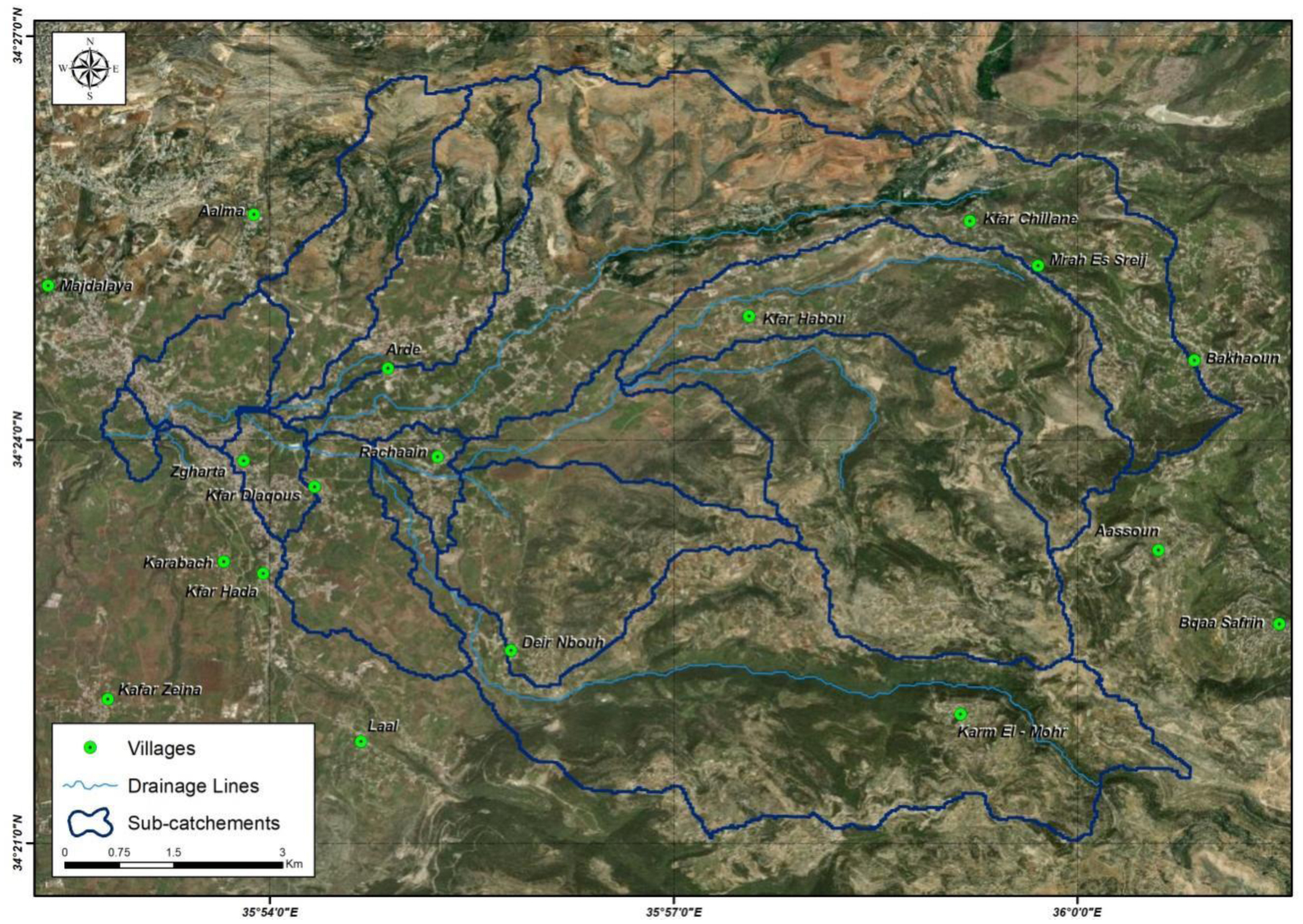
Global navigation satellite system reflectometry (GNSS-R) is based on satellite signals' multipath interference effect and has developed as one of the important remote sensing technologies in sea ice detection. An isometric mapping (ISOMAP)-based method is proposed in this paper as a development in sea ice detection approaches. The integral delay waveforms (IDWs), selected from February to April in 2018, derived from TechDemoSat-1 (TDS-1) Delay-Doppler maps (DDMs) are applied to open water and sea ice classification. In the first, the model for extracting low-dimensional coordinates of IDWs employs the randomly selected 187666 IDW samples, which are 30% of the whole IDW dataset. Then, low-dimensional coordinates of IDWs are used to train three different classifiers of support vector machine (SVM) and gradient boosting decision tree (GBDT), linear discriminant algorithm (LDA) and K-nearest neighbors (KNN) for determining the sea ice and sea water. The remaining 437889 samples, about 70% of the whole datasets, are used to validate with the ground surface type from the National Snow and Ice Data Center (NSIDC) data provided by the National Oceanic and Atmospheric Administration (NOAA). The algorithm performance is evaluated, and the overall accuracy of SVM, GBDT, LDA and KNN are 99.44%, 85.58%, 91.88% and 98.82%, respectively. The sea ice detection methods are developed, and the accuracy of detection is improved in this paper.
Citation: Yuan Hu, Zhihao Jiang, Xintai Yuan, Xifan Hua, Wei Liu. Isometric mapping algorithm based GNSS-R sea ice detection[J]. Metascience in Aerospace, 2024, 1(1): 38-52. doi: 10.3934/mina.2024002
[1] | Wei Wu, Shicheng Luo, Hongying Wang . Design of an automatic landscape design system in smart cities based on vision computing. Mathematical Biosciences and Engineering, 2023, 20(9): 16383-16400. doi: 10.3934/mbe.2023731 |
[2] | Yujing Qiao, Ning Lv, Baoming Jia . Multiview intelligent networking based on the genetic evolution algorithm for precise 3D measurements. Mathematical Biosciences and Engineering, 2023, 20(8): 14260-14280. doi: 10.3934/mbe.2023638 |
[3] | Colella Ylenia, De Lauri Chiara, Improta Giovanni, Rossano Lucia, Vecchione Donatella, Spinosa Tiziana, Giordano Vincenzo, Verdoliva Ciro, Santini Stefania . A Clinical Decision Support System based on fuzzy rules and classification algorithms for monitoring the physiological parameters of type-2 diabetic patients. Mathematical Biosciences and Engineering, 2021, 18(3): 2654-2674. doi: 10.3934/mbe.2021135 |
[4] | Zhan Cui, Jinjin Chu . Design and assessment of a vision computing-based multimedia interaction system for hybrid teaching. Mathematical Biosciences and Engineering, 2023, 20(8): 14718-14733. doi: 10.3934/mbe.2023658 |
[5] | Wenzheng Wei, Ying Jin . A novel Internet of Things-supported intelligent education management system implemented via collaboration of knowledge and data. Mathematical Biosciences and Engineering, 2023, 20(7): 13457-13473. doi: 10.3934/mbe.2023600 |
[6] | Mubashir Ahmad, Saira, Omar Alfandi, Asad Masood Khattak, Syed Furqan Qadri, Iftikhar Ahmed Saeed, Salabat Khan, Bashir Hayat, Arshad Ahmad . Facial expression recognition using lightweight deep learning modeling. Mathematical Biosciences and Engineering, 2023, 20(5): 8208-8225. doi: 10.3934/mbe.2023357 |
[7] | Hao Li, Xiaoyu Wen, Haoqi Wang, Guofu Luo, Steve Evans . A methodology for the modular structure planning of product-service systems. Mathematical Biosciences and Engineering, 2019, 16(3): 1489-1524. doi: 10.3934/mbe.2019072 |
[8] | Jin Zhang, Nan Ma, Zhixuan Wu, Cheng Wang, Yongqiang Yao . Intelligent control of self-driving vehicles based on adaptive sampling supervised actor-critic and human driving experience. Mathematical Biosciences and Engineering, 2024, 21(5): 6077-6096. doi: 10.3934/mbe.2024267 |
[9] | Boyi Zeng, Jun Zhao, Shantian Wen . A textual and visual features-jointly driven hybrid intelligent system for digital physical education teaching quality evaluation. Mathematical Biosciences and Engineering, 2023, 20(8): 13581-13601. doi: 10.3934/mbe.2023606 |
[10] | Yuanyao Lu, Kexin Li . Research on lip recognition algorithm based on MobileNet + attention-GRU. Mathematical Biosciences and Engineering, 2022, 19(12): 13526-13540. doi: 10.3934/mbe.2022631 |
Global navigation satellite system reflectometry (GNSS-R) is based on satellite signals' multipath interference effect and has developed as one of the important remote sensing technologies in sea ice detection. An isometric mapping (ISOMAP)-based method is proposed in this paper as a development in sea ice detection approaches. The integral delay waveforms (IDWs), selected from February to April in 2018, derived from TechDemoSat-1 (TDS-1) Delay-Doppler maps (DDMs) are applied to open water and sea ice classification. In the first, the model for extracting low-dimensional coordinates of IDWs employs the randomly selected 187666 IDW samples, which are 30% of the whole IDW dataset. Then, low-dimensional coordinates of IDWs are used to train three different classifiers of support vector machine (SVM) and gradient boosting decision tree (GBDT), linear discriminant algorithm (LDA) and K-nearest neighbors (KNN) for determining the sea ice and sea water. The remaining 437889 samples, about 70% of the whole datasets, are used to validate with the ground surface type from the National Snow and Ice Data Center (NSIDC) data provided by the National Oceanic and Atmospheric Administration (NOAA). The algorithm performance is evaluated, and the overall accuracy of SVM, GBDT, LDA and KNN are 99.44%, 85.58%, 91.88% and 98.82%, respectively. The sea ice detection methods are developed, and the accuracy of detection is improved in this paper.
Bacterial antimicrobial resistance (AMR) has emerged as a global public health concern, especially in clinical and veterinary settings. Recently, increasing attention has been focused on the pervasive distribution of AMR in anthropogenically impacted environments such as air, soil, wastewater, surface water from rivers and lakes, and other recreational waters [1]–[3]. Interestingly, aquatic environments are recognized as one of the natural reservoirs and ideal settings for the acquisition and spread of antibiotic-resistant bacteria (ARB) from urban, industrial, medical, and agricultural waste. Wastewater is a favorable environment for microbial proliferation and gene exchange between species thanks to the plethora of bacteria, high nutrient levels, and optimal temperature [4]. Furthermore, surface waters have been recognized as potential reservoirs for ARB. Studies have shown that an estimated 30–90% of antibiotics administered to humans and animals are excreted as active substances and discharged into the environment [5]. In the aquatic system, these antibiotics may exert selective pressure on bacteria, leading to microbial resistance and serious health consequences [3]. In addition, the potential of disseminating multidrug-resistant bacteria (MDR) into the population is quite significant, posing a serious public health risk resulting from improper sewage disposal and treatment. MDR is defined as acquired non-susceptibility to at least one agent in more than three antimicrobial classes [6]. Interestingly, human behaviors associated with agricultural pollution and urbanization exacerbate water environment pollution and deterioration, worsening river and lake water quality. An estimated 80% of industrial and municipal wastewater worldwide is released into the environment without any previous treatment, which harms ecosystems and poses risks to human and animal health. Because of the severe lack of sanitation and wastewater treatment systems, this percentage is greater in developing countries [7].
Enterobacteriaceae, a large family of Gram-negative and non-spore-forming rods, includes potential pathogens of several genera such as Escherichia, Enterobacter, Klebsiella, Proteus, Citrobacter, Serratia, Salmonella, and Shigella. These bacteria can be transmitted through fecal material and can be found in various environments such as soil, water, and food [8]. Escherichia coli (E. coli), part of the normal flora of the gastrointestinal tract, spreads in natural environments either directly through fecal waste or indirectly through poorly managed wastewater, making it a primary indicator of fecal bacterial contamination in water, food, and soil [4]. It usually contaminates water systems by various routes including human activities and animal, hospital, and municipal discharges. An increased prevalence of extended-spectrum β-lactamases (ESBLs) has been recently reported [9]. ESBLs are a cluster of enzymes that effectively break down third-generation oxyimino-cephalosporins such as cefotaxime, ceftazidime, and ceftriaxone, as well as monobactams like aztreonam, but not cephamycins (cefoxitin, cefotetan) and carbapenems (imipenem, ertapenem, biapenem, meropenem, and doripenem) [10]. Most ESBLs are encoded by antibiotic resistance genes (ARGs) frequently found on mobile genetic elements of a variety of Gram-negative bacteria. Biofilms of the aquatic system can serve as hotspots for disseminating these ARGs, contributing to increased pathogenicity and antimicrobial resistance [11]. More than 400 ESBL subtypes have been identified so far, most commonly derived from the TEM (Temoniera), SHV (Sulfhydryl Variable), OXA (Oxacillinase), and CTX-M (Cefotaximase-München) types [12]. Notably, the CTX-M type (mainly CTX-M-15) is among the most prevalent of ESBL genes described in communities, clinical settings, and water networks, followed by the TEM and SHV types [13]. ESBL-producing E. coli has been found in recreational waters, wastewater, and surface water from rivers and lakes over the past decade [14]. Furthermore, the discovery of ESBL-producing E. coli in companions, livestock, and wild animals has hypothesized its role in spreading ESBL-producing bacteria in the environment [15].
Lebanon, a developing country on the Mediterranean Sea, has struggled with post-civil war infrastructure, waste, and water management issues, in addition to a population growth since 2011 including an influx of 1.5 million refugees that exerted a significant strain on existing resources and services [16]. Surface water in Lebanon is intended for human consumption, recreational activities, electricity generation, and irrigation [17]. In 2016, only 58.54% of buildings were properly connected to a sewerage system; the remaining buildings either used septic tanks or cesspools or directly discharged untreated sewage into the aquatic system. It was also reported that 92% of the collected wastewater is discarded into aquatic environments without any prior treatment [18]. The majority of water sources are contaminated with industrial waste and sewage, posing a significant risk to public health and the economy. Many studies conducted in Lebanon showed a deterioration pattern in domestic water and seawater quality [19],[20]. In addition, recent reports have highlighted the contamination of spring and well waters intended for human consumption, as well as estuaries that harbor unique plant and animal communities [21]. ESBL-producing E. coli has been also isolated from river effluents in Lebanon, including refugee camps, highlighting the magnitude of the problem associated with the dissemination of drug-resistant Gram-negative bacteria [16]. Thus, the present study aimed to assess the water quality and occurrence of antibiotic-resistant bacteria in water samples collected from different surface water sites in the North Governorate of Lebanon and to estimate the occurrence and molecular characterization of ESBL-producing E. coli isolates.
A total of 100 water samples were collected from 25 different surface water sites (spaced approximately 200 m apart) in the North Governorate of Lebanon between July 2020 and April 2021. The sampling sites were chosen to achieve a possible compromise between the representativeness of the contaminated areas and operational feasibility. The selected sites are presented in Figure 1 and designated as follows: upstream area (S1 to S5), midstream area (S6 to S14), and downstream area (S15 to S25). Many sites (Al Merdechyeh, Rachaaine, Kfar Dlaqous, Zgharta, and Tripoli) were in the discontinuous urbanized upstream area; this area is influenced by agricultural and anthropogenic activities, in addition to high population size estimated at 331,500 [22]. A 50 mL sample was collected from each site in a sterile glass bottle, kept at 4 °C, and processed within six hours of sampling.
Physicochemical parameters including temperature and pH were measured. Turbidity in surface water indicates the presence of suspended solids and organic matter. It was measured by a Lovibond water testing turbidimeter. Dissolved oxygen (DO) is an important indicator of water quality by measuring oxygen concentration. A Thermo Orion was employed to evaluate the DO and biochemical oxygen demand (BOD) for 5 days.
Samples were processed to determine the concentrations of total coliforms (TC), fecal coliforms (FC), and enterococci (ENT), using a membrane filtration method for testing TC (protocol 9222B), FC (protocol 9222D17), and ENT (US EPA Method 1600) [23]. Membrane filters were then transferred to Petri dishes and placed on Endo agar, membrane fecal coliform agar, and Enterococcus selective agar (Sigma-Aldrich, USA) for TC, FC, and ENT bacteria respectively. They were incubated for 24 h at 37 °C for the total coliforms. Fecal coliforms were incubated at 44.5 °C for 24 h. Each isolated colony was examined for its morphological characteristics, and the viable bacterial count was enumerated in terms of colony-forming units (CFU) per 100 milliliters. The French SEQ-EAU-2003 and the US EPA standards [24] were used to evaluate irrigation and recreational water quality, respectively. According to SEQ-EAU-2003, the acceptable limit for thermo-tolerant fecal coliforms (fecal coliforms or E. coli) is 100 CFU/100 mL, which aligns with the standards set by other European Union countries [25]. Thus, we adopted these standards to assess the suitability of water sources for irrigation and recreation in Lebanon.
Enterobacteriaceae resistant to broad-spectrum cephalosporins were selected by directly plating 100 µL of water on ESBL ChromoSelect agar plates (Sigma-Aldrich, USA) and incubated at 37 °C for 24 h. Typical E. coli colonies were randomly picked from selective plates and identified using Api 20E (BioMerieux SA, Lyon, France).
Antibiotic susceptibility was tested using 27 different antibiotics belonging to 11 different antibiotic classes, including the penicillins amoxicillin (AMX, 25 µg), ticarcillin (TIC, 75 µg), and piperacillin (PIP, 100 µg); the beta-lactamase inhibitor combinations amoxicillin/clavulanic acid (AMC, 20 µg/10 µg), ticarcillin/clavulanic acid (TIM, 75 µg/10 µg ), and piperacillin–tazobactam (TZP, 100 µg/10 µg); the cephalosporins cefuroxime (CXM, 30 µg), cefoxitin (FOX, 30 µg), cefotaxime (CTX, 30 µg), ceftriaxone (CRO, 30 µg), ceftazidime (CAZ, 30 µg), cefepime (FEP, 30 µg), and cefixime (CFM, 5 µg); the carbapenems imipenem (IPM, 10 µg) and ertapenem (ETP, 10 µg); the monobactams aztreonam (ATM, 30 µg); the aminoglycosides streptomycin (SMN, 10 µg), tobramycin (TOB, 30 µg), gentamicin (GEN, 10 µg), and amikacin (AMK, 30 µg); the tetracycline tetracycline (TET, 30 µg); the fluoroquinolone ciprofloxacin (CIP, 5 µg); the sulfonamides sulfonamide (SSS, 300 µg) and trimethoprim/sulfamethoxazole (SXT, 25 µg); the phenicol chloramphenicol (CHL, 30 µg); the quinolones nalidixic acid (NAL, 30 µg); and the non-sulfonamide trimethoprim (TMP, 5 µg) (Bio-Rad, USA). ESBL production, when suspected, was confirmed by the double-disc synergy test, with third-generation cephalosporins (cefotaxime, ceftazidime, and cefixime). Results were interpreted according to the Clinical Laboratory Standards Institute guidelines [26]. Bacteria were classified as susceptible, intermediate, or resistant according to the approved clinical breakpoints. E. coli ATCC 25922 was used for quality control.
Antibiotic resistance profiles of ESBL-producing E. coli isolates were performed by the disc diffusion method according to the guidelines of the Antibiogram Committee of the French Society for Microbiology (https://www.sfm-microbiologie.org/).
Genomic DNA extraction was performed using a NucleoSpin Tissue kit (Macherey-Nagel, Hoerdt, France) for each isolate. The genomic DNA samples were stored at −20 °C for downstream molecular analysis.
Phylogenetic typing was performed to determine the main phylogenetic groups (A, B1, B2, C, D, E, and F) of ESBL-producing E. coli isolates by polymerase chain reaction (PCR) amplification of chuA, yjaA, tspE4C2, and arpA genes as described previously [27]. The presence of β-lactamase-encoding genes was tested in all ESBL-producing E. coli isolates by PCR amplification of blaTEM, blaSHV, blaCTX-M, blaCTX-M15, blaOXA, and blaOXA-48 genes (Table 1) [16],[28],[29]. The PCR mixture for each reaction contained 12.5 µL of 2 × REDTaq® ReadyMix™ (Sigma-Aldrich, USA), 1 µL of each primer (10 µM), 1 µL of template DNA, and double-distilled water (ddH2O) to a final volume of 25 µL. The amplification products were separated in 2% agarose gel containing ethidium bromide and then visualized under ultraviolet (UV) light.
PCR target | Primer sequence (5′-3′) | Annealing Temperature (°C) | Length (bp) | Reference | ||
Phylogenetic | ChuA | chuA.1b | ATGGTACCGGACGAACCAAC | 59 | 288 | [27] |
chuA.2 | TGCCGCCAGTACCAAAGACA | |||||
yjaA | yjaA.1b | CAAACGTGAAGTGTCAGGAG | 59 | 211 | ||
yjaA.2b | AATGCGTTCCTCAACCTGTG | |||||
TspE4.C | TspE4C2.1 | CACTATTCGTAAGGTCATCC | 59 | 152 | ||
TspE4C2.2b | AGTTTATCGCTGCGGGTCGC | |||||
arpA | AceK.f | AACGCTATTCGCCAGCTTGC | 59 | 400 | ||
ArpA1.r | TCTCCCCATACCGTACGCTA | |||||
β-lactam resistance | blaTEM | TemA1 | ATAAAATTCTTGAAGAC | 42 | 1075 | [16] |
TemB1 | TTACCAATGCTTAATCA | |||||
blaSHV | SHV-F | CACTCAAGGATGTATTGTG | 49 | 822 | ||
SHV-R | TTAGCGTTGCCAGTGCTCG | |||||
blaCTX-M | CTX-C1 | ATGTGCAGCACCAGTAAAGT | 54 | 545 | ||
CTX-C2 | ACCGCGATATCGTTGGTGG | |||||
blaOXA | OXA-F | ACACAATACATATCAACTTCGC | 61 | 813 | ||
OXA-R | AGTGTGTTTAGAATGGTGATC | |||||
blaCTX-M-15 | F | CACACGTGGAATTTAGGGACT | 50 | 995 | [28] | |
R | GCCGTCTAAGGCGATAAACA | |||||
Carbapenem resistance | blaOXA-48 | F | GCTTGATCGCCCTCGATT | 60 | 238 | [29] |
R | GATTTGCTCCGTGGCCGAAA |
Data entry and descriptive statistics were performed using IBM SPSS (Statistical Package for the Social Sciences) version 29. Student's t-test was performed for means comparison. A p-value ≤ 0.05 was considered significant.
Physicochemical parameters were measured in the water samples. The mean values of these parameters are presented in Table 2. Recorded temperatures ranged from 17.2 to 20.8 °C during the dry season and from 11.8 to 17.2 °C during the wet season. The recorded pH levels of all sites were within the permissible limits (6.5 to 8.5) set by the World Health Organization (WHO) for potable water [30]. The upstream water samples (S1 to S10) had the lowest turbidity, while the downstream samples (S15 to S25) exceeded the WHO guideline value of 5 NTU. Moreover, the highest average of DO levels (10.64) was observed during the dry sample period. However, the BOD5 content was lower during the dry season (2.76) than in the wet season (3.1).
The results of microbial analysis at the different sampling sites showed that total coliforms ranged from 1.9 × 101 ± 1.7 × 101 to 5.3 × 106 ± 7.8 × 106 CFU/100 mL. In contrast, fecal coliform levels ranged from 5.4 ± 0.58 to 1.6 × 106 ± 2.1 × 106 CFU/100 mL during both seasons. E. coli concentrations ranged from 5.0 ± 4.2 to 3.4 × 105 ± 4.6 × 105 CFU/100 mL, and the counts of fecal enterococci ranged from 0 to 5.1 × 104 ± 5.9 × 104 CFU/100 mL. A significant difference in bacterial load was recorded among the different sampling sites (p < 0.001).
Fecal coliforms were detected in 98/100 (98%) of water samples and 84/100 (84%) of sampling sites. In positive sites, the average number of fecal coliforms ranged from 1 × 100 to 4.7 × 106 CFU/100 mL. E. coli isolates were recovered from 90/100 (90%) of water samples. E. coli concentration ranged from 1 to 1 × 106 CFU/100 mL in the positive sites. The highest average counts of fecal coliforms (1.6 × 106 ± 2.1 × 106 CFU/100 mL) and E. coli (3.4 × 105 ± 4.6 × 105) were recorded in samples collected from the midstream and downstream sites (S6 to S25). E. coli and fecal coliform counts were then compared to the SEQ-EAU standard (100 CFU/100 mL) for irrigation water quality. Results showed that water samples exceeded the standard based on the standard related to fecal coliforms (84/100, 84%) and E. coli counts (80/100, 80%). Overall, the majority of these samples, collected from the midstream and downstream areas, were present in regions with relatively concentrated agricultural practices; only upstream samples were found to be acceptable.
The suitability of water for recreational activities was assessed through the US EPA standard based on a threshold of fecal coliforms of 800 CFU/100 mL. Subsequently, 70/100 (70%) of the samples and 80/100 (80%) of the sites exceeded the recommended safety standard for recreational usage. Additionally, it was noted that samples collected from midstream and downstream sites exceeded the standard (S6 to S25).
Total Coliforms CFU/100 mL |
Fecal Coliforms CFU/100 mL |
Enterococci CFU/100 mL |
E. coli CFU/100 mL |
pH | DO mg/L |
BOD 5 mg/L |
Temperature (°C) |
Turbidity NTU |
|
S1 | 1.9 × 101 ± 1.7 × 101 | 5.4 ± 0.58 | 0 ± 0 | 5.0 ± 4.2 | 7.9 ± 0.1 | 10.9 ± 0.5 | 0.8 ± 0.4 | 14.5 ± 5.8 | 0.06 ± 0.01 |
S2 | 1.6 × 102 ± 2.4 × 102 | 10.25 ± 9.74 | 1.25 ± 1.9 | 1.4 ± 1.7 × 101 | 7.8 ± 0.3 | 10.9 ± 0.3 | 1.5 ± 0.9 | 14.9 ± 4.5 | 0.56 ± 0.24 |
S3 | 3.5 × 101 ± 3.3 × 101 | 9.8 ± 6.9 | 4.3 ± 4.3 | 1.6 × 101 ± 1.8 × 101 | 8 ± 0.3 | 10.4 ± 1.7 | 1.8 ± 1.5 | 17.9 ± 2.7 | 0.6 ± 0.3 |
S4 | 3.6 × 101 ± 3.9 × 101 | 7.5 ± 6 | 3.8 ± 4.3 | 8 ± 8.5 | 7.9 ± 0.3 | 10.6 ± 2 | 1.9 ± 1.5 | 16.7 ± 3.3 | 0.56 ± 0.23 |
S5 | 1.4 × 103 ± 1.2 × 103 | 82 ± 36.2 | 4.5 × 101 ± 3.6 × 101 | 5 × 102 ± 4.5 × 102 | 7.9 ± 0.4 | 10.6 ± 2.5 | 1.3 ± 1 | 14.9 ± 4.6 | 1.05 ± 0.57 |
S6 | 9.9 × 103 ± 6.0 × 103 | 4.1 × 103 ± 4.1 × 103 | 1.2 × 102 ± 7.5 × 101 | 4 × 103 ± 3.7 × 103 | 7.6 ± 0.3 | 10.2 ± 1.3 | 1 ± 0.4 | 15.9 ± 4.1 | 0.94 ± 0.37 |
S7 | 5.7 × 103 ± 5.0 × 103 | 4.6 × 103 ± 2.5 × 103 | 3.2 × 102 ± 3.4 × 102 | 4 × 103 ± 4.1 × 103 | 7.7 ± 0.2 | 10.7 ± 1.5 | 1 ± 0.4 | 17.6 ± 2.1 | 1.43 ± 0.59 |
S8 | 1.0 × 104 ± 5.9 × 103 | 7.9 × 103 ± 1.7 × 103 | 2.2 × 102 ± 2 × 102 | 5.4 × 103 ± 3 × 103 | 7.7 ± 0.3 | 10.5 ± 0.9 | 1 ± 0.6 | 17.5 ± 3 | 1.5 ± 0.5 |
S9 | 2.1 × 104 ± 8.7 × 103 | 4.6 × 103 ± 4.2 × 103 | 4.3 × 102 ± 5.2 × 102 | 1.1 × 103 ± 6.8 × 103 | 7.8 ± 0.3 | 10.5 ± 0.5 | 1.1 ± 0.8 | 17.9 ± 3 | 1.5 ± 0.54 |
S10 | 1.6 × 104 ± 1.3 × 104 | 5.7 × 103 ± 5.4 × 103 | 2 × 102 ± 1.5 × 102 | 3.4 × 103 ± 2.2 × 103 | 7.8 ± 0.1 | 10.9 ± 1.3 | 1.9 ± 1.1 | 17.5 ± 3.8 | 1.57 ± 0.42 |
S11 | 4.3 × 104 ± 3.9 × 104 | 2.4 × 104 ± 2 × 103 | 1.3 × 103 ± 1.5 × 103 | 2.4 × 104 ± 2.4 × 104 | 7.9 ± 0.1 | 10.6 ± 1.5 | 1.5 ± 0.6 | 17.8 ± 3.9 | 1.74 ± 0.12 |
S12 | 1.0 × 105 ± 9.8 × 104 | 3.4 × 104 ± 4.7 × 104 | 6.1 × 10 ± 5.5 × 103 | 5.8 × 104 ± 5.9 × 104 | 7.9 ± 0.2 | 10.4 ± 0.8 | 1.7 ± 0.3 | 16.3 ± 4.1 | 2.36 ± 0.5 |
S13 | 2.0 × 105 ± 2.1 × 105 | 4.5 × 104 ± 4.3 × 104 | 6 × 103 ± 4.9 × 103 | 1.8 × 104 ± 1.5 × 104 | 7.9 ± 0.2 | 10.4 ± 1.2 | 1.2 ± 0.3 | 17 ± 3.9 | 2.36 ± 0.6 |
S14 | 3.0 × 105 ± 3.5 × 105 | 5.4 × 104 ± 5.3 × 104 | 5.9 × 103 ± 4.9 × 103 | 2 × 104 ± 2.5 × 104 | 8 ± 0.5 | 10.4 ± 2 | 2.5 ± 1.3 | 17.8 ± 4.4 | 2.39 ± 0.7 |
S15 | 1.0 × 106 ± 6.3 × 105 | 1.4 × 105 ± 5.1 × 104 | 2.6 × 104 ± 2.1 × 104 | 3.8 × 104 ± 2 × 104 | 7.7 ± 0.2 | 9.1 ± 3.2 | 4.4 ± 1.5 | 19.4 ± 3.4 | 3.69 ± 1.65 |
S16 | 1.5 × 105 ± 1.1 × 105 | 2.1 × 104 ± 3.3 × 104 | 2.9 × 104 ± 2.7 × 104 | 1.1 × 104 ± 8.7 × 104 | 7.7 ± 0.2 | 9.6 ± 0.6 | 3.8 ± 1.8 | 17.8 ± 2.3 | 7.69 ± 5.15 |
S17 | 8.9 × 105 ± 9.1 × 105 | 9.3 × 104 ± 8.7 × 104 | 4.5 × 104 ± 4.5 × 104 | 3 × 104 ± 2.5 × 104 | 7.8 ± 0.1 | 8.1 ± 1.6 | 4.3 ± 0.8 | 17.6 ± 3.7 | 6.15 ± 3.25 |
S18 | 2.4 × 106 ± 1.7 × 106 | 3.6 × 105 ± 3.7 × 105 | 7.8 × 104 ± 6.8 × 104 | 1.2 × 105 ± 7.8 × 105 | 7.9 ± 0.4 | 7.1 ± 3.3 | 5.7 ± 1.7 | 17.5 ± 4.5 | 4.94 ± 1.97 |
S19 | 3.4 × 106 ± 3.2 × 106 | 1.2 × 106 ± 1.4 × 106 | 9.3 × 104 ± 8 × 104 | 2.4 × 105 ± 2.6 × 105 | 7.9 ± 0.4 | 7.8 ± 2.2 | 4.5 ± 1.4 | 16.4 ± 3.7 | 5.07 ± 2.2 |
S20 | 5.3 × 106 ± 7.8 × 106 | 1.6 × 106 ± 2.1 × 106 | 1.3 × 105 ± 1.2 × 105 | 3.4 × 105 ± 4.6 × 105 | 7.9 ± 0.4 | 8.6 ± 1.6 | 5.8 ± 1.2 | 17.9 ± 3.4 | 5.07 ± 2.92 |
S21 | 3.3 × 106 ± 5.5 × 106 | 2.1 × 105 ± 2.1 × 105 | 5.1 × 104 ± 3.4 × 104 | 2.9 × 105 ± 4.9 × 105 | 7.6 ± 0.3 | 8.7 ± 3.6 | 5.1 ± 1.1 | 16.4 ± 3.7 | 5.29 ± 2.53 |
S22 | 1.7 × 106 ± 1.9 × 106 | 2.1 × 105 ± 1.1 × 105 | 4.2 × 104 ± 2.7 × 104 | 1.5 × 105 ± 2.1 × 105 | 7.7 ± 0.1 | 8.5 ± 2 | 4.3 ± 1.3 | 17.6 ± 3.8 | 6.74 ± 2.26 |
S23 | 1.3 × 106 ± 8.4 × 105 | 2.5 × 105 ± 1 × 105 | 3.5 × 104 ± 1.1 × 104 | 1.7 × 105 ± 1.8 × 105 | 7.8 ± 0.3 | 8.4 ± 1 | 5.5 ± 1 | 18.9 ± 4.2 | 9.17 ± 3.89 |
S24 | 1.3 × 106 ± 4.5 × 105 | 3.3 × 105 ± 2.7 × 105 | 3.9 × 104 ± 3.3 × 104 | 6 × 104 ± 2.4 × 104 | 7.7 ± 0.1 | 8.8 ± 0.6 | 4.5 ± 1.1 | 18.1 ± 4.1 | 11.36 ± 2.81 |
S25 | 1.1 × 106 ± 5.5 × 105 | 3.7 × 105 ± 4.2 × 105 | 5.1 × 104 ± 5.9 × 104 | 6.2 × 104 ± 3.7 × 104 | 7.6 ± 0.2 | 9.5 ± 0.9 | 4.8 ± 1.8 | 18.7 ± 4.6 | 18.86 ± 11.01 |
A consistent pattern of strong positive correlations between bacterial contamination indicators (total coliforms, fecal coliforms, E. coli, and Enterococci) was observed in both seasons. DO consistently showed negative correlations with bacterial contamination indicators. BOD5 showed positive correlations with bacterial indicators, turbidity, and temperature.
Table 3 summarizes the correlation coefficients between the various water quality parameters during the dry and wet seasons.
During the dry season, total coliform and E. coli showed a strong positive correlation with BOD5 (r = 0.766, r = 0.760 respectively) and positive moderate correlation with turbidity (r = 0.497, r = 0.415). Fecal coliforms showed a very strong positive correlation with Enterococci (r = 0.878) and BOD5 (r = 0.669), and a moderate correlation with turbidity (r = 0.397). Negative correlations were observed between DO and total coliforms (r = −0.646), E. coli (r = −0.596), and fecal coliforms (r = −0.569). BOD5 showed a positive correlation with temperature (r = 0.537) and a negative correlation with pH (r = −0.487). A strong positive correlation was observed between pH and E. coli (r = 0.704), but not with fecal coliforms, fecal enterococci, and total coliforms. During the wet season, total coliforms showed a strong positive correlation with BOD5 (r = 0.719) and a weak positive correlation with turbidity (r = 0.288). A strong positive correlation was observed between E. coli and BOD5 (r = 0.736) and a moderate correlation between E. coli and turbidity (r = 0.478). Fecal coliform showed a moderate positive correlation with BOD5 (r = 0.539) and a weak correlation with turbidity (r = 0.256). Enterococci showed a very strong positive correlation with BOD5 (r = 0.875) and a moderate correlation with turbidity (r = 0.549). DO had negative correlations with total coliforms (r = −0.674), E. coli (r = −0.705), fecal coliforms (r = −0.444), and Enterococci (r = −0.800).
Of the 100 water samples, 56 ESBL-producing E. coli isolates were recovered. Resistance to penicillins (amoxicillin, ticarcillin, and piperacillin) was detected in all ESBL-producing E. coli isolates. Resistance to beta-lactamase inhibitor combinations was also observed including amoxicillin/clavulanic acid (31/56, 55%), ticarcillin/clavulanic acid (56/56, 100%), and piperacillin–tazobactam (46/56, 82%). All the ESBL-producing E. coli isolates were resistant to at least six out of the seven tested cephalosporins, with 35/56 (63%) exhibiting additional resistance to cefoxitin. All the ESBL-producing E. coli isolates were also resistant to monobactam, aztreonam, amikacin, ertapenem, and sulfonamide. Different levels of resistance were observed for the remaining antibiotics, including aminoglycosides (streptomycin (56/56, 100%), tobramycin (52/56, 93%), gentamicin (48/56, 86%)), fluoroquinolones (ciprofloxacin 40/56, 71%), and trimethoprim/sulfamethoxazole (42/56, 75%). Furthermore, only 2/56 (4%) of the ESBL-producing E. coli isolates were found to be resistant to imipenem. Additional resistance patterns are shown in Figure 2.
Total Coliforms | Fecal Coliforms | Enterococci | E. coli | pH | DO | BOD 5 | Temperature | Turbidity | ||
Total coliforms | dry season | 1 | 0.940** | 0.757** | 0.895** | −0.368 | −0.646** | 0.766** | 0.363 | 0.497* |
wet season | 1 | 0.862** | 0.786** | 0.807** | 0.142 | −0.674** | 0.719** | 0.144 | 0.288 | |
Fecal coliforms | dry season | 0.940** | 1 | 0.878** | 0.844** | −0.394 | −0.569** | 0.669** | 0.234 | 0.397* |
wet season | 0.862** | 1 | 0.755** | 0.813** | 0.289 | −0.444* | 0.539** | 0.141 | 0.256 | |
Enterococci | dry season | 0.757** | 0.878** | 1 | 0.729** | −0.511** | −0.566** | 0.717** | 0.199 | 0.445* |
wet season | 0.786** | 0.755** | 1 | 0.773** | 0.178 | −0.800** | 0.875** | 0.210 | 0.549** | |
E. coli | dry season | 0.895** | 0.844** | 0.729** | 1 | 0.704** | −0.596** | 0.760** | 0.363 | 0.415* |
wet season | 0.807** | 0.813** | 0.773** | 1 | 0.715** | −0.705** | 0.736** | 0.117 | 0.478* | |
pH | dry season | −0.368 | −0.394 | −0.511** | 0.704** | 1 | 0.598** | −0.487* | −0.178 | −0.432* |
wet season | 0.142 | 0.289 | 0.178 | 0.715** | 1 | −0.158 | −0.037 | −0.175 | −0.444* | |
DO | dry season | −0.646** | −0.569** | −0.566** | −0.596** | 0.598** | 1 | −0.789** | −0.459* | −0.732** |
wet season | −0.674** | −0.444* | −0.800** | −0.705** | −0.158 | 1 | −0.783** | −0.217 | −0.315 | |
BOD 5 | dry season | 0.766** | 0.669** | 0.717** | 0.760** | −0.487* | −0.789** | 1 | 0.537** | 0.648** |
wet season | 0.719** | 0.539** | 0.875** | 0.736** | −0.037 | −0.783** | 1 | 0.415* | 0.674** | |
Tem | dry season | 0.363 | 0.234 | 0.199 | 0.363 | −0.178 | −0.459* | 0.537** | 1 | 0.671** |
wet season | 0.144 | 0.141 | 0.210 | 0.117 | −0.175 | −0.217 | 0.415* | 1 | 0.378 | |
Turbidity | dry season | 0.497* | 0.397* | 0.445* | 0.415* | −0.432* | −0.732** | 0.648** | 0.671** | 1 |
wet season | 0.288 | 0.256 | 0.549** | 0.478* | −0.444* | −0.315 | 0.674** | 0.378 | 1 |
* significant Pearson correlation
The predominant phylogenetic group in the 56 ESBL-producing E. coli isolates was B2 (40/56, 71.4%), followed by A (10/56, 17.9%), non-typeable (NT) (3/56, 5.4%), D (2/56, 3.6%), and E (1/56, 1.8%). Among the B2 phylogroup isolates (n = 40), 25/40 (62.5%) possessed blaCTX-M, 14/50 (35%) possessed blaTEM, 26/40 (65%) possessed blaSHV, and 11/40 (27.5%) possessed the blaOXA gene. Out of the A phylogroup isolates (n = 10), 8/10 (80%) harbored blaCTX-M, 7/10 (70%) harbored blaTEM, 3/10 (30%) harbored blaSHV, and 3/10 (30%) harbored the blaOXA gene (Table 4).
ESBL genes were detected in all 56 ESBL-producing E. coli isolates. The blaCTX-M gene (40/56, 71%) was the most prevalent ARG, followed by blaTEM (36/56, 64%), blaSHV (20/56, 36%), and blaOXA (16/56, 29%). Co-occurrence of blaCTX-M with other ESBL genes (blaTEM, blaSHV, or blaOXA) was also detected in 31/56 (55%) of the MDR isolates in this study. Among them, blaCTX-M was co-carried with blaTEM (13/56, 23%), blaSHV (6/56, 11%), and blaOXA (2/56, 4%) ARGs. In addition, 5/56 (9%) of the MDR isolates carried the 4 genes, 7/56 (12%) carried blaCTX-M alone, 5/56 (9%) carried blaTEM alone, and none of the isolates carried blaSHV or blaOXA alone (Table 4).
![]() |
AMX = Amoxicillin, AMC = Amoxicillin/Clavulanic Acid, PIP = Piperacillin, TZP = Piperacillin/Tazobactam, TIC = Ticarcillin, TIM = Ticarcillin/Clavulanic Acid, IPM = Imipenem, ETP = Ertapenem, ATM = Aztreonam, CXM = Cefuroxime, FOX = Cefoxitin, CTX = Cefotaxime, CRO = Ceftriaxone, CAZ = Ceftazidime, FEP = Cefepime, CFM = Cefixime, SMN = Streptomycin, TOB = Tobramycin, GEN = Gentamicin, AMK = Amikacin, CIP = Ciprofloxacin, TET = Tetracycline, SSS = Sulfonamide, SXT = Trimethoprim/Sulfamethoxazole, NAL = Nalidixic Acid, CHL = Chloramphenicol, TMP = Trimethoprim. NT = Non-Typeable. Dark blue = resistant, white = susceptible, green = intermediate, and red = present.
It is widely acknowledged that untreated sewage and various agricultural and industrial pollutants have severe impacts on aquatic environments. However, there is a scarcity of studies focusing on fecal pollution and microbial safety of surface water in Lebanon, and in the Northern region specifically. Therefore, monitoring the quality of water used for irrigation is of utmost importance to control the spread of diseases in Lebanon, which is particularly vulnerable due to ongoing medical and economic crises.
The present study revealed that 98/100 (98%) of the water samples contained fecal coliforms including E. coli (90/100, 90%). When considering both fecal bacteria, it was observed that 80/100 (80%) and 84/100 (84%) of the water samples exceeded the SEQ-EAU-2003 standard for irrigation and recreational activities. The widespread presence of fecal indicators is not surprising, considering that 92% of the collected wastewater in Lebanon is discharged untreated into aquatic environments [18]. Our findings are higher than those reported in a previous study conducted across all rivers in Lebanon by Dagher et al. [31]. Pollution levels, expressed by the density of fecal indicators, tend to increase in densely populated and agriculturally/industrially active sites. This can be attributed to the fact that surface water carries sewage from different villages, as well as effluents from informal refugee settlements, various industries, and poultry farms. Notably, surface water serves as the primary source of irrigation for agricultural lands and is known to be contaminated with pollutants, including pesticides and human and animal waste [31]. Previous studies have reported contamination of products, such as spinach, parsley, cabbage, and lettuce, collected from the Beqaa Valley with fecal bacteria [32]. Considering that fresh produce is often consumed raw, the risk of foodborne diseases in the Northern region is heightened.
Physicochemical characteristics including pH, temperature, conductivity, and turbidity can significantly impact the aquatic ecology. Water temperature is influenced by local climate and plays a crucial role in aquatic ecosystems, influencing various physicochemical characteristics of the water environment and affecting the reproduction and metabolism of aquatic organisms [33]. The variation in pH along different sampling points could be attributed to the geological composition of the riverbed and pollution through surface runoff. In addition, turbidity levels increased from upstream to downstream sites, exceeding the guideline values. This difference may be due to soil erosion and water runoff from human activities. The highest average DO levels were observed during the dry sample period, which could be attributed to nutrient enrichment from nearby fields and the discharge of chemicals or pollutants from surrounding industries. During the dry season, the BOD5 content is lower than in the wet season due to increased surface runoff and rainfall discharge into the water. Moreover, excessive bacterial load in water indicates high BOD5 levels, which can result from the consumption of dissolved oxygen, leading to a decrease in DO concentration values. Consequently, fish and other aquatic organisms of Lebanese rivers may struggle to survive in such water conditions [34]. The Pearson correlation coefficient (r) is a statistical tool used to assess the relationship between different water quality parameters by measuring the strength and direction of the linear relationship between variables [35]. In this study, strong positive correlations were observed during both seasons between bacterial contaminants (total coliforms, fecal coliforms, Enterococci) and water quality parameters like BOD5 and turbidity. Additionally, DO levels appear to be negatively affected by bacterial contamination. Temperature seems to influence BOD5 and turbidity positively, suggesting that temperature may similarly influence water quality parameters throughout the year. High correlation coefficients between variables indicate that they share similar sources of contamination or undergo similar geochemical processes. Furthermore, the correlation strength differences between the seasons may be attributed to environmental factors, such as rainfall and runoff or increased water quantity in the wet season, that influence water quality.
The presence of fecal contamination in water samples was assessed by isolating E. coli strains, as an indicator of fecal contamination and the potential presence of other pathogenic microorganisms living in communities. Moreover, the isolated antibiotic-resistant E. coli in surface water could be an important carrier and a rich source of emerging and spreading antimicrobial-resistant determinants to other surrounding counterparts [36]. E. coli strains can be classified into different phylogroups, namely, A, B1, B2, C, D, E, and F. Phylogroups A and B1 are sister groups, while phylogroups B2 and D are known to be more virulent [37]. It should be highlighted that isolates from aquatic habitats have been detected in phylogenetic groups A and B1 more frequently than isolates from phylogenetic groups B2 and D [38]. In this study, phylogroup B2 was the most prevalent, followed by phylogroups A, D, and E, respectively. E. coli strains related to the B2 and D phylogroups carry more virulence-associated genes compared to the other phylogroups and are primarily associated with extraintestinal infections, including urinary tract infections [37]. However, our findings are in contrast with previous studies conducted in Portugal, Malaysia, and Iraq that showed a predominance of isolates belonging to the A and B1 phylogenetic groups [39]–[41]. Moreover, a recent study conducted by Diab et al. [21] reported that phylogroup A was the most prevalent in Lebanon's water, while phylogroup B2 was the least prevalent. These discrepancies could be attributed to differences in water sources (wells, springs, estuaries, or rivers) and geographical locations.
Given the release of untreated sewage and other contaminants into Lebanese surface water and the widespread ARB in other crucial matrices in Lebanon [42], addressing ARB in our samples was necessary. Moreover, our findings showed that approximately 56/100 (56%) of the E. coli isolates were MDR, which is consistent with the findings of Bong et al. [36], who reported the presence of MDR ESBLs in anthropogenic-impacted rivers. The percentage of MDR E. coli in Lebanese surface water is slightly lower than what has been reported for sewage-contaminated rivers in Romania (60.34%) and Ethiopia (78%) [43],[44]. However, considering that Lebanon is one of the smallest countries in Asia in terms of total area (~10,450 km2) and population (~6.8 million), the prevalence of MDR E. coli in surface water is still a significant concern. Our finding aligns with a previous study by Tokajian et al. [16], which examined bacterial loads in sewage-contaminated surface water samples from Lebanon. In another study, ESBL-producing E. coli constituted 77.3% of all isolates and was also the predominant organism in various water sources in Lebanon, including wells, estuaries, and rivers [21]. In Lebanon, the increasing prevalence of antibiotic-resistant bacteria in surface water has become a growing concern due to the absence of efficient strategies for collection and domestic wastewater management. The Central Administration of Statistics reports that only 37% of buildings in Lebanon are connected to a sewer network, while the rest rely on cesspools and septic tanks or directly release untreated sewage into the environment [22]. The North district, which hosts a large number of refugees, also encompasses the most impoverished and vulnerable communities. Moreover, 30% of these refugees reside in densely populated areas, exacerbating issues such as overcrowding, infrastructure damage, and limited access to water and sanitation services [22]. Consequently, this population increase has intensified the pressure on various resources in Lebanon, including water. According to the International Institute for Environment and Development, there has been a 30% increase in water demand since the onset of the Syrian conflict. Additionally, research published by the Ministry of Environment in 2014 indicated a 33% rise in wastewater pollution since 2011, which can be attributed to the population growth around water sources [22].
All the ESBL-producing E. coli isolates in this study exhibited resistance to third-generation cephalosporins and aztreonam. Thus, we have investigated the distribution and characterization of ESBL-producing E. coli. Interestingly, 62% of strains showed additional resistance to cefoxitin. The resistance patterns varied within the different phylogroups. The phylogroups B2 and D exhibited lower levels of antimicrobial resistance compared to the phylogroups A and B1 [45]. These findings reflect the influence of antibiotic overuse and inadequate wastewater management, which contribute to the development and spread of antimicrobial resistance in the environment. We then investigated the presence of ESBL-encoding genes in these isolates, including blaCTX-M, blaTEM, blaOXA, and blaSHV. The blaCTX-M, blaTEM, and blaSHV genes were more prevalent than blaOXA. These findings are consistent with a recent study that reported the recovery of ESBL-producing E. coli from natural water sources [46]. The presence of blaCTX-M genes in water is often associated with highly self-transferable plasmids, indicating the transfer of ESBL-producing E. coli from sewage to rivers [47]. The most common ESBL gene identified in our study was blaCTX-M-15 (32/56, 57%), which is consistent with recent studies conducted in South Africa and Portugal and with studies in Lebanon covering estuaries, wells, springs, and rivers [16],[21],[48],[49]. This frequency is higher than that shown in Thailand (44.7%) [50], but a higher prevalence was reported in Tunisia (85%) and Germany (81%) [51],[52]. blaCTX-M-15 is also the predominant ESBL gene in Lebanon and has been detected in isolates recovered from animals as well as in healthy and hospitalized patients [21],[53]. The presence of untreated sewage and industrial water in surface water may contribute to the high prevalence of this resistance determinant [42]. The dissemination of blaCTX-M-15 has been globally linked to the spread of highly virulent strains of E. coli, such as ST131 (belonging to the B2 phylogenetic group and serotype O25:H4) and ST405 (phylogroup D) [54]. Co-occurrence of blaCTX-M-15 with other ESBL genes (blaTEM, blaSHV, or blaOXA-48) was also observed in 32/56 (57%) of the MDR isolates in our study. This phenomenon is common among Gram-negative organisms isolated from surface water [55]. In addition to blaCTX-M-15, blaTEM was found in 36/56 (64%) of isolates. This gene has been commonly detected in sewage and river water samples in different countries such as Poland and China and even in river water samples from Lebanon [16],[56],[57]. Previous studies have shown that blaTEM has been frequently associated with IncF plasmids, implicated in the dissemination of resistance determinants, and widely distributed among Enterobacteriaceae [16]. The presence of this gene indicates the contamination of surface waters by antibiotic-resistant organisms originating from human activities or hospital effluents, as they are a major source of the blaTEM gene [55]. Moreover, the carbapenemase gene, blaOXA-48, was observed in 11/56 (20%) of the ESBL-producing E. coli isolates. This finding is greater than other recent studies that reported the presence of gene encoding in 3.9% of US drinking water and 4% of water bodies in India [55],[58] but less than Kuwait, where blaOXA-48 was found in 30% of marine environment samples [59]. In Lebanon, carbapenemase-producing E. coli were recovered from water samples with blaOXA-48 being the most commonly identified gene [21]. This gene is commonly associated with the spread of carbapenem resistance and has been reported in Enterobacteriaceae from various sources, including humans, animals, and the environment [60]. The blaCTX-M-15 gene was co-present with blaTEM, blaSHV, and blaOXA genes, thus promoting the evolution and dissemination of MDR and extensively drug-resistant (XDR) strains [61]. It has been reported that bacteria may integrate ARGs as residential genes in their chromosome, ensuring a stable propagation of ESBL Enterobacteriaceae [62].
Moreover, we aimed to study the phylogenetic classification of ESBL-producing E. coli isolates and investigate their antimicrobial resistance patterns for further information on the regional E. coli phylogenetic grouping and association between the phylogenetic groups and their antimicrobial resistance patterns. In the present study, the highest MDR frequency was detected in phylogenetic group B2, followed by groups A and D. Lower resistance levels were observed in isolates belonging to group E. In contrast, the highest MDR frequency was found in phylogenetic group A in Portugal, Malaysia, and Iraq [39]–[41]. In addition, there was lower prevalence of MDR in isolates in phylogenetic group B2 in Spain [63]. The disparity in these findings may be explained by the geographical differences as well as the origins of isolates found at various locations.
Although this study had limitations in terms of the number of resistance genes studied, the observed patterns of genetic resistance are intriguing and motivate more comprehensive investigations. Hence, future studies with higher sample size should consider various ESBL-producing coliforms. This will provide a deeper understanding of the current distribution of resistance genes and their dissemination among different organisms. Further genomic analyses of clinical and environmental isolates are of the utmost importance to uncover possible circulation of strains between the hospital and the community settings.
Antibiotics are consistently used for medical and agricultural purposes. These antibiotics are excreted from humans and animals unmetabolized, leading to the emergence of ARB, which are then disseminated by water to humans and animals. Our study highlights the wide spread of antibiotic-resistant bacteria and resistance genes in surface water in Northern Lebanon, particularly in areas with inadequate wastewater management and high population density. This situation is alarming since ARB poses a potential threat to public health, and surface water is directly intended for human consumption without any further treatment or is used to water animals and irrigate crops. These findings highlight the urgency for implementing collaborative strategies between the water, health, environment, and agricultural sectors to reduce human exposure to pathogens and pinpoint the causes of environmental pollution, including the alarming issue of antimicrobial resistance. This is in line with the One Health project, spearheaded by the WHO, which includes actions to safeguard the wellbeing of humans, animals, and ecosystems. Sustainable solutions such as improved wastewater treatment systems and proper sanitation infrastructure are required. Additionally, monitoring antibiotic use in human health, but also in animal health, and implementing antibiotic stewardship programs can help reduce the selection pressure for resistance and preserve the effectiveness of antibiotics.
Use of AI tools declaration
The authors declare they have not used Artificial Intelligence (AI) tools in the creation of this article.
[1] |
Screen JA, Simmonds I (2010) The central role of diminishing sea ice in recent Arctic temperature amplification. Nature 464: 1334–1337. https://doi.org/10.1038/nature09051 doi: 10.1038/nature09051
![]() |
[2] | Park JW, Korosov AA, Babiker M, et al. (2019) Classification of sea ice types in Sentinel-1 SAR images. Cryosphere Discuss. 2019: 1–23. |
[3] |
Dabboor M, Geldsetzer T (2014) Towards sea ice classification using simulated RADARSAT Constellation Mission compact polarimetric SAR imagery. Remote Sens Environ 140: 189–195. https://doi.org/10.1016/j.rse.2013.08.035 doi: 10.1016/j.rse.2013.08.035
![]() |
[4] |
Cardellach E, Fabra F, Nogués-Correig O, et al. (2011) GNSS-R ground-based and airborne campaigns for ocean, land, ice, and snow techniques: Application to the GOLD-RTR data sets. Radio Sci 46: 1–16. https://doi.org/10.1029/2011rs004683 doi: 10.1029/2011rs004683
![]() |
[5] | Martin-Neira M (1993) A passive reflectometry and interferometry system (PARIS): Application to ocean altimetry. ESA J 17: 331–355. |
[6] | Hall C, Cordey R (1988) Multistatic scatterometry, International Geoscience and Remote Sensing Symposium, Remote Sensing: Moving Toward the 21st Century', IEEE. |
[7] |
Liu Y, Collett I, Morton YJ (2019) Application of Neural Network to GNSS-R Wind Speed Retrieval. IEEE T Geosc Remote 57: 9756–9766. https://doi.org/10.1109/tgrs.2019.2929002 doi: 10.1109/tgrs.2019.2929002
![]() |
[8] |
Garrison JL, Komjathy A, Zavorotny VU, et al. (2022) Wind speed measurement using forward scattered GPS signals. IEEE T Geosc Remote 40: 50–65. https://doi.org/10.1109/36.981349 doi: 10.1109/36.981349
![]() |
[9] |
Clarizia M P, Ruf C S, Jales P, et al. (2014) Gommenginger. Spaceborne GNSS-R Minimum Variance Wind Speed Estimator. IEEE T Geosc Remote 52: 6829–6843. https://doi.org/10.1109/Tgrs.2014.2303831 doi: 10.1109/Tgrs.2014.2303831
![]() |
[10] |
Yu K, Li Y, Chang X (2019) Chang. Snow Depth Estimation Based on Combination of Pseudorange and Carrier Phase of GNSS Dual-Frequency Signals. IEEE T Geosc Remote 57: 1817–1828. https://doi.org/10.1109/tgrs.2018.2869284 doi: 10.1109/tgrs.2018.2869284
![]() |
[11] |
Camps A, Park H, Pablos M, et al. (2016) Sensitivity of GNSS-R Spaceborne Observations to Soil Moisture and Vegetation. IEEE J-Stars 9: 4730–4742. https://doi.org/10.1109/jstars.2016.2588467 doi: 10.1109/jstars.2016.2588467
![]() |
[12] |
Rodriguez-Alvarez N, Bosch-Lluis X, Camps A, et al. (2009) Soil Moisture Retrieval Using GNSS-R Techniques: Experimental Results Over a Bare Soil Field. IEEE T Geosc Remote 47: 3616–3624. https://doi.org/10.1109/tgrs.2009.2030672 doi: 10.1109/tgrs.2009.2030672
![]() |
[13] |
Di Simone A, Park H, Riccio D, et al. (2017) Sea Target Detection Using Spaceborne GNSS-R Delay-Doppler Maps: Theory and Experimental Proof of Concept Using TDS-1 Data. IEEE J-Stars 10: 4237–4255. https://doi.org/10.1109/jstars.2017.2705350 doi: 10.1109/jstars.2017.2705350
![]() |
[14] |
Alonso-Arroyo A, Zavorotny VU, Camps A (2017) Sea ice detection using UK TDS-1 GNSS-R data. IEEE J-Stars 55: 4989–5001. https://doi.org/10.1109/TGRS.2017.2699122 doi: 10.1109/TGRS.2017.2699122
![]() |
[15] |
Yan Q, Huang W (2016) Sea ice detection from GNSS-R delay-Doppler map. 2016 17th International Symposium on Antenna Technology and Applied Electromagnetics (ANTEM), 2016: 1–2. https://doi.org/10.1109/ANTEM.2016.7550123 doi: 10.1109/ANTEM.2016.7550123
![]() |
[16] |
Yan Q, Huang W (2016) Spaceborne GNSS-R Sea Ice Detection Using Delay-Doppler Maps: First Results From the U.K. TechDemoSat-1 Mission. IEEE J-Stars 9: 4795–4801. https://doi.org/10.1109/jstars.2016.2582690 doi: 10.1109/jstars.2016.2582690
![]() |
[17] |
Munoz-Martin J F, Perez A, Camps A, et al. (2020) Snow and Ice Thickness Retrievals Using GNSS-R: Preliminary Results of the MOSAiC Experiment. Rem Sen 12: 4038. https://doi.org/10.3390/rs12244038 doi: 10.3390/rs12244038
![]() |
[18] |
Zavorotny VU, Voronovich AG (2000) Scattering of GPS signals from the ocean with wind remote sensing application. IEEE T Geosci Remote 38: 951–964. https://doi.org/10.1109/36.841977 doi: 10.1109/36.841977
![]() |
[19] |
Zhu Y, Yu K, Zou J, et al. (2017) Sea Ice Detection Based on Differential Delay-Doppler Maps from UK TechDemoSat-1. Sensors (Basel) 17: 1614. https://doi.org/10.3390/s17071614 doi: 10.3390/s17071614
![]() |
[20] |
Yan Q, Huang W (2018) Sea Ice Sensing From GNSS-R Data Using Convolutional Neural Networks. IEEE Geosci Remote Sens Lett 15: 1510–1514. https://doi.org/10.1109/lgrs.2018.2852143 doi: 10.1109/lgrs.2018.2852143
![]() |
[21] |
Hu Y, Jiang Z, Liu W, et al. (2023) GNSS-R Sea Ice Detection Based on Linear Discriminant Analysis. IEEE T Geosci Remote. https://doi.org/10.1109/TGRS.2023.3269088 doi: 10.1109/TGRS.2023.3269088
![]() |
[22] | Eustice D, Baylis C, Marks RJ (2015) Woodward's ambiguity function: From foundations to applications, 2015 Texas Symposium on Wireless and Microwave Circuits and Systems (WMCS); 2015: IEEE. 2015: 1–17. https://doi.org/10.1109/WMCaS.2015.7233208 |
[23] | Jales P, Unwin M (2015) MERRByS product manual: GNSS reflectometry on TDS-1 with the SGR-ReSI. Surrey Satellite Technol. Ld. Guildford, UK. |
[24] |
Samko O, Marshall A D, Rosin P L (2006) Rosin. Selection of the optimal parameter value for the Isomap algorithm. Pattern Recogn Lett 27: 968–979. https://doi.org/10.1016/j.patrec.2005.11.017 doi: 10.1016/j.patrec.2005.11.017
![]() |
[25] |
Heinzel S, Berg D, Gasser T, et al. (2019) Update of the MDS research criteria for prodromal Parkinson's disease. Mov Disord. 34: 1464–1470. https://doi.org/10.1002/mds.27802 doi: 10.1002/mds.27802
![]() |
[26] |
Hougardy S (2010) The Floyd–Warshall algorithm on graphs with negative cycles. Inform Process Lett 110: 279–281. https://doi.org/10.1016/j.ipl.2010.02.001 doi: 10.1016/j.ipl.2010.02.001
![]() |
[27] |
Cortes C, Vapnik V (1995) Support-Vector Networks. Mach Learn 20: 273–297. https://doi.org/10.1007/Bf00994018 doi: 10.1007/Bf00994018
![]() |
[28] |
Liang W, Luo S, Zhao G, et al. (2020) Predicting Hard Rock Pillar Stability Using GBDT, XGBoost, and LightGBM Algorithms. Mathematics 8: 765. https://doi.org/10.3390/math8050765 doi: 10.3390/math8050765
![]() |
[29] | Izenman A (2013) Linear Discriminant Analysis, In: Modern Multivariate Statistical Techniques, Springer Texts in Statistics: Springer, 237–280. |
[30] |
Turk M, Pentland A (1991) Eigenfaces for recognition. J Cogn Neurosci 3: 71–86. https://doi.org/10.1162/jocn.1991.3.1.71 doi: 10.1162/jocn.1991.3.1.71
![]() |
[31] |
Wang L, Zhang Y, Feng J (2005) On the Euclidean distance of images. IEEE Trans Pattern Anal Mach Intel 27: 1334–1339. https://doi.org/10.1109/TPAMI.2005.165 doi: 10.1109/TPAMI.2005.165
![]() |
[32] | Guo G, Wang H, Bell D, et al. (2003) KNN model-based approach in classification, On The Move to Meaningful Internet Systems 2003: CoopIS, DOA, and ODBASE: OTM Confederated International Conferences, CoopIS, DOA, and ODBASE 2003, Catania, Sicily, Italy, November 3–7. Proceedings; Springer. |
[33] | Kraemer HC (2014) Kappa coefficient. Wiley StatsRef: statistics reference online: 1–4. https://doi.org/10.1002/9781118445112.stat00365.pub2 |
[34] |
Alonso-Arroyo A, Zavorotny V U, Camps A (2017) Sea Ice Detection Using U.K. TDS-1 GNSS-R Data. IEEE T Geosci Remot 55: 4989–5001. https://doi.org/10.1109/tgrs.2017.2699122 doi: 10.1109/tgrs.2017.2699122
![]() |
[35] |
Yan Q, Huang W (2019) Detecting Sea Ice From TechDemoSat-1 Data Using Support Vector Machines With Feature Selection. IEEE J--STARS 12: 1409–1416. https://doi.org/10.1109/jstars.2019.2907008 doi: 10.1109/jstars.2019.2907008
![]() |
[36] |
Yan Q, Huang W (2017) Neural Networks Based Sea Ice Detection and Concentration Retrieval From GNSS-R Delay-Doppler Maps. IEEE J--STARS 10: 3789–3798. https://doi.org/10.1109/jstars.2017.2689009 doi: 10.1109/jstars.2017.2689009
![]() |
PCR target | Primer sequence (5′-3′) | Annealing Temperature (°C) | Length (bp) | Reference | ||
Phylogenetic | ChuA | chuA.1b | ATGGTACCGGACGAACCAAC | 59 | 288 | [27] |
chuA.2 | TGCCGCCAGTACCAAAGACA | |||||
yjaA | yjaA.1b | CAAACGTGAAGTGTCAGGAG | 59 | 211 | ||
yjaA.2b | AATGCGTTCCTCAACCTGTG | |||||
TspE4.C | TspE4C2.1 | CACTATTCGTAAGGTCATCC | 59 | 152 | ||
TspE4C2.2b | AGTTTATCGCTGCGGGTCGC | |||||
arpA | AceK.f | AACGCTATTCGCCAGCTTGC | 59 | 400 | ||
ArpA1.r | TCTCCCCATACCGTACGCTA | |||||
β-lactam resistance | blaTEM | TemA1 | ATAAAATTCTTGAAGAC | 42 | 1075 | [16] |
TemB1 | TTACCAATGCTTAATCA | |||||
blaSHV | SHV-F | CACTCAAGGATGTATTGTG | 49 | 822 | ||
SHV-R | TTAGCGTTGCCAGTGCTCG | |||||
blaCTX-M | CTX-C1 | ATGTGCAGCACCAGTAAAGT | 54 | 545 | ||
CTX-C2 | ACCGCGATATCGTTGGTGG | |||||
blaOXA | OXA-F | ACACAATACATATCAACTTCGC | 61 | 813 | ||
OXA-R | AGTGTGTTTAGAATGGTGATC | |||||
blaCTX-M-15 | F | CACACGTGGAATTTAGGGACT | 50 | 995 | [28] | |
R | GCCGTCTAAGGCGATAAACA | |||||
Carbapenem resistance | blaOXA-48 | F | GCTTGATCGCCCTCGATT | 60 | 238 | [29] |
R | GATTTGCTCCGTGGCCGAAA |
Total Coliforms CFU/100 mL |
Fecal Coliforms CFU/100 mL |
Enterococci CFU/100 mL |
E. coli CFU/100 mL |
pH | DO mg/L |
BOD 5 mg/L |
Temperature (°C) |
Turbidity NTU |
|
S1 | 1.9 × 101 ± 1.7 × 101 | 5.4 ± 0.58 | 0 ± 0 | 5.0 ± 4.2 | 7.9 ± 0.1 | 10.9 ± 0.5 | 0.8 ± 0.4 | 14.5 ± 5.8 | 0.06 ± 0.01 |
S2 | 1.6 × 102 ± 2.4 × 102 | 10.25 ± 9.74 | 1.25 ± 1.9 | 1.4 ± 1.7 × 101 | 7.8 ± 0.3 | 10.9 ± 0.3 | 1.5 ± 0.9 | 14.9 ± 4.5 | 0.56 ± 0.24 |
S3 | 3.5 × 101 ± 3.3 × 101 | 9.8 ± 6.9 | 4.3 ± 4.3 | 1.6 × 101 ± 1.8 × 101 | 8 ± 0.3 | 10.4 ± 1.7 | 1.8 ± 1.5 | 17.9 ± 2.7 | 0.6 ± 0.3 |
S4 | 3.6 × 101 ± 3.9 × 101 | 7.5 ± 6 | 3.8 ± 4.3 | 8 ± 8.5 | 7.9 ± 0.3 | 10.6 ± 2 | 1.9 ± 1.5 | 16.7 ± 3.3 | 0.56 ± 0.23 |
S5 | 1.4 × 103 ± 1.2 × 103 | 82 ± 36.2 | 4.5 × 101 ± 3.6 × 101 | 5 × 102 ± 4.5 × 102 | 7.9 ± 0.4 | 10.6 ± 2.5 | 1.3 ± 1 | 14.9 ± 4.6 | 1.05 ± 0.57 |
S6 | 9.9 × 103 ± 6.0 × 103 | 4.1 × 103 ± 4.1 × 103 | 1.2 × 102 ± 7.5 × 101 | 4 × 103 ± 3.7 × 103 | 7.6 ± 0.3 | 10.2 ± 1.3 | 1 ± 0.4 | 15.9 ± 4.1 | 0.94 ± 0.37 |
S7 | 5.7 × 103 ± 5.0 × 103 | 4.6 × 103 ± 2.5 × 103 | 3.2 × 102 ± 3.4 × 102 | 4 × 103 ± 4.1 × 103 | 7.7 ± 0.2 | 10.7 ± 1.5 | 1 ± 0.4 | 17.6 ± 2.1 | 1.43 ± 0.59 |
S8 | 1.0 × 104 ± 5.9 × 103 | 7.9 × 103 ± 1.7 × 103 | 2.2 × 102 ± 2 × 102 | 5.4 × 103 ± 3 × 103 | 7.7 ± 0.3 | 10.5 ± 0.9 | 1 ± 0.6 | 17.5 ± 3 | 1.5 ± 0.5 |
S9 | 2.1 × 104 ± 8.7 × 103 | 4.6 × 103 ± 4.2 × 103 | 4.3 × 102 ± 5.2 × 102 | 1.1 × 103 ± 6.8 × 103 | 7.8 ± 0.3 | 10.5 ± 0.5 | 1.1 ± 0.8 | 17.9 ± 3 | 1.5 ± 0.54 |
S10 | 1.6 × 104 ± 1.3 × 104 | 5.7 × 103 ± 5.4 × 103 | 2 × 102 ± 1.5 × 102 | 3.4 × 103 ± 2.2 × 103 | 7.8 ± 0.1 | 10.9 ± 1.3 | 1.9 ± 1.1 | 17.5 ± 3.8 | 1.57 ± 0.42 |
S11 | 4.3 × 104 ± 3.9 × 104 | 2.4 × 104 ± 2 × 103 | 1.3 × 103 ± 1.5 × 103 | 2.4 × 104 ± 2.4 × 104 | 7.9 ± 0.1 | 10.6 ± 1.5 | 1.5 ± 0.6 | 17.8 ± 3.9 | 1.74 ± 0.12 |
S12 | 1.0 × 105 ± 9.8 × 104 | 3.4 × 104 ± 4.7 × 104 | 6.1 × 10 ± 5.5 × 103 | 5.8 × 104 ± 5.9 × 104 | 7.9 ± 0.2 | 10.4 ± 0.8 | 1.7 ± 0.3 | 16.3 ± 4.1 | 2.36 ± 0.5 |
S13 | 2.0 × 105 ± 2.1 × 105 | 4.5 × 104 ± 4.3 × 104 | 6 × 103 ± 4.9 × 103 | 1.8 × 104 ± 1.5 × 104 | 7.9 ± 0.2 | 10.4 ± 1.2 | 1.2 ± 0.3 | 17 ± 3.9 | 2.36 ± 0.6 |
S14 | 3.0 × 105 ± 3.5 × 105 | 5.4 × 104 ± 5.3 × 104 | 5.9 × 103 ± 4.9 × 103 | 2 × 104 ± 2.5 × 104 | 8 ± 0.5 | 10.4 ± 2 | 2.5 ± 1.3 | 17.8 ± 4.4 | 2.39 ± 0.7 |
S15 | 1.0 × 106 ± 6.3 × 105 | 1.4 × 105 ± 5.1 × 104 | 2.6 × 104 ± 2.1 × 104 | 3.8 × 104 ± 2 × 104 | 7.7 ± 0.2 | 9.1 ± 3.2 | 4.4 ± 1.5 | 19.4 ± 3.4 | 3.69 ± 1.65 |
S16 | 1.5 × 105 ± 1.1 × 105 | 2.1 × 104 ± 3.3 × 104 | 2.9 × 104 ± 2.7 × 104 | 1.1 × 104 ± 8.7 × 104 | 7.7 ± 0.2 | 9.6 ± 0.6 | 3.8 ± 1.8 | 17.8 ± 2.3 | 7.69 ± 5.15 |
S17 | 8.9 × 105 ± 9.1 × 105 | 9.3 × 104 ± 8.7 × 104 | 4.5 × 104 ± 4.5 × 104 | 3 × 104 ± 2.5 × 104 | 7.8 ± 0.1 | 8.1 ± 1.6 | 4.3 ± 0.8 | 17.6 ± 3.7 | 6.15 ± 3.25 |
S18 | 2.4 × 106 ± 1.7 × 106 | 3.6 × 105 ± 3.7 × 105 | 7.8 × 104 ± 6.8 × 104 | 1.2 × 105 ± 7.8 × 105 | 7.9 ± 0.4 | 7.1 ± 3.3 | 5.7 ± 1.7 | 17.5 ± 4.5 | 4.94 ± 1.97 |
S19 | 3.4 × 106 ± 3.2 × 106 | 1.2 × 106 ± 1.4 × 106 | 9.3 × 104 ± 8 × 104 | 2.4 × 105 ± 2.6 × 105 | 7.9 ± 0.4 | 7.8 ± 2.2 | 4.5 ± 1.4 | 16.4 ± 3.7 | 5.07 ± 2.2 |
S20 | 5.3 × 106 ± 7.8 × 106 | 1.6 × 106 ± 2.1 × 106 | 1.3 × 105 ± 1.2 × 105 | 3.4 × 105 ± 4.6 × 105 | 7.9 ± 0.4 | 8.6 ± 1.6 | 5.8 ± 1.2 | 17.9 ± 3.4 | 5.07 ± 2.92 |
S21 | 3.3 × 106 ± 5.5 × 106 | 2.1 × 105 ± 2.1 × 105 | 5.1 × 104 ± 3.4 × 104 | 2.9 × 105 ± 4.9 × 105 | 7.6 ± 0.3 | 8.7 ± 3.6 | 5.1 ± 1.1 | 16.4 ± 3.7 | 5.29 ± 2.53 |
S22 | 1.7 × 106 ± 1.9 × 106 | 2.1 × 105 ± 1.1 × 105 | 4.2 × 104 ± 2.7 × 104 | 1.5 × 105 ± 2.1 × 105 | 7.7 ± 0.1 | 8.5 ± 2 | 4.3 ± 1.3 | 17.6 ± 3.8 | 6.74 ± 2.26 |
S23 | 1.3 × 106 ± 8.4 × 105 | 2.5 × 105 ± 1 × 105 | 3.5 × 104 ± 1.1 × 104 | 1.7 × 105 ± 1.8 × 105 | 7.8 ± 0.3 | 8.4 ± 1 | 5.5 ± 1 | 18.9 ± 4.2 | 9.17 ± 3.89 |
S24 | 1.3 × 106 ± 4.5 × 105 | 3.3 × 105 ± 2.7 × 105 | 3.9 × 104 ± 3.3 × 104 | 6 × 104 ± 2.4 × 104 | 7.7 ± 0.1 | 8.8 ± 0.6 | 4.5 ± 1.1 | 18.1 ± 4.1 | 11.36 ± 2.81 |
S25 | 1.1 × 106 ± 5.5 × 105 | 3.7 × 105 ± 4.2 × 105 | 5.1 × 104 ± 5.9 × 104 | 6.2 × 104 ± 3.7 × 104 | 7.6 ± 0.2 | 9.5 ± 0.9 | 4.8 ± 1.8 | 18.7 ± 4.6 | 18.86 ± 11.01 |
Total Coliforms | Fecal Coliforms | Enterococci | E. coli | pH | DO | BOD 5 | Temperature | Turbidity | ||
Total coliforms | dry season | 1 | 0.940** | 0.757** | 0.895** | −0.368 | −0.646** | 0.766** | 0.363 | 0.497* |
wet season | 1 | 0.862** | 0.786** | 0.807** | 0.142 | −0.674** | 0.719** | 0.144 | 0.288 | |
Fecal coliforms | dry season | 0.940** | 1 | 0.878** | 0.844** | −0.394 | −0.569** | 0.669** | 0.234 | 0.397* |
wet season | 0.862** | 1 | 0.755** | 0.813** | 0.289 | −0.444* | 0.539** | 0.141 | 0.256 | |
Enterococci | dry season | 0.757** | 0.878** | 1 | 0.729** | −0.511** | −0.566** | 0.717** | 0.199 | 0.445* |
wet season | 0.786** | 0.755** | 1 | 0.773** | 0.178 | −0.800** | 0.875** | 0.210 | 0.549** | |
E. coli | dry season | 0.895** | 0.844** | 0.729** | 1 | 0.704** | −0.596** | 0.760** | 0.363 | 0.415* |
wet season | 0.807** | 0.813** | 0.773** | 1 | 0.715** | −0.705** | 0.736** | 0.117 | 0.478* | |
pH | dry season | −0.368 | −0.394 | −0.511** | 0.704** | 1 | 0.598** | −0.487* | −0.178 | −0.432* |
wet season | 0.142 | 0.289 | 0.178 | 0.715** | 1 | −0.158 | −0.037 | −0.175 | −0.444* | |
DO | dry season | −0.646** | −0.569** | −0.566** | −0.596** | 0.598** | 1 | −0.789** | −0.459* | −0.732** |
wet season | −0.674** | −0.444* | −0.800** | −0.705** | −0.158 | 1 | −0.783** | −0.217 | −0.315 | |
BOD 5 | dry season | 0.766** | 0.669** | 0.717** | 0.760** | −0.487* | −0.789** | 1 | 0.537** | 0.648** |
wet season | 0.719** | 0.539** | 0.875** | 0.736** | −0.037 | −0.783** | 1 | 0.415* | 0.674** | |
Tem | dry season | 0.363 | 0.234 | 0.199 | 0.363 | −0.178 | −0.459* | 0.537** | 1 | 0.671** |
wet season | 0.144 | 0.141 | 0.210 | 0.117 | −0.175 | −0.217 | 0.415* | 1 | 0.378 | |
Turbidity | dry season | 0.497* | 0.397* | 0.445* | 0.415* | −0.432* | −0.732** | 0.648** | 0.671** | 1 |
wet season | 0.288 | 0.256 | 0.549** | 0.478* | −0.444* | −0.315 | 0.674** | 0.378 | 1 |
* significant Pearson correlation
![]() |
AMX = Amoxicillin, AMC = Amoxicillin/Clavulanic Acid, PIP = Piperacillin, TZP = Piperacillin/Tazobactam, TIC = Ticarcillin, TIM = Ticarcillin/Clavulanic Acid, IPM = Imipenem, ETP = Ertapenem, ATM = Aztreonam, CXM = Cefuroxime, FOX = Cefoxitin, CTX = Cefotaxime, CRO = Ceftriaxone, CAZ = Ceftazidime, FEP = Cefepime, CFM = Cefixime, SMN = Streptomycin, TOB = Tobramycin, GEN = Gentamicin, AMK = Amikacin, CIP = Ciprofloxacin, TET = Tetracycline, SSS = Sulfonamide, SXT = Trimethoprim/Sulfamethoxazole, NAL = Nalidixic Acid, CHL = Chloramphenicol, TMP = Trimethoprim. NT = Non-Typeable. Dark blue = resistant, white = susceptible, green = intermediate, and red = present.
PCR target | Primer sequence (5′-3′) | Annealing Temperature (°C) | Length (bp) | Reference | ||
Phylogenetic | ChuA | chuA.1b | ATGGTACCGGACGAACCAAC | 59 | 288 | [27] |
chuA.2 | TGCCGCCAGTACCAAAGACA | |||||
yjaA | yjaA.1b | CAAACGTGAAGTGTCAGGAG | 59 | 211 | ||
yjaA.2b | AATGCGTTCCTCAACCTGTG | |||||
TspE4.C | TspE4C2.1 | CACTATTCGTAAGGTCATCC | 59 | 152 | ||
TspE4C2.2b | AGTTTATCGCTGCGGGTCGC | |||||
arpA | AceK.f | AACGCTATTCGCCAGCTTGC | 59 | 400 | ||
ArpA1.r | TCTCCCCATACCGTACGCTA | |||||
β-lactam resistance | blaTEM | TemA1 | ATAAAATTCTTGAAGAC | 42 | 1075 | [16] |
TemB1 | TTACCAATGCTTAATCA | |||||
blaSHV | SHV-F | CACTCAAGGATGTATTGTG | 49 | 822 | ||
SHV-R | TTAGCGTTGCCAGTGCTCG | |||||
blaCTX-M | CTX-C1 | ATGTGCAGCACCAGTAAAGT | 54 | 545 | ||
CTX-C2 | ACCGCGATATCGTTGGTGG | |||||
blaOXA | OXA-F | ACACAATACATATCAACTTCGC | 61 | 813 | ||
OXA-R | AGTGTGTTTAGAATGGTGATC | |||||
blaCTX-M-15 | F | CACACGTGGAATTTAGGGACT | 50 | 995 | [28] | |
R | GCCGTCTAAGGCGATAAACA | |||||
Carbapenem resistance | blaOXA-48 | F | GCTTGATCGCCCTCGATT | 60 | 238 | [29] |
R | GATTTGCTCCGTGGCCGAAA |
Total Coliforms CFU/100 mL |
Fecal Coliforms CFU/100 mL |
Enterococci CFU/100 mL |
E. coli CFU/100 mL |
pH | DO mg/L |
BOD 5 mg/L |
Temperature (°C) |
Turbidity NTU |
|
S1 | 1.9 × 101 ± 1.7 × 101 | 5.4 ± 0.58 | 0 ± 0 | 5.0 ± 4.2 | 7.9 ± 0.1 | 10.9 ± 0.5 | 0.8 ± 0.4 | 14.5 ± 5.8 | 0.06 ± 0.01 |
S2 | 1.6 × 102 ± 2.4 × 102 | 10.25 ± 9.74 | 1.25 ± 1.9 | 1.4 ± 1.7 × 101 | 7.8 ± 0.3 | 10.9 ± 0.3 | 1.5 ± 0.9 | 14.9 ± 4.5 | 0.56 ± 0.24 |
S3 | 3.5 × 101 ± 3.3 × 101 | 9.8 ± 6.9 | 4.3 ± 4.3 | 1.6 × 101 ± 1.8 × 101 | 8 ± 0.3 | 10.4 ± 1.7 | 1.8 ± 1.5 | 17.9 ± 2.7 | 0.6 ± 0.3 |
S4 | 3.6 × 101 ± 3.9 × 101 | 7.5 ± 6 | 3.8 ± 4.3 | 8 ± 8.5 | 7.9 ± 0.3 | 10.6 ± 2 | 1.9 ± 1.5 | 16.7 ± 3.3 | 0.56 ± 0.23 |
S5 | 1.4 × 103 ± 1.2 × 103 | 82 ± 36.2 | 4.5 × 101 ± 3.6 × 101 | 5 × 102 ± 4.5 × 102 | 7.9 ± 0.4 | 10.6 ± 2.5 | 1.3 ± 1 | 14.9 ± 4.6 | 1.05 ± 0.57 |
S6 | 9.9 × 103 ± 6.0 × 103 | 4.1 × 103 ± 4.1 × 103 | 1.2 × 102 ± 7.5 × 101 | 4 × 103 ± 3.7 × 103 | 7.6 ± 0.3 | 10.2 ± 1.3 | 1 ± 0.4 | 15.9 ± 4.1 | 0.94 ± 0.37 |
S7 | 5.7 × 103 ± 5.0 × 103 | 4.6 × 103 ± 2.5 × 103 | 3.2 × 102 ± 3.4 × 102 | 4 × 103 ± 4.1 × 103 | 7.7 ± 0.2 | 10.7 ± 1.5 | 1 ± 0.4 | 17.6 ± 2.1 | 1.43 ± 0.59 |
S8 | 1.0 × 104 ± 5.9 × 103 | 7.9 × 103 ± 1.7 × 103 | 2.2 × 102 ± 2 × 102 | 5.4 × 103 ± 3 × 103 | 7.7 ± 0.3 | 10.5 ± 0.9 | 1 ± 0.6 | 17.5 ± 3 | 1.5 ± 0.5 |
S9 | 2.1 × 104 ± 8.7 × 103 | 4.6 × 103 ± 4.2 × 103 | 4.3 × 102 ± 5.2 × 102 | 1.1 × 103 ± 6.8 × 103 | 7.8 ± 0.3 | 10.5 ± 0.5 | 1.1 ± 0.8 | 17.9 ± 3 | 1.5 ± 0.54 |
S10 | 1.6 × 104 ± 1.3 × 104 | 5.7 × 103 ± 5.4 × 103 | 2 × 102 ± 1.5 × 102 | 3.4 × 103 ± 2.2 × 103 | 7.8 ± 0.1 | 10.9 ± 1.3 | 1.9 ± 1.1 | 17.5 ± 3.8 | 1.57 ± 0.42 |
S11 | 4.3 × 104 ± 3.9 × 104 | 2.4 × 104 ± 2 × 103 | 1.3 × 103 ± 1.5 × 103 | 2.4 × 104 ± 2.4 × 104 | 7.9 ± 0.1 | 10.6 ± 1.5 | 1.5 ± 0.6 | 17.8 ± 3.9 | 1.74 ± 0.12 |
S12 | 1.0 × 105 ± 9.8 × 104 | 3.4 × 104 ± 4.7 × 104 | 6.1 × 10 ± 5.5 × 103 | 5.8 × 104 ± 5.9 × 104 | 7.9 ± 0.2 | 10.4 ± 0.8 | 1.7 ± 0.3 | 16.3 ± 4.1 | 2.36 ± 0.5 |
S13 | 2.0 × 105 ± 2.1 × 105 | 4.5 × 104 ± 4.3 × 104 | 6 × 103 ± 4.9 × 103 | 1.8 × 104 ± 1.5 × 104 | 7.9 ± 0.2 | 10.4 ± 1.2 | 1.2 ± 0.3 | 17 ± 3.9 | 2.36 ± 0.6 |
S14 | 3.0 × 105 ± 3.5 × 105 | 5.4 × 104 ± 5.3 × 104 | 5.9 × 103 ± 4.9 × 103 | 2 × 104 ± 2.5 × 104 | 8 ± 0.5 | 10.4 ± 2 | 2.5 ± 1.3 | 17.8 ± 4.4 | 2.39 ± 0.7 |
S15 | 1.0 × 106 ± 6.3 × 105 | 1.4 × 105 ± 5.1 × 104 | 2.6 × 104 ± 2.1 × 104 | 3.8 × 104 ± 2 × 104 | 7.7 ± 0.2 | 9.1 ± 3.2 | 4.4 ± 1.5 | 19.4 ± 3.4 | 3.69 ± 1.65 |
S16 | 1.5 × 105 ± 1.1 × 105 | 2.1 × 104 ± 3.3 × 104 | 2.9 × 104 ± 2.7 × 104 | 1.1 × 104 ± 8.7 × 104 | 7.7 ± 0.2 | 9.6 ± 0.6 | 3.8 ± 1.8 | 17.8 ± 2.3 | 7.69 ± 5.15 |
S17 | 8.9 × 105 ± 9.1 × 105 | 9.3 × 104 ± 8.7 × 104 | 4.5 × 104 ± 4.5 × 104 | 3 × 104 ± 2.5 × 104 | 7.8 ± 0.1 | 8.1 ± 1.6 | 4.3 ± 0.8 | 17.6 ± 3.7 | 6.15 ± 3.25 |
S18 | 2.4 × 106 ± 1.7 × 106 | 3.6 × 105 ± 3.7 × 105 | 7.8 × 104 ± 6.8 × 104 | 1.2 × 105 ± 7.8 × 105 | 7.9 ± 0.4 | 7.1 ± 3.3 | 5.7 ± 1.7 | 17.5 ± 4.5 | 4.94 ± 1.97 |
S19 | 3.4 × 106 ± 3.2 × 106 | 1.2 × 106 ± 1.4 × 106 | 9.3 × 104 ± 8 × 104 | 2.4 × 105 ± 2.6 × 105 | 7.9 ± 0.4 | 7.8 ± 2.2 | 4.5 ± 1.4 | 16.4 ± 3.7 | 5.07 ± 2.2 |
S20 | 5.3 × 106 ± 7.8 × 106 | 1.6 × 106 ± 2.1 × 106 | 1.3 × 105 ± 1.2 × 105 | 3.4 × 105 ± 4.6 × 105 | 7.9 ± 0.4 | 8.6 ± 1.6 | 5.8 ± 1.2 | 17.9 ± 3.4 | 5.07 ± 2.92 |
S21 | 3.3 × 106 ± 5.5 × 106 | 2.1 × 105 ± 2.1 × 105 | 5.1 × 104 ± 3.4 × 104 | 2.9 × 105 ± 4.9 × 105 | 7.6 ± 0.3 | 8.7 ± 3.6 | 5.1 ± 1.1 | 16.4 ± 3.7 | 5.29 ± 2.53 |
S22 | 1.7 × 106 ± 1.9 × 106 | 2.1 × 105 ± 1.1 × 105 | 4.2 × 104 ± 2.7 × 104 | 1.5 × 105 ± 2.1 × 105 | 7.7 ± 0.1 | 8.5 ± 2 | 4.3 ± 1.3 | 17.6 ± 3.8 | 6.74 ± 2.26 |
S23 | 1.3 × 106 ± 8.4 × 105 | 2.5 × 105 ± 1 × 105 | 3.5 × 104 ± 1.1 × 104 | 1.7 × 105 ± 1.8 × 105 | 7.8 ± 0.3 | 8.4 ± 1 | 5.5 ± 1 | 18.9 ± 4.2 | 9.17 ± 3.89 |
S24 | 1.3 × 106 ± 4.5 × 105 | 3.3 × 105 ± 2.7 × 105 | 3.9 × 104 ± 3.3 × 104 | 6 × 104 ± 2.4 × 104 | 7.7 ± 0.1 | 8.8 ± 0.6 | 4.5 ± 1.1 | 18.1 ± 4.1 | 11.36 ± 2.81 |
S25 | 1.1 × 106 ± 5.5 × 105 | 3.7 × 105 ± 4.2 × 105 | 5.1 × 104 ± 5.9 × 104 | 6.2 × 104 ± 3.7 × 104 | 7.6 ± 0.2 | 9.5 ± 0.9 | 4.8 ± 1.8 | 18.7 ± 4.6 | 18.86 ± 11.01 |
Total Coliforms | Fecal Coliforms | Enterococci | E. coli | pH | DO | BOD 5 | Temperature | Turbidity | ||
Total coliforms | dry season | 1 | 0.940** | 0.757** | 0.895** | −0.368 | −0.646** | 0.766** | 0.363 | 0.497* |
wet season | 1 | 0.862** | 0.786** | 0.807** | 0.142 | −0.674** | 0.719** | 0.144 | 0.288 | |
Fecal coliforms | dry season | 0.940** | 1 | 0.878** | 0.844** | −0.394 | −0.569** | 0.669** | 0.234 | 0.397* |
wet season | 0.862** | 1 | 0.755** | 0.813** | 0.289 | −0.444* | 0.539** | 0.141 | 0.256 | |
Enterococci | dry season | 0.757** | 0.878** | 1 | 0.729** | −0.511** | −0.566** | 0.717** | 0.199 | 0.445* |
wet season | 0.786** | 0.755** | 1 | 0.773** | 0.178 | −0.800** | 0.875** | 0.210 | 0.549** | |
E. coli | dry season | 0.895** | 0.844** | 0.729** | 1 | 0.704** | −0.596** | 0.760** | 0.363 | 0.415* |
wet season | 0.807** | 0.813** | 0.773** | 1 | 0.715** | −0.705** | 0.736** | 0.117 | 0.478* | |
pH | dry season | −0.368 | −0.394 | −0.511** | 0.704** | 1 | 0.598** | −0.487* | −0.178 | −0.432* |
wet season | 0.142 | 0.289 | 0.178 | 0.715** | 1 | −0.158 | −0.037 | −0.175 | −0.444* | |
DO | dry season | −0.646** | −0.569** | −0.566** | −0.596** | 0.598** | 1 | −0.789** | −0.459* | −0.732** |
wet season | −0.674** | −0.444* | −0.800** | −0.705** | −0.158 | 1 | −0.783** | −0.217 | −0.315 | |
BOD 5 | dry season | 0.766** | 0.669** | 0.717** | 0.760** | −0.487* | −0.789** | 1 | 0.537** | 0.648** |
wet season | 0.719** | 0.539** | 0.875** | 0.736** | −0.037 | −0.783** | 1 | 0.415* | 0.674** | |
Tem | dry season | 0.363 | 0.234 | 0.199 | 0.363 | −0.178 | −0.459* | 0.537** | 1 | 0.671** |
wet season | 0.144 | 0.141 | 0.210 | 0.117 | −0.175 | −0.217 | 0.415* | 1 | 0.378 | |
Turbidity | dry season | 0.497* | 0.397* | 0.445* | 0.415* | −0.432* | −0.732** | 0.648** | 0.671** | 1 |
wet season | 0.288 | 0.256 | 0.549** | 0.478* | −0.444* | −0.315 | 0.674** | 0.378 | 1 |
![]() |