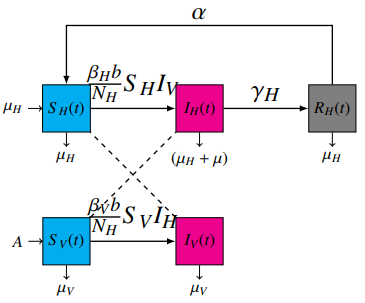
Traditional lectures are commonly understood to be a teacher-centered mode of instruction where the main aim is a provision of explanations by an educator to the students. Recent literature in higher education overwhelmingly depicts this mode of instruction as inferior compared to the desired student-centered models based on active learning techniques. First, using a four-quadrant model of educational environments, we address common confusion related to a conflation of two prevalent dichotomies by focusing on two key dimensions: (1) the extent to which students are prompted to engage actively and (2) the extent to which expert explanations are provided. Second, using a case study, we describe an evolution of tertiary mathematics education, showing how traditional instruction can still play a valuable role, provided it is suitably embedded in a student-centered course design. We support our argument by analyzing the teaching practice and learning environment in a third-year abstract algebra course through the lens of Stanislas Dehaene's theoretical framework for effective teaching and learning. The framework, comprising "four pillars of learning", is based on a state-of-the-art conception of how learning can be facilitated according to cognitive science, educational psychology and neuroscience findings. In the case study, we illustrate how, over time, the unit design and the teaching approach have evolved into a learning environment that aligns with the four pillars of learning. We conclude that traditional lectures can and do evolve to optimize learning environments and that the erection of the dichotomy "traditional instruction versus active learning" is no longer relevant.
Citation: Heiko Dietrich, Tanya Evans. Traditional lectures versus active learning – A false dichotomy?[J]. STEM Education, 2022, 2(4): 275-292. doi: 10.3934/steme.2022017
[1] | Anita T. Kurniawati, Fatmawati, Chidozie W. Chukwu, Windarto, Faishal F. Herdicho . Optimal control of dengue fever model with a logistically growing human population. Mathematical Modelling and Control, 2025, 5(1): 48-60. doi: 10.3934/mmc.2025004 |
[2] | Rashid Jan, Normy Norfiza Abdul Razak, Sania Qureshi, Imtiaz Ahmad, Salma Bahramand . Modeling Rift Valley fever transmission: insights from fractal-fractional dynamics with the Caputo derivative. Mathematical Modelling and Control, 2024, 4(2): 163-177. doi: 10.3934/mmc.2024015 |
[3] | Yanchao He, Yuzhen Bai . Finite-time stability and applications of positive switched linear delayed impulsive systems. Mathematical Modelling and Control, 2024, 4(2): 178-194. doi: 10.3934/mmc.2024016 |
[4] | S. Y. Tchoumi, Y. Kouakep-Tchaptchie, D. J. Fotsa-Mbogne, J. C. Kamgang, J. M. Tchuenche . Optimal control of a malaria model with long-lasting insecticide-treated nets. Mathematical Modelling and Control, 2021, 1(4): 188-207. doi: 10.3934/mmc.2021018 |
[5] | Mlyashimbi Helikumi, Paride O. Lolika . Dynamics and analysis of COVID-19 disease transmission: The effect of vaccination and quarantine. Mathematical Modelling and Control, 2023, 3(3): 192-209. doi: 10.3934/mmc.2023017 |
[6] | Erick Manuel Delgado Moya, Diego Samuel Rodrigues . Fractional order modeling for injectable and oral HIV pre-exposure prophylaxis. Mathematical Modelling and Control, 2023, 3(2): 139-151. doi: 10.3934/mmc.2023013 |
[7] | Sifan Song, Huilan Li . A Hopf algebra on (0,1)-matrices. Mathematical Modelling and Control, 2025, 5(2): 193-201. doi: 10.3934/mmc.2025014 |
[8] | Abdul-Fatawu O. Ayembillah, Baba Seidu, C. S. Bornaa . Mathematical modeling of the dynamics of maize streak virus disease (MSVD). Mathematical Modelling and Control, 2022, 2(4): 153-164. doi: 10.3934/mmc.2022016 |
[9] | Yaxin Ren, Yakui Xue . Modeling and optimal control of COVID-19 and malaria co-infection based on vaccination. Mathematical Modelling and Control, 2024, 4(3): 316-335. doi: 10.3934/mmc.2024026 |
[10] | J. O. Akanni, S. Ajao, S. F. Abimbade, Fatmawati . Dynamical analysis of COVID-19 and tuberculosis co-infection using mathematical modelling approach. Mathematical Modelling and Control, 2024, 4(2): 208-229. doi: 10.3934/mmc.2024018 |
Traditional lectures are commonly understood to be a teacher-centered mode of instruction where the main aim is a provision of explanations by an educator to the students. Recent literature in higher education overwhelmingly depicts this mode of instruction as inferior compared to the desired student-centered models based on active learning techniques. First, using a four-quadrant model of educational environments, we address common confusion related to a conflation of two prevalent dichotomies by focusing on two key dimensions: (1) the extent to which students are prompted to engage actively and (2) the extent to which expert explanations are provided. Second, using a case study, we describe an evolution of tertiary mathematics education, showing how traditional instruction can still play a valuable role, provided it is suitably embedded in a student-centered course design. We support our argument by analyzing the teaching practice and learning environment in a third-year abstract algebra course through the lens of Stanislas Dehaene's theoretical framework for effective teaching and learning. The framework, comprising "four pillars of learning", is based on a state-of-the-art conception of how learning can be facilitated according to cognitive science, educational psychology and neuroscience findings. In the case study, we illustrate how, over time, the unit design and the teaching approach have evolved into a learning environment that aligns with the four pillars of learning. We conclude that traditional lectures can and do evolve to optimize learning environments and that the erection of the dichotomy "traditional instruction versus active learning" is no longer relevant.
Dengue fever, which is caused by the dengue virus, is known as one of the most important tropical diseases. There is a circle of dengue virus transmission that concerns humans and mosquitoes; therefore, dengue fever is also called a mosquito-borne disease. The spread of the virus occurs through the bite of an infected female Aedes Aegypti mosquito. So far, four serotypes have been detected for the dengue virus: DEN1, DEN2, DEN3, and DEN4 [1]. Humans can be immune to one serotype but can be reinfected by another serotype. Thus, humans living in areas endemic to dengue disease can be reinfected 3 or 4 times by viruses with different serotypes. Since the period of mosquito infection ends through death, the mosquitoes never recover from the infection [1]. The virus has an incubation period of 4 to 10 days for the mosquito, during which, it becomes an infected vector. Suppose the infected mosquito bites a human who is susceptible to the virus. In that case, the virus will enter the human body until it can be transmitted back to another mosquito. Infected humans can transmit the virus through the Aedes Aegypti mosquito for 4 to 5 days after their first symptoms appear [2].
Every year, approximately 100–400 million people are infected by the dengue virus [3]. The COVID-19 pandemic more or less changed the dynamics of dengue fever cases. Medical reports which focused on this outbreak showed that the dengue disease had lower reporting rates. Dengue fever cases experienced a decline between 2020–2022 (during the COVID-19 pandemic). As a result, in 2023, the dengue fever cases significantly increased globally. In particular, Indonesia has become one of the Southeast Asian countries, ranked among the world's 30 most susceptible to an endemic [4]. This condition becomes a challenge for researchers to reduce the endemicity of dengue fever that occurs year to year.
Understanding the transmission of disease from a mathematical perspective involves the use of mathematical models. Large-scale data-based simulations can be conducted using mathematical models to estimate the spread of epidemics [5,6]. However, it is also crucial to theoretically study epidemic models to understand their qualitative behavior. This understanding is valuable to inform the control policies. Disease epidemics have another point of view in memory and can have wide-ranging effects in the fractional models using integer order, as studied by [7,8]. In particular, mathematical modeling concerning the cycle of transmission of the dengue virus has been explored, such as in the host-vector model by the author in [9,10,11,12]. The author in [10] modified the model in [9] regarding the incubation period, that is, a percentage of infected humans who are incapable of transmitting the disease. Based on [10], the authors in [11] considered mathematical modeling to predict and control dengue transmission. Furthermore, the optimal control by [12] was performed to take precautions. Utilizing the optimal control theory to model the spread of disease is valuable for researchers and policymakers. This approach helps to explore evidence-based disease prevention and control strategies. The findings from this analysis can contribute to more impactful public health interventions and improved outcomes for populations affected by infectious diseases [13,14]. Optimal control within the host-vector dengue model is often used to assess the effectiveness of various intervention strategies [15,16].
Optimal control in dynamical systems is one of the mathematical theories that elaborate on determining the control policy to achieve certain optimality criteria [17]. The optimality procedures involve assigning manipulation system inputs or controls over time to achieve the intended behavior while minimizing or maximizing the objective function. Some notes that must be provided in the theory of optimal control in dynamic systems are control variables, objective functions, constraints, and optimality conditions. For continuous time problems, Pontryagin's Maximum Principle provides the necessary conditions for optimal solutions [18]. It involves Hamilton functions, adjoint variables, and control laws.
Different from the previous studies, in this study, we analyze the mathematical model of dengue virus transmission to control the infected class. Due to the high death rate of dengue fever, we modify the mathematical model in [11] by adding a death rate parameter. There is a possibility that someone will be reinfected with another serotype of the dengue virus; therefore, we enhance a parameter to represent this condition. Additionally, we develop the analysis of the model in [11] by discussing the global stability for both disease-free and endemic equilibrium points. The most commonly used methods for global stability analyses are the Lyapunov stability theorem and LaSalle's invariance principle (see, for example, [19,20]). However, it is often challenging to construct Lyapunov functions, since there is no general method available. Remarkable mathematical models can contribute to reducing both the infected host and the infected vector population. It is very promising to use host-vector models from a mathematical perspective to answer this.
There are five main findings from this work. First, we develop a new mathematical model that includes deaths due to the dengue virus and considers reinfection of the dengue virus to a person. Second, a global stability analysis is performed using the tricky Lyapunov function. Third, a sensitivity analysis is presented to understand the parameters that influence the spread of the virus. Fourth, the model is developed to provide control over the virus transmission. Fifth, through theoretical and numerical analyses, we can conclude that the presence of control makes the solution behavior move to a disease-free state more quickly.
In this study, we divide the population into the host and the vector. The total host (human) population is denoted by NH. The host population itself has three classes: susceptible, infected, and recovered at time t. Meanwhile, the vector population is divided into two classes: susceptible and infected at time t. We denote NV as the total vector (mosquito) population. The host population on the susceptible class at t is denoted by SH(t), the host population that is infected at time t is denoted by IV(t), and the host population that is recovered at time t is denoted by RH(t). Furthermore, we denote SV(t) to represent the susceptible vector population at time t, and IV(t) to denote the infected vector population at time t.
We use the notation μH to represent the birth rate of the host population. In this model, we assume a closed host population; thus, the birth rate is equal to the natural death rate. Due to the high death rate due to dengue fever, we modify the mathematical model in [11] by adding the death rate of the host population caused by the virus, which is denoted by μ. If the number of mosquito bites per day is denoted by b, then each day there will be bNV mosquito bites. Therefore, the host will receive bNVNHIVNV infected mosquito bites per day. If βH denotes the transmission rate from the mosquito to the host, then βHbNVNHIVNV denotes the infection rate of the susceptible hosts. We use α to represent the susceptible host, who can be susceptible again after recovering from dengue fever. Furthermore, we denote γH as the recovery rate.
The population of the vector has a growth rate as much as A, meanwhile μV denotes the mortality rate for the vector. Since a mosquito will bite bNH per day per person, then one mosquito will get the virus by bNHIH per day. If the transmission rate from the host to the mosquito is denoted by βV, then the infection rate per susceptible vector is βVbNHIH. Figure 1 portrays the mathematical modeling.
The mathematical model for dengue virus transmission is a complex system that takes various factors that contribute to the spread of the virus into account, which can be described as follows:
dSH(t)dt=μHNH−βHbNHSHIV−μHSH+αRH,SH(0)>0,dIH(t)dt=βHbNHSHIV−(μH+μ+γH)IH,IH(0)≥0,dRH(t)dt=γHIH−μHRH−αRH,RH(0)≥0,dSV(t)dt=A−βVbNHSVIH−μVSV,SV(0)>0,dIV(t)dt=βVbNHSVIH−μVIV,IV(0)≥0, | (2.1) |
where SH+IH+RH=NH and SV+IV=NV.
To ensure that the model solution reflects the real-world population, it is important to determine the non-negative solutions of System (2.1). Because the negative populations are not feasible, we prove the non-negativity of System (2.1) solutions by Theorem 2.1.
Theorem 2.1. Solutions of System (2.1) in the set of a vector of a non-negative real number R5+ are always non-negative for all time.
Proof. Let the solutions of System (2.1) rely on [0,τ), where 0<τ<∞. Based on the first equation of System (2.1), let SH(t)>0 for every t∈[0,τ). If it is not satisfied, then there exist t1∈(0,τ) such that SH(t1)≤0; therefore, dSH(t)dt|t=t1≤0, and we have SH(t)>0 for every t∈[0,t1). We do the same for the second equation of System (2.1). Let IH(t)≥0 for every t∈[0,t1). If it is not held, then there exist t2∈(0,t1) such that IH(t2)<0; therefore, dIH(t)dt|t=t2<0, and we have IH(t)≥0 for every t∈[0,t1).
Furthermore, for the third equation of System (2.1), we claim that RH(t)≥0 for every t∈[0,t2) if dRH(t)dt|t=t3<0. To prove this statement, we use reductio ad absurdum. Suppose that there exist t3∈[0,t2) such that RH(t3)<0. Then, we obtain the following:
dRH(t)dt|t=t3=γHIH(t3)−μHRH(t3)−αRH(t3)≥0, |
since RH(t3)<0, μH>0, and α>0. It is a contradiction with the fact that dRH(t)dt|t=t3<0. It implies that if dRH(t)dt|t=t3<0, then RH(t)≥0 for every t∈[0,t2).
In a similar way, we can observe that SV>0 and IV≥0. Now, we come to the first equation of System (2.1); if dSH(t)dt|t=t1<0, then SH(t)>0 for every t∈[0,τ). Suppose that there exist t1∈(0,τ) such that SH(t1)≤0,
dSH(t)dt|t=t1=μHNH(t1)−βHbNHSH(t1)IV(t1)−μHSH(t1)+αRH(t1)≥0. |
It is a contradiction to our sufficient condition that dSH(t)dt|t=t1<0; therefore, our supposition should be SH(t)>0 for every t∈[0,τ).
Using the same manner, we obtain that IH≥0. Hence, all of the solutions of System (2.1) are non-negative for all the time t on [0,τ), where 0<τ<∞.
The intuition of the boundedness of the solution can be explained as follows. We note that NH remains constant. Furthermore, since μV denotes the per capita death rate of the vector population, then μVNV is the total deaths of the vector. The rate of change of the vector population is represented by the following:
dNVdt=A−μVNV. |
As t approaches infinity, NV approaches AμV. Thus, SV+IV=AμV. Therefore, for NH and NV, which are constant, and for convenience, we introduce the following transformation:
sH=SHNH,iH=IHNH,rH=RHNH,sV=SVA/μV,iV=IVA/μV. | (2.2) |
Additionally, we use the relation rH=1−(sH+iH) and sV=1−iV. Using transformation (2.2), System (2.1) can be written as follows:
dsH(t)dt=μH−βHb(A/μV)NHsHiV−μHsH+α(1−(sH+iH)), | (2.3a) |
diH(t)dt=βHb(A/μV)NHsHiV−(μH+μ+γH)iH, | (2.3b) |
diVdt=βVbsViH−μViV=βVb(1−iV)iH−μViV. | (2.3c) |
In the next section, we examine the equilibrium points of System (2.3) and their stability. Generally, we obtain two types of equilibrium: disease-free and endemic. The local stability of the equilibrium can be studied through the value of the basic reproduction number. Meanwhile, La-Salle Lyapunov can be applied to analyze the global stability of the equilibrium point.
The equilibrium points of System (2.3) will be explained in the following discussion. For convenience, we use the relation (3.1) for our analysis. Let
M1=βVbμV(μH+α),M2=βHb(A/μV)NHβVbμV,ˆR0=βHb(A/μV)NH(μH+μ+γH)βVbμV. | (3.1) |
The equilibrium solutions are determined by setting each equation in System (2.3) equal to zero. Based on (2.3a), (2.3c), and (3.1), we obtain the following:
sH=(μH+α−αiH)((βVb/μV)iH+1)M2iH+(α+μH)((βVb/μV)iH+1), | (3.2a) |
iV=βVbiHβVbiH+μV. | (3.2b) |
By substituting Eq (3.2) into (2.3b), then we have that iH must satisfy the following quadratic equation:
−(αˆR0+M1+M2)i2H+(μH+α)(ˆR0−1)iH=0. | (3.3) |
Therefore, the disease-free equilibrium point can be summarized by the following lemma.
Lemma 3.1. If iH=0, then System (2.3) has a disease free equilibrium point, E0=(1,0,0).
Proof. One of the solutions of (3.3) is iH=0. By taking the right-hand side of (2.3c) to zero and substituting iH=0, we come to have iV=0. Moreover, since iH=0 and iV=0, then we obtain sH=1 by taking zero to the right-hand side of (2.3a).
Now, we will discuss the basic reproduction number that indicates the value of secondary infections resulting from the first infection. This value is determined using the next-generation matrix method [21]. In this study, matrix F1 is defined as the matrix whose entries consist of the first derivative of all terms that represent the rate of change of all factors contributing to the infectious class at the disease-free equilibrium E0. Therefore,
F1=[∂∂iH(βHb(A/μV)NHsHiV)∂∂iH(βVbiH)∂∂iV(βHb(A/μV)NHsHiV)∂∂iV(βVbiH)]at(1,0,0)=[0βVbβHb(A/μH)NH0]. | (3.4) |
We create the matrix F2 as a matrix of loss terms for each class, which is evaluated at the disease-free equilibrium as follows:
F2=[∂∂iH(μH+μ+γH)iH∂∂iH(μV+βVbiH)iV∂∂iV(μH+μ+γH)iH∂∂iV(μV+βVbiH)iV]at(1,0,0)=[(μH+μ+γH)00(μV)]. | (3.5) |
The inverse form of (3.5) is as follows:
F2−1=1(μH+μ+γH)μV[μV00μH+μ+γH]=[1μH+μ+γH001μV]. | (3.6) |
Based on Matrices (3.4) and (3.6), we obtain Matrix P, which is a product of F1 and F−12; then,
P=F1F2−1=[0βVbμVβHb(A/μV)NH(μH+μ+γH)0]. | (3.7) |
The largest eigenvalue of the matrix P is as follows:
√βVb(A/μV)NH(μH+μ+γH)βHbμV. |
If R0 is a notation for this value, then, in this study, R0 is referred to as the basic reproduction number of System (2.3). Furthermore, if
ˆR0=βVb(A/μV)NH(μH+μ+γH)βHbμV, | (3.8) |
then
R0=√ˆR0. | (3.9) |
This can be interpreted as the average number of secondary cases in the susceptible population caused by a single case. In a detailed biological interpretation, this can be seen as follows: an infected host that exists in the susceptible population is bitten during his/her infective period by b(A/μV)NH(μH+μ+γH) susceptible vectors. This results in a portion βVb(A/μV)NH(μH+μ+γH) of the vectors becoming infected. On the other hand, an infected vector administers bμV of bites to the susceptible hosts. These bites lead to a portion βHbμV of new infections to the host population.
The next section will describe the stability near the disease-free equilibrium point.
We describe the stability analysis of the disease-free equilibrium in Theorem 3.1.
Theorem 3.1. Given R0 in (3.9):
i. If ˆR0>1, then E0 is unstable.
ii. If ˆR0<1, then E0 is locally asymptotically stable.
Proof. The Jacobian matrix at E0 is as follows:
J|(1,0,0)=[−μH−α−α−βHbA/μVNH0−μH−μ−γHβHA/μVNH0βVb−μV]. | (3.10) |
If λ denotes the eigenvalue and I denotes the identity matrix, then the eigenvalues of the matrix (3.10) are determined by solving the characteristic equation |J(E0)−λI|=0. It implies that
(−μH−α−λ)[λ2+(μH+μ+γH+μV)λ+(μH+μ+γH)μV−(βHbA/μVNH)(βVb)]=0. |
Therefore, λ1=−(μH+α) and
λ2,3=−(μH+μ+γH+μV)2±√(μH+μ+γH+μV)2−4(μH+μ+γH)μV(1−ˆR0)2, | (3.11) |
where ˆR0 is written as in (3.8). Thus,
i. If ˆR0>1, then one of the eigenvalue in (3.11) is positive. Since we have a positive eigenvalue, then E0 is unstable.
ii. If ˆR0<1, then the eigenvalues in (3.11) are negative. Since all the eigenvalues are negative, then E0 is locally asymptotically stable.
The stability of the disease-free equilibrium point can be interpreted whenever ˆR0<1; then, there is no virus transmission in the population, and all humans are free of a dengue virus infection. Next, we analyze the global stability of the disease-free equilibrium points using La Salle Lyapunov [22]. In this study, we consider the Lyapunov function V:R3+,0→R3, where R3+,0={(sH,iH,iV)∈R3:sH>0,iH,iV≥0}, and
V=iH+βHb(A/μV)μVNHiV. | (3.12) |
It is easy to show that V and its partial derivatives are continuous in R3. Additionally, V is positive definite. The orbital derivative of function (3.12) is determined by the following:
˙V=∂V∂sHdsHdt+∂V∂iHdiHdt+∂V∂iVdiVdt=βHb(A/μV)NHsHiV−(μH+μ+γH)iH+βHb(A/μV)μVNH[βVb(1−iV)iH−μViV]=−βHb(A/μV)NH[1−sH]iV−(μH+μ+γH)[1−ˆR0(1−iV)]iH. |
Therefore, we achieve the global stability of the disease-free equilibrium point in Theorem 3.2.
Theorem 3.2. If ˆR0<1, then the disease-free equilibrium point is globally asymptotically stable.
Proof. If ˆR0<1, then we have ˙V<0. We also note that ˙V(E0)=0. Therefore, based on La Salle Lyapunov, the disease-free equilibrium E0 is globally asymptotically stable.
Recall the solution of Eq (3.3). We obtained iH=0, as the solution coincides with the disease-free equilibrium point; therefore, the other solution, iH≠0, corresponds to the endemic equilibrium point. Based on (3.3), we obtain the following:
iH=(μH+α)(ˆR0−1)M1+M2+αˆR0. | (3.13) |
The solution iH>0 in (3.13) exists if ˆR0>1. Consequently, the existence of the endemic equilibrium point can be concluded through the following Lemma 3.2.
Lemma 3.2. If ˆR0>1, then the endemic equilibrium point exists.
Proof. Note that ˆR0>1. The solution in (3.13) can be substituted into (3.2); then, we acquire the endemic equilibrium point, E1=(s∗H,i∗H,i∗V), where
s∗H=M1+α+μH+μ+γHM1+M2+αˆR0,i∗H=(μH+α)(ˆR0−1)M1+M2+αˆR0,i∗V=M1(ˆR0−1)(M1+α)ˆR0+M2. |
Furthermore, a stability analysis of the endemic equilibrium point can be explained by Theorem 3.3.
Theorem 3.3. If ˆR0>1, then the endemic equilibrium point E1 is locally asymptotically stable.
Proof. We consider that ˆR0>1. Based on this and (3.1), we derive the Jacobian matrix at E1 as follows:
J|(s∗H,i∗H,i∗V)=[a11a12a13a21a22a23a31a32a33], | (3.14) |
where
a11=−βHb(A/μV)NHM1(ˆR0−1)(M1+α)ˆR0+M2−(μH+α),a12=−α,a13=−βHb(A/μV)NHM1+α+μH+μ+γHM1+M2+αˆR0,a21=βHb(A/μV)NHM1(ˆR0−1)(M1+α)ˆR0+M2,a22=−(μH+μ+γH),a23=βHb(A/μV)NHM1+α+μH+μ+γHM1+M2+αˆR0,a31=0,a32=βVb(1−M1(ˆR0−1)(M1+α)ˆR0+M2),a33=−βVb(μH+α)(ˆR0−1)M1+M2+αˆR0−μV. |
The eigenvalues of matrix (3.14) are determined by solving the characteristic equation |J(E1)−λI|=0, where λ denotes the eigenvalues; we solve λ in (3.15) as follows:
[−βHb(A/μV)NHM1(ˆR0−1)(M1+α)ˆR0+M2−(μH+α)−λ]×[−(μH+μ+γH)−λ][−βVb(μH+α)(ˆR0−1)M1+M2+αˆR0−μV−λ]+[−βHb(A/μV)NHM1+α+μH+μ+γHM1+M2+αˆR0]×βHb(A/μV)NHM1(ˆR0−1)(M1+α)ˆR0+M2×βVb(1−M1(ˆR0−1)(M1+α)ˆR0+M2)−[−βHb(A/μV)NHM1(ˆR0−1)(M1+α)ˆR0+M2−(μH+α)−λ]×βHb(A/μV)NHM1+α+μH+μ+γHM1+M2+αˆR0×βVb(1−M1(ˆR0−1)(M1+α)ˆR0+M2)−βHb(A/μV)NHM1(ˆR0−1)(M1+α)ˆR0+M2×(βVb(μH+α)(ˆR0−1)M1+M2+αˆR0+μV+λ)α=0. | (3.15) |
Solving (3.15) means that we solve
λ3+Q1λ2+Q2λ+Q3=0, |
where
Q1=βHb(A/μV)NHM1(ˆR0−1)(M1+α)ˆR0+M2+βVb(μH+α)(ˆR0−1)M1+M2+αˆR0+μV+(μH+α)+(μH+μ+γH),Q2=(βHb(A/μV)NHM1(ˆR0−1)(M1+α)ˆR0+M2+(μH+α))×(μH+μ+γH)+(βHb(A/μV)NHM1(ˆR0−1)(M1+α)ˆR0+M2+(μH+α)(μH+μ+γH))×(βVb(μH+α)(ˆR0−1)M1+M2+αˆR0+μV)+βHb(A/μV)NHM1+α+μH+μ+γHM1+M2+αˆR0×βVb(M1(ˆR0−1)(M1+α)ˆR0+M2−1)+αβHb(A/μV)NHM1(ˆR0−1)(M1+α)ˆR0+M2,Q3=(βHb(A/μV)NHM1(ˆR0−1)(M1+α)ˆR0+M2+(μH+α))(μH+μ+γH)×(βVb(μH+α)(ˆR0−1)M1+M2+αˆR0+μV)+[βHb(A/μV)NHM1+α+μH+μ+γHM1+M2+αˆR0]βHb(A/μV)NH×M1(ˆR0−1)(M1+α)ˆR0+M2βVb(M1(ˆR0−1)(M1+α)ˆR0+M2−1)+(βHb(A/μV)NHM1(ˆR0−1)(M1+α)ˆR0+M2+(μH+α))×βHb(A/μV)NHM1+α+μH+μ+γHM1+M2+αˆR0×βVb(M1(ˆR0−1)(M1+α)ˆR0+M2−1)+αβHb(A/μV)NHM1(ˆR0−1)(M1+α)ˆR0+M2×(βVb(μH+α)(ˆR0−1)M1+M2+αˆR0+μV). |
In this case, if ˆR0>1, then it implies Q1,Q2,Q3>0. By manipulating the algebra, we also obtain that Q1,Q2>Q3. Based on the Routh Hurwitz criterion, the endemic equilibrium point E1 is locally asymptotically stable.
The discussion in this article continues regarding the global stability around the endemic equilibrium point, particularly when α=0 represents a population with permanent immunity. The dynamic model for the spread of dengue fever is as follows:
dsH(t)dt=μH−βHb(A/μV)NHsHiV−μHsH,diH(t)dt=βHb(A/μV)NHsHiV−(μH+μ+γH)iH,diVdt=βVbsViH−μViV=βVb(1−iV)iH−μViV. | (3.16) |
The endemic equilibrium (s∗∗H,i∗∗H,i∗∗V) satisfies the following:
μH=βHb(A/μV)NHs∗∗Hi∗∗V+μHs∗∗H,(μH+μ+γH)i∗∗H=βHb(A/μV)NHs∗∗Hi∗∗V,μVi∗∗V=βVb(1−i∗∗V)i∗∗H. | (3.17) |
Let the function
W=sH−s∗∗H−s∗∗Hln(sHs∗∗H)+iH−i∗∗H−i∗∗Hln(iHi∗∗H)+iV−i∗∗V−i∗∗Vln(iVi∗∗V), | (3.18) |
and note that g(x)=x−1−lnx≥g(1)=0 for x>0. We introduce y1=sHs∗∗H,y2=iHi∗∗H, and y3=iVi∗∗V. The derivative of W along the solution (3.16), and using (3.17), we obtain the following:
∂W∂sHdsHdt=(1−s∗∗HsH)(βHb(A/μV)NHs∗∗Hi∗∗V+μHs∗∗H−βHb(A/μV)NHsHiV−μHsH)=−βHb(A/μV)NHs∗∗Hi∗∗V(y1y3−1)+βHb(A/μV)NHs∗∗Hi∗∗V(y3−1y1)−μHs∗∗H(y1−1), | (3.19) |
∂W∂iHdiHdt=(1−i∗∗HiH)(βHb(A/μV)NHsHiV−βHb(A/μV)NHs∗∗Hi∗∗Vi∗∗HiH)=βHb(A/μV)NHs∗∗Hi∗∗V(y1y3−y1y3y2), | (3.20) |
∂W∂iVdiVdt=(1−i∗∗ViV)(βVb(1−iV)iH−βVb(1−i∗∗V)i∗∗Hi∗∗ViV)=−βVbi∗∗Vi∗∗H(y2y3+1−y2−y3)−βVby3(1+1y23). | (3.21) |
If (3.19)–(3.21) are substituted into (3.18), then we have the following:
˙W=∂W∂sHdsHdt+∂W∂iHdiHdt+∂W∂iVdiVdt=−βHb(A/μV)NHs∗∗Hi∗∗V(y1y3y2−y3+1y1−1)−μHs∗∗H(y1−1)−βVbi∗∗Vi∗∗H(y2y3+1−y2−y3)−βVby3(1+1y23)≤−βHb(A/μV)NHs∗∗Hi∗∗V(y1y3y2−y3+1y1−1)−βVbi∗∗Vi∗∗H(y2y3+1−y2−y3)−βVby3(1+1y23)≤−βHb(A/μV)NHs∗∗Hi∗∗V(g(y1y3y2)+g(1y1))−βVbi∗∗Vi∗∗Hg(y2y3)−βVby3(1+1y23), | (3.22) |
where we use the inequality 1−x≤−lnx and g(x)≥0. Therefore, we come to the global stability of the endemic equilibrium point in Theorem 3.4.
Theorem 3.4. If ˆR0>1, then the endemic equilibrium point is globally asymptotically stable.
Proof. Based on Lemma 3.2, the endemic equilibrium exists if ˆR0>1. Moreover, based on (3.22), we have ˙W≤0. Furthermore, ˙W=0 is satisfied at (s∗∗H,i∗∗H,i∗∗V). The claim follows by LaSalle's Invariance Principle [22].
A sensitivity analysis explains the impact of changes in the parameter values on the system behavior. We study the sensitivity of the basic reproduction number to determine the robustness of the model to the parameter values measured by the sensitivity index. Next, the sensitivity index is defined as follows.
Definition 4.1. [23,24] The sensitivity index of variable y is the relative change ratio of y to the relative change of parameter s, and is defined as follows:
Υys=∂y∂s×sy. | (4.1) |
We analyze the sensitivity index of ˆR0 using the formula (4.1), especially for the parameters βV, βH, b, μ, and α that influence R0. The sensitivity index of ˆR0 can either depend on the values of other parameters or be a constant that does not depend on the parameter values. Based on Definition 4.1 and Eq (3.9), we obtain the following:
ΥˆR0βV=∂ˆR0∂βV×βVˆR0=1,ΥˆR0βH=∂ˆR0∂βH×βHˆR0=1,ΥˆR0b=∂ˆR0∂b×bˆR0=2,ΥˆR0μ=∂ˆR0∂μ×μˆR0=−μ(μH+μ+γH),ΥˆR0α=∂ˆR0∂α×αˆR0=0. |
Based on the signs of the sensitivity index, the value of ˆR0 increases by βV, βH, and b. On the contrary, it decreases by μ. Moreover, α does not impact the value of ˆR0. We note that the most sensitive parameter corresponds to the largest sensitivity index. In this case, b is the most sensitive parameter; an increase of b by 10% increases ˆR0 by 10%. Recall that in this case, b represents the number of mosquito bites per day. Based on the sensitivity index, the value of ˆR0 decreases if the value of b decreases. Biologically, the value of b can be a target to reduce as the intervention of dengue transmission. We can reduce the value of b by reducing the presence of mosquitoes. In addition, increasing the value of μ decreases ˆR0. Changing the value of an individual who can be re-infected cannot alter the value of ˆR0.
This section will delve deeper into the dengue virus transmission and discuss how it can be controlled using the prevention control variable. We denote u1, where 0≤u1≤1, as a control variable to reduce the contact between the host and the vector. We have derived the following model:
dSH(t)dt=μHNH−(1−u1)βHbSHIVNH−μHSH+αRH,dIH(t)dt=(1−u1)βHbSHIVNH−μHIH−μIH−γHIH,dRH(t)dt=γHIH−μHRH−αRH,dSV(t)dt=A−(1−u1)βVbSVIHNH−μVSV,dIV(t)dt=(1−u1)βVbSVIHNH−μVIV. | (5.1) |
To investigate the optimality of these efforts, we utilize the objective function G, which minimizes the infected host class and the cost of implementing the u1 control. In this study, if B is the weight for the infected hosts class, and C is the weight for prevention efforts, then the solution of (5.1) minimizes the number of infected hosts with a minimal control cost u1(t) such that
G(u1)=T∫0(BIH(t)+Cu21(t))dt. | (5.2) |
To further elaborate, the Hamiltonian of this study is as follows:
H=BIH(t)+Cu21(t)+δ1(μHNH−(1−u1)βHbSHIVNH−μHSH+αRH)+δ2((1−u1)βHbSHIVNH−μHIH−μIH−γHIH)+δ3(γHIH−μHRH−αRH)+δ4(A−(1−u1)βVbSVIHNH−μVSV)+δ5((1−u1)βVbSVIHNH−μVIV), |
where δ1,δ2,...,δ5 are costate variables. We use the Maximum Pontryagin principle to determine the existence of an optimal control [17,18,24,25]. The following theorem provides an idea.
Theorem 5.1. If u∗1 is the optimal control that minimizes G(u1) with System (5.1) as the constraint, then there exist costate variables δ1,δ2,...,δ5 such that
dδ1dt=δ1((1−u1)βHbIVNH+μH)−δ2(1−u1)βHbIVNH,dδ2dt=−B+δ2(μH+μ+γH)−δ3γH+δ4(1−u1)βVbSVNH−δ5(1−u1)βVbSVNH,dδ3dt=−αδ1+δ3(μH+α),dδ4dt=δ4(1−u1)βVbIHNH−δ5(1−u1)βVbIHNH,dδ5dt=δ1(1−u1)βHbSHNH−δ2(1−u1)βHbSHNH+δ5μV, |
with transversality conditions δi(T)=0,i=1,...,5, and the u∗1 satisfies the optimality condition
u∗1=min{max(0,(δ2−δ1)βHbS∗HI∗VNH+(δ5−δ4)βVbS∗VI∗HNH2C),1}. |
Proof. The costate equations are obtained by differentiating the Hamiltonian function, evaluated at the optimal control, as follows:
dδ1dt=−∂H∂SH=δ1((1−u1)βHbIVNH+μH)−δ2(1−u1)βHbIVNH,dδ2dt=−∂H∂IH=−B+δ2(μH+μ+γH)−δ3γH+δ4(1−u1)βVbSVNH−δ5(1−u1)βVbSVNH,dδ3dt=−∂H∂RH=−αδ1+δ3(μH+α),dδ4dt=−∂H∂SV=δ4(1−u1)βVbIHNH−δ5(1−u1)βVbIHNH,dδ5dt=−∂H∂IV=δ1(1−u1)βHbSHNH−δ2(1−u1)βHbSHNH+δ5μV. |
The optimal control at the solutions S∗H,I∗H,R∗H,S∗V,I∗V at System (5.1) is obtained by the following:
∂H∂u1=0⇔2Cu∗1+δ1βHbS∗HI∗VNH−δ2βHbS∗HI∗VNH+δ4βVbS∗VI∗HNH−δ5βVbS∗VI∗HNH=0⇔u∗1=(δ2−δ1)βHbS∗HI∗VNH+(δ5−δ4)βVbS∗VI∗HNH2C, |
and
u∗1=min{max(0,(δ2−δ1)βHbS∗HI∗VNH+(δ5−δ4)βVbS∗VI∗HNH2C),1}. |
To illustrate the analytical results, in this simulation, we select parameter values based on prior research, see [9,11] and the references cited therein. We use the following parameters: α=0,9619; μV=0,071428; b=0,5;βV=1;βH=0,75; μH=0,391389;μ=0,8; and γH=0,1428. Using these parameter values, using A=400, and NH=1000, we obtain ˆR0≈22,036>1. On the other hand, if we use A=40;NH=10000, then we have ˆR0≈0,22<1.
Figure 2 shows the illustration of Theorems 3.1 and 3.3. Using different initial values in Figure 3 (i.e., (0, 45;0, 22;0, 3), (0, 5;0, 25;0,165), and (0, 4;0, 24;0, 2) shows us that the solutions tend to the disease-free equilibrium point. On the other hand, we aim to reduce the number of infected humans and infected vectors in the population. Therefore, the endemicity should be controllable. Figure 4 showcases a dynamic tornado plot which illustrates the parameter sensitivity index. We calculate the sensitivity index using the given values and obtain the following values: βV=βH=1, b=2, μ=−0,59962, and α=0. In this case, the number of mosquito bites per day is the most crucial parameter to reduce the spread of the dengue virus.
Introducing control enables the solution to reach the disease-free equilibrium point more rapidly. Figures 5–7 illustrate the dynamics of the system solutions, both without control and with control. The proportion of susceptible humans in the controlled system is higher due to lower transmission rates from control measures. Conversely, the proportion of infected humans in the controlled system is lower throughout the simulation period, highlighting the success of the implemented control strategies in restricting disease transmission. Moreover, a significant decrease in infected vectors indicates that the intervention suppresses the spread within the vector population.
In Figure 8, we present the profiles of infected humans and mosquitoes using several control values. It can be explained that for a system without control, the virus transmission rate is high enough to cause dengue fever to spread rapidly, so that the number of infected humans increases in a short time. This condition is a cycle; the high number of infected humans also causes the high number of infected mosquitoes, and vice versa.
The impact of the control measures aims to reduce the infected host population (i.e., minimize G(u1)). These measures include providing vaccinations, community education regarding the importance of cleanliness to prevent the proliferation of mosquitoes, and promoting awareness of dengue fever symptoms. Therefore, we present the numerical solution of the optimal control u∗1 in Figure 9 to understand the control behavior. It shows that the control measured significantly reduced the infected hosts at day 30.
Meanwhile, in a system with control, strategic intervention in the prevention efforts is optimally implemented to control the spread of disease and to reduce costs. This treatment makes the behavior of the system solution in infected humans and infected mosquitoes decrease more quickly toward a disease-free condition.
This paper presents a detailed mathematical model of dengue virus spread in the form of a host-vector that considers the role of humans as hosts and mosquitoes as vectors. The model analysis is generally divided into two, namely the model analysis without control and the model with vector control. Based on the results of the model analysis without control, the population will be free from dengue virus infection if the basic reproduction number is less than one. Mathematically, this is proven by La-Salle Lyapunov in Theorem 3.2. On the contrary, the endemicity holds if the basic reproduction number is more than one, see Theorem 3.4.
The model is further enriched by providing control strategies through the effective prevention of the dengue virus spread. Mathematically, we use the Pontryagin Maximum Principle in Theorem 5.1, which is a powerful optimization tool in the field of control theory. Through a numerical analysis, we showed that the control strategy can significantly reduce the population of infectious classes for both the hosts and the vectors. This is a significant contribution to developing effective strategies to control the spread of dengue fever, which is a major public health problem in many parts of the world.
Our research focused on the potential for a person to be re-infected without considering the different susceptible classes. In fact, a person can be re-infected by various types of dengue viruses. It is important to remember that there are four types of viruses, and being infected with one kind of virus does not confer immunity to the other. Therefore, it can be considered that susceptible host populations to have different dengue virus infections. In terms of memory effects, utilizing a fractional model can significantly advance the research in understanding the behavior of dengue spread [7,8,15]. In future research, we can further develop the mathematical model for dengue by including compartments such as Exposed [26], Quarantined [27], and Treated [28]. Control measures, such as vaccination strategies [26], and Wolbachia bacterium [29] are considerable to future studies.
The authors declare they have not used Artificial Intelligence (AI) tools in the creation of this article.
The first author would like to thank Universitas Negeri Yogyakarta for the financial support. We also thank the Mathematical Biology team at Universitas Negeri Yogyakarta for the discussion.
Authors declare that they have no conflicts of interest.
Four-quadrant model of educational environments