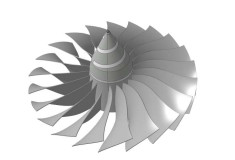
In the intelligent manufacturing environment, modern industry is developing at a faster pace, and there is an urgent need for reasonable production scheduling to ensure an organized production order and a dependable production guarantee for enterprises. Additionally, production cooperation between enterprises and different branches of enterprises is increasingly common, and distributed manufacturing has become a prevalent production model. In light of these developments, this paper presents the research background and current state of distributed shop scheduling. It summarizes relevant research on issues that align with the new manufacturing model, explores hot topics and concerns and focuses on the classification of distributed parallel machine scheduling, distributed flow shop scheduling, distributed job shop scheduling and distributed assembly shop scheduling. The paper investigates these scheduling problems in terms of single-objective and multi-objective optimization, as well as processing constraints. It also summarizes the relevant optimization algorithms and their limitations. It also provides an overview of research methods and objects, highlighting the development of solution methods and research trends for new problems. Finally, the paper analyzes future research directions in this field.
Citation: Jianguo Duan, Mengting Wang, Qinglei Zhang, Jiyun Qin. Distributed shop scheduling: A comprehensive review on classifications, models and algorithms[J]. Mathematical Biosciences and Engineering, 2023, 20(8): 15265-15308. doi: 10.3934/mbe.2023683
[1] | Yicang Zhou, Zhien Ma . Global stability of a class of discrete age-structured SIS models with immigration. Mathematical Biosciences and Engineering, 2009, 6(2): 409-425. doi: 10.3934/mbe.2009.6.409 |
[2] | Dong-Me Li, Bing Chai, Qi Wang . A model of hepatitis B virus with random interference infection rate. Mathematical Biosciences and Engineering, 2021, 18(6): 8257-8297. doi: 10.3934/mbe.2021410 |
[3] | Xichao Duan, Sanling Yuan, Kaifa Wang . Dynamics of a diffusive age-structured HBV model with saturating incidence. Mathematical Biosciences and Engineering, 2016, 13(5): 935-968. doi: 10.3934/mbe.2016024 |
[4] | Tingting Xue, Long Zhang, Xiaolin Fan . Dynamic modeling and analysis of Hepatitis B epidemic with general incidence. Mathematical Biosciences and Engineering, 2023, 20(6): 10883-10908. doi: 10.3934/mbe.2023483 |
[5] | Bao-Zhu Guo, Li-Ming Cai . A note for the global stability of a delay differential equation of hepatitis B virus infection. Mathematical Biosciences and Engineering, 2011, 8(3): 689-694. doi: 10.3934/mbe.2011.8.689 |
[6] | Maysaa Al Qurashi, Saima Rashid, Fahd Jarad . A computational study of a stochastic fractal-fractional hepatitis B virus infection incorporating delayed immune reactions via the exponential decay. Mathematical Biosciences and Engineering, 2022, 19(12): 12950-12980. doi: 10.3934/mbe.2022605 |
[7] | Tahir Khan, Fathalla A. Rihan, Muhammad Ibrahim, Shuo Li, Atif M. Alamri, Salman A. AlQahtani . Modeling different infectious phases of hepatitis B with generalized saturated incidence: An analysis and control. Mathematical Biosciences and Engineering, 2024, 21(4): 5207-5226. doi: 10.3934/mbe.2024230 |
[8] | Jiying Ma, Shasha Ma . Dynamics of a stochastic hepatitis B virus transmission model with media coverage and a case study of China. Mathematical Biosciences and Engineering, 2023, 20(2): 3070-3098. doi: 10.3934/mbe.2023145 |
[9] | C. Connell McCluskey . Global stability for an SEI model of infectious disease with age structure and immigration of infecteds. Mathematical Biosciences and Engineering, 2016, 13(2): 381-400. doi: 10.3934/mbe.2015008 |
[10] | Tailei Zhang, Hui Li, Na Xie, Wenhui Fu, Kai Wang, Xiongjie Ding . Mathematical analysis and simulation of a Hepatitis B model with time delay: A case study for Xinjiang, China. Mathematical Biosciences and Engineering, 2020, 17(2): 1757-1775. doi: 10.3934/mbe.2020092 |
In the intelligent manufacturing environment, modern industry is developing at a faster pace, and there is an urgent need for reasonable production scheduling to ensure an organized production order and a dependable production guarantee for enterprises. Additionally, production cooperation between enterprises and different branches of enterprises is increasingly common, and distributed manufacturing has become a prevalent production model. In light of these developments, this paper presents the research background and current state of distributed shop scheduling. It summarizes relevant research on issues that align with the new manufacturing model, explores hot topics and concerns and focuses on the classification of distributed parallel machine scheduling, distributed flow shop scheduling, distributed job shop scheduling and distributed assembly shop scheduling. The paper investigates these scheduling problems in terms of single-objective and multi-objective optimization, as well as processing constraints. It also summarizes the relevant optimization algorithms and their limitations. It also provides an overview of research methods and objects, highlighting the development of solution methods and research trends for new problems. Finally, the paper analyzes future research directions in this field.
Aeroengine is an important equipment to propel the aircraft forward and its total thrust is the sum of the thrust generated by the core engine and the turbine fan. The air flow state between adjacent blades of the turbine fan determines the thrust value of aeroengine. Since the experiments cost a lot, numerical simulations become an essential part of the blade geometry design and optimization [5,10,2,12,7]. Nevertheless, many difficulties arise in numerical simulation such as nonlinearity, high Reynolds number, complex three-dimensional (3D) geometrical domains, and boundary layer effect (see, e.g., [9]). In order to alleviate these difficulties, we will use the dimension splitting method to simulate the airflow state of aeroengine blade fan.
Based on the article [6], we establish a semi-geodesic coordinate system (called R-coordinate system), whose two basis vectors are on the manifold, and the other is along the hub circle. Thus, Navier-Stokes equations (NSEs) in the R-coordinate system can be rewritten as a set of membrane operator equations on the blade surface, and the bending operator equations along the hub circle [8]. By using Euler central difference scheme to approximate the third variable, the 3D NSEs become a series of two-dimensional (2D) equations with three variables. After successively iterations, the approximate solution to the NSEs can be obtained. Obviously, the significant feature of this new method is this method only solves the 2D problem in each sub-domain. In addition, it can alleviate the boundary layer effect by approaching adjacent surfaces, and the parameterized surface provides convenience for blade design and optimization.
The purpose of this work is to introduce our proposed method to simulate the flow state of the channel of aeroengine turbine fan. A lot of work has been carried out in the field of viscous flow and its applications in aeroengine turbine [16,15,4,11,14,13,3]. In this paper, a toy model is designed to give a comparison between our novel method and traditional methods. It turns out the new method shows a good performance for the toy model. Then we apply the proposed method to simulate the flow state of aeroengine turbine fan.
The present paper is built up as follows. In Section 2, some essential differential geometry knowledge is briefly introduced, then the R-coordinate system is established. Meanwhile, the NSEs' new form, splitting method and the variational form are formulated in Sections 3. Furthermore, we derive the finite element form in Section 4. Section 5 presents the numerical results, which contain the comparison of the new method and traditional methods, and the simulation results of aeroengine turbine fan.
In this section, we establish a new coordinate system according to the geometric shape of the blade and give the relationship between the new coordinate system and the rectangular coordinate system and the cylindrical coordinate system. To express concisely and clearly, we let Greek letters
Compared with the size of the aircraft engine fan, the thickness of the fan blade can be neglected. Thus the blade surface
ℜ(x)=rer+rΘ(r,z)eθ+zk. | (2.1) |
Here
The channel
ℜ(x;ξ)=rer+rθeθ+zk, | (2.2) |
where
Let
x1=z,x2=r,ξ=ε−1(θ−Θ(x)), |
it is clear that the Jacobian matrix
(r,θ,z)→(x1,x2,ξ):x1=z,x2=r,ξ=ε−1(θ−Θ(x)). | (2.3) |
In the R-coordinate system, the fixed region
Since the new coordinate is established, these basis vectors become a bridge to communicate different coordinate systems. Let
{er=cosθi+sinθj,eθ=−sinθi+cosθj,i=cosθer−sinθeθ,j=sinθer+cosθeθ. |
The basis vectors of the R-coordinate system are denoted as
{eα=∂αℜ=∂αxi+∂αyj+∂αzk,α=1,2,e3=∂∂ξ(ℜ)=∂x∂ξi+∂y∂ξj+∂z∂ξk, | (2.4) |
where
{x:=x(x1,x2,ξ)=rcosθ=x2cos(εξ+Θ(x1,x2)),y:=y(x1,x2,ξ)=rsinθ=x2sin(εξ+Θ(x1,x2)),z:=z(x1,x2,ξ)=x1. |
By straightforward calculation, the relationship between base vectors is as follows
{e1=x2Θ1eθ+k=−x2sinθΘ1i+x2cosθΘ1j+k,e2=Θ2x2eθ+er=(cosθ−x2sinθΘ2)i+(sinθ+x2cosθΘ2)j,e3=x2εeθ=−εx2sinθi+εx2cosθj,er=e2−ε−1Θ2e3,eθ=(εx2)−1e3,k=e1−ε−1Θ1e3,i=cosθe2−(ε−1cosθΘ2+(εx2)−1sinθ)e3,j=sinθe2+((εx2)−1cosθ−ε−1Θ2sinθ)e3, | (2.5) |
where
The metric tensor
aαβ:=eαeβ=δαβ+r2ΘαΘβ, |
and it is easy to see that
a=det(aαβ)=1+r2|∇Θ|2>0, | (2.6) |
where
Similarly, the covariant and contravariant components of metric tensor are
gij=eiej,gij=eiej. |
By calculation, they can be expressed as (cf.[5])
{gαβ=aαβ,g3β=gβ3=εr2Θβ,g33=ε2r2,gαβ=δαβ,g3β=gβ3=−ε−1Θβ,g33=(εr)−2a. | (2.7) |
As described in our previous article [6], for given
In this section, we derive the flows state governed by the incompressible rotational NSEs through employing differential operators in the R-coordinate system. Because of the lighter mass of air, its body force is neglected and the rotational NSEs can be expressed as
{∂u∂t−νΔu+(u∇)u+2ω×u+∇p=−ω×(ω×r),divu=0. | (3.1) |
For a time-dependent problem, we usually discretize the problem in time and solve the static problem at each time step, then the time-dependent problem is reduced to a static problem at each time step. If we consider an implicit time discretization (e.g., the backward Euler method) to equation (3.1) with time-step size
{u−νκΔu+κ(u∇)u+2κω×u+κ∇p=−κω×(ω×r)+fu,divu=0, | (3.2) |
where
Assume that
Γijk=ei⋅ejk,∇kui=∂ui∂xk+Γikmum,∗Γαβσ=eα⋅eβλ,∗∇βuα=∂uα∂xβ+∗Γαβσuσ, |
where
Lemma 3.1. [6] By denoting
1). The Laplace operator
Δui=˜Δui+2g3γ∗∇γ∂ui∂ξ+g33∂2ui∂ξ2+Li3γ∂uγ∂ξ+Li33∂u3∂ξ+Liσγ∗∇σuγ+Li0γuγ+δi3L3σ3∗∇σu3, | (3.3) |
where
{Lα3γ=−(εr)−1(Θ2δαγ+2aδ2αΘγ)−˜ΔΘδαγ,L33γ=−2(εr)−2(r2ΘβΘβγ−r−1δ2γ),Lα33=−2rδ2α,L333=−(εr)−1(r˜ΔΘ+Θ2),Lασγ=2rδ2αΘσΘγ−(r|∇Θ|2−r−1)δ2σδαγ,L3σγ=2(εr)−1(δ2σΘγ+rΘγσ)Lα0γ={Θγ[(a+1)Θ2+r˜ΔΘ]−r−2δ2γ+rΘβΘβγ}δ2α,L30γ=(εr)−1(3Θ2γ+2(r2Θ2αΘα+rΘ22)Θγ+)rΘααγ+r−2Θ2δ2γ,L3σ3=(3r−1−r|∇Θ|2)δ2σ, | (3.4) |
and
2). Its related items coriolis force and centrifugal force satisfy, respectively,
C=2κω×u=κCijujei, | (3.5) |
fic=−κεijkωj(→ω×r)k=κεijkgjmωmεklnωlrn=κεijkgjmωmεklnωlgn2r, | (3.6) |
where
3). The pressure gradient is
∇p=[gαβ∂p∂xβ+gα3∂p∂ξg3β∂p∂xβ+g33∂p∂ξ]=[∂p∂xα−ε−1Θα∂p∂ξ−ε−1Θβ∂p∂xβ+(rε)−2a∂p∂ξ]. |
4). The nonlinear term in equation (3.1) is
B(u,u)=κ(u∇)u=κBi(u)ei=κ[uβ∗∇βuα+u3∂uα∂ξ+nαkmukumuβ∗∇βu3+u3∂u3∂ξ+n3kmukum], | (3.7) |
where
{nαλσ=0,nα3β=nαβ3=−rεδ2αΘβ,nα33=−rε2δ2α,n333=rεΘ2,n3λσ=(rε)−1(a2λΘσ+δ2σΘλ)+ε−1Θλσ,n33β=n3β3=r−1a2β. |
5). The mass conservation formula can be rewritten as
div u=∂uα∂xα+∂u3∂ξ+u2r,∗divu=∂uα∂xα−rΘ2Θσuσ. | (3.8) |
By Lemma 3.1, the incompressible rotational NSEs in the R-coordinate system can be rewritten as
{ui+κ{uβ∗∇βui+u3∂ui∂ξ+nikmukum+Cijuj−ν[˜Δui+2g3γ∗∇γ∂ui∂ξ+g33∂2ui∂ξ2+Li3γ∂uγ∂ξ+Li33∂u3∂ξ+Liσγ∗∇σuγ+Li0γuγ+δi3L3σ3∗∇σu3]+giβ∂p∂xβ+gi3∂p∂ξ}=Fi,∂uβ∂xβ+∂u3∂ξ+u2r=0, | (3.9) |
where
Fi=fic+fiu. |
And its channel region and boundaries are
{Ω={(x1,x2,ξ)|(x1,x2)∈D,−1≤ξ≤1,},∂Ω=Γin∪Γout∪ℑ+∪ℑ−∪Γt∪Γb. | (3.10) |
The initial and boundary value conditions are
u|t=0=uo,u|ℑ+∪ℑ−=0,u|Γin=uin. | (3.11) |
In this section, the finite-element-difference method will be presented. The first step is to divide the interval
[−1,+1]=∪N−1k=0[ξk,ξk+1],ξk=−1+kτ,k=0,1,⋯N−1. |
Then the domain
∂w∂ξ≃wk+1−wk−12τ,∂2w∂ξ2≃wk+1−2wk+wk−1τ2. | (3.12) |
where
[w]+k:=wk+1+wk−1τ2,[w]−k:=wk+1−wk−12τ. | (3.13) |
Plugging (3.13) into (3.9), we obtain
{ui+κ{uβ∗∇βui+u3[ui]−k+nikmukum−ν[˜Δui+2g3γ∗∇γ[ui]−k+g33([ui]+k−2uiτ2)+Li3γ[uγ]−k+Li33[u3]−k+Liσγ∗∇σuγ+Li0γuγ+δi3L3σ3∗∇σu3]+giβ∂p∂xβ+gi3[p]−k+Cijuj}=˜Fik,∂uα∂xα+[u3]−k+u2r=0, | (3.14) |
where
˜Fik:=1τ∫ξk+1ξkFi. | (3.15) |
And its boundary conditions are
uk|γ0=0,uk|γin=uin,∂D=γ0∪γin∪γout, | (3.16) |
where
Introduce the Hilbert space
V(D)={u∈H1(D)×H1(D)×H1(D),u=0|γ0∪γin}, |
then its inner product and norms are given, respectively, by
(w,v)D=∫Ωaijwivj√adx,aij={aαβ=aαβ,aα3=a3α=0,a33=1},|w|21,D=∑α∑j‖∂αwj‖20,D,‖w‖20,D=∑j‖wj‖20,D,‖w‖21,D=|w|21,D+‖w‖20,D. |
Without the ambiguity, the index `D' is often omitted.
For clarity and simplicity, we denote
{Li(k):=−κν(˜Δuik+Liσγ∗∇σuγk+Li0γuγk−2g33τ−2uik+δi3L3σ3∗∇σu3k)+uik,Bi(k):=κ(uβk∗∇βuik+nilmulkumk),Ci(k):=κCimumk,Si:=−κν(2g3γ∗∇γ[ui]−k+g33[ui]+k+Li3γ[uγ]−k+Li33[u3]−k)+κu3k[ui]−k,Pi:=κgi3[p]−k. | (3.17) |
Meanwhile, we denote
{Lα(k)+Bα(k)+Cα(k)+κgαβ∂pk∂xβ=ˆFαk,L3(k)+B3(k)+C3(k)+κg3β∂pk∂xβ=ˆF3k,∂uα∂xα+[u3]−k+u2r=0. | (3.18) |
Thus, the variational problem corresponding to the boundary value problem of the NSEs is as follows:
{Seek uk∈L∞(0,T;V(D))+uin, pk∈L2(D),k=0,1,2,⋯,N−1,s.t.a(uk,v)+(C(uk),v)+(L(uk),v)+b(uk,uk,v)−(pk,m(v))=(ˆFk,v)−<h,v>γout,∀v∈V(D),(∂uαk∂xα+u2kr,q)=([u3]−k,q),∀q∈L2(D), | (3.19) |
where the linear, bilinear and trilinear forms are given, respectively,
{a(uk,v)=νκ(aij∂λuik,∂λvj)+νκ(ˆaijuik,vj)+(aijui,vj),ˆaij=aijr−2aα2τ,(C(uk),v)=κ(C∗i,vi),C∗β=aαβCαmumk,C∗3=C3mumk,(L(uk),v)=νκ(L∗i,vi),L∗β=∂λaαβ∂λuαk−aαβ(Lασν∗∇σuνk+Lα0νuνk),L∗3=−(L3σλ∗∇σuλk+L3σ3∗∇σu3k+L30σuσk),b(uk,uk,v)=(κaijBi(k),vj),(ˆFk,v)=(aijˆFik,vj),<h,v>γout=−∫γoutκμaij∂λuikvjnλdl+∫γoutκpk(aαλvα−εΘβv3)nλdl,m(v)=κ(∂αvα−εΘβ∂βv3−ε˜ΔΘv3). | (3.20) |
In this section, we apply the Taylor-Hood elements, i.e.,
Vh:={vh∈C0(ˉΩ);vh|K∈P2(K),∀K∈Th},Mh:={ph∈C0(ˉΩ);ph|K∈P1(K),∀K∈Th}. | (4.1) |
The product space
{Seek wh∈Vh, ph∈Mh s.t.a(wh,vh)+(C(wh),vh)+(L(wh),vh)+b(wh,wh,vh)−(ph,m(vh))=(ˆFk,vh)−<h,vh>γout,∀vh∈Vh,(∂wαh∂xα+w2hr,qh)=−([w3h]−k,qh),∀qh∈Mh. | (4.2) |
Suppose the finite element basis functions are denoted as
φi(x),i=1,2,⋯,NG1, ϕi(x), i=1,2,⋯NG2, |
where
wmh=NG1∑i=1Ximφi(x),ph=NG2∑i=1Piϕi(x),m=1,2,3,vkh=NG1∑i=1Yikφi(x),qh=NG2∑i=1Qiϕi(x),k=1,2,3. | (4.3) |
Assume solution vector
Wm={X1m,X2m,⋯XNG1m}T,W={W1,W2,W3}T,Vk={Y1k,Y2k,⋯YNG1k}T,V={V1,V2,V3}T,P={P1,⋯,PNG2}T,Q={Q1,⋯,QNG2}T. | (4.4) |
Substitute (4.4) into (4.2), we obtain the result on 2D membrane operator:
{a(wh,vh)=KαβijXiαYjβ,(L(wh),vh)=LτβijXiτYjβ,(C(wh,ω),vh)=CτβijXiτYjβ+C3βijXi3Yjβ,A0(wh,vh)=a(wh,vh)+(L(wh),vh)+(C(wh,ω),vh)=[KτβijXiτ+K3βijXi3]Yjβ,b(wh,wh,vh)=blm,βik,jXilXkmYjβ,(ph,m(vh))=B∗βijPiYjβ | (4.5) |
where
{Kαβij=κν[(aαβ∂λφi,∂λφj)+α2τ(aαβar−1φi,r−1φj)]+(aαβφi,φj),Lτβij=κν(∂λaαβ∂λφiδατ,φj)−κνaαβ((Lασν∂σδντ+LασνΓνσλδλτ+Lα0νδντ)φi,φj),Cτβij=(κaαβCατφi,φj),C3βij=(κaαβCα3φi,φj),Kτβij=Kαβijδτα+Lτβij+Cτβij,K3βij=C3βij,blm,βik,j=κ(aαβ{δlλφi[∂λφkδαm+Γαλσφkδσm]+nαlmφiφk},φj),B∗βij=κ(ϕi,∂βφj). |
Proof. In this proof, we only prove
∗∇σwν=∂σwν+Γνσλwλ,∗∇σw3=∂σw3, |
we obtain
(L(wh),vh)=κ(−νaαβLασν∗∇σwνh−νaαβLα0νwνh,vβh)+κ(ν∂λaαβ∂λwαh,vβh)=κ(−νaαβLασν[∂σwνh+Γνσλwλh]−νaαβLα0νwνh,vβh)+κ(ν∂λaαβ∂λwαh,vβh)=κν(∂λaαβ∂λwαh−aαβLασν∂σwνh,vβh)−κν(aαβLασνΓνσλwλh+aαβLα0νwνh,vβh)=κν(∂λaαβ∂λφiδατ−aαβLασν∂σφiδντ,φj)XiτYjβ−κν(aαβLασνΓνσλφiδλτ+aαβLα0νφiδντ,,φj)XiτYjβ=LτβijXiτYjβ. |
The remainder of the argument is analogous and is left to the reader.
Next, we will give the discrete scheme on the right side of the formula (4.2). According to the definition of inner product in space
{(ˆFk,v)=(aαβˆFαk,vβ)=ˆFβjYjβ,<h,v>γout=∫−κνaαβ∂λφiXiαφjYjβnλdl+∫κaαβϕiPiφjYjαnβdl=ˆHβjYjβ. |
Thus, we obtain
KτβijXiτ+K3βijXi3+blm,βik,jXilXkm−B∗βijPi=Fβj, | (4.6) |
where
{a(w3h,v3h)=K33ijXi3Yj3,(L(w3h),v3h)=Lτ3ijXiτYj3+L33ijXi3Yj3,(C(w3h,ω),v3h)=C3αijXiαYj3+C33ijXi3Yj3,A0(w3h,v3h)=[Kα3ijXiα+K33ijXi3]Yj3,b(w3,w3,v3)=blm,3ik,jXilXkmYj3,(ph,m(vh))=B∗3ijPiYj3, | (4.7) |
where
{K33ij=κν(∂λφi,∂λφj)+κνα2τ(ar−1φi,r−1φj)+(φi,φj),Lτ3ij=−(κν[L3σλ(∂σφiδλτ+Γλστφi)+L30τφi],φj),L33ij=−(κνL3σ3∂σφi,φj),Cα3ij=(κC3αφi,φj),C33ij=(κC33φi,φj),K33ij=K33ij+L33ij+C33ij,Kα3ij=Lα3ij+Cα3ij,blm,3ik,j=κ(φiδβlδ3m∂βφk+n3lmφiφk,φj),B∗3ij=−κ(ϕi,εΘβ∂βφj+ε˜ΔΘφj). |
And its right terms are
{(ˆF3k,v3)=(ˆF3k,Yj3φj)=ˆF3jYj3,<h3,v3>γout=−∫κν∂λφiXi3φjYj3nλdl−∫κεΘβϕiPiφjYj3nλdl=ˆH3jYj3. |
It is easy to verify it, so its proof will not be given here. Then the bending operators becomes
Kα3ijXiα+K33ijXi3+bml,3ik,jXimXkl−B∗3ijPi=F3j, | (4.8) |
where
Finally, we obtain the finite element algebraic equations of equation (3.19), i.e.,
{KτβijXiτ+K3βijXi3+blm,βik,jXilXkm−B∗βijPi=Fβj,Kα3ijXiα+K33ijXi3+bml,3ik,jXimXkl−B∗3ijPi=F3j,MijXiα=−(φi[Xi3]−k,ϕj), | (4.9) |
where
Mα∗ij=(∂αφi+r−1φiδα2). |
In this section, for certain examples, the results of the new algorithm program and the traditional algorithm program will be compared to verify the accuracy of the new algorithm program. And the new algorithm program shows good performance. Then, based on the new algorithm, the flow state of the gas in the fan channel of an aeroengine is given.
In this part, a simple model is provided to give the comparison between results obtained by the dimension splitting method (DS method) and traditional 3D method (T3D method). In this example, we adopt stationary model and assume
51 2D-manifolds and 4987 elements per surface are used to partition the channel and for DS method while 254867 elements are used for T3D method. In this model, we assume
As mentioned above, the flow state determines the maximum thrust that aeroengine turbine fan can provide. Our main purpose is using the DS method to simulate the flow state of the aeroengine turbine fan. In addition to the alleviation of boundary layer effects and make parallelism easier, DS method can give one clear design objective
In this article, we adopt the blades provided by the partner airlines which shape can be shown in Figure 15. Its meshes in the R-coordinate system are shown in Figure 1, and 51 2D-manifolds are used in this case. The velocity at inlet is 80 m/s and the rotating angular velocity is 80 rad/s. Figure 11 – Figure 12 show the pressure distribution on the blade surface. Because of the rotation, positive pressure surface and negative pressure surface have different pressure distribution. The pressure distribution of the positive pressure surface is higher than that of the negative pressure surface. Because the blade is cocked up at the bottom (see Figure 15), the pressure distribution near the bottom of the blade is obviously different from other places. Through formula transformation, we present the 3D model of blade pressure and velocity in Figure 13 – Figure 14.
Meanwhile, we show the velocity distribution at outlet in Figure 16. The velocity on the outside is significantly higher than that on the inside, and the velocity in the middle of the channel is also higher than that near the blade, which is consistent with common sense. From Figure 16, we can find that the maximum velocity is located at the edge of the blade, which can reach 130 m/s.
In this work, we conduct numerical simulations for 3D flow in the flow channel of the aeroengine turbine fan based on the dimension splitting method. However, the simulation of the flow states between the blades is not the ultimate goal. Our ultimate goal is to use the simulation results to design or optimize the blades. Meanwhile, the parameterized blade surface provides convenience for blade design and optimization. Thus, it will be the focus of follow-up research to optimize the blade by combining the inverse problem. In addition, compressible flow and boundary layer phenomenons are also the focus of the follow-up research.
The work of G. Ju was partially supported by the NSF of China (No. 11731006), the Shenzhen Sci-Tech Fund (No. JCYJ20170818153840322), and Guangdong Provincial Key Laboratory of Computational Science and Material Design No. 2019B030301001. The work of R. Chen was supported by the NSF of China (No. 61531166003) and Shenzhen Sci-Tech fund (No. JSGG20170824154458183 and ZDSYS201703031711426). The work of J. Li was partially supported by the NSF of China (No. 11971221) and Shenzhen Sci-Tech fund (No. JCYJ20190809150413261). The work of K. Li was supported by the NSF of China under the grant No. 10971165 and 10771167.
[1] |
G. Tang, H. Mai, How does manufacturing intelligentization influence innovation in china from a nonlinear perspective and economic servitization background?, Sustainability, 14 (2022), 14032–14032. https://doi.org/10.3390/su142114032 doi: 10.3390/su142114032
![]() |
[2] |
H. Zhang, G. Xu, R. Pan, H. Ge, A novel heuristic method for the energy-efficient flexible job-shop scheduling problem with sequence-dependent set-up and transportation time, Eng. Optim., 54 (2022), 1646–1667. https://doi.org/10.1080/0305215X.2021.1949007 doi: 10.1080/0305215X.2021.1949007
![]() |
[3] |
Ö. Tosun, M. K. Marichelvam, N. Tosun, A literature review on hybrid flow shop scheduling, J. Oper. Manage., 12 (2020), 156–194. https://doi.org/10.1504/IJAOM.2020.108263 doi: 10.1504/IJAOM.2020.108263
![]() |
[4] | L. Wang, Shop Scheduling with Genetic Algorithms, Tsinghua University & Springer Press, Beijing, 2003. |
[5] |
W. Xu, R. Wu, L. Wang, X. Zhao, X. Li, Solving a multi-objective distributed scheduling problem for building material equipment group enterprises by measuring quality indicator with a product gene evaluation approach, Comput. Ind. Eng., 168 (2022), 108142. https://doi.org/10.1016/j.cie.2022.108142 doi: 10.1016/j.cie.2022.108142
![]() |
[6] |
Y. Koren, S. J. Hu, P. Gu, M. Shpitalni, Open-architecture products, CIRP Ann., 62 (2013), 719–729. https://doi.org/10.1016/j.cirp.2013.06.001 doi: 10.1016/j.cirp.2013.06.001
![]() |
[7] |
F. Tao, Q. Qi, A. Liu, A. Kusiak, Data-driven smart manufacturing, J. Manuf. Syst., 48 (2018), 157–169. https://doi.org/10.1016/j.jmsy.2018.01.006 doi: 10.1016/j.jmsy.2018.01.006
![]() |
[8] |
J. Leng, Z. Chen, W. Sha, Z. Lin, J. Lin, Q. Liu, Digital twins-based flexible operating of open architecture production line for individualized manufacturing, Adv. Eng. Inf., 53 (2022), 101676. https://doi.org/10.1016/j.aei.2022.101676 doi: 10.1016/j.aei.2022.101676
![]() |
[9] |
W. Shen, L. Wang, Q. Hao, Agent-based distributed manufacturing process planning and scheduling: a state-of-the-art survey, IEEE Trans. Syst. Man Cybern. Syst., 36 (2006), 563–577. https://doi.org/10.1109/TSMCC.2006.874022 doi: 10.1109/TSMCC.2006.874022
![]() |
[10] | R. Agarwal, P. De, C. E. Wells, Cooperative distributed problem solving: an investigation in the domain of job shop scheduling, in Proceedings of the Twenty-Eighth Annual Hawaii International Conference on System Sciences, IEEE, 3 (1995), 4–12. https://doi.org/10.1109/HICSS.1995.375579 |
[11] | C. P. Gomes, A. Tate, L. Thomas, A distributed scheduling framework, in Proceedings Sixth International Conference on Tools with Artificial Intelligence. TAI 94, IEEE, (1994), 49–55. https://doi.org/10.1109/TAI.1994.346514 |
[12] |
A. Toptal, I. Sabuncuoglu, Distributed scheduling: a review of concepts and applications, Int. J. Prod. Res., 48 (2010), 5235–5262. https://doi.org/10.1080/00207540903121065 doi: 10.1080/00207540903121065
![]() |
[13] |
Z. Shao, W. Shao, D. Pi, Effective heuristics and metaheuristics for the distributed fuzzy blocking flow-shop scheduling problem, Swarm Evol. Comput., 59 (2020), 100747. https://doi.org/10.1016/j.swevo.2020.100747 doi: 10.1016/j.swevo.2020.100747
![]() |
[14] | S. Apte, N. Petrovsky, Will blockchain technology revolutionize excipient supply chain management?, J. Excipients Food Chem., 7 (2016), 76–78. |
[15] |
F. Tao, J. Cheng, Q. Qi, M. Zhang, H. Zhang, F. Sui, Digital twin-driven product design, manufacturing and service with big data, Int. J. Adv. Manuf. Technol., 94 (2018), 3563–3576. https://doi.org/10.1007/s00170-017-0233-1 doi: 10.1007/s00170-017-0233-1
![]() |
[16] |
J. Yli-Huumo, D. Ko, S. Choi, S. Park, K. Smolander, Where is current research on blockchain technology?—a systematic review, PLoS One, 11 (2016), e163477. https://doi.org/10.1371/journal.pone.0163477 doi: 10.1371/journal.pone.0163477
![]() |
[17] |
J. Leng, P. Jiang, K. Xu, Q. Liu, J. L. Zhao, Y. Bian, et al., Makerchain: A blockchain with chemical signature for self-organizing process in social manufacturing, J. Clean. Prod., 234 (2019), 767–778. https://doi.org/10.1016/j.jclepro.2019.06.265 doi: 10.1016/j.jclepro.2019.06.265
![]() |
[18] |
Y. Wu, Cloud-edge orchestration for the internet of things: architecture and AI-powered data processing, IEEE Internet Things, 8 (2021), 12792–12805. https://doi.org/10.1109/JIOT.2020.3014845 doi: 10.1109/JIOT.2020.3014845
![]() |
[19] |
J. Leng, Z. Chen, W. Sha, S. Ye, Q. Liu, X. Chen, Cloud-edge orchestration-based bi-level autonomous process control for mass individualization of rapid printed circuit boards prototyping services, J. Manuf. Syst., 63 (2022), 143–161. https://doi.org/10.1016/j.jmsy.2022.03.008 doi: 10.1016/j.jmsy.2022.03.008
![]() |
[20] | L. Wang, W. Shen, Process Planning and Scheduling for Distributed Manufacturing, Springer Science & Business Media, 2007. |
[21] |
P. Wu, Y. Wang, J. Cheng, Y. Li, An improved mixed-integer programming approach for bi-objective parallel machine scheduling and location, Comput. Ind. Eng., 174 (2022), 108813. https://doi.org/10.1016/j.cie.2022.108813 doi: 10.1016/j.cie.2022.108813
![]() |
[22] |
V. Heinz, A. Novák, M. Vlk, Z. Hanzálek, Constraint programming and constructive heuristics for parallel machine scheduling with sequence-dependent setups and common servers, Comput. Ind. Eng., 172 (2022), 108586. https://doi.org/10.1016/j.cie.2022.108586 doi: 10.1016/j.cie.2022.108586
![]() |
[23] |
G. Song, R. Leus, Parallel machine scheduling under uncertainty: Models and exact algorithms, INFORMS J. Comput., 34 (2022), 3059–3079. https://doi.org/10.1287/ijoc.2022.1229 doi: 10.1287/ijoc.2022.1229
![]() |
[24] |
N. Farmand, H. Zarei, M. Rasti-Barzoki, Two meta-heuristic algorithms for optimizing a multi-objective supply chain scheduling problem in an identical parallel machines environment, Int. J. Ind. Eng. Comput., 12 (2021), 249–272. https://doi.org/10.5267/j.ijiec.2021.3.002 doi: 10.5267/j.ijiec.2021.3.002
![]() |
[25] | M. Abedi, H. Seidgar, H. Fazlollahtabar, R. Bijani, Bi-objective optimisation for scheduling the identical parallel batch-processing machines with arbitrary job sizes, unequal job release times and capacity limits, Int. J. Prod. Res., 53 (2015), 1680–1711. https://doi.org/10.1080/00207543.2014.952795 |
[26] |
Y. Zheng, Y. Yuan, Q. Zheng, D. Lei, A hybrid imperialist competitive algorithm for the distributed unrelated parallel machines scheduling problem, Symmetry, 14 (2022), 204. https://doi.org/10.3390/sym14020204 doi: 10.3390/sym14020204
![]() |
[27] |
R. Logendran, B. McDonell, B. Smucker, Scheduling unrelated parallel machines with sequence-dependent setups, Comput. Oper. Res., 34 (2007), 3420–3438. https://doi.org/10.1016/j.cor.2006.02.006 doi: 10.1016/j.cor.2006.02.006
![]() |
[28] |
J. Behnamian, S. F. Ghomi, The heterogeneous multi-factory production network scheduling with adaptive communication policy and parallel machine, Inf. Sci., 219 (2013), 181–196. https://doi.org/10.1016/j.ins.2012.07.020 doi: 10.1016/j.ins.2012.07.020
![]() |
[29] |
D. Lei, M. Liu, An artificial bee colony with division for distributed unrelated parallel machine scheduling with preventive maintenance, Comput. Ind. Eng., 141 (2020), 106320–106320. https://doi.org/10.1016/j.cie.2020.106320 doi: 10.1016/j.cie.2020.106320
![]() |
[30] | G. Bektur, T. Saraç, A mathematical model and heuristic algorithms for an unrelated parallel machine scheduling problem with sequence-dependent setup times, machine eligibility restrictions and a common server, Comput. Ind. Eng., 103 (2018), 46–63. https://doi.org/10.1016/j.cor.2018.10.010 |
[31] |
H. Jouhari, D. Lei, M. A. Al-qaness, , M. A. Elaziz, A. A. Ewees, O. Farouk, Sine-cosine algorithm to enhance simulated annealing for unrelated parallel machine scheduling with setup times, Mathematics, 7 (2019), 1120. https://doi.org/10.3390/math7111120 doi: 10.3390/math7111120
![]() |
[32] |
D. Lei, Y. Yuan, J. Cai, D. Bai, An imperialist competitive algorithm with memory for distributed unrelated parallel machines scheduling, Int. J. Prod. Res., 58 (2020), 597–614. https://doi.org/10.1080/00207543.2019.1598596 doi: 10.1080/00207543.2019.1598596
![]() |
[33] | D. Li, J. Wang, R. Qiang, R. Chiong, A hybrid differential evolution algorithm for parallel machine scheduling of lace dyeing considering colour families, sequence-dependent setup and machine eligibility, Int. J. Prod. Res., 59 (2020), 1–17. https://doi.org/10.1080/00207543.2020.1740341 |
[34] |
D. Lei, Y. Yuan, J. Cai, An improved artificial bee colony for multi-objective distributed unrelated parallel machine scheduling, Int. J. Prod. Res., 59 (2020), 1–13. https://doi.org/10.1080/00207543.2020.1775911 doi: 10.1080/00207543.2020.1775911
![]() |
[35] |
B. Shahidi-Zadeh, R. Tavakkoli-Moghaddam, A. Taheri-Moghadam, I. Rastgar, Solving a bi-objective unrelated parallel batch processing machines scheduling problem: A comparison study, Comput. Ind. Eng., 88 (2017), 71–90. https://doi.org/10.1016/j.cor.2017.06.019 doi: 10.1016/j.cor.2017.06.019
![]() |
[36] |
Y. He, C. W. Hui, Automatic rule combination approach for single-stage process scheduling problems, AIChE J., 53 (2007), 2026–2047. https://doi.org/10.1002/aic.11236 doi: 10.1002/aic.11236
![]() |
[37] |
Z. Pan, D. Lei, L. Wang, A knowledge-based two-population optimization algorithm for distributed energy-efficient parallel machines scheduling, IEEE Trans. Cybern., 52 (2020), 5051–5063. https://doi.org/10.1109/TCYB.2020.3026571 doi: 10.1109/TCYB.2020.3026571
![]() |
[38] |
L. Zhang, Q. Deng, Y. Zhao, Q. Fan, X. Liu, G. Gong, Joint optimization of demand-side operational utility and manufacture-side energy consumption in a distributed parallel machine environment, Comput. Ind. Eng., 164 (2022), 107863. https://doi.org/10.1016/j.cie.2021.107863 doi: 10.1016/j.cie.2021.107863
![]() |
[39] |
X. Wu, A. Che, A memetic differential evolution algorithm for energy-efficient parallel machine scheduling, Omega, 82 (2018), 155–165. https://doi.org/10.1016/j.omega.2018.01.001 doi: 10.1016/j.omega.2018.01.001
![]() |
[40] | J. Behnamian, M. Zandieh, S. F. Ghomi, Parallel-machine scheduling problems with sequence-dependent setup times using an ACO, SA and VNS hybrid algorithm, Expert Syst. Appl., 36 (2009), 9637–9644. https://doi.org/10.1016/j.eswa.2008.10.007 |
[41] |
M. Yazdani, S. Gohari, B. Naderi, Multi-factory parallel machine problems: Improved mathematical models and artificial bee colony algorithm, Comput. Ind. Eng., 81 (2015), 36–45. https://doi.org/10.1016/j.cie.2014.12.023 doi: 10.1016/j.cie.2014.12.023
![]() |
[42] |
J. Behnamian, S. F. Ghomi, The heterogeneous multi-factory production network scheduling with adaptive communication policy and parallel machine, Inf. Sci., 219 (2013), 181–196. https://doi.org/10.1016/j.ins.2012.07.020 doi: 10.1016/j.ins.2012.07.020
![]() |
[43] |
J. Behnamian, Matheuristic for the decentralized factories scheduling problem, Appl. Math. Model., 47 (2017), 668–684. https://doi.org/10.1016/j.apm.2017.02.033 doi: 10.1016/j.apm.2017.02.033
![]() |
[44] |
J. Behnamian, Heterogeneous networked cooperative scheduling with anarchic particle swarm optimization, IEEE Trans. Eng. Manage., 64 (2017), 166–178. https://doi.org/10.1016/j.apm.2017.02.033 doi: 10.1016/j.apm.2017.02.033
![]() |
[45] |
J. Behnamian, S. M. T. F. Ghomi, Multi-objective multi-factory scheduling, RAIRO-Oper. Res., 55 (2021), S1447–S1467. https://doi.org/10.1051/ro/2020044 doi: 10.1051/ro/2020044
![]() |
[46] |
J. Behnamian, H. Asgari, A hyper-heuristic for distributed parallel machine scheduling with machine-dependent processing and sequence-dependent setup times, RAIRO-Oper. Res., 56 (2022), 4129–4143. https://doi.org/10.1051/ro/2022194 doi: 10.1051/ro/2022194
![]() |
[47] | S. Hatami, R. Ruiz, C. Andrés-Romano, Heuristics for a distributed parallel machine assembly scheduling problem with eligibility constraints, in 2015 International Conference on Industrial Engineering and Systems Management (IESM), (2015), 145–153. https://doi.org/10.1109/IESM.2015.7380149 |
[48] |
S. Hatami, R. R. García, C. A. Romano, The Distributed Assembly Parallel Machine Scheduling Problem with eligibility constraints, Int. J. Eng. Sci., 3 (2015), 13–23. https://doi.org/10.4995/ijpme.2015.3345 doi: 10.4995/ijpme.2015.3345
![]() |
[49] |
A. Hamzadayı, An effective benders decomposition algorithm for solving the distributed permutation flowshop scheduling problem, Comput. Oper. Res., 123 (2020), 105006. https://doi.org/10.1016/j.cor.2020.105006 doi: 10.1016/j.cor.2020.105006
![]() |
[50] |
V. Fernandez-Viagas, J. M. Framinan, A bounded-search iterated greedy algorithm for the distributed permutation flowshop scheduling problem, Int. J. Prod. Res., 53 (2015), 1111–1123. https://doi.org/10.1080/00207543.2014.948578 doi: 10.1080/00207543.2014.948578
![]() |
[51] |
J. Y. Mao, Q. K. Pan, Z. H. Miao, L. Gao, S. Chen, A hash map-based memetic algorithm for the distributed permutation flowshop scheduling problem with preventive maintenance to minimize total flowtime, Knowl. Based Syst., 242 (2022), 108413. https://doi.org/10.1016/j.knosys.2022.108413 doi: 10.1016/j.knosys.2022.108413
![]() |
[52] |
K. Wang, Y. Huang, H. Qin, A fuzzy logic-based hybrid estimation of distribution algorithm for distributed permutation flowshop scheduling problems under machine breakdown, J. Oper. Res. Soc., 67 (2016), 68–82. https://doi.org/10.1057/jors.2015.50 doi: 10.1057/jors.2015.50
![]() |
[53] |
B. Naderi, R. Ruiz, The distributed permutation flowshop scheduling problem, Comput. Oper. Res., 37 (2010), 754–768. https://doi.org/10.1016/j.cor.2009.06.019 doi: 10.1016/j.cor.2009.06.019
![]() |
[54] |
E. Taillard, Some efficient heuristic methods for the flow shop sequencing problem, Eur. J. Oper. Res., 47 (1990), 65–74. https://doi.org/10.1016/0377-2217(90)90090-X doi: 10.1016/0377-2217(90)90090-X
![]() |
[55] |
J. Gao, R. Chen, W. Deng, An efficient tabu search algorithm for the distributed permutation flowshop scheduling problem, Int. J. Prod. Res., 51 (2013), 641–651. https://doi.org/10.1080/00207543.2011.644819 doi: 10.1080/00207543.2011.644819
![]() |
[56] |
S. Y. Wang, L. Wang, M. Liu, Y. Xu, An effective estimation of distribution algorithm for solving the distributed permutation flow-shop scheduling problem, Int. J. Prod. Econ., 145 (2013), 387–396. https://doi.org/10.1016/j.ijpe.2013.05.004 doi: 10.1016/j.ijpe.2013.05.004
![]() |
[57] |
D. Ferone, S. Hatami, E. M. González‐Neira, A. A. Juan, P. Festa, A biased‐randomized iterated local search for the distributed assembly permutation flow‐shop problem, Int. Trans. Oper. Res., 27 (2020), 1368–1391. https://doi.org/10.1111/itor.12719 doi: 10.1111/itor.12719
![]() |
[58] |
J. P. Huang, Q. K. Pan, L. Gao, An effective iterated greedy method for the distributed permutation flowshop scheduling problem with sequence-dependent setup times, Swarm Evol. Comput., 59 (2020), 100742. https://doi.org/10.1016/j.swevo.2020.100742 doi: 10.1016/j.swevo.2020.100742
![]() |
[59] |
Y. Xu, L. Wang, S. Wang, M. Liu, An effective hybrid immune algorithm for solving the distributed permutation flow-shop scheduling problem, Eng. Optim., 46 (2014), 1269–1283. https://doi.org/10.1080/0305215X.2013.827673 doi: 10.1080/0305215X.2013.827673
![]() |
[60] | A. Ali, Y. Gajpal, T. Y. Elmekkawy, Distributed permutation flowshop scheduling problem with total completion time objective, Opsearch, (2020), 1–23. https://doi.org/10.1007/s12597-020-00484-3 |
[61] |
A. Hamzadayı, An effective benders decomposition algorithm for solving the distributed permutation flowshop scheduling problem, Comput. Oper. Res., 123 (2020), 105006. https://doi.org/10.1016/j.cor.2020.105006 doi: 10.1016/j.cor.2020.105006
![]() |
[62] |
J. Y. Mao, Q. K. Pan, Z. H. Miao, L. Gao, S. Chen, A hash map-based memetic algorithm for the distributed permutation flowshop scheduling problem with preventive maintenance to minimize total flowtime, Knowl. Based Syst., 242 (2022), 108413. https://doi.org/10.1016/j.knosys.2022.108413 doi: 10.1016/j.knosys.2022.108413
![]() |
[63] |
Y. Yu, F. Q. Zhang, G. D. Yang, Y. Wang, J. P. Huang, Y. Y. Han, A discrete artificial bee colony method based on variable neighborhood structures for the distributed permutation flowshop problem with sequence-dependent setup times, Swarm Evol. Comput., 75 (2022), 101179. https://doi.org/10.1016/j.swevo.2022.101179 doi: 10.1016/j.swevo.2022.101179
![]() |
[64] |
V. Fernandez-Viagas, P. Perez-Gonzalez, J. M. Framinan, The distributed permutation flow shop to minimise the total flowtime, Comput. Ind. Eng., 118 (2018), 464–477. https://doi.org/10.1016/j.cie.2018.03.014 doi: 10.1016/j.cie.2018.03.014
![]() |
[65] |
A. Khare, S. Agrawal, Effective heuristics and metaheuristics to minimise total tardiness for the distributed permutation flowshop scheduling problem, Int. J. Prod. Res., 59 (2021), 7266–7282. https://doi.org/10.1080/00207543.2020.1837982 doi: 10.1080/00207543.2020.1837982
![]() |
[66] |
Y. Z. Li, Q. K. Pan, X. He, H. Y. Sang, K. Z. Gao, X. L. Jing, The distributed flowshop scheduling problem with delivery dates and cumulative payoffs, Comput. Ind. Eng., 165 (2022), 107961. https://doi.org/10.1016/j.cie.2022.107961 doi: 10.1016/j.cie.2022.107961
![]() |
[67] |
P. A. Villarinho, J. Panadero, L. S. Pessoa, A. A. Juan, F. L. C. Oliveira, A simheuristic algorithm for the stochastic permutation flow‐shop problem with delivery dates and cumulative payoffs, Int. Trans. Oper. Res., 28 (2021), 716–737. https://doi.org/10.1111/itor.12862 doi: 10.1111/itor.12862
![]() |
[68] | Q. Li, J. Li, X. Zhang, B. Zhang, A wale optimization algorithm for distributed flow shop with batch delivery, Soft Comput., 25 (2021), 1–14. |
[69] |
A. P. Rifai, H. T. Nguyen, S. Z. M. Dawal, Multi-objective adaptive large neighborhood search for distributed reentrant permutation flow shop scheduling, Appl. Soft Comput., 40 (2016), 42–57. https://doi.org/10.1016/j.asoc.2015.11.034 doi: 10.1016/j.asoc.2015.11.034
![]() |
[70] |
J. Deng, L. Wang, A competitive memetic algorithm for multi-objective distributed permutation flow shop scheduling problem, Swarm Evol. Comput., 32 (2017), 121–131. https://doi.org/10.1016/j.swevo.2016.06.002 doi: 10.1016/j.swevo.2016.06.002
![]() |
[71] |
Z. Yan, R. Shi, K. Du, L. Yi, The role of green production process innovation in green manufacturing: empirical evidence from OECD countries, Appl. Econ., 54 (2022), 6755–6767. https://doi.org/10.1080/00036846.2022.2083569 doi: 10.1080/00036846.2022.2083569
![]() |
[72] |
C. Zhang, W. Ji, Digital twin-driven carbon emission prediction and low-carbon control of intelligent manufacturing job-shop, Procedia CIRP, 83 (2019), 624–629. https://doi.org/10.1016/j.procir.2019.04.095 doi: 10.1016/j.procir.2019.04.095
![]() |
[73] |
X. Wu, A. Che, Energy-efficient no-wait permutation flow shop scheduling by adaptive multi-objective variable neighborhood search, Omega, 94 (2020), 102117–102117. https://doi.org/10.1016/j.omega.2019.102117 doi: 10.1016/j.omega.2019.102117
![]() |
[74] |
J. F. Chen, L. Wang, Z. P. Peng, A collaborative optimization algorithm for energy-efficient multi-objective distributed no-idle flow-shop scheduling, Swarm Evol. Comput., 50 (2019), 100557–100557. https://doi.org/10.1016/j.swevo.2019.100557 doi: 10.1016/j.swevo.2019.100557
![]() |
[75] |
T. Meng, Q. K. Pan, L. Wang, A distributed permutation flowshop scheduling problem with the customer order constraint, Knowl. Based Syst., 184 (2019), 104894–104894. https://doi.org/10.1016/j.knosys.2019.104894 doi: 10.1016/j.knosys.2019.104894
![]() |
[76] |
K. Wang, Y. Huang, H. Qin, A fuzzy logic-based hybrid estimation of distribution algorithm for distributed permutation flowshop scheduling problems under machine breakdown, J. Oper. Res. Soc., 67 (2016), 68–82. https://doi.org/10.1057/jors.2015.50 doi: 10.1057/jors.2015.50
![]() |
[77] |
J. Y. Mao, Q. K. Pan, Z. H. Miao, L. Gao, An effective multi-start iterated greedy algorithm to minimize makespan for the distributed permutation flowshop scheduling problem with preventive maintenance, Expert Syst. Appl., 169 (2021), 114495. https://doi.org/10.1016/j.eswa.2020.114495 doi: 10.1016/j.eswa.2020.114495
![]() |
[78] |
W. Shao, D. Pi, Z. Shao, Optimization of makespan for the distributed no-wait flow shop scheduling problem with iterated greedy algorithms, Knowl. Based Syst., 137 (2017), 163–181. https://doi.org/10.1016/j.knosys.2017.09.026 doi: 10.1016/j.knosys.2017.09.026
![]() |
[79] |
S. W. Lin, K. C. Ying, Minimizing makespan for solving the distributed no-wait flowshop scheduling problem, Comput. Ind. Eng., 99 (2016), 202–209. https://doi.org/10.1016/j.cie.2016.07.027 doi: 10.1016/j.cie.2016.07.027
![]() |
[80] |
G. Zhang, K. Xing, Differential evolution metaheuristics for distributed limited-buffer flowshop scheduling with makespan criterion, Comput. Oper. Res., 108 (2019), 33–43. https://doi.org/10.1016/j.cor.2019.04.002 doi: 10.1016/j.cor.2019.04.002
![]() |
[81] |
F. Zhao, L. Zhao, L. Wang, H. Song, An ensemble discrete differential evolution for the distributed blocking flowshop scheduling with minimizing makespan criterion, Expert Syst. Appl., 160 (2020), 113678. https://doi.org/10.1016/j.eswa.2020.113678 doi: 10.1016/j.eswa.2020.113678
![]() |
[82] |
X. Han, Y. Han, Q. Chen, J. Li, H. Sang, Y. Liu, et al., Distributed flow shop scheduling with sequence-dependent setup times using an improved iterated greedy algorithm, Complex Syst., 1 (2021), 198–217. https://doi.org/10.23919/CSMS.2021.0018 doi: 10.23919/CSMS.2021.0018
![]() |
[83] |
X. Han, Y. Han, B. Zhang, H. Qin, J. Li, Y. Liu, et al., An effective iterative greedy algorithm for distributed blocking flowshop scheduling problem with balanced energy costs criterion, Appl. Soft Comput., 129 (2022), 109502. https://doi.org/10.1016/j.asoc.2022.109502 doi: 10.1016/j.asoc.2022.109502
![]() |
[84] |
W. Shao, D. Pi, Z. Shao, A Pareto-based estimation of distribution algorithm for solving multiobjective distributed no-wait flow-shop scheduling problem with sequence-dependent setup time, IEEE Trans. Autom. Sci. Eng., 16 (2019), 1344–1360. https://doi.org/10.1109/TASE.2018.2886303 doi: 10.1109/TASE.2018.2886303
![]() |
[85] |
J. P. Huang, Q. K. Pan, L. Gao, An effective iterated greedy method for the distributed permutation flowshop scheduling problem with sequence-dependent setup times, Swarm Evol. Comput., 59 (2020), 100742. https://doi.org/10.1016/j.swevo.2020.100742 doi: 10.1016/j.swevo.2020.100742
![]() |
[86] |
J. P. Huang, Q. K. Pan, Z. H. Miao, L. Gao, Effective constructive heuristics and discrete bee colony optimization for distributed flowshop with setup times, Eng. Appl. Artif. Intell., 97 (2021), 104016. https://doi.org/10.1016/j.engappai.2020.104016 doi: 10.1016/j.engappai.2020.104016
![]() |
[87] |
T. Meng, Q. K. Pan, L. Wang, A distributed permutation flowshop scheduling problem with the customer order constraint, Knowl. Based Syst., 184 (2019), 104894. https://doi.org/10.1016/j.knosys.2019.104894 doi: 10.1016/j.knosys.2019.104894
![]() |
[88] |
S. Cai, K. Yang, K. Liu, Multi-objective optimization of the distributed permutation flow shop scheduling problem with transportation and eligibility constraints, J. Oper. Res. Soc. China, 6 (2018), 391–416. https://doi.org/10.1007/s40305-017-0165-3 doi: 10.1007/s40305-017-0165-3
![]() |
[89] | S. Hatami, R. Ruiz, C. A. Romano, Two simple constructive algorithms for the distributed assembly permutation flowshop scheduling problem, in Managing Complexity: Challenges for Industrial Engineering and Operations Management, (2014), 139–145. |
[90] |
Q. K. Pan, L. Gao, L. X. Yu, F. M. Jose, Effective constructive heuristics and meta-heuristics for the distributed assembly permutation flowshop scheduling problem, Appl. Soft Comput., 81 (2019), 105492. https://doi.org/10.1016/j.asoc.2019.105492 doi: 10.1016/j.asoc.2019.105492
![]() |
[91] | X. Li, X. Zhang, M. Yin, J. Wang, A genetic algorithm for the distributed assembly permutation flowshop scheduling problem, in 2015 IEEE Congress on Evolutionary Computation (CEC), IEEE, (2015), 3096–3101. https://doi.org/10.1109/CEC.2015.7257275 |
[92] |
D. Ferone, S. Hatami, E. M. González‐Neira, A. A. Juan, P. Festa, A biased‐randomized iterated local search for the distributed assembly permutation flow‐shop problem, Int. Trans. Oper. Res., 27 (2020), 1368–1391. https://doi.org/10.1111/itor.12719 doi: 10.1111/itor.12719
![]() |
[93] |
J. Lin, Z. J. Wang, X. Li, A backtracking search hyper-heuristic for the distributed assembly flow-shop scheduling problem, Swarm Evol. Comput., 36 (2017), 124–135. https://doi.org/10.1016/j.swevo.2017.04.007 doi: 10.1016/j.swevo.2017.04.007
![]() |
[94] |
G. Zhang, K. Xing, F. Cao, Scheduling distributed flowshops with flexible assembly and set-up time to minimise makespan, Int. J. Prod. Res., 56 (2018), 3226–3244. https://doi.org/10.1080/00207543.2017.1401241 doi: 10.1080/00207543.2017.1401241
![]() |
[95] |
J. Deng, L. Wang, S. Y. Wang, X. L. Zheng, A competitive memetic algorithm for the distributed two-stage assembly flow-shop scheduling problem, Int. J. Prod. Res., 54 (2016), 3561–3577. https://doi.org/10.1080/00207543.2015.1084063 doi: 10.1080/00207543.2015.1084063
![]() |
[96] |
H. Y. Sang, Q. K. Pan, J. Q. Li, P. Wang, Y. Y. Han, K. Z. Gao, et al., Effective invasive weed optimization algorithms for distributed assembly permutation flowshop problem with total flowtime criterion, Swarm Evol. Comput., 44 (2019), 64–73. https://doi.org/10.1016/j.swevo.2018.12.001 doi: 10.1016/j.swevo.2018.12.001
![]() |
[97] |
F. Zhao, X. Hu, L.Wang, Z. Li, A memetic discrete differential evolution algorithm for the distributed permutation flow shop scheduling problem, Complex Intell. Syst., 8 (2022), 141–161. https://doi.org/10.1007/s40747-021-00354-5 doi: 10.1007/s40747-021-00354-5
![]() |
[98] |
F. Xiong, K. Xing, Meta-heuristics for the distributed two-stage assembly scheduling problem with bi-criteria of makespan and mean completion time, Int. J. Prod. Res., 52 (2014), 2743–2766. https://doi.org/10.1080/00207543.2014.884290 doi: 10.1080/00207543.2014.884290
![]() |
[99] |
X. Wu, X. Liu, N. Zhao, An improved differential evolution algorithm for solving a distributed assembly flexible job shop scheduling problem, Memet Comput., 11 (2019), 335–355. https://doi.org/10.1007/s12293-018-00278-7 doi: 10.1007/s12293-018-00278-7
![]() |
[100] |
Y. Y. Huang, Q. K. Pan, J. P. Huang, P. N. Suganthan, L. Gao, An improved iterated greedy algorithm for the distributed assembly permutation flowshop scheduling problem, Comput. Ind. Eng., 152 (2021), 107021. https://doi.org/10.1016/j.cie.2020.107021 doi: 10.1016/j.cie.2020.107021
![]() |
[101] |
G. Wang, X. Li, L. Gao, P. Li, Energy-efficient distributed heterogeneous welding flow shop scheduling problem using a modified MOEA/D, Swarm Evol. Comput., 62 (2021), 100858. https://doi.org/10.1016/j.swevo.2021.100858 doi: 10.1016/j.swevo.2021.100858
![]() |
[102] |
G. Wang, X. Li, L. Gao, P. Li, An effective multi-objective whale swarm algorithm for energy-efficient scheduling of distributed welding flow shop, Ann. Oper. Res., 310 (2022), 223–255. https://doi.org/10.1007/s10479-021-03952-1 doi: 10.1007/s10479-021-03952-1
![]() |
[103] |
J. Cai, D. Lei, M. Li, A shuffled frog-leaping algorithm with memeplex quality for bi-objective distributed scheduling in hybrid flow shop, Int. J. Prod. Res., 59 (2020), 1–18. https://doi.org/10.1080/00207543.2020.1780333 doi: 10.1080/00207543.2020.1780333
![]() |
[104] |
J. Cai, R. Zhou, D. Lei, Dynamic shuffled frog-leaping algorithm for distributed hybrid flow shop scheduling with multiprocessor tasks, Appl. Artif. Intell., 90 (2020), 103540–103540. https://doi.org/10.1016/j.engappai.2020.103540 doi: 10.1016/j.engappai.2020.103540
![]() |
[105] |
C. Lu, Q. Liu, B. Zhang, L. Yin, A Pareto-based hybrid iterated greedy algorithm for energy-efficient scheduling of distributed hybrid flowshop, Expert Syst. Appl., 204 (2022), 117555. https://doi.org/10.1016/j.eswa.2022.117555 doi: 10.1016/j.eswa.2022.117555
![]() |
[106] |
H. Qin, T. Li, Y. Teng, K. Wang, Integrated production and distribution scheduling in distributed hybrid flow shops, Memet Comput., 13 (2021), 185–202. https://doi.org/10.1007/s12293-021-00329-6 doi: 10.1007/s12293-021-00329-6
![]() |
[107] |
C. Lu, L. Gao, J. Yi, X. Li, Energy-efficient scheduling of distributed flow shop with heterogeneous factories: A real-world case from automobile industry in China, IEEE Trans. Ind. Inf., 17 (2020), 6687–6696. https://doi.org/10.1109/TⅡ.2020.3043734 doi: 10.1109/TⅡ.2020.3043734
![]() |
[108] |
E. Jiang, L. Wang, J. Wang, Decomposition-based multi-objective optimization for energy-aware distributed hybrid flow shop scheduling with multiprocessor tasks, Tsinghua Sci. Technol., 26 (2021), 646–663. https://doi.org/10.26599/TST.2021.9010007 doi: 10.26599/TST.2021.9010007
![]() |
[109] |
J. Cai, D. Lei, A cooperated shuffled frog-leaping algorithm for distributed energy-efficient hybrid flow shop scheduling with fuzzy processing time, Complex Intell. Syst., 7 (2021), 2235–2253. https://doi.org/10.1007/s40747-021-00400-2 doi: 10.1007/s40747-021-00400-2
![]() |
[110] |
J. Zheng, L. Wang, J. J. Wang, A cooperative coevolution algorithm for multi-objective fuzzy distributed hybrid flow shop, Knowl. Based Syst., 194 (2020), 105536. https://doi.org/10.1016/j.knosys.2020.105536 doi: 10.1016/j.knosys.2020.105536
![]() |
[111] |
J. J. Wang, L. Wang, A knowledge-based cooperative algorithm for energy-efficient scheduling of distributed flow-shop, IEEE Trans. Syst. Man Cybern.: Syst., 50 (2018), 1805–1819. https://doi.org/10.1109/TSMC.2017.2788879 doi: 10.1109/TSMC.2017.2788879
![]() |
[112] |
G. Zhang, K. Xing, Differential evolution metaheuristics for distributed limited-buffer flowshop scheduling with makespan criterion, Comput. Oper. Res., 108 (2019), 33–43. https://doi.org/10.1016/j.cor.2019.04.002 doi: 10.1016/j.cor.2019.04.002
![]() |
[113] |
C. Lu, Y. Huang, L. Meng, L. Gao, B. Zhang, J. Zhou, A Pareto-based collaborative multi-objective optimization algorithm for energy-efficient scheduling of distributed permutation flow-shop with limited buffers, Robot Comput. Integr. Manuf., 74 (2022), 102277. https://doi.org/10.1016/j.rcim.2021.102277 doi: 10.1016/j.rcim.2021.102277
![]() |
[114] |
K. Geng, C. Ye, A memetic algorithm for energy-efficient distributed re-entrant hybrid flow shop scheduling problem, J. Intell. Fuzzy Syst., 41 (2021), 3951–3971. https://doi.org/10.3233/JIFS-202963 doi: 10.3233/JIFS-202963
![]() |
[115] |
J. Dong, C. Ye, Green scheduling of distributed two-stage reentrant hybrid flow shop considering distributed energy resources and energy storage system, Comput. Ind. Eng., 169 (2022), 108146. https://doi.org/10.1016/j.cie.2022.108146 doi: 10.1016/j.cie.2022.108146
![]() |
[116] |
Y. Alaouchiche, Y. Ouazene, F. Yalaoui, Economic and energetic performance evaluation of unreliable production lines: An integrated analytical approach, IEEE Access, 8 (2020), 185330–185345. https://doi.org/10.1109/ACCESS.2020.3029761 doi: 10.1109/ACCESS.2020.3029761
![]() |
[117] |
B. Naderi, A. Azab, Modeling and heuristics for scheduling of distributed job shops, Expert Syst. Appl., 41 (2014), 7754–7763. https://doi.org/10.1016/j.eswa.2014.06.023 doi: 10.1016/j.eswa.2014.06.023
![]() |
[118] |
M. L. R. Varela, G. D. Putnik, M. M. Cruz-Cunha, Web-based technologies integration for distributed manufacturing scheduling in a virtual enterprise, Int. J. Web Portals (IJWP), 4 (2012), 19–34. https://doi.org/10.4018/jwp.2012040102 doi: 10.4018/jwp.2012040102
![]() |
[119] |
H. Z. Jia, J. Y. Fuh, A. Y. Nee, Y. F. Zhang, Web-based multi-functional scheduling system for a distributed manufacturing environment, Concurr. Eng., 10 (2002), 27–39. https://doi.org/10.1177/1063293X02010001054 doi: 10.1177/1063293X02010001054
![]() |
[120] |
H. Z. Jia, A. Y. Nee, J. Y. Fuh, Y. F. Zhang, A modified genetic algorithm for distributed scheduling problems, J. Intell. Manuf., 14 (2003), 351–362. https://doi.org/10.1023/A:1024653810491 doi: 10.1023/A:1024653810491
![]() |
[121] |
H. Z. Jia, J. Y. Fuh, A. Y. Nee, Y. F. Zhang, Integration of genetic algorithm and Gantt chart for job shop scheduling in distributed manufacturing systems, Comput. Ind. Eng., 53 (2007), 313–320. https://doi.org/10.1016/j.cie.2007.06.024 doi: 10.1016/j.cie.2007.06.024
![]() |
[122] |
F. T. Chan, S. H. Chung, L. Y. Chan, G. Finke, M. K. Tiwari, Solving distributed FMS scheduling problems subject to maintenance: Genetic algorithms approach, Robot Comput. Integr. Manuf., 22 (2006), 493–504. https://doi.org/10.1016/j.rcim.2005.11.005 doi: 10.1016/j.rcim.2005.11.005
![]() |
[123] |
I. J. Jeong, S. B. Yim, A job shop distributed scheduling based on Lagrangian relaxation to minimise total completion time, Int. J. Prod. Res., 47 (2009), 6783–6805. https://doi.org/10.1080/00207540701824217 doi: 10.1080/00207540701824217
![]() |
[124] |
L. De Giovanni, F. Pezzella, An improved genetic algorithm for the distributed and flexible job-shop scheduling problem, Eur. J. Oper. Res., 200 (2010), 395–408. https://doi.org/10.1016/j.ejor.2009.01.008 doi: 10.1016/j.ejor.2009.01.008
![]() |
[125] |
P. Lou, S. K. Ong, A. Y. C. Nee, Agent-based distributed scheduling for virtual job shops, Int. J. Prod. Res., 48 (2010), 3889–3910. https://doi.org/10.1080/00207540902927918 doi: 10.1080/00207540902927918
![]() |
[126] |
B. Naderi, A. Azab, An improved model and novel simulated annealing for distributed job shop problems, J. Adv. Manuf. Technol., 81 (2015), 693–703. https://doi.org/10.1007/s00170-015-7080-8 doi: 10.1007/s00170-015-7080-8
![]() |
[127] |
T. K. Liu, Y. P. Chen, J. H. Chou, Solving distributed and flexible job-shop scheduling problems for a real-world fastener manufacturer, IEEE Access, 2 (2014), 1598–1606. https://doi.org/10.1109/ACCESS.2015.2388486 doi: 10.1109/ACCESS.2015.2388486
![]() |
[128] |
I. Chaouch, O. B. Driss, K. Ghedira, A novel dynamic assignment rule for the distributed job shop scheduling problem using a hybrid ant-based algorithm, Appl. Intell., 49 (2019), 1903–1924. https://doi.org/10.1007/s10489-018-1343-7 doi: 10.1007/s10489-018-1343-7
![]() |
[129] |
Q. Luo, Q. Deng, G. Gong, L. Zhang, W. Han, K. Li, An efficient memetic algorithm for distributed flexible job shop scheduling problem with transfers, Expert Syst. Appl., 160 (2020), 113721. https://doi.org/10.1016/j.eswa.2020.113721 doi: 10.1016/j.eswa.2020.113721
![]() |
[130] |
J. Q. Li, P. Duan, J. Cao, X. P. Lin, Y. Y. Han, A hybrid Pareto-based tabu search for the distributed flexible job shop scheduling problem with E/T criteria, IEEE Access, 6 (2018), 58883–58897. https://doi.org/10.1109/ACCESS.2018.2873401 doi: 10.1109/ACCESS.2018.2873401
![]() |
[131] |
F. T. Chan, S. H. Chung, P. L. Y. Chan, An adaptive genetic algorithm with dominated genes for distributed scheduling problems, Expert Syst. Appl., 29 (2005), 364–371. https://doi.org/10.1016/j.eswa.2005.04.009 doi: 10.1016/j.eswa.2005.04.009
![]() |
[132] |
L. Meng, C. Zhang, Y. Ren, B. Zhang, C. Lv, Mixed-integer linear programming and constraint programming formulations for solving distributed flexible job shop scheduling problem, Comput. Ind. Eng., 142 (2020), 106347. https://doi.org/10.1016/j.cie.2020.106347 doi: 10.1016/j.cie.2020.106347
![]() |
[133] |
B. Marzouki, O. B. Driss, K. Ghédira, Solving distributed and flexible job shop scheduling problem using a chemical reaction optimization metaheuristic, Procedia Comput. Sci., 126 (2018), 1424–1433. https://doi.org/10.1016/j.procs.2018.08.114 doi: 10.1016/j.procs.2018.08.114
![]() |
[134] |
M. Zandieh, A. R. Khatami, S. H. A. Rahmati, Flexible job shop scheduling under condition-based maintenance: improved version of imperialist competitive algorithm, Appl. Soft Comput., 58 (2017), 449–464. https://doi.org/10.1016/j.asoc.2017.04.060 doi: 10.1016/j.asoc.2017.04.060
![]() |
[135] |
P. H. Lu, M. C. Wu, H. Tan, Y. H. Peng, C. F. Chen, A genetic algorithm embedded with a concise chromosome representation for distributed and flexible job-shop scheduling problems, J. Intell. Manuf., 29 (2018), 19–34. https://doi.org/10.1007/s10845-015-1083-z doi: 10.1007/s10845-015-1083-z
![]() |
[136] |
M. C. Wu, C. S. Lin, C. H. Lin, C. F. Chen, Effects of different chromosome representations in developing genetic algorithms to solve DFJS scheduling problems, Comput. Oper. Res., 80 (2017), 101–112. https://doi.org/10.1016/j.cor.2016.11.021 doi: 10.1016/j.cor.2016.11.021
![]() |
[137] |
H. C. Chang, T. K. Liu, Optimisation of distributed manufacturing flexible job shop scheduling by using hybrid genetic algorithms, J. Intell. Manuf., 28 (2017), 1973–1986. https://doi.org/10.1007/s10845-015-1084-y doi: 10.1007/s10845-015-1084-y
![]() |
[138] |
W. Xu, Y. Hu, W. Luo, L. Wang, R. Wu, A multi-objective scheduling method for distributed and flexible job shop based on hybrid genetic algorithm and tabu search considering operation outsourcing and carbon emission, Comput. Ind. Eng., 157 (2021), 107318. https://doi.org/10.1016/j.cie.2021.107318 doi: 10.1016/j.cie.2021.107318
![]() |
[139] |
L. Meng, Y. Ren, B. Zhang, J. Q. Li, H. Sang, C. Zhang, MILP modeling and optimization of energy-efficient distributed flexible job shop scheduling problem, IEEE Access, 8 (2020), 191191–191203. https://doi.org/10.1109/ACCESS.2020.3032548 doi: 10.1109/ACCESS.2020.3032548
![]() |
[140] |
S. Zhang, X. Li, B. Zhang, S. Wang, Multi-objective optimisation in flexible assembly job shop scheduling using a distributed ant colony system, Eur. J. Oper. Res., 283 (2020), 441–460. https://doi.org/10.1016/j.ejor.2019.11.016 doi: 10.1016/j.ejor.2019.11.016
![]() |
[141] |
F. Xiong, K. Xing, Meta-heuristics for the distributed two-stage assembly scheduling problem with bi-criteria of makespan and mean completion time, Int. J. Prod. Res., 52 (2014), 2743–2766. https://doi.org/10.1080/00207543.2014.884290 doi: 10.1080/00207543.2014.884290
![]() |
[142] |
S. Yang, Z. Xu, The distributed assembly permutation flowshop scheduling problem with flexible assembly and batch delivery. Int. J. Prod. Res., 59 (2021), 4053-4071. https://doi.org/10.1080/00207543.2020.1757174 doi: 10.1080/00207543.2020.1757174
![]() |
[143] |
Z. Shao, W. Shao, D. Pi, Effective constructive heuristic and metaheuristic for the distributed assembly blocking flow-shop scheduling problem, Appl. Intell., 50 (2020), 4647–4669. https://doi.org/10.1007/s10489-020-01809-x doi: 10.1007/s10489-020-01809-x
![]() |
[144] |
W. Niu, J. Q. Li, A two-stage cooperative evolutionary algorithm for energy-efficient distributed group blocking flow shop with setup carryover in precast systems, Knowl. Based Syst., 257 (2022), 109890. https://doi.org/10.1016/j.knosys.2022.109890 doi: 10.1016/j.knosys.2022.109890
![]() |
[145] |
F. Jolai, H. Asefi, M. Rabiee, P. Ramezani, Bi-objective simulated annealing approaches for no-wait two-stage flexible flow shop scheduling problem, Sci. Iran., 20 (2013), 861–872. https://doi.org/10.1016/j.scient.2012.10.044 doi: 10.1016/j.scient.2012.10.044
![]() |
[146] |
J. Deng, L. Wang, S. Y. Wang, X. L. Zheng, A competitive memetic algorithm for the distributed two-stage assembly flow-shop scheduling problem, Int. J. Prod. Res., 54 (2016), 3561–3577. https://doi.org/10.1080/00207543.2015.1084063 doi: 10.1080/00207543.2015.1084063
![]() |
[147] |
F. Nikzad, J. Rezaeian, I. Mahdavi, I. Rastgar, Scheduling of multi-component products in a two-stage flexible flow shop, Appl. Soft Comput., 32 (2015), 132–143. https://doi.org/10.1016/j.asoc.2015.03.006 doi: 10.1016/j.asoc.2015.03.006
![]() |
[148] |
A. Azadeh, M. Jeihoonian, B. M. Shoja, S. H. Seyedmahmoudi, An integrated neural network-simulation algorithm for performance optimisation of the bi-criteria two-stage assembly flow-shop scheduling problem with stochastic activities, Int. J. Prod. Res., 50 (2012), 7271–7284. https://doi.org/10.1080/00207543.2011.645511 doi: 10.1080/00207543.2011.645511
![]() |
[149] |
F. Zhao, D. Shao, L. Wang, T. Xu, N. Zhu, An effective water wave optimization algorithm with problem-specific knowledge for the distributed assembly blocking flow-shop scheduling problem, Knowl. Based Syst., 243 (2022), 108471. https://doi.org/10.1016/j.knosys.2022.108471 doi: 10.1016/j.knosys.2022.108471
![]() |
[150] |
G. Zhang, K. Xing, Memetic social spider optimization algorithm for scheduling two-stage assembly flowshop in a distributed environment, Comput. Ind. Eng., 125 (2018), 423–433. https://doi.org/10.1016/j.cie.2018.09.007 doi: 10.1016/j.cie.2018.09.007
![]() |
[151] |
D. Lei, B. Su, M. Li, Cooperated teaching-learning-based optimisation for distributed two-stage assembly flow shop scheduling, Int. J. Prod. Res., 59 (2021), 7232–7245. https://doi.org/10.1080/00207543.2020.1836422 doi: 10.1080/00207543.2020.1836422
![]() |
[152] |
N. Shoaardebili, P. Fattahi, Multi-objective meta-heuristics to solve three-stage assembly flow shop scheduling problem with machine availability constraints, Int. J. Prod. Res., 53 (2015), 944–968. https://doi.org/10.1080/00207543.2014.948575 doi: 10.1080/00207543.2014.948575
![]() |
[153] |
Y. Du, J. Q. Li, C. Luo, L. L. Meng, A hybrid estimation of distribution algorithm for distributed flexible job shop scheduling with crane transportations, Swarm Evol. Comput., 62 (2021), 100861. https://doi.org/10.1016/j.swevo.2021.100861 doi: 10.1016/j.swevo.2021.100861
![]() |
[154] |
X. Wu, X. Liu, N. Zhao, An improved differential evolution algorithm for solving a distributed assembly flexible job shop scheduling problem, Memet. Comput., 11 (2019), 335–355. https://doi.org/10.1007/s12293-018-00278-7 doi: 10.1007/s12293-018-00278-7
![]() |
[155] |
G. Zhang, K. Xing, G. Zhang, Z. He, Memetic algorithm with meta-Lamarckian learning and simplex search for distributed flexible assembly permutation flowshop scheduling problem, IEEE Access, 8 (2020), 96115–96128. https://doi.org/10.1109/ACCESS.2020.2996305 doi: 10.1109/ACCESS.2020.2996305
![]() |
1. | Jean Pierre II Kouenkam, Joseph Mbang, Yves Emvudu, Global dynamics of a model of hepatitis B virus infection in a sub-Saharan African rural area, 2020, 13, 1793-5245, 2050054, 10.1142/S1793524520500540 | |
2. | Getachew Teshome Tilahun, Woldegebriel Assefa Woldegerima, Nesredin Mohammed, A fractional order model for the transmission dynamics of hepatitis B virus with two-age structure in the presence of vaccination, 2021, 28, 2576-5299, 87, 10.1080/25765299.2021.1896423 | |
3. | Chao Liu, Peng Chen, Qiyu Jia, Lora Cheung, Effects of Media Coverage on Global Stability Analysis and Optimal Control of an Age-Structured Epidemic Model with Multi-Staged Progression, 2022, 10, 2227-7390, 2712, 10.3390/math10152712 | |
4. | Jianquan Li, Yuming Chen, Peijun Zhang, Dian Zhang, Global Stability of a Viral Infection Model with Defectively Infected Cells and Latent Age, 2024, 45, 0252-9599, 555, 10.1007/s11401-024-0028-2 | |
5. | Salah Boulaaras, Aasim Ullah Jan, Tahir Hassan, Taha Radwan, Mathematical modeling and computational analysis of hepatitis B virus transmission using the higher-order Galerkin scheme, 2024, 13, 2192-8029, 10.1515/nleng-2024-0048 |