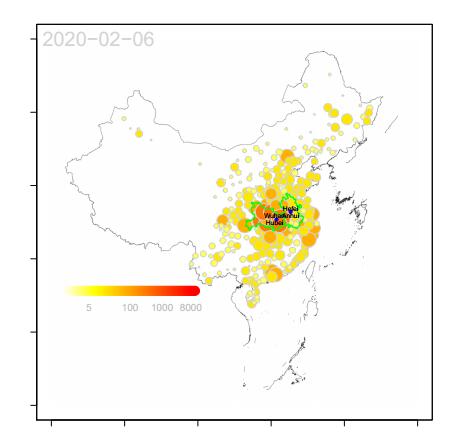
The Aquila optimizer (AO) is a recently developed swarm algorithm that simulates the hunting behavior of Aquila birds. In complex optimization problems, an AO may have slow convergence or fall in sub-optimal regions, especially in high complex ones. This paper tries to overcome these problems by using three different strategies: restart strategy, opposition-based learning and chaotic local search. The developed algorithm named as mAO was tested using 29 CEC 2017 functions and five different engineering constrained problems. The results prove the superiority and efficiency of mAO in solving many optimization issues.
Citation: Huangjing Yu, Heming Jia, Jianping Zhou, Abdelazim G. Hussien. Enhanced Aquila optimizer algorithm for global optimization and constrained engineering problems[J]. Mathematical Biosciences and Engineering, 2022, 19(12): 14173-14211. doi: 10.3934/mbe.2022660
[1] | Weike Zhou, Aili Wang, Fan Xia, Yanni Xiao, Sanyi Tang . Effects of media reporting on mitigating spread of COVID-19 in the early phase of the outbreak. Mathematical Biosciences and Engineering, 2020, 17(3): 2693-2707. doi: 10.3934/mbe.2020147 |
[2] | Hamdy M. Youssef, Najat A. Alghamdi, Magdy A. Ezzat, Alaa A. El-Bary, Ahmed M. Shawky . A new dynamical modeling SEIR with global analysis applied to the real data of spreading COVID-19 in Saudi Arabia. Mathematical Biosciences and Engineering, 2020, 17(6): 7018-7044. doi: 10.3934/mbe.2020362 |
[3] | Haonan Zhong, Wendi Wang . Mathematical analysis for COVID-19 resurgence in the contaminated environment. Mathematical Biosciences and Engineering, 2020, 17(6): 6909-6927. doi: 10.3934/mbe.2020357 |
[4] | Fang Wang, Lianying Cao, Xiaoji Song . Mathematical modeling of mutated COVID-19 transmission with quarantine, isolation and vaccination. Mathematical Biosciences and Engineering, 2022, 19(8): 8035-8056. doi: 10.3934/mbe.2022376 |
[5] | Pannathon Kreabkhontho, Watchara Teparos, Thitiya Theparod . Potential for eliminating COVID-19 in Thailand through third-dose vaccination: A modeling approach. Mathematical Biosciences and Engineering, 2024, 21(8): 6807-6828. doi: 10.3934/mbe.2024298 |
[6] | Lin Feng, Ziren Chen, Harold A. Lay Jr., Khaled Furati, Abdul Khaliq . Data driven time-varying SEIR-LSTM/GRU algorithms to track the spread of COVID-19. Mathematical Biosciences and Engineering, 2022, 19(9): 8935-8962. doi: 10.3934/mbe.2022415 |
[7] | Yujie Sheng, Jing-An Cui, Songbai Guo . The modeling and analysis of the COVID-19 pandemic with vaccination and isolation: a case study of Italy. Mathematical Biosciences and Engineering, 2023, 20(3): 5966-5992. doi: 10.3934/mbe.2023258 |
[8] | Ahmed Alshehri, Saif Ullah . A numerical study of COVID-19 epidemic model with vaccination and diffusion. Mathematical Biosciences and Engineering, 2023, 20(3): 4643-4672. doi: 10.3934/mbe.2023215 |
[9] | Dipo Aldila, Meksianis Z. Ndii, Brenda M. Samiadji . Optimal control on COVID-19 eradication program in Indonesia under the effect of community awareness. Mathematical Biosciences and Engineering, 2020, 17(6): 6355-6389. doi: 10.3934/mbe.2020335 |
[10] | Tianfang Hou, Guijie Lan, Sanling Yuan, Tonghua Zhang . Threshold dynamics of a stochastic SIHR epidemic model of COVID-19 with general population-size dependent contact rate. Mathematical Biosciences and Engineering, 2022, 19(4): 4217-4236. doi: 10.3934/mbe.2022195 |
The Aquila optimizer (AO) is a recently developed swarm algorithm that simulates the hunting behavior of Aquila birds. In complex optimization problems, an AO may have slow convergence or fall in sub-optimal regions, especially in high complex ones. This paper tries to overcome these problems by using three different strategies: restart strategy, opposition-based learning and chaotic local search. The developed algorithm named as mAO was tested using 29 CEC 2017 functions and five different engineering constrained problems. The results prove the superiority and efficiency of mAO in solving many optimization issues.
Coronavirus belongs to the Coronaviridae family in phylogeny. It was first identified as a pathogenic microorganism of human disease in the mid-1960s. The name comes from the fact that the ratchet protein on the surface of the virus envelope protrudes like a crown under an electron microscope. Before 2019, six known coronaviruses types have been discovered: α genus of 229E, β genus of OC43, HKU1, Middle East respiratory syndrome coronavirus (MERS-COV) and severe acute respiratory syndrome (SARS-COV) [1,2,3]. In December 2019, an unidentified pneumonia was found in Wuhan, Hubei province, China. The responsible virus was later confirmed as a new coronavirus [4]. The National Board of Health name the disease as a novel coronavirus pneumonia (NCP). The World Health Organization (WHO) temporarily named the virus as the novel coronavirus 2019 (2019-nCov), and the disease as coronavirus disease 2019 (COVID-19) on 11 February. At the same time, the International Committee for the classification of viruses named the virus as severe acute respiratory syndrome coronavirus-2 (SARS-COV2) [5]. The disease spread rapidly from Wuhan to all parts of the country and overseas. Till March 12, 2020 at 5:44 p.m, a total of 80,981 people had been confirmed infected in China, and the total death toll had reached 3173. New cases of COVID-19 have emerged in 109 countries overseas (including Japan, South Korea and so on), with 38,620 confirmed cases and 1465 deaths get rid of China from the WHO [6].
Anhui, a neighbouring province of Hubei, is vulnerable to the disease. Since the first confirmed case reported on January 10, 2020 in Anhui, a total of 990 cases have been confirmed and 6 deaths have been reported by March 12. Figure 1 shows the heat map of the total number of confirmed cases in China on February 6. The epidemic poses a challenge to the prevention and control of the epidemic in Anhui.
In the face of the severe epidemic, the Chinese government promptly adopted a series of policies to mitigate the epidemic. The lockdown of the epicenter Wuhan has been implemented since January 23, 2020. On February 6, Anhui province started to adopt the centralized quarantine measures for close contacts of cases. These policies effectively prevented the spread of the disease in Anhui. This is the first work to use mathematical modeling techniques combined with observed data to evaluate the control effectiveness in Anhui. As the outbreak of COVID-19 now became a pandemic, the lesson learnt in Anhui, China is surely valuable for mitigation in other localities. Data-driven mathematical modelling plays an important role in epidemic mitigation, in preparedness for future epidemic and in the evaluation of control effectiveness. The basic reproduction numbers (number of secondary cases may be caused by a typical primary case in a wholly susceptible population, R0) is one of the key epidemiological parameters in quantifying the rate of disease transmission, the size of the outbreak and the needed control effort [7,8]. Many data-driven modelling works in different regions have been done [9,10,11]. In this work, we focused on the epidemic in Anhui based on daily morbidity data which reflect the epidemic more accurately and promptly than laboratory confirmed data. Different from previous studies [8,9,10,11,12,13,14,15], we adopted relatively simple models with flexible transmission rates and model structures.
The interrupted time series analysis is a quasi-experimental research design. By collecting and analyzing relevant data of the outcome indicators measured at multiple time points before and after the implementation of an intervention, the change trend of the outcome indicators before and after the implementation of the intervention is compared to evaluate the impact of the intervention on the outcome indicators [16,17]. We obtained the daily morbidity data from January 10 to February 11, 2020 from the Anhui Province Center of Disease Control and Prevention. During this period, two interventions occurred, Wuhan lockdown on January 23 (i.e., no imported cases from Wuhan to Anhui thereafter), and centralized quarantine management measures for close contacts implemented since February 6. We adopted an interrupted time series model to evaluate the effects of these two interventions.
We define the interrupted time series model as follows: Yt=β0+β1+timet+β2∗intervention1t+β3∗timeafterintervention1t+β4+intervention2t+β5∗timeafterintervention2t+et, where Yt is the daily morbidity index value, β0 is the baseline value (also initial value at t=0); β1 is the baseline slope value (before any intervention); β2 refers to the contribution of the first intervention on the baseline value; β3 is the contribution of the first intervention on the baseline slope; That is, β1+β3 is the trend after the implementation of the first intervention; similarly, β4,5 are contribution of the second intervention on the baseline value and baseline slope; β4,5 replaced β1,2 after the implementation of the second intervention. Thus, β1+β5 is the trend of daily morbidity after the second intervention.
As shown in Figure 2, the model prediction (the black curve) is divided into three sections, before January 23 (phase Ⅰ), between January 23 and February 6 (phase Ⅱ) and after February 6 (phase Ⅲ). The slopes of in phases Ⅰ-Ⅲ are β1, β1+β3, β1+β5, respectively. The instantaneous and lasting effects of the two interventions are clearly demonstrated.We fit the model with Eviews and find the r-squared R2=0.92 and the Durbin-Watson statistic DW=2.32, which indicates high fitting performance of the model. Parameter estimates are listed in the Table 1.
Parameter | Estimate | p-value |
β0 | 7.076923 | 0.0778 |
β1 | 2.923077 | 0.0000 |
β2 | 38.29670 | 0.0000 |
β3 | -6.057143 | 0.0000 |
β4 | -4.742857 | 0.5012 |
β5 | -0.323077 | 0.8442 |
According to Table 1, the linear growth trend of the morbidity number during phase Ⅰ was β1=2.9 (p−value<1e−5). The morbidity number had a sudden lift β2=38 (p−value<1e−5) on January 23, 2020. After that the slope of the morbidity number decreased by β3=6.057 (p−value<1e−5). These results imply that right before the lockdown of Wuhan a large number of imported cases from Wuhan or other Hubei cities arrived into Anhui. The lockdown effect was substantial as the morbidity data showed an immediate down trend. However, the effect of the second intervention (after February 6) was noticeable but no significant. As shown in Figure 2, the daily number of cases continued to decrease after February 6. To sum up, it can be seen that the lockdown measures in Wuhan as well as the centralized quarantine management measures in Anhui had a great impact against the spread of COVID-19 in Anhui.
According to the Anhui Provincial Center for Disease Control and Prevention, the onset of the first case in Anhui was on January 10, 2020 and the case was confirmed on January 22, 2020. There is a 12 days confirmation delay. According to the report of the National Health Commission, both pre-symptomatic patients and symptomatic patients may be contagious. Recent studies showed that the mean serial interval (time delay between the symptom onset of a primary case and a second case) is short, and could be shorter than the incubation period (time delay from infection to symptom onset). Thus pre-symptomatic transmission (one day or two prior symptom onset) is fully justified [9,10].
Our interrupted time series analysis showed that both Wuhan lockdown and Anhui centralized quarantine management had an impact on the daily morbidity number in Anhui. During the initial phase of the COVID-19 outbreak, the rate of exposure could be high due to lack of awareness and protection. Subsequently, the relevant announcement and measures issued by the government attracted public's attention and enhanced level of self-protection, and strict quarantine measures were adopted. The rate of exposure gradually declined, and the form of epidemic transmission (main transmitter) may have changed accordingly. We consider these factors and formulate our mechanistic models for the three phases.
Phase Ⅰ (prior to January 23, 2020): During this period public awareness of the severity of the disease was low and cases (especially those of mild symptom) might be treated as common cold. Both pre-symptomatic cases (especially right before symptom onset) and symptomatic cases were infectious [8]. Considering the infectivity before and after the symptom onset might be different, we divided the infected people into two categories: E and I in phase Ⅰ, and allowed the infectivity of class E to be less than that of class I. However, the mobility of class I might be lower than that of class E, thus the infectivity level of E might be comparable to I. The population N can be divided into four categories: susceptible (S), exposed and pre-symptomatic population (E), symptomatic population (I), and recovered population (R). The total population N equals S+E+I+R. The transmission dynamic model reads:
{dSdt=−βS(kE+I)N,dEdt=βS(kE+I)N−qE,dIdt=qE−γI,dRdt=γI. | (2.1) |
where, β is the transmission rate of disease, q is the rate at which exposed individual showing symptom, {k controls the infectiousness of exposed pre-symptomatic individuals relative to symptomatic individuals, γ is the cure rate at which symptomatic individuals moving to recovered class. We ignore COVID-19 induced death since there were no deaths from diseases in the early stage and few deaths in the later stage in Anhui.
Phase Ⅱ (between January 23 and February 6, 2020): public began to realize the seriousness of the epidemic after Wuhan lockdown on January 23 and lockdown in other cities in Hubei shortly after. The country also issued the epidemic situation announcement, and began to take quarantine measures, symptomatic cases went to the hospital to see a doctor and would be quarantined before confirmation. Therefore, after January 23, only pre-symptomatic cases in E class can effectively contact susceptibles to spread the disease. And there was also a decrease in the rate of exposure after January 23. Therefore, on the basis of model (2.1), we incorporate the policy intervention factors, and change the exposure rate to α1β. We assume all symptomatic cases were quarantined in quarantined class (Q), and σ is the cure rate at which quarantined cases moving to recovered class. Then SEQR model (2.2) reads:
{dSdt=−α1βSEN,dEdt=α1βSEN−qE,dQdt=qE−σQ,dRdt=σQ. | (2.2) |
Phase Ⅲ (after February 6, 2020): The centralized quarantined management measures for close contacts was adopted on February 6 in Anhui and resulted in a decline in the chance of contact among the population. Therefore, after February 6, the rate of exposure rate was changed to α2β. The model is the same as (2.2), except for α2 replacing α1.
We adopted MCMC method implemented in R package to estimate parameters in models (2.1 and 2.2). We fit I(t) or Q(t) to the observed morbidity data. Note that our morbidity are daily current symptomatic cases. We obtained β=0.4029, q=0.41 (per day), k=0.78 and γ=0.182 (per day) in the first stage. Since during the first phase, individuals in E and I were not quarantined, both of them may spread the disease. We obtained the basic reproduction number during the phase 1: R0=kβq+βγ=2.9764. We did not distinguish imported cases and local cases and treat all cases as local. A substantial proportion of cases in phase Ⅰ would be imported cases from Wuhan or other cities in Hubei. If the proportion of imported cases over the time during the phase Ⅰ was relatively constant, our method is still relatively reasonable since the mortality is still a proxy of the local epidemic. Given that most of the infections occurred among family members, we presume the ratio of imported versus local case were relatively stable during phase Ⅰ, and gradually vanished later. The actual and predicted values of the morbidity number during phase Ⅰ are compared in Figure 3.
We used MCMC to fit model 2 to the morbidity number between January 23 and February 6, 2020. We obtained α1β=0.208, q=0.24, σ=0.04. Using generation matrix method, the basic reproduction number was estimated R0=α1βq=0.8667. This reduction in R0 was due to lockdown effects, holiday effects, and public being vigilant. The actual and predicted values of the morbidity number during phase Ⅱ are compared Figure 4.
Similarly, we fitted model (2.2) (with α2 replacing α1) to the morbidity number after February 6, 2020. We obtained α2β=0.1311, q=0.229, σ=0.051. The basic reproduction number is R0=α2βq=0.5725. This reduction in R0 was due to further strengthened quarantine measure in Auhui.
The comparison between actual value and predicted value of daily morbidity in phase Ⅲ is show in Figure 5. Under the policies of Wuhan lockdown and centralized quarantined management in Anhui, the morbidity number has been reduced and sharply decreased.
The parameter descriptions and estimates are listed in Table 2.
Parameter | Description | Values | Methods |
β | phase Ⅰ transmission rate per capita per day | 0.4029 | MCMC |
q | rate of symptom onset of pre-symptomatic exposed cases | 0.229-0.41 | Data and MCMC |
α1β | phase Ⅱ transmission rate per capita per day | 0.208 | MCMC |
σ | The cure rate | 0.04-0.051 | Data and MCMC |
α2β | phase Ⅲ transmission rate per capita per day | 0.1311 | MCMC |
Finally, the real data during the whole epidemic period compared to the predicted value of the three-stages model is shown in Figures 6 & 7.
In order to view the effect of relevant measures taken by the government on epidemic prevention and control more intuitively, we considered a hypothetical scenario that neither Wuhan lockdown nor centralized quarantine measures were in place and compared the morbidity number with and without government measures in Figure 8.
Furthermore, we considered a second hypothetical scenario when the Wuhan lockdown was in place but no centralized quarantine measures in Anhui. The comparison of morbidity number of patients in Anhui is shown in Figure 9. It can be seen from the graph that the number of new cases of COVID-19 would continue for a long time, rather than decrease as seen now.
To sum up, it can be seen that Wuhan lockdown and centralized quarantine measure in Anhui had played a timely and effective role in the prevention and control of the epidemic.
Based on the results of time series analysis, and the different measures taken by the government as time nodes, this paper establishes a transmission dynamics model which is divided into three phases. It can be seen from the analysis results of each stage that under the gradual implementation of various government measures, the basic reproduction number of the transmission of COVID-19 in Anhui decreased gradually, from 2.9764 to 0.8667 and then to 0.5725. These result of simulations indicate that the epidemic in Anhui has been controlled and is now in a critical period of transmission interruption. At the same time, this also further showed that when a major epidemic occurs, the timely implementation of national policies plays an important role in the control of the epidemic.
In addition, from the analysis of the data and model results, it can be concluded that the Wuhan lockdown in the second phase reduced the rate of exposure of transmission substantially in Anhui province by 48.37%. A centralized quarantine measure adopted in Anhui reduced the rate of exposure by 67.46% or 36.97%) compared with the rate of exposure rate in phase Ⅰ or phase Ⅱ, respectively. Therefore, both Wuhan lockdown and Anhui timely centralized quarantine measures have greatly reduced the speed of the transmission. The concentration quarantine measures in Anhui caused a substantial decrease in the rate of exposure, thus should be considered timely and effective. From the perspective of public health, this work further illustrated the impact of national and provincial policies in public health.
This research is supported by National Natural Science Foundation of China (11401002, 11771001), Natural Science Fund for Colleges and Universities in Anhui Province (KJ2018A0029), Teaching Research Project of Anhui University (ZLTS2016065) and Anhui Provincial Department of Science and Technology, Anhui Provincial Health Commission Emergency Research Project "Epidemiological and clinical characteristics of new coronavirus pneumonia" (No. 202004a07020002).
The authors declare there is no conflicts of interest.
[1] |
Y. J. Zhang, Y. F. Wang, Y. X. Yan, J. Zhao, Z. M. Gao, Lmraoa: An improved arithmetic optimization algorithm with multi-leader and high-speed jumping based on opposition-based learning solving engineering and numerical problems, Alexandria Eng. J., 61 (2022), 12367–12403. https://doi.org/10.1016/j.aej.2022.06.017 doi: 10.1016/j.aej.2022.06.017
![]() |
[2] |
S. Singh, H. Singh, N. Mittal, A. G. Hussien, F. Sroubek, A feature level image fusion for night-vision context enhancement using arithmetic optimization algorithm based image segmentation, Expert Syst. Appl., 209 (2022), 118272. https://doi.org/10.1016/j.eswa.2022.118272 doi: 10.1016/j.eswa.2022.118272
![]() |
[3] |
A. G. Hussien, A. E. Hassanien, E. H. Houssein, M. Amin, A. T. Azar, New binary whale optimization algorithm for discrete optimization problems, Eng. Optimiz., 52 (2020), 945–959. https://doi.org/10.1080/0305215X.2019.1624740 doi: 10.1080/0305215X.2019.1624740
![]() |
[4] |
L. D. Giovanni, F. Pezzella, An improved genetic algorithm for the distributed and flexible job-shop scheduling problem, Eur. J. Oper. Res., 200 (2010), 395–408. https://doi.org/10.1016/j.ejor.2009.01.008 doi: 10.1016/j.ejor.2009.01.008
![]() |
[5] | A. G. Hussien, E. H. Houssein, A. E. Hassanien, A binary whale optimization algorithm with hyperbolic tangent fitness function for feature selection, in IEEE 2017 Eighth international conference on intelligent computing and information systems (ICICIS), (2017), 166–172. https://doi.org/10.1109/INTELCIS.2017.8260031 |
[6] |
A. G. Hussien, D. Oliva, E. H. Houssein, A. A. Juan, X. Yu, Binary whale optimization algorithm for dimensionality reduction, Mathematics, 8 (2020), 1821. https://doi.org/10.3390/math8101821 doi: 10.3390/math8101821
![]() |
[7] |
A. G. Hussien, M. Amin, A self-adaptive harris hawks optimization algorithm with opposition-based learning and chaotic local search strategy for global optimization and feature selection, Int. J. Mach. Learn. Cyb., 13 (2022), 309–336. https://doi.org/10.1007/s13042-021-01326-4 doi: 10.1007/s13042-021-01326-4
![]() |
[8] |
Q. Liu, N. Li, H. Jia, Q. Qi, L. Abualigah, Modified remora optimization algorithm for global optimization and multilevel thresholding image segmentation, Mathematics, 10 (2022), 1014. https://doi.org/10.3390/math10071014 doi: 10.3390/math10071014
![]() |
[9] |
A. A. Ewees, L. Abualigah, D. Yousri, A. T. Sahlol, M. A. Al-qaness, S. Alshathri, et al., Modified artificial ecosystem-based optimization for multilevel thresholding image segmentation, Mathematics, 9 (2021), 2363. https://doi.org/10.3390/math9192363 doi: 10.3390/math9192363
![]() |
[10] |
M. Besnassi, N. Neggaz, A. Benyettou, Face detection based on evolutionary haar filter, Pattern Anal. Appl., 23 (2020), 309–330. https://doi.org/10.1007/s10044-019-00784-5 doi: 10.1007/s10044-019-00784-5
![]() |
[11] | E. H. Houssein, M. Amin, A. G. Hussien, A. E. Hassanien, Swarming behaviour of salps algorithm for predicting chemical compound activities, in IEEE 2017 eighth international conference on intelligent computing and information systems (ICICIS), (2017), 315–320. https://doi.org/10.1109/INTELCIS.2017.8260072 |
[12] | H. Fathi, H. AlSalman, A. Gumaei, I. I. Manhrawy, A. G. Hussien, P. El-Kafrawy, An efficient cancer classification model using microarray and high-dimensional data, Comput. Intell. Neurosci., 2021 (2021). https://doi.org/10.1155/2021/7231126 |
[13] |
L. Abualigah, A. H. Gandomi, M. A. Elaziz, A. G. Hussien, A. M. Khasawneh, M. Alshinwan, et al., Nature-inspired optimization algorithms for text document clustering—a comprehensive analysis, Algorithms, 13 (2020), 345. https://doi.org/10.3390/a13120345 doi: 10.3390/a13120345
![]() |
[14] |
A. S. Sadiq, A. A. Dehkordi, S. Mirjalili, Q. V. Pham, Nonlinear marine predator algorithm: A cost-effective optimizer for fair power allocation in noma-vlc-b5g networks, Expert Syst. Appl., 203 (2022), 117395. https://doi.org/10.1016/j.eswa.2022.117395 doi: 10.1016/j.eswa.2022.117395
![]() |
[15] |
A. A. Dehkordi, A. S. Sadiq, S. Mirjalili, K. Z. Ghafoor, Nonlinear-based chaotic harris hawks optimizer: algorithm and internet of vehicles application, Appl. Soft Comput., 109 (2021), 107574. https://doi.org/10.1016/j.asoc.2021.107574 doi: 10.1016/j.asoc.2021.107574
![]() |
[16] | A. S. Sadiq, A. A. Dehkordi, S. Mirjalili, J. Too, P. Pillai, Trustworthy and efficient routing algorithm for iot-fintech applications using non-linear lévy brownian generalized normal distribution optimization, IEEE Internet Things, 2021. https://doi.org/10.1109/JIOT.2021.3109075 |
[17] |
H. Faris, S. Mirjalili, I. Aljarah, Automatic selection of hidden neurons and weights in neural networks using grey wolf optimizer based on a hybrid encoding scheme, Int. J. Mach. Learn. Cyb., 10 (2019), 2901–2920. https://doi.org/10.1007/s13042-018-00913-2 doi: 10.1007/s13042-018-00913-2
![]() |
[18] |
B. Cao, J. Zhao, P. Yang, Y. Gu, K. Muhammad, J. J. Rodrigues, et al., Multiobjective 3-d topology optimization of next-generation wireless data center network, IEEE Trans. Ind. Inf., 16 (2019), 3597–3605. https://doi.org/10.1109/TII.2019.2952565 doi: 10.1109/TII.2019.2952565
![]() |
[19] |
X. Fu, P. Pace, G. Aloi, L. Yang, G. Fortino, Topology optimization against cascading failures on wireless sensor networks using a memetic algorithm, Comput. Networks, 177 (2020), 107327. https://doi.org/10.1016/j.comnet.2020.107327 doi: 10.1016/j.comnet.2020.107327
![]() |
[20] |
L. Abualigah, A. Diabat, A comprehensive survey of the grasshopper optimization algorithm: results, variants, and applications, Neural Comput. Appl., 32 (2020), 15533–15556. https://doi.org/10.1007/s00521-020-04789-8 doi: 10.1007/s00521-020-04789-8
![]() |
[21] |
H. Chen, H. Qiao, L. Xu, Q. Feng, K. Cai, A fuzzy optimization strategy for the implementation of rbf lssvr model in vis–nir analysis of pomelo maturity, IEEE Trans. Ind. Inf., 15 (2019), 5971–5979. https://doi.org/10.1109/TII.2019.2933582 doi: 10.1109/TII.2019.2933582
![]() |
[22] |
H. G. Beyer, B. Sendhoff, Robust optimization–-a comprehensive survey, Comput. Method Appl. M., 196 (2007), 3190–3218. https://doi.org/10.1016/J.CMA.2007.03.003 doi: 10.1016/J.CMA.2007.03.003
![]() |
[23] |
D. Oliva, A. A. Ewees, M. A. E. Aziz, A. E. Hassanien, M. P. Cisneros, A chaotic improved artificial bee colony for parameter estimation of photovoltaic cells, Energies, 10 (2017), 865. https://doi.org/10.3390/en10070865 doi: 10.3390/en10070865
![]() |
[24] | J. Kennedy, R. Eberhart, Particle swarm optimization, in IEEE Proceedings of ICNN'95-International Conference on Neural Networks, 4 (1995), 1942–1948. https://doi.org/10.1109/ICNN.1995.488968 |
[25] |
D. Karaboga, C. Ozturk, A novel clustering approach: Artificial bee colony (abc) algorithm, Appl. Soft Comput., 11 (2011), 652–657. https://doi.org/10.1016/j.asoc.2009.12.025 doi: 10.1016/j.asoc.2009.12.025
![]() |
[26] | R. R. Mostafa, A. G. Hussien, M. A. Khan, S. Kadry, F. A. Hashim, Enhanced coot optimization algorithm for dimensionality reduction, in IEEE 2022 Fifth International Conference of Women in Data Science at Prince Sultan University (WiDS PSU), (2022), 43–48. https://10.1109/WiDS-PSU54548.2022.00020 |
[27] | J. H. Holland, Adaptation in Natural and Artificial Systems: An Introductory Analysis with Applications to Biology, Control, and Artificial Intelligence, MIT press, 1992. |
[28] |
A. H. Gandomi, A. H. Alavi, Krill herd: A new bio-inspired optimization algorithm, Commun. Nonlinear Sci., 17 (2012), 4831–4845. https://10.1016/j.cnsns.2012.05.010 doi: 10.1016/j.cnsns.2012.05.010
![]() |
[29] | Z. W. Geem, J. H. Kim, G. V. Loganathan, A new heuristic optimization algorithm: harmony search, Simulation, 76 (2001), 60–68. https://doi.org/0037-5497(2001)l:2<60:ANHOAH>2.0.TX;2-3 |
[30] |
F. A. Hashim, A. G. Hussien, Snake optimizer: A novel meta-heuristic optimization algorithm, Knowl.-Based Syst., 242 (2022), 108320. https://doi.org/10.1016/j.knosys.2022.108320 doi: 10.1016/j.knosys.2022.108320
![]() |
[31] |
G. G. Wang, S. Deb, Z. Cui, Monarch butterfly optimization, Neural Comput. Appl., 31 (2019), 1995–2014. https://doi.org/10.1007/s00521-015-1923-y doi: 10.1007/s00521-015-1923-y
![]() |
[32] |
S. Li, H. Chen, M. Wang, A. A. Heidari, S. Mirjalili, Slime mould algorithm: A new method for stochastic optimization, Future Gener. Comput. Syst., 111 (2020), 300–323. https://doi.org/10.1016/j.future.2020.03.055 doi: 10.1016/j.future.2020.03.055
![]() |
[33] |
G. G. Wang, Moth search algorithm: a bio-inspired metaheuristic algorithm for global optimization problems, Memet. Comput., 10 (2018), 151–164. https://doi.org/10.1007/s12293-016-0212-3 doi: 10.1007/s12293-016-0212-3
![]() |
[34] |
Y. Yang, H. Chen, A. A. Heidari, A. H. Gandomi, Hunger games search: Visions, conception, implementation, deep analysis, perspectives, and towards performance shifts, Expert Syst. Appl., 177 (2021), 114864. https://doi.org/10.1016/j.eswa.2021.114864 doi: 10.1016/j.eswa.2021.114864
![]() |
[35] |
I. Ahmadianfar, A. A. Heidari, A. H. Gandomi, X. Chu, H. Chen, Run beyond the metaphor: An efficient optimization algorithm based on runge kutta method, Expert Syst. Appl., 181 (2021), 115079. https://doi.org/10.1016/j.eswa.2021.115079 doi: 10.1016/j.eswa.2021.115079
![]() |
[36] |
I. Ahmadianfar, A. A. Heidari, S. Noshadian, H. Chen, A. H. Gandomi, Info: An efficient optimization algorithm based on weighted mean of vectors, Expert Syst. Appl., 195 (2022), 116516. https://doi.org/10.1016/j.eswa.2022.116516 doi: 10.1016/j.eswa.2022.116516
![]() |
[37] | A. G. Hussien, A. A. Heidari, X. Ye, G. Liang, H. Chen, Z. Pan, Boosting whale optimization with evolution strategy and gaussian random walks: an image segmentation method, Eng. Comput., (2022), 1–45. https://doi.org/10.1007/s00366-021-01542-0 |
[38] |
L. Abualigah, M. A. Elaziz, A. G. Hussien, B. Alsalibi, S. M. J. Jalali, A. H. Gandomi, Lightning search algorithm: A comprehensive survey, Appl. Intell., 51 (2021), 2353–23760. https://doi.org/10.1007/s10489-020-01947-2 doi: 10.1007/s10489-020-01947-2
![]() |
[39] |
A. S. Assiri, A. G. Hussien, M. Amin, Ant lion optimization: variants, hybrids, and applications, IEEE Access, 8 (2020), 77746–77764. https://doi.org/10.1109/ACCESS.2020.2990338 doi: 10.1109/ACCESS.2020.2990338
![]() |
[40] |
A. G. Hussien, M. Amin, M. Wang, G. Liang, A. Alsanad, A. Gumaei, et al., Crow search algorithm: Theory, recent advances, and applications, IEEE Access, 8 (2020), 173548–173565. https://doi.org/10.1109/ACCESS.2020.3024108 doi: 10.1109/ACCESS.2020.3024108
![]() |
[41] |
A. G. Hussien, M. Amin, M. A. E. Aziz, A comprehensive review of moth-flame optimisation: variants, hybrids, and applications, J. Exp. Theor. Artif., 32 (2020), 705–725. https://doi.org/10.1080/0952813X.2020.1737246 doi: 10.1080/0952813X.2020.1737246
![]() |
[42] |
R. Zheng, A. G. Hussien, H. M. Jia, L. Abualigah, S. Wang, D. Wu, An improved wild horse optimizer for solving optimization problems, Mathematics, 10 (2022), 1311. https://doi.org/10.3390/math10081311 doi: 10.3390/math10081311
![]() |
[43] |
S. Wang, A. G. Hussien, H. Jia, L. Abualigah, R. Zheng, Enhanced remora optimization algorithm for solving constrained engineering optimization problems, Mathematics, 10 (2022), 1696. https://doi.org/10.3390/math10101696 doi: 10.3390/math10101696
![]() |
[44] |
L. Wang, Q. Cao, Z. Zhang, S. Mirjalili, W. Zhao, Artificial rabbits optimization: A new bio-inspired meta-heuristic algorithm for solving engineering optimization problems, Eng. Appl. Artif. Intell., 114 (2022), 105082. https://doi.org/10.1016/j.engappai.2022.105082 doi: 10.1016/j.engappai.2022.105082
![]() |
[45] |
W. Zhao, Z. Zhang, S. Mirjalili, L. Wang, N. Khodadadi, S. M. Mirjalili, An effective multi-objective artificial hummingbird algorithm with dynamic elimination-based crowding distance for solving engineering design problems, Comput. Method. Appl. M., 398 (2022), 115223. https://doi.org/10.1016/j.cma.2022.115223 doi: 10.1016/j.cma.2022.115223
![]() |
[46] |
S. Saremi, S. Mirjalili, A. Lewis, Grasshopper optimisation algorithm: theory and application, Adv. Eng. Software., 105 (2017), 30–47. https://doi.org/10.1016/j.advengsoft.2017.01.004 doi: 10.1016/j.advengsoft.2017.01.004
![]() |
[47] |
S. Mirjalili, S. M. Mirjalili, A. Lewis, Grey wolf optimizer, Adv. Eng. Software, 69 (2014), 46–61. https://doi.org/10.1016/j.advengsoft.2013.12.007 doi: 10.1016/j.advengsoft.2013.12.007
![]() |
[48] | E. H. Houssein, A. G. Hussien, A. E. Hassanien, S. Bhattacharyya, M. Amin, S-shaped binary whale optimization algorithm for feature selection, in First International Symposium on Signal and Image Processing (ISSIP 2017), 2017. 79–87. |
[49] |
L. Abualigah, D. Yousri, M. A. Elaziz, A. A. Ewees, M. A. Al-Qaness, A. H. Gandomi, Aquila optimizer: a novel meta-heuristic optimization algorithm, Comput. Ind. Eng., 157 (2021), 107250. https://doi.org/10.1016/j.cie.2021.107250 doi: 10.1016/j.cie.2021.107250
![]() |
[50] |
S. Wang, H. Jia, L. Abualigah, Q. Liu, R. Zheng, An improved hybrid aquila optimizer and harris hawks algorithm for solving industrial engineering optimization problems, Processes, 9 (2021), 1551. https://doi.org/10.3934/mbe.2021352 doi: 10.3934/mbe.2021352
![]() |
[51] |
S. Mahajan, L. Abualigah, A. K. Pandit, M. Altalhi, Hybrid aquila optimizer with arithmetic optimization algorithm for global optimization tasks, Soft Comput., 26 (2022), 4863–4881. https://doi.org/10.1007/s00500-022-06873-8 doi: 10.1007/s00500-022-06873-8
![]() |
[52] |
L. Abualigah, A. Diabat, S. Mirjalili, M. A. Elaziz, A. H. Gandomi, The arithmetic optimization algorithm, Comput. Methods Appl. Mech. Eng., 376 (2021), 113609. https://doi.org/10.1016/j.cma.2020.113609 doi: 10.1016/j.cma.2020.113609
![]() |
[53] |
Y. J. Zhang, Y. X. Yan, J. Zhao, Z. M. Gao, Aoaao: The hybrid algorithm of arithmetic optimization algorithm with aquila optimizer, IEEE Access, 10 (2022), 10907–10933. https://doi.org/10.1109/ACCESS.2022.3144431 doi: 10.1109/ACCESS.2022.3144431
![]() |
[54] |
J. Zhao, Z. M. Gao, H. F. Chen, The simplified aquila optimization algorithm, IEEE Access, 10 (2022), 22487–22515. https://doi.org/10.1109/ACCESS.2022.3153727 doi: 10.1109/ACCESS.2022.3153727
![]() |
[55] |
C. Ma, H. Huang, Q. Fan, J. Wei, Y. Du, W. Gao, Grey wolf optimizer based on aquila exploration method, Expert Syst. Appl., 205 (2022), 117629. https://doi.org/10.1016/j.eswa.2022.117629 doi: 10.1016/j.eswa.2022.117629
![]() |
[56] |
B. Gao, Y. Shi, F. Xu, X. Xu, An improved aquila optimizer based on search control factor and mutations, Processes, 10 (2022), 1451. https://doi.org/10.3390/pr10081451 doi: 10.3390/pr10081451
![]() |
[57] |
A. M. AlRassas, M. A. Al-qaness, A. A. Ewees, S. Ren, M. A. Elaziz, R. Damaševičius, et al., Optimized anfis model using aquila optimizer for oil production forecasting, Processes, 9 (2021), 1194. https://doi.org/10.3390/pr9071194 doi: 10.3390/pr9071194
![]() |
[58] |
M. A. Elaziz, A. Dahou, N. A. Alsaleh, A. H. Elsheikh, A. I. Saba, M. Ahmadein, Boosting covid-19 image classification using mobilenetv3 and aquila optimizer algorithm, Entropy, 23 (2021), 1383. https://doi.org/10.3390/e23111383 doi: 10.3390/e23111383
![]() |
[59] |
A. Fatani, A. Dahou, M. A. Al-Qaness, S. Lu, M. A. Elaziz, Advanced feature extraction and selection approach using deep learning and aquila optimizer for iot intrusion detection system, Sensors, 22 (2021), 140. https://doi.org/10.3390/s22010140 doi: 10.3390/s22010140
![]() |
[60] | G. G. Wang, S. Deb, L. D. S. Coelho, Elephant herding optimization, in IEEE 2015 3rd International Symposium on Computational and Business Intelligence (ISCBI), (2015), 1–5. https://doi.org/10.1109/ISCBI.2015.8 |
[61] | R. Tanabe, A. S. Fukunaga, Improving the search performance of shade using linear population size reduction, in 2014 IEEE Congress on Evolutionary Computation (CEC), (2014), 1658–1665. https://doi.org/10.1109/CEC.2014.6900380 |
[62] | N. H. Awad, M. Z. Ali, P. N. Suganthan, Ensemble sinusoidal differential covariance matrix adaptation with euclidean neighborhood for solving cec2017 benchmark problems, in 2017 IEEE Congress on Evolutionary Computation (CEC), (2017), 372–379. https://doi.org/10.1109/CEC.2017.7969336 |
[63] |
S. Mirjalili, Moth-flame optimization algorithm: A novel nature-inspired heuristic paradigm, Knowl.-Based Syst., 89 (2015), 228–249. https://doi.org/10.1016/j.knosys.2015.07.006 doi: 10.1016/j.knosys.2015.07.006
![]() |
[64] |
S. Mirjalili, S. M. Mirjalili, A. Hatamlou, Multi-verse optimizer: a nature-inspired algorithm for global optimization, Neural Comput. Appl., 27 (2016), 495–513. https://doi.org/10.1007/s00521-015-1870-7 doi: 10.1007/s00521-015-1870-7
![]() |
[65] | K. Steenhof, M. N. Kochert, T. L. Mcdonald, Interactive effects of prey and weather on golden eagle reproduction, J. Anim. Ecol., 66 (1997), 350–362. |
[66] | H. R. Tizhoosh, Opposition-based learning: a new scheme for machine intelligence, in IEEE International Conference on Computational Intelligence for Modelling, Control and Automation and International Conference on Intelligent Agents, Web Technologies and Internet Commerce (CIMCA-IAWTIC'06), 1 (2005), 695–701. https://doi.org/10.1109/CIMCA.2005.1631345 |
[67] |
A. G. Hussien, An enhanced opposition-based salp swarm algorithm for global optimization and engineering problems, J. Amb. Intell. Hum. Comput., 13 (2022), 129–150. https://doi.org/10.1007/s12652-021-02892-9 doi: 10.1007/s12652-021-02892-9
![]() |
[68] |
H. Chen, Y. Xu, M. Wang, X. Zhao, A balanced whale optimization algorithm for constrained engineering design problems, Appl. Math. Modell., 71 (2019), 45–59. https://doi.org/10.1016/j.apm.2019.02.004 doi: 10.1016/j.apm.2019.02.004
![]() |
[69] |
Y. Yu, S. Gao, S. Cheng, Y. Wang, S. Song, F. Yuan, Cbso: A memetic brain storm optimization with chaotic local search, Memet. Comput., 10 (2018), 353–367. https://doi.org/10.1007/s12293-017-0247-0 doi: 10.1007/s12293-017-0247-0
![]() |
[70] |
J. Zhao, Y. Zhang, S. Li, Y. Wang, Y. Yan, Z. Gao, A chaotic self-adaptive jaya algorithm for parameter extraction of photovoltaic models, Math. Biosci. Eng., 19 (2022), 5638–5670. https://doi.org/10.3934/mbe.2022264 doi: 10.3934/mbe.2022264
![]() |
[71] |
H. Zhang, Z. Wang, W. Chen, A. A. Heidari, M. Wang, X. Zhao, et al., Ensemble mutation-driven salp swarm algorithm with restart mechanism: Framework and fundamental analysis, Expert Syst. Appl., 165 (2021), 113897. https://doi.org/10.1016/j.eswa.2020.113897 doi: 10.1016/j.eswa.2020.113897
![]() |
[72] |
Y. Zhang, Y. Wang, S. Li, F. Yao, L. Tao, Y. Yan, et al., An enhanced adaptive comprehensive learning hybrid algorithm of rao-1 and jaya algorithm for parameter extraction of photovoltaic models, Math. Biosci. Eng., 19 (2022), 5610–5637. https://doi.org/10.3934/mbe.2022263 doi: 10.3934/mbe.2022263
![]() |
[73] |
Y. J. Zhang, Y. X. Yan, J. Zhao, Z. M. Gao, Cscahho: Chaotic hybridization algorithm of the sine cosine with harris hawk optimization algorithms for solving global optimization problems, Plos One, 17 (2022), e0263387. https://doi.org/10.1371/journal.pone.0263387 doi: 10.1371/journal.pone.0263387
![]() |
[74] |
M. Y. Cheng, D. Prayogo, A novel fuzzy adaptive teaching–learning-based optimization (fatlbo) for solving structural optimization problems, Eng. Comput., 33 (2017), 55–69. https://doi.org/10.1007/s00366-016-0456-z doi: 10.1007/s00366-016-0456-z
![]() |
[75] |
H. Samma, J. Mohamad-Saleh, S. A. Suandi, B. Lahasan, Q-learning-based simulated annealing algorithm for constrained engineering design problems, Neural Comput. Appl., 32 (2020), 5147–5161. https://doi.org/10.1007/s00521-019-04008-z doi: 10.1007/s00521-019-04008-z
![]() |
[76] |
C. A. C. Coello, Use of a self-adaptive penalty approach for engineering optimization problems, Comput. Ind., 41 (2000), 113–127. https://doi.org/10.1016/S0166-3615(99)00046-9 doi: 10.1016/S0166-3615(99)00046-9
![]() |
[77] |
K. Deb, Optimal design of a welded beam via genetic algorithms, AIAA J., 29 (1991), 2013–2015. https://doi.org/10.2514/3.10834 doi: 10.2514/3.10834
![]() |
[78] |
S. Mirjalili, A. H. Gandomi, S. Z. Mirjalili, S. Saremi, H. Faris, S. M. Mirjalili, Salp swarm algorithm: A bio-inspired optimizer for engineering design problems, Adv. Eng. Software, 114 (2017), 163–191. https://doi.org/10.1016/j.advengsoft.2017.07.002 doi: 10.1016/j.advengsoft.2017.07.002
![]() |
[79] |
A. Faramarzi, M. Heidarinejad, S. Mirjalili, A. H. Gandomi, Marine predators algorithm: A nature-inspired metaheuristic, Expert Syst. Appl., 152 (2020), 113377. https://doi.org/10.1016/j.eswa.2020.113377 doi: 10.1016/j.eswa.2020.113377
![]() |
[80] |
A. A. Heidari, S. Mirjalili, H. Faris, I. Aljarah, M. Mafarja, H. Chen, Harris hawks optimization: Algorithm and applications, Future Gener. Comput. Syst., 97 (2019), 849–872. https://doi.org/10.1016/j.future.2019.02.028 doi: 10.1016/j.future.2019.02.028
![]() |
[81] |
A. G. Hussien, L. Abualigah, R. A. Zitar, F. A. Hashim, M. Amin, A. Saber, et al., Recent advances in harris hawks optimization: A comparative study and applications, Electronics, 11 (2022), 1919. https://doi.org/10.3390/electronics11121919 doi: 10.3390/electronics11121919
![]() |
[82] |
S. Mirjalili, A. Lewis, The whale optimization algorithm, Adv. Eng. Software, 95 (2016), 51–67. https://doi.org/10.1016/j.advengsoft.2016.01.008 doi: 10.1016/j.advengsoft.2016.01.008
![]() |
[83] |
B. Kannan, S. N. Kramer, An augmented lagrange multiplier based method for mixed integer discrete continuous optimization and its applications to mechanical design, J. Mech. Design, 116 (1994), 405–411. https://doi.org/10.1115/1.2919393 doi: 10.1115/1.2919393
![]() |
[84] |
H. Liu, Z. Cai, Y. Wang, Hybridizing particle swarm optimization with differential evolution for constrained numerical and engineering optimization, Appl. Soft Comput., 10 (2010), 629–640. https://doi.org/10.1016/j.asoc.2009.08.031 doi: 10.1016/j.asoc.2009.08.031
![]() |
[85] |
M. Mahdavi, M. Fesanghary, E. Damangir, An improved harmony search algorithm for solving optimization problems, Appl. Math. Comput., 188 (2007), 1567–1579. https://doi.org/10.1016/j.amc.2006.11.033 doi: 10.1016/j.amc.2006.11.033
![]() |
[86] | J. Zhao, Z. M. Gao, W. Sun, The improved slime mould algorithm with levy flight, in Journal of Physics: Conference Series, 1617 (2020), 012033. https://doi.org/10.1088/1742-6596/1617/1/012033 |
[87] |
Q. He, L. Wang, An effective co-evolutionary particle swarm optimization for constrained engineering design problems, Eng. Appl. Artif. Intell., 20 (2007), 89–99. https://doi.org/10.1016/j.engappai.2006.03.003 doi: 10.1016/j.engappai.2006.03.003
![]() |
[88] |
A. Kaveh, A. Dadras, A novel meta-heuristic optimization algorithm: Thermal exchange optimization, Adv. Eng. Software, 110 (2017), 69–84. https://doi.org/10.1016/j.advengsoft.2017.03.014 doi: 10.1016/j.advengsoft.2017.03.014
![]() |
[89] | J. S. Arora, Introduction to optimum design, Elsevier, 2004. |
[90] |
A. Kaveh, M. Khayatazad, A new meta-heuristic method: Ray optimization, Comput. Struct., 112 (2012), 283–294. https://doi.org/10.1016/j.compstruc.2012.09.003 doi: 10.1016/j.compstruc.2012.09.003
![]() |
[91] |
E. Mezura-Montes, C. A. C. Coello, An empirical study about the usefulness of evolution strategies to solve constrained optimization problems, Int. J. Gen. Syst., 37 (2008), 443–473. https://doi.org/10.1080/03081070701303470 doi: 10.1080/03081070701303470
![]() |
[92] |
M. A. Elaziz, D. Oliva, S. Xiong, An improved opposition-based sine cosine algorithm for global optimization, Expert Syst. Appl., 90 (2017), 484–500. https://doi.org/10.1016/j.eswa.2017.07.043 doi: 10.1016/j.eswa.2017.07.043
![]() |
[93] |
E. Rashedi, H. Nezamabadi-Pour, S. Saryazdi, Gsa: A gravitational search algorithm, Inf. Sci, 179 (2009), 2232–2248. https://doi.org/10.1016/j.ins.2009.03.004 doi: 10.1016/j.ins.2009.03.004
![]() |
[94] | E. Mezura-Montes, C. A. C. Coello, Useful infeasible solutions in engineering optimization with evolutionary algorithms, in Mexican International Conference on Artificial Intelligence, 3789 (2005), 652–662. https://doi.org/10.1007/11579427_66 |
[95] | S. Stephen, D. Christu, A. Dalvi, Design optimization of weight of speed reducer problem through matlab and simulation using ansys, Int. J. Mech. Eng. Technol., 9 (2018), 339–349. |
[96] |
S. Lu, H. M. Kim, A regularized inexact penalty decomposition algorithm for multidisciplinary design optimization problems with complementarity constraints, J. Mech. Design, 132 (2010), 041005. https://doi.org/10.1115/1.4001206 doi: 10.1115/1.4001206
![]() |
[97] |
S. Mirjalili, Sca: A sine cosine algorithm for solving optimization problems, Knowl.-Based Syst., 96 (2016), 120–133. https://doi.org/10.1016/j.knosys.2015.12.022 doi: 10.1016/j.knosys.2015.12.022
![]() |
[98] | E. Mezura-Montes, C. C. Coello, R. Landa-Becerra, Engineering optimization using simple evolutionary algorithm, in Proceedings. 15th IEEE International Conference on Tools with Artificial Intelligence, (2003), 149–156. https://doi.org/10.1109/TAI.2003.1250183 |
[99] |
S. Akhtar, K. Tai, T. Ray, A socio-behavioural simulation model for engineering design optimization, Eng. Optimiz., 34 (2002), 341–354. https://doi.org/10.1080/03052150212723 doi: 10.1080/03052150212723
![]() |
[100] |
V. K. Kamboj, A. Nandi, A. Bhadoria, S. Sehgal, An intensify harris hawks optimizer for numerical and engineering optimization problems, Appl. Soft Comput., 89 (2020), 106018. https://doi.org/10.1016/j.asoc.2019.106018 doi: 10.1016/j.asoc.2019.106018
![]() |
[101] | H. Nowacki, Optimization in pre-contract ship design, In International Conference on Computer Applications in the Automation of Shipyard Operation and Ship Design, 1973. |
[102] |
A. H. Gandomi, X. S. Yang, A. H. Alavi, Cuckoo search algorithm: a metaheuristic approach to solve structural optimization problems, Eng. Comput., 29 (2013), 17–35. https://doi.org/10.1007/s00366-011-0241-y doi: 10.1007/s00366-011-0241-y
![]() |
[103] |
M. Zhang, W. Luo, X. Wang, Differential evolution with dynamic stochastic selection for constrained optimization, Inf. Sci., 178 (2008), 3043–3074. https://doi.org/10.1016/j.ins.2008.02.014 doi: 10.1016/j.ins.2008.02.014
![]() |
[104] |
A. Sadollah, A. Bahreininejad, H. Eskandar, M. Hamdi, Mine blast algorithm: A new population based algorithm for solving constrained engineering optimization problems, Appl. Soft Comput., 13 (2013), 2592–2612. https://doi.org/10.1016/j.asoc.2012.11.026 doi: 10.1016/j.asoc.2012.11.026
![]() |
[105] | A. E. YILDIRIM, A. Karci, Application of three bar truss problem among engineering design optimization problems using artificial atom algorithm, in IEEE 2018 International Conference on Artificial Intelligence and Data Processing (IDAP), (2018), 1–5. https://doi.org/10.1109/IDAP.2018.8620762 |
1. | Guo-Rong Xing, Ming-Tao Li, Li Li, Gui-Quan Sun, The Impact of Population Migration on the Spread of COVID-19: A Case Study of Guangdong Province and Hunan Province in China, 2020, 8, 2296-424X, 10.3389/fphy.2020.587483 | |
2. | Wei Yang, Modeling COVID-19 Pandemic with Hierarchical Quarantine and Time Delay, 2021, 2153-0785, 10.1007/s13235-021-00382-3 | |
3. | Bootan Rahman, Evar Sadraddin, Annamaria Porreca, The basic reproduction number of SARS‐CoV‐2 in Wuhan is about to die out, how about the rest of the World?, 2020, 30, 1052-9276, 10.1002/rmv.2111 | |
4. | Roman Cherniha, Vasyl’ Davydovych, A Mathematical Model for the COVID-19 Outbreak and Its Applications, 2020, 12, 2073-8994, 990, 10.3390/sym12060990 | |
5. | Ghulam Hussain, Tahir Khan, Amir Khan, Mustafa Inc, Gul Zaman, Kottakkaran Sooppy Nisar, Ali Akgül, Modeling the dynamics of novel coronavirus (COVID-19) via stochastic epidemic model, 2021, 60, 11100168, 4121, 10.1016/j.aej.2021.02.036 | |
6. | Haitao Song, Feng Li, Zhongwei Jia, Zhen Jin, Shengqiang Liu, Using traveller-derived cases in Henan Province to quantify the spread of COVID-19 in Wuhan, China, 2020, 101, 0924-090X, 1821, 10.1007/s11071-020-05859-1 | |
7. | Tingzhe Sun, Yan Wang, Modeling COVID-19 epidemic in Heilongjiang province, China, 2020, 138, 09600779, 109949, 10.1016/j.chaos.2020.109949 | |
8. | Dhiraj Kumar Das, Anupam Khatua, T.K. Kar, Soovoojeet Jana, The effectiveness of contact tracing in mitigating COVID-19 outbreak: A model-based analysis in the context of India, 2021, 00963003, 126207, 10.1016/j.amc.2021.126207 | |
9. | Wei Wei, Bowen Duan, Min Zuo, Quanmin Zhu, An extended state observer based U-model control of the COVID-19, 2021, 00190578, 10.1016/j.isatra.2021.02.039 | |
10. | Prasantha Bharathi Dhandapani, Dumitru Baleanu, Jayakumar Thippan, Vinoth Sivakumar, On stiff, fuzzy IRD-14 day average transmission model of COVID-19 pandemic disease, 2020, 7, 2375-1495, 208, 10.3934/bioeng.2020018 | |
11. | Tahir Khan, Gul Zaman, Youssef El Khatib, Modeling the dynamics of novel coronavirus (COVID-19) via stochastic epidemic model, 2021, 22113797, 104004, 10.1016/j.rinp.2021.104004 | |
12. | Haitao Song, Zhongwei Jia, Zhen Jin, Shengqiang Liu, Estimation of COVID-19 outbreak size in Harbin, China, 2021, 0924-090X, 10.1007/s11071-021-06406-2 | |
13. | Orwa Al-Abdulla, Agneta Kallström, Camilo Valderrama, Jussi Kauhanen, Simulation of the Progression of the COVID-19 Outbreak in Northwest Syria Using a Basic and Adjusted SIR Model, 2022, 2, 2813-0227, 44, 10.3390/zoonoticdis2020006 | |
14. | S. A. Alblowi, M. El Sayed, M. A. El Safty, Decision Making Based on Fuzzy Soft Sets and Its Application in COVID-19, 2021, 30, 1079-8587, 961, 10.32604/iasc.2021.018242 | |
15. | Caitriona Murphy, Jessica Y. Wong, Benjamin J. Cowling, Nonpharmaceutical interventions for managing SARS-CoV-2, 2023, Publish Ahead of Print, 1070-5287, 10.1097/MCP.0000000000000949 | |
16. | Wenjing Zhao, Lin Zhang, Junling Wang, Lili Wang, How has academia responded to the urgent needs created by COVID-19? A multi-level global, regional and national analysis, 2022, 0165-5515, 016555152210846, 10.1177/01655515221084646 | |
17. | Juan Luis Fernández-Martínez, Zulima Fernández-Muñiz, Ana Cernea, Andrzej Kloczkowski, Ezequiel López-Rubio, Predictive Mathematical Models of the Short-Term and Long-Term Growth of the COVID-19 Pandemic, 2021, 2021, 1748-6718, 1, 10.1155/2021/5556433 | |
18. | M. El Sayed, M. A. El Safty, M. K. El-Bably, Topological approach for decision-making of COVID-19 infection via a nano-topology model, 2021, 6, 2473-6988, 7872, 10.3934/math.2021457 | |
19. | ROMAN M. CHERNIHA, VASYL V. DAVYDOVYCH, A reaction–diffusion system with cross-diffusion: Lie symmetry, exact solutions and their applications in the pandemic modelling, 2022, 33, 0956-7925, 785, 10.1017/S095679252100022X | |
20. | Junaid Iqbal Khan, Jebran Khan, Furqan Ali, Farman Ullah, Jamshid Bacha, Sungchang Lee, Artificial Intelligence and Internet of Things (AI-IoT) Technologies in Response to COVID-19 Pandemic: A Systematic Review, 2022, 10, 2169-3536, 62613, 10.1109/ACCESS.2022.3181605 | |
21. | Haitao Song, Fang Liu, Feng Li, Xiaochun Cao, Hao Wang, Zhongwei Jia, Huaiping Zhu, Michael Y. Li, Wei Lin, Hong Yang, Jianghong Hu, Zhen Jin, Modeling the second outbreak of COVID-19 with isolation and contact tracing, 2022, 27, 1531-3492, 5757, 10.3934/dcdsb.2021294 | |
22. | Haiyin Li, Yan Wu, Toshikazu Kuniya, Dynamics of SCIR Modeling for COVID-19 with Immigration, 2022, 2022, 1099-0526, 1, 10.1155/2022/9182830 | |
23. | Bootan Rahman, Sarbaz H. A. Khoshnaw, Grace O. Agaba, Fahad Al Basir, How Containment Can Effectively Suppress the Outbreak of COVID-19: A Mathematical Modeling, 2021, 10, 2075-1680, 204, 10.3390/axioms10030204 | |
24. | Ting Zeng, Zhidong Teng, Ramziya Rifhat, Xiaodong Wang, Lei Wang, Kai Wang, Analysis and simulation of a stochastic COVID-19 model with large-scale nucleic acid detection and isolation measures: A case study of the outbreak in Urumqi, China in August, 2022, 2023, 24680427, 10.1016/j.idm.2023.03.004 | |
25. | S. Sankaranarayanan, An approach to statistical analysis-using the average transmission model of Covid 19, 2020, 8, 23193786, 2158, 10.26637/MJM0804/0141 | |
26. | Alaa Falih Mahdi, Hussein K. Asker, A Sensitivity Analysis of the PSITPS Epidemic Model’s Parameters for COVID-19, 2024, 11, 2518-0010, 79, 10.31642/JoKMC/2018/110210 | |
27. | Akash Pal, Garima Jain, Ishita Roy, Sumit Sharma, 2023, Chapter 37, 978-981-19-5190-9, 453, 10.1007/978-981-19-5191-6_37 |
Parameter | Estimate | p-value |
β0 | 7.076923 | 0.0778 |
β1 | 2.923077 | 0.0000 |
β2 | 38.29670 | 0.0000 |
β3 | -6.057143 | 0.0000 |
β4 | -4.742857 | 0.5012 |
β5 | -0.323077 | 0.8442 |
Parameter | Description | Values | Methods |
β | phase Ⅰ transmission rate per capita per day | 0.4029 | MCMC |
q | rate of symptom onset of pre-symptomatic exposed cases | 0.229-0.41 | Data and MCMC |
α1β | phase Ⅱ transmission rate per capita per day | 0.208 | MCMC |
σ | The cure rate | 0.04-0.051 | Data and MCMC |
α2β | phase Ⅲ transmission rate per capita per day | 0.1311 | MCMC |
Parameter | Estimate | p-value |
β0 | 7.076923 | 0.0778 |
β1 | 2.923077 | 0.0000 |
β2 | 38.29670 | 0.0000 |
β3 | -6.057143 | 0.0000 |
β4 | -4.742857 | 0.5012 |
β5 | -0.323077 | 0.8442 |
Parameter | Description | Values | Methods |
β | phase Ⅰ transmission rate per capita per day | 0.4029 | MCMC |
q | rate of symptom onset of pre-symptomatic exposed cases | 0.229-0.41 | Data and MCMC |
α1β | phase Ⅱ transmission rate per capita per day | 0.208 | MCMC |
σ | The cure rate | 0.04-0.051 | Data and MCMC |
α2β | phase Ⅲ transmission rate per capita per day | 0.1311 | MCMC |