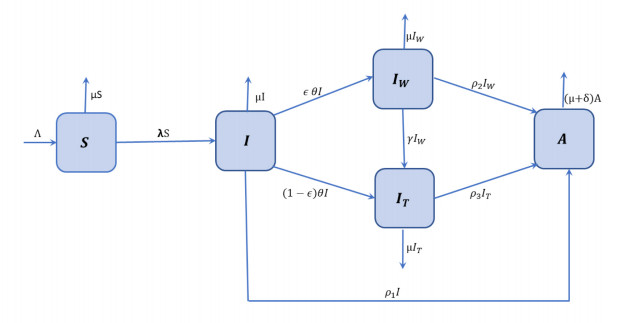
The management of HIV/AIDS has evolved ever since advent of the disease in the past three decades. Many countries have had to revise their policies as new information on the virus, and its transmission dynamics emerged. In this paper, we track the changes in Botswana's HIV/AIDS response and treatment policies using a piece-wise system of differential equations. The policy changes are easily tracked in three epochs. Models for each era are formulated from a "grand model" that can be linked to all the epochs. The grand model's steady states are determined and analysed in terms of the model reproduction number, R0. The model exhibits a backward bifurcation, where a stable disease-free equilibrium coexists with a stable endemic equilibrium when R0<1. The stability of the models for the other epochs can be derived from that of the grand model by setting some parameters to zero. The models are fitted to HIV/AIDS prevalence data from Botswana for the past three decades. The changes in the populations in each compartment are tracked as the response to the disease and treatment policy changed over time. Finally, projections are made to determine the possible trajectory of HIV/AIDS in Botswana. The implications of the policy changes are easily seen, and a discussion on how these changes impacted the epidemic are articulated. The results presented have crucial impact on how policy changes affected and continue to influence the trajectory of the HIV/AIDS epidemic in Botswana.
Citation: Tefa Kaisara, Farai Nyabadza. Modelling Botswana's HIV/AIDS response and treatment policy changes: Insights from a cascade of mathematical models[J]. Mathematical Biosciences and Engineering, 2023, 20(1): 1122-1147. doi: 10.3934/mbe.2023052
[1] | Esther Chigidi, Edward M. Lungu . HIV model incorporating differential progression for treatment-naive and treatment-experienced infectives. Mathematical Biosciences and Engineering, 2009, 6(3): 427-450. doi: 10.3934/mbe.2009.6.427 |
[2] | Wenshuang Li, Shaojian Cai, Xuanpei Zhai, Jianming Ou, Kuicheng Zheng, Fengying Wei, Xuerong Mao . Transmission dynamics of symptom-dependent HIV/AIDS models. Mathematical Biosciences and Engineering, 2024, 21(2): 1819-1843. doi: 10.3934/mbe.2024079 |
[3] | Federico Papa, Francesca Binda, Giovanni Felici, Marco Franzetti, Alberto Gandolfi, Carmela Sinisgalli, Claudia Balotta . A simple model of HIV epidemic in Italy: The role of the antiretroviral treatment. Mathematical Biosciences and Engineering, 2018, 15(1): 181-207. doi: 10.3934/mbe.2018008 |
[4] | Divine Wanduku . A nonlinear multi-population behavioral model to assess the roles of education campaigns, random supply of aids, and delayed ART treatment in HIV/AIDS epidemics. Mathematical Biosciences and Engineering, 2020, 17(6): 6791-6837. doi: 10.3934/mbe.2020354 |
[5] | M. Hadjiandreou, Raul Conejeros, Vassilis S. Vassiliadis . Towards a long-term model construction for the dynamic simulation of HIV infection. Mathematical Biosciences and Engineering, 2007, 4(3): 489-504. doi: 10.3934/mbe.2007.4.489 |
[6] | Gigi Thomas, Edward M. Lungu . A two-sex model for the influence of heavy alcohol consumption on the spread of HIV/AIDS. Mathematical Biosciences and Engineering, 2010, 7(4): 871-904. doi: 10.3934/mbe.2010.7.871 |
[7] | Miguel Atencia, Esther García-Garaluz, Gonzalo Joya . The ratio of hidden HIV infection in Cuba. Mathematical Biosciences and Engineering, 2013, 10(4): 959-977. doi: 10.3934/mbe.2013.10.959 |
[8] | Nawei Chen, Shenglong Chen, Xiaoyu Li, Zhiming Li . Modelling and analysis of the HIV/AIDS epidemic with fast and slow asymptomatic infections in China from 2008 to 2021. Mathematical Biosciences and Engineering, 2023, 20(12): 20770-20794. doi: 10.3934/mbe.2023919 |
[9] | Gordon Akudibillah, Abhishek Pandey, Jan Medlock . Optimal control for HIV treatment. Mathematical Biosciences and Engineering, 2019, 16(1): 373-396. doi: 10.3934/mbe.2019018 |
[10] | Helong Liu, Xinyu Song . Stationary distribution and extinction of a stochastic HIV/AIDS model with nonlinear incidence rate. Mathematical Biosciences and Engineering, 2024, 21(1): 1650-1671. doi: 10.3934/mbe.2024072 |
The management of HIV/AIDS has evolved ever since advent of the disease in the past three decades. Many countries have had to revise their policies as new information on the virus, and its transmission dynamics emerged. In this paper, we track the changes in Botswana's HIV/AIDS response and treatment policies using a piece-wise system of differential equations. The policy changes are easily tracked in three epochs. Models for each era are formulated from a "grand model" that can be linked to all the epochs. The grand model's steady states are determined and analysed in terms of the model reproduction number, R0. The model exhibits a backward bifurcation, where a stable disease-free equilibrium coexists with a stable endemic equilibrium when R0<1. The stability of the models for the other epochs can be derived from that of the grand model by setting some parameters to zero. The models are fitted to HIV/AIDS prevalence data from Botswana for the past three decades. The changes in the populations in each compartment are tracked as the response to the disease and treatment policy changed over time. Finally, projections are made to determine the possible trajectory of HIV/AIDS in Botswana. The implications of the policy changes are easily seen, and a discussion on how these changes impacted the epidemic are articulated. The results presented have crucial impact on how policy changes affected and continue to influence the trajectory of the HIV/AIDS epidemic in Botswana.
The first official case of HIV in Botswana was identified in 1985. The nation's response to the epidemic dates from the late 1980s with the Short Term Plan (STP) for the years 1987-1989, followed by the Medium Term Plan Ⅰ (MTP Ⅰ) for the years 1991–1996, which outlined a medical and health system response to HIV/AIDS [1]. The Medium Term Plan Ⅱ (MTP Ⅱ) for 1997–2002 developed the institutional structures necessary to organise and manage the national response. The national policy was revised in 1998 following new scientific, epidemiological and medical knowledge about the distribution and transmission of HIV and other sexually transmitted diseases (STDs) and proven effective interventions in prevention and care. The evolution of the national response in Botswana's continued with developing the National Strategic Framework (NSF) for HIV/AIDS 2003–2009. The review of MTP Ⅱ informed the NSF. The second national strategic framework (NSF Ⅱ) established in 2010 outlined the national priorities for the national response from 2010–2016. NSF Ⅱ focused on a costed multi-sectoral and rolling operational plan that guided the implementation of the national response. Due to increased global research on HIV/AIDS dynamics, new knowledge and information on the field rapidly kept changing. Therefore, a review of the HIV/AIDS policy was needed as new findings emerged. As a result, the Botswana national policy on HIV and AIDS was revised in 2012.
Botswana is now on its third national strategic framework (NSF Ⅲ) for 2019–2023. The NSF Ⅲ targets ending AIDS by 2030. This strategic framework has been informed by relevant global, regional and national policies and strategic frameworks. NSF Ⅲ goals include; prioritising an HIV combination prevention strategy, targeting and exceeding the global target (90-90-90) of HIV testing and treatment, reduce HIV related stigma, discrimination and gender vulnerability while promoting and protecting the human rights of people living with HIV.
According to the World Health Organization (WHO), health policy refers to decisions, plans, and actions undertaken to achieve specific health care goals within a society. An explicit health policy can achieve several objectives; it defines a vision for the future, outlines priorities and the expected roles of different population groups, and builds consensus and informs people [2]. Health policy categories may be global, national, local, public and workplace-based. National health policies, strategies and plans play an essential role in defining a country's vision, policy directions, and strategies for ensuring her population's health. For example, an HIV/AIDS policy is a health policy that provides the basic framework for reducing the spread of HIV/AIDS and managing its impacts. Countries have adopted targets for achieving universal access to HIV prevention, treatment, care and support by producing national HIV/AIDS policies.
Mathematical models have a vital role in assisting policymakers in developing HIV/AIDS prevention and treatment strategies. They help in bridging the gap between theory and policy. Some of the several ways models can assist HIV/AIDS policymakers are; comparing the impact and cost-effectiveness of different interventions, generalising the results of randomised controlled trials to different settings, identifying threats to programme success and opportunities for maximising intervention efficiency, evaluating and motoring the extent to which observed trends in HIV prevalence are attributable to HIV/AIDS programme success [3]. Mathematical models have also been used to obtain insights into other HIV/AIDS policy research. They include; evaluation of treatment as a prevention strategy [4,5], the scale-up of microbicides [6], the impact and cost-effectiveness of HIV Pre-exposure Prophylaxis (PrEP) targeting key populations [7,8], the efficacy of the Post-Exposure Prophylaxis (PEP) [9], projecting HIV diagnoses among children and adolescents [10,11], HIV vaccines in future with the presence of current interventions [12], the impact of voluntary medical male circumcision (VMMC) [13] and other recent recent developments in modelling techniques for HIV/AIDS models [14,15,16]. Many mathematical models of HIV/AIDS, with different structures, complexity and parameter choices have contributed to HIV/AIDS research for many years. However, none of the models including the recent work, has tracked how the change in HIV/AIDS policy over the years have impacted or influenced the trajectory of the epidemic. We thus present a unique model that traces the impact of policy changes over time.
In this study, we track the changes in policy for HIV/AIDS testing and treatment in Botswana. In the first phase of the national HIV/AIDS policy, voluntary counselling and testing are encouraged. However, the policy is silent on treatment but rather focusing on behavioural change. Botswana was the first African country to establish a national HIV/AIDS treatment programme, called "Masa", the Setswana word for "a new dawn", in 2002, leading to the second phase of national HIV/AIDS policies. Since the start of the Masa programme, the national guidelines changed to take into account the improved understanding of the biology of HIV, reduce adverse side effects associated with treatment, and accommodate the availability of improved drugs [17]. In 2008, the eligibility criteria threshold for Antiretroviral therapy (ART) changed from a CD4 cell count of 200 cells per cubic millimetre to 250 cells per cubic millimetre. In 2013, it changed again to 350 cells per cubic millimetre. In June 2016, Botswana launched the "Treat All" strategy, promoting universal health coverage and ensuring that all individuals who test positive for HIV get treatment immediately regardless of their viral load or CD4 count. Mathematical models have been developed and fitted to HIV/AIDS data in different countries, see for instance [18,19]. All these articles fit one model to data spanning over a long period. However, none of the models account for the change in dynamics due to the policy changes. We aim to develop deterministic models to integrate HIV/AIDS prevalence data amongst adults, 15 years and above, for Botswana and the change in policies. The objective of this study is to examine the impacts of change in HIV treatment policy over time.
This paper is arranged as follows: The models are developed in the next section and analysed in Section 3. Numerical simulations are presented in Section 4 and the paper is concluded in Section 5.
The HIV/AIDS treatment policy for Botswana can thus be divided into three epochs with respect to the treatment policy. It is important to note that additional epochs can be considered depending on the nature of the response being considered. The first epoch captures the dynamics before introducing the universal ART programme (1990–2001). The second epoch captures the period from which the universal ART programme was rolled out (2002–2015) with criterion eligibility of CD4 count to enrol. Finally, the third epoch captures the era (2017–to date) where eligibility to enrol on ART is a positive test. Comparing the three models, it is notable that the first model changed by introducing treatment in 2002. As a result, two compartments were added in the second epoch to cater for the treatment policy. It is important to note that this modelling framework is similar to that used in [20,21], where individuals were classified according to their immunological staging when they test positive. In 2016, when the treat all policy was launched, the model changed by dropping out of the waiting compartment. We note that the second epoch model is the biggest, and the other two models can be deduced from it by setting some parameters to zero. Given that the first and third epochs can be obtained from the second epoch from a modelling perspective, we begin by looking at the model for the second epoch, which we dub here the "grand model".
We propose a model that captures the dynamics of HIV/AIDS with an ART programme that considers CD4 cell count as an eligibility criterion for treatment. The population of sexually active adults, N(t), is divided into five classes: S(t), for the adult population at risk of being infected by HIV and I(t), for individuals who have contracted HIV and are unaware of their status. Due to unavailable data on the CD4 cell count of the infected individuals, screened individuals are divided into two groups, IW(t) and IT(t), where IW(t) represents those who tested positive for HIV but with a CD4 cell count above the threshold, therefore not legible to enrol in ART and IT(t) represents those individuals who tested positive for HIV and are legible to enrol into ART. A(t), represent HIV infected individuals who will progress to the AIDS stage. Thus,
N(t)=S(t)+I(t)+IW(t)+IT(t)+A(t). |
We assume that susceptible individuals are recruited, as they become sexually active, at a rate Λ. Susceptible individuals are infected at a rate λ, whose full description is given with system (2.1). Infected individuals in class I can either develop AIDS or get into treatment upon testing if their CD 4 cell count is below a set threshold or they wait till their CD4 count level decreases to the required threshold. A proportion ϵ of those tested at a rate θ will have a CD4 count level above the threshold while the remaining proportion will be treated upon testing. Individuals in IW will progress to the class IT after a decrease in their CD4 cell count meets the required CD4 cell count level at a rate γ. All individuals with HIV, i.e those in I, IW(t) and IT(t) will progress to the AIDS class A(t), at rates ρ1, ρ2 and ρ3 respectively. Individuals in the AIDS class are assumed to transmit infection and they also die due to the disease at a rate δ. Individuals in each class are assumed to die naturally at a rate μ. The forces of infection are defined by λ=(λ1,λ2,λ3) for the three epochs. The explicit expressions are given in the description of each epoch. The dynamics of the second epoch are presented in Figure 1.
A summary of the model parameters is given in Table 1.
Parameter | Description | Units |
Λ | Recruitment rate | per time |
μ | Natural mortality rate | per time |
δ | Mortality rate due to HIV/AIDS | per time |
β | Transmission rate from Infected but unaware | per person per time |
ˆβ | Effective infectiousness per contact | per person per time |
η1 | Amplification factor of transmission rate from infected awaiting treatment | unitless |
η2 | Amplification factor of transmission rate from aware Infected awaiting treatment | unitless |
η3 | Amplification factor of transmission rate from Infected on treatment | unitless |
ρ1 | Progression to AIDS status by unaware infected | per time |
ρ2 | Progression to AIDS status by infected awaiting treatment | per time |
ρ3 | Progression to AIDS status by infected on treatment | per time |
θ | Testing rate | per time |
ϵ | Proportion not legible for treatment | unitless |
γ | Rate of enrolling into treatment after waiting to be legible | per time |
m | The fear of infection parameter that results in behavioural change | per person |
p | Level of protection by condoms | unitless |
n | The number of sexual partners | per person per time |
λi | Force of infection for each epoch for i=1,2,3. | per time |
The following system of ordinary differential equations thus governs the second epoch model,
˙X2=f(X2)=(Λ−(λ2+μ)Sλ2S−(μ+θ+ρ1)IϵθI−(μ+γ+ρ2)IW(1−ϵ)θI+γIW−(μ+ρ3)ITρ1I+ρ2IW+ρ3IT−(μ+δ)A), | (2.1) |
where X2=(S,I,IW,IT,A)T and the force of infection λ2(t) is given by
λ2=(β1+m(I+IW+IT+A))(I+η1A+η2IW+η3ITN)⋅ |
Here, β reflects not only the infectiousness per contact and the rate of sexual contact per unit time but also incorporates the impact of condom use as a primary prevention tool. Assuming that the level of protection by condoms is given by p, where 0≤p≤1. If p=0 then condoms do not offer any protection while p=1 implies perfect protection. Thus, (1−p) measures condom failure in preventing HIV transmission, see [22] for a detailed explanation. The number of sexual contacts in which condoms fail is represented by c=n(1−p) where n is the number of sexual partners per unit time. We thus define β=ˆβn(1−p), where ˆβ is the effective infectiousness per contact. This force of infection is reasonable for HIV since as the infection spreads, it is most likely that the remaining susceptible individuals become more cautious about their contacts and potential exposure to HIV, see also [23]. The force of infection will begin to decline as the number of HIV/AIDS cases and deaths due to the disease cases increases due to self-imposed fear [24]. Therefore m represents the fear of infection that will result in behavioural change, driven by the prevalence of the infection in the population. The parameter can be viewed as the measure in the reduction of the infection rate induced by the fear generated per infected case.
Given that there was no treatment in the period 1990 to 2001. We set the parameters related to treatment to zero in the grand model to obtain the first epoch model. The parameters related to treatment in the grand model are θ,γ,ρ2,ρ3 and setting them to zero results an SIA model for the first epoch.
Our model is thus governed by the following system of ordinary differential equations represented as follows,
˙X1=f(X1)=(Λ−(λ1+μ)Sλ1S−(μ+ρ1)Iρ1I−(μ+δ)A), | (2.2) |
where X1=(S,I,A)T and
λ1=(β1+m(I+A))(I+η1AN)⋅ |
Note that the mathematical analysis of system (2.2) is presented in [25].
In June 2016, the Botswana government launched the "Treat All" strategy, promoting universal health coverage and ensuring that all individuals who test positive for HIV enrol to treatment immediately regardless of their viral load or CD4 cell count. An assumption is made that all individuals in IW will move to IT since they already know their status and have no reason to wait. Setting the parameters, ϵ, ρ2 and γ, related to the non-legible to treatment compartment IW to zero in the grand model, we obtain the third epoch model. As a result, we have a four-compartment deterministic model of susceptible individuals (S), infected individuals who are unaware of their HIV infection status (I), infected individuals who have tested and immediately enrolled into treatment (IT) and infected who develop AIDS (A).
The third epoch model is thus governed by the following system of ordinary differential equations,
˙X3=f(X3)=(Λ−(λ3+μ)Sλ3S−(μ+θ+ρ1)IθI−(μ+ρ2)TTρ1I+ρ3TT−(μ+δ)A), | (2.3) |
where X3=(S,I,TT,A)T. The force of infection λ3(t) is given by
λ3=(β1+m(I+IT+A))(I+η1A+η3ITN)⋅ | (2.4) |
The parameters are as described in Table 1.
Given that the first and the third epochs can be obtained from the grand model, we consider the mathematical analysis of system (2.1). The mathematical analysis of the other two models can be drawn from the big model by setting relevant parameters to zero. We present the mathematical analysis of model (2.1) subject to the initial conditions:
S(0)=S0≥0,I(0)=I0≥0,IW(0)=IW0≥0,IT(0)=IT0≥0,A(0)=A0≥0,andN(0)=N0. | (3.1) |
It is important to note that the analysis results of the grand model captures those of the models of first and third epochs given that they are "sub-models" of the grand model.
Lemma 3.1. The model (2.1) with the initial conditions (3.1), has non-negative solutions and the solution of the system will remain positive for all t>0.
Proof. From the first equation of the model system (2.1), let λ=λ2, we have
dSdt=Λ−(λ2+μ)S≥−(λ2+μ)S. |
We thus have
S(t)≥S0exp[−∫t0(λ2+μ)dt]>0. |
From the second equation of the model system (2.1) we obtain
dIdt=λ2S−(μ+θ+ρ1)I≥−(μ+θ+ρ1)I, |
whose solution is given by
I(t)≥I0exp[−∫t0(μ+θ+ρ1)t]>0. |
In a similar manner we can demonstrate that IW(t)>0, IT(t)>0 and A(t)>0 for all t>0.
Lemma 3.2. The model (2.1) solutions are uniformly bounded in the set
Ω={(S(t),I(t),Iw(t),IT(t),A(t))∈R5+ | 0≤N≤Λμ}. |
Proof. All parameters and initial conditions in the system (2.1) are assumed to be positive.
The equations of system (2.1) gives
dNdt=Λ−μN−δA,≤Λ−μN. |
The solution of the differential inequality is given by
N(t)≤Λμ+(N0−Λμ)e−μt⋅ |
If N0>Λμ, the solutions of N(t) decreases to Λμ and if N0<Λμ then N(t)→Λμ as t→∞. The region Ω is thus positively invariant and solutions are bounded. This means that every solution of (2.1) with initial conditions in Ω remain in Ω for all t≥0. The model is thus epidemiologically and mathematically well-posed in the region
Ω={(S(t),I(t),Iw(t),IT(t),A(t))∈R5+ | 0≤N≤Λμ}. |
The steady states of the model are obtained by setting the equation of system (2.1) to zero such that
˙X2=(Λ−(λ2+μ)Sλ2S−σ1IϵθI−σ2IW(1−ϵ)θI+γIW−σ3ITρ1I+ρ2IW+ρ3IT−σ4A)=0 | (3.2) |
where σ1=θ+ρ1+μ, σ2=γ+ρ2+μ, σ3=ρ3+μ and σ4=δ+μ.
The steady state variables expressed in terms of I∗ are given by,
I∗W=ψ1I∗,I∗T=ψ2I∗,A∗=ψ3I∗ | (3.3) |
where
ψ1=ϵθσ2, ψ2=(1−ϵ)θ+γψ1σ3andψ3=ρ1+ρ2ψ1+ρ3ψ2σ4⋅ |
We also have
λ∗2=ϕ2I∗(1+mϕ1I∗)N∗, | (3.4) |
where ϕ1=1+ψ1+ψ2+ψ3 and ϕ2=β(1+η1ψ3+η2ψ1+η3ψ2).
Substituting (3.4) into the second equation (3.2) and solving for I∗ we obtain
I∗=0andS∗N∗=σ1(1+mϕ1I∗)ϕ2⋅ | (3.5) |
If I∗=0 then we have I∗W=I∗T=A∗0=0 and S∗=Λμ. This corresponds to the disease-free state. The model thus has a disease-free equilibrium (DFE) given by
D0=(Λμ,0,0,0,0). |
The reproduction number, R0, gives a threshold condition to the stability of the disease free disease-free equilibrium [23]. We compute the reproduction number of the grand model system 2.1 using the next generation method. Following [26], and adopting the matrix notation, the matrices for new infection terms, F, and the transfer terms, V, at the DFE are given by
F=[ββη2βη3βη1000000000000] and V=[σ1000−ϵθσ200−(1−ϵ)θ−γσ30−ρ1−ρ2−ρ3σ4]. |
FV−1 is the next generation matrix. The spectral radius, of the matrix FV−1 is the reproduction number of the model. Thus
R0=R1+R2+R3+R4, | (3.6) |
where
R1=βσ1,R2=βη2ϵθσ1σ2,R3=βη3(ϵθγ+(1−ϵ)θσ2)σ1σ2σ3,R4=βη1(ρ1σ2σ3+ϵθρ2σ3+ϵθγρ3+(1−ϵ)θρ3σ2)σ1σ2σ3σ4. |
R0 is the sum of four terms representing the contribution of infection by classes, I,IW,IT and A. We note that the R0 for the first epoch model can be found by setting the parameters, θ,γ,ρ2,ρ3, in Eq (3.6) to zero. The same applies to the reproduction number of the third epoch, which is obtained by setting the parameters ϵ,γ and ρ2 in Eq (3.6) to zero. It is defined as the expected number of secondary cases produced by a typical infected individual during its entire period of infectiousness in a completely susceptible population and is mathematically defined as the dominant eigenvalue of a positive linear operator.
Following Theorem 2 in [26], we state the stability of the disease free equilibrium, D0, as follows:
Theorem 3.2.1. The DFE, D0, is locally asymptotically stable if R0<Rc0<1 and unstable otherwise.
Note that the expression for Rc0 is defined in the next subsection.
The total population at the steady state is given by
N∗=S∗+ϕ1I∗⋅ | (3.7) |
Substituting (3.7) into Eq (3.5) yields
S∗=−σ1ϕ1I∗(1+mϕ1I∗)σ1+mσ1ϕ1I∗−ϕ2⋅ | (3.8) |
Substituting Eqs (3.4), (3.7) and (3.8) into the first equation of the system (3.2) we obtain the quadratic polynomial in I∗ given by
ν2I∗2+ν1I∗+ν0=0, | (3.9) |
with
ν2=mσ1ϕ1(σ1−μϕ1),ν1=σ21−σ1ϕ2−σ1ϕ1(mΛ+μ),ν0=Λσ1(1−R0). |
Here, R0 is the model reproduction number given by the simplified expression
R0=ϕ2σ1=R1+R2+R3+R4, |
where R1,R2,R3 and R4 are define in (3.6).
The existence of the endemic equilibrium (EE) is subjected to the roots of the quadratic equation (3.9) being positive, we thus have
I∗=−ν1±√ν21−4ν2ν02ν2. |
σ1−μϕ1=μϵθρ2δ+θρ3δ[γ+ρ2+μ(1−ϵ)]+ρ1δ(μ+γ+ρ2)(μ+ρ3)(δ+μ)(μ+γ+ρ2)(μ+ρ3)>0. |
The coefficient of I∗ in Eq (3.9) can either be positive or negative. Also, ν0 can be either positive or negative depending on whether R0 is less or greater than unity. The results are presented in Table 2.
ν2>0 | |||
ν1>0 | ν1<0 | ||
ν0>0 (R0<1) | ν0<0 (R0>1) | ν0>0 (R0<1) | ν0<0 (R0>1) |
no positive roots | 1 positive root | 2 positive roots | 1 positive root |
We note from Table 2 that for the existence and uniqueness of the endemic equilibrium, ν1 and ν0 must be negative as ν2 is always positive for R0>1. Thus, we have the following result.
Theorem 3.2.2. The model has:
i. a unique endemic equilibrium if ν0<0,R0>1,
ii. a unique endemic equilibrium if ν21−4ν2ν0=0,
iii. two endemic equilibria if ν1<0 and ν0>0,R0<1,
iv. no endemic equilibrium otherwise.
From case (i) of Theorem 3.2.2, there exist a unique endemic equilibrium whenever R0>1. Furthermore, case (iii) indicates the possibility of backward bifurcation. Backward bifurcation in epidemic models implies a co-existence of a stable DFE with a stable EE when the associated reproduction number is less than unity, see also [27]. The epidemiological implications of this phenomenon is that the requirement R0<1 is not sufficient for the disease elimination effort rather the effort is described by the value of the critical parameter at the turning point [28]. We set the discriminant of Eq (3.9) to zero and solve for the critical value of R0, denoted RC0 so that
Rc0=1−ν214Λσ1ν2. |
The backward bifurcation is depicted in Figure 2 for the parameter values given in the caption. The backward bifurcation shows a locally stable DFE and an unstable and stable EE for R0<1. To effectively control the disease, a globally stable DFE is desired for R0<1. In this case, the DFE is globally stable below the threshold Rc0≈0.6 making it challenging to eliminate the disease because it is far from unity for the chosen parameter values. Backward bifurcation has been observed in models for disease dynamics such as those for behavioural responses to perceived risk, treatment, in-host dynamics, the imperfect vaccine, public health education campaigns and reinfection in the transmission dynamics [27].
Theorem 3.2.3.
The endemic equilibrium point D1 of the model system equations is globally asymptotically stable if R0>1.
The proof of the theorem in given in Appendix A.
This section estimates the model's unknown parameters by calibration or curve fitting. Our data is extracted from the UNAIDS website (https://aidsinfo.unaids.org/). We consider data on the number of adults (aged 15 year and above, living with HIV in Botswana from 1990 to 2019. We first attempt to fit each of the three models, described by systems (2.1), (2.2) and (2.3), to the prevalence data of Botswana for the entire modelling period. A Matlab built-in function ode45 is used to solve the systems and the fminsearch algorithm is used for the minimisation routine. The data points are to be compared with simulation results by minimizing the sum of square difference of the models' prevalence P(t), (i.e the number of all HIV and AIDS-infected people over the modelling time) and the HIV/AIDS prevalence for the adults population data (ti,Xi) given as
SSE=30∑i=1(Xi−P(ti))2⋅ | (4.1) |
For all the models, we use the pre-estimated and parameters ranges in Table 4. Figure 3 shows the model fits to data from UNAIDS website for Botswana from 1990–2019 for the three epoch models.
Year | 1990 | 1991 | 1992 | 1993 | 1994 | 1995 | 1996 | 1997 | 1998 | 1999 |
ALWHIV | 40000 | 59000 | 82000 | 110000 | 140000 | 170000 | 190000 | 220000 | 230000 | 250000 |
Year | 2000 | 2001 | 2002 | 2003 | 2004 | 2005 | 2006 | 2007 | 2008 | 2009 |
ALWHIV | 260000 | 260000 | 270000 | 270000 | 270000 | 280000 | 290000 | 290000 | 300000 | 310000 |
Year | 2010 | 2011 | 2012 | 2013 | 2014 | 2015 | 2016 | 2017 | 2018 | 2019 |
ALWHIV | 320000 | 320000 | 330000 | 340000 | 340000 | 350000 | 360000 | 360000 | 370000 | 370000 |
Parameter | Range | Nominal Value |
Λ | μ×S0 | |
μ | 0.01818 | |
δ | (0.1, 0.4) | 0.15477 |
β | (0, 1) | 0.7 |
η1 | (1, 3) | 2 |
η2 | (1, 2) | 1.0002 |
η3 | (0, 1) | 0.9998 |
ρ1 | (0.0667, 0.125) | 0.097980 |
ρ2 | (0.0667, 1) | 0.16998 |
ρ3 | (0.01, 0.0667) | 0.01 |
θ | (0, 1) | 0.99997 |
ϵ | (0, 1) | 0.0010232 |
γ | (0, 1) | 0.44029 |
m | (0, 1) | 0.015 |
Figure 3(a) shows the fitting the epoch 1 model (SIA) to the data using the inital conditions S(0)=722000, I(0)=35000 and A(0)=5000, the SSE is equal to 8.1×103. Figure 3(b) shows the fitting of the grand model (SIIWITA) to the data with inital conditions S(0)=722000, I(0)=35000, IW=0, IT=0 and A(0)=5000. The SSE equal to 3.5×103. Figure 3(c) shows the fitting of epoch 3 model (SIITA) to the data with inital conditions S(0)=722000, I(0)=35000, IT=0 and A(0)=5000 to data. The SSE in this case is equal to 7.01×103. The best-fit curves for all the three models do not simulate the data ideally, as shown by Figure 3.
We note that UNAIDS collected the data for almost three decades for various responses to HIV/AIDS. In order to achieve a simulated best fit to a series of data points that captures the change in HIV treatment policy in Botswana for almost three decades, we propose to calibrate the second epoch model and allow some parameters to vary over the modelling period. We vary these parameters based solely on the implementation strategy of ART in Botswana.The second epoch model fit the data to Botswana's treatment policy for the period 2002–2016. The implementation of antiretroviral therapy in Botswana started in 2002. We, therefore, render all the parameters related to treatment (ϵ,θ,γ,η2,η3,ρ2 and ρ3) to zero for the period before 2002 because there was no HIV treatment. Botswana launched a "Treat All" strategy in 2016, that is anyone who tests positive for HIV enrols on treatment immediately. We, therefore, render all parameters related to waiting for eligibility to treatment based on CD4 count (ϵ,γ,η2 and ρ2) to zero.
Below we show the implementation of shutting down parameters of the grandmodel to obtain the models of epoch 1 and 3 using Matlab;
![]() |
We thus have a piecewise system,
˙X={f(X1),1990≤t<2002,f(X2),2002≤t<2016,f(X3),2016≤t≤2019. | (4.2) |
Although many mathematical modelers have fitted models to data see for instance [29], they have ignored the fact that factors influencing disease transmission change during the modelling period. We aim to incorporate changes in policy in the fitting to data. We estimate the model parameters guided by the Botswana statistics, literature and curve fitting algorithm. The natural death rate, μ, is taken to be the reciprocal of the average life expectancy of Botswana [30]. The recruitment rate, Λ, is estimated by considering that our initial modelling time is 1990. The World Population Prospects (www.population.un.org) estimates the total population of Botswana in the year 1990 to be 1 382 000 of which the adults (aged 15+) accounted for 55.2%. Therefore the total adult population, N=762864. The UNAIDS estimated the total number of adults living with HIV to be 40 000 in year 1990. Thus we have I+A=40000 since the data does not distinguish between infectious and AIDS staged individuals. We thus estimate the initial conditions as follows;
∙ N = 762 864, adult population found in the year 1990.
∙ I(0)+A(0) = 40 000, HIV adults prevalence in 1990.
∙ I(0) = 35 000, assumed number of infected staged individuals.
∙ A(0) = 5000, assumed number of AIDS patients.
∙ S(0)=N−I(0)−IT(0)−ID(0)−A(0) = 722 864.
Figure 4 shows the fit of all three epoch models to the data of adults living with HIV/AIDS for Botswana. It shown from Figure 4(a) that the model fits very well to the data and the SSE = 9.07×102. The estimated parameters from the fitting are given in Table 4. Figure 4(b) shows a steady increase in the number of infected adults by 2030, provided the HIV/AIDS policy for Botswana does not change.
Figure 5 shows the time series plots of all state variables of the model. Figure 5(a) shows infected increasing from 1990 and starts falling after 2002 before stabilizing. From 1990 to before 2002, the number of individuals not legible for treatment is zero. After 2002 the number of individuals increased starts falling after 2007 and stabilize from 2007 as shown in Figure 5(b). Individuals on treatment kick off from 2002, shown in Figure 5(c), and have been growing since. Figure 5(d) shows an increase of individuals on the AIDS stage, from 1990 to around 2002 before decreasing.
Figure 6 shows the projections of all state variables of the model provided the policy does not change. Figure 6(a), shows that susceptible individuals would continue to decrease steadily. Figure 5(b) shows that the infected population also continue to decrease, infected individuals on treatment would continue to increase every year as shown in Figure 6(c). The infected progressing to AIDS stage would steadily decrease as seen in Figure 6(d).
The estimation of parameters through curve fitting is subjected to variation since the parameters are selected from a range. Sensitivity analysis aims to quantify the influence of parameters variation in the model output. We perform global sensitivity analysis to examine the sensitivity of R0 to variation in parameters using the Latin Hypercube Sampling (LHS) and the partial rank correlation coefficients (PRCCs). LHS is a statistical sampling method that allows for an efficient analysis of parameter variation across simultaneous uncertainty ranges in each parameter [31]. PRCCs illustrate the degree of the effect that each parameter has on the outcome. Fixing μ=1/55 while taking ranges of the rest of the other parameters from Table 4, we compute the PRCCs of the parameters with respect to the R0. The PRCCs for each parameter against R0 obtained after 1000 LHS samples is presented in Figure 7. Parameters that have high absolute values of PRCCs are the most influential to the reproduction number. The sign of the PRCCS gives the qualitative relationship between the input parameters and the output variable, R0. The positive PRCCs imply that as the parameter value increases, the reproduction number will also increase and vice versa. On the other hand, negative PRCCs imply that as the parameter value increases, the R0 will decrease and vice versa. The parameters β,η1,η2,η3,ρ1 and ϵ have the potential to cause a burden to the disease if not mitigated. Whilst the rest of the parameters, when enhanced, can help ease the disease burden.
Figure 7(a) shows that the most influential parameters of the model are the transmission rate, β and the AIDS induced deaths, δ. The box plot Figure 7(b) shows the five number distribution of obtained values of R0, the lower quartile, the median and the upper quartile being about 2.19, 4.29 and 6.53, respectively. The best fit parameter values yield an R0=6.8. The median value of R0 in African countries is 4.5, the 90th percentile value of R0 is 6.3, and the maximum being 9.5 [32]. The best fit parameter values yield an R0=6.8 for Botswana, which is within the estimated calculations given in [32]. We note that although some parameters may have small magnitudes of PRCCs, they may still make significant changes in the disease spread.
To investigate the effects of Botswana's HIV/AIDS policies, we vary the parameters linked to behavioural change and treatment. The first epoch policy was mainly focused on behavioural change. However, the second and third epochs policies included treatment at different rates. We, therefore, vary the parameters m and θ to evaluate their effects on the dynamics of HIV/AIDS for Botswana.
Figure 8 shows the projected change in the number of HIV/AIDS infected adults. Increasing both the testing and treatment rate and behavioural change. A decrease in HIV/AIDS infected adults is observed as we keep doubling the testing and therapy efforts and behavioural change rate.
Figure 9(a) shows that increasing θ only, decreases the number of infected adults just from 2002 when treatment was introduced. Figure 9(b) shows that increasing both m and θ would decrease the infected cases from the beginning of the modelling period. The shaded regions show the number of cases averted by the increase in treatment and behavioral change. More cases would be averted by increasing both m and θ.
Figure 10(a) shows that increasing θ only, increases the number of infected adults enrolled on treatment and the shaded area shows the number of individuals added into ART. Interestingly, Figure 10(b) shows that increasing both m and θ would decrease the number of infected adults enrolled on treatment; this is as a result of more averted infected cases shown by Figure 9(b). The shaded regions show the number of treated cases averted by increasing both m and θ interventions. Therefore, this decrease can be interpreted as a cost-saving measure for the country.
Figure 11(a) shows that increasing θ only decreases the number of infected adults progressing to the aids stage just from 2002 when treatment was introduced. The shaded regions show the number of averted AIDS cases. On the other hand, Figure 10(b) shows that increasing both m and θ decrease the number of infected adults progressing to the aids stage from the beginning of the modelling period. This result shows that more AIDS cases are averted by increasing both behavioral change and treatment.
In this paper, a piecewise system of three models is proposed and analysed to track Botswana's HIV/AIDS policy changes. Introducing policy change in mathematical models is critical for a holistic view of an epidemic influenced by policy dynamics. The structure of the models become dynamic to capture the changes in policy and response to the disease. In this study, we looked at how the treatment policies of Botswana evolved. As a result, this led to the development of models over three epochs. In the 1990s, there was no treatment, and many of the models only involved those at risk, the infected and those who would have developed AIDS. With the advent of treatment that depended on the CD4 cell count, the models had to adapt to capture the rollout of ART programmes. Many researchers developed several mathematical models, but the model presented in this paper represents the perfect summary of the general structure of models that capture how the response to the epidemic should be modelled. The treatment policies further developed into the "Treat All" strategy, which assumed that treatment had the potential to reduce the viral load to undetectable levels, resulting in reduced transmission. We also present the model that captures this policy.
Of the three models developed in this paper, the model of the second epoch captures the dynamics of the first and third epochs if some chosen parameters values are set to zero. The analysis of the grand model thus captures the study of the other two models. We, therefore, focused on the analysis of the grand model. The steady states were determined and analysed in terms of the model reproduction number R0. The model is fitted to data from UNAIDS over three decades. It is clear that the use of a piece-wise models, captures the ideal scenario in which the disease transmission process is dynamic, changing as the policies change.
Many of the mathematical models presented with data fitting ignore aspects of changes in policies over time. They thus consider the parameter values that are averaged over the entire modelling period. It is however important to track the changes in the policies over time that will result in changes in the parameter values over time. We fitted the three models and showed that the fitting in which policy changes are not taken into consideration do not perfectly fit to the data. To address the changes in the parameters over time, we then fitted the grand model to prevalence data from UNAIDS on Botswana. Some of the parameters were considered as continuous piecewise functions to generate a perfect fit, as shown in Figure 4. Sensitivity analysis is performed to determine how each of the parameters impacts Botswana's HIV/AIDS epidemic.
The early policies were based on campaigns to target behavioural change, and the later policies were focused on the uptake of testing and treatment. However, the simulations results showed that a combination of policies leads to effective management of the disease. Therefore, the policymakers need to evaluate the policies and use the information to update the new policies adding to the latest research findings and recommendations. Also, it is imperative to ensure that when new policies are implemented, the earlier ones are not left behind but updated. Earlier policies may be updated to meet the latest trends. For example, the testing and treatment enrollment campaigns to reach the 95-95-95 target should also carry a message of prevention from contracting the disease targeting behavioural change as a package.
The models presented here capture the most straightforward scenarios in each epoch. It is thus essential to note that further refinements can be done by including additional compartments to capture the challenges of managing HIV/AIDS in Botswana. In particular, aspects of drop-out from treatment programmes, defaulting, delays in accessing health care services, and excluding the migrant population from accessing free HIV/AIDS treatment before September 2019 should be considered to give a more realistic picture of how policies have impacted the epidemics in Botswana. Our study, however, provides a significant impetus in the modelling of HIV/AIDS dynamics in the presence of policy changes.
Proof. We propose a Lyapunov function of the form
V=(S−S∗−S∗lnSS∗)+a2(I−I∗−I∗lnII∗)+b2(IW−I∗W−I∗WlnIWI∗W)+c2(IT−I∗T−I∗TlnITI∗T)+d2(A−A∗−A∗lnAA∗), |
where a2, b2, c2 and d2 are positive constants to be determined. Note that V=0 when (S1,I1,IW1,IT1,A1)=(S∗,I∗,I∗W,I∗T,A∗) otherwise V>0. We now show that the time derivative of V is negative. Therefore, the time derivative of the Lyapunov function is given by
˙V=(1−S∗S)˙S+a2(1−I∗I)˙I+b2(1−I∗WIW)˙IW+c2(1−I∗TIT)˙IT+d2(1−A∗A)˙A,=(1−S∗S)(Λ−λS−μS)+a2(1−I∗I)(λS−σ1I)+b2(1−I∗WIW)(ϵθI−σ2IW)+c2(1−I∗TIT)((1−ϵ)θI+γIW−σ3IT)+d2(1−A∗A)(ρ1I+ρ2IW+ρ3IT−σ4A)⋅ |
From the system (3.2), we also have
Λ=(λ∗+μ)S∗,σ1=λ∗S∗I∗,σ2=ϵθI∗I∗W,σ3=(1−ϵ)θI∗+γI∗WI∗T,σ4=ρ1I∗+ρ2I∗W+ρ3I∗TA∗. | (A.1) |
We express the derivative of the Lyapunov function as the sum of its components, ˙V=˙V1+˙V2+˙V3+˙V4, and evaluating the components separately, we obtain
˙V1≤μS∗(1−S∗S)(1−SS∗)+β(1−S∗S)[I∗S∗(1−ISI∗S∗)+η1A∗S∗(1−ASA∗S∗)+η2I∗WS∗(1−IWSI∗WS∗)+η3I∗TS∗(1−ITSI∗TS∗)].˙V2≤a2β(1−I∗I)[I∗S∗(ISI∗S∗−II∗)+η1A∗S∗(ASA∗S∗−II∗)+η2I∗WS∗(IWSI∗WS∗−II∗)+η3I∗TS∗(ITSI∗TS∗−II∗)].˙V3=b2(1−I∗WIW)[ϵθI∗(II∗−IWI∗W)].˙V4=c2(1−I∗TIT)[(1−ϵ)θI∗(II∗−ITI∗T)+γI∗W(IWI∗W−ITI∗T)].˙V5=d2(1−A∗A)[ρ1I∗(II∗−AA∗)+ρ2I∗W(IWI∗W−AA∗)+ρ3I∗T(ITI∗T−AA∗)]. |
Letting
SS∗=E, II∗=F, IWI∗W=G, ITI∗T=H, AA∗=J, | (A.2) |
the derivative of V them reduces to
˙V≤−μS∗E(E−1)2+Q(E,F,G,H,J), |
where
Q(E,F,G,H,J)=βI∗S∗+βη1A∗S∗+βη2I∗WS∗+βη3I∗TS∗+a2βI∗S∗+a2βη1A∗S∗+a2βη2I∗WS∗+a2βη3I∗TS∗+b2ϵθI∗+c2γI∗W+c2(1−ϵ)θI∗+d2ρ1I∗+d2ρ2I∗W+d2ρ3I∗T+E(−a2βI∗S∗)+F(βI∗S∗−a2βI∗S∗−a2βη1A∗S∗−a2βη2I∗WS∗−a2βη3I∗TS∗+b2ϵθI∗+c2(1−ϵ)θI∗+d2ρ1I∗)+G(βη2I∗WS∗−b2ϵθI∗+c2γI∗W+d2ρ2I∗W)+H(βη3I∗TS∗−c2(1−ϵ)θI∗−c2γI∗W+d2ρ3I∗T)+J(βη1A∗S∗−d2ρ1I∗−d2ρ2I∗W−d2ρ3I∗T)+1E(−βI∗S∗−βη1A∗S∗−βη2I∗WS∗−βη3I∗TS∗)+EF(−βS∗I∗+a2βS∗I∗)+EG(−βη2I∗WS∗+a2βη2I∗WS∗)+EH(−βη3I∗TS∗+a2βη3I∗TS∗)+EJ(−βη1A∗S∗+a2βη1A∗S∗)++EHF(−aβη3I∗TS∗)+FH(−c2(1−ϵ)θI∗)+GH(−cγI∗W)+FG(−b2ϵθI∗)+EJF(−a2βη1A∗S∗)+EGF(−a2βη2I∗WS∗)+FJ(−d2ρI∗)+GJ(−dρ2I∗W)+HJ(−dρ3I∗T)⋅ | (A.3) |
By setting
a2=1,b2=βS∗[(γη3I∗TI∗w+η2 I∗W)(I∗ρ1+I∗Wρ2+I∗Tρ3)+η1A∗(I∗Wρ2+γI∗TI∗wρ3)]ϵθI∗(I∗ρ1+I∗Wρ2+I∗Tρ3),c2=βη3I∗TS∗(1−ϵ)θI∗+γI∗w+βη1A∗S∗ρ3I∗T((1−ϵ)θI∗+γI∗w)(ρ1I∗+ρ2I∗W+ρ3I∗T),d2=βη1A∗S∗ρ1I∗+ρ2I∗W+ρ3I∗T, |
the coefficients of F,G,H,J,EF,EG,EH and EJ become zero and
Q(E,F,G,H,J)=βI∗S∗(2−1E−E)+βη1A∗S∗(2−EJF−1E)+βη2I∗WS∗(2−EGF−1E)+βη3I∗TS∗(2−EHF−1E)+b2ϵθI∗(1−FG)+c2(1−ϵ)θI∗(1−FH)+c2γI∗w(1−GH)+d2ρ1I∗(1−FJ)+d2ρ2I∗W(1−GJ)+d2ρ3I∗T(1−HJ). | (A.4) |
We note that (2−1E−E) is less than or equal to zero by the arithmetic-mean-geometric-mean inequality, with equality if and only if E=1. Also, the following expressions;
(2−EJF−1E),(2−EGF−1E)and(2−EHF−1E) |
are less than or equal to zero by the arithmetic-mean-geometric-mean inequality, with equality if and only if E=1 and F=G=J=H. After some tedious algebraic manipulations of replacing the constants b2,c2 and d2 in Eq (6.4), we draw similar conclusions. We thus have
˙V=−μS∗E(E−1)2+Q(E,F,G,H,J)≤0and˙V=0 |
if and only if S=S∗ and II∗=IWI∗W=ITI∗T=AA∗. Since S must remain constant at S∗ then ˙S=0. Therefore, II∗=IWI∗W=ITI∗T=AA∗=1. Thus, the invariant set in Ω is the singleton (S∗,I∗,I∗W,I∗T,A∗). Thus the endemic equilibrium D1 is globally asymptotically stable if R0>1 by the LaSalle invariance principle [33].
The authors declare there is no conflict of interest.
[1] | Botswana National Policy on HIV/AIDS, 2012, available from: https://www.undp.org/content/dam/botswana/docs/HIVAIDS/Botswana%20HIV%20and%20AIDS%20Policy%202012.pdf (accessed 15 August 2021) |
[2] | World Health Organization, Health Policy, available from: https://www.who.int/alliance-hpsr/alliancehpsr_reader.pdf (accessed 22 March 2011) |
[3] |
L. F. Johnson, P. J. White, A review of mathematical models of HIV/AIDS interventions and their implications for policy, Sex. Transm. Infect., 87 (2011), 629–634. http://dx.doi.org/10.1136/sti.2010.045500 doi: 10.1136/sti.2010.045500
![]() |
[4] |
K. Nah, H. Nishiura, N. Tsuschiya, X. Sun, A. Imamura, Test-and-treat approach to HIV/AIDS: a primer for mathematical modeling, Theor. Biol. Med. Model., 14 (2017), 16. https://doi.org/10.1186/s12976-017-0062-9 doi: 10.1186/s12976-017-0062-9
![]() |
[5] |
R. M. Granich, C. F. Gilks, C. Dye, K. M. De Cock, B. G. Williams, Universal voluntary HIV testing with immediate antiretroviral therapy as a strategy for elimination of HIV transmission: a mathematical model, Lancet, 373 (2009), 48–57. https://doi.org/10.1016/s0140-6736(08)61697-9 doi: 10.1016/s0140-6736(08)61697-9
![]() |
[6] |
A. M. Foss, P. T. Vickerman, M. Alary, C. H. Watts, How much could a microbicide's sexually transmitted infection efficacy contribute to reducing HIV risk and the level of condom use needed to lower risk? Model estimates, Sex. Transm. Dis., 85 (2009), 276–282. https://doi.org/10.1136/sti.2008.032458 doi: 10.1136/sti.2008.032458
![]() |
[7] |
L. Zhang, P. Peng, Y. Wu, X. Ma, N. N. Soe, X. Huang, et al., Modelling the epidemiological impact and cost-effectiveness of PrEP for HIV transmission in MSM in China, AIDS Behav., 23 (2019), 523–533. https://doi.org/10.1007/s10461-018-2205-3 doi: 10.1007/s10461-018-2205-3
![]() |
[8] |
I. Cremin, L. McKinnon, J. Kimani, P. Cherutich, G. Gakii, F. Muriuki, et al., PrEP for key populations in combination HIV prevention in Nairobi: a mathematical modelling study, Lancet HIV, 4 (2017), e214–e222. https://doi.org/10.1016/s2352-3018(17)30021-8 doi: 10.1016/s2352-3018(17)30021-8
![]() |
[9] |
C. Pinto, A. R. Carvalho, D. Baleanu, H. M. Srivastava, Efficacy of the post-exposure prophylaxis and of the HIV latent reservoir in HIV infection, Mathematics, 7 (2019), 515. https://doi.org/10.3390/math7060515 doi: 10.3390/math7060515
![]() |
[10] |
D. E. Gordon, L. R. Ghazaryan, J. Maslak, B. J. Anderson, K. S. Brousseau, A. F. Carrascal, L. C. Smith, Projections of diagnosed HIV infection in children and adolescents in New York State, Paediatr. Perinat. Ep., 26 (2012), 131–139. https://doi.org/10.1111/j.1365-3016.2011.01243.x doi: 10.1111/j.1365-3016.2011.01243.x
![]() |
[11] |
M. Ronoh, F. Chirove, J. Wairimu, W. Ogana, Evidence-based modeling of combination control on Kenyan youth HIV/AIDS dynamics, PloS One, 17 (2020), e0242491. https://doi.org/10.1371/journal.pone.0242491 doi: 10.1371/journal.pone.0242491
![]() |
[12] |
J. A. Smith, S. J. Anderson, K. L. Harris, J. B. McGillen, E. Lee, G. P. Garnett, et al., Maximising HIV prevention by balancing the opportunities of today with the promises of tomorrow: a modelling study, Lancet HIV, 3 (2016), e289–e296. https://doi.org/10.1016/s2352-3018(16)30036-4 doi: 10.1016/s2352-3018(16)30036-4
![]() |
[13] |
N. J. Nagelkerke, S. Moses, S. J. de Vlas, R. C. Bailey, Modelling the public health impact of male circumcision for HIV prevention in high prevalence areas in Africa, BMC Infect. Dis., 7 (2007), 1–5. https://doi.org/10.1186/1471-2334-7-16 doi: 10.1186/1471-2334-7-16
![]() |
[14] | P. A. Naik, M. Ghoreishi, J. Zu, Approximate solution of a nonlinear fractional-Order HIV model using momotopy analysis method, Int. J. Numer. Anal. Model., 19 (2022), 52–84. |
[15] |
P. A. Naik, K. M. Owolabi, M. Yavuz, J. Zu, Chaotic dynamics of a fractional order HIV-1 model involving AIDS-related cancer cells, Chaos Soliton Fract., 140 (2020), 110272. https://doi.org/10.1016/j.chaos.2020.110272 doi: 10.1016/j.chaos.2020.110272
![]() |
[16] |
P. A. Naik, M. Yavuz, J. Zu, The role of prostitution on HIV transmission with memory: A modeling approach, Alex. Eng. J., 59 (2020), 2513–2531. https://doi.org/10.1016/j.aej.2020.04.016 doi: 10.1016/j.aej.2020.04.016
![]() |
[17] |
M. Farahani, A. Vable, R. Lebelonyane, K. Seipone, M. Anderson, A. Avalos, et al. Outcomes of the Botswana national HIV/AIDS treatment programme from 2002 to 2010: a longitudinal analysis, Lancet Glob. Health, 2 (2014), e44–e50. https://doi.org/10.1016/s2214-109x(13)70149-9 doi: 10.1016/s2214-109x(13)70149-9
![]() |
[18] |
F. Nyabadza, Z. Mukandavire, Modelling HIV/AIDS in the presence of an HIV testing and screening campaign, J. Theor. Biol., 280 (2011), 167–179. https://doi.org/10.1016/j.jtbi.2011.04.021 doi: 10.1016/j.jtbi.2011.04.021
![]() |
[19] |
S. M. Rahman, N. K. Vaidya, X. Zou, Impact of early treatment programs on HIV epidemics: An immunity-based mathematical model, Math. Biosci., 280 (2016), 38–49. https://doi.org/10.1016/j.mbs.2016.07.009 doi: 10.1016/j.mbs.2016.07.009
![]() |
[20] |
J. M. Hyman, J. Li, The reproductive number for an HIV model with differential infectivity and staged progression, Linear Algebra Appl., 398 (2005), 101–116. https://doi.org/10.1016/j.laa.2004.07.017 doi: 10.1016/j.laa.2004.07.017
![]() |
[21] |
C. C. McCluskey, A model of HIV/AIDS with staged progression and amelioration, Math. Biosci., 181 (2003), 1–16. https://doi.org/10.1016/s0025-5564(02)00149-9 doi: 10.1016/s0025-5564(02)00149-9
![]() |
[22] |
F. Nyabadza, Z. Mukandavire, S. D. Hove-Musekwa, Modelling the HIV/AIDS epidemic trends in South Africa: Insights from a simple mathematical model, Nonlinear Anal. Real World Appl., 12 (2011), 2091–2104. https://doi.org/10.1016/j.nonrwa.2010.12.024 doi: 10.1016/j.nonrwa.2010.12.024
![]() |
[23] | M. Martcheva, An introduction to Mathematical Epidemiology, Vol. 61. Springer, New York, 2015. https://doi.org/10.1007/978-1-4899-7612-3 |
[24] | I. Ghosh, P. K. Tiwari, S. Samanta, I. M. Elmojtaba, N. Al-Salti, J. Chattopadhyay, A simple SI-type model for HIV/AIDS with media and self-imposed psychological fear, Math. Biosci., 306 (2018), 160–169. |
[25] |
F. Nyabadza, C. Chiyaka, Z. Mukandavire, S. D. Hove-Musekwa, Analysis of an HIV/AIDS model with public-health information campaigns and individual withdrawal, J. Biol. Syst., 18 (2010), 357–375. https://doi.org/10.1142/S0218339010003329 doi: 10.1142/S0218339010003329
![]() |
[26] |
P. van den Driessche, J. Watmough, Reproduction numbers and sub-threshold endemic equilibrium for compartmental models of disease transmission, Math. Biosci., 180 (2002), 29–48. https://doi.org/10.1016/S0025-5564(02)00108-6 doi: 10.1016/S0025-5564(02)00108-6
![]() |
[27] |
A. B. Gumel, Causes of backward bifurcations in some epidemiological models, J. Math. Anal. Appl., 395 (2012), 355–365. https://doi.org/10.1016/j.jmaa.2012.04.077 doi: 10.1016/j.jmaa.2012.04.077
![]() |
[28] |
K. P. Hadeler, P. Van den Driessche, Backward bifurcation in epidemic control, Math. Biosci., 146 (1997), 15–35. https://doi.org/10.1016/s0025-5564(97)00027-8 doi: 10.1016/s0025-5564(97)00027-8
![]() |
[29] |
T. Kaisara, F. Nyabadza, On the future of HIV/AIDS in Botswana in the presence of drop-outs: Insights from a simple mathematical model, Int. J. Appl. Computat. Math., 6 (2020), 1–20. https://doi.org/10.1007/s40819-020-00843-0 doi: 10.1007/s40819-020-00843-0
![]() |
[30] | World Health Organization, World health rankings: live longer live better, 2018. |
[31] |
S. M. Blower, H. Dowlatabadi, Sensitivity and uncertainty analysis of complex models of disease transmission: An HIV model, as an example, Int. Stat. Rev., 62 (1994), 229–243. https://doi.org/10.2307/1403510 doi: 10.2307/1403510
![]() |
[32] | B. G. Williams, E. Gouws, R0 and the elimination of HIV in Africa: Will 90-90-90 be sufficient?, available from: https://arXiv.org/ftp/arXiv/papers/1304/1304.3720.pdf (accessed 14 May 2019). |
[33] | J. P. LaSalle, The stability of dynamical systems, regional conference series in applied mathematics, Society for industrial and applied mathematics, Philadelphia, USA, 1976. |
1. | Chouaib Bounkaicha, Karam Allali, Modelling disease spread with spatio-temporal fractional derivative equations and saturated incidence rate, 2023, 2363-6203, 10.1007/s40808-023-01773-8 | |
2. | A. E. Matouk, Ismail Gad Ameen, Yasmeen Ahmed Gaber, Analyzing the dynamics of fractional spatio-temporal SEIR epidemic model, 2024, 9, 2473-6988, 30838, 10.3934/math.20241489 | |
3. | Syeda Alishwa Zanib, Sehrish Ramzan, Muzamil Abbas Shah, Nadeem Abbas, Wasfi Shatanawi, Comprehensive analysis of mathematical model of HIV/AIDS incorporating fisher-folk community, 2024, 10, 2363-6203, 6323, 10.1007/s40808-024-02099-9 |
Parameter | Description | Units |
Λ | Recruitment rate | per time |
μ | Natural mortality rate | per time |
δ | Mortality rate due to HIV/AIDS | per time |
β | Transmission rate from Infected but unaware | per person per time |
ˆβ | Effective infectiousness per contact | per person per time |
η1 | Amplification factor of transmission rate from infected awaiting treatment | unitless |
η2 | Amplification factor of transmission rate from aware Infected awaiting treatment | unitless |
η3 | Amplification factor of transmission rate from Infected on treatment | unitless |
ρ1 | Progression to AIDS status by unaware infected | per time |
ρ2 | Progression to AIDS status by infected awaiting treatment | per time |
ρ3 | Progression to AIDS status by infected on treatment | per time |
θ | Testing rate | per time |
ϵ | Proportion not legible for treatment | unitless |
γ | Rate of enrolling into treatment after waiting to be legible | per time |
m | The fear of infection parameter that results in behavioural change | per person |
p | Level of protection by condoms | unitless |
n | The number of sexual partners | per person per time |
λi | Force of infection for each epoch for i=1,2,3. | per time |
ν2>0 | |||
ν1>0 | ν1<0 | ||
ν0>0 (R0<1) | ν0<0 (R0>1) | ν0>0 (R0<1) | ν0<0 (R0>1) |
no positive roots | 1 positive root | 2 positive roots | 1 positive root |
Year | 1990 | 1991 | 1992 | 1993 | 1994 | 1995 | 1996 | 1997 | 1998 | 1999 |
ALWHIV | 40000 | 59000 | 82000 | 110000 | 140000 | 170000 | 190000 | 220000 | 230000 | 250000 |
Year | 2000 | 2001 | 2002 | 2003 | 2004 | 2005 | 2006 | 2007 | 2008 | 2009 |
ALWHIV | 260000 | 260000 | 270000 | 270000 | 270000 | 280000 | 290000 | 290000 | 300000 | 310000 |
Year | 2010 | 2011 | 2012 | 2013 | 2014 | 2015 | 2016 | 2017 | 2018 | 2019 |
ALWHIV | 320000 | 320000 | 330000 | 340000 | 340000 | 350000 | 360000 | 360000 | 370000 | 370000 |
Parameter | Range | Nominal Value |
Λ | μ×S0 | |
μ | 0.01818 | |
δ | (0.1, 0.4) | 0.15477 |
β | (0, 1) | 0.7 |
η1 | (1, 3) | 2 |
η2 | (1, 2) | 1.0002 |
η3 | (0, 1) | 0.9998 |
ρ1 | (0.0667, 0.125) | 0.097980 |
ρ2 | (0.0667, 1) | 0.16998 |
ρ3 | (0.01, 0.0667) | 0.01 |
θ | (0, 1) | 0.99997 |
ϵ | (0, 1) | 0.0010232 |
γ | (0, 1) | 0.44029 |
m | (0, 1) | 0.015 |
Parameter | Description | Units |
Λ | Recruitment rate | per time |
μ | Natural mortality rate | per time |
δ | Mortality rate due to HIV/AIDS | per time |
β | Transmission rate from Infected but unaware | per person per time |
ˆβ | Effective infectiousness per contact | per person per time |
η1 | Amplification factor of transmission rate from infected awaiting treatment | unitless |
η2 | Amplification factor of transmission rate from aware Infected awaiting treatment | unitless |
η3 | Amplification factor of transmission rate from Infected on treatment | unitless |
ρ1 | Progression to AIDS status by unaware infected | per time |
ρ2 | Progression to AIDS status by infected awaiting treatment | per time |
ρ3 | Progression to AIDS status by infected on treatment | per time |
θ | Testing rate | per time |
ϵ | Proportion not legible for treatment | unitless |
γ | Rate of enrolling into treatment after waiting to be legible | per time |
m | The fear of infection parameter that results in behavioural change | per person |
p | Level of protection by condoms | unitless |
n | The number of sexual partners | per person per time |
λi | Force of infection for each epoch for i=1,2,3. | per time |
ν2>0 | |||
ν1>0 | ν1<0 | ||
ν0>0 (R0<1) | ν0<0 (R0>1) | ν0>0 (R0<1) | ν0<0 (R0>1) |
no positive roots | 1 positive root | 2 positive roots | 1 positive root |
Year | 1990 | 1991 | 1992 | 1993 | 1994 | 1995 | 1996 | 1997 | 1998 | 1999 |
ALWHIV | 40000 | 59000 | 82000 | 110000 | 140000 | 170000 | 190000 | 220000 | 230000 | 250000 |
Year | 2000 | 2001 | 2002 | 2003 | 2004 | 2005 | 2006 | 2007 | 2008 | 2009 |
ALWHIV | 260000 | 260000 | 270000 | 270000 | 270000 | 280000 | 290000 | 290000 | 300000 | 310000 |
Year | 2010 | 2011 | 2012 | 2013 | 2014 | 2015 | 2016 | 2017 | 2018 | 2019 |
ALWHIV | 320000 | 320000 | 330000 | 340000 | 340000 | 350000 | 360000 | 360000 | 370000 | 370000 |
Parameter | Range | Nominal Value |
Λ | μ×S0 | |
μ | 0.01818 | |
δ | (0.1, 0.4) | 0.15477 |
β | (0, 1) | 0.7 |
η1 | (1, 3) | 2 |
η2 | (1, 2) | 1.0002 |
η3 | (0, 1) | 0.9998 |
ρ1 | (0.0667, 0.125) | 0.097980 |
ρ2 | (0.0667, 1) | 0.16998 |
ρ3 | (0.01, 0.0667) | 0.01 |
θ | (0, 1) | 0.99997 |
ϵ | (0, 1) | 0.0010232 |
γ | (0, 1) | 0.44029 |
m | (0, 1) | 0.015 |