim sup inequality, thanks to (2.9) and the fact that in the dilute limit, |Pε|→0. Theorem 2.1 follows from Proposition 2.2 by general properties of the Γ-convergence.
Throughout the paper, we will write A≲B as a short-hand for A≤CB, where C is a positive constant, depending only on the domain, the boundary datum and the free energy functional (2.1), but not on ε.
The main technical tool is the following trace inequality, which is adapted from [7,Lemma 4.1].
Lemma 2.3 ([12,Lemma 3.1]). Let P⊆R3 be a compact, convex set whose interior contains the origin. Then, there exists a constant C=C(P)>0 such that, for any a>0, b≥2a and any u∈H1(bP∖aP), there holds
∫∂(aP)|u|4dσ≤C∫bP∖aP(|∇u|2+|u|6)dx+Ca2b3∫bP∖aP|u|4dx. |
Given an inclusion Pi,jε=xi,jε+εαRi,jεPj, we consider ˆPi,jε:=xi,jε+μεRi,jεPj, where μ>0 is a small (but fixed) parameter. By taking μ small enough, we can make sure that the ˆPi,jε's are pairwise disjoint. Then, by applying Lemma 2.3 component-wise on ˆPi,jε∖Pi,jε and summing the corresponding inequalities over i and j, we deduce
Lemma 2.4. For any Q∈H1(Ωε,S0), there holds
ε3−2α∫∂Pε|Q|4dσ≲ε3−2α∫Ωε(|∇Q|2+|Q|6)dx+∫Ωε|Q|4dx. |
Another tool is the harmonic extension operator, Eε:H1(Ωε,S0)→H1(Ω,S0), defined by (2.7).
Lemma 2.5. The operator Eε:H1(Ωε,S0)→H1(Ω,S0) satisfies the following properties.
(i). There exists a constant C>0 such that ‖∇(EεQ)‖L2(Ω)≤C‖∇Q‖L2(Ωε) for any ε>0 and any Q∈H1(Ωε,S0).
(ii). If the maps Qε∈H1(Ω,S) converge H1(Ω)-strongly to Q as ε→0, then Eε(Qε|Ωε)→Q strongly in H1(Ω) as ε→0, too.
Proof. For any i, j, consider the inclusion Pi,jε=xi,jε+εαRi,jεPj and let Ri,jε:=xi,jε+2εαRi,jεPj. Let Rε:=∪i,jRi,jε. The properties of Laplace equation, combined with a scaling argument (see, e.g., [12,Lemma 3.4]), imply that
‖∇(EεQ)‖L2(Pε)≲‖∇Q‖L2(Rε∖Pε). | (2.10) |
Statement (ⅰ) then follows immediately. To prove Statement (ⅱ), take a sequence Qε∈H1(Ω,S0) that converges strongly to Q as ε→0. Then,
‖∇Qε−∇(Eε(Qε|Ωε))‖L2(Ω)≤‖∇Qε‖L2(Pε)+‖∇(Eε(Qε|Ωε))‖L2(Pε)(2.10)≲‖∇Qε‖L2(Rε)≲‖∇Q‖L2(Rε)+‖∇Q−∇Qε‖L2(Ω). |
Both terms in the right-hand side converge to 0 as ε→0, because |Rε|≲ε3α−3→0, and Statement (ii) follows.
The proof of Theorem 2.1 is largely similar to that of [12, Theorem 1.1]. We reproduce here only some steps of the proof, either because there require a modification or because they will be useful in Section 4.
Remarks on the lower semi-continuity of Fε. Even before we address the asymptotic analysis as ε→0, we should make sure that, for fixed ε>0, the functional Fε is sequentially lower semi-continuous with respect to the weak topology on H1(Ωε,S0). If the surface energy density fs is bounded from below, then the surface integral is lower semi-continuous by Fatou lemma. If fs has subcritical growth, that is |fs(Q)|≲|Q|p+1 for some p<4, then the lower semi-continuity of the surface integral follows from the compact Sobolev embedding H1/2(∂Ωε,S0)↪Lp(∂Ωε,S0) and Lebesgue's dominated convergence theorem. However, our assumption (H8) allows for surface energy densities that have quartic growth and are unbounded from below, e.g. fs(Q):=−|Q|4. In this case, the surface integral alone may not be sequentially weakly lower-semi continuous [12, Lemma 3.10]. However, lower semi-continuity may be restored at least on bounded subsets of H1(Ωε,S0), when ε is small:
Proposition 2.6 ([12,Proposition 3.9]). Suppose that the assumptions (H1)–(H8) are satisfied. For any M>0 there exists ε0(M)>0 such that following statement holds: if 0<ε≤ε0(M), Qk⇀Q weakly in H1g(Ωε,S0) and if ‖∇Qk‖L2(Ωε)≤M for any k, then
Fε[Q]≤lim infk→+∞Fε[Qk]. |
The proof carries over from [12], almost word by word, using Lemma 2.4. Essentially, the loss of lower semi-continuity that may arise from the surface integral can be quantified, with the help of Lemma 2.4 and the bound on ∇Qk. However, since the surface integral is multiplied by a small factor ε3−2α, this loss of lower semi-continuity is compensated by the strong convexity of the elastic term fe, for ε sufficiently small.
Pointwise convergence of the surface energy terms. For ease of notation, let us define
Jε[Q]:=ε3−2αJ∑j=1∫∂Pjεfjs(Q,ν)dσ | (2.11) |
J0[Q]:=∫Ωfhom(Q,x)dxforQ∈H1g(Ω,S0) | (2.12) |
We state some properties of the functions fjhom:S0ׯΩ→R, fhom:S0ׯΩ→R, defined by (2.5), (2.4) respectively.
Lemma 2.7. For any j∈{1,…,J}, the function fjhom is locally Lispchitz-continuous, and there holds
|fjhom(Q,x)|≲|Q|4+1,|∇fjhom(Q,x)|≲|Q|3+1 | (2.13) |
for any (Q,x)∈S0ׯΩ. Moroever, the function fhom satisfies
|fhom(Q1,x)−fhom(Q2,x)|≲(|Q1|3+|Q2|3+1)|Q1−Q2| | (2.14) |
for any Q1, Q2∈S0 and any x∈¯Ω.
Proof. Using the definition (2.4) of fjhom, and the assumption (H8), we obtain
|fjhom(Q1,x1)−fjhom(Q2,x2)|≤∫∂Pj|fjs(Q1,Rj∗(x1)νPj)−fjs(Q2,Rj∗(x2)νPj)|dσ≲∫∂Pj(|Q1|3+|Q2|3+1)(|Q1−Q2|+|(Rj∗(x1)−Rj∗(x2))νPj|)dσ |
Since Rj∗ is Lipschitz-continuous by (H4), we deduce
|fjhom(Q1,x1)−fjhom(Q2,x2)|≲(|Q1|3+|Q2|3+1)(|Q1−Q2|+|x1−x2|) | (2.15) |
and (2.14) follows. We multiply the previous inequality by ξj, where ξj is given by (H3), take x1=x2=x, and sum over j. Since ξj∈L∞(Ω) by Assumption (H3), we obtain
|fhom(Q1,x)−fhom(Q2,x)|(2.5)≤J∑j=1‖ξj‖L∞(Ω)|fjhom(Q1,x)−fjhom(Q2,x)|≲(|Q1|3+|Q2|3+1)|Q1−Q2|. |
Let us introduce the auxiliary quantity
˜Jε[Q]:=ε3−2αJ∑j=1Njε∑i=1∫∂Pi,jεfjs(Q(xi,jε),ν)dσ. | (2.16) |
Lemma 2.8. For any bounded, Lipschitz map Q:¯Ω→S0, there holds
˜Jε[Q]=J∑j=1∫R3fjhom(Q(x),x)dμjε(x) | (2.17) |
(where the measures μjε are defined by (H3)), and
|Jε[Q]−˜Jε[Q]|≲εα(‖Q‖3L∞(Ω)+1)‖∇Q‖L∞(Ω). | (2.18) |
Proof. For any i and j, consider the single inclusion Pi,jε:=xi,jε+εαRi,jεPjε. Since ν(x)=Ri,jενPj(ε−α(Ri,jε)T(x−xi,jε)) for any x∈∂Pi,jε, by a change of variable we obtain
˜Jε[Q]=ε3J∑j=1Njε∑i=1∫∂Pjfjs(Q(xi,jε),Ri,jενP)dσ(H4)=ε3J∑j=1Njε∑i=1∫∂Pjfjs(Q(xi,jε),Rj∗(xi,jε)νP)dσ(2.5)=ε3J∑j=1Njε∑i=1fjhom(Q(xi,jε),xi,jε). |
Now, (2.17) follows from the definition of μjε, (H3). On the other hand, by decomposing the integral on ∂Pj as a sum of integrals over the boundary of each inclusion, we obtain
|Jε[Q]−˜Jε[Q]|≲ε3−2αJ∑j=1Njε∑i=1∫∂Pi,jε|fjs(Q(x),ν)−fjs(Q(xi,jε),ν)|dσ(x)(H8)≲ε3−2αNjε∑i=1J∑j=1∫∂Pi,jε(|Q(x)|3+|Q(xi,jε)|3+1)|Q(x)−Q(xi,jε)|dσ(x) |
Since Q is assumed to be Lipschitz continuous and the diameter of Pi,jε is ≲εα, we have |Q(x)−Q(xi,jε)|≲εα‖∇Q‖L∞(Ω). This implies
|Jε[Q]−˜Jε[Q]|≲ε3−ασ(∂Pε)(‖Q‖3L∞(R3)+1)‖∇Q‖L∞(Ω). |
Finally, we note that σ(∂Pε)≲ε2α−3, because there are O(ε−3) inclusions (as a consequence of (H2)) and the surface area of each inclusion is O(ε2α). Thus, the lemma follows.
Since μjε⇀∗ξjdx by Assumption (H3), as an immediate consequence of Lemma 2.8 and (2.5), (2.12) we obtain
Proposition 2.9. For any bounded, Lipschitz map Q:¯Ω→S0, there holds Jε[Q]→J0[Q] as ε→0.
Once Proposition 2.9 is proved, the rest of the proof of Proposition 2.2 and Theorem 2.1 follows exactly as in [12].
Proposition 3.1. There exist (possibly disconnected) shapes Pk⊂R3, k∈{1,2,3,4,5,6} such that taking as surface energy the Rapini-Papoular surface energy fs(Q,ν)=tr(Q−Qν)2 with Qν=ν⊗ν−13Id where ν is the exterior unit-normal, we have:
fPkhom(Q)=(23+tr(Q2))σ(∂Pk)−2tr(QMk) | (3.1) |
where
Mk=(π3+π2)Id−π2ek⊗ek,k∈{1,2,3} |
M4=(π3+π2)Id−π2e3⊗e3+23(e1⊗e2+e2⊗e1), |
M5=(π3+π2)Id−π2e2⊗e2+23(e1⊗e3+e3⊗e1), |
M6=(π3+π2)Id−π2e1⊗e1+23(e2⊗e3+e3⊗e2), |
with Mk,k∈{1,2,3,4,5,6} a basis in the linear space of 3×3 symmetric matrices.
Proof. By formula (2.4) we have:
fPkhom(Q)=∫∂Pk(tr(Q2)−2tr(QQν)+tr(Qν)2)dσ | (3.2) |
hence we readily get (3.1) with Mk=∫∂Pkν(x)⊗ν(x)dσ(x).
Let us take for 1≤i,j≤3 with i≠j the 'potato wedges' domains
Ω+ij:={x=(x1,x2,x3)∈R3:|x|≤1, xi≥0, xj≥0} | (3.3) |
as candidates for 'parts of' our shapes Pk's (see Figure 1).
We calculate the term
∫∂Ω+12ν⊗νdσ=∫Ω+12∩{x1=0}ν⊗νdσ⏟:=I1+∫Ω+12∩{x2=0}ν⊗νdσ⏟:=I2+∫∂Ω+12∩{x1⋅x2>0}ν⊗νdσ⏟:=I3 |
Then:
I1=e1⊗e1∫{x22+x23≤1,x1=0}dσ=π2e1⊗e1 | (3.4) |
where e1:=(1,0,0). Similarly we get I2=π2e2⊗e2 with e2:=(0,1,0). Finally:
(I3)ij=∫∂Ω+12∩{x1⋅x2>0}xixjdσ(x),∀i,j∈{1,2,3}. | (3.5) |
Because x1x3, respectively x2x3 are odd functions in the variable x3 along the domain Ω+12∩{x1⋅x2>0} we have that (I3)13=(I3)31=(I3)23=(I3)32=0. Furthermore:
(I3)12=(I3)21=∫∂Ω+12∩{x1⋅x2>0}x1x2dσx=∫π0(∫π20sin3θcosφsinφdφ)dθ=∫π0sin3θdθ⋅∫π20cosφsinφdφ=43⋅12=23 | (3.6) |
Similarly we get: (I3)11=(I3)22=(I3)33=π3. Summarizing the last calculations, we get:
∫∂Ω+12ν⊗νdσ=(π3+π223023π3+π2000π3) | (3.7) |
Analogous calculations provide
∫∂Ω+13ν⊗νdσ=(π3+π20230π30230π3+π2) | (3.8) |
∫∂Ω+23ν⊗νdσ=(π3000π3+π223023π3+π2) | (3.9) |
Similarly we define, for 1≤i<j≤3,
Ω−ij:={x=(x1,x2,x3)∈R3:|x|≤1, xi≤0, xj≥0} | (3.10) |
and we have:
∫∂Ω−12ν⊗νdσ=(π3+π2−230−23π3+π2000π3) | (3.11) |
respectively
∫∂Ω−13ν⊗νdσ=(π3+π20−230π30−230π3+π2) | (3.12) |
∫∂Ω−23ν⊗νdσ=(π3000π3+π2−230−23π3+π2) | (3.13) |
We take then:
P1:=Ω+23∪(Ω−23−(0,1,0)),P2:=Ω+13∪(Ω−13−(1,0,0)),P3:=Ω+12∪(Ω−12−(1,0,0)) |
and, respectively
P4:=Ω+12,P5:=Ω+13,P6:=Ω+23. |
Proposition 3.2. Let P be a 3×3 symmetric matrix, not necessarily traceless, and W∈R. There exists a family of JP∈N shapes Pj and corresponding surface energy strengths ij,j∈{1,…,JP} such that, taking for each shape the Rapini-Papoular surface energy with corresponding intensity ij, i.e.,
fjs(Q,ν)=Wijtr(Q−Qν)2 | (3.14) |
with Qν:=ν⊗ν−13Id and ν is the exterior unit-normal, the corresponding homogenised potential is:
fPhom(Q)=−WαP(13+12tr(Q2))+Wtr(QP) | (3.15) |
with αP∈R explicitly computable in terms of the shapes volumes and the surface energy strengths.
Proof. We take Mk, k∈{1,…,6}, as provided in Proposition 3.1, to be a linear basis in the spaces of 3×3 symmetric matrices. Then there exists ak:=P⋅Mk, k∈{1,…,6} such that
P=6∑k=1akMk |
Let P1,…,PJP be the connected components of P1,…,Pk. Each Pj is a compact, convex set of the form (3.3) or (3.10) (see Figure 1). For j∈{1,…,JP}, we define the corresponding intensities as ij=−12ak where k=k(j) is such that Pj⊆Pk. Then, noting that the homogenised potentials corresponding to each species will add together to provide the homogenised porential corresponding to all the species, we get:
fPhom(Q)=−127∑k=1akfPkhom(Q)=−W(13+12tr(Q2))6∑k=1ikσ(∂Pk)+Wtr(QP) | (3.16) |
hence we obtain the claimed (3.15) with αP:=∑6k=1ikσ(∂Pk).
Remark 3.3. We can, without loss of generality, drop the constant term in a bulk potential, since adding a constant to an energy functional does not change the minimiser. In particular in fPhom we can ignore the term −W3αP.
We wish now to choose the surface energy densities fjs of Rapini-Papoular type and the shapes of the colloidal particles, in such a way that given the symmetric 3×3 matrix P and W∈R, local minimisers of the Landau-de Gennes functional
Fε[Q]=∫Ωε(fLdGe(∇Q)+atr(Q2)−btr(Q3)+c(tr(Q2))2)dx+ε3−2αJ∑j=1∫∂Pjεfjs(Q,ν)dσ | (3.17) |
(with fLdGe given by (2.2)) converge to local minimisers of the homogenised functional
F0[Q]=∫Ω(fLdGe(∇Q)+a′tr(Q2)−btr(Q3)+c(tr(Q2))2+Wtr(PQ))dx. | (3.18) |
We will assume that 1<α<3/2 and the centres of the inclusions, xi,jε, satisfy (H2) and that they are uniformly distributed, i.e., they satify (H3) with ξj=1. We also assume that all inclusions of the same family are parallel to each other, that is, we take Ri,jε=Id for any i, j, ε (in particular, (H4) is satisfied with Rj∗=Id).
Remark 3.4. One could also choose colloidal particles and corresponding surface energies that modify the b and c coefficients, but for this it would not suffice to use Rapini-Papoular type of surface energies (see for instance Section 2.2 in [12]).
Corollary 3.5. Let (a,b,c)∈R3 with c>0. Let a′∈R, W>0, and let P be a symmetric, 3×3 matrix. Suppose that the inequalities (2.3) are satisfied. Then, there exists a family of shapes Pj and a corresponding surface energy fjs for each of them, such that for any isolated local minimiser Q0 of the functional F0 defined by (3.18), and for ε>0 small enough, there exists a local minimiser Qε of the functional Fε, defined by (3.17), such that EεQε→Q0 strongly in H1(Ω,S0).
Proof. This statement is a particular case of our main result, Theorem 2.1. If (2.3) holds and c>0, c′>0, then the conditions (H6)–(H7) are satisfied.
We take JP species Pj,j∈{1,…,JP} and surface energies given by (3.14), as in Proposition 3.1. Each Pj is a compact, convex set of the form (3.3) or (3.10), so (H5) is satisfied (up to translations) and (H8) is satisfied too. The homogenised potential corresponding to these is:
fPhom(Q)=−WαP(13+12tr(Q2))+Wtr(QP) | (3.19) |
where αP:=∑6k=1P⋅Mk2σ(∂Pk) (and the Mk,k∈{1,…,6} are those from Proposition 3.1). We further take one more species, of spherical colloids PJP+1:=B1, and define the surface energy density
fJP+1s(Q,ν):=14π(a′+WαP2−a)(ν⋅Q2ν). | (3.20) |
This produces (see also for instance Remark 2.9 in [12]) a homogenised potential
fsphhom(Q):=(a′+WαP2−a)tr(Q2) |
Then the homogenised potential for all the JP+1 species is
fhom(Q)=fsphhom(Q)+fPhom(Q)=(a′−a)tr(Q2)+btr(Q3)+c(tr(Q2))2+Wtr(PQ)−W3αP |
and, since we can, without loss of generality, see Remark 3.3 drop the constant term −W3αP, the corollary follows from Theorem 2.1.
The purpose of this section is to study the asymptotic behaviour, as ε→0, of minimisers of a functional with a different choice of the scaling for the surface anchoring strength. We consider the free energy functional:
Fε,γ[Q]:=∫Ωε(fe(∇Q)+fb(Q))dx+ε3−2α−γJ∑j=1∫∂Pjεfjs(Q,ν)dσ. | (4.1) |
(where ν(x) denotes as usually the exterior normal at the point x on the boundary), with α∈(1,32) and
K1. 0<γ<1/4.
Due to the extra factor ε−γ in front of the surface integral, we cannot apply Proposition 2.6 to obtain the lower semi-continuity of Fε,γ for fixed ε. Therefore, in contrast with the previous sections, we assume boundedness from below on the surface term.
K2. fs≥0.
Remark 4.1. Under the assumption (K2), the sequential weak lower semi-continuity of Fε (for fixed ε) follows from the compact embedding H1/2(∂Ωε)↪L2(∂Ωε) and Fatou's lemma. Therefore, a routine application of the direct method of the Calculus of Variations shows that minimisers of Fε exist, for any ε>0.
As a consequence of (K2) and of (H3), the function fhom is non-negative, too. In fact, we will also assume that
K3. inf{fhom(Q,x):Q∈S0}=0 for any x∈¯Ω.
Recall that, for any j∈{1,…,J}, the measures μjε:=ε−3∑iδxi,jε are supposed to converge weakly∗ to a non-negative function ξj∈L∞(Ω). We need to prescribe a rate of convergence. We express the rate of convergence in terms of the W−1,1-norm (that is, the dual Lipschitz norm, also known as flat norm in some contexts):
Fε:=maxj=1,2,…,Jsup{∫Ωφdμjε−∫Ωφξjdx:φ∈W1,∞(Ω),‖∇φ‖L∞(Ω)+‖φ‖L∞(Ω)≤1}. | (4.2) |
K4. There exists a constant λflat>0 such that Fε≤λflatε for any ε.
Remark 4.2. The assumption (K4) is satisfied if the inclusions are periodically distributed. Consider, for simplicity, the case J=1, and suppose that the centres of the inclusions, xiε, are exactly the points y∈(εZ)3 such that y+[−ε/2,ε/2]3⊆Ω. Let Ωε:=∪i(xiε+[−ε/2,ε/2]3). Then, for any φ∈W1,∞(Ω), we have
|∫Ωφdμε−∫Ωφdx|≤Nε∑i=1∫xiε+[−ε/2,ε/2]3|φ−φ(xiε)|+∫Ω∖Ωε|φ|≤√3ε2‖∇φ‖L∞(Ω)|Ωε|+‖φ‖L∞(Ω)|Ω∖Ωε|. |
Moreover, |Ω∖Ωε|≲ε, because Ω∖Ωε⊆{y∈Ω:dist(y,∂Ω)≤√3ε}. Therefore, (K4) holds.
Finally, we assume some regularity on the boundary datum g:∂Ω→S0.
K5. g is bounded and Lipschitz.
Γ-convergence to a constrained problem. We can now define the candidate limit functional. Let
A:={Q∈H1g(Ω,S0):fhom(x,Q(x))=0 fora.e.x∈Ω}, | (4.3) |
and ˜F:L2(Ω,S0)→(−∞,+∞],
˜F(Q):={∫Ω(fe(∇Q)+fb(Q))dxifQ∈A+∞otherwise. |
Theorem 4.3. Suppose that the assumptions (H1)–(H8), (K1)–(K5) are satisfied. Then, the following statements hold.
i. Given a family of maps Qε∈H1g(Ωε,S0) such that supεFε,γ(Q)<+∞, there exists a non-relabelled sequence and Q0∈A such that EεQε⇀Q0 weakly in H1(Ω),
˜F(Q0)≤lim infε→0Fε,γ(Qε). |
ii. For any Q0∈A, there exists a sequence of maps Qε∈H1g(Ωε,S0) such that EεQε→Q0 strongly in H1(Ω) and
lim supε→0Fε,γ(Qε)≤˜F(Q0). |
Remark 4.4. The theorem is only meaningful when A is non-empty, and it may happen that A is empty even if fhom(g(x),x)=0 for any x∈∂Ω.
Before we give the proof of Theorem 4.3, we state some auxiliary results. We first recall some properties of the convolution, which will be useful in constructing the recovery sequence.
Lemma 4.5. For any P∈H1(R3,S0) and σ>0, there exists a smooth map Pσ:R3→S0 that satisfies the following properties:
‖Pσ‖L∞(R3)≲σ−1/2‖P‖L6(R3),‖∇Pσ‖L∞(R3)≲σ−3/2‖∇P‖L2(R3) | (4.4) |
‖P−Pσ‖L2(R3)≲σ‖∇P‖L2(R3) | (4.5) |
‖∇P−∇Pσ‖L2(R3)→0asσ→0. | (4.6) |
Moreover, if U⊆U′ are Borel subsets of R3 such that dist(U,R3∖U′)>σ, then
‖Pσ‖L2(U)≤‖P‖L2(U′). | (4.7) |
Proof. Let us take a non-negative, even function φ∈C∞c(R3), supported in the unit ball B1, such that ‖φ‖L1(R3)=1. Let φσ(x):=σ−3φ(x/σ). By a change of variable, we see that
‖φσ‖Lp(R3)=σ3/p−3‖φ‖Lp(R3)foranyp∈[1,+∞). | (4.8) |
Let Pσ be defined as the convolution Pσ:=P∗φσ. Then, by Young's inequality, we have
‖∇Pσ‖L∞(R3)=‖(∇P)∗φσ‖L∞(R3)≤‖∇P‖L2(R3)‖φσ‖L2(R3)(4.8)≲σ−3/2‖∇P‖L2(R3). |
The other inequality in (4.4) is obtained in a similar way. The condition (4.6) is a well-known property of convolutions.
Let us prove (4.5). Let ψ be the Fourier transform* of φ. Then, ψ is smooth and rapidly decaying (that is, it belongs to the Schwartz space S(R3)) and, in particular, it is Lipschitz continuous. Moreover, ψ(0)=∫R3φ(x)dx=1. By the properties of the Fourier transform, we have ^φσ(ξ)=ψ(σξ). By applying Plancherel theorem, we obtain
‖P−Pσ‖2L2(R3)=∫R3|ˆP(ξ)|2(1−ψ(σξ))2dξ=∫R3|ˆP(ξ)|2(ψ(0)−ψ(σξ))2dξ≤σ2‖∇ψ‖2L∞(R3)∫R3|ξ|2|ˆP(ξ)|2dξ=σ24π2‖∇ψ‖2L∞(R3)‖∇P‖2L2(R3). |
*We adopt the convention ˆφ(ξ)=∫R3φ(x)exp(−2πix⋅ξ)dx.
It only remains to prove (4.7). Let χ be the indicator function of U′ (i.e., χ=1 on U′ and χ=0 elsewhere). Observe that Pσ=(χP)∗φσ on U, because φσ is supported on the ball Bσ of radius σ and, by assumption, dist(U,R3∖U′)>σ. Then, Young inequality implies
‖P‖L2(U)≤‖χP‖L2(R3)‖φσ‖L1(R3)=‖P‖L2(U′). |
Lemma 4.6. Let \Omega\subseteq \mathbb{R}^3 a bounded, smooth domain, and let g\colon\Omega\to{\mathscr {S}}_0 be a bounded, Lipschitz map. For any Q\in H^1_g(\Omega, \, {\mathscr {S}}_0) and \sigma\in (0, \, 1) , there exists a bounded, Lipschitz map Q_\sigma\colon\overline{\Omega}\to{\mathscr {S}}_0 that satisfies the following properties:
\begin{gather} Q_\sigma = g \qquad {\rm{on }}\; \partial\Omega \end{gather} | (4.9) |
\begin{gather} \left\| {Q_\sigma} \right\|_{L^\infty(\Omega)} \lesssim \sigma^{-1/2}\left(\left\| {Q} \right\|_{H^1(\Omega)} + \left\| {g} \right\|_{L^\infty(\Omega)}\right) \end{gather} | (4.10) |
\begin{gather} \left\| {\nabla Q_\sigma} \right\|_{L^\infty(\Omega)} \lesssim \sigma^{-3/2} \left(\left\| {Q} \right\|_{H^1(\Omega)} + \left\| {g} \right\|_{W^{1,\infty}(\Omega)}\right) \end{gather} | (4.11) |
\begin{gather} \left\| {Q - Q_\sigma} \right\|_{L^2(\Omega)} \lesssim \sigma\left\| {Q} \right\|_{H^1(\Omega)} \end{gather} | (4.12) |
\begin{gather} \left\| {\nabla Q - \nabla Q_\sigma} \right\|_{L^2(\Omega)} \to 0 \qquad {\rm{as }}\; \sigma\to 0. \end{gather} | (4.13) |
Proof. Since \Omega is bounded and smooth, we can extend g to a bounded, Lipschitz map \mathbb{R}^3 \to{\mathscr {S}}_0 , still denoted g for simplicity, in such a way that \left\| {g} \right\|_{L^\infty(\mathbb{R}^3)} \lesssim \left\| {g} \right\|_{L^\infty(\Omega)} , \left\| {\nabla g} \right\|_{L^\infty(\mathbb{R}^3)} \lesssim \left\| {\nabla g} \right\|_{L^\infty(\Omega)} . Let P : = Q - g . Then, P\in H^1_0(\Omega, \, {\mathscr {S}}_0) , and we extend P to a new map P\in H^1(\mathbb{R}^3, \, {\mathscr {S}}_0) by setting P : = 0 on \mathbb{R}^3\setminus\Omega . By applying Lemma 4.5 to P , we construct a family of smooth maps (P_\sigma)_{\sigma > 0} that satisfies (4.4)–(4.7). We define
\tilde{P}_\sigma(x) : = \min\left(1, \, \sigma^{-1}{{\rm{dist}}}(x, \, \partial\Omega) \right) P_\sigma(x) \qquad {\rm{for }} \;x\in\Omega. |
The map \tilde{P}_\sigma\colon\overline{\Omega}\to{\mathscr {S}}_0 is bounded, Lipschitz, and \tilde{P}_\sigma = 0 on \partial\Omega . We claim that \tilde{P}_\sigma satisfies (4.10)–(4.13) with g\equiv 0 ; the lemma will follow by taking Q_\sigma : = \tilde{P}_\sigma + g . First, we note that (4.10) is a consequence of the extension of P to the whole \mathbb{R}^3 and (4.4) together with the Gagliardo-Nirenberg-Soboleve inequality. Then we check (4.11). Clearly |\tilde{P}_\sigma|\leq |P_\sigma| . Using the chain rule, and keeping in mind that the function {{\rm{dist}}}(\cdot, \, \partial\Omega) is 1 -Lipschitz, we see that
\begin{equation} |\nabla\tilde{P}_\sigma| \leq |\nabla P_\sigma| + \sigma^{-1}|P_\sigma| \qquad {\rm{a.e.\; on }}\; \Omega \end{equation} | (4.14) |
and (4.11) follows, with the help of (4.4).
We pass to the proof of (4.12). Let \Gamma_\sigma : = \{x\in \mathbb{R}^3\colon {{\rm{dist}}}(x, \, \partial\Omega) < \sigma\} . Since \partial\Omega is a compact, smooth manifold, for sufficiently small \sigma the set \Gamma_\sigma is diffeomorphic to the product \partial\Omega\times(-\sigma, \, \sigma) . We identify \Gamma_\sigma\simeq\partial\Omega\times(-\sigma, \, \sigma) and denote the variable in \Gamma_\sigma as x = (y, \, t)\in\partial\Omega\times(-\sigma, \, \sigma) . We apply Poincaré inequality to the map P on each slice \{y\}\times(-\sigma, \, \sigma) :
\int_{-\sigma}^\sigma \left| {P(y, \, t)} \right|^2 \mathrm{d} t = \int_0^\sigma \left| {P(y, \, t) - P(y, \, 0)} \right|^2 \mathrm{d} t \lesssim \sigma^2 \int_0^\sigma \left| {\partial_t P(y, \, t)} \right|^2 \mathrm{d} t. |
By integrating with respect to y\in\partial\Omega , we obtain
\begin{equation} \left\| {P} \right\|_{L^2(\Gamma_\sigma)} \lesssim \sigma\left\| {\nabla P} \right\|_{L^2(\Gamma_\sigma)} \end{equation} | (4.15) |
and hence,
\begin{split} \|\tilde{P}_\sigma - P_\sigma\|_{L^2(\Omega)} \lesssim \left\| {P_\sigma} \right\|_{L^2(\Gamma_\sigma)} \lesssim \left\| {P} \right\|_{L^2(\Gamma_\sigma)} + \left\| {P - P_\sigma} \right\|_{L^2(\Omega)} \stackrel{(4.5), (4.15)}{\lesssim} \sigma \left\| {\nabla P} \right\|_{L^2(\Omega)} \! . \end{split} |
Finally, let us prove (4.13). Combining (4.7) and (4.15), we deduce
\begin{equation} \left\| {P_\sigma} \right\|_{L^2(\Gamma_{\sigma})} \leq \left\| {P} \right\|_{L^2(\Gamma_{2\sigma})} \lesssim \sigma\left\| {\nabla P} \right\|_{L^2(\Gamma_{2\sigma})} \! . \end{equation} | (4.16) |
Therefore, we have
\begin{split} \|\nabla\tilde{P}_\sigma - \nabla P_\sigma\|_{L^2(\Omega)} &\leq \|\nabla\tilde{P}_\sigma\|_{L^2(\Gamma_\sigma)} + \left\| {\nabla P_\sigma} \right\|_{L^2(\Gamma_\sigma)} \\ &\stackrel{(4.14)}{\lesssim} \left\| {\nabla P_\sigma} \right\|_{L^2(\Gamma_\sigma)} + \sigma^{-1} \left\| {P_\sigma} \right\|_{L^2(\Gamma_{\sigma})} \\ &\stackrel{(4.16)}{\lesssim} \left\| {\nabla P} \right\|_{L^2(\Gamma_{2\sigma})} + \left\| {\nabla P_\sigma - \nabla P} \right\|_{L^2(\Omega)} \end{split} |
and both terms in the right-hand side converge to zero as \sigma\to 0 , due to (4.6).
Lemma 4.7. For any Q\in H^1_g(\Omega, \, {\mathscr {S}}_0) , there exists a sequence (Q_{\varepsilon})_{{\varepsilon} > 0} in H^1_g(\Omega, \, {\mathscr {S}}_0) that converges H^1(\Omega) -strongly to Q and satisfies
\left| {J_{\varepsilon}[Q_{\varepsilon}] - J_0[Q]} \right| \lesssim {\varepsilon}^{1/4} \left(\left\| {Q} \right\|^4_{H^1(\Omega)} + 1\right) |
(the functionals J_{\varepsilon} , J_0 are defined in (2.11), (2.12) respectively). The constant implied in front of the right-hand side depends on the L^\infty(\partial\Omega) -norms of g and \nabla g , as well as \Omega , f_s^j , {\mathscr P}^j , R_*^j with j\in\{1, \dots, J\} .
Proof. Let us fix a small {\varepsilon} > 0 . Let \beta be a positive parameter, to be chosen later, and let Q_{\varepsilon} : = Q_{{\varepsilon}^\beta}\in H^1_g(\Omega, \, {\mathscr {S}}_0) be the Lipschitz map given by Lemma 4.6. We have
\begin{equation} \begin{split} \left| {J_{\varepsilon}[Q_{\varepsilon}] - J_0[Q]} \right| \leq |J_{\varepsilon}[Q_{\varepsilon}] - \tilde{J}_{\varepsilon}[Q_{\varepsilon}]| + |\tilde{J}_{\varepsilon}[Q_{\varepsilon}] - J_0[Q_{\varepsilon}]| + \left| {J_0[Q_{\varepsilon}] - J_0[Q]} \right| \end{split} \end{equation} | (4.17) |
where \tilde{J}_{\varepsilon} is defined by (2.16). We will estimate separately all the terms in the right-hand side.
First, let us estimate the difference J_{\varepsilon}[Q_{\varepsilon}] - \tilde{J}_{\varepsilon}[Q_{\varepsilon}] . This can be achieved with the help of Lemma 2.8:
\begin{equation} \begin{split} |J_{\varepsilon}[Q_{\varepsilon}] - \tilde{J}_{\varepsilon}[Q_{\varepsilon}]| &\stackrel{(2.18)}{\lesssim} {\varepsilon}^\alpha \left(\left\| {Q_{\varepsilon}} \right\|_{L^\infty(\Omega)}^3 + 1\right) \left\| {\nabla Q_{\varepsilon}} \right\|_{L^\infty(\Omega)} \\ &\stackrel{(4.10), (4.11)}{\lesssim} {\varepsilon}^{\alpha - 3\beta} \left(\left\| {Q} \right\|_{H^1(\Omega)}^4 + 1\right) \end{split} \end{equation} | (4.18) |
(here and througout the rest of the proof, the constant implied in front of the right-hand side may depend on the L^\infty -norms of g and \nabla g ).
As for the second term, \tilde{J}_{\varepsilon}[Q_{\varepsilon}] - J_0[Q_{\varepsilon}] , we write \tilde{J}_{\varepsilon} in the form (2.17) and we re-write J_0 using (2.5), (2.12):
\begin{equation} \begin{split} |\tilde{J}_{\varepsilon}[Q_{\varepsilon}] - J_0[Q_{\varepsilon}]| &\leq \sum\limits_{j = 1}^J \left| {\int_{\Omega} f_{hom}^j(Q_{\varepsilon}, \, x) \, \mathrm{d}\mu_{\varepsilon}^j - \int_{\Omega} f_{hom}^j(Q_{\varepsilon}, \, x) \, \xi^j \, \mathrm{d} x} \right| \\ &\stackrel{(4.2)}{\leq} \mathbb{F}_{\varepsilon} \sum\limits_{j = 1}^J \left(\|\nabla (f_{hom}^j(Q_{\varepsilon}, \, \cdot))\|_{L^\infty(\Omega)} + \|f_{hom}^j(Q_{\varepsilon}, \, \cdot)\|_{L^\infty(\Omega)}\right) \! . \end{split} \end{equation} | (4.19) |
To estimate the terms at the right-hand side, we apply Lemma 2.7 and Lemma 4.6:
\begin{split} \|\nabla (f_{hom}^j(Q_{\varepsilon}, \, \cdot))\|_{L^\infty(\Omega)} &\stackrel{(2.13)}{\lesssim} \left(\left\| {Q_{\varepsilon}} \right\|_{L^\infty(\Omega)}^3 + 1 \right) \left\| {\nabla Q_{\varepsilon}} \right\|_{L^\infty(\Omega)} \\ &\stackrel{(4.11)}{\lesssim} {\varepsilon}^{- 3\beta} \left(\left\| {Q} \right\|_{H^1(\Omega)}^4 + 1\right) \! , \end{split} |
and
\begin{split} \|f_{hom}^j(Q_{\varepsilon}, \, \cdot)\|_{L^\infty(\Omega)} &\stackrel{(2.13)}{\lesssim} \left\| {Q_{\varepsilon}} \right\|_{L^\infty(\Omega)}^4 + 1 \stackrel{(4.11)}{\lesssim} {\varepsilon}^{- 2\beta} \left(\left\| {Q} \right\|_{H^1(\Omega)}^4 + 1\right) \! . \end{split} |
Injecting these inequalities into (4.19), and using that \mathbb{F}_{\varepsilon}\lesssim{\varepsilon} by Assumption (4.2), we obtain
\begin{equation} \begin{split} |\tilde{J}_{\varepsilon}[Q_{\varepsilon}] - J_0[Q_{\varepsilon}]| &\lesssim {\varepsilon}^{1- 3\beta} \left(\left\| {Q} \right\|_{H^1(\Omega)}^4 + 1\right) \!. \end{split} \end{equation} | (4.20) |
Finally, the term J_0[Q_{\varepsilon}] - J_0[Q] . We apply Lemma 2.7 and the Hölder inequality:
\begin{equation*} \begin{split} \left| {J_0[Q_{\varepsilon}] - J_0[Q]} \right| &\leq \int_{\Omega} \left| {f_{hom}(Q_{\varepsilon}, \, \cdot) - f_{hom}(Q, \, \cdot)} \right| \\ &\stackrel{(2.14)}{\leq} \int_{\Omega} \left(\left| {Q} \right|^3 + \left| {Q_{\varepsilon}} \right|^3 + 1\right) \left| {Q - Q_{\varepsilon}} \right| \\ &\lesssim \left(\left\| {Q} \right\|_{L^6(\Omega)}^3 + \left\| {Q_{\varepsilon}} \right\|_{L^6(\Omega)}^3 + 1 \right) \left\| {Q - Q_{\varepsilon}} \right\|_{L^2(\Omega)} \end{split} \end{equation*} |
The sequence Q_{\varepsilon} is bounded in L^6(\Omega) , thanks to Sobolev embedding and to Lemma 4.6. Therefore,
\begin{equation} \begin{split} \left| {J_0[Q_{\varepsilon}] - J_0[Q]} \right| &\stackrel{(4.12)}{\lesssim} {\varepsilon}^\beta \left\| {Q} \right\|_{H^1(\Omega)}^4 + {\varepsilon}^\beta \left\| {Q} \right\|_{H^1(\Omega)} \! . \end{split} \end{equation} | (4.21) |
Combining (4.17), (4.18), (4.20) and (4.21), we deduce
\left| {J_{\varepsilon}[Q_{\varepsilon}] - J_0[Q]} \right| \lesssim {\varepsilon}^{\min(\alpha - 3\beta, \, 1 - 3\beta, \, \beta)} \left(\left\| {Q} \right\|^4_{H^1(\Omega)} + 1\right) \! . |
Keeping into account that \alpha > 1 , we see that the optimal choice of \beta is \beta = 1/4 , and the lemma follows.
Lemma 4.8. Let Q_{\varepsilon}\in H^1(\Omega_{\varepsilon}, \, {\mathscr {S}}_0) be a family of maps, such that E_{\varepsilon} Q_{\varepsilon}\to Q strongly in H^1(\Omega) , as {\varepsilon}\to 0 . Then,
\begin{equation*} \limsup\limits_{{\varepsilon}\to 0} \int_{\Omega_{\varepsilon}} \left(f_e(\nabla Q_{\varepsilon}) + f_b(Q_{\varepsilon})\right) \mathrm{d} x \leq \int_{\Omega} \left(f_e(\nabla Q) + f_b(Q)\right) \mathrm{d} x. \end{equation*} |
Proof. By Sobolev embedding, we have E_{\varepsilon} Q_{\varepsilon}\to Q strongly in L^6(\Omega) . Then, up to extraction of a non-relabelled subsequence, we find functions h_e\in L^2(\Omega) , h_b\in L^6(\Omega) such that
\begin{equation} \left| {\nabla(E_{\varepsilon} Q_{\varepsilon})} \right| \leq h_e, \quad \left| {E_{\varepsilon} Q_{\varepsilon}} \right| \leq h_b \qquad {\rm{a.e. \;on }}\; \Omega, {\rm{ for \;any }} {\varepsilon}. \end{equation} | (4.22) |
Let \chi_{\varepsilon} be the the indicator function of \Omega_{\varepsilon} (i.e., \chi_{\varepsilon} : = 1 on \Omega_{\varepsilon} and \chi_{\varepsilon} : = 0 elsewhere). Thanks to (H6), (H7) and to (4.22), we have
\left(f_e(\nabla Q_{\varepsilon}) + f_b(Q_{\varepsilon})\right) \chi_{\varepsilon} \lesssim h_e^2 + h_b^6 + 1 \in L^1(\Omega) \qquad {\rm{a.e. \;on } }\;\Omega, {\rm{ for\; any }}\; {\varepsilon}. |
Moreover, since |{\mathscr P}_{\varepsilon}|\lesssim {\varepsilon}^{3\alpha - 3}\to 0 , \chi_{{\varepsilon}} converges to 1 strongly in L^1(\Omega) and we may extract a further subsequence so to have \chi_{\varepsilon}\to 1 a.e. Then, the lemma follows from Lebesgue's dominated converge theorem.
Proof of Theorem 4.3. Let Q_{\varepsilon}\in H^1_g(\Omega_{\varepsilon}, \, {\mathscr {S}}_0) , for {\varepsilon} > 0 , be a family of maps such that \sup_{\varepsilon}{\mathscr F}_{{\varepsilon}, \gamma}(Q) < +\infty . We first extract a (non-relabelled) subsequence {\varepsilon}\to 0 , so that \limsup_{{\varepsilon}\to 0}{\mathscr F}_{{\varepsilon}, \gamma}(Q_{\varepsilon}) is achieved as a limit; this allows us to pass freely to subsequences, in what follows. Thanks to (H6), (H7), (K2), we have \sup_{\varepsilon}\|Q_{\varepsilon}\|_{L^2(\Omega_{\varepsilon})} < +\infty . By Lemma 2.5, there is a (non-relabelled) subsequence and Q_0\in H^1_g(\Omega, \, {\mathscr {S}}_0) such that E_{\varepsilon} Q_{\varepsilon}\rightharpoonup Q_0 weakly in H^1(\Omega) . By Proposition 2.2, there holds
\widetilde{{\mathscr F}}(Q) \leq \liminf\limits_{{\varepsilon}\to 0} \int_{\Omega_{\varepsilon}}\left(f_e(\nabla Q_{\varepsilon}) + f_b(Q_{\varepsilon})\right) \leq \liminf\limits_{{\varepsilon}\to 0} {\mathscr F}_{{\varepsilon},\gamma}(Q_{\varepsilon}) |
and
J_0[Q_0] = \lim\limits_{{\varepsilon}\to 0} J_{\varepsilon}[Q_{\varepsilon}] \leq \limsup\limits_{{\varepsilon}\to 0} {\varepsilon}^\gamma {\mathscr F}_{{\varepsilon},\gamma}(Q_{\varepsilon}) = 0, |
so Q_0 belongs to the class {\mathscr A} defined by (4.3). Thus, Statement (i) is proved.
We now prove Statement (ⅱ). Let Q_0\in H^1_g(\Omega, \, \Omega) be fixed. We can suppose without loss of generality that Q\in{\mathscr A} , otherwise the statement is trivial. Due to Lemma 4.7, there is a sequence \tilde{Q}_{\varepsilon}\in H^1_g(\Omega, \, {\mathscr {S}}_0) such that \tilde{Q}_{\varepsilon}\to Q_0 strongly in H^1(\Omega) and
\begin{equation} \left| {J_{\varepsilon}[\tilde{Q}_{\varepsilon}]} \right| = {\varepsilon}^{3-2\alpha} \left| {\sum\limits_{j = 1}^J \int_{\partial{\mathscr P}^j} f_s^j(\tilde{Q}_{\varepsilon}, \, \nu) \, \mathrm{d}\sigma} \right| \lesssim {\varepsilon}^{1/4} \left(\left\| {Q} \right\|_{L^4(\Omega)}^4 + 1\right) \! . \end{equation} | (4.23) |
Let Q_{\varepsilon} : = \tilde{Q}_{{\varepsilon}|\Omega_{\varepsilon}} . By Lemma 2.5, E_{\varepsilon} Q_{\varepsilon}\to Q_0 strongly in H^1(\Omega) . Using Lemma 4.8 and (4.23), and recalling that \gamma < 1/4 , we conclude that
\limsup\limits_{{\varepsilon}\to 0} {\mathscr F}_{{\varepsilon},\gamma}(Q_{\varepsilon}) = \limsup\limits_{{\varepsilon}\to 0} \int_{\Omega_{\varepsilon}}\left(f_e(\nabla Q_{\varepsilon}) + f_b(Q_{\varepsilon})\right) +\underbrace{\limsup\limits_{{\varepsilon}\to 0} {\varepsilon}^{-\gamma} J_{\varepsilon}[Q_{\varepsilon}]}_{ = 0, \rm{ by (4.23)}} \leq \widetilde{{\mathscr F}}(Q_0), |
so the proof is complete.
The work of A. Z. is supported by the Basque Government through the BERC 2018-2021 program, by Spanish Ministry of Economy and Competitiveness MINECO through BCAM Severo Ochoa excellence accreditation SEV-2017-0718 and through project MTM2017-82184-R funded by (AEI/FEDER, UE) and acronym "DESFLU".
The authors declare that there is no conflict of interest regarding the publication of this article.
[1] |
D. E. Hornung, M. P. Enns, The contributions of smell and taste to overall intensity: A model, Percept. Psychophys., 39 (1986), 385–391. https://doi.org/10.3758/BF03207066 doi: 10.3758/BF03207066
![]() |
[2] | B. Lorrain, J. Ballester, T. Thomas-Danguin, J. Blanquet, J. M. Meunier, Y. Le Fur, Selection of potential impact odorants and sensory validation of their importance in typical chardonnay wines, J. Agri. Food Chem., 54 (2006), 3973–3981. |
[3] |
C. Flavián, S. Ibáñez-Sánchez, C. Orús, The influence of scent on virtual reality experiences: The role of aroma-content congruence, J. Business Res., 123 (2021), 289–301. https://doi.org/10.1016/j.jbusres.2020.09.036 doi: 10.1016/j.jbusres.2020.09.036
![]() |
[4] |
R. Hudson, Editorial note, Chem. Senses, 25 (2000), 693–693. https://doi.org/10.1046/j.1442-9993.2000.00002.x doi: 10.1046/j.1442-9993.2000.00002.x
![]() |
[5] | H. Stone, R. N. Bleibaum, H. A. Thomas, Sensory evaluation practices, Academic press, 2012. |
[6] | G. Ferrari, O. Lablanquie, R. Cantagrel, J. Ledauphin, T. Payot, N. Fournier, et al., Determination of key odorant compounds in freshly distilled cognac using GC-o, GC-MS, and sensory evaluation, J. Agri. Food Chem., 52 (2004), 5670–5676. |
[7] | S.-J. Lee, A. C. Noble, Characterization of odor-active compounds in californian chardonnay wines using GC-olfactometry and GC-mass spectrometry, J. Agri. Food Chem., 51 (2003), 8036–8044. |
[8] |
A. Pérez-Silva, E. Odoux, P. Brat, F. Ribeyre, G. Rodriguez-Jimenes, V. Robles-Olvera, et al., GC–MS and GC–olfactometry analysis of aroma compounds in a representative organic aroma extract from cured vanilla (vanilla planifolia g. jackson) beans, Food Chem, 99 (2006), 728–735. https://doi.org/10.1016/j.foodchem.2005.08.050 doi: 10.1016/j.foodchem.2005.08.050
![]() |
[9] |
M. Brattoli, E. Cisternino, P. Dambruoso, G. de Gennaro, P. Giungato, A. Mazzone, et al., Gas chromatography analysis with olfactometric detection (GC-o) as a useful methodology for chemical characterization of odorous compounds, Sensors, 13 (2013), 16759–16800. https://doi.org/10.3390/s131216759 doi: 10.3390/s131216759
![]() |
[10] | T. Thomas-Danguin, C. Sinding, S. Romagny, F. E. Mountassir, B. Atanasova, E. Le Berre, et al., The perception of odor objects in everyday life: A review on the processing of odor mixtures, Front. Psychol., 5 (2014). |
[11] |
W. Grosch, Evaluation of the key odorants of foods by dilution experiments, aroma models and omission, Chem. Senses, 26 (2001), 533–545. https://doi.org/10.1093/chemse/26.5.533 doi: 10.1093/chemse/26.5.533
![]() |
[12] | H. Guth, Quantitation and sensory studies of character impact odorants of different white wine varieties, J. Agri. Food Chem., 45 (1997), 3027–3032. |
[13] | V. Ferreira, N. Ortín, A. Escudero, R. López, J. Cacho, Chemical characterization of the aroma of grenache rosé wines: aroma extract dilution analysis, quantitative determination, and sensory reconstitution studies, J. Agri. Food Chem., 50 (2002), 4048–4054. |
[14] | A. Escudero, B. Gogorza, M. A. Melús, N. Ortín, J. Cacho, V. Ferreira, Characterization of the aroma of a wine from maccabeo. key role played by compounds with low odor activity values, J. Agri. Food Chem., 52 (2004), 3516–3524. |
[15] |
V. Ferreira, M. Sáenz-Navajas, E. Campo, P. Herrero, A. de la Fuente, P. Fernández-Zurbano, Sensory interactions between six common aroma vectors explain four main red wine aroma nuances, Food Chem., 199 (2016), 447–456. https://doi.org/10.1016/j.foodchem.2015.12.048 doi: 10.1016/j.foodchem.2015.12.048
![]() |
[16] | Y. Zheng, B. G. Sun, M. M. Zhao, F. P. Zheng, M. Q. Huang, J. Y. Sun, et al., Characterization of the key odorants in chinese zhima aroma-type baijiu by gas chromatography–olfactometry, quantitative measurements, aroma recombination, and omission studies, J. Agri. Food Chem., 64 (2016), 5367–5374. |
[17] |
W. S. Cain, Odor intensity: Differences in the exponent of the psychophysical function, Percept. Psychophys., 6 (1969), 349–354. https://doi.org/10.3758/BF03212789 doi: 10.3758/BF03212789
![]() |
[18] |
P. A. Edwards, P. C. Jurs, Correlation of odor intensities with structural properties of odorants, Chem. Senses, 14 (1989), 281–291. https://doi.org/10.1093/chemse/14.2.281 doi: 10.1093/chemse/14.2.281
![]() |
[19] |
M. H. Abraham, R. Sanchez-Moreno, J. E. Cometto-Muniz, W. S. Cain, An algorithm for 353 odor detection thresholds in humans, Chemical Senses, 37 (2012), 207–218. https://doi.org/10.1093/chemse/bjr094 doi: 10.1093/chemse/bjr094
![]() |
[20] |
A. Keller, R. C. Gerkin, Y. F. Guan, A. Dhurandhar, G. Turu, B. Szalai, et al., Predicting human olfactory perception from chemical features of odor molecules, Science, 355 (2017), 820–826. https://doi.org/10.1126/science.aal2014 doi: 10.1126/science.aal2014
![]() |
[21] |
R. M. Khan, C.-H. Luk, A. Flinker, A. Aggarwal, H. Lapid, R. Haddad, et al., Predicting odor pleasantness from odorant structure: Pleasantness as a reflection of the physical world, J. Neurosci., 27 (2007), 10015–10023. https://doi.org/10.1523/JNEUROSCI.1158-07.2007 doi: 10.1523/JNEUROSCI.1158-07.2007
![]() |
[22] |
A. Arshamian, R. C. Gerkin, N. Kruspe, E. Wnuk, S. Floyd, C. O'Meara, et al., The perception of odor pleasantness is shared across cultures, Current Biol., 32 (2022), 2061–2066. https://doi.org/10.1016/j.cub.2022.02.062 doi: 10.1016/j.cub.2022.02.062
![]() |
[23] |
L. Shang, C. J. Liu, Y. Tomiura, K. S. Hayashi, Machine-learning-based olfactometer: Prediction of odor perception from physicochemical features of odorant molecules, Anal. Chem., 89 (2017), 11999–12005. https://doi.org/10.1021/acs.analchem.7b02389 doi: 10.1021/acs.analchem.7b02389
![]() |
[24] |
K. Snitz, A. Yablonka, T. Weiss, I. Frumin, R. M. Khan, N. Sobel, Predicting odor perceptual similarity from odor structure, PLoS Comput. Biol., 9 (2013), e1003184. https://doi.org/10.1371/journal.pcbi.1003184 doi: 10.1371/journal.pcbi.1003184
![]() |
[25] |
A. C. Noble, S. E. Ebeler, Use of multivariate statistics in understanding wine flavor, Food Rev. Int., 18 (2002), 1–21. https://doi.org/10.1081/FRI-120003414 doi: 10.1081/FRI-120003414
![]() |
[26] | M. Aznar, R. López, J. Cacho, V. Ferreira, Prediction of aged red wine aroma properties from aroma chemical composition. partial least squares regression models, J. Agri. Food Chem., 51 (2003), 2700–2707. |
[27] |
S. G. Penella, R. Boulanger, I. Maraval, G. Kopp, M. Corno, B.Fontez, et al., Link between flavor perception and volatile compound composition of dark chocolates derived from trinitario cocoa beans from dominican republic, Molecules, 28 (2023), 3805. https://doi.org/10.3390/molecules28093805 doi: 10.3390/molecules28093805
![]() |
[28] | B Veinand, Sensory testing with flavourists: challenges and solutions, In Rapid Sensory Profiling Techniques, pages 383–399. Elsevier, 2015. |
[29] | J.-N. Jaubert, C. Tapiero, J.-C. Dore, The field of odors: toward a universal language for odor relationships, Perfumer & Flavor., 20 (1995), 1–16. |
[30] | S. Mirri, C. Prandi, M. Roccetti, P. Salomoni, Handmade Narrations: Handling digital narrations on food and gastronomic culture, J. Comput. Cultural Herit., 10 (2017), 1–17. |
[31] | M. Sicard, C. Baudrit, M. N. Leclerc-Perlat, P. H. Wuillemin, N. Perrot, Expert knowledge integration to model complex food processes. application on the camembert cheese ripening process, Expert Syst. Appl., 38 (2011), 11804–11812. |
[32] | N. Perrot, I. C. Trelea, C. Baudrit, G. Trystram, P. Bourgine, Modelling and analysis of complex food systems: State of the art and new trends, Trends Food Sci. Technol., 22 (2011), 304–314. |
[33] | L. A. Zadeh, Fuzzy sets, Inform. Control, 8 (1965), 338–353. https://doi.org/10.1016/S0019-9958(65)90241-X |
[34] |
N. Perrot, I. Ioannou, I. Allais, C. Curt, J. Hossenlopp, G. Trystram, Fuzzy concepts applied to food product quality control: A review, Fuzzy Sets Syst., 157 (2006), 1145–1154. https://doi.org/10.1016/j.fss.2005.12.013 doi: 10.1016/j.fss.2005.12.013
![]() |
[35] |
J. Tan, X. Gao, D. E. Gerrard, application of fuzzy sets and neural networks in sensory analysis, J. Sensory Studies, 14 (1999), 119–138. https://doi.org/10.1111/j.1745-459X.1999.tb00108.x doi: 10.1111/j.1745-459X.1999.tb00108.x
![]() |
[36] | V. J. Davidson, J. Ryks, T. Chu, Fuzzy models to predict consumer ratings for biscuits based on digital image features, IEEE Transact. Fuzzy Syst., 9 (2001), 62–67. |
[37] |
I. Ioannou, N. Perrot, J. Hossenlopp, G. Mauris, G. Trystram, The fuzzy set theory: a helpful tool for the estimation of sensory properties of crusting sausage appearance by a single expert, Food Quality Prefer., 13 (2002), 589–595. https://doi.org/10.1016/S0950-3293(02)00045-9 doi: 10.1016/S0950-3293(02)00045-9
![]() |
[38] | S. Mukhopadhyay, G. C. Majumdar, T. K. Goswami, H. N. Mishra, Fuzzy logic (similarity analysis) approach for sensory evaluation of chhana podo, LWT - Food Sci. Technol., 53 (2013), 204–210. |
[39] | C. Debjani, S. Das, H. Das, Aggregation of sensory data using fuzzy logic for sensory quality evaluation of food, J. Food Sci. Technol., 50 (2011), 1088–1096. |
[40] | K. J. Shinde, I. L. Pardeshi, Fuzzy logic model for sensory evaluation of commercially available jam samples, J. Ready Eat Food, 1 (2014), 78–84. |
[41] |
O. Folorunso, Y. Ajayi, T. Shittu, Fuzzy-rule-based approach for modeling sensory acceptabitity of food products, Data Sci. J., 8 (2009), 70–77. https://doi.org/10.2481/dsj.007-006 doi: 10.2481/dsj.007-006
![]() |
[42] |
A. Villière, R. Symoneaux, A. Roche, A. Eslami, N. Perrot, Y. Le Fur, et al., Comprehensive sensory and chemical data on the flavor of 16 red wines from two varieties: sensory descriptive analysis, hs-spme-gc-ms volatile compounds quantitative analysis, and odor-active compounds identification by hs-spme-gc-ms-o, Data Brief, 24 (2019), 103725. https://doi.org/10.1016/j.dib.2019.103725 doi: 10.1016/j.dib.2019.103725
![]() |
[43] |
A. Loison, R. Symoneaux, P. Deneulin, T. Thomas-Danguin, C. Fant, L. Guérin, et al., Exemplarity measurement and estimation of the level of interjudge agreement for two categories of french red wines, Food Quality Prefer., 40 (2015), 240–251. https://doi.org/10.1016/j.foodqual.2014.10.001 doi: 10.1016/j.foodqual.2014.10.001
![]() |
[44] | P. Pollien, A. Ott, F. Montigon, M. Baumgartner, R. Muñoz-Box, A. Chaintreau, Hyphenated headspace-gas chromatography-sniffing technique: Screening of impact odorants and quantitative aromagram comparisons, J. Agri. Food Chem., 45 (1997), 2630–2637. |
[45] | S. Arctander, Perfume and flavor chemicals, Montlcair, N.J. USA, 1969. |
[46] | W. Luebke, the good scents company, https://WWW.thegoodscentscompany.com/index.html, 1980. |
[47] |
L. A. Zadeh, Fuzzy logic and approximate reasoning, Synthese, 30 (1975), 407–428. https://doi.org/10.1007/BF00485052 doi: 10.1007/BF00485052
![]() |
[48] | D. Dubois, H. Prade, Fuzzy sets and systems: Theory and applications, Academic press, NY, USA, 1969. |
[49] | S. Arlot, A. Celisse, A survey of cross-validation procedures for model selection, Statistics Surveys, 4 (2010), 40–79. |
[50] |
W. Guang, M. Baraldo, M. Furlanut, Calculating percentage prediction error: A user's note, Pharmacolog. Res., 32 (1995), 241–248. https://doi.org/10.1016/S1043-6618(05)80029-5 doi: 10.1016/S1043-6618(05)80029-5
![]() |
[51] |
A. Roche, N. M. Perrot, T. Thomas-Danguin, Oops, the ontology for odor perceptual space: From molecular composition to sensory attributes of odor objects, Molecules, 2022. https://doi.org/10.3390/molecules272227888 doi: 10.3390/molecules272227888
![]() |
[52] | N. Hansen, Towards a New Evolutionary Computation Studies in Fuzziness and Soft Computing, In chapter The CMA evolution strategy: A comparing review, (eds) J.A. Lozano, P. Larrañaga, I. Inza, I. Bengoetxea, 192 (2006), Springer, 75–102. https://doi.org/10.1080/02693445.1948.12035642 |