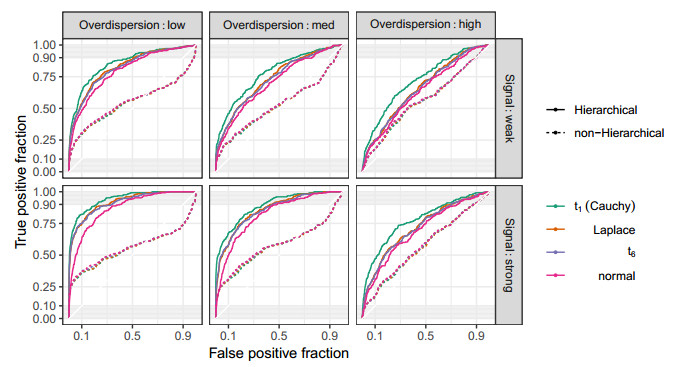
Citation: Ignacio Alvarez-Castro, Jarad Niemi. Fully Bayesian analysis of allele-specific RNA-seq data[J]. Mathematical Biosciences and Engineering, 2019, 16(6): 7751-7770. doi: 10.3934/mbe.2019389
[1] | Yonghua Zhuang, Kristen Wade, Laura M. Saba, Katerina Kechris . Development of a tissue augmented Bayesian model for expression quantitative trait loci analysis. Mathematical Biosciences and Engineering, 2020, 17(1): 122-143. doi: 10.3934/mbe.2020007 |
[2] | Dao-Peng Chen, Fangyuan Zhang, Shili Lin . AIJ: joint test for simultaneous detection of imprinting and non-imprinting allelic expression imbalance. Mathematical Biosciences and Engineering, 2020, 17(1): 366-386. doi: 10.3934/mbe.2020020 |
[3] | Saskya Mary Soemartojo, Titin Siswantining, Yoel Fernando, Devvi Sarwinda, Herley Shaori Al-Ash, Sarah Syarofina, Noval Saputra . Iterative bicluster-based Bayesian principal component analysis and least squares for missing-value imputation in microarray and RNA-sequencing data. Mathematical Biosciences and Engineering, 2022, 19(9): 8741-8759. doi: 10.3934/mbe.2022405 |
[4] | Yong Ding, Jian-Hong Liu . The signature lncRNAs associated with the lung adenocarcinoma patients prognosis. Mathematical Biosciences and Engineering, 2020, 17(2): 1593-1603. doi: 10.3934/mbe.2020083 |
[5] | Shaoyu Li, Su Xu, Xue Wang, Nilüfer Ertekin-Taner, Duan Chen . An augmented GSNMF model for complete deconvolution of bulk RNA-seq data. Mathematical Biosciences and Engineering, 2025, 22(4): 988-1018. doi: 10.3934/mbe.2025036 |
[6] | Jiaxin Luo, Lin Wu, Dinghui Liu, Zhaojun Xiong, Linli Wang, Xiaoxian Qian, Xiaoqiang Sun . Gene regulatory network analysis identifies key genes and regulatory mechanisms involved in acute myocardial infarction using bulk and single cell RNA-seq data. Mathematical Biosciences and Engineering, 2021, 18(6): 7774-7789. doi: 10.3934/mbe.2021386 |
[7] | Jinqi He, Wenjing Zhang, Faxiang Li, Yan Yu . Development of metastasis-associated seven gene signature for predicting lung adenocarcinoma prognosis using single-cell RNA sequencing data. Mathematical Biosciences and Engineering, 2021, 18(5): 5959-5977. doi: 10.3934/mbe.2021298 |
[8] | Sijie Lu, Juan Xie, Yang Li, Bin Yu, Qin Ma, Bingqiang Liu . Identification of lncRNAs-gene interactions in transcription regulation based on co-expression analysis of RNA-seq data. Mathematical Biosciences and Engineering, 2019, 16(6): 7112-7125. doi: 10.3934/mbe.2019357 |
[9] | Francisco Julian Ariza-Hernandez, Juan Carlos Najera-Tinoco, Martin Patricio Arciga-Alejandre, Eduardo Castañeda-Saucedo, Jorge Sanchez-Ortiz . Bayesian inverse problem for a fractional diffusion model of cell migration. Mathematical Biosciences and Engineering, 2024, 21(4): 5826-5837. doi: 10.3934/mbe.2024257 |
[10] | Kaiyu Shen, Shuaiyi Ke, Binyu Chen, Tiantian Zhang, Hongtai Wang, Jianhui Lv, Wencang Gao . Identification and validation of biomarkers for epithelial-mesenchymal transition-related cells to estimate the prognosis and immune microenvironment in primary gastric cancer by the integrated analysis of single-cell and bulk RNA sequencing data. Mathematical Biosciences and Engineering, 2023, 20(8): 13798-13823. doi: 10.3934/mbe.2023614 |
Over the past decade, RNA-sequencing (RNA-seq) has been replacing microarray technology as the primary high-throughput method used to measure gene expression [1]. In a biological sample, the amount of messenger RNA (transcript abundance) derived from a gene is known as the gene's expression level in that sample. For each gene, RNA-seq is a count positively correlated with the gene's transcript abundance. A diploid genome has two sets of chromosomes, one from each parent, so every gene has two copies. RNA-seq can be used to measure the expression of each gene copy separately when the two gene copies exhibit sequence differences. These two separate measures of expression for a single gene are known as allele-specific expression (ASE) measures, which can be obtained using single nucleotide polymorphism (SNPs) that makes it possible to distinguish the expression of the two alleles [2]. The study of ASE may provide some explanation for so-called heterosis effects. In plant breeding, phenotypic heterosis occurs when hybrid lines show improvements in several phenotype traits compared with their inbred parent lines [3]. Heterozygous hybrid varieties might take advantage of having two alleles with different genotypes in order to adapt to environmental conditions by promoting the selection of the superior allele. The uneven expression of alleles might be related to the superior adaptation of hybrids, so it might be related to the occurrence of gene heterosis [4,5]. Other biological questions where ASE is relevant may include identifying imprinting or parent-of-origin effects, which occurs in genes where only one parental allele is expressed, the distinction between cis-acting and trans-acting regulation DNA relies on ASE since cis-acting is associated with differentially expressed alleles while trans-acting has effects both alleles [2].
Several modelling strategies has been proposed to analyze ASE data. Given the total ASE, i.e., the sum of counts in both alleles, the so-called reference allele count can be modeled as binomially distributed [5], or use Beta-binomial distribution which includes gene-specific overdispersion [6,7,8,9]. Instead of modeling ASE counts based on a binomial distribution, it is possible to adapt models originally designed for dealing with total RNA-seq transcript abundance counts, Poisson [4], generalized Poisson [10,11] and negative binomial distributions [12] has been proposed. [13] provide an extensive review of the methods to detect differential expression for total RNA-seq data. Differentially expressed genes can be obtained applying a binomial test for each gene and adjusting p-values to control false discovery rate (FDR). Total RNA-seq expression and ASE can be combined to distinguish factors that affect the gene expression in an allele-specific manner (cis-QTL) from factors that affect the gene expression of the two alleles at the same time (trans-QTL). A likelihood ratio test distinguishes cis and trans regulation by combining ASE beta-binomial model with a model for the total RNA-seq counts [2]. The model is extended in [14] to incorporate isoform-specific information and haplotype modeling.
In this paper, a hierarchical overdispersed count regression model is proposed to study allele-specific expression. This modeling framework allows easy generalization to include additional genotypes, tissue types, and additional alleles. These more complex models will allow researches to address more complex biological questions. The method is applicable whenever heterozygous genotype expression is available. In cases with uncertainty about the genotype, an initial stage is needed to determine sites from heterozygous genotype before inference about ASE is possible.
The hierarchical aspect of the approach is very important, we learn the gene-specific parameters hierarchical distribution from data, i.e. perform full Bayesian inference. The proposed model is able to capture the key features of ASE data such as reference allele bias, and is more flexible in the modelling of biological sample random effects. In addition, fully Bayesian inference allow to detect relevant genes based on summaries of the posterior probability of being differentially expressed between alleles with no need of multiplicity corrections. The main problem to obtain full Bayesian inference in this problem is computational, we use GPU-accelerated algorithm to obtain posterior samples [15].
The next Section describe the main characteristic ASE data different from the total RNA-seq expression. Section 3 describes the statistical model we propose to analyze ASE data. Sections 4 and 5 presents results from a simulation study and a real ASE data set analysis, respectively. Finally, 6 presents a summary of the main findings and comments on the next steps in this line of research.
ASE counts from RNA-seq data are typically obtained by first mapping the short RNA-seq reads to a reference genome and then assigning those reads to a particular allele using known single nucleotide polymorphisms (SNPs). If no SNPs are known in a particular short read, then there is no ASE information for that read and that read cannot be assigned to a particular allele. The proportion of genes having some ASE information available depends on genomic similarity and RNA-seq read length [2].
Assume ASE counts are available for a single variety BM whose parents are the varieties B and M. The ASE counts are formed by two transcript abundance counts per gene each sample. In plant breeding experiments, it is common that the parental varieties, are inbred lines and thus haplotypes are known. While we focus on a plant breeding experiment where the parents are inbred lines with haplotypes known, the methodology could be utilized whenever sample are taken from a single variety and there are two possible alleles for each gene corresponding to two different varieties.
To set some ideas, we perform an initial data exploration letting mga be the mean expression level for allele a∈{B,M} of gene g∈{1,…,G} over all available samples. Then let Ag=mgB+mgM be the average gene abundance and Rg=mgB/mgM be the allele ratio. Figure 1, based on plant breeding experiment more fully described in Section 5, illustrates some characteristics usually present in ASE gene transcript abundance data using the summary measures Ag and Rg.
The left panel in Figure 1 presents a histogram of Rg with a reference Gaussian density constructed using the sample mean and sample variance. The empirical distribution of Rg is more concentrated around 0 and has heavier tails than a normal distribution. This characteristic is not exclusive to ASE counts, differential expression measures in total RNA-seq counts and microarrays are typically similar.
The right panel in Figure 1 shows a two-dimensional histogram of the (Ag,Rg) pairs. The most frequent cells are close to zero allele difference (black line) for any level of average ASE suggesting that most of the genes have small differences in the ASE counts. In addition, more genes fall above zero allele difference than below and the gene-wide average ratio (red line) is also positive suggesting that allele B has higher ASE counts than allele M on average.
While there could be some biological reason to observe one of the alleles more expressed than the other one on average, it is known the ASE process can result in increased counts, on average, for the reference genome [16].
The reference genome is (almost) fully known, and many times it is not possible to distinguish mismatches due to errors from genuine mismatches due to the read corresponding to a non-reference genome. A read that truly matches the reference genome is more likely to be counted than a read matching the non-reference genome, creating a bias towards reference allele counts. Alternative ASE processes can be implemented to eliminate this reference genome bias [17,18,19]. Alternatively, a conservative analysis would be to only consider those genes with significant allele imbalance against the reference allele [4]. In the following section, we develop methodology in the modeling stage that allows the analysis to adjust for this bias.
We introduce a hierarchical overdispersed count regression model for the allele-specific counts for each sample and borrows information across genes to learn about gene-specific parameters. To estimate parameters we will utilize a Markov chain Monte Carlo (MCMC) approach utilizing an overall Gibbs sampling structure with slice sampling for intractable conditional distributions. To ameliorate the computational difficulties of sampling from the high dimensional posterior, we utilize an algorithm constructed to run on a graphics processing unit (GPU) which provides within-iteration acceleration of the algorithm [15].
Each hybrid sample in the experiment has two sub-samples: one with RNA-seq counts for allele B and one with RNA-seq counts for allele M.
Let Ygn be the allele-specific RNA-seq count of gene g in sub-sample n for g={1,2,…,G} and n={1,2,…,N}. Let X by an N×P model matrix that contains information regarding which allele each sub-sample is associated with as well as any additional relevant experimental conditions and let xn be the nth row of X. We follow the approach of [15] and assume
Ygnind∼Po(hnex⊤nβg+ϵgn),ϵgnind∼N(0,γ2g) | (3.1) |
where hn is a measure of the library size for sub-sample n and βg and γg are gene-specific model parameters.
There might be many columns in the model matrix X specific to particular applications, for instance to represent blocking factors or relevant covariate effects. However, there are three columns that should be present in models dealing with ASE counts. We assume the first column corresponds to an intercept term and denote its associated coefficient as βg,1. Moreover, we assume the second model matrix column take value 1 for observed counts from the reference allele and the value -1 for observed counts of the non-reference allele. Then, the regression coefficient associated with the second column, βg,2, represents the half difference of gene ASE, genes with βg,2=0 providing evidence of equally expressed alleles. Lastly, if there is more than one biological replicate (which is usually the case), a third column should be included to represent the grouping effect of the allele-specific sub-sampling. We assume this effect it corresponds to the last column in X, its associated coefficient is βg,P.
The ϵgn terms provide gene-specific overdispersion through a normal hierarchical distribution with mean 0 and variance γ2g. This effect implies a quadratic mean-variance relationship that could differ across genes, and admits the partition of the total gene variability into technical and biological components similar to the Poisson-gamma mixture [20].
As we have many genes, but generally few biological samples, we wish to borrow information across the genes about the gene-specific parameters βg and γg. One feature common to RNA-seq and ASE counts is that, in many cases, the large effect of interest are only present for a small group of genes while the remaining genes have small to negligible effects as demonstrated in the left panel of Figure 1. This pattern can be modeled using shrinkage distributions, i.e. distributions that have more mass around the location parameter but with heavier tails. Several of these distributions can be written as a scale mixture of normals, i.e. βgpind∼N(θp,σ2pξgp) and ξgpind∼p(ξ) where the marginal distribution for βgp can be normal, Student-t, Laplace [21], or horseshoe distribution [22] by assuming a point-mass, inverse-gamma, exponential, and half-Cauchy distribution for the ξgp, respectively.
A second set of gene-specific parameters are the normal variances that control overdispersion, γg. We model these variances as independent inverse-gamma distributions conditional on ν and τ, and independent from the regression coefficients βg, i.e. γgind∼IG(ν/2,ντ/2). With this parametrization, we have E(1/γg)=1/τ and the coefficient of variation is CV(γg)=√2/(ν−4), and therefore τ is related to the location of the distribution while ν controls the amount of shrinkage around that location.
Prior distribution for the hyperparameters of regression coefficients are set as normals for the means, θkind∼N(0,ck), and uniform for the standard deviations σkind∼Unif(0,sk) [23]. Parameters controlling overdispersion effect have uniform prior, ν∼Unif(0,d), and gamma prior, τ∼Ga(a,b).
Normal prior for location parameters θk is widely used choice [24], it can be weakly informative maintaining conditional conjugacy. Similarly the gamma prior for a location-related parameter τ represents a good balance between computation convenience and being weakly informative. The prior parameters, indicated with Roman letters, are set to obtain diffuse distributions. As the number of genes is very large, there is a lot of information about the hyperparameters in the data and thus, these diffuse priors will not have a large impact on the hyperparameter estimation [25].
Models where gene-specific parameters have fully specified distributions, i.e. non-hierarchical models, can be estimated using MCMC methods [26]. However, fully Bayesian inference of high-dimensional hierarchical models is computationally demanding since the number of groups (or genes) is large. Usually, approximations like empirical Bayes [27] or integrated nested Laplace approximation [28] are used to obtain inference results. We instead utilize the fbseq package [29] which uses graphics processing units (GPUs) to take advantage of the embarrassingly parallel MCMC steps and parallel reductions in each iteration of the MCMC algorithm, convergence is assessed using potential scale reduction factor statistic. For computational reasons, fbseq provides posterior means and standard deviations for gene-specific parameters and full MCMC samples for all hyperparameters and few gene-specific parameters.
An important characteristic present in some ASE data is to observe a higher transcription for one of the alleles on average across all genes, due to the positive bias towards the reference allele mentioned in Section 2 and observed in Figure 1. These systematic difference among alleles are not of interest as the goal is to identify genes showing differences among allele larger than what is explained by systematic factors.
We consider the overall mean across all genes, θ2, as a measure of the systematic difference among alleles commonly due to bias towards the reference allele. Then, we define the allele effect to be the difference between alleles that is not due to bias, i.e. Δg=βg2−θ2. Since this is a function of a gene-specific parameter and a hyperparameter, we are not able to obtain the posterior distribution directly from the fbseq output.
In order to obtain inference about the gene-specific regression parameters, the posterior mean and variance from the MCMC samples can be used to create a normal approximation of its posterior distribution [15]. A similar strategy could be used to obtain credible intervals for the allele effect, Δg. In this case the posterior mean and variance of Δg are
E(Δg|y)=E(βg2|y)−E(θ2|y)Var(Δg|y)=Var(βg2|y)+Var(θ2|y)−2Cov(βg2,θ2|y) |
As we mentioned before, fbseq does not compute this covariance. However, the variability of the hyperparameters is negligible compared to the variability of the gene-specific parameters, i.e. Var(Δg|y)≈Var(βg2|y) since Var(θ2|y)−2Cov(βg2,θ2|y)<<Var(βg2|y). Therefore, a normal approximation for Δg has mean E(Δg|y) and variance Var(βg2|y). We show this approximation is reasonable in Figure 6 in supplemental material.
The main goal of the proposed model is to identify genes with differentially expressed alleles (DEA). We say a gene has DEA when |Δg|≥c where c>0 represents a threshold that must be adapted to specific applications or experiments. Here we follow [30] in setting as the DEA threshold a 25% increase in the expression level, i.e., c=log(1.25)/2.
Unfortunately, P(|Δg|≥c|y) will be large for genes with large posterior uncertainty for Δg even when E(Δg|y)≈0. To avoid this issue, we use the statistic pg=min{P(Δg<c),P(Δg>−c)} where small pg provide evidence in favor of DEA [28].
A Bayesian false discovery rate correction has been proposed [28,31]. However, since we use a fully hierarchical Bayesian model and the null hypothesis has a positive probability multiplicity corrections are not needed [32,33]. Alternatively, we could minimize the following expected loss
E[L(d,y)]=q∑gdg(1−pg)+∑g(1−dg)pg=qFD+FN |
where dg is an indicator that gene g has DEA, FD and FN are the posterior expected false discoveries and false negatives respectively, and q is the relative cost associated to FD. [34] shows the optimal rule that minimizes E[L(d,y)] is
dg=I(pg≤1q+1). |
Setting q=19 we would declare as DEA every gene with pg lower than 5%.
A simulation study is performed to explore how the model captures several characteristics of interest in the data, and evaluate model performance in finding genes where the allele effect is present. In this Section, we describe the data sets simulation scenarios, the analysis of each simulated data, and present simulation study results.
In order to obtain simulated data sets close to the real data we have, we fit an initial model and use it to simulate new data. We obtain point estimates of the gene-specific regression coefficients and gene-specific overdispersion parameters using edgeR [12]. In addition, we obtain normalization factors h∗n based on the method proposed by [35]. These point estimates and normalization values are used to obtain the simulated data sets. This corresponds to a negative binomial model for the ASE counts, Ygnind∼NB(h∗nex⊤nβg,ϕg), where h∗gn are normalization factors and ϕg control the overdispersion.
The specific {data set} we use later in Section 5 as an application example, has 8 allele-specific observations per gene, corresponding to 4 biological replicates of a single hybrid genotype distributed in two blocks. Let yg=(yg1,…,yg8) such that yg1 and yg2 represent the ASE counts for the two alleles from the first sample, yg3 and yg4 represent the ASE counts for the two alleles from the second sample, etc. In order to obtain approximate independence among regression coefficients (which is an assumption in hierarchical distributions), we use a zero-sum parametrization for X as shown in Eq (4.1).
X=[111101−1110111−101−11−1011−1011−1−10111−10−11−1−10−1] | (4.1) |
This particular choice of X matrix implies βg1 corresponds to the intercept and βg2 to the half allele difference, as in the general model presented previously. Here we include a column to capture the difference between the two blocks, associated with coefficient βg3. Also, two columns for block and replicate interaction, (β4g,β5g) are included, which represent the half difference between replicates within each block. Note that usually the set of effects related with grouping factors as biological samples, share a common variance, while the model proposed here allows σ4≠σ5 which encompasses the common variance case.
A simulation scenario is defined by four simulation design parameters: sparsity (w) and strength of the allele effect (s), bias toward reference allele (p), and overdispersion effects (T). Table 1 shows the design parameters values, in total there are 24 scenarios as a full factorial combination of the design parameters values. Each scenario is formed by the simulated ASE count for G=5000 genes with 8 observations per gene, and is replicated 2 times.
Description | Sparsity | Strength | Bias | Overdispersion |
Parameter | w | s | p | T |
Values | .5,.95 | 1,1.8 | 1,.5 | 0.25,1,4 |
The estimates (h∗n,ˆβg1,ˆβg2,ˆϕg) from NB model described above are used to obtain simulated data set. We construct two groups of genes depending on whether the estimated allele difference is larger than a threshold, |ˆβg2|>c or not, and we obtain a stratified random sample with (1−w) proportion of genes with large allele difference. With the selected genes, we obtain 8 counts per gene as follows:
Ygnind∼NB(ˉheEgn,Tˆϕg)Egn=ˆβg1+ψ(s)xaˆβg2+log(p)I(xa=−1) |
where ˉh is the average of normalization factors, ψ(s)=s when |ˆβg2|>c and ψ(s)=1 in other case, and xa takes value 1 for the reference allele and −1 in the non-reference allele. The design parameter s controls the signal strength, we set s=(1,1.8) as weak and strong signal cases respectively. Lastly, overdispersion effects are computed as Tˆϕg, three overdispersion scenarios are determined by the value of T=(.25,1,4).
Reference allele bias is created by the log(p) factor, to better understand why this might match with the biologic characteristic of this effect consider an intermediate step where Y∗gn is a simulated count without bias (i.e. log(p)=0) and then Ygn∼Bin(Y∗gn,p) when xa=−1, integrating out Y∗gn we can recover the negative binomial distribution. Design parameter p is the probability of actually assigning one short read to the non-reference allele, so on average (1−p) non-reference reads are lost. This implies that the mean of βg2 coefficients, θ2, should be close to −log(p)/2 since βg2 captures the gene-specific half difference among the two alleles and log(p) represent the allele between alleles averaging all genes. But not necessarily for each individual count, since the initial negative binomial simulation is independent for the two alleles within a gene.
Every simulated data set is analyzed using data model (3.1) with five hierarchical distributions with normal scale mixture described in 3.2. We use normal, Laplace, Student-t6, Cauchy (Student-t1), and horseshoe. The main reason for this is to assess the impact of the hierarchical distribution of the regression coefficients on the posterior inference. We ran three MCMC chains with 40,000 iterations with thinning value of 5 in Cauchy and horseshoe cases. Still, horseshoe distribution shows lack of convergence in many scenarios for θk parameters, therefore we do not present horseshoe distribution simulation results nor consider it for the real data analysis. It have beem recently pointed out that horseshoe distribution may have poor mixing in high-dimensional problems, and propose to use an elliptical slice sampler to improve it [36].
Additionally, we fit a non-hierarchical counterpart for each version of the proposed model, i.e., fixing hyperparameters values so distribution for gene-specific parameter is no longer learned from data. In non-hierarchical Bayesian models are set with values θk=0, σ2k=32, τ=.1, ν=1, and inference is obtained with 3 MCMC chains with 20000 iterations and no thinning.
Figure 2 presents receiver operating characteristic (ROC) curves for only one replicate in simulation scenarios where only 5% of genes are truly DEA and reference allele bias is present. ROC curves are computed with plotROC package [37]. Statistic pg is used is used as a continuous score to compute the ROC curves, we set DEA threshold in c=log(1.25)/2, as in [30]. Results indicates that increasing the signal strength and decreasing the overdispersion level produce better detection rates for all methods. The non-hierarchical models fail into account the bias towards reference allele. Among hierarchical models, Figure 2 suggests a Cauchy distribution for gene-specific regression parameters has slightly better detection rates (other simulation scenarios present similar patterns).
Several alternative methods are used to analyze each simulated data set for performance comparison with model (3.1) results. First, we use a Poisson generalized linear model with overdispersion and biological sample effects, for each gene, the model is estimated via maximum likelihood. Second, a beta-binomial distribution is used to perform a likelihood ratio test for each gene. Finally, negative binomial model is estimated via empirical Bayes methods using edgeR package [12]. In all these three methods a p-value is obtained from testing if each gene shows evidence of DEA and then a false discovery rate correction is applied, using the method proposed in [38].
ROC curves can be summarize computing the area under the ROC curve (AUC), a perfect detection rate would have AUC value of 1. Figure 3 shows AUC measure results only for hierarchical Bayesian models and the three alternative methods just described. The facets combine the signal strength level and sparsity level (columns) with the presence of reference allele bias (rows), overdispersion level is represented by the the color and type of the lines. Each line corresponds to one simulation scenario.
Similarly to Figure 2 overdispersion level and signal strength have the largest impact on the signal detection performance measured with AUC across all models. There might be some interaction among the simulation design factors, for instance, signal strength shows almost no effect in AUC when overdispersion level is high.
Figure 3 shows that Cauchy as hierarchical distribution for the regression parameters have the largest AUC measure in most simulation scenarios, particularly when 95% of gene having true effect lower than the threshold. Cauchy accommodates a lot of probability mass close to zero and its heavy tails can capture the genes with real effects. Performance of Laplace and t distributions appear to be slightly worst than Cauchy models. This might suggest degrees of freedom parameter in student-t distribution impact how the model borrows information across genes. Next, using a normal distribution for regression coefficients has the poorest AUC results among Bayesian hierarchical models, and its lower than edgeR method for most cases. The empirical Bayes method (edgeR) shows equal (or slightly better) AUCs than hierarchical models in many scenarios while is somewhat worse in highly sparse and weak signal cases. Finally, the two frequentist methods shows lowest AUC measures in every simulation scenario, and are specially affected by the presence of reference allele bias.
We finish this Section showing how the proposed model captures the bias towards reference allele. Above we mentioned that parameter θ2 should capture half of the bias in log scale, i.e., we expect i.e. E(θ2|y)≈−log(p)/2. Figure 4 shows a scatter plot of E(θ2|Y) against log(p). The plot suggests posterior expectation of θ2 captures the bias towards the reference allele, is possible to use it as an estimate of the bias and remove it when making inference about the allele effect.
In this Section we apply the methods described in Section 3 to a RNA-seq data set with allele-specific counts from maize that constitute a portion of the experimental data obtained by [4]. Data set includes four replicate samples of a hybrid genotype (B73xMo17) distributed in 2 flow cell blocks and two allele count measures per sample. RNA-seq transcript abundance count information for 39656 genes is obtained using Iliumna® technology and B73 genome as the reference allele [39]. However, many of them have little or no ASE information. To avoid genes with extremely low observed expression, only use genes were the average of allele-specific counts is bigger than 1. The resulting data set corresponds to the ASE counts of 16380 genes, which is 41% of the total.
An initial exploration of this data, based on expression averages per gene, was presented in Section 2 to illustrate the main features of an ASE data set, here we use the expression for each replicate without averaging. The specific model matrix we use is the same presented in (4.1) used to create a model to simulate data. Gene-specific intercept is normally distributed while the rest of regression parameters are Cauchy distributed. The choice of βgk∼Ca(θk,σk) is based on the results from simulation study, the models using Cauchy hierarchical distribution results in better partial AUC measures in particular in sparse cases or cases with large overdispersion levels.
We present the main results from the analysis, we start with some remarks about the posterior inference relative to the hyperparameters of the model, and after that we focus on the results relative to gene-specific allele effects. We present credible intervals for Δg and identify genes differentially expressed between alleles.
Table 2 presents posterior summaries for all hyperparameter in the model. Posterior means and credible interval for (ν,τ) suggest most genes show very little or none overdispersion present, but there are a few genes with large overdispersion effects. Posterior mean of θ2 is positive representing the bias towards reads from B73 allele. The results suggest that expression from Mo17 allele is only 78% of the expression count from allele B73 on average across all genes. In other words, 1 out of 5 reads from Mo17 is lost presumably because is compared with a different genome. Finally, results suggest the variances of common biological sample effects are different with σ24>σ25 by a factor of 100.
Parameter | Posterior Mean | Credible Interval (95%) |
ν | 3.6 | (3,4.3) |
τ | 0.0023 | (0.0019, 0.0028) |
θ1 | 2.4 | (2.4, 2.4) |
θ2 | 0.12 | (0.12, 0.13) |
θ3 | -0.025 | (-0.029, -0.021) |
θ4 | -0.026 | (-0.029, -0.024) |
θ5 | 0.002 | (0.00015, 0.0038) |
σ21 | 1.7 | (1.7, 1.8) |
σ22 | 0.012 | (0.011, 0.013) |
σ23 | 0.013 | (0.013, 0.014) |
σ24 | 0.0011 | (0.00094, 0.0012) |
σ25 | 0.000015 | (0.0000095, 0.000023) |
Figure 5 shows allele effect posterior inference results for each gene. Left panel presents 95% credible intervals of allele effects against expected gene expression with color highlighting genes with differentially expressed alleles. The expected gene expression (in logs) is computed as βg1+hn, i.e. the posterior mean of the gene-specific intercept plus the offset value. Genes with large expected expression show smaller allele effects and shorter credible intervals than genes with low expression. There are some genes flagged as differentially expressed among alleles with very low expression level.
The right panel of Figure 5 shows the observed allele ratio (Rg) against the evidence of DEA measured as 1−pg, color of the points represents the total ASE observed count. This figure relates model results with observed data, it suggest the model results are reasonable given the observed data. Genes with large absolute value of the allele ratio presents larger probabilities of having DEA, there also some genes with relatively small allele effect but with large probability, this occurs when the total expression level is high (darker points). Additionally Figure 10 (in supplemental material) shows the allele effects estimated by the model are highly correlated with the observed allele ratio with some shrinkage towards zero value, this behavior is expected for hierarchical models.
Results indicate that 17% of the genes shows allele differential expression. When the observed allele ratio in logs is negative (favor the non-reference allele), the list of genes with DEA is contained within the list of genes previously flagged by [4]. There are many genes flagged in [4] that are discarded by our proposed model. This makes sense because we define a region of non-differential expression instead of a point value, so this smaller proportion of DEA is reasonable since the null region is larger. On the other hand, genes where the observed allele ratio favor the reference allele were previously discarded, our analysis flagged is capable to find genes high probability of DEA since correct the reference bias from the allele effect.
Allele-specific expression refers to a transcript abundance count associated with each gene copy (allele). We propose a hierarchical overdispersed count regression model for ASE data from heterozygous genotypes to detect genes with differential expression between alleles. This model address the main characteristics of ASE data. A measure of allele effect corrected for reference allele bias and a method to obtain credible intervals for this measure are described. The proposed statistical method can be applied to multi-allelic scenarios or situations that require to model total and allele expression simultaneously. Model inference is performed in a fully Bayesian fashion. The specific MCMC algorithm is embarrassingly parallel when updating the gene-specific parameters. A parallel strategy computing is then used for computational efficiency.
Simulation experiments suggested there are performance gains in learning the hierarchical distributions of gene-specific parameter from data. Hierarchical Bayesian models show slightly better performance than empirical Bayes approach and much better results than frequentist and non-hierarchical methods. Non-hierarchical Bayesian models performance is more heavily affected by to overdispersion and sparsity level and cannot accommodate the reference allele bias. Caution is needed for the comparison with frequentist methods since they are calibrated for p-values distribution under a point mass null hypothesis, but the simulated data had small but different from zero effects in non-DEA genes. Among hierarchical models, the better performance results in terms of signals detection were showed by Cauchy distribution. Cauchy is informative enough to produce information sharing across genes and at the same time is flexible enough (due to the heavy tails) to accommodate large true signals.
A real data set is analyzed with the Poisson hierarchical model, using Cauchy as hierarchical distribution for gene specific regression parameters. The application consist in ASE data from four maize hybrid plants. We found evidence of DEA for 17% of the genes, results are consistent with previous analysis of the same data, our method allow to reference bias thus some of the genes were not consider before, otherwise our is somewhat more conservative. The model suggest variances of biological samples are different for each sample, the usual corrections with random effects restrict these variances to be equal. Could be relevant to explore the consequences of this restriction in the model results.
As we mentioned earlier, the method proposed in the paper could serve as the base for a more general model. Some relevant generalizations are straightforward to incorporate. A careful construction of the design matrix (4.1) the only piece needed to include more varieties (other genomes) and total RNA-seq expression or to deal with multiple alleles data. These generalizations allow to study more relevant contrast other than differential expression among alleles. For instance, in plant breeding applications, we could study allelic imbalance, i.e. compare the allele expression ratio in hybrid with the total expression ratio among parental lines, and the relationship among hybrid vigor and allelic imbalance. Other generalizations maybe harder to incorporate, in order to work under uncertainty about the genotype, a new model stage is needed. It could be possible to use a finite mixture of Poisson distribution in (3.1), where the mixing probabilities corresponds to the probability of each genotype.
Horseshoe distribution results showed lack-of-convergence problems so it was excluded from the proposed models. Recently, it was pointed out the poor mixing of a horseshoe implementation based on a scale mixture of normals (which is the one used in this work) and propose to use an elliptical slice sampler instead in [36]. We would like to continue working analyzing the effect of the elliptical sample for the horseshoe distribution in the proposed models.
This research was supported by National Institute of General Medical Sciences (NIGMS) of the National Institutes of Health and the joint National Science Foundation / NIGMS Mathematical Biology Program under award number R01GM109458. The content is solely the responsibility of the authors and does not necessarily represent the official views of the National Institutes of Health or the National Science Foundation.
The authors declare there is no conflict of interest.
Allele effect variance approximation
In Section 3 we stated that Var(Δg|y)≈Var(βg2|y), we can test the approximation from a few genes having the complete MCMC samples. Figure 6 presents scatter plots of the variance of the allele effect against the variance of the regression coefficient βg2, the facets represents the hierarchical distribution used in the model and color of points represent the overdispersion level. There is a close relationship among the two plotted variances, suggesting the approximation Var(Δg|y)≈Var(βg2|y) is reasonable.
ROC curves for complementary scenarios
Figures 7, 8 and 9 shows ROC curves for simulation scenarios that complete the scenarios presented in the main text (Figure 2). In all three figures, row facets corresponds to overdispersion level, while column facets combine signal strength and bias. Hierarchical models are plotted with continuous lines and dashed lines correspond to non-hierarchical models. Line color indicates the hierarchical. distribution.
Allele effects in real data analysis
Figure 10 illustrates the relationship among estimated allele effects (Δg) and the observed data.
[1] | S. Datta and D. Nettleton, Statistical Analysis of Next Generation Sequencing Data, Springer, 2014. Available from: http://link.springer.com/content/pdf/10.1007/978-3-319-07212-8.pdf. |
[2] | W. Sun and Y. Hu, Mapping of expression quantitative trait loci using RNA-seq data, in Statistical Analysis of Next Generation Sequencing Data (eds. D. Nettleton and S. Datta), 2014, 25–50. |
[3] | P. S. Schnable and N. M. Springer, Progress toward understanding heterosis in crop plants, Annu. Rev. Plant Biol., 64 (2013), 71–88. |
[4] | A. Paschold, Y. Jia, C. Marcon, et al., Complementation contributes to transcriptome complexity in maize (Zea mays L.) hybrids relative to their inbred parents., Genome Res., 22 (2012), 2445–2454. |
[5] | G. D. M. Bell, N. C. Kane, L. H. Rieseberg, et al., RNA-Seq analysis of allele-specific expression, hybrid effects, and regulatory divergence in hybrids compared with their parents from natural populations, Genome Biol. Evol., 5 (2013), 1309–1323. |
[6] | J. K. Pickrell, J. C. Marioni, A. A. Pai, et al., Understanding mechanisms underlying human gene expression variation with rna sequencing, Nature, 464 (2010), 768–772. |
[7] | W. Sun and Y. Hu, eQTL Mapping Using RNA-seq Data, Stat. Biosci., 5 (2013), 198–219. |
[8] | C. T. Harvey, G. A. Moyerbrailean, G. O. Davis, et al., Quasar: quantitative allele-specific analysis of reads, Bioinformatics, 31 (2014), 1235–1242. |
[9] | N. Raghupathy, K. Choi, M. J. Vincent, et al., Hierarchical analysis of RNA-seq reads improves the accuracy of allele-specific expression, Bioinformatics, 34 (2018), 2177–2184. |
[10] | S. Srivastava and L. Chen, A two-parameter generalized Poisson model to improve the analysis of RNA-seq data., Nucleic Acids Res., 38 (2010), e170. |
[11] | X. Wei and X. Wang, A computational workflow to identify allele-specific expression and epigenetic modification in maize., Genom. Proteom. Bioinf., 11 (2013), 247–252. |
[12] | M. D. Robinson, D. J. McCarthy and G. K. Smyth, edgeR: a Bioconductor package for differential expression analysis of digital gene expression data., Bioinformatics (Oxford, England), 26 (2010), 139–140. |
[13] | D. J. Lorenz, R. S. Gill, R. Mitra, et al., Using RNA-seq Data to Detect Differentially Expressed Genes, in Statistical Analysis of Next Generation Sequencing Data (eds. S. Datta and D. Nettleton), 2014, chapter 2, 25–49. |
[14] | Y.-J. Hu, W. Sun, J.-Y. Tzeng, et al., Proper use of allele-specific expression improves statistical power for cis -eQTL mapping with RNA-seq data, J. Am. Stat. Assoc., 110 (2015), 962–974. |
[15] | W. Landau, J. Niemi and D. Nettleton, Fully bayesian analysis of rna-seq counts for the detection of gene expression heterosis, J. Am. Stat. Assoc., 114 (2019), 601–612. |
[16] | N. I. Panousis, M. Gutierrez-Arcelus, E. T. Dermitzakis, et al., Allelic mapping bias in RNA-sequencing is not a major confounder in eQTL studies, Genome. Biol., 15 (2014), 467. |
[17] | J. F. Degner, J. C. Marioni, A. A. Pai, et al., Effect of read-mapping biases on detecting allele-specific expression from RNA-sequencing data, Bioinformatics, 25 (2009), 3207–3212. |
[18] | R. Vijaya Satya, N. Zavaljevski and J. Reifman, A new strategy to reduce allelic bias in RNA-Seq readmapping, Nucleic Acids Res., 40 (2012), 1–9. |
[19] | K. R. Stevenson, J. D. Coolon and P. J. Wittkopp, Sources of bias in measures of allele-specific expression derived from RNA-sequence data aligned to a single reference genome., BMC Genom., 14 (2013), 536. |
[20] | Y. Chen, A. T. L. Lun and G. K. Smyth, Differential expression analysis of complex RNA-seq experiments using edgeR, in Statistical Analysis of Next Generation Sequencing Data, Springer, Cham, 2014, 51–74. |
[21] | T. Park and G. Casella, The Bayesian lasso, J. Am. Stat. Assoc., 103 (2008), 681–686. |
[22] | C. M. Carvalho, N. G. Polson and J. G. Scott, Handling Sparsity via the Horseshoe, J. Mach. Learn. Res., 5 (2009), 73–80. |
[23] | A. Gelman, Prior distributions for variance parameters in hierarchical models, Bayesian Analysis, 1 (2006), 515–533. |
[24] | A. Gelman, J. B. Carlin, H. S. Stern,et al., Bayesian Data Analysis, CRC press, 2013. |
[25] | J. K. Ghosh, M. Delampady and T. Samanta, An Introduction to Bayesian Analysis, Springer, 2006. Available from: http://onlinelibrary.wiley.com/doi/10.1002/9781118684818.ch16/summary. |
[26] | L. G. León-Novelo, L. M. McIntyre, J. M. Fear, et al., A flexible Bayesian method for detecting allelic imbalance in RNA-seq data, BMC Genom., 15 (2014), 920. |
[27] | J. Niemi, E. Mittman, W. Landau, et al., Empirical Bayes analysis of RNA-seq data for detection of gene expression heterosis, J. Agr. Biol. Envir. St., 20 (2015), 614–628. |
[28] | M. A. Van De Wiel, G. G. R. Leday, L. Pardo, et al., Bayesian analysis of RNA sequencing data by estimating multiple shrinkage priors, Biostatistics, 14 (2013), 113–128. |
[29] | W. Landau and J. Niemi, A fully Bayesian strategy for high-dimensional hierarchical modeling using massively parallel computing, 2016. Available from: http://arxiv.org/abs/1606.06659. |
[30] | A. Lithio and D. Nettleton, Hierarchical modeling and differential expression analysis for RNA-seq experiments with inbred and hybrid genotypes, J. Agr. Biol. Envir. St., 20 (2015), 598–613. |
[31] | M. Ventrucci, E. M. Scott and D. Cocchi, Multiple testing on standardized mortality ratios: a Bayesian hierarchical model for FDR estimation, Biostatistics, 12 (2011), 51–67. |
[32] | P. Muller, G. Parmigiani and K. Rice, FDR and Bayesian multiple comparisons ules, 2006. Available from: http://biostats.bepress.com/jhubiostat/paper115. |
[33] | H. Y. Bar, J. G. Booth and M. T. Wells, A bivariate model for simultaneous testing in bioinformatics data, J. Am. Stat. Assoc., 109 (2014), 537–547. |
[34] | P. Müller, G. Parmigiani, C. Robert, et al., Optimal sample size for multiple testing: the case of gene expression microarrays, J. Am. Stat. Assoc., 99 (2004), 990–1001. |
[35] | S. Anders and W. Huber, Differential expression analysis for sequence count data, Genome Biol., 11 (2010), R106. |
[36] | P. R. Hahn and J. He, Elliptical slice sampling for Bayesian shrinkage regression with applications to causal inference, 2016. Available from: http://faculty.chicagobooth.edu/richard. hahn/JCGS_submit.pdf. |
[37] | M. C. Sachs, plotROC: A tool for plotting roc curves, J. Stat. Software, 79 (2017), 1–19. |
[38] | Y. Benjamini and Y. Hochberg, Controlling the false discovery rate: A practical and powerful approach to multiple testing, J. R. Stat. Soc-B, 57 (1995), 289–300. |
[39] | P. S. Schnable, D. Ware, R. S. Fulton, et al., The B73 maize genome: complexity, diversity, and dynamics, Science, 326 (2009), 1112–1115. |
1. | Joshua P. Zitovsky, Michael I. Love, Fast effect size shrinkage software for beta-binomial models of allelic imbalance, 2020, 8, 2046-1402, 2024, 10.12688/f1000research.20916.2 |
Description | Sparsity | Strength | Bias | Overdispersion |
Parameter | w | s | p | T |
Values | .5,.95 | 1,1.8 | 1,.5 | 0.25,1,4 |
Parameter | Posterior Mean | Credible Interval (95%) |
ν | 3.6 | (3,4.3) |
τ | 0.0023 | (0.0019, 0.0028) |
θ1 | 2.4 | (2.4, 2.4) |
θ2 | 0.12 | (0.12, 0.13) |
θ3 | -0.025 | (-0.029, -0.021) |
θ4 | -0.026 | (-0.029, -0.024) |
θ5 | 0.002 | (0.00015, 0.0038) |
σ21 | 1.7 | (1.7, 1.8) |
σ22 | 0.012 | (0.011, 0.013) |
σ23 | 0.013 | (0.013, 0.014) |
σ24 | 0.0011 | (0.00094, 0.0012) |
σ25 | 0.000015 | (0.0000095, 0.000023) |
Description | Sparsity | Strength | Bias | Overdispersion |
Parameter | w | s | p | T |
Values | .5,.95 | 1,1.8 | 1,.5 | 0.25,1,4 |
Parameter | Posterior Mean | Credible Interval (95%) |
ν | 3.6 | (3,4.3) |
τ | 0.0023 | (0.0019, 0.0028) |
θ1 | 2.4 | (2.4, 2.4) |
θ2 | 0.12 | (0.12, 0.13) |
θ3 | -0.025 | (-0.029, -0.021) |
θ4 | -0.026 | (-0.029, -0.024) |
θ5 | 0.002 | (0.00015, 0.0038) |
σ21 | 1.7 | (1.7, 1.8) |
σ22 | 0.012 | (0.011, 0.013) |
σ23 | 0.013 | (0.013, 0.014) |
σ24 | 0.0011 | (0.00094, 0.0012) |
σ25 | 0.000015 | (0.0000095, 0.000023) |