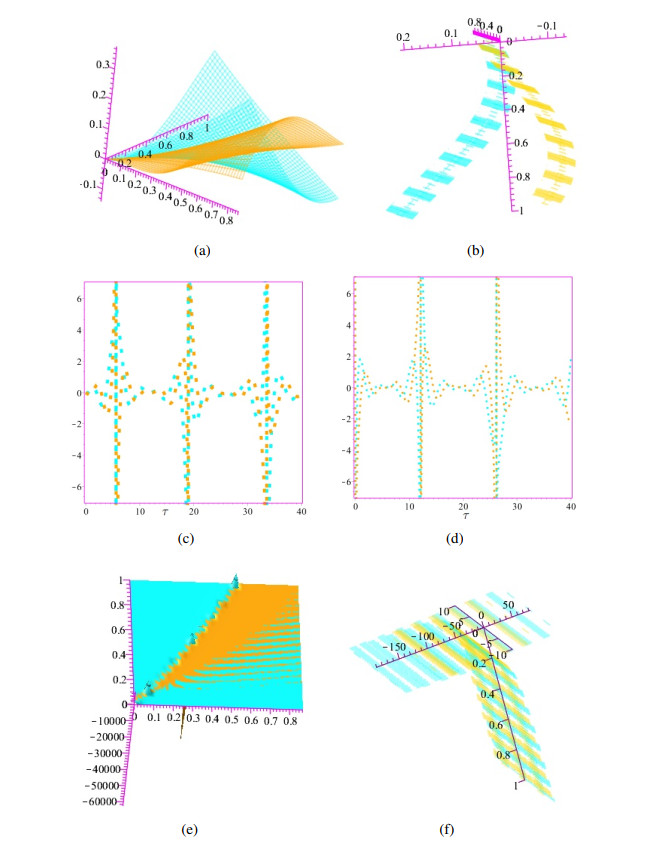
Taxi detour is a chronic problem in urban transport systems, which largely undermines passengers' riding experience and the city's image while unnecessarily worsening traffic congestion. Tourists unfamiliar with city roads often encounter detour problems. Therefore, it is important for regulatory authorities to develop a tool for detour behavior detection in order to discover or identify detours. This study proposes a detour trajectory detection model framework based on the trajectory data of taxis that can identify taxi driving detour fraud at the microscopic level and analyze the characteristics of detouring trajectories from the perspective of microscopic motion traits. The deviation from normal driving trajectories provides a framework for the automatic detection of detour trajectories for the off-site supervision platform of the taxis. Considering drawbacks of the isolation-Based Anomalous Trajectory (iBAT) algorithm, this paper made further improvements in trajectory anomaly detection. In this study, three methods including the iBAT, iBAT + Dynamic Time Warping (DTW), and iBAT + DTW algorithms considering the driving distance and time are compared using the relevant experimental data. The case studies verify that the proposed method outperforms the other methods. Verified by the experiments based on the trajectory data coming from Nanjing, the false positive rate of this framework is only 1.64%.
Citation: Jian Wan, Peiyun Yang, Wenbo Zhang, Yaxing Cheng, Runlin Cai, Zhiyuan Liu. A taxi detour trajectory detection model based on iBAT and DTW algorithm[J]. Electronic Research Archive, 2022, 30(12): 4507-4529. doi: 10.3934/era.2022229
[1] | Yaya Wang, Md Nurul Raihen, Esin Ilhan, Haci Mehmet Baskonus . On the new sine-Gordon solitons of the generalized Korteweg-de Vries and modified Korteweg-de Vries models via beta operator. AIMS Mathematics, 2025, 10(3): 5456-5479. doi: 10.3934/math.2025252 |
[2] | Wei Gao, Gulnur Yel, Haci Mehmet Baskonus, Carlo Cattani . Complex solitons in the conformable (2+1)-dimensional Ablowitz-Kaup-Newell-Segur equation. AIMS Mathematics, 2020, 5(1): 507-521. doi: 10.3934/math.2020034 |
[3] | Zeliha Korpinar, Mustafa Inc, Ali S. Alshomrani, Dumitru Baleanu . The deterministic and stochastic solutions of the Schrodinger equation with time conformable derivative in birefrigent fibers. AIMS Mathematics, 2020, 5(3): 2326-2345. doi: 10.3934/math.2020154 |
[4] | Maheen Waqar, Khaled M. Saad, Muhammad Abbas, Miguel Vivas-Cortez, Waleed M. Hamanah . Diverse wave solutions for the (2+1)-dimensional Zoomeron equation using the modified extended direct algebraic approach. AIMS Mathematics, 2025, 10(6): 12868-12887. doi: 10.3934/math.2025578 |
[5] | M. Ali Akbar, Norhashidah Hj. Mohd. Ali, M. Tarikul Islam . Multiple closed form solutions to some fractional order nonlinear evolution equations in physics and plasma physics. AIMS Mathematics, 2019, 4(3): 397-411. doi: 10.3934/math.2019.3.397 |
[6] | Mustafa Inc, Hadi Rezazadeh, Javad Vahidi, Mostafa Eslami, Mehmet Ali Akinlar, Muhammad Nasir Ali, Yu-Ming Chu . New solitary wave solutions for the conformable Klein-Gordon equation with quantic nonlinearity. AIMS Mathematics, 2020, 5(6): 6972-6984. doi: 10.3934/math.2020447 |
[7] | M. Hafiz Uddin, M. Ali Akbar, Md. Ashrafuzzaman Khan, Md. Abdul Haque . New exact solitary wave solutions to the space-time fractional differential equations with conformable derivative. AIMS Mathematics, 2019, 4(2): 199-214. doi: 10.3934/math.2019.2.199 |
[8] | Abdullahi Yusuf, Tukur A. Sulaiman, Mustafa Inc, Sayed Abdel-Khalek, K. H. Mahmoud . M−truncated optical soliton and their characteristics to a nonlinear equation governing the certain instabilities of modulated wave trains. AIMS Mathematics, 2021, 6(9): 9207-9221. doi: 10.3934/math.2021535 |
[9] | Muhammad Bilal, Javed Iqbal, Ikram Ullah, Aditi Sharma, Hasim Khan, Sunil Kumar Sharma . Novel optical soliton solutions for the generalized integrable (2+1)- dimensional nonlinear Schrödinger system with conformable derivative. AIMS Mathematics, 2025, 10(5): 10943-10975. doi: 10.3934/math.2025497 |
[10] | M. TarikulIslam, M. AliAkbar, M. Abul Kalam Azad . Traveling wave solutions in closed form for some nonlinear fractional evolution equations related to conformable fractional derivative. AIMS Mathematics, 2018, 3(4): 625-646. doi: 10.3934/Math.2018.4.625 |
Taxi detour is a chronic problem in urban transport systems, which largely undermines passengers' riding experience and the city's image while unnecessarily worsening traffic congestion. Tourists unfamiliar with city roads often encounter detour problems. Therefore, it is important for regulatory authorities to develop a tool for detour behavior detection in order to discover or identify detours. This study proposes a detour trajectory detection model framework based on the trajectory data of taxis that can identify taxi driving detour fraud at the microscopic level and analyze the characteristics of detouring trajectories from the perspective of microscopic motion traits. The deviation from normal driving trajectories provides a framework for the automatic detection of detour trajectories for the off-site supervision platform of the taxis. Considering drawbacks of the isolation-Based Anomalous Trajectory (iBAT) algorithm, this paper made further improvements in trajectory anomaly detection. In this study, three methods including the iBAT, iBAT + Dynamic Time Warping (DTW), and iBAT + DTW algorithms considering the driving distance and time are compared using the relevant experimental data. The case studies verify that the proposed method outperforms the other methods. Verified by the experiments based on the trajectory data coming from Nanjing, the false positive rate of this framework is only 1.64%.
The notion of fractional derivative (FD) is more than three thousand years old, the role of fractional calculus has been increasing due to its application zone in various domains including biology, semiconductor industry, optical communication, energy quantization, quantum chemistry, wave propagation, protein folding and bending, condensed matter physics, solid state physics, nanotechnology and industry, laser propagation, nonlinear optics etc. the fractional differential equations (FDEs), have received a great deal of interest from scholars and researchers. Many mathematicians presented diverse types of FDs, such as that given in [1,2]. The most famous ones are Hadamard, Marchaud, Riemann-Liouville, Grunwald-Letnikov, Kober, Caputo, Riesz and Erdelyi.
Lately, a novel FD has been presented by Khalil et al. [3] and others [4,5,6], called conformable FD. Due to the significance of the exact solutions of NLSEs, plenty of mathematicians solved them with conformable derivative, who used the different methods like first integral method (FIM) [7,8], functional variable method (FVM) [7,9], trial equation method (TEM) [10], modified trial equation method (MTEM) [11], direct algebraic method (DAM) [12] and sine-Gordon expansion method (SGEM) [13] to find the exact solutions to NLSEs.
In [14], Younas et al. presented CTFMNLSE and studied the exact solutions of it by the generalized exponential rational function method. Also in [15,16], authors introduced some new solutions of CTFMNLSE via FIM, FVM, TEM and MTEM.
Motivated by the work done in [14,15,16], we consider the following CTFMNLSE:
iDατΨ+σ1ΨXX+σ2|Ψ|2Ψ=iδ1ΨXXX+iδ2Ψ2Ψ∗X−iδ3|Ψ|2ΨX+δ4Ψ0<α≤1, | (1.1) |
where σ1=P08K20(−3cos(Θ)+2), σ2=−P0K202, δ1=P0cos(Θ)16K30(−5cos2(Θ)−6), δ2=P0K0cos(Θ)4, δ3=3P0K02,δ4=K0|Ψ|2X|X=0, P0 and K0 are the frequency and the wave number of the carrier wave, respectively, and the operator Dα of order α, where α∈(0,1] represents the conformable fractional derivative.
In this section, we present some properties and definitions of the conformal derivative and other Preliminaries.
Definition 2.1. [3] Suppose Ω:(0,∞)→R is a function. Therefore the conformal fractional derivative of Ω of order α is as follows
Tα(τ)=limϵ→0Ω(τ+ϵτ1−α)−Ω(τ)ϵ | (2.1) |
for all 0<α<1,0<τ.
Definition 2.2. [3] Suppose ι≥0 and τ≥ι. Also, suppose Ω is a function defined on (ι,τ] and α∈R. Therefore, the α-fractional integral of Ω is defined by,
IαιΩ(τ)=∫τιΩ(ς)ς1−αdς, | (2.2) |
if the Riemann improper integral exists.
Theorem 2.3. [3]Suppose 0<α≤1, and Ω and ℧ are α−differentiable at a point τ, therefore
(i) Tα(ϖ1Ω+ϖ2℧)=ϖ1Tα(Ω)+ϖ2Tα(℧),∀ϖ1,ϖ2∈R.
(ii) Tα(tϖ)=ϖτϖ−α,∀ϖ∈R.
(iii) τTα(Ω℧)=ΩTα(℧)+℧Tα(Ω).
(iv) Tα(Ω℧)=℧Tα(Ω)−ΩTα(℧)℧2.
Furthermore, if Ω is differentiable, then Tα(Ω)(τ)=τ1−αdΩdτ.
Theorem 2.4. [3] Suppose Ω:(0,∞)→R is a function s.t. Ω is differentiable and also α-differentiable. Suppose ℧ is a function defined in the range of Ω and also differentiable; therefore, one has the following rule
Tα(Ωo℧)(τ)=τ1−α℧(τ)Ω′(℧(τ)). |
Remark 2.5. Let
Q′(ξ)=Ln(A)(P0+P1Q(ξ)+P2Q2(ξ)),A≠0,1. | (2.3) |
The solutions of ODE (2.3) are:
(1) When P21−4P0P2<0 and P2≠0,
Q1(ξ)=−P12P2+√−(P21−4P0P2)2P2tanA(√−(P21−4P0P2)2ξ), |
Q2(ξ)=−P12P2−√−(P21−4P0P2)2P2cotA(√−(P21−4P0P2)2ξ), |
Q3(ξ)=−P12P2+√−(P21−4P0P2)2P2tanA(√−(P21−4P0P2)ξ)±√−sr(P21−4P0P2)2P2secA(√−(P21−4P0P2)ξ), |
Q4(ξ)=−P12P2−√−(P21−4P0P2)2P2cotA(√−(P21−4P0P2)ξ)±√−sr(P21−4P0P2)2P2cscA(√−(P21−4P0P2)ξ), |
Q5(ξ)=−P12P2+√−(P21−4P0P2)4P2tanA(√−(P21−4P0P2)4ξ)−√−(P21−4P0P2)4P2cotA(√−(P21−4P0P2)4ξ), |
(2) When P21−4P0P2>0 and P2≠0,
Q6(ξ)=−P12P2−√P21−4P0P22P2tanhA(√P21−4P0P22ξ), |
Q7(ξ)=−P12P2−√P21−4P0P22P2cothA(√P21−4P0P22ξ), |
Q8(ξ)=−P12P2−√P21−4P0P22P2tanhA(√P21−4P0P2ξ)±i√sr(P21−4P0P2)2P2sechA(√P21−4P0P2ξ), |
Q9(ξ)=−P12P2−√P21−4P0P22P2cothA(√P21−4P0P2ξ)±√sr(P21−4P0P2)2P2cschA(√P21−4P0P2ξ), |
Q10(ξ)=−P12P2−√P21−4P0P24P2tanhA(√P21−4P0P24ξ)−√P21−4P0P24P2cothA(√P21−4P0P24ξ), |
where generalized hyperbolic and triangular functions are given by
coshA(θ)=sAθ+rA−θ2,sinhA(θ)=sAθ−rA−θ2, |
cothA(θ)=sAθ+rA−θseθ−re−θ,tanhA(θ)=sAθ−rA−θseθ+re−θ, |
cschA(θ)=2sAθ−rA−θ,sechA(θ)=2sAθ+rA−θ, |
cosA(θ)=sAiθ+rA−iθ2i,sinA(θ)=sAiθ−rA−iθ2i, |
cotA(θ)=isAiθ+rA−iθsAiθ−rA−iθ,tanA(θ)=−isAiθ−rA−iθsAiθ+rA−iθ, |
cscA(θ)=2isAiθ−rA−iθ,secA(θ)=2sAiθ+rA−iθ, |
where θ is an independent variable, A≠0,1, and s and r are arbitrary constants greater than zero and are called deformation parameters.
In this section, we present the first step of the DAM and the SGEM, for finding analytical solutions of CTFMNLSE defined as (1.1). Suppose a CTFNLPDE,
Γ(Φ,Φτ,ΦX,DατΦ,DβXΦ,D2ατ,D2βX,…)=0,0<α,β≤1, | (3.1) |
where Γ and Φ are a polynomial and an unknown function in its arguments, respectively. Using a fractional travelling wave transformation
Φ(X,τ)=Λ(ξ),ξ=X−Vατα | (3.2) |
where V is velocity and substituting (3.2) into (3.1), we have a NLODE given by
Υ(Λ,Λ′,Λ′′,Λ′′′,…)=0, | (3.3) |
in which ′ signifies the derivative with respect to ξ.
Since Ψ=Ψ(X,τ) in (1.1) is a complex function, we begin with the following travelling wave assumption
Ψ(X,τ)=Λ(ξ)eiψ | (3.4) |
where ξ=η(X−Vατα) and ψ=−KX+Pατα+ζ, and ζ,P and K are parameters, represent the phase constant, frequency and wave number respectively. Substitute (3.4) into (1.1), we get real and imaginary parts as follows
η2(σ1−3δ1K)Λ′′+(σ2+(δ2+δ3)K)Λ3+(−P−σ1K2+δ1K3−δ4)Λ=0, | (3.5) |
and
(3δ1K2−V−2σ1K)Λ′−δ1η2Λ′′′+(δ3−δ2)Λ2Λ′=0. | (3.6) |
Now integrating the imaginary part of the equation and taking constant equal to zero one may have
3(3δ1K2−V−2σ1K)Λ−3δ1η2Λ′′+(δ3−δ2)Λ3=0. | (3.7) |
From (3.5) and (3.7), it can be followed that
K3δ1−P−σ1K2−δ43(3δ1K2−V−2σ1)K=σ1−3δ1K−3δ1=Kδ2+Kδ3+σ2δ3−δ2. | (3.8) |
From above, it can be followed that
V=−δ1P+δ1δ4+2K(σ1−2δ1K)2σ1−3δ1K, |
K=σ1(δ2−δ3)−3σ2δ16δ1δ2. |
Rewrite (3.5) into following form
Λ′′+λ1Λ3−λ2Λ=0 | (3.9) |
or
Λ′′=λ2Λ−λ1Λ3 | (3.10) |
where λ1=σ2+(δ2+δ3)Kη2(σ1−3δ1K) and λ2=−−P−σ1K2+δ1K3−δ4η2(σ1−3δ1K).
In the next two subsections, we investigate the primary steps for detecting the exact solution of (3.5) by using DAM and the SGEM. Similarly we can find the exact solution of (3.7).
Firstly, CTFNLPDE (3.1) is reduced to NLODE (3.3) under the transformation (3.2). Secondly, let us consider that Eq (3.3) has a formal solution of the form
Λ(ξ)=N∑j=0bjQj(ξ),bN≠0, | (3.11) |
where bj(j=0,…,N) are constant coefficients to be detected later and Q(ξ) satisfies the ODE in the form (2.3). Now, we are able to determine the value N in (3.11) by balancing the highest order derivative term and the highest order nonlinear term in (3.3). Substitute (3.11) along with its required derivatives into (3.3) and compares the coefficients of powers of Q(ξ) in the resultant equation for getting the set of algebraic equation. In the end, we {solve} the set of algebraic equations and put the results generated in (3.11) to obtain the exact solutions of (3.1).
Now, balancing the order of Λ′′ and Λ3 in (3.10), we get N=1. Therefore, Eq (3.11) is presented by
Λ(ξ)=b0+b1Q(ξ). | (3.12) |
By substituting (3.12) into (3.10) and gathering all terms with the same order of Q(ξ) together, the left-hand side of (3.10) are converted into polynomial in Q(ξ). {Putting} every coefficient of every polynomial to zero, we get a set of algebraic equations for b0 and b1. Now, we have
Λ3=b30+3b20b1Q+3b0b21Q2+b31Q3andΛ′′=b1Q′′, | (3.13) |
where
Q′′=Ln2(A)(P0+P1Q+P2Q2)[P1+2P2Q]. | (3.14) |
Coefficients of Q(ξ) as follows:
∁0:b1Ln2(A)P0P1+λ1b30−λ2b0=0∁1:b1Ln2(A)[P21+2P0P2]+3λ1b20b1−λ2b1=0∁2:3b1Ln2(A)P1P2+3λ1b0b21=0∁3:2b1Ln2(A)P22+λ1b31=0. |
We earn the following values, by solving the above system of equations for b0 and b1:
b0=±i√2λ1Ln(A)P1,b1=±i√2λ1Ln(A)P2. | (3.15) |
The solutions of (1.1) corresponding to (3.4), (3.12) and (3.15) are:
(1) When P21−4P0P2<0, and P2≠0,
Ψ1,2(X,τ)=(±i)ln(A)√−(P21−4P0P2)√2λ1ei(−KX+Pατα+ζ)tanA(√−(P21−4P0P2)2(η(X−Vατα)+ξ0)), |
Ψ1,3(X,τ)=(±i)ln(A)√−(P21−4P0P2)√2λ1ei(−KX+Pατα+ζ)cotA(√−(P21−4P0P2)2(η(X−Vατα)+ξ0)), |
Ψ1,4(X,τ)=(±i)ln(A)ei(−KX+Pατα+ζ)[√−(P21−4P0P2)√2λ1tanA(√−(P21−4P0P2)(η(X−Vατα)+ξ0))±√−sr(P21−4P0P2)√2λ1secA(√−(P21−4P0P2)(η(X−Vατα)+ξ0))], |
Ψ1,5(X,τ)=(±i)ln(A)ei(−KX+Pατα+ζ)[−√−(P21−4P0P2)√2λ1cotA(√−(P21−4P0P2)(η(X−Vατα)+ξ0))±√−sr(P21−4P0P2)√2λ1cscA(√−(P21−4P0P2)(η(X−Vατα)+ξ0)), |
Ψ1,6(X,τ)=(±i)ln(A)ei(−KX+Pατα+ζ)[√−(P21−4P0P2)√8λ1tanA(√−(P21−4P0P2)4(η(X−Vατα)+ξ0))−√−(P21−4P0P2)√8λ1cotA(√−(P21−4P0P2)4(η(X−Vατα)+ξ0))], |
(2) When P21−4P0P2>0, and P2≠0,
Ψ1,7(X,τ)=(±i)ln(A)√P21−4P0P2√2λ1ei(−KX+Pατα+ζ)tanhA(√P21−4P0P22(η(X−Vατα)+ξ0)), |
Ψ1,8(X,τ)=(±i)ln(A)√P21−4P0P2√2λ1ei(−KX+Pατα+ζ)cothA(√P21−4P0P22(η(X−Vατα)+ξ0)), |
Ψ1,9(X,τ)=(±i)ln(A)ei(−KX+Pατα+ζ)[−√P21−4P0P2√2λ1tanhA(√P21−4P0P2(η(X−Vατα)+ξ0))±i√sr(P21−4P0P2)√2λ1sechA(√P21−4P0P2(η(X−Vατα)+ξ0))], |
Ψ1,10(X,τ)=±ei(−KX+Pατα+ζ)[−√P21−4P0P2√2λ1cothA(√P21−4P0P2(η(X−Vατα)+ξ0))±√sr(P21−4P0P2)√2λ1cschA(√P21−4P0P2(η(X−Vατα)+ξ0))], |
Ψ1,11(X,τ)=(±i)ln(A)ei(−KX+Pατα+ζ)[√P21−4P0P2√8λ1tanhA(√P21−4P0P24(η(X−Vατα)+ξ0))+√P21−4P0P2√8λ1cothA(√P21−4P0P24(η(X−Vατα)+ξ0))], |
where ξ0 is an arbitrary constant. For more details see [17,18].
Consider the sine-Gordon equation from [19,20]
ΨXX−Ψττ=m2sin(Ψ), | (3.16) |
where Ψ=Ψ(X,τ) and m is a constant. To solve the equation through sine-Gordon expansion algorithm, first we use the transformation Ψ(X,τ)=Λ(ξ) where ξ=μ(X−cτ) which reduce (3.16) to the following NLODE:
Λ′′=m2μ2(1−c2)sin(Λ). | (3.17) |
After that, we multiply Λ′ on both sides of (3.17) and integrate it once which gives
[(Λ2)]2=m2μ2(1−c2)sin2(Λ2)+k, | (3.18) |
in which k is an integration constant. Therefore by putting k=0,Λ2=w(ξ), and m2μ2(1−c2)=a2 in (3.18), we get
w′=asin(w), | (3.19) |
which by setting a=1 in (3.19), we have
w′=sin(w). | (3.20) |
Equation (3.20) is a simplified form of the sine-Gordon Eq (3.16). Thus, it has the following solutions:
sin(w)=sech(ξ),cos(w)=tanh(ξ), | (3.21) |
and
sin(w)=icsch(ξ),cos(w)=coth(ξ). | (3.22) |
Here, firstly, CTFNLPDE (3.1) is reduced to NLODE (3.3) under the transformation (3.2). Secondly, we apply the following transformation
Λ(w)=N∑j=1cosj−1(w)[Bjsin(w)+Ajcos(w)]+A0. | (3.23) |
It is supposed that the solution Λ(ξ) of the nonlinear (3.3) along with (3.21) and (3.22) can be demonstrated as
Λ(ξ)=N∑j=1tanhj−1(ξ)[Bjsech(ξ)+Ajtanh(ξ)]+A0. | (3.24) |
and
Λ(ξ)=N∑j=1cothj−1(ξ)[Bjcsch(ξ)+Ajcoth(ξ)]+A0. | (3.25) |
After detecting the value of N by means of using the homogeneous balance principle, substituting its value into (3.23) and setting the result into the reduced ODE (3.20) give a nonlinear algebraic system. Equating the coefficients of sinj(w) and cosj(w) equal to zero and solving the acquired system yield the values of Aj and Bj. Finally, after substituting the values of Aj and Bj into (3.24) and (3.25), we are able to earn the solitary wave solutions for (3.1).
We used the balancing technique to Eq (3.10) by considering the highest derivative Λ′′ and the highest power nonlinear term Λ3, which the value of N is gained as N+2=3N or N=1. Thus, we have the following equations
Λ(w)=B1sin(w)+A1cos(w)+A0, | (3.26) |
Λ′(w)=B1cos(w)sin(w)−A1sin2(w), | (3.27) |
and
Λ′′(w)=B1[cos2(w)sin(w)−sin3(w)]−2A1sin2(w)cos(w). | (3.28) |
Also we have
Λ3=B31sin3(w)+A31cos3(w)+3A0B21sin2(w)+3A21A0cos2(w)+3A20B1sin(w)+3A20A1cos(w)+3A1B21sin2(w)cos(w)+3A21B1cos2(w)sin(w)+6A0A1B1sin(w)cos(w)+A30. | (3.29) |
By substituting (3.26)–(3.29) into (3.10), we obtain the following nonlinear algebraic system:
sin3(w):−B1+λ1B31=0,cos3(w):λ1A31=0,sin2(w):3λ1A0B21=0,cos2(w)3λ1A1A0=0,sin(w):3λ1A20B1−λ2B1=0cos(w):3λ1A20A1−λ2A1=0,sin2(w)cos(w):−2A1+3λ1A1B21=0,cos2(w)sin(w):B1+3λ1A21B1=0,sin(w)cos(w):6λ1A0A1B1=0,sin0(w)cos0(w):λ1A30−λ2A0=0. |
Using (3.21) and (3.26), we earn the following traveling wave solutions:
Case 1: A0=±√λ2λ1or±√λ23λ1or0,A1=±√−13λ1,B1=0.
Ψ2,1=ei(−KX+Pατα+ζ)[±√−13λ1tanh(η(X−Vατα)+ξ0)+A0], | (3.30) |
Ψ2,2=ei(−KX+Pατα+ζ)[±√−13λ1coth(η(X−Vατα)+ξ0)+A0]. | (3.31) |
Case 2: A0=±√λ2λ1or±√λ23λ1or0,A1=0,B1=±√23λ1.
Ψ2,3=ei(−KX+Pατα+ζ)[±√23λ1sech(η(X−Vατα)+ξ0)+A0], | (3.32) |
Ψ2,4=ei(−KX+Pατα+ζ)[±i√23λ1csch(η(X−Vατα)+ξ0)+A0]. | (3.33) |
Case 3: A0=±√λ2λ1or±√λ23λ1or0,A1=0,B1=±√1λ1.
Ψ2,5=ei(−KX+Pατα+ζ)[±√1λ1sech(η(X−Vατα)+ξ0)+A0], | (3.34) |
Ψ2,6=ei(−KX+Pατα+ζ)[±i√1λ1csch(η(X−Vατα)+ξ0)+A0]. | (3.35) |
Case 4: A0=±√λ2λ1or±√λ23λ1or0,A1=±√−13λ1,B1=±√1λ1.
Ψ2,7=ei(−KX+Pατα+ζ)[±√1λ1sech(η(X−Vατα)+ξ0)±√−13λ1tanh(η(X−Vατα)+ξ0)+A0], | (3.36) |
Ψ2,8=ei(−KX+Pατα+ζ)[±i√1λ1csch(η(X−Vατα)+ξ0)±√−13λ1coth(η(X−Vατα)+ξ0)+A0]. | (3.37) |
Case 5: A0=±√λ2λ1or±√λ23λ1or0,A1=±√−13λ1,B1=±√23λ1.
Ψ2,9=ei(−KX+Pατα+ζ)[±√23λ1sech(η(X−Vατα)+ξ0)±√−13λ1tanh(η(X−Vατα)+ξ0)+A0], | (3.38) |
Ψ2,10=ei(−KX+Pατα+ζ)[±i√23λ1csch(η(X−Vατα)+ξ0)±√−13λ1coth(η(X−Vατα)+ξ0)+A0], | (3.39) |
where ξ0 is an arbitrary constant. For more details, see [21,22].
Let δ1=δ3=η=s=r=P0=P1=P2=1,P=δ2=δ4=σ2=A=2,ζ=V=0.5,K=0.25,σ1=−1,α=0.90,ξ0=A0=0. Therefore, we have λ1=−1.57143 and λ2=−2.24107. We now present numerical results in tables and charts.
Figure 1 (a) and (e) show the 3D with the both real and imaginary parts of the solution Ψ1,1 and Ψ1,3 for different values of X and τ and also, Figure 1 (b), (f) and (c), (d) show the 3D and 2D with the both real and imaginary part of the solution Ψ1,1 and Ψ1,3 for fixed X and different values of τ through DAM, using the above values. Now, Figure 2 (a) and (c) show the 3D with the both real and imaginary parts of the solution Ψ2,1 and Ψ2,3 for different values of X and τ and also Figure 2 (b) and (d) show the 3D with the both real and imaginary part of the solution Ψ2,1 and Ψ2,3 for fixed X and different values of τ through SGEM, using the above values.
Moreover, Figure 3 displays the 3D with the real and imaginary part of solution Ψ2,1 for fixed X and different values of α(=0.50−0.09), obtained via SGEM.
Figure 4 (a) shows the 3D with the differences between the real and also the imaginary part of solutions Ψ1,1 and Ψ2,1, and also Figure 4 (b) shows the 3D with the differences between the real and also the imaginary part of solutions Ψ1,3 and Ψ2,3, for fixed X and fixed α. Note that, these differences are minor in a wide range of domains. which implies that both methods leads to similar results except for some values.
Tables 1–4 present the numerical results of the solutions of CTFMNLSE (1.1) obtained by DAM and SGEM with several point sources trough arbitrary.
X | τ | Ψℜ1,1(X,τ) | Ψℜ1,2(X,τ) | Ψℜ1,3(X,τ) | Ψℜ1,4(X,τ) |
0.012 | 0.00058 | -0.00058 | 587.19354 | -587.19354 | |
0.012 | 0.037 | -0.00550 | 0.00550 | -55.91444 | 55.91444 |
0.062 | -0.01056 | 0.01056 | -26.29075 | 26.29075 | |
0.012 | 0.00933 | -0.00933 | 36.46445 | -36.46445 | |
0.037 | 0.037 | 0.00284 | -0.00284 | 109.34814 | -109.34814 |
0.062 | -0.00266 | 0.00266 | -105.25186 | 105.25186 | |
0.012 | 0.01816 | -0.01816 | 18.87874 | -18.87874 | |
0.062 | 0.037 | 0.01124 | -0.01124 | 27.81915 | -27.81915 |
0.062 | 0.00531 | -0.00531 | 53.34247 | -53.34247 |
X | τ | Ψℑ1,1(X,τ) | Ψℑ1,2(X,τ) | Ψℑ1,3(X,τ) | Ψℑ1,4(X,τ) |
0.012 | 0.00034 | -0.00034 | 350.78189 | -350.78189 | |
0.012 | 0.037 | -0.00385 | 0.00385 | -39.18969 | 39.18969 |
0.062 | -0.00852 | 0.00852 | -21.21368 | 21.21368 | |
0.012 | 0.00549 | -0.00549 | 21.47530 | -21.47530 | |
0.037 | 0.037 | 0.00196 | -0.00196 | 75.62594 | -75.62594 |
0.062 | -0.00212 | 0.00212 | -83.84577 | 83.84577 | |
0.012 | 0.01054 | -0.01054 | 10.96007 | -10.96007 | |
0.062 | 0.037 | 0.00767 | -0.00767 | 18.98398 | -18.98398 |
0.062 | 0.00417 | -0.00417 | 41.95143 | -41.95143 |
X | τ | Ψℜ2,1(X,τ) | Ψℜ2,2(X,τ) | Ψℜ2,3(X,τ) | Ψℜ2,4(X,τ) |
0.012 | 0.00065 | -0.00065 | 241.48348 | -241.48348 | |
0.012 | 0.037 | -0.00624 | 0.00624 | -22.75660 | 22.75660 |
0.062 | -0.01197 | 0.01197 | -10.70930 | 10.70930 | |
0.012 | 0.01055 | -0.01055 | 14.88481 | -14.88481 | |
0.037 | 0.037 | 0.00320 | -0.00320 | 44.79798 | -44.79798 |
0.062 | -0.00304 | 0.00304 | -42.62661 | 42.62661 | |
0.012 | 0.02052 | -0.02052 | 7.71125 | -7.71125 | |
0.062 | 0.037 | 0.01270 | -0.01270 | 11.36482 | -11.36482 |
0.062 | 0.00599 | -0.00599 | 21.83731 | -21.83731 |
X | τ | Ψℑ2,1(X,τ) | Ψℑ2,2(X,τ) | Ψℑ2,3(X,τ) | Ψℑ2,4(X,τ) |
0.012 | 0.00038 | -0.00038 | 144.25913 | -144.25913 | |
0.012 | 0.037 | -0.00437 | 0.00437 | -15.94980 | 15.94980 |
0.062 | -0.00965 | 0.00965 | -8.64120 | 8.64120 | |
0.012 | 0.00621 | -0.00621 | 8.76623 | -8.76623 | |
0.037 | 0.037 | 0.00221 | -0.00221 | 30.98259 | -30.98259 |
0.062 | -0.00241 | 0.00241 | -33.95722 | 33.95722 | |
0.012 | 0.01191 | -0.01191 | 4.47677 | -4.47677 | |
0.062 | 0.037 | 0.00866 | -0.00866 | 7.75543 | -7.75543 |
0.062 | 0.00471 | -0.00471 | 17.17404 | -17.17404 |
In Table 5, based on Tables 1–4, separately, we calculate the differences between solutions Ψ1,1,Ψ1,3,Ψ2,1, and Ψ2,3, represented by ΔΨ1,1,ΔΨ2,1,ΔΨ2,1, and ΔΨ2,3, for fixed X=0.012 and different values of τ. Note that ℜ and ℑ show the real and imaginary part of solutions. As we can observe, for fixed X by changing the value of τ, both DAM and SGEM result major changes for solutions Ψ1,3 and Ψ2,3, and here we are not dealing with an advantageous result. Nevertheless, SGEM results more minor changes than DAM.
X=0.012 | |||||||
τ=0.012,0.037,0.062 | |||||||
DAM | SGEM | ||||||
ΔΨℜ1,1 | ΔΨℑ1,1 | ΔΨℜ1,3 | ΔΨℑ1,3 | ΔΨℜ2,1 | ΔΨℑ2,1 | ΔΨℜ2,3 | ΔΨℑ2,3 |
0.00608 | 0.00419 | 643.10798 | 389.97158 | 0.00689 | 0.00475 | 264.24008 | 160.20893 |
0.01114 | 0.00886 | 613.48429 | 771.99557 | 0.01262 | 0.01003 | 252.19278 | 152.90033 |
0.00506 | 0.00467 | 29.62369 | 17.97601 | 0.00573 | 0.00528 | 12.04730 | 7.30860 |
Tables 6–9 propose the real and imaginary part of exact solutions of CTFMNLSE (1.1) obtained by six different methods: FIM, FVM, TEM, MTEM [15,16] and also DAM and SGEM, with several point sources trough arbitrary. For some values the results obtained through DAM and SGEM, are near to the results obtained in four other methods.
X | τ | FIM⏞Ψℜ1,2(X,τ) | FVM⏞Ψℜ1,2(X,τ) | TEM⏞Ψℜ1,2(X,τ) | MTEM⏞Ψℜ1,2(X,τ) | DAM⏞Ψℜ1,2(X,τ) | SGEM⏞Ψℜ1,2(X,τ) |
0.012 | 0.012 | ∓ 0.00099 | ∓ 0.86524 | ± 1.44911 | ∓ 0.00083 | ± 0.00057 | ± 0.00064 |
0.037 | ± 0.01209 | ∓ 0.96849 | ± 1.38186 | ± 0.00941 | ∓ 0.00549 | ∓ 0.00623 | |
0.062 | ± 0.02666 | ∓ 1.06066 | ± 1.31204 | ± 0.02081 | ∓ 0.01055 | ∓ 0.01196 | |
0.087 | ± 0.04264 | ∓ 1.14551 | ± 1.23880 | ± 0.03333 | ∓ 0.01482 | ∓ 0.01679 | |
0.037 | 0.012 | ∓ 0.01689 | ∓ 0.85415 | ± 1.45334 | ∓ 0.01342 | ± 0.00933 | ± 0.01055 |
0.037 | ∓ 0.00583 | ∓ 0.95628 | ± 1.38820 | ∓ 0.00478 | ± 0.00283 | ± 0.00319 | |
0.062 | ± 0.00691 | ∓ 1.04758 | ± 1.32017 | ± 0.00518 | ∓ 0.00266 | ∓ 0.00303 | |
0.087 | ± 0.02124 | ∓ 1.13177 | ± 1.24849 | ± 0.01639 | ∓ 0.00738 | ∓ 0.00838 | |
0.062 | 0.012 | ∓ 0.03247 | ∓ 0.84187 | ± 1.45547 | ∓ 0.02575 | ± 0.01815 | ± 0.02052 |
0.037 | ∓ 0.02346 | ∓ 0.94274 | ± 1.39254 | ∓ 0.01873 | ± 0.01124 | ± 0.01270 | |
0.062 | ∓ 0.01251 | ∓ 1.03303 | ± 1.32641 | ∓ 0.01019 | ± 0.00530 | ± 0.00598 | |
0.087 | ± 0.00016 | ∓ 1.11640 | ± 1.25641 | ∓ 0.00028 | ± 0.00013 | ± 0.00012 | |
0.087 | 0.012 | ∓ 0.04776 | ∓ 0.82848 | ± 1.45550 | ∓ 0.03784 | ± 0.02705 | ± 0.03053 |
0.037 | ∓ 0.04079 | ∓ 0.92793 | ± 1.39486 | ∓ 0.03244 | ± 0.01972 | ± 0.02227 | |
0.062 | ∓ 0.03165 | ∓ 1.01707 | ± 1.33074 | ∓ 0.02533 | ± 0.01336 | ± 0.01509 | |
0.087 | ∓ 0.02062 | ∓ 1.09949 | ±1.26251 | ∓ 0.01673 | ± 0.00773 | ± 0.00872 |
X | τ | FIM⏞Ψℜ3,4(X,τ) | FVM⏞Ψℜ3,4(X,τ) | TEM⏞Ψℜ3,4(X,τ) | MTEM⏞Ψℜ3,4(X,τ) | DAM⏞Ψℜ3,4(X,τ) | SGEM⏞Ψℜ3,4(X,τ) |
0.012 | 0.012 | ∓ 378.21073 | ∓70.07373 | ±351.38054 | ∓351.20376 | ±587.19354 | ±241.48348 |
0.037 | ± 38.81779 | ∓30.84863 | ∓39.15773 | ±39.17920 | ∓55.91443 | ∓22.75659 | |
0.062 | ± 21.08640 | ∓19.36377 | ∓21.19424 | ±21.20629 | ∓26.29074 | ∓10.70930 | |
0.087 | ± 15.32460 | ∓14.48859 | ∓15.38516 | ±15.39533 | ∓16.74259 | ∓6.82515 | |
0.037 | 0.012 | ∓ 21.73603 | ∓28.35492 | ±21.46931 | ∓21.47600 | ±36.46444 | ±14.88481 |
0.037 | ∓ 78.99292 | ∓24.09697 | ±75.68598 | ∓75.67674 | ±109.34813 | ±44.79797 | |
0.062 | ± 80.05677 | ∓18.81347 | ∓83.68725 | ±83.77139 | ∓105.25185 | ∓42.62660 | |
0.087 | ± 30.34363 | ∓15.03660 | ∓30.84459 | ±30.86541 | ∓33.98710 | ∓13.82036 | |
0.062 | 0.012 | ∓11.06619 | ∓16.76490 | ±10.95095 | ∓10.95634 | ±18.87873 | ±7.71124 |
0.037 | ∓ 19.30540 | ∓16.27571 | ±18.97941 | ∓18.98481 | ±27.8191 | ±11.36481 | |
0.062 | ∓43.52313 | ∓14.65664 | ±41.97445 | ∓41.97341 | ±53.34247 | ±21.83730 | |
0.087 | ±3894.75067 | ∓12.88518 | ±1783.25721 | ∓1751.00331 | ±1903.01800 | ±950.20622 | |
0.087 | 0.012 | ∓7.36271 | ∓11.81796 | ±7.29069 | ∓7.29620 | ±12.76218 | ± 5.21999 |
0.037 | ∓10.90080 | ∓11.82677 | ±10.75601 | ∓10.76155 | ±15.99035 | ±6.53613 | |
0.062 | ∓16.93629 | ∓11.28924 | ±16.60991 | ∓16.61494 | ±21.40072 | ±8.74788 | |
0.087 | ∓30.39447 | ∓10.50579 | ±29.40686 | ∓29.40818 | ±33.17835 | ±13.57924 |
X | τ | FIM⏞Ψℑ1,2(X,τ) | FVM⏞Ψℑ1,2(X,τ) | TEM⏞Ψℑ1,2(X,τ) | MTEM⏞Ψℑ1,2(X,τ) | DAM⏞Ψℑ1,2(X,τ) | SGEM⏞Ψℑ1,2(X,τ) |
0.012 | 0.012 | ± 0.00165 | ±1.44929 | ±0.86568 | ±0.00140 | ±0.00034 | ±0.00038 |
0.037 | ∓ 0.01726 | ±1.38406 | ±0.96853 | ∓0.01342 | ∓0.00385 | ∓0.00437 | |
0.062 | ∓0.033041 | ±1.31780 | ±1.05867 | ∓0.02579 | ∓0.00851 | ∓0.00965 | |
0.087 | ∓0.04635 | ±1.24922 | ±1.13976 | ∓0.03622 | ∓0.01364 | ∓0.01544 | |
0.037 | 0.012 | ± 0.02868 | ±1.45317 | ±0.85592 | ±0.02279 | ±0.00549 | ±0.00621 |
0.037 | ± 0.00844 | ±1.38971 | ±0.96009 | ±0.00691 | ±0.00196 | ±0.00220 | |
0.062 | ∓ 0.00867 | ±1.32525 | ±1.05167 | ∓0.00651 | ∓0.00212 | ∓0.00241 | |
0.087 | ∓ 0.02338 | ±1.25853 | ±1.13434 | ∓0.01804 | ∓0.00671 | ∓0.00762 | |
0.062 | 0.012 | ± 0.05593 | ±1.45494 | ±0.84497 | ±0.04436 | ±0.01054 | ±0.01191 |
0.037 | ± 0.03438 | ±1.39330 | ±0.95027 | ±0.02745 | ±0.00767 | ±0.00866 | |
0.062 | ± 0.01591 | ±1.33069 | ±1.04316 | ±0.01295 | ±0.00417 | ±0.00470 | |
0.087 | ∓0.00018 | ±1.26590 | ±1.12728 | ±0.00031 | ±0.00011 | ±0.00011 | |
0.087 | 0.012 | ± 0.08346 | ±1.45459 | ±0.83287 | ±0.06613 | ±0.01548 | ±0.01747 |
0.037 | ± 0.06058 | ±1.39481 | ±0.93913 | ±0.04818 | ±0.01327 | ±0.01499 | |
0.062 | ±0.04077 | ±1.33411 | ±1.03317 | ±0.03263 | ±0.01037 | ±0.01171 | |
0.087 | ±0.02328 | ±1.27129 | ±1.11860 | ±0.01889 | ±0.00685 | ±0.00773 |
X | τ | FIM⏞Ψℑ3,4(X,τ) | FVM⏞Ψℑ3,4(X,τ) | TEM⏞Ψℑ3,4(X,τ) | MTEM⏞Ψℑ3,4(X,τ) | DAM⏞Ψℑ3,4(X,τ) | SGEM⏞Ψℑ3,4(X,τ) |
0.012 | 0.012 | ±633.10822 | ±12.28177 | ∓588.19565 | ±587.89974 | ±350.78189 | ±144.25913 |
0.037 | ∓55.38382 | ±19.40644 | ±55.86884 | ∓55.89947 | ∓39.18969 | ∓15.94980 | |
0.062 | ∓26.13300 | ±14.23268 | ±26.26665 | ∓26.28158 | ∓21.21368 | ∓8.64120 | |
0.087 | ∓16.65633 | ±10.67754 | ±16.72216 | ∓16.73321 | ∓15.40396 | ∓6.27945 | |
0.037 | 0.012 | ±36.90715 | ∓7.51554 | ∓36.45427 | ±36.46563 | ±21.47530 | ±8.76623 |
0.037 | ±114.21647 | ±1.26633 | ∓109.43494 | ±109.42158 | ±75.62594 | ±30.98259 | |
0.062 | ∓100.49552 | ±4.11852 | ±105.05287 | ∓105.15849 | ∓83.84576 | ∓33.95722 | |
0.087 | ∓33.39707 | ±4.55124 | ±33.94843 | ∓33.97135 | ∓30.87972 | ∓12.55679 | |
0.062 | 0.012 | ±19.06153 | ∓6.31111 | ∓18.86303 | ±18.87231 | ±10.96007 | ±4.47677 |
0.037 | ±28.29015 | ∓2.73162 | ∓27.81244 | ±27.82036 | ±18.98398 | ±7.75543 | |
0.062 | ±55.34093 | ∓0.47397 | ∓53.37174 | ±53.37043 | ±41.95143 | ±17.17404 | |
0.087 | ∓4340.87665 | ±0.69068 | ∓1987.52122 | ±1951.57277 | ±1707.43866 | ±852.55044 | |
0.087 | 0.012 | ±12.86682 | ∓5.00514 | ∓12.74097 | ±12.75059 | ±7.30283 | ±2.98701 |
0.037 | ±16.19046 | ∓3.32263 | ∓15.97541 | ±15.98364 | ±10.76607 | ±4.40068 | |
0.062 | ±21.81420 | ∓2.01126 | ∓21.39382 | ±21.40030 | ±16.61527 | ±6.79175 | |
0.087 | ±34.30495 | ∓1.10820 | ∓33.19028 | ±33.19177 | ±29.39629 | ±12.03132 |
Using the DAM and SGEM, firstly we found the exact solutions of CTFMNLSE (1.1) and finally, we presented numerical results in tables and charts. Also, we compared the real and imaginary parts of the exact solutions of CTFMNLSE (1.1) obtained by DAM and SGEM with four other different methods: FIM, FVM, TEM, MTEM. For some values the results obtained through DAM and SGEM, were near to the results obtained in four other methods. Overall, the performance of the proposed methods (DAM and SGEM) is reliable and effective and gives more solutions. These methods are direct and concise. Therefore, we conclude these methods can be extended to solve many nonlinear conformable fractional PDEs which are arising in the theory of solitons and other areas.
The authors T. Abdeljawad and N. Mlaiki would like to thank Prince Sultan University for paying the APC and for the support through the TAS research lab.
The authors declare no conflicts of interest.
[1] |
Q. Cheng, Z. Liu, Y. Lin, X. S. Zhou, An s-shaped three-parameter (S3) traffic stream model with consistent car following relationship, Transp. Res. Part B Methodol., 153 (2021), 246-271. https://doi.org/10.1016/j.trb.2021.09.004 doi: 10.1016/j.trb.2021.09.004
![]() |
[2] |
H. Wang, Transportation-enabled urban services: A brief discussion, Mutimodal Transp., 1 (2022), 100007. https://doi.org/10.1016/j.multra.2022.100007 doi: 10.1016/j.multra.2022.100007
![]() |
[3] | M. Ester, H. P. Kriegel, J. Sander, X. Xu, A density-based algorithm for discovering clusters in large spatial databases with noise, in Kdd, AAAI, 96 (1996), 226-231. |
[4] |
Q. Cheng, Z. Liu, J. Guo, X. Wu, R. Pendyala, B. Belezamo, et al., Estimating key traffic state parameters through parsimonious spatial queue models, Transp. Res. Part C Emerging Technol., 137 (2022), 103596. https://doi.org/10.1016/j.trc.2022.103596 doi: 10.1016/j.trc.2022.103596
![]() |
[5] |
Z. Liu, Y. Wang, Q. Cheng, H. Yang, Analysis of the information entropy on traffic flows, IEEE Trans. Intell. Transp. Syst., 2022 (2022), 1-12. https://doi.org/10.1109/TITS.2022.3155933 doi: 10.1109/TITS.2022.3155933
![]() |
[6] |
D. Huang, J. Xing, Z. Liu, Q. An, A multi-stage stochastic optimization approach to the stop-skipping and bus lane reservation schemes, Transportmetrica A Transp. Sci., 17 (2021), 1272-1304. https://doi.org/10.1080/23249935.2020.1858206 doi: 10.1080/23249935.2020.1858206
![]() |
[7] | F. Giannotti, M. Nanni, F. Pinelli, D. Pedreschi, Trajectory pattern mining, in Proceedings of the 13th ACM SIGKDD International Conference on Knowledge Discovery and Data Mining, ACM, San Jose, USA, (2007), 330-339. https://doi.org/10.1145/1281192.1281230 |
[8] | I. Syarif, A. Prugel-Bennett, G. Wills, Data mining approaches for network intrusion detection: from dimensionality reduction to misuse and anomaly detection, J. Inf. Technol. Rev., 3 (2012), 70-83. |
[9] |
Y. Yue, H. D. Wang, B. Hu, Q. Li, Y. G. Li, A. G. Yeh, Exploratory calibration of a spatial interaction model using taxi GPS trajectories, Comput. Environ. Urban Syst., 36 (2012), 140-153. https://doi.org/10.1016/j.compenvurbsys.2011.09.002 doi: 10.1016/j.compenvurbsys.2011.09.002
![]() |
[10] |
Q. Cheng, Y. Chen, Z. Liu, A bi-level programming model for the optimal lane reservation problem, Expert Syst. Appl., 189 (2022), 116147. https://doi.org/10.1016/j.eswa.2021.116147 doi: 10.1016/j.eswa.2021.116147
![]() |
[11] | G. Münz, S. Li, G. Carle, Traffic anomaly detection using k-means clustering, in GI/ITG Workshop MMBnet, 7 (2007), 9. |
[12] | I. N. Junejo, O. Javed, M. Shah, Multi feature path modeling for video surveillance, in Proceedings of the 17th International Conference on Pattern Recognition, IEEE, Cambridge, UK, 2 (2004), 716-719. https://doi.org/10.1109/ICPR.2004.1334359 |
[13] |
Q. Meng, P. Liu, Z. Liu, Integrating multimodal transportation research, J. Multimodal Transport., 1 (2022), 100001. https://doi.org/10.1016/j.multra.2022.100001 doi: 10.1016/j.multra.2022.100001
![]() |
[14] |
Y. Zheng, Trajectory data mining: an overview, ACM Trans. Intell. Syst. Technol. (TIST), 6 (2015), 1-41. https://doi.org/10.1145/2743025 doi: 10.1145/2743025
![]() |
[15] |
Z. Feng, Y. Zhu, A survey on trajectory data mining: techniques and applications, IEEE Access, 4 (2016), 2056-2067. https://doi.org/10.1109/ACCESS.2016.2553681 doi: 10.1109/ACCESS.2016.2553681
![]() |
[16] |
N. Paragios, R. Deriche, Geodesic active regions: a new framework to deal with frame partition problems in computer vision, J. Visual Commun. Image Represent., 13 (2002), 249-268. https://doi.org/10.1006/jvci.2001.0475 doi: 10.1006/jvci.2001.0475
![]() |
[17] | C. Stauffer, W. E. Grimson, Adaptive background mixture models for real-time tracking, in 1999 IEEE Computer Society Conference on Computer Vision and Pattern Recognition (Cat. No PR00149), IEEE, Collins, USA, 2 (1999), 246-252. https://doi.org/10.1109/CVPR.1999.784637 |
[18] |
S. Coşar, G. Donatiello, V. Bogorny, C. Garate, L. O. Alvares, F. Bremond, Toward abnormal trajectory and event detection in video surveillance, IEEE Trans. Circuits Syst. Video Technol., 27 (2016), 683-695. https://doi.org/10.1109/TCSVT.2016.2589859 doi: 10.1109/TCSVT.2016.2589859
![]() |
[19] |
J. Huo, X. Fu, Z. Liu, Q. Zhang, Short-term estimation and prediction of pedestrian density in urban hot spots based on mobile phone data, IEEE Trans. Intell. Transp. Syst., 23 (2022), 10827-10838. https://doi.org/10.1109/TITS.2021.3096274 doi: 10.1109/TITS.2021.3096274
![]() |
[20] |
D. Huang, Y. Wang, S. Jia, Z. Liu, A Lagrangian relaxation approach for the electric bus charging scheduling optimisation problem, Transportmetrica A Transp. Sci., 2022 (2022), 1-24. https://doi.org/10.1080/23249935.2021.2023690 doi: 10.1080/23249935.2021.2023690
![]() |
[21] |
J. Simon, Remote supply revisited: the jeep problem with costly transfer points, Multimodal Transp., 1 (2022), 100019. https://doi.org/10.1016/j.multra.2022.100019 doi: 10.1016/j.multra.2022.100019
![]() |
[22] |
J. Qiu, K. Huang, J. Hawkins, The taxi sharing practices: matching, routing and pricing methods, Multimodal Transp., 1 (2022), 100003. https://doi.org/10.1016/j.multra.2022.100003 doi: 10.1016/j.multra.2022.100003
![]() |
[23] | A. T. Palma, V. Bogorny, B. Kuijpers, L. O. Alvares, A clustering-based approach for discovering interesting places in trajectories, in Proceedings of the 2008 ACM Symposium on Applied Computing, ACM, Fortaleza, Brazil, (2008), 863-868. https://doi.org/10.1145/1363686.1363886 |
[24] |
L. Grady, E. L. Schwartz, Isoperimetric graph partitioning for image segmentation, IEEE Trans. Pattern Anal. Mach. Intell., 28 (2006), 469-475. https://doi.org/10.1109/TPAMI.2006.57 doi: 10.1109/TPAMI.2006.57
![]() |
[25] | L. Zhao, G. Shi, J. Yang, An adaptive hierarchical clustering method for ship trajectory data based on DBSCAN algorithm, in 2017 IEEE 2nd International Conference on Big Data Analysis (ICBDA), IEEE, Beijing, China, (2017), 329-336. https://doi.org/10.1109/ICBDA.2017.8078834 |
[26] |
Y. Xi, D. Huang, Y. Yuan, Z. Liu, K. Anish, N. Zheng, Improved dynamic time warping algorithm for bus route trajectory curve fitting, J. Transp. Eng., 147 (2021), 04021044. https://doi.org/10.1061/JTEPBS.0000544 doi: 10.1061/JTEPBS.0000544
![]() |
[27] | R. F. Ibrahim, A recommendation system based on clustering and classification for optimal trajectory analysis, PhD thesis, Carleton University, 2019. https://doi.org/10.22215/etd/2019-13400 |
[28] |
M. Khashei, M. Bijari, A novel hybridization of artificial neural networks and ARIMA models for time series forecasting, Appl. Soft Comput., 11 (2011), 2664-2675. https://doi.org/10.1016/j.asoc.2010.10.015 doi: 10.1016/j.asoc.2010.10.015
![]() |
[29] |
Y. Yuan, W. Zhang, X. Yang, Y. Liu, Z. Liu, W. Wang, Traffic state classification and prediction based on trajectory data, J. Intell. Transp. Syst., 2021 (2021), 1-15. https://doi.org/10.1080/15472450.2021.1955210 doi: 10.1080/15472450.2021.1955210
![]() |
[30] |
V. Hodge, J. Austin, A survey of outlier detection methodologies, Artif. Intell. Rev., 22 (2004), 85-126. https://doi.org/10.1023/B:AIRE.0000045502.10941.a9 doi: 10.1023/B:AIRE.0000045502.10941.a9
![]() |
[31] | S. Y. Huang, Y. N. Huang, Network traffic anomaly detection based on growing hierarchical SOM, in 2013 43rd Annual IEEE/IFIP International Conference on Dependable Systems and Networks (DSN), IEEE, Budapest, Hungary, (2013), 1-2. https://doi.org/10.1109/DSN.2013.6575338 |
[32] | A. S. da Silva, J. A. Wickboldt, L. Z. Granville, A. Schaeffer-Filho, ATLANTIC: A framework for anomaly traffic detection, classification, and mitigation in SDN, in NOMS 2016-2016 IEEE/IFIP Network Operations and Management Symposium, IEEE, Istanbul, Turkey, (2016), 27-35. https://doi.org/10.1109/NOMS.2016.7502793 |
[33] |
K. K. Santhosh, D. P. Dogra, P. P. Roy, Anomaly detection in road traffic using visual surveillance: A survey, ACM Comput. Surv. (CSUR), 53 (2020), 1-26. https://doi.org/10.1145/3417989 doi: 10.1145/3417989
![]() |
[34] | S. Chawla, Y. Zheng, J. Hu, Inferring the root cause in road traffic anomalies, in 2012 IEEE 12th International Conference on Data Mining, IEEE, Brussels, Belgium, (2012), 141-150. https://doi.org/10.1109/ICDM.2012.104 |
[35] |
P. R. Lei, A framework for anomaly detection in maritime trajectory behavior, Knowl. Inf. Syst., 47 (2016), 189-214. https://doi.org/10.1007/s10115-015-0845-4 doi: 10.1007/s10115-015-0845-4
![]() |
[36] |
J. Wang, I. C. Paschalidis, Statistical traffic anomaly detection in time-varying communication networks, IEEE Trans. Control Network Syst., 2 (2014), 100-111. https://doi.org/10.1109/TCNS.2014.2378631 doi: 10.1109/TCNS.2014.2378631
![]() |
[37] |
E. M. Knorr, R. T. Ng, V. Tucakov, Distance-based outliers: Algorithms and applications, VLDB J., 8 (2000), 237-253. https://doi.org/10.1007/s007780050006 doi: 10.1007/s007780050006
![]() |
[38] | E. M. Knorr, R.T. Ng, Finding intensional knowledge of distance-based outliers, in Vldb, 99 (1999), 211-222. |
[39] | J. G. Lee, J. Han, X. Li, Trajectory outlier detection: A partition-and-detect framework, in 2008 IEEE 24th International Conference on Data Engineering, IEEE, Cancun, Mexico, (2008), 140-149. https://doi.org/10.1109/ICDE.2008.4497422 |
[40] |
S. A. Ahmed, D. P. Dogra, S. Kar, P. P. Roy, Surveillance scene representation and trajectory abnormality detection using aggregation of multiple concepts, Expert Syst. Appl., 101 (2018), 43-55. https://doi.org/10.1016/j.eswa.2018.02.013 doi: 10.1016/j.eswa.2018.02.013
![]() |
[41] | Y. Ge, H. Xiong, Z. Zhou, H. Ozdemir, J. Yu, K. C. Lee, Top-eye: top-k evolving trajectory outlier detection, in Proceedings of the 19th ACM International Conference on Information and Knowledge Management, ACM, Toronto, Canada, (2010), 1733-1736. https://doi.org/10.1145/1871437.1871716 |
[42] | W. Qin, J. Tang, C. Lu, S. Lao, A trajectory abnormal detection method based on segmentation and clustering, in Journal of Physics: Conference Series, 2010 (2021), 012188. https://doi.org/10.1088/1742-6596/2010/1/012188 |
[43] |
X. Zhao, Y. Rao, J. Cai, W. Ma, Abnormal trajectory detection based on a sparse subgraph, IEEE Access, 8 (2020), 29987-30000. https://doi.org/10.1109/ACCESS.2020.2972299 doi: 10.1109/ACCESS.2020.2972299
![]() |
[44] |
Z. Ding, M. Fei, An anomaly detection approach based on isolation forest algorithm for streaming data using sliding window, IFAC Proc. Vol., 46 (2013), 12-17. https://doi.org/10.3182/20130902-3-CN-3020.00044 doi: 10.3182/20130902-3-CN-3020.00044
![]() |
[45] | D. Xu, Y. Wang, Y. Meng, Z. Zhang, An improved data anomaly detection method based on isolation forest, in 2017 10th International Symposium on Computational Intelligence and Design (ISCID), IEEE, Hangzhou, China, 2 (2017), 287-291. https://doi.org/10.1109/ISCID.2017.202 |
[46] | Z. Cheng, C. Zou, J. Dong, Outlier detection using isolation forest and local outlier factor, in Proceedings of the Conference on Research in Adaptive and Convergent Systems, ACM, Chongqing, China, (2019), 161-168. https://doi.org/10.1145/3338840.3355641 |
1. | Muhammad Shakeel, Aysha Bibi, Dean Chou, Asim Zafar, Study of optical solitons for Kudryashov’s Quintuple power-law with dual form of nonlinearity using two modified techniques, 2023, 273, 00304026, 170364, 10.1016/j.ijleo.2022.170364 | |
2. | Shaofu Wang, Novel soliton solutions of CNLSEs with Hirota bilinear method, 2023, 0972-8821, 10.1007/s12596-022-01065-x | |
3. | Safoura Rezaei Aderyani, Reza Saadati, Stability and controllability results by n–ary aggregation functions in matrix valued fuzzy n–normed spaces, 2023, 643, 00200255, 119265, 10.1016/j.ins.2023.119265 | |
4. | Safoura Rezaei Aderyani, Reza Saadati, Chenkuan Li, Tofigh Allahviranloo, 2024, Chapter 9, 978-3-031-55563-3, 231, 10.1007/978-3-031-55564-0_9 | |
5. | Safoura Rezaei Aderyani, Reza Saadati, Javad Vahidi, Multiple exp-function method to solve the nonlinear space–time fractional partial differential symmetric regularized long wave (SRLW) equation and the (1+1)-dimensional Benjamin–Ono equation, 2023, 37, 0217-9792, 10.1142/S0217979223502132 | |
6. | Saad Abdelkebir, Brahim Nouiri, Analytical method for solving a time-conformable fractional telegraph equation, 2023, 37, 0354-5180, 2773, 10.2298/FIL2309773A | |
7. | Jamshad Ahmad, Kanza Noor, Sonia Akram, Stability analysis and solitonic behaviour of Schrödinger’s nonlinear (2+1) complex conformable time fractional model, 2024, 56, 1572-817X, 10.1007/s11082-024-06521-5 | |
8. | Donal O’Regan, Safoura Rezaei Aderyani, Reza Saadati, Mustafa Inc, Soliton Solution of the Nonlinear Time Fractional Equations: Comprehensive Methods to Solve Physical Models, 2024, 13, 2075-1680, 92, 10.3390/axioms13020092 | |
9. | Donal O’Regan, Safoura Rezaei Aderyani, Reza Saadati, Tofigh Allahviranloo, Solving the Fornberg–Whitham Model Derived from Gilson–Pickering Equations by Analytical Methods, 2024, 13, 2075-1680, 74, 10.3390/axioms13020074 |
X | τ | Ψℜ1,1(X,τ) | Ψℜ1,2(X,τ) | Ψℜ1,3(X,τ) | Ψℜ1,4(X,τ) |
0.012 | 0.00058 | -0.00058 | 587.19354 | -587.19354 | |
0.012 | 0.037 | -0.00550 | 0.00550 | -55.91444 | 55.91444 |
0.062 | -0.01056 | 0.01056 | -26.29075 | 26.29075 | |
0.012 | 0.00933 | -0.00933 | 36.46445 | -36.46445 | |
0.037 | 0.037 | 0.00284 | -0.00284 | 109.34814 | -109.34814 |
0.062 | -0.00266 | 0.00266 | -105.25186 | 105.25186 | |
0.012 | 0.01816 | -0.01816 | 18.87874 | -18.87874 | |
0.062 | 0.037 | 0.01124 | -0.01124 | 27.81915 | -27.81915 |
0.062 | 0.00531 | -0.00531 | 53.34247 | -53.34247 |
X | τ | Ψℑ1,1(X,τ) | Ψℑ1,2(X,τ) | Ψℑ1,3(X,τ) | Ψℑ1,4(X,τ) |
0.012 | 0.00034 | -0.00034 | 350.78189 | -350.78189 | |
0.012 | 0.037 | -0.00385 | 0.00385 | -39.18969 | 39.18969 |
0.062 | -0.00852 | 0.00852 | -21.21368 | 21.21368 | |
0.012 | 0.00549 | -0.00549 | 21.47530 | -21.47530 | |
0.037 | 0.037 | 0.00196 | -0.00196 | 75.62594 | -75.62594 |
0.062 | -0.00212 | 0.00212 | -83.84577 | 83.84577 | |
0.012 | 0.01054 | -0.01054 | 10.96007 | -10.96007 | |
0.062 | 0.037 | 0.00767 | -0.00767 | 18.98398 | -18.98398 |
0.062 | 0.00417 | -0.00417 | 41.95143 | -41.95143 |
X | τ | Ψℜ2,1(X,τ) | Ψℜ2,2(X,τ) | Ψℜ2,3(X,τ) | Ψℜ2,4(X,τ) |
0.012 | 0.00065 | -0.00065 | 241.48348 | -241.48348 | |
0.012 | 0.037 | -0.00624 | 0.00624 | -22.75660 | 22.75660 |
0.062 | -0.01197 | 0.01197 | -10.70930 | 10.70930 | |
0.012 | 0.01055 | -0.01055 | 14.88481 | -14.88481 | |
0.037 | 0.037 | 0.00320 | -0.00320 | 44.79798 | -44.79798 |
0.062 | -0.00304 | 0.00304 | -42.62661 | 42.62661 | |
0.012 | 0.02052 | -0.02052 | 7.71125 | -7.71125 | |
0.062 | 0.037 | 0.01270 | -0.01270 | 11.36482 | -11.36482 |
0.062 | 0.00599 | -0.00599 | 21.83731 | -21.83731 |
X | τ | Ψℑ2,1(X,τ) | Ψℑ2,2(X,τ) | Ψℑ2,3(X,τ) | Ψℑ2,4(X,τ) |
0.012 | 0.00038 | -0.00038 | 144.25913 | -144.25913 | |
0.012 | 0.037 | -0.00437 | 0.00437 | -15.94980 | 15.94980 |
0.062 | -0.00965 | 0.00965 | -8.64120 | 8.64120 | |
0.012 | 0.00621 | -0.00621 | 8.76623 | -8.76623 | |
0.037 | 0.037 | 0.00221 | -0.00221 | 30.98259 | -30.98259 |
0.062 | -0.00241 | 0.00241 | -33.95722 | 33.95722 | |
0.012 | 0.01191 | -0.01191 | 4.47677 | -4.47677 | |
0.062 | 0.037 | 0.00866 | -0.00866 | 7.75543 | -7.75543 |
0.062 | 0.00471 | -0.00471 | 17.17404 | -17.17404 |
X=0.012 | |||||||
τ=0.012,0.037,0.062 | |||||||
DAM | SGEM | ||||||
ΔΨℜ1,1 | ΔΨℑ1,1 | ΔΨℜ1,3 | ΔΨℑ1,3 | ΔΨℜ2,1 | ΔΨℑ2,1 | ΔΨℜ2,3 | ΔΨℑ2,3 |
0.00608 | 0.00419 | 643.10798 | 389.97158 | 0.00689 | 0.00475 | 264.24008 | 160.20893 |
0.01114 | 0.00886 | 613.48429 | 771.99557 | 0.01262 | 0.01003 | 252.19278 | 152.90033 |
0.00506 | 0.00467 | 29.62369 | 17.97601 | 0.00573 | 0.00528 | 12.04730 | 7.30860 |
X | τ | FIM⏞Ψℜ1,2(X,τ) | FVM⏞Ψℜ1,2(X,τ) | TEM⏞Ψℜ1,2(X,τ) | MTEM⏞Ψℜ1,2(X,τ) | DAM⏞Ψℜ1,2(X,τ) | SGEM⏞Ψℜ1,2(X,τ) |
0.012 | 0.012 | ∓ 0.00099 | ∓ 0.86524 | ± 1.44911 | ∓ 0.00083 | ± 0.00057 | ± 0.00064 |
0.037 | ± 0.01209 | ∓ 0.96849 | ± 1.38186 | ± 0.00941 | ∓ 0.00549 | ∓ 0.00623 | |
0.062 | ± 0.02666 | ∓ 1.06066 | ± 1.31204 | ± 0.02081 | ∓ 0.01055 | ∓ 0.01196 | |
0.087 | ± 0.04264 | ∓ 1.14551 | ± 1.23880 | ± 0.03333 | ∓ 0.01482 | ∓ 0.01679 | |
0.037 | 0.012 | ∓ 0.01689 | ∓ 0.85415 | ± 1.45334 | ∓ 0.01342 | ± 0.00933 | ± 0.01055 |
0.037 | ∓ 0.00583 | ∓ 0.95628 | ± 1.38820 | ∓ 0.00478 | ± 0.00283 | ± 0.00319 | |
0.062 | ± 0.00691 | ∓ 1.04758 | ± 1.32017 | ± 0.00518 | ∓ 0.00266 | ∓ 0.00303 | |
0.087 | ± 0.02124 | ∓ 1.13177 | ± 1.24849 | ± 0.01639 | ∓ 0.00738 | ∓ 0.00838 | |
0.062 | 0.012 | ∓ 0.03247 | ∓ 0.84187 | ± 1.45547 | ∓ 0.02575 | ± 0.01815 | ± 0.02052 |
0.037 | ∓ 0.02346 | ∓ 0.94274 | ± 1.39254 | ∓ 0.01873 | ± 0.01124 | ± 0.01270 | |
0.062 | ∓ 0.01251 | ∓ 1.03303 | ± 1.32641 | ∓ 0.01019 | ± 0.00530 | ± 0.00598 | |
0.087 | ± 0.00016 | ∓ 1.11640 | ± 1.25641 | ∓ 0.00028 | ± 0.00013 | ± 0.00012 | |
0.087 | 0.012 | ∓ 0.04776 | ∓ 0.82848 | ± 1.45550 | ∓ 0.03784 | ± 0.02705 | ± 0.03053 |
0.037 | ∓ 0.04079 | ∓ 0.92793 | ± 1.39486 | ∓ 0.03244 | ± 0.01972 | ± 0.02227 | |
0.062 | ∓ 0.03165 | ∓ 1.01707 | ± 1.33074 | ∓ 0.02533 | ± 0.01336 | ± 0.01509 | |
0.087 | ∓ 0.02062 | ∓ 1.09949 | ±1.26251 | ∓ 0.01673 | ± 0.00773 | ± 0.00872 |
X | τ | FIM⏞Ψℜ3,4(X,τ) | FVM⏞Ψℜ3,4(X,τ) | TEM⏞Ψℜ3,4(X,τ) | MTEM⏞Ψℜ3,4(X,τ) | DAM⏞Ψℜ3,4(X,τ) | SGEM⏞Ψℜ3,4(X,τ) |
0.012 | 0.012 | ∓ 378.21073 | ∓70.07373 | ±351.38054 | ∓351.20376 | ±587.19354 | ±241.48348 |
0.037 | ± 38.81779 | ∓30.84863 | ∓39.15773 | ±39.17920 | ∓55.91443 | ∓22.75659 | |
0.062 | ± 21.08640 | ∓19.36377 | ∓21.19424 | ±21.20629 | ∓26.29074 | ∓10.70930 | |
0.087 | ± 15.32460 | ∓14.48859 | ∓15.38516 | ±15.39533 | ∓16.74259 | ∓6.82515 | |
0.037 | 0.012 | ∓ 21.73603 | ∓28.35492 | ±21.46931 | ∓21.47600 | ±36.46444 | ±14.88481 |
0.037 | ∓ 78.99292 | ∓24.09697 | ±75.68598 | ∓75.67674 | ±109.34813 | ±44.79797 | |
0.062 | ± 80.05677 | ∓18.81347 | ∓83.68725 | ±83.77139 | ∓105.25185 | ∓42.62660 | |
0.087 | ± 30.34363 | ∓15.03660 | ∓30.84459 | ±30.86541 | ∓33.98710 | ∓13.82036 | |
0.062 | 0.012 | ∓11.06619 | ∓16.76490 | ±10.95095 | ∓10.95634 | ±18.87873 | ±7.71124 |
0.037 | ∓ 19.30540 | ∓16.27571 | ±18.97941 | ∓18.98481 | ±27.8191 | ±11.36481 | |
0.062 | ∓43.52313 | ∓14.65664 | ±41.97445 | ∓41.97341 | ±53.34247 | ±21.83730 | |
0.087 | ±3894.75067 | ∓12.88518 | ±1783.25721 | ∓1751.00331 | ±1903.01800 | ±950.20622 | |
0.087 | 0.012 | ∓7.36271 | ∓11.81796 | ±7.29069 | ∓7.29620 | ±12.76218 | ± 5.21999 |
0.037 | ∓10.90080 | ∓11.82677 | ±10.75601 | ∓10.76155 | ±15.99035 | ±6.53613 | |
0.062 | ∓16.93629 | ∓11.28924 | ±16.60991 | ∓16.61494 | ±21.40072 | ±8.74788 | |
0.087 | ∓30.39447 | ∓10.50579 | ±29.40686 | ∓29.40818 | ±33.17835 | ±13.57924 |
X | τ | FIM⏞Ψℑ1,2(X,τ) | FVM⏞Ψℑ1,2(X,τ) | TEM⏞Ψℑ1,2(X,τ) | MTEM⏞Ψℑ1,2(X,τ) | DAM⏞Ψℑ1,2(X,τ) | SGEM⏞Ψℑ1,2(X,τ) |
0.012 | 0.012 | ± 0.00165 | ±1.44929 | ±0.86568 | ±0.00140 | ±0.00034 | ±0.00038 |
0.037 | ∓ 0.01726 | ±1.38406 | ±0.96853 | ∓0.01342 | ∓0.00385 | ∓0.00437 | |
0.062 | ∓0.033041 | ±1.31780 | ±1.05867 | ∓0.02579 | ∓0.00851 | ∓0.00965 | |
0.087 | ∓0.04635 | ±1.24922 | ±1.13976 | ∓0.03622 | ∓0.01364 | ∓0.01544 | |
0.037 | 0.012 | ± 0.02868 | ±1.45317 | ±0.85592 | ±0.02279 | ±0.00549 | ±0.00621 |
0.037 | ± 0.00844 | ±1.38971 | ±0.96009 | ±0.00691 | ±0.00196 | ±0.00220 | |
0.062 | ∓ 0.00867 | ±1.32525 | ±1.05167 | ∓0.00651 | ∓0.00212 | ∓0.00241 | |
0.087 | ∓ 0.02338 | ±1.25853 | ±1.13434 | ∓0.01804 | ∓0.00671 | ∓0.00762 | |
0.062 | 0.012 | ± 0.05593 | ±1.45494 | ±0.84497 | ±0.04436 | ±0.01054 | ±0.01191 |
0.037 | ± 0.03438 | ±1.39330 | ±0.95027 | ±0.02745 | ±0.00767 | ±0.00866 | |
0.062 | ± 0.01591 | ±1.33069 | ±1.04316 | ±0.01295 | ±0.00417 | ±0.00470 | |
0.087 | ∓0.00018 | ±1.26590 | ±1.12728 | ±0.00031 | ±0.00011 | ±0.00011 | |
0.087 | 0.012 | ± 0.08346 | ±1.45459 | ±0.83287 | ±0.06613 | ±0.01548 | ±0.01747 |
0.037 | ± 0.06058 | ±1.39481 | ±0.93913 | ±0.04818 | ±0.01327 | ±0.01499 | |
0.062 | ±0.04077 | ±1.33411 | ±1.03317 | ±0.03263 | ±0.01037 | ±0.01171 | |
0.087 | ±0.02328 | ±1.27129 | ±1.11860 | ±0.01889 | ±0.00685 | ±0.00773 |
X | τ | FIM⏞Ψℑ3,4(X,τ) | FVM⏞Ψℑ3,4(X,τ) | TEM⏞Ψℑ3,4(X,τ) | MTEM⏞Ψℑ3,4(X,τ) | DAM⏞Ψℑ3,4(X,τ) | SGEM⏞Ψℑ3,4(X,τ) |
0.012 | 0.012 | ±633.10822 | ±12.28177 | ∓588.19565 | ±587.89974 | ±350.78189 | ±144.25913 |
0.037 | ∓55.38382 | ±19.40644 | ±55.86884 | ∓55.89947 | ∓39.18969 | ∓15.94980 | |
0.062 | ∓26.13300 | ±14.23268 | ±26.26665 | ∓26.28158 | ∓21.21368 | ∓8.64120 | |
0.087 | ∓16.65633 | ±10.67754 | ±16.72216 | ∓16.73321 | ∓15.40396 | ∓6.27945 | |
0.037 | 0.012 | ±36.90715 | ∓7.51554 | ∓36.45427 | ±36.46563 | ±21.47530 | ±8.76623 |
0.037 | ±114.21647 | ±1.26633 | ∓109.43494 | ±109.42158 | ±75.62594 | ±30.98259 | |
0.062 | ∓100.49552 | ±4.11852 | ±105.05287 | ∓105.15849 | ∓83.84576 | ∓33.95722 | |
0.087 | ∓33.39707 | ±4.55124 | ±33.94843 | ∓33.97135 | ∓30.87972 | ∓12.55679 | |
0.062 | 0.012 | ±19.06153 | ∓6.31111 | ∓18.86303 | ±18.87231 | ±10.96007 | ±4.47677 |
0.037 | ±28.29015 | ∓2.73162 | ∓27.81244 | ±27.82036 | ±18.98398 | ±7.75543 | |
0.062 | ±55.34093 | ∓0.47397 | ∓53.37174 | ±53.37043 | ±41.95143 | ±17.17404 | |
0.087 | ∓4340.87665 | ±0.69068 | ∓1987.52122 | ±1951.57277 | ±1707.43866 | ±852.55044 | |
0.087 | 0.012 | ±12.86682 | ∓5.00514 | ∓12.74097 | ±12.75059 | ±7.30283 | ±2.98701 |
0.037 | ±16.19046 | ∓3.32263 | ∓15.97541 | ±15.98364 | ±10.76607 | ±4.40068 | |
0.062 | ±21.81420 | ∓2.01126 | ∓21.39382 | ±21.40030 | ±16.61527 | ±6.79175 | |
0.087 | ±34.30495 | ∓1.10820 | ∓33.19028 | ±33.19177 | ±29.39629 | ±12.03132 |
X | τ | Ψℜ1,1(X,τ) | Ψℜ1,2(X,τ) | Ψℜ1,3(X,τ) | Ψℜ1,4(X,τ) |
0.012 | 0.00058 | -0.00058 | 587.19354 | -587.19354 | |
0.012 | 0.037 | -0.00550 | 0.00550 | -55.91444 | 55.91444 |
0.062 | -0.01056 | 0.01056 | -26.29075 | 26.29075 | |
0.012 | 0.00933 | -0.00933 | 36.46445 | -36.46445 | |
0.037 | 0.037 | 0.00284 | -0.00284 | 109.34814 | -109.34814 |
0.062 | -0.00266 | 0.00266 | -105.25186 | 105.25186 | |
0.012 | 0.01816 | -0.01816 | 18.87874 | -18.87874 | |
0.062 | 0.037 | 0.01124 | -0.01124 | 27.81915 | -27.81915 |
0.062 | 0.00531 | -0.00531 | 53.34247 | -53.34247 |
X | τ | Ψℑ1,1(X,τ) | Ψℑ1,2(X,τ) | Ψℑ1,3(X,τ) | Ψℑ1,4(X,τ) |
0.012 | 0.00034 | -0.00034 | 350.78189 | -350.78189 | |
0.012 | 0.037 | -0.00385 | 0.00385 | -39.18969 | 39.18969 |
0.062 | -0.00852 | 0.00852 | -21.21368 | 21.21368 | |
0.012 | 0.00549 | -0.00549 | 21.47530 | -21.47530 | |
0.037 | 0.037 | 0.00196 | -0.00196 | 75.62594 | -75.62594 |
0.062 | -0.00212 | 0.00212 | -83.84577 | 83.84577 | |
0.012 | 0.01054 | -0.01054 | 10.96007 | -10.96007 | |
0.062 | 0.037 | 0.00767 | -0.00767 | 18.98398 | -18.98398 |
0.062 | 0.00417 | -0.00417 | 41.95143 | -41.95143 |
X | τ | Ψℜ2,1(X,τ) | Ψℜ2,2(X,τ) | Ψℜ2,3(X,τ) | Ψℜ2,4(X,τ) |
0.012 | 0.00065 | -0.00065 | 241.48348 | -241.48348 | |
0.012 | 0.037 | -0.00624 | 0.00624 | -22.75660 | 22.75660 |
0.062 | -0.01197 | 0.01197 | -10.70930 | 10.70930 | |
0.012 | 0.01055 | -0.01055 | 14.88481 | -14.88481 | |
0.037 | 0.037 | 0.00320 | -0.00320 | 44.79798 | -44.79798 |
0.062 | -0.00304 | 0.00304 | -42.62661 | 42.62661 | |
0.012 | 0.02052 | -0.02052 | 7.71125 | -7.71125 | |
0.062 | 0.037 | 0.01270 | -0.01270 | 11.36482 | -11.36482 |
0.062 | 0.00599 | -0.00599 | 21.83731 | -21.83731 |
X | τ | Ψℑ2,1(X,τ) | Ψℑ2,2(X,τ) | Ψℑ2,3(X,τ) | Ψℑ2,4(X,τ) |
0.012 | 0.00038 | -0.00038 | 144.25913 | -144.25913 | |
0.012 | 0.037 | -0.00437 | 0.00437 | -15.94980 | 15.94980 |
0.062 | -0.00965 | 0.00965 | -8.64120 | 8.64120 | |
0.012 | 0.00621 | -0.00621 | 8.76623 | -8.76623 | |
0.037 | 0.037 | 0.00221 | -0.00221 | 30.98259 | -30.98259 |
0.062 | -0.00241 | 0.00241 | -33.95722 | 33.95722 | |
0.012 | 0.01191 | -0.01191 | 4.47677 | -4.47677 | |
0.062 | 0.037 | 0.00866 | -0.00866 | 7.75543 | -7.75543 |
0.062 | 0.00471 | -0.00471 | 17.17404 | -17.17404 |
X=0.012 | |||||||
τ=0.012,0.037,0.062 | |||||||
DAM | SGEM | ||||||
ΔΨℜ1,1 | ΔΨℑ1,1 | ΔΨℜ1,3 | ΔΨℑ1,3 | ΔΨℜ2,1 | ΔΨℑ2,1 | ΔΨℜ2,3 | ΔΨℑ2,3 |
0.00608 | 0.00419 | 643.10798 | 389.97158 | 0.00689 | 0.00475 | 264.24008 | 160.20893 |
0.01114 | 0.00886 | 613.48429 | 771.99557 | 0.01262 | 0.01003 | 252.19278 | 152.90033 |
0.00506 | 0.00467 | 29.62369 | 17.97601 | 0.00573 | 0.00528 | 12.04730 | 7.30860 |
X | τ | FIM⏞Ψℜ1,2(X,τ) | FVM⏞Ψℜ1,2(X,τ) | TEM⏞Ψℜ1,2(X,τ) | MTEM⏞Ψℜ1,2(X,τ) | DAM⏞Ψℜ1,2(X,τ) | SGEM⏞Ψℜ1,2(X,τ) |
0.012 | 0.012 | ∓ 0.00099 | ∓ 0.86524 | ± 1.44911 | ∓ 0.00083 | ± 0.00057 | ± 0.00064 |
0.037 | ± 0.01209 | ∓ 0.96849 | ± 1.38186 | ± 0.00941 | ∓ 0.00549 | ∓ 0.00623 | |
0.062 | ± 0.02666 | ∓ 1.06066 | ± 1.31204 | ± 0.02081 | ∓ 0.01055 | ∓ 0.01196 | |
0.087 | ± 0.04264 | ∓ 1.14551 | ± 1.23880 | ± 0.03333 | ∓ 0.01482 | ∓ 0.01679 | |
0.037 | 0.012 | ∓ 0.01689 | ∓ 0.85415 | ± 1.45334 | ∓ 0.01342 | ± 0.00933 | ± 0.01055 |
0.037 | ∓ 0.00583 | ∓ 0.95628 | ± 1.38820 | ∓ 0.00478 | ± 0.00283 | ± 0.00319 | |
0.062 | ± 0.00691 | ∓ 1.04758 | ± 1.32017 | ± 0.00518 | ∓ 0.00266 | ∓ 0.00303 | |
0.087 | ± 0.02124 | ∓ 1.13177 | ± 1.24849 | ± 0.01639 | ∓ 0.00738 | ∓ 0.00838 | |
0.062 | 0.012 | ∓ 0.03247 | ∓ 0.84187 | ± 1.45547 | ∓ 0.02575 | ± 0.01815 | ± 0.02052 |
0.037 | ∓ 0.02346 | ∓ 0.94274 | ± 1.39254 | ∓ 0.01873 | ± 0.01124 | ± 0.01270 | |
0.062 | ∓ 0.01251 | ∓ 1.03303 | ± 1.32641 | ∓ 0.01019 | ± 0.00530 | ± 0.00598 | |
0.087 | ± 0.00016 | ∓ 1.11640 | ± 1.25641 | ∓ 0.00028 | ± 0.00013 | ± 0.00012 | |
0.087 | 0.012 | ∓ 0.04776 | ∓ 0.82848 | ± 1.45550 | ∓ 0.03784 | ± 0.02705 | ± 0.03053 |
0.037 | ∓ 0.04079 | ∓ 0.92793 | ± 1.39486 | ∓ 0.03244 | ± 0.01972 | ± 0.02227 | |
0.062 | ∓ 0.03165 | ∓ 1.01707 | ± 1.33074 | ∓ 0.02533 | ± 0.01336 | ± 0.01509 | |
0.087 | ∓ 0.02062 | ∓ 1.09949 | ±1.26251 | ∓ 0.01673 | ± 0.00773 | ± 0.00872 |
X | τ | FIM⏞Ψℜ3,4(X,τ) | FVM⏞Ψℜ3,4(X,τ) | TEM⏞Ψℜ3,4(X,τ) | MTEM⏞Ψℜ3,4(X,τ) | DAM⏞Ψℜ3,4(X,τ) | SGEM⏞Ψℜ3,4(X,τ) |
0.012 | 0.012 | ∓ 378.21073 | ∓70.07373 | ±351.38054 | ∓351.20376 | ±587.19354 | ±241.48348 |
0.037 | ± 38.81779 | ∓30.84863 | ∓39.15773 | ±39.17920 | ∓55.91443 | ∓22.75659 | |
0.062 | ± 21.08640 | ∓19.36377 | ∓21.19424 | ±21.20629 | ∓26.29074 | ∓10.70930 | |
0.087 | ± 15.32460 | ∓14.48859 | ∓15.38516 | ±15.39533 | ∓16.74259 | ∓6.82515 | |
0.037 | 0.012 | ∓ 21.73603 | ∓28.35492 | ±21.46931 | ∓21.47600 | ±36.46444 | ±14.88481 |
0.037 | ∓ 78.99292 | ∓24.09697 | ±75.68598 | ∓75.67674 | ±109.34813 | ±44.79797 | |
0.062 | ± 80.05677 | ∓18.81347 | ∓83.68725 | ±83.77139 | ∓105.25185 | ∓42.62660 | |
0.087 | ± 30.34363 | ∓15.03660 | ∓30.84459 | ±30.86541 | ∓33.98710 | ∓13.82036 | |
0.062 | 0.012 | ∓11.06619 | ∓16.76490 | ±10.95095 | ∓10.95634 | ±18.87873 | ±7.71124 |
0.037 | ∓ 19.30540 | ∓16.27571 | ±18.97941 | ∓18.98481 | ±27.8191 | ±11.36481 | |
0.062 | ∓43.52313 | ∓14.65664 | ±41.97445 | ∓41.97341 | ±53.34247 | ±21.83730 | |
0.087 | ±3894.75067 | ∓12.88518 | ±1783.25721 | ∓1751.00331 | ±1903.01800 | ±950.20622 | |
0.087 | 0.012 | ∓7.36271 | ∓11.81796 | ±7.29069 | ∓7.29620 | ±12.76218 | ± 5.21999 |
0.037 | ∓10.90080 | ∓11.82677 | ±10.75601 | ∓10.76155 | ±15.99035 | ±6.53613 | |
0.062 | ∓16.93629 | ∓11.28924 | ±16.60991 | ∓16.61494 | ±21.40072 | ±8.74788 | |
0.087 | ∓30.39447 | ∓10.50579 | ±29.40686 | ∓29.40818 | ±33.17835 | ±13.57924 |
X | τ | FIM⏞Ψℑ1,2(X,τ) | FVM⏞Ψℑ1,2(X,τ) | TEM⏞Ψℑ1,2(X,τ) | MTEM⏞Ψℑ1,2(X,τ) | DAM⏞Ψℑ1,2(X,τ) | SGEM⏞Ψℑ1,2(X,τ) |
0.012 | 0.012 | ± 0.00165 | ±1.44929 | ±0.86568 | ±0.00140 | ±0.00034 | ±0.00038 |
0.037 | ∓ 0.01726 | ±1.38406 | ±0.96853 | ∓0.01342 | ∓0.00385 | ∓0.00437 | |
0.062 | ∓0.033041 | ±1.31780 | ±1.05867 | ∓0.02579 | ∓0.00851 | ∓0.00965 | |
0.087 | ∓0.04635 | ±1.24922 | ±1.13976 | ∓0.03622 | ∓0.01364 | ∓0.01544 | |
0.037 | 0.012 | ± 0.02868 | ±1.45317 | ±0.85592 | ±0.02279 | ±0.00549 | ±0.00621 |
0.037 | ± 0.00844 | ±1.38971 | ±0.96009 | ±0.00691 | ±0.00196 | ±0.00220 | |
0.062 | ∓ 0.00867 | ±1.32525 | ±1.05167 | ∓0.00651 | ∓0.00212 | ∓0.00241 | |
0.087 | ∓ 0.02338 | ±1.25853 | ±1.13434 | ∓0.01804 | ∓0.00671 | ∓0.00762 | |
0.062 | 0.012 | ± 0.05593 | ±1.45494 | ±0.84497 | ±0.04436 | ±0.01054 | ±0.01191 |
0.037 | ± 0.03438 | ±1.39330 | ±0.95027 | ±0.02745 | ±0.00767 | ±0.00866 | |
0.062 | ± 0.01591 | ±1.33069 | ±1.04316 | ±0.01295 | ±0.00417 | ±0.00470 | |
0.087 | ∓0.00018 | ±1.26590 | ±1.12728 | ±0.00031 | ±0.00011 | ±0.00011 | |
0.087 | 0.012 | ± 0.08346 | ±1.45459 | ±0.83287 | ±0.06613 | ±0.01548 | ±0.01747 |
0.037 | ± 0.06058 | ±1.39481 | ±0.93913 | ±0.04818 | ±0.01327 | ±0.01499 | |
0.062 | ±0.04077 | ±1.33411 | ±1.03317 | ±0.03263 | ±0.01037 | ±0.01171 | |
0.087 | ±0.02328 | ±1.27129 | ±1.11860 | ±0.01889 | ±0.00685 | ±0.00773 |
X | τ | FIM⏞Ψℑ3,4(X,τ) | FVM⏞Ψℑ3,4(X,τ) | TEM⏞Ψℑ3,4(X,τ) | MTEM⏞Ψℑ3,4(X,τ) | DAM⏞Ψℑ3,4(X,τ) | SGEM⏞Ψℑ3,4(X,τ) |
0.012 | 0.012 | ±633.10822 | ±12.28177 | ∓588.19565 | ±587.89974 | ±350.78189 | ±144.25913 |
0.037 | ∓55.38382 | ±19.40644 | ±55.86884 | ∓55.89947 | ∓39.18969 | ∓15.94980 | |
0.062 | ∓26.13300 | ±14.23268 | ±26.26665 | ∓26.28158 | ∓21.21368 | ∓8.64120 | |
0.087 | ∓16.65633 | ±10.67754 | ±16.72216 | ∓16.73321 | ∓15.40396 | ∓6.27945 | |
0.037 | 0.012 | ±36.90715 | ∓7.51554 | ∓36.45427 | ±36.46563 | ±21.47530 | ±8.76623 |
0.037 | ±114.21647 | ±1.26633 | ∓109.43494 | ±109.42158 | ±75.62594 | ±30.98259 | |
0.062 | ∓100.49552 | ±4.11852 | ±105.05287 | ∓105.15849 | ∓83.84576 | ∓33.95722 | |
0.087 | ∓33.39707 | ±4.55124 | ±33.94843 | ∓33.97135 | ∓30.87972 | ∓12.55679 | |
0.062 | 0.012 | ±19.06153 | ∓6.31111 | ∓18.86303 | ±18.87231 | ±10.96007 | ±4.47677 |
0.037 | ±28.29015 | ∓2.73162 | ∓27.81244 | ±27.82036 | ±18.98398 | ±7.75543 | |
0.062 | ±55.34093 | ∓0.47397 | ∓53.37174 | ±53.37043 | ±41.95143 | ±17.17404 | |
0.087 | ∓4340.87665 | ±0.69068 | ∓1987.52122 | ±1951.57277 | ±1707.43866 | ±852.55044 | |
0.087 | 0.012 | ±12.86682 | ∓5.00514 | ∓12.74097 | ±12.75059 | ±7.30283 | ±2.98701 |
0.037 | ±16.19046 | ∓3.32263 | ∓15.97541 | ±15.98364 | ±10.76607 | ±4.40068 | |
0.062 | ±21.81420 | ∓2.01126 | ∓21.39382 | ±21.40030 | ±16.61527 | ±6.79175 | |
0.087 | ±34.30495 | ∓1.10820 | ∓33.19028 | ±33.19177 | ±29.39629 | ±12.03132 |