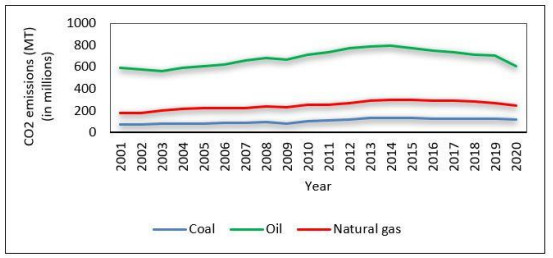
Energy consumption increases daily across the world. Electricity is the best means that humankind has found for transmitting energy. This can be said regardless of its origin. Energy transmission is crucial for ensuring the efficient and reliable distribution of electricity from power generation sources to end-users. It forms the backbone of modern societies, supporting various sectors such as residential, commercial, and industrial activities. Energy transmission is a fundamental enabler of well-functioning and competitive electricity markets, supporting reliable supply, market integration, price stability, and the integration of renewable energy sources. Electric energy sourced from various regions worldwide is routinely traded within these electricity markets on a daily basis. This paper presents a review of forecasting techniques for intraday electricity markets prices, volumes, and price volatility. Electricity markets operate in a sequential manner, encompassing distinct components such as the day-ahead, intraday, and balancing markets. The intraday market is closely linked to the timely delivery of electricity, as it facilitates the trading and adjustment of electricity supply and demand on the same day of delivery to ensure a balanced and reliable power grid. Accurate forecasts are essential for traders to maximize profits within intraday markets, making forecasting a critical concern in electricity market management. In this review, statistical and econometric approaches, involving various machine learning and ensemble/hybrid techniques, are presented. Overall, the literature highlights the superiority of machine learning and ensemble/hybrid models over statistical models.
Citation: Sameer Thakare, Neeraj Dhanraj Bokde, Andrés E. Feijóo-Lorenzo. Forecasting different dimensions of liquidity in the intraday electricity markets: A review[J]. AIMS Energy, 2023, 11(5): 918-959. doi: 10.3934/energy.2023044
[1] | Cohen Ang'u, Nzioka John Muthama, Mwanthi Alexander Mutuku, Mutembei Henry M'IKiugu . Carbon monoxide and nitrogen dioxide patterns associated with changes in energy use during the COVID-19 pandemic in Kenya. AIMS Environmental Science, 2022, 9(3): 244-259. doi: 10.3934/environsci.2022017 |
[2] | Ernyasih, Anwar Mallongi, Anwar Daud, Sukri Palutturi, Stang, Abdul RazakThaha, Erniwati Ibrahim, Wesam Al Madhoun, Andriyani . Strategy for mitigating health and environmental risks from vehicle emissions in South Tangerang. AIMS Environmental Science, 2023, 10(6): 794-808. doi: 10.3934/environsci.2023043 |
[3] | Sayali Sandbhor, Sayali Apte, Vaishnavi Dabir, Ketan Kotecha, Rajkumar Balasubramaniyan, Tanupriya Choudhury . AI-based carbon emission forecast and mitigation framework using recycled concrete aggregates: A sustainable approach for the construction industry. AIMS Environmental Science, 2023, 10(6): 894-910. doi: 10.3934/environsci.2023048 |
[4] | Novi Sylvia, Aden Syahrullah Tarigan, Rozanna Dewi, Yunardi Yunardi, Yazid Bindar, Mutia Reza . A simulation study of CO2 gas adsorption with bottom ash adsorbent from palm oil mill waste using computational fluid dynamic (CFD). AIMS Environmental Science, 2024, 11(3): 444-456. doi: 10.3934/environsci.2024022 |
[5] | Jordan Brown, Corona Chen, Deborah Carr . Endolithic microbes may alter the carbon profile of concrete. AIMS Environmental Science, 2024, 11(2): 221-247. doi: 10.3934/environsci.2024011 |
[6] | Anwar Khan, Benjamin Razis, Simon Gillespie, Carl Percival, Dudley Shallcross . Global analysis of carbon disulfide (CS2) using the 3-D chemistry transport model STOCHEM. AIMS Environmental Science, 2017, 4(3): 484-501. doi: 10.3934/environsci.2017.3.484 |
[7] | A. Fraser-McDonald, C. Boardman, T. Gladding, S. Burnley, V. Gauci . Nitrous oxide emissions from trees planted on a closed landfill site. AIMS Environmental Science, 2023, 10(2): 313-324. doi: 10.3934/environsci.2023018 |
[8] | Hedi Indra Januar, Neviaty Putri Zamani, Dedi Soedarma, Ekowati Chasanah . Changes in soft coral Sarcophyton sp. abundance and cytotoxicity at volcanic CO2 seeps in Indonesia. AIMS Environmental Science, 2016, 3(2): 239-248. doi: 10.3934/environsci.2016.2.239 |
[9] | Dominic Bowd, Campbell McKay, Wendy S. Shaw . Urban greening: environmentalism or marketable aesthetics. AIMS Environmental Science, 2015, 2(4): 935-949. doi: 10.3934/environsci.2015.4.935 |
[10] | Guillermo Samperio-Ramos, J. Magdalena Santana-Casiano, Melchor González-Dávila, Sonia Ferreira, Manuel A. Coimbra . Variability in the organic ligands released by Emiliania huxleyi under simulated ocean acidification conditions. AIMS Environmental Science, 2017, 4(6): 788-808. doi: 10.3934/environsci.2017.6.788 |
Energy consumption increases daily across the world. Electricity is the best means that humankind has found for transmitting energy. This can be said regardless of its origin. Energy transmission is crucial for ensuring the efficient and reliable distribution of electricity from power generation sources to end-users. It forms the backbone of modern societies, supporting various sectors such as residential, commercial, and industrial activities. Energy transmission is a fundamental enabler of well-functioning and competitive electricity markets, supporting reliable supply, market integration, price stability, and the integration of renewable energy sources. Electric energy sourced from various regions worldwide is routinely traded within these electricity markets on a daily basis. This paper presents a review of forecasting techniques for intraday electricity markets prices, volumes, and price volatility. Electricity markets operate in a sequential manner, encompassing distinct components such as the day-ahead, intraday, and balancing markets. The intraday market is closely linked to the timely delivery of electricity, as it facilitates the trading and adjustment of electricity supply and demand on the same day of delivery to ensure a balanced and reliable power grid. Accurate forecasts are essential for traders to maximize profits within intraday markets, making forecasting a critical concern in electricity market management. In this review, statistical and econometric approaches, involving various machine learning and ensemble/hybrid techniques, are presented. Overall, the literature highlights the superiority of machine learning and ensemble/hybrid models over statistical models.
Over the years, Africa has experienced an upward trend in CO2 emissions driven mainly by increased energy demands and heavy reliance on nonrenewable energy. Given the vulnerability of the African continent to climate change and external shocks such as extreme weather conditions and food insecurity, it is essential to tackle the issue in earnest. Without adequate regulatory measures, attaining global climate objectives becomes exceedingly difficult [1]. According to the Intergovernmental Panel on Climate Change, anthropogenic climate change results from almost a century of net greenhouse gas emissions due to energy use, land use, production, and consumption patterns [2]. Suboptimal energy consumption can lead to an increase in carbon emission levels. Therefore, economic structures, energy frameworks, and environmental measures should be based on the efficient use of resources [3].
Nonrenewable energy is mainly associated with increased CO2 emissions. Research suggests that as countries prioritize economic growth, there is an increase in the deterioration of the ecological environment [4]. As several African countries advance their economies through industrialization and construction, there is heightened demand for energy, especially fossil fuels, resulting in high CO2 emissions. Excessive energy usage directly and indirectly affects the natural environment [5]. As shown in Figure 1, oil remains the dominant fossil fuel in Africa because of its use in transportation, electricity generation, and different industrial processes. Countries such as Nigeria and Angola are the major oil-producing countries and, thus, contribute significantly to the overall emissions of the continent. These resources enhance economic growth, especially for oil-producing countries [6]. Natural gas is Africa's second-largest source of CO2 emissions. Natural gas utilization has increased significantly in recent years due to its perceived cleaner-burning features compared to coal and oil. Algeria and Egypt have invested considerably in natural gas extraction and infrastructure development to raise their share of energy output [7]. Coal is Africa's third-largest source of CO2 emissions and is predominantly used in South Africa [8]. Due to its high carbon intensity compared to natural gas, coal is generally viewed as a last-resort fuel supply alternative. To attain the 1.5 ℃ goal, South Africa must cut its share of coal power by around 30% every decade [9,10]. According to the International Energy Agency, global subsidies for fossil fuel consumption surged to over one trillion US dollars, marking the highest annual value recorded to date [11]. The Glasgow Climate Pact 2021 urged countries to eliminate ineffective fossil fuel subsidies [12]. Africa has developed a reliance on petroleum products without adequately addressing the associated consequences of greenhouse gas emissions and other climate change-related issues [13]. Vehicle fuel efficiency, economic activities, and population growth rates are often regarded as significant drivers of transportation energy demand [14]. The energy consumption required for road passenger transport can vary significantly depending on various factors, including vehicle type, load factor, average speed, driving style, technology, and vehicle age [15]. Under the 2050 Scenario, transportation-related CO2 emissions must decrease by more than 3% annually by 2030 to reach net zero emissions [16]. Africa faces a challenge in balancing economic growth and sustainable environmental standards. Numerous countries are experiencing energy shortages while attempting to fulfill international climate commitments. Given that energy facilitates economic growth, Africa should transition to clean energy sources to meet the increasing energy demands across different sectors [17]. Policymakers should focus on advancing renewable energy technologies, including wind and solar power, to reduce reliance on fossil fuels [18].
Access to clean energy remains a challenge in Africa, as 46% of the total population still has no access to basic electricity [19]. Most households still rely on conventional fuel for cooking and heating, although the continent has significant renewable resources such as solar, wind, hydro, and geothermal [20]. For Africa to transition to clean energy sources, investment in modern energy should be increased and sustained. To ensure a smooth transition to clean energy, stakeholders should outline effective alternative means of energy generation [21]. Li and Haneklaus [22] stated that using clean energy and stricter environmental regulations are two realistic ways to reduce CO2 emissions. Studies have shown the possibility of digitalizing Africa's sustainable energy transition by adopting digital technologies [23]. Artificial intelligence (AI) is gaining momentum within the energy sector. Digital technologies can make energy use more efficient and less polluting [24]. AI boosts green economic efficiency by streamlining supply chains, reducing waste, and using less energy [25]. Developing countries face financial, technological, and infrastructure barriers that obstruct decarbonization initiatives [26]. A critical policy strategy is encouraging households and businesses to adopt clean energy rather than conventional polluting ones [27].
Most research on energy use and its environmental effects focuses on the separate effects of CO2 emissions, nonrenewable energy, and transport energy usage. Previous studies, most of which were carried out in industrialized countries, have only examined these indicators individually. Therefore, there is currently a study gap regarding the mediating effect of transport energy usage on the relationship between nonrenewable consumption and CO2 emissions in Africa. Although it is recognized that Africa contributes a negligible amount to global CO2 emissions, the continent is at risk of unique vulnerabilities due to its socioeconomic conditions and dependence on nonrenewable energy sources. According to the International Energy Agency [28], many economic activities in Africa are still dependent on fossil fuels. Moreover, most of the literature has ignored the possible mediating role that transport energy plays in defining the relationship between nonrenewable energy consumption and CO2 emissions. Most transport systems in Africa remain underdeveloped and greatly rely on fossil fuel sources, potentially increasing the negative environmental effect caused by the increased use of nonrenewable energy.
This study examines an important gap in the current literature by investigating the mediating effect of transport energy on the relationship between nonrenewable energy consumption and CO2 emissions in Africa. The random effect model will be used to determine the mediating effect of transport energy. The fixed effect model is utilized to test for the moderating impact of clean energy. Additionally, different econometric approaches will be employed to examine the reliability of the research estimates. The Driscoll and Kraay standard error [29] estimator is used to check the robustness of the estimates. This study offers empirical evidence that may guide future policy decisions on climate change and energy demand. The remainder of the paper is outlined as follows: Section 2 presents the literature review. Section 3 outlines the material and methods. Section 4 shows the results. Section 5 outlines the discussion. Finally, section 6 presents the conclusion with policy recommendations.
The consumption of nonrenewable energy, such as fossil fuels, significantly affects the environment. Nonrenewable energy refers to energy sources that reduce in quantity as consumption increases. The prioritization of economic growth might lead to an increase in the consumption of nonrenewable energy, which could deteriorate the environment [30]. Energy consumption levels differ in intensity and volume in various countries, regions, and continents. Developed countries typically exhibit higher energy consumption levels than developing countries. This is due to variations in economic structures, technological advancements, and industrialization. Previous studies have examined the effect of nonrenewable energy on CO2 emissions, and the outcomes vary across different economic structures. Chen et al. [31] investigated the impact of energy on CO2 emissions per capita using a sample of 95 countries from 1995 to 2015. The results revealed that the growth in nonrenewable energy consumption positively correlates with increased CO2 emissions. Similarly, AlNemer et al. [32] investigated co-movements between renewable and nonrenewable energy sources and CO2 emissions in Saudi Arabia. The findings suggest that nonrenewable energy sources lead to a rise in CO2 emissions. The impact of nonrenewable energy on total carbon productivity is exacerbated by income inequality [33]. The significant deterioration of global climatic conditions has led to increased attention to environmental issues [34]. To achieve carbon neutrality, countries should systematically reduce the proportion of nonrenewable energy in their energy mix while enhancing the contribution of renewable energy sources [35]. Based on the literature stated above, this study outlines the first hypothesis.
Hypothesis 1. Nonrenewable energy consumption has a positive impact on CO2 emissions.
Transport energy consumption can influence nonrenewable energy consumption and, eventually, CO2 emissions. As countries develop their energy infrastructure, they increasingly rely on nonrenewable energy sources for transportation, increasing CO2 emissions. The high cost of clean technologies in transport remains one of the main barriers to achieving a low carbon footprint [36]. Several studies have highlighted that transport energy consumption increases the consumption of nonrenewable energy, which translates to high CO2 emissions. Wang et al. [37] suggested that total transport energy consumption is projected to rise to 636 million tons of oil equivalent (Mtoe) by 2050, leading to 1602 million tons of CO2 emissions. Without significant policy intervention, future energy consumption and CO2 emissions from transport will escalate significantly, and the sector will continue to depend predominantly on fossil fuels [38]. Ağbulut [39] examined the relationship between transportation-related energy demand and CO2 emissions in Turkey. The research indicates that energy demand and CO2 emissions from Turkey's transport industry will rise approximately 3.4 times by the year 2050 compared to current levels. A study by Chandran and Tang [40] revealed a bidirectional causality between transport energy consumption and CO2 emissions.
Mraihi et al. [41] claimed that the fuel intensity of automobiles is the primary factor influencing the energy consumption of road transportation in Tunisia. On the other hand, Peng and Wu [42] demonstrated that the increase in CO2 emissions in the transportation sector in China is linked to the increased use of essential energy resources, particularly petroleum products. The need to enhance economic production among nations brought about the extraction and exploitation of natural resources like minerals, petroleum, coal, and natural gas [43]. Wu et al. [44] documented that the transport sector in China utilizes more energy than the United States. Yadav et al. [45] concluded that fuel stacking will likely remain essential to the socio-cultural energy tradition, influencing progress toward a low-carbon and greener energy transition. Therefore, decarbonizing the transportation sector depends on technological, behavioral, economic, and societal trends [46]. The transportation industry is the fastest-growing energy end-use sector in many countries [47], relying heavily on petroleum products. Therefore, the transport industry accounts for increasing global greenhouse gas emissions. Between 2010 and 2019, the transport sector was the world's fastest-growing combustion (fossil fuel-burning) industry [48]. Saidi [49] indicated that freight transportation and energy consumption contribute significantly to CO2 emissions in 14 Middle Eastern and North African (MENA) nations. In the Philippines, Lopez et al. [50] discovered that transport activity has an inhibitory force, whereas energy intensity is the main contributor. Based on the prepositions above, the second hypothesis is formulated.
Hypothesis 2. Nonrenewable energy consumption significantly affects CO2 emissions through changes in transport energy consumption.
Clean energy characterizes the availability and use of sustainable, renewable, and environmentally friendly energy sources. As of 2022, 2.3 billion people still rely on toxic and hazardous energy sources for cooking [51]. Modifying the energy framework and utilizing clean energy sources can reduce pollutant emissions. Carbon dioxide emissions, the main greenhouse gas contributing to climate change, may be significantly reduced using renewable energy sources and energy efficiency measures [52]. Cai et al. [53] examined the relationship between clean energy consumption, economic growth, and CO2 emissions in G7 countries. The study suggests that clean energy consumption significantly affects CO2 emissions in the US. The integration of sustainable technologies is also facilitated by the interaction of different energy sources [54]. Li and Haneklaus [55] investigated the nexus between clean energy consumption and CO2 emissions from 1979 to 2019. The findings revealed that a 1% increase in clean energy consumption reduces emissions by 0.099%. The energy ladder hypothesis emphasizes increasing access to clean energy to promote sustainable development. The nexus between increased household incomes and the transition to modern fuels is apparent. This transition occurs within a structure of significant fuel stacking [56].
Zambrano-Monserrate [57] studied the relationship between CO2 emissions and clean energy in the OECD countries. According to the authors, increasing clean energy production will reduce CO2 emissions by 0.23% and 0.33%. Xue et al. [58] suggested that developing more green energy sources is crucial to solving today's climate challenges. Also, Yang and Wang [59] investigated the relationship between clean energy, emission trading policies, and CO2 emissions in China. The authors discovered an inverse relationship between clean energy and CO2 emissions. They concluded that carbon trading pilots significantly promoted clean energy in China. Wang et al. [60] noted that high-income countries can utilize AI technology to accelerate their energy transition more effectively because of better infrastructure and technical expertise. Ahmed et al. [61] indicated that clean energy reduces CO2 emissions. Additionally, Tangato [62] studied the impact of clean technologies on carbon emissions in developed and developing countries. The findings indicate that access to renewable energy, clean fuels, and cooking technologies lowers carbon dioxide emissions in developed and developing countries. Bo et al. [63] pointed out that renewable energy resources can reduce emissions. In another study, Ummalla and Goyari [64] examined the impact of clean energy consumption on economic growth and CO2 emissions in BRICS countries. Based on the results, they recommended tax incentives for clean energy initiatives in emerging economies. Clean energy investment is growing much faster than that of fossil fuels. It rose from almost USD 1.13 trillion to USD 1.74 trillion between 2017 and 2023 [65]. Based on the factors mentioned above, the third hypothesis is formulated.
Hypothesis 3. Clean energy significantly moderates the relationship between nonrenewable energy consumption and CO2 emissions.
The conceptual model is presented in Figure 2.
This study employs panel data for 22 African countries from 2001 to 2020. The countries included in the sample represent different economic settings, specifically resource-intensive and non-resource-intensive countries. The sample size consists of 440 observations (20 per country), ensuring variability within the dataset. Countries and variables were selected based on data consistency and emission levels. Countries included in the sample are Angola, Botswana, Cameroon, Congo, Democratic Republic of the Congo, Côte d'Ivoire, Egypt, Ethiopia, Gabon, Ghana, Kenya, Morocco, Mozambique, Niger, Nigeria, Rwanda, Senegal, South Africa, Tanzania, Uganda, Tunisia, and Zambia. The research dataset was retrieved from the World Bank and the International Energy Agency databanks. Table 1 presents a description of the study variables.
Variables | Variable name | Indicator | Unit of measurement | Sources |
Dependent variable | CO2 per capita | lnCO2pc | Metric tons per capita | WDI [66] |
Independent variable | Oil products (nonrenewable energy) | lnNREpc | Terajoules per capita | IEA [67] WDI [66] |
Mediating variable | Transport energy consumption | lnETRpc | Terajoules per capita | IEA [67] WDI [66] |
Moderating variable | Clean energy access (residential) | lnCLE | % of the total population | WDI [66] |
Control variables | GDP per capita | lnGDPpc | Constant 2015 US$ | WDI [66] |
Industrialization | lnIND | % of GDP | WDI [66] | |
Renewable energy consumption | lnREC | % of final energy consumption | WDI [66] | |
Agricultural land | lnAGL | % of land area | WDI [66] | |
Trade | TR | % of GDP | WDI [66] | |
Urbanization | lnURB | % of the total population | WDI [66] |
The countries included in the study are among Africa's top contributors to CO2 emissions. Many of Africa's carbon emissions can be traced to a few countries such as South Africa, Egypt, Algeria, Libya, and Nigeria due to their heavy energy usage, industrial activities, and large population. Therefore, limiting the sample to high-emission countries in Africa allows for an in-depth understanding of their emission patterns and overall impact on world CO2 emissions. Also, due to a lack of complete data on some African nations, only 22 countries will be considered in this study. Several nations, like the Central African Republic, Comoros, Guinea Bissau, Djibouti, and Somalia, have low CO2 emissions. Therefore, their addition would have little influence. Due to several recent changes affecting data collection in Africa, such as the COVID-19 pandemic and a change in the economic framework of certain countries, the research period was not extended to recent years. Political instability in some African countries makes it challenging to provide accurate statistics.
Similar to Li et al. [68], this research utilizes CO2 emissions per capita (measured in metric tons per capita) as the dependent variable. This variable is consistent and complete for all countries included in the sample. Data for the dependent variable was retrieved from the World Bank indicator databank. Using per capita CO2 emissions facilitates comparisons across countries with varying population sizes and economic frameworks.
The primary independent variable is nonrenewable energy consumption. In this study, the total oil consumption serves as an indicator of nonrenewable energy consumption. Oil products include petrol, diesel, motor petrol, liquefied petroleum gas (LPG), ethane, crude oil, natural gas liquids (NGL), jet kerosene, fuel oil, other kerosene, naphtha, and other oil products. The variable for total oil consumption was sourced from the International Energy Agency databank, while the total population variable was obtained from the World Bank indicator databank. The calculation of this variable involves dividing the total consumption of nonrenewable energy by the total population. The measurement formula is stated as follows:
NREpc=(totalnon−renewableenergyconsumptiontotalpopulation) |
This study used transport energy consumption as a mediating variable. Transport energy consumption includes energy consumed by different means of transport such as vehicles, trains, ships, and aircraft. The indicator was retrieved from the International Energy Agency databank. The calculation of this variable involves dividing the total transport energy consumption by the total population. The measurement formula is stated as follows:
ETRpc=(totaltransportenergyconsumptiontotalpopulation) |
Clean energy is used as a moderating variable in this study. Due to the limited availability of reliable data on clean energy access in Africa, this study used access to clean fuels and technologies for cooking (% of the total population) as the indicator for clean energy. Access to clean fuels and technologies for cooking can act as a substitute for broader clean energy access in Africa due to its direct influence on environmental sustainability, especially in developing countries where traditional biomass fuels are commonly used. The variable was gathered from the World Bank indicator databank.
i. GDP per capita (GDPpc): GDP per capita refers to per capita gross domestic product in constant 2015 U.S. dollars. GDP per capita measures a country's economic performance and living standard. This variable is calculated by dividing the total gross domestic product by the total population. The GDP per capita indicator has gained momentum in empirical research due to its scope in capturing the income levels of different countries [69,70,71]. The effect of economic growth on emissions differs across African countries [72].
ii. Industrialization (IND): Industrialization is essential to a country's economic landscape. As countries engage in trade, there is an increase in industrial activities. The industry value comprises mining, manufacturing, construction, electricity, water, and gas. Industrialization is measured as a percentage of the gross domestic product [66]. Several researchers used industrialization as a variable in their studies on CO2 emissions. Using industrialization as an explanatory variable, Mentel et al. [73] revealed that industrialization positively impacts CO2 emissions in sub-Saharan Africa. This variable determines the CO2 emissions caused by nonrenewable energy during industrial expansion.
iii. Renewable energy consumption (REC): Renewable energy refers to the ratio of energy derived from renewable energy sources such as solar, wind, geothermal, hydropower, and bioenergy. Renewable energy is the primary route in achieving carbon neutrality. The low usage of modern renewable energy in Africa can be attributed to income disparities, minimal technical expertise, and unfavorable markets [74]. Several studies have employed renewable energy as an indicator in their research on CO2 emissions. Chen et al. [31] revealed that renewable energy has a profound impact on CO2 emissions. Besides that, using renewable energy consumption as a control variable is very relevant because it shows countries shifting away from the consumption of fossil fuels.
iv. Agricultural land (AGL): Agricultural land is cultivated land planted with permanent pastures and crops. It covers over one-third of the planet's land [66]. Nitrogen and agricultural CH4 emissions must be reduced by 26% and 48% by 2030 [75] to control global warming. Agricultural greenhouse gas emissions are incredibly challenging in emerging economies since agricultural transformation is associated with industrialization [76].
v. Trade (TR): Trade refers to the buying and selling of goods between two or more parties. According to the World Bank [66], trade openness encompasses the total value of exports and imports of goods and services, expressed as a percentage of gross domestic product. Due to the relevance of trade in the global economy, several studies have adopted the variable as a measure of trade openness and its effect on the environment. Haug and Ucal [77] used trade as one of their main independent variables and found that it significantly impacts CO2 emissions. This variable is crucial since it allows researchers to control the economic setting in which energy consumption happens.
vi. Urbanization (URB): Urbanization refers to the population living in a particular metropolitan area or city. In recent years, cities have expanded, prompting people to relocate from rural to urban locations [66]. As a result, metropolitan areas have higher population densities and require more infrastructure, which increases their energy demand and the rate of CO2 emissions from transportation, residential, and commercial activities. In industrialized nations, urbanization is frequently related to economic growth and environmental degradation [78].
The subsequent models were constructed to address the research hypotheses. All the research variables except for trade were transformed into natural logarithms to achieve a constant variance.
To test for the direct impact of nonrenewable energy consumption on CO2 emissions, this study constructs Model (1) as shown in Eq 1:
lnCO2pci,t=δ+β0lnNREpci,t+φiControlsi,t+λi+γi+εi,t | (1) |
where CO2pci, t represents CO2 per capita (dependent variable) of country i in year t. NREpci, t denotes nonrenewable energy consumption per capita of country i (N = 22) in year t (T = 20). Controlsi, t represents the control variables of country i in year t. δ represents the intercept, and β0 and φi are the regression parameters. λi and γi represent the year and country dummies, respectively. εi,t is the residual term.
This study employs Eqs 2 and 3 to test for the mediating effect of transport energy consumption. This study follows the approach of Baron and Kenny [79] to assess the mediating effect of transport energy consumption on the nexus between nonrenewable energy consumption and CO2 emissions. Based on the framework, three key conditions must be satisfied to establish mediation. First, nonrenewable energy consumption must significantly affect CO2 emissions. Second, nonrenewable energy consumption must significantly affect transport energy consumption. Finally, the direct effect of nonrenewable energy on CO2 emissions should be significantly or non-significantly reduced when transport energy consumption is added to the model. Therefore, Eqs 2 and 3 show the mediating effect models.
lnETRpci,t=δ+β1lnNREpci,t+φiControlsi,t+λi+γi+εi,t | (2) |
lnCO2pci,t=δ+β2lnNREpci,t+β3lnETRpci,t+φiControlsi,t |
+λi+γi+εi,t | (3) |
In these equations, ETRpci, t represents the transport energy consumption of country i in year t.
This study utilizes Model (4) to test for the moderating effect of clean energy. The model is presented in Eq 4.
lnCO2pci,t=δ+β0lnNREpci,t+β1lnCLEi,t+β2(lnNREpci,t×lnCLEi,t) |
+φiControlsi,t+λi+γi+εi,t | (4) |
where CLEi, t represents the clean energy of country i in year t. A significant interaction term (β2) indicates that the moderator influences the strength of the relationship between nonrenewable energy consumption and CO2 emissions.
Table 2 presents the descriptive statistics of the research variables. For the entire sample, this study revealed that the mean value and standard deviation for CO2 per capita (CO2pc), nonrenewable energy consumption (NREpc), transport energy consumption (ETRpc), clean energy (CLE), and GDP per capita (GDPpc) are 1.146 (1.719), 0.005 (0.004), 0.003 (0.002), 31.993 (34.913), and 2160.890 (1864.198), respectively. For the resource groups, resource-intensive countries (RIC) account for the highest mean values for CO2 per capita, nonrenewable energy consumption, transport energy consumption, clean energy, and GDP per capita at 1.499, 0.006, 0.004, 33.636, and 2650.406, respectively. Conversely, non-resource-intensive countries account for the lowest mean values for CO2 per capita (0.635), nonrenewable energy consumption (0.003), transport energy consumption (0.002), clean energy (29.619), and GDP per capita (1453.812).
Variables | Full sample mean (st. dev) | RIC mean (st. dev) | NRIC Mean (st. dev) |
CO2pc | 1.146 (1.719) |
1.499 (2.066) |
0.635 (0.791) |
NREpc | 0.005 (0.004) |
0.006 (0.006) |
0.003 (0.003) |
ETRpc | 0.003 (0.002) |
0.004 (0.004) |
0.002 (0.002) |
CLE | 31.993 (34.913) |
33.636 (32.756) |
29.619 (37.780) |
GDPpc | 2160.890 (1864.198) |
2650.406 (2124.403) |
1453.812 (1068.710) |
IND | 29.071 (12.513) |
34.886 (12.905) |
20.673 (4.798) |
REC | 63.516 (30.276) |
63.198 (29.860) |
63.974 (30.946) |
AGL | 45.254 (22.066) |
36.408 (22.350) |
58.031 (13.911) |
TR | 63.367 (26.170) |
66.541 (28.597) |
58.783 (21.460) |
URB | 44.359 (18.688) |
49.865 (17.341) |
36.407 (17.714) |
*Note: RIC represents resource-intensive countries; NRIC indicates non-resource-intensive countries. St. dev represents the standard deviation (in parentheses). |
Some tests were conducted between models to determine the appropriate model for estimating our variables. The results of the Breusch-Pagan Lagrange multiplier and Hausman tests are presented in Table 3. The Breusch-Pagan Lagrange multiplier assessed the most compatible model between the pooled ordinary least squares (OLS) and the random effect model [80]. The test outlines a significant probability value at the 1% level. Therefore, the null hypothesis is rejected, suggesting that the random effect is much better than the pooled OLS. The Hausman test was adopted to examine the most accurate model between the fixed and random effect models [81]. The probability value of the Hausman test for the baseline and mediating effect regressions suggested that the random effect is the best. Conversely, the moderating effect model indicated that the fixed effect is the most appropriate estimator. Both the fixed effect and random effect models control for individual-specific effects [82]. According to Baltagi [83], the random effect model does not allow for endogenous regressors or individual effects, whereas the fixed effect model does. The random effect model can be further extended to include random coefficients, cross-level interactions, and complex variance functions [84]. These models make fundamentally different assumptions, and selecting the right model is crucial [85].
Model | Breusch-Pagan Lagrange multiplier test |
Hausman test | Conclusion |
Baseline model | 2268.97*** [0.0000] |
18.70 [0.8112] |
Random effect |
Mediating effect model | 2163.78*** [0.0000] |
20.04 [0.7895] |
Random effect |
Moderating effect model | 2298.92*** [0.0000] |
43.81** [0.0290] |
Fixed effect |
*Note: p<0.05: reject null hypothesis; P≥0.05: fail to reject the null hypothesis. |
The findings of the baseline model where lnCO2pc is the dependent variable are outlined in Table 4, column 1. The results revealed that nonrenewable energy consumption (NREpc) significantly affects CO2 emissions at the 1% level. A 1% rise in nonrenewable energy consumption leads to an increase in CO2 emissions of 0.34%. The findings show that the usage of nonrenewable energy increases carbon emissions. Similarly, Apergis et al. [86] postulated that nonrenewable energy positively impacts the level of per capita CO2 emissions in Uzbekistan. This is a significant discovery for policymakers and stakeholders because it underscores the adverse effect of nonrenewable energy on the environment and the need to transition to clean energy sources. This finding supports hypothesis 1 and aligns with several studies [87,88], which indicate that nonrenewable energy consumption deteriorates the environment. The consumption of nonrenewable energy substantially contributes to pollution and climate change. Historically, fossil fuels have been the primary source of energy in Africa. However, excessive use of nonrenewable energy presents various environmental challenges [89]. Carbon dioxide emissions from fossil fuels account for approximately 68% of current greenhouse gas emissions. These emissions are generated by coal, oil, and gas combustion in the energy sector and by industrial processes related to metal, cement, and other materials [90]. The control variables indicate that GDPpc (β=0.557,p<0.01), IND (β=0.119,p<0.05), TR (β=0.004,p<0.01), and URB (β=0.473,p<0.01) increase CO2 emissions. Conversely, REC (β=−0.303,p<0.01) reduces CO2 emissions.
Variables | Model (1) | Model (2) | Model (3) |
lnCO2pc | lnETRpc | lnCO2pc | |
lnNREpc | 0.340*** | 0.929*** | 0.184** |
(0.041) | (0.033) | (0.076) | |
lnETRpc | 0.181*** | ||
(0.067) | |||
lnGDPpc | 0.557*** | 0.119* | 0.418*** |
(0.073) | (0.061) | (0.080) | |
lnIND | 0.119** | 0.103** | 0.173*** |
(0.054) | (0.045) | (0.060) | |
lnREC | -0.303*** | -0.192*** | -0.150 |
(0.067) | (0.068) | (0.091) | |
lnAGL | 0.071 | 0.705*** | -0.459* |
(0.083) | (0.187) | (0.251) | |
TR | 0.004*** | -0.001* | 0.004*** |
(0.001) | (0.000) | (0.001) | |
lnURB | 0.473*** | 0.339** | 0.475*** |
(0.134) | (0.134) | (0.179) | |
Constant | -4.139*** | -4.854*** | -1.866 |
(0.781) | (0.876) | (1.199) | |
Year dummies | YES | YES | YES |
Country dummies | YES | YES | YES |
Observations | 440 | 440 | 440 |
R-squared | 0.648 | 0.879 | 0.659 |
*Note: Standard errors are in parentheses. *** P < 0.01, ** P < 0.05, * P < 0.10. |
Columns 2 and 3 of Table 4 show the results of Eqs 2 and 3 using the random effects generalized least squares (RE-GLS) regression. In column 2, ETRpc is the dependent variable, and the findings reveal a positive and significant relationship between NREpc and transport energy consumption. A 1% increase in nonrenewable energy consumption leads to an increase in transport energy consumption of 0.93%. Column 3 (Table 4) presents the estimation results of Eq 3, where CO2pc is the dependent variable. The results show that the estimate of ETRpc is positive and significant at the 1% level (β=0.181,p<0.01). Therefore, increased transport energy consumption leads to a rise in CO2 emissions. The coefficient of NREpc (column 3, Table 4) is positive and significant at the 5% level (β=0.184,p<0.05). The coefficient of nonrenewable energy is significantly reduced once transport energy consumption is included in the model. Therefore, transport energy consumption mediates the relationship between nonrenewable energy consumption and CO2 emissions. Over the years, African countries have experienced an influx of different transportation means, most of which require fossil fuel energy. Transportation is a vital tool for economic development, but emissions from transportation means are becoming more intense. A change in the transport system is required to ensure sustainable mobility and carbon neutrality.
The regression outcome suggested that nonrenewable energy consumption affects CO2 emissions through changes in transportation energy consumption, thus confirming Hypothesis 2. The effect of NREpc on CO2 emissions shows a direct effect of 0.184, an indirect effect of 0.168 (0.929×0.181), and a total effect of 0.352 (0.184+0.168). This indicates that transport energy usage is a major driver in rising CO2 emissions. These results highlight that increased reliance on fossil fuels for transportation leads to an increase in CO2 emissions. The performance of transport systems influences the emissions produced for each unit of nonrenewable energy consumed. An improvement in technology and a transition toward sustainable methods can potentially reduce emission levels [91,92,93]. The energy consumption theory suggests that as economies expand, there is an increase in energy consumption levels due to a rise in economic activities. In column 3 (Table 4), all the control variables are significant except for REC. The findings revealed that GDPpc (β=0.418,p<0.01), IND (β=0.173,p<0.01), TR (β=0.004,p<0.01), and URB (β=0.475,p<0.01) have a positive and significant effect on CO2 emissions. In contrast, AGL (β=−0.459,p<0.10) exhibits an inverse relationship with CO2 emissions.
The moderating effect result of Eq 4 is shown in Table 5. Column 1 reveals the moderating effect of clean energy on the relationship between nonrenewable energy consumption and CO2 emissions. The findings suggested that CLE (β=−0.129,p<0.01) has a negative and significant impact on CO2 emissions. Additionally, the interaction term (NREpc×CLE) also shows a negative and significant effect. A percentage increase in the interaction value (NREpc×CLE) reduces CO2 emissions by 0.078%. This result validates Hypothesis 3. The finding aligns with the energy ladder hypothesis, which posits that as household income increases, there is a transition to cleaner energy sources. When households or industries have dependable access to clean energy sources, such as solar, wind, or hydroelectric power, their dependence on fossil fuels for energy diminishes. This transition decreases total CO2 emissions and modifies energy consumption dynamics. The controlled variables are all significant at the 1% level. GDPpc (β=0.448,p<0.01), IND (β=0.195,p<0.01), TR (β=0.002,p<0.01), and URB (β=0.439,p<0.01) are shown to have a positive effect on CO2 emissions. Conversely, REC (β=−0.224,p<0.05) and AGL (β=−1.027,p<0.01) exhibit an inverse relationship with CO2 emissions. These findings suggest that GDPpc, IND, TR, and URB increase CO2 emissions. In contrast, renewable energy consumption and agricultural land usage have been shown to reduce CO2 emissions. These results align with previous studies [94,95,96].
Variables | Model (4) | Resource | Non-resource |
Moderator: lnCLE | intensive countries | intensive countries | |
lnNREpc | 0.380*** | 0.362*** | 0.310*** |
(0.043) | (0.058) | (0.065) | |
lnCLE | -0.129*** | -0.105*** | 0.044 |
(0.021) | (0.034) | (0.027) | |
lnNREpc×lnCLE | -0.078*** | -0.111*** | 0.015 |
(0.012) | (0.015) | (0.018) | |
lnGDPpc | 0.448*** | 0.560*** | 0.107 |
(0.083) | (0.141) | (0.099) | |
lnIND | 0.195*** | 0.160* | 0.142** |
(0.057) | (0.085) | (0.061) | |
lnREC | -0.224** | -0.488*** | -0.020 |
(0.093) | (0.143) | (0.105) | |
lnAGL | -1.027*** | -1.667*** | 0.473 |
(0.253) | (0.338) | (0.356) | |
TR | 0.002*** | 0.002*** | 0.007*** |
(0.001) | (0.001) | (0.001) | |
lnURB | 0.439*** | -0.453 | 1.786*** |
(0.168) | (0.275) | (0.215) | |
Constant | -1.717 | 3.878** | -10.524*** |
(1.191) | (1.723) | (1.550) | |
Year dummies | YES | YES | YES |
Country dummies | YES | YES | YES |
Observations | 440 | 260 | 180 |
R-squared | 0.695 | 0.614 | 0.897 |
*Note: Standard errors are in parentheses. *** P < 0.01, ** P < 0.05, * P < 0.10. |
As shown in column 2 of Table 5, CLE (β=−0.105,p<0.01) and the interaction term (NREpc×CLE) (β=−0.111,p<0.01) reduce CO2 emissions for resource-intensive countries. This suggests that clean energy significantly moderates the nexus between NREpc and CO2 emissions in resource-intensive countries. Conversely, the CLE coefficient and the interaction value are positive and insignificant for non-resource-intensive countries. The estimates of the resource-intensity groups revealed that limited access to clean energy increases CO2 emissions in non-resource-intensive countries. The limited access to clean energy in non-resource-intensive countries results from various interconnected factors, such as economic limitations, insufficient infrastructure, and restricted investment in renewable technologies.
This section examines the mediating effect of transport energy consumption based on resource levels. The panel is divided into two groups: resource-intensive countries and non-resource-intensive countries (see Table A1). As shown in the results in column 3 of Table 6, NREpc exhibits a positive and significant effect on CO2 emissions in resource-intensive countries (β=0.329,p<0.01). The effect of NREpc on CO2 emissions shows a direct impact of 0.329, an indirect effect of 0.024 (0.873×0.027), and a total effect of 0.353 (0.329+0.024). Additionally, column 6 (Table 6) revealed that NREpc has a positive and significant effect on CO2 emissions (β=0.075,p<0.01) in non-resource-intensive countries. The effect of NREpc on CO2 emissions shows a direct effect of 0.075, an indirect effect of 0.068 (0.174×0.389), and a total effect of 0.143 (0.075+0.068). In summary, transport energy consumption is a significant mediator in resource-intensive and non-resource-intensive countries. Nonrenewable energy consumption has a greater influence on CO2 emissions in resource-intensive countries. International financial aid initiatives for developing countries, such as the Green Climate Fund, are critical to reducing greenhouse gas emissions [97].
Variable | Resource intensive countries | Non-resource intensive countries | ||||
lnCO2pc (1) |
lnETRpc (2) |
lnCO2pc (3) |
lnCO2pc (4) |
lnETRpc (5) |
lnCO2pc (6) |
|
lnNREpc | 0.351*** | 0.873*** | 0.329*** | 0.297*** | 0.174*** | 0.075*** |
(0.056) | (0.044) | (0.092) | (0.057) | (0.028) | (0.020) | |
lnETRpc | 0.027* | 0.389*** | ||||
(0.084) | (0.051) | |||||
lnGDPpc | 0.785*** | 0.258** | 0.759*** | 0.254*** | 0.389*** | 0.591*** |
(0.130) | (0.104) | (0.134) | (0.075) | (0.050) | (0.037) | |
lnIND | 0.059 | 0.199*** | 0.060 | 0.188*** | 0.746*** | 0.148** |
(0.085) | (0.067) | (0.087) | (0.062) | (0.093) | (0.070) | |
lnREC | -0.375*** | -0.065 | -0.380*** | -0.054 | -0.360*** | -0.304*** |
(0.109) | (0.090) | (0.114) | (0.075) | (0.046) | (0.034) | |
lnAGL | 0.020 | 0.336*** | -0.004 | 0.121 | -0.253*** | -0.437*** |
(0.117) | (0.103) | (0.131) | (0.221) | (0.070) | (0.046) | |
TR | 0.004*** | -0.001* | 0.004*** | 0.005*** | 0.004*** | 0.002** |
(0.001) | (0.001) | (0.001) | (0.001) | (0.001) | (0.001) | |
lnURB | -0.155 | -0.084 | -0.164 | 1.258*** | 0.105 | 0.292*** |
(0.238) | (0.192) | (0.244) | (0.149) | (0.080) | (0.051) | |
Constant | -2.734** | -4.150*** | -2.374* | -6.516*** | -8.591*** | -0.832 |
(1.144) | (0.932) | (1.227) | (1.105) | (0.487) | (0.536) | |
Year dummies | YES | YES | YES | YES | YES | YES |
Country dummies | YES | YES | YES | YES | YES | YES |
Observations | 260 | 260 | 260 | 180 | 180 | 180 |
R-squared | 0.505 | 0.866 | 0.507 | 0.890 | 0.718 | 0.836 |
*Note: Standard errors are in parentheses. *** P < 0.01, ** P < 0.05, * P < 0.10. |
Nigeria, Angola, and South Africa are among the African countries heavily reliant on fossil fuel exports. According to Oladunni et al. [98], Nigeria and Angola play an important role in upstream fossil fuel production. These nations' transportation sectors are mostly made up of heavy-duty diesel-powered vehicles. Non-resource-intensive countries like Kenya, Uganda, and Rwanda depend on agriculture and other services for economic growth. Additionally, some non-resource-intensive countries are inclined to use public transportation. Nonetheless, these countries face issues of urbanization and increased automobile ownership, which increases CO2 emissions.
The presence of autocorrelation, heteroskedasticity, and cross-sectional dependence in the sample can cause inconsistent and biased estimates. Therefore, this study adopts the Wooldridge [99] test for autocorrelation and the modified Wald statistics for groupwise heteroskedasticity [100]. The Wooldridge test identifies first-order autocorrelation in the residuals. The Wald test detects the issue of group-wise heteroskedasticity, especially when dealing with grouped data. Table 7 presents the results of the autocorrelation, heteroskedasticity, and cross-sectional dependence tests. The heteroskedasticity and autocorrelation tests show statistic values of 2281.48 and 111.65, respectively. These values are statistically significant at the 1% level. Therefore, the null hypothesis of no autocorrelation and heteroskedasticity is rejected. Additionally, the Pesaran [101] test for cross-sectional dependence was adopted. The findings revealed the presence of cross-sectional dependence in the sample.
Test | Statistic | Conclusion |
Modified Wald test for group-wise heteroskedasticity chi2 (22) | 2281.48*** [0.0000] |
Present |
Wooldridge test for autocorrelation F (1, 21) | 111.65*** [0.0000] |
Present |
Pesaran's test of cross-sectional dependence | -2.702*** [0.0069] |
Present |
*Note: *** P < 0.01, ** P < 0.05, * P < 0.10. |
To test for robustness, this study employed the Driscoll and Kraay standard errors (DKSE) estimator to address issues of heteroskedasticity and autocorrelation in the sample [29]. This test can be used in both balanced and unbalanced panel data. Additionally, this estimator is suitable for panels with larger cross-sections and small-time dimensions (N > T). Table 8 presents the robustness test result. The result in column (1) supports the findings of the baseline regression and confirms Hypothesis 1, indicating that nonrenewable energy consumption has a positive and significant effect on CO2 emissions (β=0.174,p<0.01). The findings revealed that for every percentage increase in the consumption of nonrenewable energy, there is a corresponding increase in CO2 emissions of 0.17%. The coefficients of GDPpc (β=1.079,p<0.01), AGL (β=0.049,p<0.10), and URB (β=0.118,p<0.01) revealed a positive and significant relationship with CO2 emissions. Conversely, REC (β=−0.339,p<0.01) showed an inverse relationship with CO2 emissions. Column 2 indicates that the coefficient of NREpc (β=0.224,p<0.01) has a positive and significant effect on transport energy consumption. After including ETRpc in Column (3), the result shows that the coefficient of NREpc (β=0.103,p<0.01) is significantly reduced. The result revealed a direct effect of 0.103, an indirect effect of 0.071(0.224×0.317), and a total effect of 0.174 (0.103+0.071). The result supports Hypothesis 2, suggesting that transport energy consumption mediates the relationship between nonrenewable energy consumption and CO2 emissions.
(1) | (2) | (3) | |
Variables | lnCO2pc | lnETRpc | lnCO2pc |
lnNREpc | 0.174*** | 0.224*** | 0.103*** |
(0.026) | (0.030) | (0.027) | |
lnETRpc | 0.317*** | ||
(0.048) | |||
lnGDPpc | 1.079*** | 0.471*** | 0.930*** |
(0.026) | (0.040) | (0.033) | |
lnIND | -0.063 | 0.305*** | -0.159 |
(0.106) | (0.063) | (0.100) | |
lnAGL | 0.049* | 0.241*** | -0.028 |
(0.028) | (0.029) | (0.034) | |
lnREC | -0.339*** | -0.394*** | -0.214*** |
(0.016) | (0.026) | (0.018) | |
TR | 0.003 | -0.001 | 0.003* |
(0.002) | (0.001) | (0.001) | |
lnURB | 0.118*** | 0.080 | 0.093** |
(0.039) | (0.051) | (0.039) | |
Constant | -6.716*** | -8.995*** | -3.867*** |
(0.216) | (0.358) | (0.604) | |
Year dummies | YES | YES | YES |
Country dummies | YES | YES | YES |
Observations | 440 | 440 | 440 |
R-squared | 0.960 | 0.923 | 0.964 |
*Note: Standard errors are in parentheses. *** P < 0.01, ** P < 0.05, * P < 0.10. |
Endogeneity tests are a major requirement in research as they analyze the degree of correlation between a specific explanatory variable and the error term in a regression model, which may cause bias and inconsistent estimates [102]. This correlation could be due to omitted variable bias. The endogeneity issue in conventional stochastic frontier models may result in inconsistent parameter estimates [103]. This study used the instrumental variable method, as stated in the study of Wang et al. [104], to check for endogeneity in the sample. The lag of nonrenewable energy consumption (L.lnNREpc) is employed as the instrumental variable, which implies that past nonrenewable energy consumption dynamics can influence current patterns. Table 9 outlines the result of the two-stage least squares (2SLS) estimator. Column 1 presents the regression of the explanatory variable (lnNREpc) on the instrumental variable (L.lnNREpc) and the controlled variables. The results revealed a positive and significant coefficient for the lag of nonrenewable energy consumption. In the second stage regression presented in column 2, the study revealed a positive and significant coefficient for nonrenewable energy consumption (lnNREpc). The findings suggested that for every percentage increase in the consumption of nonrenewable energy, there is a corresponding increase in CO2 emissions of 0.17%. The coefficient of NREpc is still positive and significant. The results of the Durbin and Wu-Hausman tests showed a probability value greater than the 10% significance level. Therefore, the null hypothesis is accepted, suggesting the model has no endogeneity issue.
Variables | First stage | Second stage |
lnNREpc | lnCO2pc | |
L.lnNREpc | 0.974*** | |
(0.009) | ||
lnNREpc | 0.166*** | |
(0.026) | ||
lnGDPpc | 0.012 | 1.078*** |
(0.016) | (0.043) | |
lnIND | 0.004 | -0.102 |
(0.025) | (0.066) | |
lnAGL | 0.008 | 0.034 |
(0.009) | (0.024) | |
lnREC | -0.021** | -0.342*** |
(0.009) | (0.024) | |
TR | 0.001*** | 0.003*** |
(0.000) | (0.001) | |
lnURB | -0.029 | 0.125** |
(0.023) | (0.061) | |
Constant | -0.133 | -6.783*** |
(0.136) | (0.368) | |
Observations | 418 | 418 |
R-squared | 0.993 | 0.957 |
Endogeneity test | Statistics | P-value |
Durbin (score) | 0.0704 | 0.7908 |
Wu-Hausman | 0.0689 | 0.7931 |
*Note: Standard errors are in parentheses. *** P < 0.01, ** P < 0.05, * P < 0.10. |
The evidence obtained from the research findings suggests that nonrenewable energy positively impacts Africa's rising CO2 emissions. The findings align with several empirical studies. Rai et al. [105] indicated that the negative environmental effect of oil energy is significantly high in both industrialized and developing nations. Oil burning emits a large amount of carbon, accounting for around one-third of global emissions [18]. Nonrenewable energy sources in developing countries are often misused or poorly managed for various socioeconomic reasons [106]. Therefore, African countries must swiftly transition to renewable energy sources such as solar, wind, and hydropower.
The findings also indicate that energy used in transport mediates the relationship between nonrenewable energy consumption and CO2 emissions in Africa. For that reason, policymakers need to establish an integrated transportation policy that encourages better practices. Fossil fuels are the primary energy source for the transportation sector, so total consumption of these fuels has surged in recent decades, particularly in low- and middle-income nations. In emerging and developing countries, rising population and income levels drive demand for private vehicles [107]. Most energy used in transportation comes from fossil fuels (e.g., diesel and petrol) [108]. Satrovic et al. [43] established that transportation energy use and natural resource dependence significantly contribute to the environmental footprint of innovative countries. The South African transport industry has grown considerably but faces several infrastructural and ecological concerns, including greenhouse gas emissions (GHGs) and rising energy usage. According to Aba et al. [109], transitioning from petrol to compressed natural gas will bring long-term economic, resource, and environmental benefits. These benefits will conserve resources and reduce CO2 emissions. Transportation planning in Africa must be more integrated, including adaptation to climate change and future climate conditions [110]. Implementing energy conservation incentives, such as higher fuel prices, can have the same effect as increasing renewable energy adoption. This is particularly true in transportation, where low gasoline and diesel prices encourage more car use and public transportation [111].
The findings indicate that clean energy reduces CO2 emissions. The study further revealed that the moderating effect of clean energy is more pronounced in resource-intensive countries. The findings are practical as nations with abundant natural resources have the means and expertise to control climate change and invest in sustainable energy sources compared to non-resource-intensive countries [112]. African countries must thus increase their investment in renewable energy sources such as solar, wind, hydropower, and biomass. As of 2023,891.8 MW of geothermal capacity had been developed, accounting for around 47% of Kenya's renewable energy mix. This is essential for Africa's pursuit of a sustainable energy transformation [113]. Several other studies address the role of clean energy in the energy mix. Naeem et al. [114] emphasized the need for OECD countries to significantly increase their renewable energy and clean fuels while encouraging more efficient usage. Similarly, Zhou et al. [115] indicated that green financing, eco-regulation, and promoting clean energy projects through renewable energy sources significantly lower carbon emissions in emerging Asian countries.
The Integrated Resource Plan (IRP) developed by South African authorities is a blueprint for transitioning to a more diverse energy mix, incorporating renewable energies and encouraging investment in efficient and clean coal technology [116]. This strategy reduces emissions and improves energy security. In 2020, the Southern Africa subregion had the highest renewable energy capacity (17 gigawatts), accounting for nearly one-third of Africa's total output. North Africa had 12.6 gigawatts, accounting for one-fourth of Africa's total capacity [117]. The Noor Ouarzazate Solar Power Complex demonstrates how government support can stimulate large-scale solar projects, generating employment opportunities and reducing greenhouse gas emissions. The initiative is part of Morocco's energy policy of 2010–2030, which aims to increase energy supply and diversify production through renewable energy [118]. In contrast, the Democratic Republic of Congo is far from meeting the Sustainable Development Goals (SDGs) due to inefficient resource management, lax public policy, and political instability [119]. Filho et al. [120] noted that governance issues are among Africa's common causes of energy poverty. Investment in sustainable energy technology is essential for Africa's long-term development [65]. The green energy revolution enables the economy to achieve carbon neutrality and makes energy accessible [121].
The heterogeneity analysis reveals that transport energy usage is a key mediator between nonrenewable energy consumption and CO2 emissions in resource-intensive and non-resource-intensive countries. Countries that rely heavily on natural resources for economic growth may face high consumption of nonrenewable energy, resulting in a high demand for transportation energy and significant CO2 emissions from outdated transport infrastructure. Mohsin and Jamaani [122] argued that natural resources substantially impact environmental sustainability and greenhouse gas emissions. Similarly, Huang and Ren [123] found that excessive utilization of nonrenewable energy resources increases greenhouse gas emissions and contributes to climate change. On the other hand, in non-resource-intensive countries, the mediating effect of transport energy consumption is also observed. However, factors like urbanization rate and infrastructural development may shape the nature of that relationship.
The transportation infrastructure of North African countries, like Libya and Egypt, is much more advanced than that of many countries in Southern Africa. Past investments in infrastructure and favorable geographic location explain this development. Egypt, with the Suez Canal [124], is an important maritime route linking the Mediterranean Sea to the Red Sea and an extensive network of roads and railways. In contrast, many Southern African countries face problems of poor road networks and much less efficient train services. South Africa has a well-developed transportation infrastructure, including the Gautrain rapid rail link [125]. In contrast, the neighboring countries, Zimbabwe and Mozambique, struggle with poor infrastructure [126]. The condition and scope of transport infrastructure in Africa significantly affect energy usage, with clear differences between subregions. Western Africa struggles with poorly maintained roads and limited public transport options, which can lead to increased fuel consumption and emissions. Nigeria struggles with congested traffic and inadequate road networks [127]. On the other hand, Eastern Africa has invested more in rail networks such as the Standard Gauge Railway in Kenya, improving freight functionality, decreasing road transport, and reducing energy usage [128]. However, issues such as the underapplication of rail services because of their limited integration with other transport modes exist in Eastern Africa, affecting energy efficiency [129].
South American countries such as Venezuela and Brazil depend on their oil reserves for energy. However, countries in South America, such as Brazil and Chile, have significantly invested in hydropower and solar power. Brazil produces over 66% of its electricity from hydropower [130]. Chile is one of the leading countries in solar power production and houses one of the largest solar power plants in the Atacama Desert [131]. These figures indicate the continuous advancement of renewable energy in South America, a move Africa can adopt. The Southeast Asian region has been marked by rising energy consumption and automobile ownership [132]. As the population grows, more private cars are on the road, increasing air pollution in the region. Southeast Asian countries have adopted efficient public transportation systems such as the Bus Rapid Transit (BRT) in Bangkok and Jakarta and the Mass Rapid Transit (MRT) in Singapore to reduce the use of private vehicles and pollution.
The transport industry significantly increases greenhouse gas emissions and is expected to continue this trend unless substantial investments are made in clean energy alternatives. To meet the 2050 scenario of net zero emissions, CO2 emissions from the transportation sector must be reduced by more than 3% annually until 2030 [16]. African countries must establish a sustainable regulatory framework and invest in clean energy.
This study employed panel data from 22 African countries to examine the mediating effect of transport energy consumption on the relationship between nonrenewable energy consumption and CO2 emissions. The baseline regression supports Hypothesis 1, and the mediating effect regression validates Hypothesis 2. In the mediating effect regression, the study reveals a direct effect of 0.184, an indirect effect of 0.168 (0.929×0.181), and a total effect of 0.352 (0.184+0.168). The findings reveal that nonrenewable energy consumption significantly increases CO2 emissions, with transport energy consumption as a significant mediator. As economies grow, there is an increase in the demand for nonrenewable energy, which is cheap compared to renewable energy, therefore increasing energy usage in transport and other sectors. The increase in the consumption of fossil fuels deteriorates the environment through CO2 emissions. Furthermore, the results show that clean energy moderates the relationship between nonrenewable energy consumption and CO2 emissions, confirming Hypothesis 3. This means that clean energy can mitigate the adverse effects of fossil fuel consumption. The moderating impact of clean energy is found to be stronger for resource-intensive countries compared to non-resource-intensive countries. Non-resource-intensive countries have limited resources to transition to alternative energy sources. Conversely, resource-intensive countries may have more access to clean energy resources and modern infrastructure. This assumption confirms the Environmental Kuznets Curve (EKC) theory, which posits that as socioeconomic status improves, there is a transition to modern and advanced energy alternatives.
The resource-level analysis shows that the mediating effect of transport energy consumption is supported in resource-intensive and non-resource-intensive countries. The effect of nonrenewable energy consumption on CO2 emissions shows a direct impact of 0.329, an indirect effect of 0.024 (0.873×0.027), and a total effect of 0.353 (0.329+0.024) in resource-intensive countries. For non-resource-intensive countries, the study revealed a direct effect of 0.075, an indirect effect of 0.068 (0.174×0.389), and a total effect of 0.143 (0.075+0.068). The economies of resource-intensive countries rely heavily on natural resources, making transportation essential to support these operations. Additionally, the increase in transport energy consumption in non-resource-intensive countries can be attributed to urbanization and commercial activities. The Driscoll and Kraay standard errors (DKSE) estimator was used to test for robustness. The findings derived from the DKSE estimator revealed that nonrenewable energy consumption increases CO2 emissions, and transport energy usage mediates this relationship. The two-stage least squares (2SLS) indicated no presence of endogeneity in the sample.
The study outlines several recommendations based on the research findings. First, African countries should invest in environmentally friendly and effective public transport systems, such as electric buses and railway networks, powered by renewable energy. For example, solar and wind energy can be used to expand the Kenyan Nairobi railway network. Furthermore, investors and other stakeholders should consider investing in solar microgrids, given the immense potential for solar energy in regions like Sub-Saharan Africa. The same initiative has been tested in Rwanda with community-based solar projects. The Global Wind Energy Council [133] identified South Africa, Egypt, and Morocco as emerging offshore wind energy markets in 2024. The listed countries are expected to enhance their wind farm capacity to develop their energy portfolios. Finally, the relevant stakeholders should establish clear policies to reduce carbon emissions in the transportation and industrial sectors. This might involve setting emission targets that conform to international accords like the Paris Agreement.
The limited time dimension of the study narrows its scope to recent events beyond the stated study period and might affect some research findings. Global events like economic crises, pandemics, and significant government changes beyond 2020 may influence the variables under study. The inclusion of recent years in the study would help capture current changes in economic indicators in the aftermath of pandemic recovery processes and new economic landscapes. In addition, increasing the number of countries would strengthen the research, considering regional differences and the socio-economic contexts of the various countries. The study recommends further research into the nonlinear relationship between the main study variables. In addition, future studies can employ modern statistical tools such as machine learning technology to analyze large-scale models. Future studies may delve deeper into these areas for more comprehensive research.
The author declares she has not used Artificial Intelligence (AI) tools in the creation of this article.
The author declares no conflict of interest.
[1] |
Ciarreta A, Muniain P, Zarraga A (2017) Modeling and forecasting realized volatility in German–Austrian continuous intraday electricity prices. J Forecasting 36: 680–690. https://doi.org/10.1002/for.2463 doi: 10.1002/for.2463
![]() |
[2] | Linnet U (2005) Tools supporting wind energy trade in deregulated markets. Master's thesis. Technical University of Denmark, Department of Informatics and Mathematical Modelling. Available from: http://www2.compute.dtu.dk/pubdb/pubs/3969-full.html. |
[3] |
Green R (2006) Electricity liberalisation in Europe—How competitive will it be? Energy Policy 34: 2532–2541. https://doi.org/10.1016/j.enpol.2004.08.016 doi: 10.1016/j.enpol.2004.08.016
![]() |
[4] |
Karabiber OA, Xydis G (2019) Electricity price forecasting in the Danish day-ahead market using the TBATS, ANN and ARIMA methods. Energies 12: 928. https://doi.org/10.3390/en12050928 doi: 10.3390/en12050928
![]() |
[5] |
Pape C, Hagemann S, Weber C (2016) Are fundamentals enough? Explaining price variations in the German day-ahead and intraday power market. Energy Econ 54: 376–387. https://doi.org/10.1016/j.eneco.2015.12.013 doi: 10.1016/j.eneco.2015.12.013
![]() |
[6] |
Bokde N, Tranberg B, Andresen GB (2020) A graphical approach to carbon-efficient spot market scheduling for Power-to-X applications. Energy Convers Manage 224: 113461. https://doi.org/10.1016/j.enconman.2020.113461 doi: 10.1016/j.enconman.2020.113461
![]() |
[7] | Klein N, Smith MS, Nott DJ (2020) Deep distributional time series models and the probabilistic forecasting of intraday electricity prices. J Appl Econom. https://doi.org/10.1002/jae.2959 |
[8] |
Gürtler M, Paulsen T (2018) The effect of wind and solar power forecasts on day-ahead and intraday electricity prices in Germany. Energy Econ 75: 150–162. https://doi.org/10.1016/j.eneco.2018.07.006 doi: 10.1016/j.eneco.2018.07.006
![]() |
[9] |
Bokde ND, Yaseen ZM, Andersen GB (2020) ForecastTB—An R package as a Test-Bench for time series forecasting—Application of wind speed and solar radiation modeling. Energies 13: 2578. https://doi.org/10.3390/en13102578 doi: 10.3390/en13102578
![]() |
[10] | Bokde N, Troncoso A, Asencio-Corté G, et al. (2017) Pattern sequence similarity based techniques for wind speed forecasting. In: Proceedings of the International Work-Conference on Time Series, Granada, Spain, 18–20. |
[11] | Usaola J, Moreno MA (2009) Optimal bidding of wind energy in intraday markets. In: 6th International Conference on the European Energy Market, IEEE, 1–7. |
[12] |
Bokde ND, Tranberg B, Andresen GB (2021) Short-term CO2 emissions forecasting based on decomposition approaches and its impact on electricity market scheduling. Appl Energy 281: 6061. https://doi.org/10.1016/j.apenergy.2020.116061 doi: 10.1016/j.apenergy.2020.116061
![]() |
[13] | Jong C, Kovaleva S (2021) PPA Insights: Short-term forecasting and imbalance costs. In: KYOS, kyos.com. |
[14] |
Chaves-Ávila JP, Hakvoort RA, Ramos A (2014) The impact of European balancing rules on wind power economics and on short-term bidding strategies. Energy Policy 68: 383–393. https://doi.org/10.1016/j.enpol.2014.01.010 doi: 10.1016/j.enpol.2014.01.010
![]() |
[15] |
Van Der Veen RA, Abbasy A, Hakvoort RA (2012) Agent-based analysis of the impact of the imbalance pricing mechanism on market behavior in electricity balancing markets. Energy Econ 34: 874–881. https://doi.org/10.1016/j.eneco.2012.04.001 doi: 10.1016/j.eneco.2012.04.001
![]() |
[16] |
Kath C, Nitka W, Serafin T (2020) Balancing generation from renewable energy sources: Profitability of an energy trader. Energies 13: 205. https://doi.org/10.3390/en13010205 doi: 10.3390/en13010205
![]() |
[17] | Bokde ND, Pedersen T, Andresen GB (2021) Optimal scheduling of flexible power-to-x technologies in the day-ahead electricity market. arXiv preprint. https://doi.org/10.48550/arXiv.2110.09800 |
[18] | Borggrefe F, Neuhoff K (2011) Balancing and intraday market design: Options for wind integration. |
[19] | Hagemann S, Weber C (2013) An empirical analysis of liquidity and its determinants in the German intraday market for electricity. EWL Working Paper No. 17/2013. http://dx.doi.org/10.2139/ssrn.2349565 |
[20] |
Goodarzi S, Perera HN, Bunn D (2019) The impact of renewable energy forecast errors on imbalance volumes and electricity spot prices. Energy Policy 134: 110827. https://doi.org/10.1016/j.enpol.2019.06.035 doi: 10.1016/j.enpol.2019.06.035
![]() |
[21] |
Kiesel R, Paraschiv F (2017) Econometric analysis of 15-minute intraday electricity prices. Energy Econ 64: 77–90. https://doi.org/10.1016/j.eneco.2017.03.002 doi: 10.1016/j.eneco.2017.03.002
![]() |
[22] |
Browell J, Gilbert C (2022) Predicting electricity imbalance prices and volumes: Capabilities and opportunities. Energies 15: 3645. https://doi.org/10.3390/en15103645 doi: 10.3390/en15103645
![]() |
[23] |
Narajewski M (2022) Probabilistic forecasting of German electricity imbalance prices. Energies 15: 4976. https://doi.org/10.3390/en15144976 doi: 10.3390/en15144976
![]() |
[24] |
Bokde N, Feijóo A, N Al-Ansari, et al. (2020) The hybridization of ensemble empirical mode decomposition with forecasting models: Application of short-term wind speed and power modeling. Energies 13: 1666. https://doi.org/10.3390/en13071666 doi: 10.3390/en13071666
![]() |
[25] | Bourry F, Kariniotakis G (2009) Strategies for wind power trading in sequential short-term electricity markets. In: European wind energy conference (EWEC). |
[26] | Hagemann S (2015) Price determinants in the German intraday market for electricity: An empirical analysis. J Energy Mark, EWL Working Paper No. 18/2013. https://dx.doi.org/10.2139/ssrn.2352854 |
[27] | Michel N (2018) Analysis and forecast of intraday prices based on econometric models and machine-learning algorithms. Ph.D. thesis, University of Duisburg-Essen. |
[28] |
Weron R (2014) Electricity price forecasting: A review of the state-of-the-art with a look into the future. Int J Forecasting 30: 1030–1081. https://doi.org/10.1016/j.ijforecast.2014.08.008 doi: 10.1016/j.ijforecast.2014.08.008
![]() |
[29] | Berger J, Yalcinoz T, Rudion K (2020) Investigating the intraday continuous electricity market using auto regression integrated moving average model with exogenous inputs. In: 2020 IEEE International Conference on Environment and Electrical Engineering and 2020 IEEE Industrial and Commercial Power Systems Europe (EEEIC/I & CPS Europe), 1–6. |
[30] | Kulakov S, Ziel F (2021) The impact of renewable energy forecasts on intraday electricity prices. Econ Energy Environ Policy 10: 1–2. Available from: https://ideas.repec.org/a/aen/eeepjl/eeep10-1-kulakov.html. |
[31] | Maciejowska K, Nitka W, Weron T (2019) Day-ahead vs. intraday—Forecasting the price spread to maximize economic benefits. Energies 12: 631. https://doi.org/10.3390/en12040631 |
[32] | Kremer M, Kiesel R, Paraschiv F (2021) An econometric model for intraday electricity trading. Philos Trans Royal Soc A 379: 20190624. https://dx.doi.org/10.2139/ssrn.3489214 |
[33] | Glas S, Kiesel R, Kolkmann S, et al. (2019) Intraday renewable electricity trading: Advanced modeling and optimal control. In: Progress in industrial mathematics at ECMI 2018, Springer, 469–475. |
[34] |
Hu X, Jarait˙e J, Kažukauskas A (2021) The effects of wind power on electricity markets: A case study of the Swedish intraday market. Energy Econ 96: 105159. https://doi.org/10.1016/j.eneco.2021.105159 doi: 10.1016/j.eneco.2021.105159
![]() |
[35] |
Wozabal D, Rameseder G (2020) Optimal bidding of a virtual power plant on the Spanish day-ahead and intraday market for electricity. Eur J Oper Res 280: 639–655. https://doi.org/10.1016/j.ejor.2019.07.022 doi: 10.1016/j.ejor.2019.07.022
![]() |
[36] |
Féron O, Tankov P, Tinsi L (2020) Price formation and optimal trading in intraday electricity markets with a major player. Risks 8: 133. https://doi.org/10.3390/risks8040133 doi: 10.3390/risks8040133
![]() |
[37] |
Cramer E, Witthaut D, Mitsos A, et al. (2022) Multivariate probabilistic forecasting of intraday electricity prices using normalizing flows. Appl Energy 346: 121370. https://doi.org/10.1016/j.apenergy.2023.121370 doi: 10.1016/j.apenergy.2023.121370
![]() |
[38] | Kath C, Ziel F (2020) Optimal order execution in intraday markets: Minimizing costs in trade trajectories. arXiv preprint. https://doi.org/10.48550/arXiv.2009.07892 |
[39] |
Narajewski M, Ziel F (2019) Estimation and simulation of the transaction arrival process in intraday electricity markets. Energies 12: 4518. https://doi.org/10.3390/en12234518 doi: 10.3390/en12234518
![]() |
[40] | Coskun S, Korn R (2021) Modeling the intraday electricity demand in Germany. In: Mathematical Modeling, Simulation and Optimization for Power Engineering and Management, Springer. 34: 3–23. https://doi.org/10.1007/978-3-030-62732-4_1 |
[41] | Martin H, Otterson S (2018) German intraday electricity market analysis and modeling based on the limit order book. In: 2018 15th International Conference on the European Energy Market (EEM), 1–6. https://doi.org/10.1109/EEM.2018.8469829 |
[42] |
Kramer A, Kiesel R (2021) Exogenous factors for order arrivals on the intraday electricity market. Energy Econ 97: 105186. https://doi.org/10.1016/j.eneco.2021.105186 doi: 10.1016/j.eneco.2021.105186
![]() |
[43] | Martin H (2017) A limit order book model for the German intraday electricity market. Thesis for Master of Science, Electrical and Computer Engineering, Technical University Munich, Germany. |
[44] | Favetto B (2019) The European intraday electricity market: A modeling based on the hawkes process. hal-02089289. Available from: https://hal.science/hal-02089289/file/Hawkes_process_and_electricity_market.pdf. |
[45] |
Fatih Karanfil YL (2017) The role of continuous intraday electricity markets: The integration of large-share wind power generation in Denmark. Energy J 38: 107–130. http://dx.doi.org/10.5547/01956574.38.2.fkar doi: 10.5547/01956574.38.2.fkar
![]() |
[46] | Kolberg JK, Waage K (2018) Artificial intelligence and nord pool's intraday electricity market elbas: A demonstration and pragmatic evaluation of employing deep learning for price prediction: using extensive market data and spatio-temporal weather forecasts. Master's thesis. |
[47] |
Oksuz T, Ugurlu U (2019) Neural network based model comparison for intraday electricity price forecasting. Energies 12: 4557. https://doi.org/10.3390/en12234557 doi: 10.3390/en12234557
![]() |
[48] |
Andrade JR, Filipe J, Reis M, et al. (2017) Probabilistic price forecasting for day-ahead and intraday markets: Beyond the statistical model. Sustainability-Basel 9: 1990. https://doi.org/10.3390/su9111990 doi: 10.3390/su9111990
![]() |
[49] |
Uniejewski B, Marcjasz G, Weron R (2019) Understanding intraday electricity markets: Variable selection and very short-term price forecasting using LASSO. J Forecasting 35: 1533–1547. https://doi.org/10.1016/j.ijforecast.2019.02.001 doi: 10.1016/j.ijforecast.2019.02.001
![]() |
[50] |
Maciejowska K, Uniejewski B, Serafin T (2020) PCA forecast averaging—Predicting day-ahead and intraday electricity prices. Energies 13: 3530. https://doi.org/10.3390/en13143530 doi: 10.3390/en13143530
![]() |
[51] |
Yorulmus H, Ugurlu U, Oktay T (2018) A long short term memory application on the Turkish intraday electricity price forecasting. PressAcademia Proc 7: 126–130. https://doi.org/10.17261/Pressacademia.2018.867 doi: 10.17261/Pressacademia.2018.867
![]() |
[52] |
Kath C (2019) Modeling intraday markets under the new advances of the cross-border intraday project (XBID): Evidence from the German intraday market. Energies 12: 4339. https://doi.org/10.3390/en12224339 doi: 10.3390/en12224339
![]() |
[53] | Scholz C, Lehna M, Brauns K, et al. (2020) Towards the prediction of electricity prices at the intraday market using shallow and deep-learning methods. In: Workshop on Mining Data for Financial Applications, Springer, 101–118. |
[54] |
Kath C, Ziel F (2018) The value of forecasts: Quantifying the economic gains of accurate quarter-hourly electricity price forecasts. Energy Econ 76: 411–423. https://doi.org/10.1016/j.eneco.2018.10.005 doi: 10.1016/j.eneco.2018.10.005
![]() |
[55] |
Narajewski M, Ziel F (2020) Econometric modelling and forecasting of intraday electricity prices. J Commod Mark 19: 100107. https://doi.org/10.1016/j.jcomm.2019.100107 doi: 10.1016/j.jcomm.2019.100107
![]() |
[56] | Mohammadi S, Hesamzadeh MR (2020) Econometric modeling of intraday electricity market price with inadequate historical data. In: IEEE Workshop on Complexity in Engineering (COMPENG), 1–9. https://doi.org/10.1109/COMPENG50184.2022.9905434 |
[57] | Serafin T, Marcjasz G, Weron R (2020) Trading on short-term path forecasts of intraday electricity prices. Department of Operations Research and Business Intelligence, Wroclaw University of Science and Technology. |
[58] | Lehna M, Hoppmann B, Heinrich R, et al. (2021) A reinforcement learning approach for the continuous electricity market of Germany: Trading from the perspective of a wind park operator. arXiv preprint. https://doi.org/10.48550/arXiv.2111.13609 |
[59] | Demirtaş H (2020) Power imbalance prediction in Turkish energy market. Ph.D. thesis. |
[60] | Pozzetti L, Cartlidge J (2020) Trading electricity markets using neural networks. In: 32nd European Modelling and Simulation Symposium, 311–318. |
[61] | Ziel F (2017) Modeling the impact of wind and solar power forecasting errors on intraday electricity prices. In: 14th International Conference on the European Energy Market (EEM), 1–5. https://doi.org/10.1109/EEM.2017.7981900 |
[62] |
Narajewski M, Ziel F (2020) Ensemble forecasting for intraday electricity prices: Simulating trajectories. Appl Energy 279: 115801. https://doi.org/10.1016/j.apenergy.2020.115801 doi: 10.1016/j.apenergy.2020.115801
![]() |
[63] |
Marcjasz G, Uniejewski B, Weron R (2020) Beating the naïve—Combining LASSO with naïve intraday electricity price forecasts. Energies 13: 1667. https://doi.org/10.3390/en13071667 doi: 10.3390/en13071667
![]() |
[64] | Hamilton G, Abeygunawardana A, Jovanović DP, et al. (2018) Hybrid model for very short-term electricity price forecasting. In: 2018 IEEE Power & Energy Society General Meeting (PESGM), 1–5. |
[65] |
Manickavasagam J, Visalakshmi S, Apergis N (2020) A novel hybrid approach to forecast crude oil futures using intraday data. Technol Forecast Soc 158: 120126. https://doi.org/10.1016/j.techfore.2020.120126 doi: 10.1016/j.techfore.2020.120126
![]() |
[66] |
Kiyak C, de Vries A (2018) Electricity markets mechanism regarding the operational flexibility of power plants. Modern Econ 8: 567–589. https://doi.org/10.4236/me.2017.84043 doi: 10.4236/me.2017.84043
![]() |
[67] |
Cludius J, Hermann H, Matthes FC, et al. (2014) The merit order effect of wind and photovoltaic electricity generation in Germany 2008–2016: Estimation and distributional implications. Energy Econ 44: 302–313. https://doi.org/10.1016/j.eneco.2014.04.020 doi: 10.1016/j.eneco.2014.04.020
![]() |
[68] |
Janke T, Steinke F (2019) Forecasting the price distribution of continuous intraday electricity trading. Energies 12: 4262. https://doi.org/10.3390/en12224262 doi: 10.3390/en12224262
![]() |
[69] |
Sobri S, Koohi-Kamali S, Rahim NA (2018) Solar photovoltaic generation forecasting methods: A review. Energy Convers Manage 156: 459–497. https://doi.org/10.1016/j.enconman.2017.11.019 doi: 10.1016/j.enconman.2017.11.019
![]() |
Variables | Variable name | Indicator | Unit of measurement | Sources |
Dependent variable | CO2 per capita | lnCO2pc | Metric tons per capita | WDI [66] |
Independent variable | Oil products (nonrenewable energy) | lnNREpc | Terajoules per capita | IEA [67] WDI [66] |
Mediating variable | Transport energy consumption | lnETRpc | Terajoules per capita | IEA [67] WDI [66] |
Moderating variable | Clean energy access (residential) | lnCLE | % of the total population | WDI [66] |
Control variables | GDP per capita | lnGDPpc | Constant 2015 US$ | WDI [66] |
Industrialization | lnIND | % of GDP | WDI [66] | |
Renewable energy consumption | lnREC | % of final energy consumption | WDI [66] | |
Agricultural land | lnAGL | % of land area | WDI [66] | |
Trade | TR | % of GDP | WDI [66] | |
Urbanization | lnURB | % of the total population | WDI [66] |
Variables | Full sample mean (st. dev) | RIC mean (st. dev) | NRIC Mean (st. dev) |
CO2pc | 1.146 (1.719) |
1.499 (2.066) |
0.635 (0.791) |
NREpc | 0.005 (0.004) |
0.006 (0.006) |
0.003 (0.003) |
ETRpc | 0.003 (0.002) |
0.004 (0.004) |
0.002 (0.002) |
CLE | 31.993 (34.913) |
33.636 (32.756) |
29.619 (37.780) |
GDPpc | 2160.890 (1864.198) |
2650.406 (2124.403) |
1453.812 (1068.710) |
IND | 29.071 (12.513) |
34.886 (12.905) |
20.673 (4.798) |
REC | 63.516 (30.276) |
63.198 (29.860) |
63.974 (30.946) |
AGL | 45.254 (22.066) |
36.408 (22.350) |
58.031 (13.911) |
TR | 63.367 (26.170) |
66.541 (28.597) |
58.783 (21.460) |
URB | 44.359 (18.688) |
49.865 (17.341) |
36.407 (17.714) |
*Note: RIC represents resource-intensive countries; NRIC indicates non-resource-intensive countries. St. dev represents the standard deviation (in parentheses). |
Model | Breusch-Pagan Lagrange multiplier test |
Hausman test | Conclusion |
Baseline model | 2268.97*** [0.0000] |
18.70 [0.8112] |
Random effect |
Mediating effect model | 2163.78*** [0.0000] |
20.04 [0.7895] |
Random effect |
Moderating effect model | 2298.92*** [0.0000] |
43.81** [0.0290] |
Fixed effect |
*Note: p<0.05: reject null hypothesis; P≥0.05: fail to reject the null hypothesis. |
Variables | Model (1) | Model (2) | Model (3) |
lnCO2pc | lnETRpc | lnCO2pc | |
lnNREpc | 0.340*** | 0.929*** | 0.184** |
(0.041) | (0.033) | (0.076) | |
lnETRpc | 0.181*** | ||
(0.067) | |||
lnGDPpc | 0.557*** | 0.119* | 0.418*** |
(0.073) | (0.061) | (0.080) | |
lnIND | 0.119** | 0.103** | 0.173*** |
(0.054) | (0.045) | (0.060) | |
lnREC | -0.303*** | -0.192*** | -0.150 |
(0.067) | (0.068) | (0.091) | |
lnAGL | 0.071 | 0.705*** | -0.459* |
(0.083) | (0.187) | (0.251) | |
TR | 0.004*** | -0.001* | 0.004*** |
(0.001) | (0.000) | (0.001) | |
lnURB | 0.473*** | 0.339** | 0.475*** |
(0.134) | (0.134) | (0.179) | |
Constant | -4.139*** | -4.854*** | -1.866 |
(0.781) | (0.876) | (1.199) | |
Year dummies | YES | YES | YES |
Country dummies | YES | YES | YES |
Observations | 440 | 440 | 440 |
R-squared | 0.648 | 0.879 | 0.659 |
*Note: Standard errors are in parentheses. *** P < 0.01, ** P < 0.05, * P < 0.10. |
Variables | Model (4) | Resource | Non-resource |
Moderator: lnCLE | intensive countries | intensive countries | |
lnNREpc | 0.380*** | 0.362*** | 0.310*** |
(0.043) | (0.058) | (0.065) | |
lnCLE | -0.129*** | -0.105*** | 0.044 |
(0.021) | (0.034) | (0.027) | |
lnNREpc×lnCLE | -0.078*** | -0.111*** | 0.015 |
(0.012) | (0.015) | (0.018) | |
lnGDPpc | 0.448*** | 0.560*** | 0.107 |
(0.083) | (0.141) | (0.099) | |
lnIND | 0.195*** | 0.160* | 0.142** |
(0.057) | (0.085) | (0.061) | |
lnREC | -0.224** | -0.488*** | -0.020 |
(0.093) | (0.143) | (0.105) | |
lnAGL | -1.027*** | -1.667*** | 0.473 |
(0.253) | (0.338) | (0.356) | |
TR | 0.002*** | 0.002*** | 0.007*** |
(0.001) | (0.001) | (0.001) | |
lnURB | 0.439*** | -0.453 | 1.786*** |
(0.168) | (0.275) | (0.215) | |
Constant | -1.717 | 3.878** | -10.524*** |
(1.191) | (1.723) | (1.550) | |
Year dummies | YES | YES | YES |
Country dummies | YES | YES | YES |
Observations | 440 | 260 | 180 |
R-squared | 0.695 | 0.614 | 0.897 |
*Note: Standard errors are in parentheses. *** P < 0.01, ** P < 0.05, * P < 0.10. |
Variable | Resource intensive countries | Non-resource intensive countries | ||||
lnCO2pc (1) |
lnETRpc (2) |
lnCO2pc (3) |
lnCO2pc (4) |
lnETRpc (5) |
lnCO2pc (6) |
|
lnNREpc | 0.351*** | 0.873*** | 0.329*** | 0.297*** | 0.174*** | 0.075*** |
(0.056) | (0.044) | (0.092) | (0.057) | (0.028) | (0.020) | |
lnETRpc | 0.027* | 0.389*** | ||||
(0.084) | (0.051) | |||||
lnGDPpc | 0.785*** | 0.258** | 0.759*** | 0.254*** | 0.389*** | 0.591*** |
(0.130) | (0.104) | (0.134) | (0.075) | (0.050) | (0.037) | |
lnIND | 0.059 | 0.199*** | 0.060 | 0.188*** | 0.746*** | 0.148** |
(0.085) | (0.067) | (0.087) | (0.062) | (0.093) | (0.070) | |
lnREC | -0.375*** | -0.065 | -0.380*** | -0.054 | -0.360*** | -0.304*** |
(0.109) | (0.090) | (0.114) | (0.075) | (0.046) | (0.034) | |
lnAGL | 0.020 | 0.336*** | -0.004 | 0.121 | -0.253*** | -0.437*** |
(0.117) | (0.103) | (0.131) | (0.221) | (0.070) | (0.046) | |
TR | 0.004*** | -0.001* | 0.004*** | 0.005*** | 0.004*** | 0.002** |
(0.001) | (0.001) | (0.001) | (0.001) | (0.001) | (0.001) | |
lnURB | -0.155 | -0.084 | -0.164 | 1.258*** | 0.105 | 0.292*** |
(0.238) | (0.192) | (0.244) | (0.149) | (0.080) | (0.051) | |
Constant | -2.734** | -4.150*** | -2.374* | -6.516*** | -8.591*** | -0.832 |
(1.144) | (0.932) | (1.227) | (1.105) | (0.487) | (0.536) | |
Year dummies | YES | YES | YES | YES | YES | YES |
Country dummies | YES | YES | YES | YES | YES | YES |
Observations | 260 | 260 | 260 | 180 | 180 | 180 |
R-squared | 0.505 | 0.866 | 0.507 | 0.890 | 0.718 | 0.836 |
*Note: Standard errors are in parentheses. *** P < 0.01, ** P < 0.05, * P < 0.10. |
Test | Statistic | Conclusion |
Modified Wald test for group-wise heteroskedasticity chi2 (22) | 2281.48*** [0.0000] |
Present |
Wooldridge test for autocorrelation F (1, 21) | 111.65*** [0.0000] |
Present |
Pesaran's test of cross-sectional dependence | -2.702*** [0.0069] |
Present |
*Note: *** P < 0.01, ** P < 0.05, * P < 0.10. |
(1) | (2) | (3) | |
Variables | lnCO2pc | lnETRpc | lnCO2pc |
lnNREpc | 0.174*** | 0.224*** | 0.103*** |
(0.026) | (0.030) | (0.027) | |
lnETRpc | 0.317*** | ||
(0.048) | |||
lnGDPpc | 1.079*** | 0.471*** | 0.930*** |
(0.026) | (0.040) | (0.033) | |
lnIND | -0.063 | 0.305*** | -0.159 |
(0.106) | (0.063) | (0.100) | |
lnAGL | 0.049* | 0.241*** | -0.028 |
(0.028) | (0.029) | (0.034) | |
lnREC | -0.339*** | -0.394*** | -0.214*** |
(0.016) | (0.026) | (0.018) | |
TR | 0.003 | -0.001 | 0.003* |
(0.002) | (0.001) | (0.001) | |
lnURB | 0.118*** | 0.080 | 0.093** |
(0.039) | (0.051) | (0.039) | |
Constant | -6.716*** | -8.995*** | -3.867*** |
(0.216) | (0.358) | (0.604) | |
Year dummies | YES | YES | YES |
Country dummies | YES | YES | YES |
Observations | 440 | 440 | 440 |
R-squared | 0.960 | 0.923 | 0.964 |
*Note: Standard errors are in parentheses. *** P < 0.01, ** P < 0.05, * P < 0.10. |
Variables | First stage | Second stage |
lnNREpc | lnCO2pc | |
L.lnNREpc | 0.974*** | |
(0.009) | ||
lnNREpc | 0.166*** | |
(0.026) | ||
lnGDPpc | 0.012 | 1.078*** |
(0.016) | (0.043) | |
lnIND | 0.004 | -0.102 |
(0.025) | (0.066) | |
lnAGL | 0.008 | 0.034 |
(0.009) | (0.024) | |
lnREC | -0.021** | -0.342*** |
(0.009) | (0.024) | |
TR | 0.001*** | 0.003*** |
(0.000) | (0.001) | |
lnURB | -0.029 | 0.125** |
(0.023) | (0.061) | |
Constant | -0.133 | -6.783*** |
(0.136) | (0.368) | |
Observations | 418 | 418 |
R-squared | 0.993 | 0.957 |
Endogeneity test | Statistics | P-value |
Durbin (score) | 0.0704 | 0.7908 |
Wu-Hausman | 0.0689 | 0.7931 |
*Note: Standard errors are in parentheses. *** P < 0.01, ** P < 0.05, * P < 0.10. |
Variables | Variable name | Indicator | Unit of measurement | Sources |
Dependent variable | CO2 per capita | lnCO2pc | Metric tons per capita | WDI [66] |
Independent variable | Oil products (nonrenewable energy) | lnNREpc | Terajoules per capita | IEA [67] WDI [66] |
Mediating variable | Transport energy consumption | lnETRpc | Terajoules per capita | IEA [67] WDI [66] |
Moderating variable | Clean energy access (residential) | lnCLE | % of the total population | WDI [66] |
Control variables | GDP per capita | lnGDPpc | Constant 2015 US$ | WDI [66] |
Industrialization | lnIND | % of GDP | WDI [66] | |
Renewable energy consumption | lnREC | % of final energy consumption | WDI [66] | |
Agricultural land | lnAGL | % of land area | WDI [66] | |
Trade | TR | % of GDP | WDI [66] | |
Urbanization | lnURB | % of the total population | WDI [66] |
Variables | Full sample mean (st. dev) | RIC mean (st. dev) | NRIC Mean (st. dev) |
CO2pc | 1.146 (1.719) |
1.499 (2.066) |
0.635 (0.791) |
NREpc | 0.005 (0.004) |
0.006 (0.006) |
0.003 (0.003) |
ETRpc | 0.003 (0.002) |
0.004 (0.004) |
0.002 (0.002) |
CLE | 31.993 (34.913) |
33.636 (32.756) |
29.619 (37.780) |
GDPpc | 2160.890 (1864.198) |
2650.406 (2124.403) |
1453.812 (1068.710) |
IND | 29.071 (12.513) |
34.886 (12.905) |
20.673 (4.798) |
REC | 63.516 (30.276) |
63.198 (29.860) |
63.974 (30.946) |
AGL | 45.254 (22.066) |
36.408 (22.350) |
58.031 (13.911) |
TR | 63.367 (26.170) |
66.541 (28.597) |
58.783 (21.460) |
URB | 44.359 (18.688) |
49.865 (17.341) |
36.407 (17.714) |
*Note: RIC represents resource-intensive countries; NRIC indicates non-resource-intensive countries. St. dev represents the standard deviation (in parentheses). |
Model | Breusch-Pagan Lagrange multiplier test |
Hausman test | Conclusion |
Baseline model | 2268.97*** [0.0000] |
18.70 [0.8112] |
Random effect |
Mediating effect model | 2163.78*** [0.0000] |
20.04 [0.7895] |
Random effect |
Moderating effect model | 2298.92*** [0.0000] |
43.81** [0.0290] |
Fixed effect |
*Note: p<0.05: reject null hypothesis; P≥0.05: fail to reject the null hypothesis. |
Variables | Model (1) | Model (2) | Model (3) |
lnCO2pc | lnETRpc | lnCO2pc | |
lnNREpc | 0.340*** | 0.929*** | 0.184** |
(0.041) | (0.033) | (0.076) | |
lnETRpc | 0.181*** | ||
(0.067) | |||
lnGDPpc | 0.557*** | 0.119* | 0.418*** |
(0.073) | (0.061) | (0.080) | |
lnIND | 0.119** | 0.103** | 0.173*** |
(0.054) | (0.045) | (0.060) | |
lnREC | -0.303*** | -0.192*** | -0.150 |
(0.067) | (0.068) | (0.091) | |
lnAGL | 0.071 | 0.705*** | -0.459* |
(0.083) | (0.187) | (0.251) | |
TR | 0.004*** | -0.001* | 0.004*** |
(0.001) | (0.000) | (0.001) | |
lnURB | 0.473*** | 0.339** | 0.475*** |
(0.134) | (0.134) | (0.179) | |
Constant | -4.139*** | -4.854*** | -1.866 |
(0.781) | (0.876) | (1.199) | |
Year dummies | YES | YES | YES |
Country dummies | YES | YES | YES |
Observations | 440 | 440 | 440 |
R-squared | 0.648 | 0.879 | 0.659 |
*Note: Standard errors are in parentheses. *** P < 0.01, ** P < 0.05, * P < 0.10. |
Variables | Model (4) | Resource | Non-resource |
Moderator: lnCLE | intensive countries | intensive countries | |
lnNREpc | 0.380*** | 0.362*** | 0.310*** |
(0.043) | (0.058) | (0.065) | |
lnCLE | -0.129*** | -0.105*** | 0.044 |
(0.021) | (0.034) | (0.027) | |
lnNREpc×lnCLE | -0.078*** | -0.111*** | 0.015 |
(0.012) | (0.015) | (0.018) | |
lnGDPpc | 0.448*** | 0.560*** | 0.107 |
(0.083) | (0.141) | (0.099) | |
lnIND | 0.195*** | 0.160* | 0.142** |
(0.057) | (0.085) | (0.061) | |
lnREC | -0.224** | -0.488*** | -0.020 |
(0.093) | (0.143) | (0.105) | |
lnAGL | -1.027*** | -1.667*** | 0.473 |
(0.253) | (0.338) | (0.356) | |
TR | 0.002*** | 0.002*** | 0.007*** |
(0.001) | (0.001) | (0.001) | |
lnURB | 0.439*** | -0.453 | 1.786*** |
(0.168) | (0.275) | (0.215) | |
Constant | -1.717 | 3.878** | -10.524*** |
(1.191) | (1.723) | (1.550) | |
Year dummies | YES | YES | YES |
Country dummies | YES | YES | YES |
Observations | 440 | 260 | 180 |
R-squared | 0.695 | 0.614 | 0.897 |
*Note: Standard errors are in parentheses. *** P < 0.01, ** P < 0.05, * P < 0.10. |
Variable | Resource intensive countries | Non-resource intensive countries | ||||
lnCO2pc (1) |
lnETRpc (2) |
lnCO2pc (3) |
lnCO2pc (4) |
lnETRpc (5) |
lnCO2pc (6) |
|
lnNREpc | 0.351*** | 0.873*** | 0.329*** | 0.297*** | 0.174*** | 0.075*** |
(0.056) | (0.044) | (0.092) | (0.057) | (0.028) | (0.020) | |
lnETRpc | 0.027* | 0.389*** | ||||
(0.084) | (0.051) | |||||
lnGDPpc | 0.785*** | 0.258** | 0.759*** | 0.254*** | 0.389*** | 0.591*** |
(0.130) | (0.104) | (0.134) | (0.075) | (0.050) | (0.037) | |
lnIND | 0.059 | 0.199*** | 0.060 | 0.188*** | 0.746*** | 0.148** |
(0.085) | (0.067) | (0.087) | (0.062) | (0.093) | (0.070) | |
lnREC | -0.375*** | -0.065 | -0.380*** | -0.054 | -0.360*** | -0.304*** |
(0.109) | (0.090) | (0.114) | (0.075) | (0.046) | (0.034) | |
lnAGL | 0.020 | 0.336*** | -0.004 | 0.121 | -0.253*** | -0.437*** |
(0.117) | (0.103) | (0.131) | (0.221) | (0.070) | (0.046) | |
TR | 0.004*** | -0.001* | 0.004*** | 0.005*** | 0.004*** | 0.002** |
(0.001) | (0.001) | (0.001) | (0.001) | (0.001) | (0.001) | |
lnURB | -0.155 | -0.084 | -0.164 | 1.258*** | 0.105 | 0.292*** |
(0.238) | (0.192) | (0.244) | (0.149) | (0.080) | (0.051) | |
Constant | -2.734** | -4.150*** | -2.374* | -6.516*** | -8.591*** | -0.832 |
(1.144) | (0.932) | (1.227) | (1.105) | (0.487) | (0.536) | |
Year dummies | YES | YES | YES | YES | YES | YES |
Country dummies | YES | YES | YES | YES | YES | YES |
Observations | 260 | 260 | 260 | 180 | 180 | 180 |
R-squared | 0.505 | 0.866 | 0.507 | 0.890 | 0.718 | 0.836 |
*Note: Standard errors are in parentheses. *** P < 0.01, ** P < 0.05, * P < 0.10. |
Test | Statistic | Conclusion |
Modified Wald test for group-wise heteroskedasticity chi2 (22) | 2281.48*** [0.0000] |
Present |
Wooldridge test for autocorrelation F (1, 21) | 111.65*** [0.0000] |
Present |
Pesaran's test of cross-sectional dependence | -2.702*** [0.0069] |
Present |
*Note: *** P < 0.01, ** P < 0.05, * P < 0.10. |
(1) | (2) | (3) | |
Variables | lnCO2pc | lnETRpc | lnCO2pc |
lnNREpc | 0.174*** | 0.224*** | 0.103*** |
(0.026) | (0.030) | (0.027) | |
lnETRpc | 0.317*** | ||
(0.048) | |||
lnGDPpc | 1.079*** | 0.471*** | 0.930*** |
(0.026) | (0.040) | (0.033) | |
lnIND | -0.063 | 0.305*** | -0.159 |
(0.106) | (0.063) | (0.100) | |
lnAGL | 0.049* | 0.241*** | -0.028 |
(0.028) | (0.029) | (0.034) | |
lnREC | -0.339*** | -0.394*** | -0.214*** |
(0.016) | (0.026) | (0.018) | |
TR | 0.003 | -0.001 | 0.003* |
(0.002) | (0.001) | (0.001) | |
lnURB | 0.118*** | 0.080 | 0.093** |
(0.039) | (0.051) | (0.039) | |
Constant | -6.716*** | -8.995*** | -3.867*** |
(0.216) | (0.358) | (0.604) | |
Year dummies | YES | YES | YES |
Country dummies | YES | YES | YES |
Observations | 440 | 440 | 440 |
R-squared | 0.960 | 0.923 | 0.964 |
*Note: Standard errors are in parentheses. *** P < 0.01, ** P < 0.05, * P < 0.10. |
Variables | First stage | Second stage |
lnNREpc | lnCO2pc | |
L.lnNREpc | 0.974*** | |
(0.009) | ||
lnNREpc | 0.166*** | |
(0.026) | ||
lnGDPpc | 0.012 | 1.078*** |
(0.016) | (0.043) | |
lnIND | 0.004 | -0.102 |
(0.025) | (0.066) | |
lnAGL | 0.008 | 0.034 |
(0.009) | (0.024) | |
lnREC | -0.021** | -0.342*** |
(0.009) | (0.024) | |
TR | 0.001*** | 0.003*** |
(0.000) | (0.001) | |
lnURB | -0.029 | 0.125** |
(0.023) | (0.061) | |
Constant | -0.133 | -6.783*** |
(0.136) | (0.368) | |
Observations | 418 | 418 |
R-squared | 0.993 | 0.957 |
Endogeneity test | Statistics | P-value |
Durbin (score) | 0.0704 | 0.7908 |
Wu-Hausman | 0.0689 | 0.7931 |
*Note: Standard errors are in parentheses. *** P < 0.01, ** P < 0.05, * P < 0.10. |