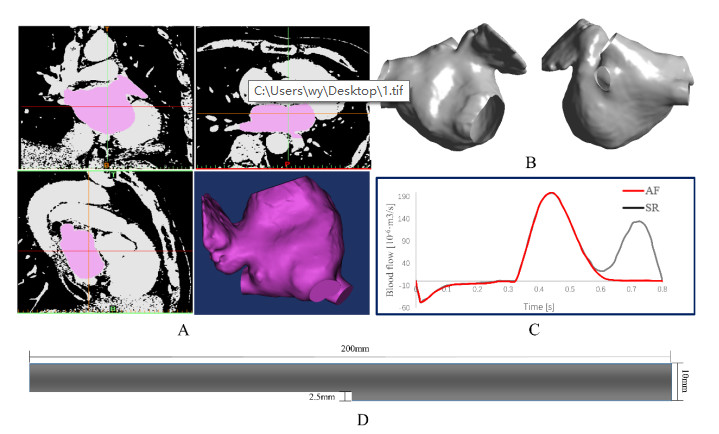
Citation: Yan Wang, Yonghui Qiao, Yankai Mao, Chenyang Jiang, Jianren Fan, Kun Luo. Numerical prediction of thrombosis risk in left atrium under atrial fibrillation[J]. Mathematical Biosciences and Engineering, 2020, 17(3): 2348-2360. doi: 10.3934/mbe.2020125
[1] | Ya'nan Wang, Sen Liu, Haijun Jia, Xintao Deng, Chunpu Li, Aiguo Wang, Cuiwei Yang . A two-step method for paroxysmal atrial fibrillation event detection based on machine learning. Mathematical Biosciences and Engineering, 2022, 19(10): 9877-9894. doi: 10.3934/mbe.2022460 |
[2] | Miao Zhu, Tao Yan, Shijie Zhu, Fan Weng, Kai Zhu, Chunsheng Wang, Changfa Guo . Identification and verification of FN1, P4HA1 and CREBBP as potential biomarkers in human atrial fibrillation. Mathematical Biosciences and Engineering, 2023, 20(4): 6947-6965. doi: 10.3934/mbe.2023300 |
[3] | Nattawan Chuchalerm, Wannika Sawangtong, Benchawan Wiwatanapataphee, Thanongchai Siriapisith . Study of Non-Newtonian blood flow - heat transfer characteristics in the human coronary system with an external magnetic field. Mathematical Biosciences and Engineering, 2022, 19(9): 9550-9570. doi: 10.3934/mbe.2022444 |
[4] | Shengjue Xiao, Yufei Zhou, Ailin Liu, Qi Wu, Yue Hu, Jie Liu, Hong Zhu, Ting Yin, Defeng Pan . Uncovering potential novel biomarkers and immune infiltration characteristics in persistent atrial fibrillation using integrated bioinformatics analysis. Mathematical Biosciences and Engineering, 2021, 18(4): 4696-4712. doi: 10.3934/mbe.2021238 |
[5] | Oualid Kafi, Nader El Khatib, Jorge Tiago, Adélia Sequeira . Numerical simulations of a 3D fluid-structure interaction model for blood flow in an atherosclerotic artery. Mathematical Biosciences and Engineering, 2017, 14(1): 179-193. doi: 10.3934/mbe.2017012 |
[6] | Huu Thuan Nguyen, Tu Anh Do, Benoît Cosson . Numerical simulation of submerged flow bridge scour under dam-break flow using multi-phase SPH method. Mathematical Biosciences and Engineering, 2019, 16(5): 5395-5418. doi: 10.3934/mbe.2019269 |
[7] | Annalisa Quaini, Sunčica Čanić, David Paniagua . Numerical characterization of hemodynamics conditions near aortic valve after implantation of left ventricular assist device. Mathematical Biosciences and Engineering, 2011, 8(3): 785-806. doi: 10.3934/mbe.2011.8.785 |
[8] | Hongtao Liu, Shuqin Liu, Xiaoxu Ma, Yunpeng Zhang . A numerical model applied to the simulation of cardiovascular hemodynamics and operating condition of continuous-flow left ventricular assist device. Mathematical Biosciences and Engineering, 2020, 17(6): 7519-7543. doi: 10.3934/mbe.2020384 |
[9] | Abdul Qadeer Khan, Azhar Zafar Kiyani, Imtiaz Ahmad . Bifurcations and hybrid control in a 3×3 discrete-time predator-prey model. Mathematical Biosciences and Engineering, 2020, 17(6): 6963-6992. doi: 10.3934/mbe.2020360 |
[10] | Li Cai, Yu Hao, Pengfei Ma, Guangyu Zhu, Xiaoyu Luo, Hao Gao . Fluid-structure interaction simulation of calcified aortic valve stenosis. Mathematical Biosciences and Engineering, 2022, 19(12): 13172-13192. doi: 10.3934/mbe.2022616 |
Atrial fibrillation (AF) is a common cardiac arrhythmia, during which the regular and orderly electrical activity in the atrium is replaced by rapid and chaotic heartbeats. The ineffective contraction of the atrium in the post-diastolic phase during AF can induce thrombosis, while the shed thrombus can cause ischemic stroke when flowing to the brain. The presence of AF is independently associated with a 5-fold increased risk of stroke [1]. The left atrial appendage (LAA) is a finger- or stump-like extension of the left atrium (LA) with lobes that may harbor up to 90% of thrombi that occur in patients with AF [2]. The remodeling process associated with AF causes the LAA to function as a static pouch, predisposing to stagnation and thrombosis. It also should be noticed that the proportion of thrombus in LAA as the origin of cardiogenic emboli is as high as 100% for non-vascular AF patients [2].
Prevention strategies of ischemic stroke caused by AF include oral anticoagulation therapy (i.e., warfarin) and minimally invasive surgeries to exclude LAA [3,4,5]. Before anticoagulation therapy, the risk of stroke is stratified according to the CHA2DS2-VASc score which refers to age, sex, stroke history and so on. A higher score means a higher risk of stroke [6]. CHA2DS2-VASc score ≡2 means a patient at high risk and needs anticoagulation therapy. However, the scoring system still has some limitations. For example, a young male with AF whose CHA2DS2-VASc score is 0, still could develop ischemic stroke. Noticed that some clinical studies have shown that LA and LAA hemodynamic information which is difficult to obtain through current medical technology, can improve stroke risk stratification [6], thus computational fluid dynamics (CFD) is widely applied to capture blood flow characteristics [7,8,9]. The study of the LA wall motion indicates that LAA is ineffective and acts as a stasis blood reservoir during AF [9]. The blood evacuation rate in LAA decreases when AF occurs which might increase thrombosis risk [8]. Comparing the lack of active atrial contraction, the occurrence of high-frequency fibrillation might have a larger impact on the blood flow stagnation [10]. We find that only a few experimental and numerical research study the flow patterns in LA [11] and hemodynamic changes induced by different LAA morphology or AF [7,8,9,12]. At the same time, the direct simulation of thrombosis in the LA, especially LAA, has yet to be produced.
The purpose of this study was to simulate the thrombosis in LA using advanced computational modeling analyses. To this end, the patient-specific geometry was reconstructed and a thrombus model was combined with constant inlet pressure and realistic outflow. To the author*s knowledge, the present study is the first to use the CFD method to directly model thrombosis in patient-specific LA. The magnitudes of thrombosis variables, blood flow distribution and wall shear stress (WSS) related indices were analyzed to explore the impact of AF on the hemodynamics.
Computed tomography (CT) images of LA and four pulmonary veins (PVs) were obtained from an adult patient with non-valvular AF at Sir Run Run Shaw Hospital, Zhejiang University School of Medicine (Hangzhou, China). The study was conducted in accordance with the ethical standards of the Local Institution Review Board and with the 1964 Helsinki declaration and its later amendments. This project was approved by the Local Bioethics Committee. All patients have given their written informed consent before the study. The patient-specific LA geometry was constructed from the CT images by using Mimics 19.0 (Materialise, Belgium) (Figure 1-A). All the geometric boundaries of inlets and outlet were cropped to get a flat surface in GeoMagic Studio (GeoMagic Inc, USA) (Figure 1-B). The fluid domain was meshed using commercial software ANSYS-ICEM (ANSYS Inc, Canonsburg, USA), while five prism layers of unstructured tetrahedral meshes were created close to the atrial wall. The computational domain had approximately 2,420,000 elements, and the unstructured tetrahedral mesh had a maximum size of 7 mm. Finer meshes with more than 4,900,000 elements were created to assess the mesh sensitivity. The differences in peak wall shear stress and averaged wall shear stress between meshes were both less than 2%.
The blood was considered to be an incompressible and non-Newtonian fluid with a constant density of 1080 kg/m3 [13]. The LA wall was assumed to be rigid with no-slip conditions. The deformation of the LA should be considered for precise results in most heart flow simulations. However, the focus of the present study is on the application of a thrombus model in LA to simulate thrombosis. In addition, the effect of the atrial kick absence, a feature of the AF, on thrombosis is also explored. To build a fully developed blood flow environment before thrombus model was employed [13], Quemada model was used to simulate the shear-thinning characteristics of the blood [14] for two complete cardiac cycles (each cardiac cycle of 0.8 s duration including 37.5% ventricular diastole and 62.5% ventricular systole). The thrombus model was developed based on a hemodynamics-based model presented by Menichini, et al. [15], which simulated thrombosis by solving transport equations of flow residence time (RT), activated platelets (AP), resting platelets (RP), bound platelets (BP) and coagulation (C). The thrombus model considers the effect of the thrombus on the blood viscosity as well. Thrombus is identified through the local BP concentration. Details of the mathematical equations can be found in the study of Menichini, et al. [15], but there are some important changes described below.
The momentum of the flow is controlled by the simplified Navier-Stokes equation. We ignore the frictional force of thrombus on the fluid. For the transport of AP and RP, a non-normalized source item Si is applied which is the summary of r1 and r2. BP and C have been normalized by their initial values for avoiding numerical ill-conditioning. Besides, the judgment threshold value of the local time average shear strain rate is 1 s-1, which determines that the initial concentration of C is 100 or 0 nmol/L.
ρ∂u∂t+ρu⋅∇u=−∇p+∇⋅(μ(∇u+(∇u)T)) | (1) |
r1=k1⋅AP⋅RPr2=k2⋅RP⋅RRT | (2) |
k1 and k2 are the kinetic constants [16]. AP and RP are the concentrations of the platelets respectively. RRT is the standardized RT which is normalized by cardiac cycle time.
The difficulty in obtaining patient-specific flowrate data in the outlet, mitral valve (MV), would be revealed when using medical measurement. The flowrate curves across the MV for the sinus rhythm (SR) cases were derived from the international regulation, which included E-wave and A-wave representing the first and the second phases, respectively [17]. The transmitral Doppler echocardiographic analysis of AF patients shows that A wave which is caused by the atrial kick in late diastole is absent in the flowrate curve [10,18]. The flowrate curves for AF cases were differed by dismissing the A wave [7]. For the four pulmonary veins inlets, constant pressure of 10 mmHg was adopted. Flowrate curves for SR and AF cases are shown in Figure 1-C, the peaks of two curves are nearly 46.9 ml/s. The maximum Reynolds number of all cases was found lower than 3000 when estimated on the basis of the peak flow rate and the MV diameter. Therefore, the blood flow was assumed as a laminar flow. Simulations were performed on ANSYS Workbench 16.1 (ANSYS Inc, USA). The time step in this transient study was 10 ms and all the cases were simulated for 20 cardiac cycles to get feasible results and the twentieth cycle data was post-processed.
Before applied to LA geometry, the thrombus model was tested in a backward-facing step (BFS) model (Figure 1-D). Quemada model with a 30% hematocrit was selected to simulate experimental blood [19], while in the LA model, the hematocrit of human blood was determined to be nearly 45% [14]. The boundary conditions were adopted from the experimental test, with 0.76 L/min at the inlets and zero pressure at the outlet [19]. Except for the bulk shear rate threshold, all other parameters were the same as in the LA model. The time step in this verified study was 0.05 s and the calculation time was 50 s. Verification results were compared with the experimental study [19] and the simulation results [15].
Figure 2 shows the thrombosis prediction results in the BFS model and they are compared with the published results. The thrombus initially appears in the downstream of the step then grows continuously. When the height of the thrombus reaches the step height (2.5 mm) and the maximum width is close to that of the step, thrombus growth almost stops in the radial direction. The thrombus growth turns slow after 14 s and almost stops growing when the total thrombus length reaches about 8.8 times the height of the step, with a maximum length of 22 mm. These results are consistent with the simulation data of Menichini, et al. [15] and match well with the experimental results [19] as we can use it for further study.
The velocity streamlines for two cases are illustrated in Figure 3. The initial streamlines seeds are averagely launched from PVs. At the first peak systole (0.4 s), the streamline distributions in the two cases are similar, the velocities in the inlets (PVs) and MV outlet are higher than in other areas of the LA. During the later peak systole (0.7 s), the blood flow gets highly disordered in the recirculating area, and the maximum velocity appears nearby the left inferior pulmonary vein (LIPV) rather than the PVs and MV in the AF case. Besides, there is little blood flowing through the MV in the AF case while blood can in the SR case. Mentioned that there is little blood flowing out from the LAA as well. Table 1 shows the proportions of blood that pass through each inlet boundaries during the last cardiac cycle. During the later peak systole for AF case, the flow ratios of the four inlets were not calculated because part of the inlet blood returned. In the SR case, the blood flow crossing the LIPV accounts for nearly 30% of the outflow while the minority of the blood (below 20%) goes through the right inferior pulmonary vein.
Inlet boundaries | 0.4 s | 0.7 s | ||
AF | SR | AF | SR | |
Left Superior Pulmonary Vein | 26.86% | 27.88% | - | 24.49% |
Left Inferior Pulmonary Vein | 30.27% | 30.56% | - | 29.02% |
Right Superior Pulmonary Vein | 26.14% | 25.35% | - | 27.20% |
Right Inferior Pulmonary Vein | 16.74% | 16.22% | - | 19.29% |
AF: atrial fibrillation; SR: sinus rhythm. |
The frictional force on the LA wall exerted by the blood flow is quite difficult to be obtained through current measurement technology but can be studied by the WSS. The distributions of WSS for two cases in peak systole phases are shown in Figure 4. Similar to the flow waveform, the distributions of WSS in two cases have little difference at the earlier peak systole. At the later peak systole, the maximum WSS values of two cases decrease greatly, especially in the AF case. Besides, the region around LIPV shows a bigger WSS than the other three inlets in the AF case (Figure 4-D), while areas around the four PVs have a similar distribution in the other three maps. The LAA has a small WSS value in all maps.
We choose BP as a thrombosis sign with a threshold of 200 nmol/L [20]. Figure 5 shows the thrombosis in the maximum cross-section of the LAA from 5.6 s with 1.6 s interval for AF case. The simulation data illustrates that thrombus forms since the seventh cardiac cycle. The thrombus first appears on the wall of the LAA, then grows inward and fills most space in the LAA at the end of the nineteenth cardiac cycle. The distributions and volume proportions (comparing with the total LA volume) of formed thrombus in the seventh and last cycles of two cases are shown in Figure 6. Thrombus only arises in the LAA with its proportions have increased tremendously for two cases from the eighth to the twentieth cycle. The difference of thrombosis ratio is clear between two cases at 16 s while AF case has a greater severe thrombosis with a 4.93% thrombus volume proportion.
The formation of BP is directly influenced by C, whose initial value is depending on the distribution of the average shear rate on the wall after the first two cardiac cycles. If the average shear rate is smaller than the judgment threshold, the initial value of C is 200 nmol/L [15]. In view of the importance of C initial value for outcomes, thrombosis in AF cases with judgment thresholds of 0.5 s-1 and 1.5 s-1 were calculated as well (Figure 7). For all cases with different thresholds, the thrombus is visible since the seventh cardiac cycle. In the end, the volume fraction of thrombus reaches 5.68% in the case with a threshold of 1.5 s-1, which is nearly twice that of the case with the judgment threshold of 0.5 s-1. Other than that, the relative thrombus fraction difference between cases with thresholds of 1.5 s-1 and 1 s-1 is 15.2%, which is smaller than that value between cases with thresholds of 1 s-1 and 0.5 s-1. Figure 7-D investigates the time-varying laws of thrombus proportions for three cases. The growth of thrombosis accelerates with the increase of judgment threshold and decelerates with the change of time. Furthermore, the difference of thrombus growth rates between cases with judgment thresholds of 1.5 s-1 and 1 s-1 is smaller than that between the latter and the case with a threshold of 0.5 s-1.
There are millions of patients suffering from non-valvular AF in China, with a 20% fatality rate and a 60% disability rate [21]. The management of AF in China is unproductive and accompanied by a high risk of mortality due to patients* low awareness and lack of proper treatment [22]. Clinical studies have shown that the usage of warfarin or new anticoagulants in AF patients with a high stroke risk can significantly reduce stroke events and improve patient outcomes [22]. Therefore, the risk assessment of thrombosis under AF conditions is crucial in the prevention of AF-related stroke. Hemodynamic characteristics of LA can improve stroke risk stratification [6], but some of them are difficult to perform high-precision measurement through non-invasive means in clinical work [7,23]. Thus we established a thrombus model to study the AF effect on the hemodynamics in the LA by numerical simulation and to build a fundament for further application of the CFD method to study thrombosis risk prediction [8].
In this study, we utilized the thrombus model to predict thrombosis in LA with the consideration of non-Newtonian properties of blood for investigating the hemodynamics difference between AF and SR cases to the same LA geometry while most previous studies just simplify blood as Newtonian flow [2,8,9,10,11,12]. The thrombus model was calibrated in the BFS model before applied to the LA model because of the absence of the thrombosis experiment in a spherical tube [19]. The results of the benchmark study were well matched with the experiment results.
Observing the streamlines in the second peak systole, in the AF case, there is little blood flowing through the MV due to the lack of atrial kick but toward the LIPV vice versa, while in the SR case, blood can pass through the MV. It will increase blood*s impact force on the wall which is consistent with the WSS distribution. The blood flow gets highly disordered in the recirculating area which means the formed thrombus is easier to separate from the wall and then move with the blood flow. Comparing to the other areas, the velocity and WSS are small in the LAA dramatically, indicating that the LAA region is susceptible to thrombosis and may be worthy of attention in the thrombosis risk assessment as previous studies have demonstrated that low WSS distributions identify regions more prone to thrombus formation [24].
There are many variables in the thrombus model, the distribution of BP predicts the thrombosis area directly while RT, C, AP, and RP are intermediate variables. C has the closest tie with the BP when compared to RT, AP and RP, as the formation of C decides the initiation of BP, in addition, BP will promote the formation of C as well. The impact of C on the thrombosis was also studied in this paper. Observation of BP above the judgment threshold shows that thrombosis mainly happens in the LAA which is consistent with the clinical statistics [2]. Thrombosis begins in the wall because of the tissue factor exposure and interaction of cells and fibrinogen there which are enhanced with a low shear rate [25]. The simulation results show that the thrombus distributions are distinguished between the different cardiac rhythms. In the AF case, thrombosis is more serious than the SR case with a ratio difference close to 18%. It also means that the impact of the occurrence of high-frequency fibrillation cannot be ignored in the simulation of AF [10]. C is an important variable that influences the initiation of thrombosis, and its initial value depends on the underlined threshold of the time-averaged shear stress rate from previous simulation results. From the sensitivity analysis of the threshold, we have found that a higher threshold means more LAA area has non zero initial C and a faster thrombosis growth rate. It can be deduced that the threshold we have chosen possessed certain rationality, noticing that the difference between thresholds of 1.5 s-1 and 1 s-1 is much smaller than that of cases with thresholds of 1 s-1 and 0.5 s-1.
Treating the LA wall as a rigid wall may be a key limitation of our simulation in this paper. In this study, we investigated the impact of the atrial kick absence by changing the outlet condition as previous researchers did [7]. For AF, there are more characters that will influence the hemodynamics in the LA, such as the high-frequency fibrillation of the LA wall. To the author*s knowledge, there are two ways to rebuild the deformation of LA, one is to set the atrial wall as the fibre-reinforced hyperplastic material and consider the fluid-structure interaction effect [9,26], while the other way is to load the deformation laws of the LA and the LAA, which can be derived from the MR or CT slice-images of the volunteers to the geometry directly [8,10,12,27]. We will consider the deformation of the LA and the LAA for the thrombosis prediction by using these two ways in our future study.
In addition, the boundary conditions are not strictly patient-specific due to the absence of clinical measurement data. The aim of the present study was the application of thrombus model to simulate the thrombosis in LA rather than the accurate thrombosis prediction in special patients. Our further study will focus on the latter strategy and PC-MRI measurement data would be used as boundary conditions for further improvement of the simulation.
LAA has stronger active contractility than LA, due to its thick pectinate muscle [28]. For healthy people, the contraction of LAA will empty the inside blood while AF patients vice versa [3,28]. The neglect of the influence of the LAA contraction may lead to thrombosis in the SR patient cases. More studies will be displayed on the LAA contraction and geometry in our future research to evaluate their impacts on thrombosis.
Thrombosis is a complex process involves the conversion of many substances [29]. In this paper, we only consider the effects of four substances. There is no doubt that other substances also have impacts on the viscosity property of the blood flow and thrombosis which should be further studied.
This is the first study that directly investigates the thrombosis in the LA with the discussion of the hemodynamics changes. The thrombus model used in this paper was calibrated in the BFS model and in good agreement with the experimental data. The hemodynamics of the AF cases and SR cases are compared, showing that the hemodynamics in LA is greatly affected by AF. Thrombosis observed in this study only occurs in the LAA which is consistent with clinical findings, thrombus first forms on the LAA wall then grows inward until filling up the inner space of LAA. AF can promote the degree of thrombosis. In the future, numerical simulation of the thrombosis and clinical statistics can be combined to improve the risk assessment mechanism of thrombosis and stroke.
All authors declare no conflicts of interest in this paper.
[1] | T. J. Wang, J. M. Massaro, D. Levy, R. S. Vasan, P. A. Wolf, R. B. D'Agostino, et al., A risk score for predicting stroke or death in individuals with new-onset atrial fibrillation in the communitythe framingham heart study, JAMA, 290 (2003), 1049-1056. |
[2] | C. Alberto, G. F. Miguel Angel, S. Horst, M. Patrizio, B. Pasquale, S. Marco, et al., Prevalence of extra-appendage thrombosis in non-valvular atrial fibrillation and atrial flutter in patients undergoing cardioversion: A large transoesophageal echo study, EuroIntervention, 15 (2019), e225-e230. |
[3] | N. Al-Saady, O. Obel, A. Camm, Left atrial appendage: Structure, function, and role in thromboembolism, Heart, 82 (1999), 547-554. |
[4] | Y. Y. Lam, B. P. Yan, S. K. Doshi, A. Li, D. Zhang, M. G. Kaya, et al., Preclinical evaluation of a new left atrial appendage occluder (lifetech lambreTM device) in a canine model, Int. J. Cardiol., 168 (2013), 3996-4001. |
[5] | J. D. Moss, Left atrial appendage exclusion for prevention of stroke in atrial fibrillation: Review of minimally invasive approaches, Curr. Cardiol. Rep., 16 (2014), 448. |
[6] | D. K. Gupta, A. M. Shah, R. P. Giugliano, C. T. Ruff, E. M. Antman, L. T. Grip, et al., Left atrial structure and function in atrial fibrillation: Engage af-timi 48, Eur. Heart J., 35 (2014), 1457-1465. |
[7] | G. M. Bosi, A. Cook, R. Rai, L. J. Menezes, S. Schievano, R. Torii, et al., Computational fluid dynamic analysis of the left atrial appendage to predict thrombosis risk, Front. Cardiov. Med., 5 (2018), 34. |
[8] | A. Masci, M. Alessandrini, L. Luca Ded, D. Forti, F. Menghini, C. Tomasi, et al., Development of a computational fluid dynamics model of the left atrium in atrial fibrillation on a patient specific basis, Computing, 44 (2017), 1. |
[9] | L. T. Zhang, M. Gay, Characterizing left atrial appendage functions in sinus rhythm and atrial fibrillation using computational models, J. Biomech., 41 (2008), 2515-2523. |
[10] | R. Koizumi, K. Funamoto, T. Hayase, Y. Kanke, M. Shibata, Y. Shiraishi, et al., Numerical analysis of hemodynamic changes in the left atrium due to atrial fibrillation, J. Biomech., 48 (2015), 472-478. |
[11] | C. Chnafa, S. Mendez, F. Nicoud, Image-based large-eddy simulation in a realistic left heart, Comput. Fluid., 94 (2014), 173-187. |
[12] | A. Masci, L. Barone, L. Dede, M. Fedele, C. Tomasi, A. Quarteroni, et al., The impact of left atrium appendage morphology on stroke risk assessment in atrial fibrillation: A computational fluid dynamics study, Front. Physiol., 9 (2018), 1938. |
[13] | F. J. H. Gijsen, E. Allanic, F. N. van de Vosse, J. D. Janssen, The influence of the non-newtonian properties of blood on the flow in large arteries: Unsteady flow in a 90° curved tube, J. Biomech., 32 (1999), 705-713. |
[14] | F. J. H. Gijsen, F. N. van de Vosse, J. D. Janssen, The influence of the non-newtonian properties of blood on the flow in large arteries: Steady flow in a carotid bifurcation model, J. Biomech., 32 (1999), 601-608. |
[15] | C. Menichini, X. Y. Xu, Mathematical modeling of thrombus formation in idealized models of aortic dissection: Initial findings and potential applications, J. Math. Biol., 73(2016), 1205-1226. |
[16] | M. Anand, K. Rajagopal, K. Rajagopal, A model incorporating some of the mechanical and biochemical factors underlying clot formation and dissolution in flowing blood, J. Theoret. Med., 5 (2003), 183-218. |
[17] | International Organization for Standardization, Iso 5840-1: 2015 cardiovascular implants-cardiac valve prostheses part 1: General requirements, Geneva, Switzerland: International Organization for Standardization, (2015), 5840-5841 |
[18] | S. Gautam, R. John, Interatrial electrical dissociation after catheter-based ablation for atrial fibrillation and flutter, Circulat. Arrhythm. Electrophysiol., 4 (2011), e26-28. |
[19] | J. O. Taylor, K. P. Witmer, T. Neuberger, B. A. Craven, R. S. Meyer, S. Deutsch, et al., In vitro quantification of time dependent thrombus size using magnetic resonance imaging and computational simulations of thrombus surface shear stresses, J. Biomech. Eng., 136 (2014). |
[20] | C. Menichini, Z. Cheng, R. G. Gibbs, X. Y. Xu, Predicting false lumen thrombosis in patient-specific models of aortic dissection, J. R. Soc. Interface, 13 (2016). |
[21] | C. E. Chiang, K. Okumura, S. Zhang, T. F. Chao, C. W. Siu, T. Wei Lim, et al., 2017 consensus of the asia pacific heart rhythm society on stroke prevention in atrial fibrillation, J. Arrhythm., 33 (2017), 345-367. |
[22] | L. H. Li, C. S. Sheng, B. C. Hu, Q. F. Huang, W. F. Zeng, G. L. Li, et al., The prevalence, incidence, management and risks of atrial fibrillation in an elderly chinese population: A prospective study, BMC Cardiovasc. Disord., 15 (2015), 31. |
[23] | I. Dentamaro, D. Vestito, E. Michelotto, D. De Santis, V. Ostuni, C. Cadeddu, et al., Evaluation of left atrial appendage function and thrombi in patients with atrial fibrillation: From transthoracic to real time 3d transesophageal echocardiography, Int. J. Cardiovasc. Imag., 33 (2017), 491-498. |
[24] | W. S. Nesbitt, E. Westein, F. J. Tovar-Lopez, E. Tolouei, A. Mitchell, J. Fu, et al., A shear gradient-dependent platelet aggregation mechanism drives thrombus formation, Nat. Med., 15 (2009), 665. |
[25] | B. Savage, E. Saldívar, Z. M. Ruggeri, Initiation of platelet adhesion by arrest onto fibrinogen or translocation on von willebrand factor, Cell, 84 (1996), 289-297. |
[26] | L. Feng, H. Gao, B. E. Griffith, S. A. Niederer, X. Luo, Analysis of a coupled fluid-structure interaction model of the left atrium and mitral valve, Int. J. Numer. Method Biomed. Eng., 0(2019), e3254. |
[27] | T. Otani, A. Al-Issa, A. Pourmorteza, E. R. McVeigh, S. Wada, H. Ashikaga, A computational framework for personalized blood flow analysis in the human left atrium, Ann. Biomed. Eng., 44 (2016), 3284-3294. |
[28] | R. Beigel, N. C. Wunderlich, S. Y. Ho, R. Arsanjani, R. J. Siegel, The left atrial appendage: Anatomy, function, and noninvasive evaluation, JACC Cardiovasc. Imag., 7(2014), 1251-1265. |
[29] | E. N. Sorensen, G. W. Burgreen, W. R. Wagner, J. F. Antaki, Computational simulation of platelet deposition and activation: I. Model development and properties, Ann. Biomed. Eng., 27(1999), 436-448. |
1. | Runxin Fang, Yang Li, Yanjuan Zhang, Qiang Chen, Quanjun Liu, Zhiyong Li, Impact of left atrial appendage location on risk of thrombus formation in patients with atrial fibrillation, 2021, 1617-7959, 10.1007/s10237-021-01454-4 | |
2. | Alejandro Gonzalo, Manuel García‐Villalba, Lorenzo Rossini, Eduardo Durán, Davis Vigneault, Pablo Martínez‐Legazpi, Oscar Flores, Javier Bermejo, Elliot McVeigh, Andrew M. Kahn, Juan C. del Alamo, Non‐Newtonian blood rheology impacts left atrial stasis in patient‐specific simulations , 2022, 38, 2040-7939, 10.1002/cnm.3597 | |
3. | Pedro Morais, Dominik Nelles, Vivian Vij, Baravan Al-Kassou, Marcel Weber, Georg Nickenig, Jan Wilko Schrickel, João L. Vilaça, Alexander Sedaghat, Assessment of LAA Strain and Thrombus Mobility and Its Impact on Thrombus Resolution—Added-Value of a Novel Echocardiographic Thrombus Tracking Method, 2022, 13, 1869-408X, 950, 10.1007/s13239-022-00629-z | |
4. | YiRen Sun, Yunfei Ling, Zijia Chen, Zhengjie Wang, Tao Li, Qi Tong, Yongjun Qian, Finding low CHA2DS2-VASc scores unreliable? Why not give morphological and hemodynamic methods a try?, 2023, 9, 2297-055X, 10.3389/fcvm.2022.1032736 | |
5. | Lida Alinezhad, Farzan Ghalichi, Majid Ahmadlouydarab, Maryam Chenaghlou, Left atrial appendage shape impacts on the left atrial flow hemodynamics: A numerical hypothesis generating study on two cases, 2022, 213, 01692607, 106506, 10.1016/j.cmpb.2021.106506 | |
6. | Wen-Jing Xiang, Jia-Dong Huo, Wei-Tao Wu, Peng Wu, Influence of Inlet Boundary Conditions on the Prediction of Flow Field and Hemolysis in Blood Pumps Using Large-Eddy Simulation, 2023, 10, 2306-5354, 274, 10.3390/bioengineering10020274 | |
7. | Ahmed Qureshi, Gregory Y. H. Lip, David A. Nordsletten, Steven E. Williams, Oleg Aslanidi, Adelaide de Vecchi, Imaging and biophysical modelling of thrombogenic mechanisms in atrial fibrillation and stroke, 2023, 9, 2297-055X, 10.3389/fcvm.2022.1074562 | |
8. | Kan Huang, Haitao Wen, Canjun Liu, Association Between Ischemic Stroke and Left Atrial Appendage Morphology in Patients With Atrial Fibrillation and Low CHA2DS2-VASc Scores, 2022, 49, 1526-6702, 10.14503/THIJ-20-7544 | |
9. | Lianru Zang, Kaihao Gu, Xingkai Ji, Hao Zhang, Shengjie Yan, Xiaomei Wu, Comparative Analysis of Temperature Rise between Convective Heat Transfer Method and Computational Fluid Dynamics Method in an Anatomy-Based Left Atrium Model during Pulsed Field Ablation: A Computational Study, 2023, 10, 2308-3425, 56, 10.3390/jcdd10020056 | |
10. | Runxin Fang, Yang Li, Jun Wang, Zidun Wang, John Allen, Chi Keong Ching, Liang Zhong, Zhiyong Li, Stroke risk evaluation for patients with atrial fibrillation: Insights from left atrial appendage, 2022, 9, 2297-055X, 10.3389/fcvm.2022.968630 | |
11. | Runxin Fang, Zidun Wang, Xie Zhao, Jun Wang, Yang Li, Yanjuan Zhang, Qiang Chen, Jiaqiu Wang, Quanjun Liu, Minglong Chen, Zhiyong Li, Stroke risk evaluation for patients with atrial fibrillation: Insights from left atrial appendage with fluid-structure interaction analysis, 2022, 148, 00104825, 105897, 10.1016/j.compbiomed.2022.105897 | |
12. | Kaihao Gu, Shengjie Yan, Xiaomei Wu, Influence of pulsating intracardiac blood flow on radiofrequency catheter ablation outcomes in an anatomy-based atrium model, 2022, 39, 0265-6736, 1064, 10.1080/02656736.2022.2108149 | |
13. | Jordi Mill, Victor Agudelo, Andy L. Olivares, Maria Isabel Pons, Etelvino Silva, Marta Nuñez-Garcia, Xabier Morales, Dabit Arzamendi, Xavier Freixa, Jérôme Noailly, Oscar Camara, Sensitivity Analysis of In Silico Fluid Simulations to Predict Thrombus Formation after Left Atrial Appendage Occlusion, 2021, 9, 2227-7390, 2304, 10.3390/math9182304 | |
14. | Yonghui Qiao, Kun Luo, Jianren Fan, Computational Prediction of Thrombosis in Food and Drug Administration’s Benchmark Nozzle, 2022, 13, 1664-042X, 10.3389/fphys.2022.867613 | |
15. | Mattia Corti, Alberto Zingaro, Luca Dede’, Alfio Maria Quarteroni, Impact of atrial fibrillation on left atrium haemodynamics: A computational fluid dynamics study, 2022, 150, 00104825, 106143, 10.1016/j.compbiomed.2022.106143 | |
16. | Lianru Zang, Kaihao Gu, Xingkai Ji, Hao Zhang, Shengjie Yan, Xiaomei Wu, Effect of Anisotropic Electrical Conductivity Induced by Fiber Orientation on Ablation Characteristics of Pulsed Field Ablation in Atrial Fibrillation Treatment: A Computational Study, 2022, 9, 2308-3425, 319, 10.3390/jcdd9100319 | |
17. | Ehsan Khalili, Cécile Daversin‐Catty, Andy L. Olivares, Jordi Mill, Oscar Camara, Kristian Valen‐Sendstad, On the importance of fundamental computational fluid dynamics toward a robust and reliable model of left atrial flows, 2024, 40, 2040-7939, 10.1002/cnm.3804 | |
18. | Carlos Albors, Andy L. Olivares, Xavier Iriart, Hubert Cochet, Jordi Mill, Oscar Camara, 2023, Chapter 50, 978-3-031-35301-7, 485, 10.1007/978-3-031-35302-4_50 | |
19. | Henrik A. Kjeldsberg, Carlos Albors, Jordi Mill, David Viladés Medel, Oscar Camara, Joakim Sundnes, Kristian Valen‐Sendstad, Impact of left atrial wall motion assumptions in fluid simulations on proposed predictors of thrombus formation, 2024, 40, 2040-7939, 10.1002/cnm.3825 | |
20. | Yan Wang, Buyun Xu, Kun Luo, Jianren Fan, Meixiang Xiang, The impact of left atrial morphology on thrombosis risk in atrial fibrillation, 2024, 36, 1070-6631, 10.1063/5.0214185 | |
21. | Qi Gao, Hongtao Lin, Jianghong Qian, Xingli Liu, Shengze Cai, He Li, Hongguang Fan, Zhe Zheng, A deep learning model for efficient end-to-end stratification of thrombotic risk in left atrial appendage, 2023, 126, 09521976, 107187, 10.1016/j.engappai.2023.107187 | |
22. | Jordi Mill, Josquin Harrison, Marta Saiz-Vivo, Carlos Albors, Xabier Morales, Andy L. Olivares, Xavier Iriart, Hubert Cochet, Jerome Noailly, Maxime Sermesant, Oscar Camara, The role of the pulmonary veins on left atrial flow patterns and thrombus formation, 2024, 14, 2045-2322, 10.1038/s41598-024-56658-2 | |
23. | Lan Ge, Yawei Xu, Jun Li, Yuan Li, Yifeng Xi, Xinyan Wang, Jing Wang, Yang Mu, Hongsen Wang, Xu Lu, Jun Guo, Zengsheng Chen, Tao Chen, Yundai Chen, The impact of contrast retention on thrombus formation risks in patients with atrial fibrillation: A numerical study, 2024, 10, 24058440, e26792, 10.1016/j.heliyon.2024.e26792 | |
24. | Sara Valvez, Manuel Oliveira-Santos, Ana P. Piedade, Lino Gonçalves, Ana M. Amaro, Computational Flow Dynamic Analysis in Left Atrial Appendage Thrombus Formation Risk: A Review, 2023, 13, 2076-3417, 8201, 10.3390/app13148201 | |
25. | Alberto Zingaro, Zan Ahmad, Eugene Kholmovski, Kensuke Sakata, Luca Dede’, Alan K. Morris, Alfio Quarteroni, Natalia A. Trayanova, A comprehensive stroke risk assessment by combining atrial computational fluid dynamics simulations and functional patient data, 2024, 14, 2045-2322, 10.1038/s41598-024-59997-2 | |
26. | Runxin Fang, Zidun Wang, Jiaqiu Wang, Jiayu Gu, Geman Yin, Qiang Chen, Xunrong Xia, Zhiyong Li, Patient-specific pulmonary venous flow characterization and its impact on left atrial appendage thrombosis in atrial fibrillation patients, 2024, 257, 01692607, 108428, 10.1016/j.cmpb.2024.108428 | |
27. | Åshild Telle, Clarissa Bargellini, Yaacoub Chahine, Juan C. Del Álamo, Nazem Akoum, Patrick M Boyle, Personalized biomechanical insights in atrial fibrillation: opportunities & challenges, 2023, 21, 1477-9072, 817, 10.1080/14779072.2023.2273896 | |
28. | João Loures Salinet, ĺtalo Sandoval Ramos de Oliveira, John Andrew Sims, João Lameu, 2023, Chapter 7, 978-3-031-38742-5, 107, 10.1007/978-3-031-38743-2_7 | |
29. | Yanlu Chen, Buyun Xu, Yuzhou Cheng, Kun Luo, Jianren Fan, Meixiang Xiang, Hemodynamic differences caused by left atrial appendage modeling contours, 2023, 35, 1070-6631, 10.1063/5.0172261 | |
30. | Ahmed Qureshi, Paolo Melidoro, Maximilian Balmus, Gregory Y.H. Lip, David A. Nordsletten, Steven E. Williams, Oleg Aslanidi, Adelaide de Vecchi, MRI-based modelling of left atrial flow and coagulation to predict risk of thrombogenesis in atrial fibrillation, 2025, 101, 13618415, 103475, 10.1016/j.media.2025.103475 | |
31. | Henrik Aasen Kjeldsberg, Renate B. Schnabel, Joakim Sundnes, Kristian Valen-Sendstad, Estimation of inlet flow rate in simulations of left atrial flows: A proposed optimized and reference-based algorithm with application to sinus rhythm and atrial fibrillation, 2025, 00219290, 112594, 10.1016/j.jbiomech.2025.112594 |
Inlet boundaries | 0.4 s | 0.7 s | ||
AF | SR | AF | SR | |
Left Superior Pulmonary Vein | 26.86% | 27.88% | - | 24.49% |
Left Inferior Pulmonary Vein | 30.27% | 30.56% | - | 29.02% |
Right Superior Pulmonary Vein | 26.14% | 25.35% | - | 27.20% |
Right Inferior Pulmonary Vein | 16.74% | 16.22% | - | 19.29% |
AF: atrial fibrillation; SR: sinus rhythm. |