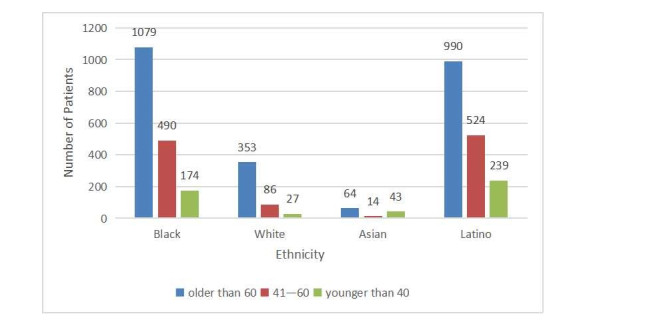
As COVID-19 continues to spread across the world and causes hundreds of millions of infections and millions of deaths, medical institutions around the world keep facing a crisis of medical runs and shortages of medical resources. In order to study how to effectively predict whether there are risks of death in patients, a variety of machine learning models have been used to learn and predict the clinical demographics and physiological indicators of COVID-19 patients in the United States of America. The results show that the random forest model has the best performance in predicting the risk of death in hospitalized patients with COVID-19, as the COVID-19 patients' mean arterial pressures, ages, C-reactive protein tests' values, values of blood urea nitrogen and their clinical troponin values are the most important implications for their risk of death. Healthcare organizations can use the random forest model to predict the risks of death based on data from patients admitted to a hospital due to COVID-19, or to stratify patients admitted to a hospital due to COVID-19 based on the five key factors this can optimize the diagnosis and treatment process by appropriately arranging ventilators, the intensive care unit and doctors, thus promoting the efficient use of limited medical resources during the COVID-19 pandemic. Healthcare organizations can also establish databases of patient physiological indicators and use similar strategies to deal with other pandemics that may occur in the future, as well as save more lives threatened by infectious diseases. Governments and people also need to take action to prevent possible future pandemics.
Citation: Qian Shen. Research of mortality risk prediction based on hospital admission data for COVID-19 patients[J]. Mathematical Biosciences and Engineering, 2023, 20(3): 5333-5351. doi: 10.3934/mbe.2023247
[1] | Vitaliy Yakovyna, Natalya Shakhovska . Modelling and predicting the spread of COVID-19 cases depending on restriction policy based on mined recommendation rules. Mathematical Biosciences and Engineering, 2021, 18(3): 2789-2812. doi: 10.3934/mbe.2021142 |
[2] | Natalya Shakhovska, Vitaliy Yakovyna, Valentyna Chopyak . A new hybrid ensemble machine-learning model for severity risk assessment and post-COVID prediction system. Mathematical Biosciences and Engineering, 2022, 19(6): 6102-6123. doi: 10.3934/mbe.2022285 |
[3] | Manfu Ma, Penghui Sun, Yong Li, Weilong Huo . Predicting the risk of mortality in ICU patients based on dynamic graph attention network of patient similarity. Mathematical Biosciences and Engineering, 2023, 20(8): 15326-15344. doi: 10.3934/mbe.2023685 |
[4] | Suzan Farhang-Sardroodi, Mohammad Sajjad Ghaemi, Morgan Craig, Hsu Kiang Ooi, Jane M Heffernan . A machine learning approach to differentiate between COVID-19 and influenza infection using synthetic infection and immune response data. Mathematical Biosciences and Engineering, 2022, 19(6): 5813-5831. doi: 10.3934/mbe.2022272 |
[5] | Lili Jiang, Sirong Chen, Yuanhui Wu, Da Zhou, Lihua Duan . Prediction of coronary heart disease in gout patients using machine learning models. Mathematical Biosciences and Engineering, 2023, 20(3): 4574-4591. doi: 10.3934/mbe.2023212 |
[6] | Hyeonjeong Ahn, Hyojung Lee . Predicting the transmission trends of COVID-19: an interpretable machine learning approach based on daily, death, and imported cases. Mathematical Biosciences and Engineering, 2024, 21(5): 6150-6166. doi: 10.3934/mbe.2024270 |
[7] | Zhihao Zhang, Ting Zeng, Yijia Wang, Yinxia Su, Xianghua Tian, Guoxiang Ma, Zemin Luan, Fengjun Li . Prediction Model of hospitalization time of COVID-19 patients based on Gradient Boosted Regression Trees. Mathematical Biosciences and Engineering, 2023, 20(6): 10444-10458. doi: 10.3934/mbe.2023459 |
[8] | Saleh I. Alzahrani, Wael M. S. Yafooz, Ibrahim A. Aljamaan, Ali Alwaleedi, Mohammed Al-Hariri, Gameel Saleh . AI-driven health analysis for emerging respiratory diseases: A case study of Yemen patients using COVID-19 data. Mathematical Biosciences and Engineering, 2025, 22(3): 554-584. doi: 10.3934/mbe.2025021 |
[9] | H. Swapnarekha, Janmenjoy Nayak, H. S. Behera, Pandit Byomakesha Dash, Danilo Pelusi . An optimistic firefly algorithm-based deep learning approach for sentiment analysis of COVID-19 tweets. Mathematical Biosciences and Engineering, 2023, 20(2): 2382-2407. doi: 10.3934/mbe.2023112 |
[10] | Peng An, Xiumei Li, Ping Qin, YingJian Ye, Junyan Zhang, Hongyan Guo, Peng Duan, Zhibing He, Ping Song, Mingqun Li, Jinsong Wang, Yan Hu, Guoyan Feng, Yong Lin . Predicting model of mild and severe types of COVID-19 patients using Thymus CT radiomics model: A preliminary study. Mathematical Biosciences and Engineering, 2023, 20(4): 6612-6629. doi: 10.3934/mbe.2023284 |
As COVID-19 continues to spread across the world and causes hundreds of millions of infections and millions of deaths, medical institutions around the world keep facing a crisis of medical runs and shortages of medical resources. In order to study how to effectively predict whether there are risks of death in patients, a variety of machine learning models have been used to learn and predict the clinical demographics and physiological indicators of COVID-19 patients in the United States of America. The results show that the random forest model has the best performance in predicting the risk of death in hospitalized patients with COVID-19, as the COVID-19 patients' mean arterial pressures, ages, C-reactive protein tests' values, values of blood urea nitrogen and their clinical troponin values are the most important implications for their risk of death. Healthcare organizations can use the random forest model to predict the risks of death based on data from patients admitted to a hospital due to COVID-19, or to stratify patients admitted to a hospital due to COVID-19 based on the five key factors this can optimize the diagnosis and treatment process by appropriately arranging ventilators, the intensive care unit and doctors, thus promoting the efficient use of limited medical resources during the COVID-19 pandemic. Healthcare organizations can also establish databases of patient physiological indicators and use similar strategies to deal with other pandemics that may occur in the future, as well as save more lives threatened by infectious diseases. Governments and people also need to take action to prevent possible future pandemics.
Since the beginning of 2020, the pandemic of COVID-19 has broken out in countries around the world. So far, more than 600 million people have been infected with COVID-19 worldwide, of which more than 6.5 million have died. The dramatic increase in the number of new infections has put healthcare facilities around the world under enormous pressure as variants of the COVID-19 virus continue to become more contagious. The study by Gabutti et al. [1] pointed out that the difficulties caused by repeatedly occuring conditions comparable to the early months of the COVID-19 pandemic have put enormous pressure on health systems in almost all European countries. Mahendradhata et al. [2] assessed the performance of the Indonesian healthcare system during the COVID-19 pandemic. They pointed out that due to the large-scale infections, the country has experienced medical supply shortages and severe difficulties in medical waste disposal throughout the pandemic, while at the same time, medical workers also faced serious mental health risks because of the pressure from their work. With the continuous emergence of new variants of COVID-19 viruses, such as delta, and the progress of COVID-19 around the world, the mortality rate of patients with COVID-19 has shown a declining trend; the relevant investigation report of the USA's Centers for Disease Control and Prevention confirmed the authenticity of this situation[3]. But, the latest Omicron variant has caused a more severe death rate for COVID-19 patients with greater infectivity.
Notari and Torrieri [4] discussed risk factors for the spread of COVID-19 and the extent to which these factors are important through the use of statistical analysis. Coccia [5] studied the effects of vaccination against COVID-19. The results of the survey show that governments can reduce confirmed cases and deaths from COVID-19 by administering an average of about 80 doses of COVID-19 vaccine per 100 inhabitants. But due to the resurgence of the COVID-19 pandemic since May 2021, this number has risen to 90. Benati and Coccia [6] have analyzed the relationship between public governance and COVID-19 vaccination in 112 countries in early 2021 to assess their readiness to respond to the pandemic crisis in a timely manner. The findings suggest that the COVID-19 vaccine doses per 100 residents are highly positively correlated with the overall governance index, and that an increase in the overall governance index improves the expected vaccination of COVID-19 vaccine doses. But other research works show that despite higher vaccination rates in all countries during the outbreak of COVID-19 Omicron variant, the total number of daily hospitalizations during this period is still higher than the number of patients hospitalized during the delta variant wave[7].
Due to the simultaneous increase in the number of infections and deaths from COVID-19 caused by the Omicron variant, there has been a new round of more serious medical runs. Hung et al. [8] believed that the Omicron variant became the dominant COVID-19 strain, resulting in a surge in hospitalizations and shortages of medical staff in every state in the United States of America, with major implications for the USA healthcare system. Based on this background, it can be considered that, for medical institutions around the world, an effective way to triage COVID-19 patients and allocate Intensive Care Unit (ICU) beds and intensive care equipment to patients with a higher risk of death to save more lives by using the tense first aid resources more effectively have became urgent and important issues in the current clinical treatment of COVID-19 patients.
The symptoms of patients infected with the new strain of COVID-19 are usually mild and limited, but at the same time, it is undeniable that COVID-19 is still very serious and even fatal to a considerable number of COVID-19 patients. Therefore, determining which of them are more likely to become seriously ill or even die is important for medical institutions that treat patients with COVID-19 to develop emergency triage strategies. This study aims to fit statistical models and machine learning models based on the basic health conditions and vital signs data of COVID-19 patients contained in historical data, hoping to effectively and accurately predict the death risk of COVID-19 patients who have just been admitted to the hospital and ultimately alleviate the impact of the COVID-19 pandemic on medical resources to a certain extent.
Research on classification models is one of the main research areas of machine learning. From logistic regression models to various new neural network models, their accuracy on various classification tasks continues to increase. Shipe et al. [9] demonstrated through research that the development of a logistic regression-based binary outcome prediction model can help healthcare professionals and patients by providing patient risk stratification analysis to support tailored clinical decisions and improve patient outcomes and quality of care. Christodoulou et al. [10] reviewed various predictive models in clinical research. They have pointed out that typical machine learning models such as classification trees, random forest, artificial neural networks and support vector machines (SVMs) have no performance advantage over logistic regression for clinical prediction models.
Shaban et al. [11] have proposed an improved K-nearest neighbor (K-NN) model i.e., an enhanced K-nearest neighbor (EKNN) model, and they used the EKNN to construct a COVID-19 patient detection strategy. The results showed that this strategy provided faster and more accurate results than other techniques at the time. Singh et al. [12] have used an SVM to generate real-time predictions of COVID-19 and explored the impact on COVID-19 identification, death, and recovery.
The random forest was officially published by Breiman [13] in the journal "Machine Learning" in 2002; this model has been the classic representative of bagging algorithms in ensemble learning for nearly two decades. A random forest integrates multiple trees for model fitting in the form of a voting decision, which effectively improves the prediction performance. Khalilia et al. [14] compared the accuracy of models such as random forests and SVMs in predicting the risk of eight diseases. It was found that the area under the receiver operating characteristic (ROC) curve of the random forest was larger than that of SVM, simple bagging algorithm and boosting algorithm. In addition, a random forest has the advantage of calculating the importance of each variable in the classification process.
Ezzoddin et al. [15] used LightGBM to predict the characteristics of the extracted chest X-ray images of COVID-19 patients. LightGBM reached 99.20 and 94.22% accuracies on the two-class (i.e., COVID-19 and No-findings) and multi-class (i.e., COVID-19, Pneumonia and No-findings) classification problems, respectively. XGBoost was proposed by Chen and Guestrin [16] in 2015. It is considered to be one of the best open-source ensemble learning models, and it is widely used in data science competitions and industry. XGBoost improves the gradient boosting decision tree (GBDT) from the regularization of the loss function and the Taylor expansion of the error part, and it optimizes the operating efficiency of each weak learner. The research of Li and Zhang [17] confirmed that the classification prediction model of the XGBoost algorithm has higher accuracy, a faster calculation speed, and high prediction accuracy when applied to orthopedic clinical data. It can deal with complex and diverse medical data, better meet the timeliness and accuracy requirements of auxiliary diagnosis, help reduce the workload of medical workers and realize real auxiliary medical services.
Since the beginning of the COVID-19 pandemic, many researchers have studied how to predict whether patients with COVID-19 are at a higher risk of death based on clinical data collected at various stages of the pandemic. Covino et al. [18] collected the clinical physiological index data of adult patients admitted to the hospital due to COVID-19 from March 1 to April 15, 2020, and they used a variety of physiological scoring systems that can quickly and quantitatively evaluate the changes of vital signs to predict the risk of admission to the ICU and death of COVID-19 patients within 48 hours and 7 days after admission. The results suggested that the early warning score can effectively predict the risk of severe illness and death in COVID-19 patients in the context of a huge demand for medical assessment and triage in emergency departments. Allenbach et al. [19] investigated how logistic regression can be used to predict changes in symptoms within 14 days of hospital admission in COVID-19 patients. Their results suggested that older age, poorer respiratory performance, higher C-reactive protein (CRP) levels, and lower lymphocyte counts in COVID-19 patients are associated with an increased risk of ICU admission or death. Mirri et al. [20] have proposed a new indicator based on a hypothesis of a relationship between viral transmission and airborne particulate pollutants such as PM2.5, PM10 and NO2. And, they have developed a machine learning model that learns data containing new metrics to predict the spread of COVID-19.
Estiri et al.[21] developed an end-to-end machine learning framework for prediction based on data from COVID-19 patients' past medical records, implementing iterative sequential representation mining, features and model selection for the prediction of patient-level hospitalizations, ICU admissions, mechanical ventilation needs and death risk. They believed that a history of previous neurological disease, cardiovascular disease (CVD), other chronic diseases, diabetes and chronic kidney disease plays a major role in whether patients with COVID-19 are at risk of death. And features with the greatest weight in predicting whether a COVID-19 patient will be admitted to the ICU was healthcare utilization events, including previous complex hospitalization episodes, hospitalization procedures and hospitalization medications. Yadaw et al. [22] collected data on 3841 patients treated at Mount Sinai Health System from March 9 to April 6, 2020, and they developed a COVID-19 mortality prediction model based on patient age, minimum oxygen saturation over the course of their medical encounter, and type of patient encounter (inpatient vs outpatient and telehealth visits). They achieved a high degree of accuracy with this model and believe that the model may help to guide the management and prognosis of patients affected by the disease in a clinical setting. Altschul et al. [23] have proposed a novel severity score specifically for COVID-19 to help predict disease severity and mortality. They believed this developed and validated novel COVID-19 severity score will aid physicians in predicting mortality during surge periods. Wang et al. [24] developed a predictive model incorporating patient data such as age, SpO2, body temperature and mean arterial pressure (MAP) based on data from the Altschul et al. [23] study. This model had good accuracy and may help with the early identification of COVID-19 patients with a high probability of death on admission.
Zhang et al. [25] proposed a new reliability estimation model based on the marshall-holguin binary Weibull distribution, which is called the multicomponent stress-strength model. The authors evaluated their proposed model through the use of simulation datasets and Monte Carlo simulations. The simulation results showed that this method performs well in terms of relative deviation, mean squared error and frequency coverage probability. Wang et al. [26] proposed a Bayesian semiparametric method to obtain the estimation of parameters and density distribution for both the cure probability and the survival distribution of the uncured patients in an accelerated failure time mixture cure; model; its performance was comparable to that of the fully parametric method according to the results of comprehensive simulation studies. The proposed approach was also adopted for the analysis of colorectal cancer clinical trial data. Zhuang et al. [27] presented a novel progressive-stress accelerated life testing model with group effects under progressive censoring. The proposed model was compared the traditional models without group effects in simulation studies; the results showed that the proposed model can detect group-to-group variation and reduce biases. An elaborate investigation of COVID-19 data for Weibull distribution under indeterminacy using time truncated repetitive sampling plan [28]. The results showed that the proposed repetitive sampling plan is more economical than the existing sampling plan.
Caillon et al. [29] studied the data of COVID-19 patients in Wuhan Seventh Hospital, and they believed that the high systolic blood pressure of patients was the cause and important comorbidity factor of end-organ damage; it was identified as covariate in both the mortality and survival prediction models. Blagosklonny [30] proposed that COVID-19 is not fatal in early life, but the mortality rate increases exponentially with age. It was the strongest predictor of mortality in elderly patients. Wang et al. [31] have collected and analyzed clinical and laboratory data on admission for non-severe adult COVID-19 patients in Changsha, China. The analysis results have proved that CRP may be a valuable marker for predicting the likelihood of exacerbation in non-severe adult COVID-19 patients. Ok et al. [32] have investigated 139 patients with COVID-19 at Siirt State Hospital. They believed that the blood urea nitrogen / creatinine ratio is an independent predictor of COVID-19 patient severity and survival. Routine evaluation of the ratio can help to identify high-risk cases with COVID-19. Lippi et al. [33] conducted a meta-analysis of clinical data from COVID-19 patients in Wuhan on March 4, 2020, and they found that clinical troponin levels in patients with severe COVID-19 infection increased significantly compared to COVID-19 patients with milder symptoms. Therefore, they believed that initial measurements of heart injury biomarkers immediately after a COVID-19 patient's hospitalization, as well as longitudinal monitoring during hospitalization, may help to identify subsets of patients who may have heart injury and thus predict the progress of COVID-19 patients toward a worse clinical situation.
The data come from the study of Altschul et al.[23], they have shared their data on https://figshare.com/s/79827c396af7df42b3d7.
Data were collected from 4711 patients who visited hospitals from March 1 to April 16 and were tested positive for SARS-CoV-2 RNA through the use of a real-time reverse transcriptase-polymerase chain reaction assay. Patients evaluated in the emergency room but not admitted, or those that died in the emergency room, were excluded from the analysis. Most patients had only one admission, and the research only considered the last hospitalization for those that had multiple admissions during this period [23].
Some of the variables included in the dataset are: length of hospital stay (LOS), myocardial infraction, peripheral vascular disease, congestive heart failure, CVD, dementia, chronic obstructive pulmonary disease, diabetes mellitus simple, diabetes mellitus complicated, oxygen saturation (OsSats), MAP, in mmHg (MAP), D-dimer, in mg/ml (Ddimer), platelets, in k per mm3 (Plts), international normalized ratio (INR), blood urea nitrogen, in mg/dL (BUN), alanine aminotransferase, in U/L (AST), white blood cells, in per mm3 (WBC) and interleukin-6, in pg/ml (IL-6).
A brief analysis of the basic demographic information of the selected patients is required first. Figure 1 shows histograms of the patients' ethnic groups and age data. The patients were mainly African and Latino Americans, with a relatively small number of white and Asian patients. The vast majority of patients were over 60 years of age. The number of patients in the 41 to 60 age group was only about half the number of patients over 60 years old, and a very small number of patients were under the age of 40. Figure 2 shows that the vast majority of patients stayed in the hospital for less than 10 days, a small number of patients stayed in the hospital between 10 and 20 days, very few patients stayed in the hospital for more than 20 days and the longest patient stay in hospital was for about 50 days. In addition, 1148 of the 4711 patients included in the data eventually died, and 3563 patients survived.
Consider that there are some numerical variables and categorical variables of patients' physiological indicators in the data, such as troponin values and categorical variables such as troponin > 0.1. In order to avoid the correlation between variables and make the model adapt to the original physiological index data, only the numerical variables of these tests were selected to construct the classification model when building the model.
Because the proportions of different ethnic groups in the data were very different, the variables related to the patient ethnic groups were not included in the final models. Considering that there were some missing values in the data, and that all missing values in the original data were 0, all data points with a value of 0 in continuous variables were considered as missing values. In order to ensure data quality, variables with more than 1000 missing data points, such as ddimer, glucose, IL6, ferritin and procalcitonin, were included in the final model. Scikit-learn's Iterative Imputer, K-NN Imputer and the means imputation method were used to deal with the missing values of data. Then, the standard deviations of all variables were calculated; the results are showed in Table 1.
Variable | Iterative imputer | K-NN imputer | Mean imputer | Original data |
LOS | 6.7489 | 6.7912 | 6.7384 | 6.9964 |
OsSats | 8.1218 | 8.0724 | 8.0423 | 8.1888 |
Temp | 4.1045 | 4.3043 | 4.1030 | 4.1713 |
MAP | 16.5238 | 16.4947 | 16.4064 | 16.8071 |
Ddimer | 5.1079 | 5.0681 | 4.9588 | 5.6648 |
Plts | 106.0403 | 106.0664 | 105.7438 | 107.8595 |
INR | 0.9250 | 0.9478 | 0.9216 | 0.9750 |
BUN | 30.8830 | 29.7391 | 29.4149 | 31.5075 |
Creatinine | 2.6093 | 2.6124 | 2.6071 | 2.6614 |
Sodium | 7.3665 | 7.3600 | 7.3400 | 7.5310 |
AST | 203.9396 | 205.5382 | 203.7963 | 210.7321 |
ALT | 108.6025 | 108.6529 | 108.4927 | 111.5664 |
WBC | 7.1725 | 7.1703 | 7.1603 | 7.3003 |
Lympho | 4.8558 | 4.8563 | 4.8556 | 4.9505 |
CrctProtein | 10.4019 | 10.2527 | 10.0813 | 11.1736 |
Troponin | 0.2688 | 0.2680 | 0.2673 | 0.2876 |
From Table 1, all methods have made their standard deviations smaller than the original data's. Although the means imputation method caused the smallest standard deviations, too much data with the same value in some variables may cause the classifier to have difficulty learning the data. For the other two methods, K-NN Imputer was able to reduce the standard deviation of the data even more, so it was chosen as the final missing value imputation method.
The goal of this study was to build a machine learning classification model to make effective risk prediction for COVID-19 patients by learning the collected physiological index data of patients, and the target variable of the model is whether the patient dies or not.
1) Introduction of models
- Logistic regression
The logistic regression model is one of the classic statistical models; it is often used in the field of medicine. The first step in building a logistic regression model is to establish the associated class of logistic regression as the entry point for the relevant parameter settings of the logistic regression model, and then we need to define the training method. Next, we need to define the training method. Set the data learned by logistic regression, the number of iterations trained and the iteration steps, initialization weights and the proportion of samples calculated for each iteration. The following conditional probabilities are used to learn the classification of data:
P(Y=1|x)=exp(w⋅x+b)1+exp(w⋅x+b) | (3.1) |
P(Y=0|x)=11+exp(w⋅x+b) | (3.2) |
The second step is to use stochastic gradient descent in the logistic regression class used by the model to optimize. This logistic regression class inherits the statistical generalized regression class in principle, and its main parts include gradient updating, gradient descent initialization and other methods. The loss function used in the gradient calculation is
J(θ)=12n∑i=1(hθ(x(t))−yt))2 | (3.3) |
The third step needs to use the method of maximum likelihood estimation in statistics to calculate the parameters according to the training of the logistic regression model. Because we assume that each sample of the data is independent of each other, we assume that the probability of all samples occurring is equal to the product of their respective probability of occurrence. Then the likelihood function for n independent samples is
L(θ)=n∏i=1f(x;θ)=n∏i=1[hθ(x)y(i)(1−hθ(x)1−y(i))] | (3.4) |
The fourth step is to learn the logistic regression model by using the inheritance generalized regression class method. First of all, we need to add the bias to the sample, initialize the weight, optimize the calculation weight and select the optimal weight. In the process of optimizing the weights, the solution of the weights needs to be carried out by using the gradient descent method. The gradient calculation is a calculation method that calls the logical gradient class, and it uses the least squares method to calculate the gradient value and loss value of the sample. Using logistic regression classes for weight updates, the updated rules are
wj:=wj+ηn∑i=1(y(i)−ϕ(z(i)))x(i)j | (3.5) |
After the logistic regression model is trained, we can use the logistic regression model class to save various parameters, including feature weight vectors and biases.
- K-NN
K-NN is a classic supervised learning model. People first use K-NN to learn the data from the training set. For the data of the test dataset, K-NN will determine the classifications of the data of the test dataset based on the votes of the K training set datasets closest to the data of the test set. K-NN typically uses Euclidean distance to calculate the distance between data; a distance of two N-dimensional data points x and y will be computed as:
d(x,y):=√(x1−y1)2+(x2−y2)2+⋅+(xn−yn)2 | (3.6) |
- Centroid displacement-based K-nearest neighbors (CDNN)
In the case of K-NN, its majority vote can be a problem if the distances between the test instance and its nearest neighbors vary widely. To overcome this problem, Nguyen et al. [34] have proposed CDNN algorithm. In the case of CDNN, the nearest neighbors are grouped into separated sets of the same class label. Then, the centroids of each set and the displacement of centroids will be calculated if the test instance x is inserted into the set. The test instance is then assigned to the set in which the centroid displacement is minimum.
CDNN addresses the simple voting issue in the classic K-NN algorithm. CDNN is adaptive to noise and class distributions, and it allows for the correct class label to be assigned to the test instance without extended tuning of the parameter K for the dataset.
- SVM
The SVM is also a classic statistical learning model. The basic model of a SVM is a linear classifier with the maximum interval defined on the feature space, and the requirement for the maximum interval makes it different from the perceptron model. SVMs also include kernel tricks, which makes it essentially a nonlinear classifier. The learning strategy of SVMs is interval maximization, which can be formalized as a problem solving convex quadratic programming. In this way, the SVM is the optimal algorithm for solving convex quadratic programming [35].
For linearly separable training datasets, the SVM learns by maximizing the interval or equivalently solving the corresponding convex quadratic programming problem, and the resulting separated hyperplane is
w∗⋅x+b∗=0 | (3.7) |
the corresponding classification decision function:
f(x)=sign(w∗⋅x+b∗) | (3.8) |
Such an SVM is called a linear separable SVM.
For the training dataset of nonlinear classification, an SVM learns through the use of kernel function and soft interval optimization, or convex quadratic programming, and the resulting classification decision function is
f(x)=sign(N∑i=1a∗iyiK(x,xi)+b∗) | (3.9) |
Such an SVM is called a nonlinear SVM.
- Random forest
The random forest is a typical sample of bagging algorithms in ensemble learning. The weak classifiers that construct the random forest algorithm are decision trees; a random forest constructs n different sample datasets by randomly sampling the data, and it establishes n decision tree models corresponding to these n datasets as its weak classifiers. After running these weak classifiers for learning, the random forest votes according to the prediction results of all weak classifiers to get the final prediction result. As an effective ensemble learning model, a random forest can also efficiently process high-dimensional data and data containing missing values.
- LightGBM
LightGBM was proposed by Ke et al. [36]. Its internal decision tree uses a leaf-wise growth strategy and sets the max_depth parameter to prevent overfitting. LightGBM also uses the histogram algorithm. According to the data binning strategy, the nodes of the decision tree can improve the calculation speed when splitting. The two learning methods of support feature parallelism and data parallelism further reduce the computational cost. In addition, the gradient-based one-side sampling and exclusive feature bundling algorithms included in LightGBM have both contributed to improving the classification accuracy.
- XGBoost
XGBoost model is a very powerful ensemble learning model which was proposed by Chen and Guestrin [16]. Compared with GBDT, XGBoost optimizes the operation efficiency of the algorithm through the parallel selection algorithm, and it also adds a regularization part to the loss, which has better generalization ability.
2) Hyperparameter tuning
For machine learning models, they usually have a lot of hyperparameters that need to be determined when the models learn data. In order to make machine learning models perform better, Grid SearchCV was used to help models determine the values of the hyperparameters.
3) Division of dataset
In machine learning tasks, researchers often divide all data into training datasets and test datasets, so that the models learn from the data from the training datasets and predict the test datasets to analyze the performance of the model. In this study, in order to control the occurrence of overfitting and underfitting, 50, 60, 70 and 80% of the data were set as the training dataset, respectively, and the effect of model learning was compared.
4) Sample balancing processing
From the exploratory data analysis in the previous part, it can be found that the classification variable of the data set—the patient died has a problem of category imbalance. Since the number of dead patients is far less than the number of surviving patients, the models may not be able to effectively learn better classification rules from the data. To eliminate this problem, the SMOTE algorithm was used for oversampling before using the models for learning. SMOTE is an effective oversampling algorithm which can effectively deal with the imbalance of data categories and avoid the overfitting problem caused by traditional oversampling methods[37]. So, SMOTE was used to oversample the training dataset before using models to learn the training dataset.
5) Data scaling
Due to the different medical tests contained in the data, the numerical sizes of the continuous variables in the data varied greatly. Regarding the models used to fit the data such as logistic regression, K-NN and the SVM, differences between the numerical sizes of variables will caused some models to consider larger arrays of variables, although these variables may not be more important. The RobustScaler algorithm was used to do the work of data scaling. Algorithms such as z-score normalization may cause data to easily lose outliers, while the RobustScaler method has stronger parameter adjustment capabilities for data centralization and data scaling robustness[38]. RobustScaler applied robust statistics on outliers to scale features, its function is
x′=x−xmedianIQR | (3.10) |
In the function, IQR is the quartile range of the data.
6) Model evaluation
The performance of machine learning models often requires researchers to analyze by calculating multiple indicators of the model. Model prediction accuracy is one of the most important indicators, and it intuitively shows the accuracy of the model in terms of predicting test data sets; the area under curve (AUC) is defined as the area under the ROC curve. As a commonly used indicator to measure the performance of machine learning classification models, the machine learning classification models with higher AUC values are better; the Kolmogorov-Smirnov (KS) statistic was proposed by A.N. Kolmogorov and N.V. Smirnov. As a standard to measure models' differentiations, the larger the value of this indicator, the stronger the risk ranking ability of the model. KS statistics can also be understood as the ability to distinguish between right and wrong categories. The larger its value, the more effectively it can give correct prediction results when the model is used for classification prediction. The KS statistic is calculated from the empirical cumulative distribution function, which is defined as
KS=Max{|cum(bad_rate)−cum(good_rate)|} | (3.11) |
The learning curve is another common way to evaluate model performance in machine learning. The learning curve is similar to the human learning curve, which can be explained as the prediction ability of the model as the data of model learning increases. The learning curve is often used to analyze whether the machine learning model has the problem of over-fitting or under-fitting.
After the data set was divided and scaled and the sample balancing of the training dataset was completed, the models were used to fit the training dataset. Then, the performances of the models on the test data set were evaluated based on the accuracy, AUC value and KS statistic value. Finally, the learning curve of the best-performing model was drawn.
After learning the training data set, the computing results for various performance indicators predicted by the model selected in this study on the test data set were as shown in Tables 2–5.
Model | Accuracy | AUC | KS |
Logistic Regression | 0.7449 | 0.7987 | 0.4698 |
K-NN | 0.7436 | 0.7148 | 0.3811 |
CDNN | 0.7008 | 0.7026 | 0.4052 |
SVM | 0.7610 | 0.5021 | 0.0054 |
Random Forest | 0.8154 | 0.8383 | 0.5279 |
LightGBM | 0.8183 | 0.8296 | 0.4946 |
XGBoost | 0.8217 | 0.8338 | 0.5048 |
Model | Accuracy | AUC | KS |
Logistic Regression | 0.7480 | 0.7983 | 0.4613 |
K-NN | 0.7434 | 0.7033 | 0.3552 |
CDNN | 0.7183 | 0.7059 | 0.4118 |
SVM | 0.7533 | 0.5022 | 0.0067 |
Random Forest | 0.8207 | 0.8317 | 0.5049 |
LightGBM | 0.8111 | 0.8178 | 0.4826 |
XGBoost | 0.8175 | 0.8300 | 0.4897 |
Model | Accuracy | AUC | KS |
Logistic Regression | 0.7405 | 0.7887 | 0.4411 |
K-NN | 0.7411 | 0.7116 | 0.3845 |
CDNN | 0.7058 | 0.6978 | 0.3956 |
SVM | 0.7504 | 0.7285 | 0.4051 |
Random Forest | 0.8147 | 0.8261 | 0.4967 |
LightGBM | 0.8190 | 0.8108 | 0.4725 |
XGBoost | 0.8140 | 0.8211 | 0.4839 |
Model | Accuracy | AUC | KS |
Logistic Regression | 0.7349 | 0.7919 | 0.4465 |
K-NN | 0.7434 | 0.7033 | 0.3552 |
CDNN | 0.7137 | 0.7270 | 0.7270 |
SVM | 0.7338 | 0.7901 | 0.4433 |
Random Forest | 0.8229 | 0.8298 | 0.5085 |
LightGBM | 0.8134 | 0.8197 | 0.4898 |
XGBoost | 0.8176 | 0.8248 | 0.4800 |
By observing the performance indicators of the above models on the test dataset, it can be found that, ensemble learning models are better than other models. First, K-NN Imputer was used to supplement the missing values of the original data, and then the random number seed was set to 933 and 50% of the data was selected. The data were oversampled by using the SMOTE method, and the oversampled data were used as the test data set. The other 50% of the original data supplemented with missing values was set as the test dataset. Using the random forest model with the hyperparameters max depth = 15 and n estimators = 150 to learn this training dataset, the resulting model would get a better area under the ROC curve on the test dataset, around 0.84.
In order to better analyze the performance of the model, the KS curve and learning curve of the random forest model were drawn as respectively shown in Figures 3 and 4.
The KS curve shows that the true positive rate is not large enough. Maybe more data need to be added to the model to fit the data better. The learning curve randomly extracts data sets of different sizes through cross validation to test the performance of the model. In Figure 4, the training score curve is always close to the level of 1; the validation score curve fluctuates between 0.82 and 0.83, but there are large distances from the training score curve, which indicates that the model needs more training samples to improve its performance.
As a powerful ensemble learning model, random forests can calculate the importance of each variable in the data to the whole model while learning the data. The sum of the importance of all variables was 1. The larger the value, the higher its importance for classification prediction. In the random forest model, the five variables with high importance to the final classification results (the importance level is greater than 0.05) were found to be the MAP in mmHg (MAP) of the COVID-19 patients at admission, the ages of the COVID-19 patients (Age), the COVID-19 patients' CRP test values in mg/L (CrctProtein), the COVID-19 patients' blood urea nitrogen in mg/dL (BUN) and the COVID-19 patients' clinical troponin value in ng/mL (troponin).
In order to verify whether there was a significant difference between the data of these features of the dead COVID-19 patients and the alive COVID-19 patients, a two-sample T-test was conducted on the data of the above characteristics of the dead patients and the surviving patients. The results are shown in Table 6.
Feature | Mean of dead patients | Mean of alive patients |
MAP∗∗∗1, 2 | 75.2601 | 89.1829 |
Age∗∗∗3 | 71.9547 | 60.6034 |
CrctProtein∗∗∗4 | 16.9394 | 10.6453 |
BUN∗∗∗5 | 44.0581 | 26.4724 |
Troponin∗∗∗6 | 0.1140 | 0.0422 |
1 *** means the p-value of two-sample T-test less than 0.001. 2 MAP means MAP in mmHg. 3 Age means ages of patients. 4 CrctProtein means CRP tests' values in mg/L. 5 BUN means blood urea nitrogen in mg/dL. 6 Troponin means clinical Troponin values in ng/mL. |
It can be seen in Table 6 that, except for diabetes mellitus, all P values from the two-sample T-test are smaller than 0.001; this means that there were significant differences in the data of the other five physiological indicators of COVID-19 patients who died and lived. Based on the above scholars' research or meta-analysis [29,30,31,32,33], it can be concluded that the five important factors in a random forest model can be used to predict whether COVID-19 patients have a risk of death.
Regarding the results of the analysis above, here are some suggestions to for healthcare organizations.
Firstly, healthcare organizations can collect data on the same physiological indicators of patients admitted to hospital with COVID-19 as the study[23] says, and use the random forest model built in this study to predict the risk of death in patients admitted to the hospital. At the same time, healthcare organizations can also determine which patients are more likely to get sicker or even die based on the data of five physiological indicators closely related to whether patients admitted to the hospital due to COVID-19 die, i.e., MAP, ages of patients, CRP test values, mean blood urea nitrogen and clinical troponin values of COVID-19 patients.
After completing an analysis of the risk of severe illness and death in patients admitted to the hospital with COVID-19, healthcare organizations can more effectively schedule limited medical resources. For example, ICU wards, ventilators and more experienced healthcare workers should be prioritized for COVID-19 admissions who are more likely to get sicker or die.
Second, healthcare organizations can take action to store and even share their patients' physiological indicator data while protecting their privacy. In the future, if people have to face the next COVID-19 pandemic or other infectious disease pandemic, healthcare organizations can build machine learning models based on stored and collected physiological indicator data of admitted patients. With high-performance models, healthcare organizations can also effectively predict a patient's risk of exacerbation or death, and allocate limited medical resources more specifically, as they did for the COVID-19 patients mentioned earlier.
Moreover, governments should also take action to help healthcare organizations build shared databases of citizens' physiological indicator data while protecting their privacy; or, they should expand the coverage of health insurance for older people to meet the next COVID-19 pandemic or other infectious disease pandemic.
Finally, people should also do more scientific physical exercise in their daily lives to enhance their resistance. At the same time, people should also have medical examinations at reasonable intervals. If there are abnormalities in physiological indicator data in physical examination results, people should consult doctors in time and improve the health problems associated with abnormal physiological indicators through treatment or other means.
The AUC value of the random forest has been improved compared with the AUC values of the previous models in the relevant studies[23,24]. But this prediction still has space for improvement; for instance, adding more data on COVID-19 admissions to the training dataset may make the model more generalizable, with higher prediction accuracy and AUC values.
Since widespread COVID-19 vaccination efforts around the world are already working, the impact of COVID-19 vaccinations on exacerbated deaths in COVID-19 patients can be analyzed by including data on COVID-19 vaccination patients. The risk of death for COVID-19 patients who have been vaccinated against COVID-19 will also be able to be effectively predicted.
Thanks to David J. Altschul, Santiago R. Unda, Joshua Benton and other authors of [23] for sharing their data on figshare.com.
The author declares that there is no conflicts of interest.
[1] |
G. Gabutti, E. d'Anchera, F. De Motoli, M. Savio, A. Stefanati, The epidemiological characteristics of the COVID-19 pandemic in Europe: Focus on Italy, Int. J. Environ. Res. Public Health, 18 (2021), 2942. https://doi.org/10.3390/ijerph18062942 doi: 10.3390/ijerph18062942
![]() |
[2] |
Y. Mahendradhata, N. L. P. E. Andayani, E. T. Hasri, M. D. Arifi, R. G. M. S. Siahaan, D. A. Solikha, et al., The capacity of the Indonesian healthcare system to respond to COVID-19, Front. Public Health, 9 (2021), 887. https://doi.org/10.3389/fpubh.2021.649819 doi: 10.3389/fpubh.2021.649819
![]() |
[3] | A. Johnson, A. B. Amin, A. R. Ali, B. Hoots, B. L. Cadwell, S. Arora, et al. COVID-19 incidence and death rates among unvaccinated and fully vaccinated adults with and without booster doses during periods of Delta and Omicron variant emergence-25 US Jurisdictions, April 4–December 25, 2021, Morb. Mortal. Wkly. Rep., 71 (2022). https://doi.org/10.15585/mmwr.mm7104e2 |
[4] |
A. Notari, G. Torrieri, COVID-19 transmission risk factors, Pathog. Glob. Health, 116 (2020), 146–177. https://doi.org/10.1080/20477724.2021.1993676 doi: 10.1080/20477724.2021.1993676
![]() |
[5] |
M. Coccia, Optimal levels of vaccination to reduce COVID-19 infected individuals and deaths: A global analysis, Environ. Res., 204 (2022), 112314. https://doi.org/10.1016/j.envres.2021.112314 doi: 10.1016/j.envres.2021.112314
![]() |
[6] | I. Benati, M. Coccia, Global analysis of timely COVID-19 vaccinations: improving governance to reinforce response policies for pandemic crises, Int. J. Health Governance, 27 (2022). https://doi.org/10.1108/IJHG-07-2021-0072 |
[7] |
B. V. Duong, P. Larpruenrudee, T. Fang, S. I. Hossain, S. C. Saha, Y. Gu, et al., Is the SARS CoV-2 omicron variant deadlier and more transmissible than delta variant?, Int. J. Environ. Res. Public Health, 19 (2022), 4586. https://doi.org/10.3390/ijerph19084586 doi: 10.3390/ijerph19084586
![]() |
[8] |
M. Hung, B. Mennell, A. Christensen, A. Mohajeri, H. Azabache, R. Moffat, Trends in COVID-19 inpatient cases and hospital capacities during the emergence of the omicron variant in the United States, COVID, 2 (2022), 1207–1213. https://doi.org/10.3390/covid2090087 doi: 10.3390/covid2090087
![]() |
[9] |
M. E. Shipe, S. A. Deppen, F. Farjah, E. L. Grogan, Developing prediction models for clinical use using logistic regression: An overview, J. Thorac. Dis., 11 (2019), S574. https://doi.org/10.21037/jtd.2019.01.25 doi: 10.21037/jtd.2019.01.25
![]() |
[10] |
E. Christodoulou, J. Ma, G. S. Collins, E. W. Steyerberg, J. Y. Verbakel, B. V. Calster, A systematic review shows no performance benefit of machine learning over logistic regression for clinical prediction models, J. Clin. Epidemiol., 110 (2019), 12–22. https://doi.org/10.1016/j.jclinepi.2019.02.004 doi: 10.1016/j.jclinepi.2019.02.004
![]() |
[11] |
W. Shaban, A. Rabie, A. Saleh, M. Abo-Elsoud, A new COVID-19 patients detection strategy (CPDS) based on hybrid feature selection and enhanced KNN classifier, Knowl.-Based Syst., 205 (2020), 106270. https://doi.org/10.1016/j.knosys.2020.106270 doi: 10.1016/j.knosys.2020.106270
![]() |
[12] |
V. Singh, R. C. Poonia, S. Kumar, P. Dass, P. Agarwal, V. Bhatnagar et al., Prediction of COVID-19 corona virus pandemic based on time series data using support vector machine, J. Discret. Math. Sci. Cryptogr., 23 (2020), 1583–1597. https://doi.org/10.1080/09720529.2020.1784535 doi: 10.1080/09720529.2020.1784535
![]() |
[13] | L. Breiman, Random forests, in Machine learning, 45 (2001), 5–32. https://doi.org/10.1023/A:1010933404324 |
[14] |
M. Khalilia, S. Chakraborty, M. Popescu, Predicting disease risks from highly imbalanced data using random forest, BMC Med. Inf. Decis. Making, 11 (2011), 1–13. https://doi.org/10.1186/1472-6947-11-51 doi: 10.1186/1472-6947-11-51
![]() |
[15] | M. Ezzoddin, H. Nasiri, M. Dorrigiv, Diagnosis of COVID-19 cases from chest X-ray images using deep neural network and LightGBM, in 2022 International Conference on Machine Vision and Image Processing (MVIP), (2022), 1–7. https://doi.org/10.1109/MVIP53647.2022.9738760 |
[16] | T. Chen, C. Guestrin, Xgboost: A scalable tree boosting system, in Proceedings of the 22nd ACM SIGKDD International Conference on Knowledge Discovery and Data Mining, (2016), 785–794. https://doi.org/10.1145/2939672.2939785 |
[17] |
S. Li, X. Zhang, Research on orthopedic auxiliary classification and prediction model based on XGBoost algorithm, Neural Comput. Appl., 32 (2020), 1971–1979. https://doi.org/10.1007/s00521-019-04378-4 doi: 10.1007/s00521-019-04378-4
![]() |
[18] |
M. Covino, C. Sandroni, M. Santoro, L. Sabia, B. Simeoni, M. Bocci, et al., Predicting intensive care unit admission and death for COVID-19 patients in the emergency department using early warning scores, Resuscitation, 156 (2020), 84–91. https://doi.org/10.1016/j.resuscitation.2020.08.124 doi: 10.1016/j.resuscitation.2020.08.124
![]() |
[19] |
Y. Allenbach, D. Saadoun, G. Maalouf, M. Vieira, A. Hellio, J. Boddaert et al., Development of a multivariate prediction model of intensive care unit transfer or death: A French prospective cohort study of hospitalized COVID-19 patients, PloS one, 15 (2020), e0240711. https://doi.org/10.1371/journal.pone.0240711 doi: 10.1371/journal.pone.0240711
![]() |
[20] |
S. Mirri, G. Delnevo, M. Roccetti, Is a COVID-19 second wave possible in emilia-romagna (Italy)? Forecasting a future outbreak with particulate pollution and machine learning, Comput., 8 (2020), 74. https://doi.org/10.3390/computation8030074 doi: 10.3390/computation8030074
![]() |
[21] |
H. Estiri, Z. Strasser, S. Murphy, Individualized prediction of COVID-19 adverse outcomes with MLHO, Sci. Rep., 11 (2021), 5322. https://doi.org/10.1038/s41598-021-84781-x doi: 10.1038/s41598-021-84781-x
![]() |
[22] |
A. S. Yadaw, Y. Li, S. Bose, R. Iyengar, S. Bunyavanich, G. Pandey, Clinical features of COVID-19 mortality: Development and validation of a clinical prediction model, Lancet Digital Health, 2 (2020), 516–525. https://doi.org/10.1016/S2589-7500(20)30217-X doi: 10.1016/S2589-7500(20)30217-X
![]() |
[23] |
D. J. Altschul, S. R. Unda, J. Benton, R. de la Garza Ramos, P. Cezayirli, M. Mehler, et al. A novel severity score to predict inpatient mortality in COVID-19 patients, Sci. Rep., 10 (2020), 1–8. https://doi.org/10.1038/s41598-020-73962-9 doi: 10.1038/s41598-020-73962-9
![]() |
[24] | H. Wang, H. Ai, Y. Fu, Q. Li, R. Cui, X. Ma, et al., Development of an early warning model for predicting the death risk of coronavirus disease 2019 based on data immediately available on admission, Front. Med., (2021), 1302. https://doi.org/10.3389/fmed.2021.699243 |
[25] | L. Zhang, A. Xu, L. An, M. Li, Bayesian inference of system reliability for multicomponent stress-strength model under marshall-olkin weibull distribution, Systems, (2022). https://doi.org/10.3390/systems10060196 |
[26] |
Y. Wang, W. Wang, Y. Tang, A Bayesian semiparametric accelerate failure time mixture cure model, Int. J. Biostat., 18 (2021), 473–485. https://doi.org/10.1515/ijb-2021-0012 doi: 10.1515/ijb-2021-0012
![]() |
[27] | L. Zhuang, A. Xu, B. Wang, Y. Xue, S. Zhang, Data analysis of progressive‐stress accelerated life tests with group effects, Qual. Technol. Quant. Manage., (2022), 1–21. https://doi.org/10.1080/16843703.2022.2147690 |
[28] | G. S. Rao, M. Aslam, Inspection plan for COVID-19 patients for weibull distribution using repetitive sampling under indeterminacy, BMC Med. Res. Methodol., 21 (2021). https://doi.org/10.1186/s12874-021-01387-7 |
[29] |
A. Caillon, K. Zhao, K. O. Klein, C. M. T. Greenwood, Z. Lu, P. Paradis, et al., High systolic blood pressure at hospital admission is an important risk factor in models predicting outcome of COVID-19 patients, Am. J. Hypertens., 34 (2021), 282–290. https://doi.org/10.1093/ajh/hpaa225 doi: 10.1093/ajh/hpaa225
![]() |
[30] |
M. V. Blagosklonny, From causes of aging to death from COVID-19, Aging, 12 (2020), 10004–10021. https://doi.org/10.18632/aging.103493 doi: 10.18632/aging.103493
![]() |
[31] | G. Wang, C. Wu, Q. Zhang, F. Wu, B. Yu, J. Lv et al., C-Reactive protein level may predict the risk of COVID-19 aggravation, Open Forum Infect. Dis., 7 (2020). https://doi.org/10.1093/ofid/ofaa153 |
[32] |
F. Ok, O. Erdogan, E. Durmus, S. Carkci, A. Canik, Predictive values of blood urea nitrogen/creatinine ratio and other routine blood parameters on disease severity and survival of COVID‐19 patients, J. Med. Virol., 93 (2020), 786–793. https://doi.org/10.1002/jmv.26300 doi: 10.1002/jmv.26300
![]() |
[33] |
G. Lippi, C. Lavie, F. Sanchis-Gomar, Cardiac troponin I in patients with coronavirus disease 2019 (COVID-19): Evidence from a meta-analysis, Prog. Cardiovasc. Dis., 63 (2020), 390–391. https://doi.org/10.1016/j.pcad.2020.03.001 doi: 10.1016/j.pcad.2020.03.001
![]() |
[34] |
B. P. Nguyen, W. Tay, C. Chui, Robust biometric recognition from palm depth images for gloved hands, IEEE Trans. Hum.-Mach. Syst., 45 (2015), 799–804. https://doi.org/10.1109/THMS.2015.2453203 doi: 10.1109/THMS.2015.2453203
![]() |
[35] |
C. Chang, C. Lin, LIBSVM: A library for support vector machines, ACM Trans. Intell. Syst. Technol., 2 (2011), 1–27. https://doi.org/10.1145/1961189.1961199 doi: 10.1145/1961189.1961199
![]() |
[36] | G. Ke, Q. Meng, T. Finley, T. Wang, W. Chen, W. Ma, et al., Lightgbm: A highly efficient gradient boosting decision tree, in Proceedings of the 31st International Conference on Neural Information Processing Systems, (2017), 3149–3157. |
[37] |
N. V. Chawla, K. W. Bowyer, L. O. Hall, W. P. Kegelmeyer, SMOTE: synthetic minority over-sampling technique, J. Artif. Intell. Res., 16 (2002), 321–357. https://doi.org/10.1613/jair.953 doi: 10.1613/jair.953
![]() |
[38] | F. Pedregosa, G. Varoquaux, A. Gramfort, V. Michel, B. Thirion, O. Grisel, et al., Scikit-learn: sklearn. preprocessing. robustscaler, J. Mach. Learn. Res., 12 (2011), 2825–2830. |
1. | Ágoston Hamar, Daryan Mohammed, Alex Váradi, Róbert Herczeg, Norbert Balázsfalvi, Béla Fülesdi, István László, Lídia Gömöri, Péter Attila Gergely, Gabor Laszlo Kovacs, Krisztián Jáksó, Katalin Gombos, COVID-19 mortality prediction in Hungarian ICU settings implementing random forest algorithm, 2024, 14, 2045-2322, 10.1038/s41598-024-62791-9 | |
2. | Olena Antonyuk , Oleksii Stavyskyi , Predicting models of inpatient death risk accompanied by coronavirus disease in healthcare establishments as an additional tool for decision-making, 2025, 152, 1996-353X, 97, 10.32345/USMYJ.1(152).2025.97-113 |
Variable | Iterative imputer | K-NN imputer | Mean imputer | Original data |
LOS | 6.7489 | 6.7912 | 6.7384 | 6.9964 |
OsSats | 8.1218 | 8.0724 | 8.0423 | 8.1888 |
Temp | 4.1045 | 4.3043 | 4.1030 | 4.1713 |
MAP | 16.5238 | 16.4947 | 16.4064 | 16.8071 |
Ddimer | 5.1079 | 5.0681 | 4.9588 | 5.6648 |
Plts | 106.0403 | 106.0664 | 105.7438 | 107.8595 |
INR | 0.9250 | 0.9478 | 0.9216 | 0.9750 |
BUN | 30.8830 | 29.7391 | 29.4149 | 31.5075 |
Creatinine | 2.6093 | 2.6124 | 2.6071 | 2.6614 |
Sodium | 7.3665 | 7.3600 | 7.3400 | 7.5310 |
AST | 203.9396 | 205.5382 | 203.7963 | 210.7321 |
ALT | 108.6025 | 108.6529 | 108.4927 | 111.5664 |
WBC | 7.1725 | 7.1703 | 7.1603 | 7.3003 |
Lympho | 4.8558 | 4.8563 | 4.8556 | 4.9505 |
CrctProtein | 10.4019 | 10.2527 | 10.0813 | 11.1736 |
Troponin | 0.2688 | 0.2680 | 0.2673 | 0.2876 |
Model | Accuracy | AUC | KS |
Logistic Regression | 0.7449 | 0.7987 | 0.4698 |
K-NN | 0.7436 | 0.7148 | 0.3811 |
CDNN | 0.7008 | 0.7026 | 0.4052 |
SVM | 0.7610 | 0.5021 | 0.0054 |
Random Forest | 0.8154 | 0.8383 | 0.5279 |
LightGBM | 0.8183 | 0.8296 | 0.4946 |
XGBoost | 0.8217 | 0.8338 | 0.5048 |
Model | Accuracy | AUC | KS |
Logistic Regression | 0.7480 | 0.7983 | 0.4613 |
K-NN | 0.7434 | 0.7033 | 0.3552 |
CDNN | 0.7183 | 0.7059 | 0.4118 |
SVM | 0.7533 | 0.5022 | 0.0067 |
Random Forest | 0.8207 | 0.8317 | 0.5049 |
LightGBM | 0.8111 | 0.8178 | 0.4826 |
XGBoost | 0.8175 | 0.8300 | 0.4897 |
Model | Accuracy | AUC | KS |
Logistic Regression | 0.7405 | 0.7887 | 0.4411 |
K-NN | 0.7411 | 0.7116 | 0.3845 |
CDNN | 0.7058 | 0.6978 | 0.3956 |
SVM | 0.7504 | 0.7285 | 0.4051 |
Random Forest | 0.8147 | 0.8261 | 0.4967 |
LightGBM | 0.8190 | 0.8108 | 0.4725 |
XGBoost | 0.8140 | 0.8211 | 0.4839 |
Model | Accuracy | AUC | KS |
Logistic Regression | 0.7349 | 0.7919 | 0.4465 |
K-NN | 0.7434 | 0.7033 | 0.3552 |
CDNN | 0.7137 | 0.7270 | 0.7270 |
SVM | 0.7338 | 0.7901 | 0.4433 |
Random Forest | 0.8229 | 0.8298 | 0.5085 |
LightGBM | 0.8134 | 0.8197 | 0.4898 |
XGBoost | 0.8176 | 0.8248 | 0.4800 |
Feature | Mean of dead patients | Mean of alive patients |
MAP∗∗∗1, 2 | 75.2601 | 89.1829 |
Age∗∗∗3 | 71.9547 | 60.6034 |
CrctProtein∗∗∗4 | 16.9394 | 10.6453 |
BUN∗∗∗5 | 44.0581 | 26.4724 |
Troponin∗∗∗6 | 0.1140 | 0.0422 |
1 *** means the p-value of two-sample T-test less than 0.001. 2 MAP means MAP in mmHg. 3 Age means ages of patients. 4 CrctProtein means CRP tests' values in mg/L. 5 BUN means blood urea nitrogen in mg/dL. 6 Troponin means clinical Troponin values in ng/mL. |
Variable | Iterative imputer | K-NN imputer | Mean imputer | Original data |
LOS | 6.7489 | 6.7912 | 6.7384 | 6.9964 |
OsSats | 8.1218 | 8.0724 | 8.0423 | 8.1888 |
Temp | 4.1045 | 4.3043 | 4.1030 | 4.1713 |
MAP | 16.5238 | 16.4947 | 16.4064 | 16.8071 |
Ddimer | 5.1079 | 5.0681 | 4.9588 | 5.6648 |
Plts | 106.0403 | 106.0664 | 105.7438 | 107.8595 |
INR | 0.9250 | 0.9478 | 0.9216 | 0.9750 |
BUN | 30.8830 | 29.7391 | 29.4149 | 31.5075 |
Creatinine | 2.6093 | 2.6124 | 2.6071 | 2.6614 |
Sodium | 7.3665 | 7.3600 | 7.3400 | 7.5310 |
AST | 203.9396 | 205.5382 | 203.7963 | 210.7321 |
ALT | 108.6025 | 108.6529 | 108.4927 | 111.5664 |
WBC | 7.1725 | 7.1703 | 7.1603 | 7.3003 |
Lympho | 4.8558 | 4.8563 | 4.8556 | 4.9505 |
CrctProtein | 10.4019 | 10.2527 | 10.0813 | 11.1736 |
Troponin | 0.2688 | 0.2680 | 0.2673 | 0.2876 |
Model | Accuracy | AUC | KS |
Logistic Regression | 0.7449 | 0.7987 | 0.4698 |
K-NN | 0.7436 | 0.7148 | 0.3811 |
CDNN | 0.7008 | 0.7026 | 0.4052 |
SVM | 0.7610 | 0.5021 | 0.0054 |
Random Forest | 0.8154 | 0.8383 | 0.5279 |
LightGBM | 0.8183 | 0.8296 | 0.4946 |
XGBoost | 0.8217 | 0.8338 | 0.5048 |
Model | Accuracy | AUC | KS |
Logistic Regression | 0.7480 | 0.7983 | 0.4613 |
K-NN | 0.7434 | 0.7033 | 0.3552 |
CDNN | 0.7183 | 0.7059 | 0.4118 |
SVM | 0.7533 | 0.5022 | 0.0067 |
Random Forest | 0.8207 | 0.8317 | 0.5049 |
LightGBM | 0.8111 | 0.8178 | 0.4826 |
XGBoost | 0.8175 | 0.8300 | 0.4897 |
Model | Accuracy | AUC | KS |
Logistic Regression | 0.7405 | 0.7887 | 0.4411 |
K-NN | 0.7411 | 0.7116 | 0.3845 |
CDNN | 0.7058 | 0.6978 | 0.3956 |
SVM | 0.7504 | 0.7285 | 0.4051 |
Random Forest | 0.8147 | 0.8261 | 0.4967 |
LightGBM | 0.8190 | 0.8108 | 0.4725 |
XGBoost | 0.8140 | 0.8211 | 0.4839 |
Model | Accuracy | AUC | KS |
Logistic Regression | 0.7349 | 0.7919 | 0.4465 |
K-NN | 0.7434 | 0.7033 | 0.3552 |
CDNN | 0.7137 | 0.7270 | 0.7270 |
SVM | 0.7338 | 0.7901 | 0.4433 |
Random Forest | 0.8229 | 0.8298 | 0.5085 |
LightGBM | 0.8134 | 0.8197 | 0.4898 |
XGBoost | 0.8176 | 0.8248 | 0.4800 |
Feature | Mean of dead patients | Mean of alive patients |
MAP∗∗∗1, 2 | 75.2601 | 89.1829 |
Age∗∗∗3 | 71.9547 | 60.6034 |
CrctProtein∗∗∗4 | 16.9394 | 10.6453 |
BUN∗∗∗5 | 44.0581 | 26.4724 |
Troponin∗∗∗6 | 0.1140 | 0.0422 |
1 *** means the p-value of two-sample T-test less than 0.001. 2 MAP means MAP in mmHg. 3 Age means ages of patients. 4 CrctProtein means CRP tests' values in mg/L. 5 BUN means blood urea nitrogen in mg/dL. 6 Troponin means clinical Troponin values in ng/mL. |