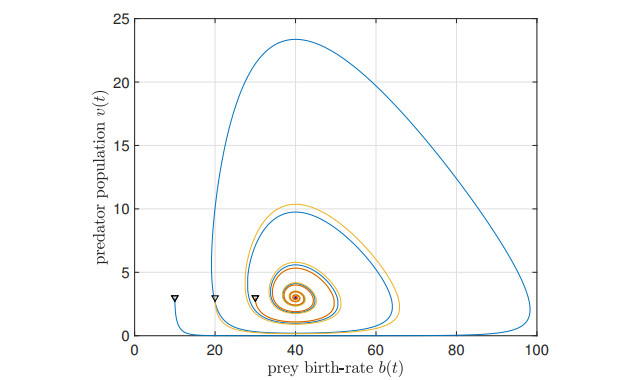
We study the impact of an age-dependent interaction in a structured predator-prey model. We present two approaches, the PDE (partial differential equation) and the renewal equation, highlighting the advantages of each one. We develop efficient numerical methods to compute the (un)stability of steady-states and the time-evolution of the interacting populations, in the form of oscillating orbits in the plane of prey birth-rate and predator population size. The asymptotic behavior when species interaction does not depend on age is completely determined through the age-profile and a predator-prey limit system of ODEs (ordinary differential equations). The appearance of a Hopf bifurcation is shown for a biologically meaningful age-dependent interaction, where the system transitions from a stable coexistence equilibrium to a collection of periodic orbits around it, and eventually to a stable limit cycle (isolated periodic orbit). Several explicit analytical solutions are used to test the accuracy of the proposed computational methods.
Citation: Jordi Ripoll, Jordi Font. Numerical approach to an age-structured Lotka-Volterra model[J]. Mathematical Biosciences and Engineering, 2023, 20(9): 15603-15622. doi: 10.3934/mbe.2023696
[1] | Lijun Wang, Chuanjun Dai, Min Zhao . Hopf bifurcation in an age-structured prey-predator model with Holling Ⅲ response function. Mathematical Biosciences and Engineering, 2021, 18(4): 3144-3159. doi: 10.3934/mbe.2021156 |
[2] | Toshikazu Kuniya, Hisashi Inaba . Hopf bifurcation in a chronological age-structured SIR epidemic model with age-dependent infectivity. Mathematical Biosciences and Engineering, 2023, 20(7): 13036-13060. doi: 10.3934/mbe.2023581 |
[3] | Wanxiao Xu, Ping Jiang, Hongying Shu, Shanshan Tong . Modeling the fear effect in the predator-prey dynamics with an age structure in the predators. Mathematical Biosciences and Engineering, 2023, 20(7): 12625-12648. doi: 10.3934/mbe.2023562 |
[4] | Shunyi Li . Hopf bifurcation, stability switches and chaos in a prey-predator system with three stage structure and two time delays. Mathematical Biosciences and Engineering, 2019, 16(6): 6934-6961. doi: 10.3934/mbe.2019348 |
[5] | S. Nakaoka, Y. Saito, Y. Takeuchi . Stability, delay, and chaotic behavior in a Lotka-Volterra predator-prey system. Mathematical Biosciences and Engineering, 2006, 3(1): 173-187. doi: 10.3934/mbe.2006.3.173 |
[6] | Kento Okuwa, Hisashi Inaba, Toshikazu Kuniya . Mathematical analysis for an age-structured SIRS epidemic model. Mathematical Biosciences and Engineering, 2019, 16(5): 6071-6102. doi: 10.3934/mbe.2019304 |
[7] | Saheb Pal, Nikhil Pal, Sudip Samanta, Joydev Chattopadhyay . Fear effect in prey and hunting cooperation among predators in a Leslie-Gower model. Mathematical Biosciences and Engineering, 2019, 16(5): 5146-5179. doi: 10.3934/mbe.2019258 |
[8] | Peter A. Braza . Predator-Prey Dynamics with Disease in the Prey. Mathematical Biosciences and Engineering, 2005, 2(4): 703-717. doi: 10.3934/mbe.2005.2.703 |
[9] | Eric M. Takyi, Kasey Cooper, Ava Dreher, Caroline McCrorey . The (De)Stabilizing effect of juvenile prey cannibalism in a stage-structured model. Mathematical Biosciences and Engineering, 2023, 20(2): 3355-3378. doi: 10.3934/mbe.2023158 |
[10] | Feng Rao, Carlos Castillo-Chavez, Yun Kang . Dynamics of a stochastic delayed Harrison-type predation model: Effects of delay and stochastic components. Mathematical Biosciences and Engineering, 2018, 15(6): 1401-1423. doi: 10.3934/mbe.2018064 |
We study the impact of an age-dependent interaction in a structured predator-prey model. We present two approaches, the PDE (partial differential equation) and the renewal equation, highlighting the advantages of each one. We develop efficient numerical methods to compute the (un)stability of steady-states and the time-evolution of the interacting populations, in the form of oscillating orbits in the plane of prey birth-rate and predator population size. The asymptotic behavior when species interaction does not depend on age is completely determined through the age-profile and a predator-prey limit system of ODEs (ordinary differential equations). The appearance of a Hopf bifurcation is shown for a biologically meaningful age-dependent interaction, where the system transitions from a stable coexistence equilibrium to a collection of periodic orbits around it, and eventually to a stable limit cycle (isolated periodic orbit). Several explicit analytical solutions are used to test the accuracy of the proposed computational methods.
Continuously structured population models describe the dynamics of animal or human populations that vary continuously with respect to some underlying physiological variable, such as body size or age; see [1,2,3,4] and [5]. Interestingly, Hopf bifurcations are sudden changes in the dynamics that may occur in ecological models, leading to the emergence of complex oscillatory behavior in the population dynamics. Specifically, a Hopf bifurcation occurs when a stable population steady-state becomes unstable, giving rise to a stable limit cycle (isolated periodic orbit) in the dynamics; see, e.g., [6,7,8,9] and also [10,11,12,13].
The Lotka-Volterra model, originally developed in the early 20th century, is a classic mathematical model for the interaction between predator and prey populations; see the books [4,14,15,16]. In the age or size structured version of this model, age or size is explicitly incorporated as a key continuous variable, allowing for richer predator-prey dynamics. The model takes into account the fact that predators preferentially target certain ages of the preys, while preys may have different reproductive successes and survival probabilities at different ages; see the papers [17,18,19]. We approach the predator-prey problem using two different formulations: the partial differential equation (PDE) approach and the renewal equation approach, [1,4,20]. Renewal equations have been used to study a wide range of phenomena in population dynamics, from the spread of infectious diseases to the dynamics of predator-prey relationships. We will analyze a non-linear renewal equation for the birth-rate of the prey population, describing how the current number of newborn preys per unit of time changes over time, based on past newborn preys and the number of predators.
On the other hand, efficient numerical methods are computational techniques designed to solve mathematical problems quickly and accurately; see the book [21], and the recent papers [22,23,24,25] and also [26,27,28]. We checked the accuracy of the methods by using available analytical solutions, and we used them to uncover the behavior of the interacting populations for the cases where analytical results are not possible.
In the present paper, we consider structured population dynamics for two interacting species where age or size of the individuals has an important impact on the dynamics. If we consider size x≥x0 (e.g., body length) as structuring variable instead of (chronological) age a≥0, under the assumptions that individuals are born at the same size x0 and the individual growth rate g is a function of size, that is, dxdt=g(x), x(0)=x0, then the size-dependent problem can be reduced to an age-dependent problem. The latter is achieved by making the change of variables a=∫xx0dsg(s), as it is well known. So, we deal with an age-structured model but keep in mind that it represents a reducible size-structured model, which is a particular case of physiologically structured populations; see [1,3,22]. Specifically, we consider the following structured predator-prey model (first-order hyperbolic system of nonlocal partial differential equations with nonlocal boundary conditions):
{∂tu(a,t)+∂au(a,t)=−(μ(a)+γ(a)∫∞0v(a,t)da)u(a,t)∂tv(a,t)+∂av(a,t)=(α∫∞0γ(a)u(a,t)da−m)v(a,t)u(0,t)=∫∞0β(a)u(a,t)da,v(0,t)=b∫∞0v(a,t)da,δ:=m−b>0, | (1.1) |
where the first equation is for the dynamics of the prey age-density with mortality μ and species interaction γ, the second one is for the dynamics of the predator age-density with mortality m and predator efficiency α, and the third one gives the newborns per unit of time of preys and predators with fertility rates β and b, respectively. Integrating the second equation over the age span, we can reduce the system and deal with the predator population size instead of its age-density.* In this model, one population (the predator/consumer) feeds on the other population (the prey/resource), and the interactions between the two populations can have important effects on their respective dynamics. Finally, let us mention that the spatial distribution of the interacting populations is neglected.
* With an abuse of notation, in the following, v will stand for the population size of predators instead of its age-density.
When dealing with interacting species, age or size of the individuals of the populations involved, has been identified as one of the key features for the joint evolution; see [17,18,19]. Let us consider the population dynamics for the interaction of predators and preys with age structure in the prey population. As explained in the introduction, we use age instead of size for simplicity, and we are implicitly assuming that the age structure of the predator population has a small impact on the dynamics. So, let u(a,t) be the density of the prey population with respect to the age a≥0 at time t≥0, and let v(t) be the total population of predators at time t≥0. The structured predator-prey model of Lotka-Volterra type in X=L1(R+)×R reads as:
{∂tu(a,t)+∂au(a,t)=−(μ(a)+γ(a)v(t))u(a,t)v′(t)=(α∫∞0γ(a)u(a,t)da−δ)v(t)u(0,t)=∫∞0β(a)u(a,t)da,t≥0, | (2.1) |
with suitable non-negative initial conditions u0(a),v0 at time t=0. Model parameters are: predator per capita mortality rate δ>0 (actually, it is a balance between fertility and mortality in the absence of preys), ingestion coefficient 0<α<1 (a measure of the efficiency of the predator) and age-specific per capita rates μ,β,γ∈L∞+(R+) as prey mortality [1/time], prey fertility [1/time] and species interaction [1/(time ⋅ ind)], respectively. Moreover, we will make the following specific assumptions:
● The age-specific mortality rate is bounded from below: μ(a)≥μ0>0, i.e., there is always a minimum mortality. In the absence of interaction between species, survival probabilities for preys and predators are e−∫a0μ(s)ds and e−ma, for some m>δ, respectively.
● The age-specific interaction rate is such that γ(a)>0 in a neighborhood of a=0, i.e., the interaction between species always takes place for the younger preys.
● We take the basic reproduction number (see, e.g., [29,30]) for the prey population, in the absence of predators, such that:
R0=∫∞0β(a)e−∫a0μ(s)dsda>1, | (2.2) |
meaning that the prey population by itself has asynchronous exponential growth. In other words, the equation for the prey population in the absence of the predators, ∂tu(a,t)+∂au(a,t)=−μ(a)u(a,t), u(0,t)=∫∞0β(a)u(a,t)da, has asynchronous exponential growth with positive Malthusian parameter r>0; see [1,3,5,31].
● The system (2.1) can be also considered in finite age-span [0,a†] with suitable assumptions on the mortality rate (unbounded case) in order to avoid (or minimize the number of) immortal individuals.
As is well known, the original Lotka-Volterra model was an ODE model for the total population of preys and predators, providing an explanation for the periodic dynamics of fish populations observed in the Adriatic sea; see the books [15,16]. The system (2.1) along with the assumptions above, can be cast into an abstract semilinear differential equation in X=L1(R+)×R, where the linear part is the infinitesimal generator of a strongly continuous semigroup of positive linear operators. We have that there exists a positive mild solution to (2.1) which is continuous in time; see [18,19,32] for the details. For the theory of semigroups applied to population dynamics; see [33].
Previous works on system (2.1) have focused on the PDE formulation. However, here we will focus on the so-called renewal formulation, which has some advantages, and we will develop efficient numerical methods when analytical results are not available.
The novelty of this paper lies in the following: Regarding the age-structured Lotka-Volterra model, we introduce a pseudospectral numerical method to compute the (un)stability of the coexistence steady-state and a predictor-corrector numerical scheme to simulate the population dynamics of predators and preys. In addition, we have uncovered exact solutions of the steady-state and the long-term asymptotic population dynamics to check the accuracy of the aforementioned computational methods, when either the age-dependent parameters are proportional or species interaction is age-independent. For the latter, we determine the asymptotic age-profile of the preys, which depends on the Malthusian parameter. Finally, for a biologically meaningful age-dependent interaction, the rightmost complex-conjugate spectral values cross the imaginary axis with positive speed, showing a Hopf bifurcation of the steady-state of the predator-prey system.
In this section, we are going to introduce a new formulation for the present predator-prey model, which simplifies the mathematical analysis. First of all, the system (2.1) can be written equivalently in integrated form as follows. Using the integration along characteristic lines in the partial differential equation (PDE) and the variation of the constants formula in the ordinary differential equation (ODE), we get to
u(a,t)={u0(a−t)e−∫t0μ(s+a−t)+γ(s+a−t)v(s)dsa≥tu(0,t−a)e−∫a0μ(s)+γ(s)v(s+t−a)dsa<t | (3.1) |
v(t)=v0e−δt+α∫t0∫∞0γ(a)u(a,s)dads,t≥0, | (3.2) |
with u(0,t−a)=∫∞0β(s)u(s,t−a)ds, a<t. Moreover, using (2.1) and (3.1) we can get a non-linear renewal equation. Indeed, defining the new variable b(t):=u(0,t) as the birth rate of the prey population (number of newborns per unit of time), we get to the renewal formulation of the present predator-prey system:
{b(t)=∫∞0β(a)e−∫a0μ(s)+γ(s)v(s+t−a)ds⋅b(t−a)dav′(t)=(α∫∞0γ(a)e−∫a0μ(s)+γ(s)v(s+t−a)ds⋅b(t−a)da−δ)v(t),t≥0, | (3.3) |
where instead of assuming a known initial condition at time t=0, we assume a known history in (−∞,0] for the birth rate of the prey population, i.e., b(τ)=ϕ(τ),τ≤0, and for the predator population v(τ)=ψ(τ),τ≤0. The first history is a non-negative locally integrable function, and the second one is a non-negative continuous function.
Interestingly, we can take advantage of this renewal equation for (b(t),v(t)), t≥0, when computing the steady-states and their linear stability— see Sections 3.1 and 5. Moreover, non-linear renewal equations like (3.3) can be readily extended to more general structured models of Lotka-Volterra type where the prey is structured by size (rather than age), and the individual growth rate and vital rates are density-dependent. See, e.g., [20] for size-structured consumer resource models.
Under the current assumptions given in Section 2, in particular, basic reproduction number (2.2) R0>1, there always exists both the trivial equilibrium of system (3.3), b∗=0, v∗=0, and the coexistence equilibrium b∗>0, v∗>0. Indeed, steady-states of (3.3) are given by:
{b∗=∫∞0β(a)e−∫a0μ(s)+γ(s)v∗dsda⋅b∗0=(α∫∞0γ(a)e−∫a0μ(s)+γ(s)v∗dsda⋅b∗−δ)v∗ | (3.4) |
Therefore, the predator population at equilibrium v∗>0 is the unique (real) solution of the non-linear equation:
1=∫∞0β(a)e−∫a0μ(s)+γ(s)v∗dsda<R0. | (3.5) |
Notice that the equation above is independent of parameters α and δ and involves the decreasing function F(a):=−∫a0γ(s)ds, a≥0. If γ∈L1(R+) then we can consider its mean value F(0)+F(∞)2=−12∫∞0γ(s)ds, to get an approximation of the solution (3.5) as:
v∗≃2ln(R0)∫∞0γ(s)ds. |
Once we have v∗>0 from (3.5), the birth rate of the prey population at equilibrium is computed from the second equation in (3.4) as
b∗=δα(∫∞0γ(a)e−∫a0μ(s)+γ(s)v∗dsda)−1>δαv∗. | (3.6) |
Moreover, the age density of the prey population at equilibrium is readily recovered from (3.1) as u∗(a)=b∗e−∫a0μ(s)+γ(s)v∗ds, a≥0, using (3.5)–(3.6). For an analogous computation of the steady-states using the PDE formulation and relaxing the assumption of R0>1, see [19].
For numerical purposes, see Sections 5 and 6, we can consider a non-trivial case with explicit solution to the coexistence equilibrium of (3.4). Namely, the age-dependent proportional case, see, e.g., [24]: generic age-specific mortality μ(a), fertility rate as β(a)=ˉβμ(a) with ˉβ>1 and interaction rate as γ(a)=ˉγμ(a), ˉγ>0. In this case we have that the basic reproduction number (2.2) is R0=ˉβ>1 and the explicit solution to (3.5)–(3.6) is v∗=ˉβ−1ˉγ>0 and b∗=δˉβαˉγ>0, respectively. In this particular case, the prey age-density at equilibrium is given by u∗(a)=b∗e−ˉβ∫a0μ(s)ds; see Figure 1 for an illustration with an age-specific mortality rate.
In this section we study the asymptotic behavior of both formulations, the PDE (2.1) and the renewal equation (3.3), through the analysis of the age-profile (i.e., normalized age-density) of the prey population.
Let the total prey population be denoted by P(t)=∫∞0u(a,t)da and let us assume for a while that the prey age-profile u(a,t)P(t) reaches a stationary age-profile ˉω(a), a≥0, with ∫∞0ˉω(a),da=1. In symbols,
limt→∞u(a,t)P(t)=ˉω(a),a≥0,(point-wise or uniformly in age). | (4.1) |
In other words, we are assuming that there exists the asymptotic age-profile for the prey population, which is independent of the initial populations.
Next, we can integrate over the age span in (2.1), dPdt=∫∞0[β(a)−μ(a)−γ(a)v(t)]u(a,t)P(t)da⋅P(t), and under the assumption above, we get to the following 2-dimensional Lotka-Volterra limit system:
{dPdt=⟨β−μ⟩ˉω⋅P−⟨γ⟩ˉω⋅vPdvdt=α⟨γ⟩ˉω⋅vP−δv,t≥0, | (4.2) |
where the brackets above ⟨⋅⟩ˉω stand for a weighted mean over the age span:
⟨ϕ⟩ˉω:=∫∞0ϕ(a)ˉω(a)da. |
This limit system is the classical predator-prey ODE Lotka-Volterra model for the sizes of the interacting populations, so the solutions (P(t),v(t)), t≥0, describe closed periodic orbits around the coexistence equilibrium
P∗=δα⟨γ⟩ˉω,v∗=⟨β−μ⟩ˉω⟨γ⟩ˉω | (4.3) |
with period and amplitude of oscillation depending on each initial condition (P0,v0) of (4.2). Taking initial conditions such that u0(a)/P0=ˉω(a), solutions of (4.2) are implicitly given, as expected, by (vv0)v∗⋅(PP0)αP∗=exp[v−v0+α(P−P0)] with period of oscillation starting at 2π√δ⟨β−μ⟩ˉω=2π√δv∗⟨γ⟩ˉω, i.e., the linearization of (4.2) around the equilibrium (4.3) has pure imaginary eigenvalues λ=±√δv∗⟨γ⟩ˉωi. See, for instance, [14,15,16]. So, the asymptotic behavior of (2.1) can be determined by the existence of the asymptotic age-profile ˉω(a) and the dynamics of the limit system (4.2).
Moreover, from (4.1) we have that
limt→∞b(t)P(t)=limt→∞u(0,t)P(t)=ˉω(0). |
So, b(t)∼ˉω(0)P(t) for large t>0, and the asymptotic behavior of the prey birth-rate b(t) and the predator population v(t) is implicitly given by
(vv0)v∗⋅(bb0)αP∗=exp[v−v0+αˉω(0)(b−b0)] | (4.4) |
with compatible initial conditions b0=ˉω(0)P0. The prey birth-rate at equilibrium is given by
b∗=δα⟨γ⟩ˉωˉω(0). | (4.5) |
So, again, the asymptotic behavior of (3.3) can be determined by the existence of the asymptotic age-profile ˉω(a) and equation (4.4).
Finally, we need to find conditions to assure the existence of the asymptotic age-profile. Taking the approach of section 2.6 in [1], we can write the system for the prey age-profile ω(a,t):=u(a,t)P(t), ∫∞0ω(a,t)da=1, t≥0, which is in general coupled with the predator and prey population sizes. Indeed, from (2.1) we get:
{∂tω(a,t)+∂aω(a,t)=−[μ(a)+γ(a)v(t)+λ(t)]ω(a,t),∫∞0ω(a,t)da=1ω(0,t)=∫∞0β(a)ω(a,t)da,λ(t):=∫∞0[β(a)−μ(a)−γ(a)v(t)]ω(a,t)daP′(t)=λ(t)P(t)v′(t)=(α∫∞0γ(a)ω(a,t)da⋅P(t)−δ)v(t),t≥0, | (4.6) |
with compatible and non-trivial (in the sense of [1], Chapter 2) initial conditions at time t=0 as ω0(a)=u0(a)/P0, P0=∫∞0u0(a)da>0 and v0>0, where u0(a) is the initial condition of (2.1). The situation where the support of the fertility rate β(a) lies to the left of the support of the initial age-density u0(a) is excluded in here, as in [1].
It is worth to mention that λ(t) defined in (4.6) is seen as a time-dependent Malthusian parameter for the preys but depending on the predator population. However, we realize from the first equation in (4.6) that the prey age-profile is independent of the predator population if and only if the interaction rate is age-independent, γ(a)≡ˉγ, a>0. Indeed, the interaction term is
[γ(a)v(t)−∫∞0γ(a)v(t)ω(a,t)da]ω(a,t)=[γ(a)−∫∞0γ(a)ω(a,t)da]v(t)ω(a,t)≡0⇔γ(a)≡ˉγ. |
Therefore, when interaction between preys and predators is age-independent, system (4.6) is uncoupled, and its asymptotic behavior is completely determined as follows:
Theorem 1 (Asymptotic behavior). Under the specific assumptions given in Section 2, if in addition γ(a)≡ˉγ, then the solution of (4.6) with non-trivial initial conditions (in the sense of Chapter 2 in [1]) is such that
limt→∞∫∞0|ω(a,t)−ˉω(a)|=0withˉω(a)=exp[−∫a0μ(s)ds−ra]∫∞0exp[−∫a0μ(s)ds−ra]da, |
where r>0 is the unique real solution to the non-linear equation 1=∫∞0β(a)e−∫a0μ(s)ds−rada<R0. Moreover, for large t>0 and a suitable constant c>0, P(t) and v(t) in (4.6) are implicitly given by the equation
vr⋅Pδ=ceˉγ(v+αP), |
describing closed periodic orbits around the steady-state P∗=δαˉγ, v∗=rˉγ.
Proof. For γ(a)≡ˉγ, the equations in (4.6) for the age-profile are uncoupled from the others:
{∂tω(a,t)+∂aω(a,t)=−[μ(a)+∫∞0[β(a)−μ(a)]ω(a,t)da]ω(a,t),ω(0,t)=∫∞0β(a)ω(a,t)da,∫∞0ω(a,t)da=1. |
Since we are assuming a non-trivial initial condition, we can apply Theorem 2.10 in [1] to assure that the asymptotic age-profile exists, and it is given by ˉω(a)=exp[−∫a0μ(s)ds−ra]∫∞0exp[−∫a0μ(s)ds−ra]da, where r is the unique real solution to 1=∫∞0β(a)e−∫a0μ(s)ds−rada. Moreover, r>0 since R0>1. So, the first part of the statement follows.
On the other hand, the equations in (4.6) for P(t) and v(t) are influenced by the age-profile:
{P′(t)=[∫∞0[β(a)−μ(a)]ω(a,t)da−ˉγv(t)]P(t)v′(t)=[αˉγP(t)−δ]v(t) | (4.7) |
Once we know the evolution of the age-profile, it is straightforward to see that
limt→∞∫∞0[β(a)−μ(a)]ω(a,t)da=∫∞0[β(a)−μ(a)]ˉω(a)da=r>0. |
Finally, if we take initial condition ω0(a)=ˉω(a) then (4.7) coincides with (4.2); otherwise (4.2) plays the role of limit system. Accordingly, the asymptotic behavior of (P(t),v(t)) in (4.6) is given by the solutions of (4.2) with ⟨β−μ⟩ˉω=r and ⟨γ⟩ˉω=ˉγ. As is well known, these are periodic orbits around the steady-state (P∗,v∗)=(δαˉγ,rˉγ), implicitly given by vv∗⋅PαP∗=c0ev+αP, c0>0, or equivalently vr⋅Pδ=cˉγ0eˉγ(v+αP), and the second part of the statement follows.
Notice that r>0 in the theorem above is the Malthusian parameter (exponential growth rate) of the preys in the absence of predators, and ˉω(a) is their asymptotic age-profile. Let us recall the well-known relationship with the basic reproduction number (2.2), sign(r)=sign(R0−1). For the computation of the basic reproduction number in structured models from the analytical and numerical points of view; see, e.g., [23,24,29,30].
Under the assumptions of Theorem 1, we can recover the asymptotic behavior of both systems (2.1) and (3.3). Namely, for large t>0, the prey age-density is u(a,t)∼P(t)ˉω(a), a≥0, and the prey birth-rate is b(t)∼P(t)ˉω(0), with P(t) and v(t) population sizes of preys and predators oscillating periodically in time. See Figure 2 for an illustration of the closed curves (4.4) oscillating around the steady-state for the constant interaction case. One can obtain similar pictures for the periodic orbits by changing μ(a) and β(a) while keeping the interaction γ as age-independent.
On the contrary, when interaction is age-dependent actually, γ(a)≢ ct., the unique stationary age-profile is then the one corresponding to the coexistence equilibrium computed in Section 3.1:
ω∗(a)=u∗(a)∫∞0u∗(a)da=exp[−∫a0μ(s)+γ(s)v∗ds]∫∞0exp[−∫a0μ(s)+γ(s)v∗ds]da |
with v∗>0 the solution of (3.5). The latter is a consequence of the fact that the equation for the age-profile is not uncoupled from the equations for the population sizes.
In this section we study the linear stability of the steady states using the renewal formulation (3.3). In particular, an efficient numerical method is introduced to tackle the (un)stability of the coexistence equilibrium for a general age-dependent interaction rate γ(a). This method is based on the finite-dimensional approximation of a differential operator; see the book [21], and the recent papers [22,23,24,25] and also [26,27,28].
First of all, let us point out that the trivial steady state (extinction equilibrium) is unstable. More precisely, under the assumption of R0>1, (0,0) is always a saddle point in the predator-prey plane, since prey population has asynchronous exponential growth in absence of predators, b(t)∼b0ert, r>0, and predator population goes to extinction in absence of preys, v(t)=v0e−δt, δ>0.
Regarding the coexistence steady-state b∗>0, v∗>0 given by (3.5)–(3.6) in Section 3.1, we can linearize (3.3) to study the behavior of solutions around the equilibrium, especially for the age-dependent interaction case.
Defining new variables B(t)=b(t)−b∗, V(t)=v(t)−v∗, from (3.3) we get to the following linear renewal equation:
{B(t)=∫∞0β∗(a)[B(t−a)−b∗∫a0γ(s)V(s+t−a)ds]daV′(t)=v∗∫∞0γ∗(a)[B(t−a)−b∗∫a0γ(s)V(s+t−a)ds]da,t≥0, | (5.1) |
where, for convenience, new age-specific functions are defined
β∗(a):=β(a)e−∫a0μ(s)+γ(s)v∗dsandγ∗(a):=αγ(a)e−∫a0μ(s)+γ(s)v∗ds, | (5.2) |
with ∫∞0β∗(a)da=1 and ∫∞0γ∗(a)da=δb∗ using (3.5)–(3.6).
Next, putting B(t)=B0eλt, V(t)=V0eλt in (5.1) we get to the following eigenvalue problem, λ∈C and (B0,V0)∈C2∖{(0,0)}:
{B0=∫∞0β∗(a)e−λa[B0−b∗∫a0γ(s)eλsds⋅V0]daλV0=v∗∫∞0γ∗(a)e−λa[B0−b∗∫a0γ(s)eλsds⋅V0]da. | (5.3) |
For an analogous computation using the PDE formulation, see [19]. From this eigenvalue problem, one can readily see that λ=0 is never an eigenvalue since ∫∞0β∗(a)da=1.
Beyond the age-independent interaction case, already analyzed in Section 4, where (5.3) has an explicit solution as expected as λ=±√δv∗ˉγi, (B0,V0)=(−b∗ˉγ,λ), we focus on the age-dependent interaction case. However, instead of analyzing the characteristic equation for the eigenvalues, i.e. (5.3) as a 2-dimensional homogeneous linear system with determinant being equal to zero, we study the eigenvalues of the differential operator related to (5.3). More precisely, the right hand side of the system above has a common factor ϕ(a):=e−λa[B0−b∗∫a0γ(s)eλsds⋅V0] which is the variation of the constants formula for the inhomogeneous ODE ϕ′(a)+λϕ(a)=−b∗γ(a)V0, ϕ(0)=B0. Therefore, (5.3) is equivalent to the eigenvalue problem
{ϕ(0)=∫∞0β∗(a)ϕ(a)daλϕ(a)=−ϕ′(a)−b∗γ(a)V0λV0=v∗∫∞0γ∗(a)ϕ(a)da, | (5.4) |
Finally we have to study the linear differential operator A:dom(A)⊂X⟶X defined by
A(φy)=(−φ′−b∗γ(⋅)yv∗∫∞0γ∗(a)φ(a)da ),dom(A)={(φ,y)∈X:φ′∈L1(R+),φ(0)=∫∞0β∗(a)φ(a)da} | (5.5) |
whose eigenvalues / eigenfunctions, λ(ϕV0)=A(ϕV0), can be approximated by the eigenvalues / eigenvectors of a suitable matrix, using pseudospectral methods. See [21].
Let us remark that thanks to the minimum mortality assumption μ(a)≥μ0>0 and the characterization of the growth bound using spectral values (see [5], [3], [19]) we can focus on the spectral bound s(A) for the (un)stability of the coexistence equilibrium (b∗,v∗). Moreover, spectral values of A with real part larger than −μ0 are actually eigenvalues, i.e., solutions to system (5.4).
For the numerical implementation, we proceed as follows; see [24] and Chapter 3 in [1]. We consider polynomial interpolation for the functions in the age interval [0,a†] with a† the maximum age, ϕ(aj)≃Φj, with aj, j=0,…,N, being the Chebyshev extremal points and N being the discretization index. Let H be the differentiation matrix of dimension (N+1)×(N+1), and we consider Clenshaw-Curtis quadrature rule with weights over the interval [−1,1], wj, j=0,…,N, 12∑Nj=0wj=1. Then, the finite-dimensional counterpart of the eigenvalue problem (5.4) is as follows:
{Φ0=a†2N∑j=0β∗jΦjwji=0λΦi=−N∑j=0HijΦj−b∗γiV0i=1,…,NλV0=v∗a†2N∑j=0γ∗jΦjwji=N+1, | (5.6) |
where β∗j=β∗(aj) and γ∗j=γ∗(aj), functions defined in (5.2). Solving Φ0 from the first equation, we get to Φ0=a†2−β∗0w0a†∑Nj=1β∗jΦjwj, and plugging into the other equations, we can reduce the linear system to dimension N+1:
{λΦi=−Hi0a†2−β∗0w0a†N∑j=1β∗jΦjwj−N∑j=1HijΦj−b∗γiV0i=1,…,NλV0=v∗a†2γ∗0w0a†2−β∗0w0a†N∑j=1β∗jΦjwj+v∗a†2N∑j=1γ∗jΦjwji=N+1. | (5.7) |
From the right hand side above, we define the entries of the matrix AN of size (N+1)×(N+1) as
{Ai,j=−Hi,0C1β∗jwj−Hi,ji=1,…,Nj=1,…NAi,N+1=−b∗γii=1,…,Nj=N+1AN+1,j=v∗a†2(C2β∗j+γ∗j)wji=N+1j=1,…,NAN+1,N+1=0i=N+1j=N+1 | (5.8) |
with constants C1=a†2−β∗0w0a† and C2=γ∗0w0a†2−β∗0w0a†, which is a finite-dimensional approximation to the linear differential operator A defined in (5.5).
The finite-dimensional eigenvalue problem λ(ˆΦV0)=AN(ˆΦV0), with ˆΦ=(Φ1,…,ΦN), is solved either by Matlab's eig or eigs.
We can check the accuracy of the proposed pseudospectral method by using the exact solution to (5.4) for the case of age-independent interaction, namely, age-independent eigenfunctions corresponding to purely imaginary eigenvalues; see Figure 3. One readily sees from (5.4) that ϕ(a)≡1 if and only if γ(a)≡ˉγ. Numerically, one readily sees from (5.6) that Φj=1, j=0…N if and only if γi=ˉγ, i=0…N since ∑Nj=0Hij=0.
In this section we introduce a numerical method to compute the time-evolution of the interacting populations with the aim of checking the results obtained in previous sections. Among many numerical schemes (see chapter 7 in [1] and the references therein and also [19,34,35]) we work on the integrated form of the present predator-prey model (3.1)–(3.2), paying attention to avoid immortal individuals.
The starting point is to consider equal age and time step-sizes Δa=Δt to get to
u(a+Δt,t+Δt)={u(a,t)e−∫t+Δttμ(s+a−t)+γ(s+a−t)v(s)dsa≥tu(a,t)e−∫a+Δtaμ(s)+γ(s)v(s+t−a)dsa<t=u(a,t)e−∫a+Δtaμ(s)+γ(s)v(s+t−a)ds |
and (3.2) can be rewritten as
v(t+Δt)=v(t)e−δΔt+α∫t+ΔttS(s)dswith S(t)=∫∞0γ(a)u(a,t)da. | (6.1) |
We recall that b(t):=u(0,t)=∫∞0β(a)u(a,t)da, and lima→∞u(a,t)=0. Now, we can discretize age (in a finite span [0,a†], with a† as the maximal age) and time by the grid points {(aj,tn):0≤j≤J,0≤n≤N} with aj=jΔa, tn=nΔt, Δt=Δa=a†/J and use quadrature rules for the integrals involved: u(0,t)=∫∞0β(a)u(a,t)da≃∫a†0β(a)u(a,t)da≃∑Jj=0wjβ(aj)u(aj,t)Δa, with weights 1J∑Jj=0wj=1. Isolating, we get
u(0,t)≃Δa1−w0β(0)ΔaJ∑j=1wjβ(aj)u(aj,t) |
with Δa small enough such that w0β(0)Δa<1 in order to assure positivity of the numerical solution. On the other hand, we can consider two approximations in (6.1): v(t+Δt)≃v(t)e−δΔt+αS(t)Δt as predictor and v(t+Δt)≃v(t)e−δΔt+α[S(t)+S(t+Δt)]Δt/2 as corrector, both with S(t)≃∑Jj=0wjγ(aj)u(aj,t)Δa.
Finally, we get to the following predictor-corrector explicit numerical scheme for the approximation of the solution of (2.1) at the grid points u(aj,tn)≃unj, v(tn)≃vn, computed from a given initial condition u(aj,0)=u0(aj), v(0)=v0:
{˜vn+1=vnexp[Δt(−δ+αSn)]un+1j+1=unjexp[−Δt(μj+γjvn+μj+1+γj+1˜vn+1)/2],j=0,…,J−2un+10=Δt1−w0β0Δt∑J−1j=1wjβjun+1jSn+1=Δt∑J−1j=0wjγjun+1jvn+1=vnexp[Δt(−δ+α(Sn+Sn+1)/2)]n≥0 | (6.2) |
where μj=μ(aj), γj=γ(aj), we set u(a†,tn)≃unJ=0, and we have used the mid-point rule for the integrals of length Δt. In addition, we can compute the prey population size over time as
P(tn)≃ΔtJ−1∑j=0wjunj |
and the prey birth-rate b(tn)=u(0,tn)≃un0. Finally, we should choose maximal age a† and mortality rate μ(a) to avoid or minimize the probability of immortal individuals, that is, from (3.1) for t>a†, u(a†,t)u(0,t−a†)≤exp[−∫a†0μ(a)da] should be zero or small enough.
We can check the accuracy of the predictor-corrector numerical scheme (6.2) using the exact solution for the case of age-independent interaction, a closed periodic orbit; see Theorem 1. Taking an initial condition such that u0(a)=P0ˉω(a)>0, b0=P0ˉω(0)>0 and v0>0, we can compute the error as ‖(b0,v0)−(bT,vT)‖, where the point (bT,vT) is the closest point to the initial condition (b0,v0) after a single revolution around the steady state (b∗,v∗); see the inset plot in Figure 4 for an illustration.
In this section, we present several numerical experiments to investigate how the spectrum of the finite-dimensional operator, the matrix AN in (5.8), approximates the point spectrum and the spectral bound of the differential operator A, defined in (5.5), determining the (un)stability of the coexistence equilibrium.
Let us consider a more realistic case than the age-dependent proportional case (i.e., β(a)=ˉβμ(a) and γ(a)=ˉγμ(a)), by assuming a finite age-span [0,a†]} with unbounded mortality μ(a)=θa†−a, θ>0, to avoid immortality in the preys, and fertility β(a)=ˉβa(a†−a), ˉβ>0 with a maximum at an intermediate age. In this scenario, the computations of previous sections are slightly different. Indeed, the basic reproduction number is computed as R0=∫a†0β(a)(a†−aa†)θda>1, and the Malthusian parameter r>0 is the solution to 1=∫a†0β(a)(a†−aa†)θe−rada. Moreover, equation (3.5) becomes 1=∫a†0β(a)(a†−aa†)θe−∫a0γ(s)ds⋅v∗da.
Finally, we can choose an age-dependent interaction rate like
γ(a)=ˉγ⋅max(1−4ka(a†−a)/a†2,0),ˉγ>0,k∈R, |
modeling three different age-dependent interactions between predators and preys:
● If k<0, the interaction is stronger at intermediate ages of the preys. γ(a) has a local maximum. Predation is focused on the most fertile preys.
● If k=0, the interaction is independent of the age of the prey. γ(a) is a constant ˉγ>0. For predators, the ages of the preys do not matter.
● If k>0, the interaction is stronger at early and late ages of the preys. γ(a) has a local minimum. Predation is focused on the less fertile preys.
Notice that increasing parameter k in this interaction rate γ, from negative to positive, predators change their target preys, from mid-aged preys to both young and old preys.
See Figure 5 for an illustration of these three scenarios with a very different predator-prey dynamics. The numerical computation of the spectral bound of the linearized operator (5.5) around the coexistence equilibrium, is in agreement with the computation of the asymptotic population dynamics (i.e. the time-evolution of the interacting populations). In particular, when an isolated stable periodic orbit arises via a super-critical Hopf bifurcation, the equilibrium becomes unstable.
We have used several mathematical methods, either analytical or numerical techniques, to quantify the impact of a predator population targeting selected age-classes (or size-classes) in a prey population.
The idealized ecological scenario of both interacting populations living in harmonic periodic oscillation (with amplitude and frequency depending on initial conditions), takes place only when their interaction is independent of the age/size of the preys; see Figures 2 and 4 and the middle panels in Figure 5. For this unrealistic case, i.e., predators do not take advantage of the preys age or size, PDE system (2.1) with age-dependent parameters cannot be reduced to an ODE system, although its asymptotic behavior is actually given by the limit system of ODEs (4.2), which turns out to be the original Lotka-Volterra model. The key-point of this fact is the computation of the asymptotic age-profile (normalized age-density) for the prey population; see Theorem 1 in Section 4.
However, for natural populations, it is more realistic to assume a predator feeding on preys when they are at certain ages or sizes. For example, smaller preys are easier to catch. In this likely scenario and for a large set of initial conditions, we have an oscillating transition, eventually stabilizing towards a steady-state population; see Figure 1 and the top panels in Figure 5. Yet, in this more realistic ecological scenario, we have also seen that, for instance, when predators focus on both young (smaller) and old (more vulnerable) preys, and preys are most fertile at intermediate ages, predator and prey populations converge to a specific periodic oscillation independently of the initial conditions; see the bottom panels in Figure 5.
With our analysis, we have contributed to the long-standing debate on the oscillations onset in ecological models and on which are the minimal model ingredients able to trigger a Hopf bifurcation of the coexistence steady-state of both species. In summary, we just need to include the preys age in the interaction term of Lotka-Volterra type (i.e., proportional to both populations) to get to a stable periodic dynamics of predator and prey populations.
The numerical results are reliable since we have checked the accuracy of the methods introduced in Sections 5 and 6, using the exact solution available for specific model parameters.
The authors declare they have not used artificial intelligence (AI) tools in the creation of this article.
Members of the Catalan research group 2021 SGR 00113. This research was funded by Ministerio de Ciencia, Inovación y Universidades, grant numbers PID2019-104437GB-I00 and PID2021-123733NB-I00.
The authors declare there is no conflict of interest.
[1] | M. Iannelli, F. Milner, The Basic Approach to Age-Structured Population Dynamics, Models, Methods and Numerics, Springer, Dordrecht, 2017. https://doi.org/10.1007/978-94-024-1146-1 |
[2] | H. Thieme, Mathematics in population biology. Princeton Series in Theoretical and Computational Biology, Princeton University Press, Princeton, NJ, 2003. |
[3] | H. Inaba, Age-structured population dynamics in demography and epidemiology, Springer, Berlin, 2017. https://doi.org/10.1007/978-981-10-0188-8 |
[4] | H. Seno, A Primer on Population Dynamics Modeling: Basic Ideas for Mathematical Formulation, Springer, Singapore, 2022. https://doi.org/10.1007/978-981-19-6016-1 |
[5] | G. F. Webb, Theory of Nonlinear Age-Dependent Population Dynamics, Marcel Dekker, New York, 1985. |
[6] |
À. Calsina, J. Ripoll, Hopf bifurcation in a structured population model for the sexual phase of monogonont rotifers, J. Math. Biol.,45(2002), 22–36. https://doi.org/10.1007/s002850200147 doi: 10.1007/s002850200147
![]() |
[7] |
T. Kuniya, H. Inaba, Hopf bifurcation in a chronological age-structured SIR epidemic model with age-dependent infectivity, Math. Biosci. Eng., 20 (2023), 13036–13060. https://doi.org/10.3934/mbe.2023581 doi: 10.3934/mbe.2023581
![]() |
[8] |
Z. Liu, P. Magal, S. Ruan, Hopf bifurcation for non-densely defined Cauchy problems, Z. Angew. Math. Phys., 62 (2011), 191–222. https://doi.org/10.1007/s00033-010-0088-x doi: 10.1007/s00033-010-0088-x
![]() |
[9] | J. Chu, P. Magal, Hopf bifurcation for a size-structured model with resting phase, Discrete Contin. Dyn. Syst. - Ser. A, 2013, 33 (2013), 4891–4921. https://doi.org/10.3934/dcds.2013.33.4891 |
[10] |
Z. Liu, H. Tang, P. Magal, Hopf bifurcation for a spatially and age structured population dynamics model, Discrete Contin. Dyn. Syst. - Ser. B, 20 (2015), 1735–1757. https://doi.org/10.3934/dcdsb.2015.20.1735 doi: 10.3934/dcdsb.2015.20.1735
![]() |
[11] |
J. Chu, A. Ducrot, P. Magal, Hopf bifurcation in a size-structured population dynamic model with random growth, J. Differ. Equat., 247 (2009), 956–1000. https://doi.org/10.1016/j.jde.2009.04.003 doi: 10.1016/j.jde.2009.04.003
![]() |
[12] |
S. Bentout, S. Djilali, A. Atangana, Bifurcation analysis of an age-structured prey–predator model with infection developed in prey, Math. Meth. Appl. Sci., 45 (2022), 1189–1208. https://doi.org/10.1002/mma.7846 doi: 10.1002/mma.7846
![]() |
[13] |
S. Bentout, S. Kumar, S. Djilali, Hopf bifurcation analysis in an age-structured heroin model, Eur. Phys. J. Plus, 136 (2021), 1–13. https://doi.org/10.1140/epjp/s13360-020-01001-7 doi: 10.1140/epjp/s13360-020-01001-7
![]() |
[14] | M. Iannelli, A. Pugliese, An Introduction to Mathematical Population Dynamics. Along the Trail of Volterra and Lotka; Springer, New York, NY, USA, 2014. https://doi.org/10.1007/978-3-319-03026-5 |
[15] | N. Bacaër, A Short History of Mathematical Population Dynamics, Springer, London, UK, 2011. https://doi.org/10.1007/978-0-85729-115-8 |
[16] | N. Bacaër, R. Bravo de la Parra, J. Ripoll, Breve Historia de los Modelos Matemáticos en Dinámica de Poblaciones, Cassini: Paris, France, 2021. (in Spanish) |
[17] |
M. Gurtin, D. Levine, On predator-prey interactions with predation dependent on age of prey, Math. Biosci., 47 (1979), 207–219. https://doi.org/10.1016/0025-5564(79)90038-5 doi: 10.1016/0025-5564(79)90038-5
![]() |
[18] |
E. Venturino, Age-structured predator-prey models, Math. Modell., 5 (1984), 117–128. https://doi.org/10.1016/0270-0255(84)90020-4 doi: 10.1016/0270-0255(84)90020-4
![]() |
[19] |
A. Perasso, Q. Richard, Implication of age-structure on the dynamics of Lotka Volterra equations, Differ. Integr. Equations, 32 (2019), 91–120. https://doi.org/10.57262/die/1544497287 doi: 10.57262/die/1544497287
![]() |
[20] |
C. Barril, À. Calsina, O. Diekmann, J. Z. Farkas, On the formulation of size-structured consumer resource models (with special attention for the principle of linearized stability), Math. Models Methods Appl. Sci., 32 (2022), 1141–1191. https://doi.org/10.1142/S0218202522500269 doi: 10.1142/S0218202522500269
![]() |
[21] | D. Breda, S. Maset, R. Vermiglio, Stability of Linear Delay Differential Equations. A Numerical Approach with MATLAB. SpringerBriefs in Electrical and Computer Engineering. SpringerBriefs in Control, Automation and Robotics. Springer, New York, (2015). https://doi.org/10.1007/978-1-4939-2107-2 |
[22] |
F. Scarabel, D. Breda, O. Diekmann, M. Gyllenberg, R. Vermiglio, Numerical Bifurcation Analysis of Physiologically Structured Population Models via Pseudospectral Approximation, Vietnam J. Math., 49 (2021), 37–67. https://doi.org/10.1007/s10013-020-00421-3 doi: 10.1007/s10013-020-00421-3
![]() |
[23] |
D. Breda, T. Kuniya, J. Ripoll, R. Vermiglio, Collocation of Next-Generation Operators for Computing the Basic Reproduction Number of Structured Populations, J. Sci. Comput., 85 (2020), 40. https://doi.org/10.1007/s10915-020-01339-1 doi: 10.1007/s10915-020-01339-1
![]() |
[24] |
D. Breda, F. Florian, J. Ripoll, R. Vermiglio, Efficient numerical computation of the basic reproduction number for structured populations, J. Comput. Appl. Math., 384 (2021), 113165. https://doi.org/10.1016/j.cam.2020.113165 doi: 10.1016/j.cam.2020.113165
![]() |
[25] |
A. Andò, S. De Reggi, D. Liessi, F. Scarabel, A pseudospectral method for investigating the stability of linear population models with two physiological structures, Math. Biosci. Eng., 20 (2023), 4493–4515. https://doi.org/10.3934/mbe.2023208 doi: 10.3934/mbe.2023208
![]() |
[26] |
D. Breda, R. Vermiglio, S. Maset, Computing the eigenvalues of Gurtin-MacCamy models with diffusion, IMA J. Numer. Anal., 32 (2012), 1030–1050. https://doi.org/10.1093/imanum/drr004 doi: 10.1093/imanum/drr004
![]() |
[27] |
D. Breda, C. Cusulin, M. Iannelli, S. Maset, R. Vermiglio, Stability analysis of age-structured population equations by pseudospectral differencing methods, J. Math. Biol., 54 (2007), 701–720. https://doi.org/10.1007/s00285-006-0064-4 doi: 10.1007/s00285-006-0064-4
![]() |
[28] |
D. Breda, M. Iannelli, S. Maset, R. Vermiglio, Stability analysis of the Gurtin-MacCamy model, SIAM J. Numer. Anal., 46 (2008), 980–995. https://doi.org/10.1137/070685658 doi: 10.1137/070685658
![]() |
[29] |
C. Barril, À. Calsina, J. Ripoll, A practical approach to R0 in continuous-time ecological models, Math. Meth. Appl. Sci., 41 (2018), 8432–8445. https://doi.org/10.1002/mma.4673 doi: 10.1002/mma.4673
![]() |
[30] |
C. Barril, À. Calsina, S. Cuadrado, J. Ripoll, On the basic reproduction number in continuously structured populations, Math. Meth. Appl. Sci., 44 (2021), 799–812. https://doi.org/10.1002/mma.6787 doi: 10.1002/mma.6787
![]() |
[31] | R. Rudnicki, Mathematical modelling of population dynamics, volume 63 of Banach Center Publications, Polish Academy of Sciences Institute of Mathematics, Warsaw, 2004. |
[32] |
M. Gyllenberg, G. F. Webb, Asynchronous exponential growth of semigroups of nonlinear operators, J. Math. Anal. Appl., 167 (1992), 443–467. https://doi.org/10.1016/0022-247X(92)90218-3 doi: 10.1016/0022-247X(92)90218-3
![]() |
[33] | K. J. Engel, R. Nagel, One-Parameter Semigroups for Linear Evolution Equations, no. 194 in Grad. Texts in Math., Springer, New York, 2000. |
[34] |
O. Angulo, J. C. López-Marcos, M. A. López-Marcos, F. A. Milner, A numerical method for nonlinear age-structured population models with finite maximum age, J. Math. Anal. Appl., 361 (2010), 150–160. https://doi.org/10.1016/j.jmaa.2009.09.001 doi: 10.1016/j.jmaa.2009.09.001
![]() |
[35] | M. Martcheva, An Introduction to Mathematical Epidemiology, Springer, New York, 2015. https://doi.org/10.1007/978-1-4899-7612-3 |
1. | Dimitri Breda, Davide Liessi, A practical approach to computing Lyapunov exponents of renewal and delay equations, 2023, 21, 1551-0018, 1249, 10.3934/mbe.2024053 | |
2. | Dimitri Breda, Davide Liessi, Lyapunov exponents of renewal equations: Numerical approximation and convergence analysis, 2024, 0, 1531-3492, 0, 10.3934/dcdsb.2024152 | |
3. | Elena V. Nikolova, On the Extended Simple Equations Method (SEsM) and Its Application for Finding Exact Solutions of the Time-Fractional Diffusive Predator–Prey System Incorporating an Allee Effect, 2025, 13, 2227-7390, 330, 10.3390/math13030330 | |
4. | Swadesh Pal, Roderick Melnik, Nonlocal Models in Biology and Life Sciences: Sources, Developments, and Applications, 2025, 15710645, 10.1016/j.plrev.2025.02.005 |