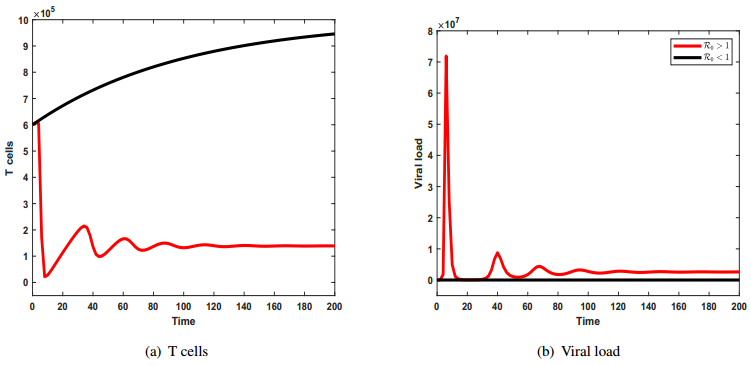
Citation: Damilola Olabode, Libin Rong, Xueying Wang. Optimal control in HIV chemotherapy with termination viral load and latent reservoir[J]. Mathematical Biosciences and Engineering, 2019, 16(2): 619-635. doi: 10.3934/mbe.2019030
[1] | Tinevimbo Shiri, Winston Garira, Senelani D. Musekwa . A two-strain HIV-1 mathematical model to assess the effects of chemotherapy on disease parameters. Mathematical Biosciences and Engineering, 2005, 2(4): 811-832. doi: 10.3934/mbe.2005.2.811 |
[2] | Divya Thakur, Belinda Marchand . Hybrid optimal control for HIV multi-drug therapies: A finite set control transcription approach. Mathematical Biosciences and Engineering, 2012, 9(4): 899-914. doi: 10.3934/mbe.2012.9.899 |
[3] | Jinliang Wang, Xiu Dong . Analysis of an HIV infection model incorporating latency age and infection age. Mathematical Biosciences and Engineering, 2018, 15(3): 569-594. doi: 10.3934/mbe.2018026 |
[4] | Urszula Ledzewicz, Shuo Wang, Heinz Schättler, Nicolas André, Marie Amélie Heng, Eddy Pasquier . On drug resistance and metronomic chemotherapy: A mathematical modeling and optimal control approach. Mathematical Biosciences and Engineering, 2017, 14(1): 217-235. doi: 10.3934/mbe.2017014 |
[5] | Gordon Akudibillah, Abhishek Pandey, Jan Medlock . Optimal control for HIV treatment. Mathematical Biosciences and Engineering, 2019, 16(1): 373-396. doi: 10.3934/mbe.2019018 |
[6] | Patrick W. Nelson, Michael A. Gilchrist, Daniel Coombs, James M. Hyman, Alan S. Perelson . An Age-Structured Model of HIV Infection that Allows for Variations in the Production Rate of Viral Particles and the Death Rate of Productively Infected Cells. Mathematical Biosciences and Engineering, 2004, 1(2): 267-288. doi: 10.3934/mbe.2004.1.267 |
[7] | Damilola Olabode, Libin Rong, Xueying Wang . Stochastic investigation of HIV infection and the emergence of drug resistance. Mathematical Biosciences and Engineering, 2022, 19(2): 1174-1194. doi: 10.3934/mbe.2022054 |
[8] | Gesham Magombedze, Winston Garira, Eddie Mwenje . Modelling the immunopathogenesis of HIV-1 infection and the effect of multidrug therapy: The role of fusion inhibitors in HAART. Mathematical Biosciences and Engineering, 2008, 5(3): 485-504. doi: 10.3934/mbe.2008.5.485 |
[9] | Divine Wanduku . A nonlinear multi-population behavioral model to assess the roles of education campaigns, random supply of aids, and delayed ART treatment in HIV/AIDS epidemics. Mathematical Biosciences and Engineering, 2020, 17(6): 6791-6837. doi: 10.3934/mbe.2020354 |
[10] | Bing Li, Yuming Chen, Xuejuan Lu, Shengqiang Liu . A delayed HIV-1 model with virus waning term. Mathematical Biosciences and Engineering, 2016, 13(1): 135-157. doi: 10.3934/mbe.2016.13.135 |
The human immuno-deficiency virus (HIV) infection has been a most significant infectious disease seen in the history of human health. Since the inception of the disease in the 1980s, the high spreading speed of infection around the world has been a great concern to the public. By the end of 2016, approximately 36.7 million people have been reported worldwide to be living with HIV, and about 1 million people died from HIV related illnesses [19]. Although tremendous efforts have been made in the prevention, intervention and control of HIV, its pandemic remains a major socio-economic burden particularly in underdeveloped countries. Without any treatment, it has been shown that the progression of HIV to AIDS (acquired immune deficiency syndrome) usually takes about 10 years on average [19].
It is extremely challenging to eradicate the disease. Some studies indicate that the infection may lay dormant in reserviors [7,11,38]. One popular treatment that came up to help manage the infection is known as highly active antiretroviral therapy (HAART). The HAART is a combination of antiretroviral agents*. This therapy has been utilized to suppress viral replication in the bloodstream for decades thereby increasing the life expectancy of individual living with HIV [3].
*These drugs are classed as nucleoside/nucleotide reverse transcriptase inhibitors (NRTIs), non-nucleoside reverse transcriptase inhibitors (NNRTIs), protease inhibitors, entry inhibitors (including fusion inhibitors), integrase inhibitors, chemokine co-receptor antagonists (CCR5 antagonists), cytochrome P4503A (CYP3A) inhibitors.
Mathematical modeling, analysis and simulations for HIV have long provided useful insights into disease dynamics that could guide the public health administration for designing effective prevention and control measures against the disease (e.g., see [2,10,20,22,23,24,25,28,29,31] and the references therein). Most of the modeling work is built on a system of ordinary differential equations (ODEs). For instance, in 1993, Perelson et al. used an ODE model of HIV to study the effects of azidothymidine (AZT), a nucleoside reverse transcriptase inhibitor, on the viral growth and the T-cell population dynamics by showing the dependence of endemic level of infected T cells on the model parameters [24]. In 2009, Rong and Perelson proposed a model that included a logistic term representing homeostatic proliferation of latently infected cells and then investigated the quantitative and integrated prospective in terms of long-term dynamics of HIV and the latent reservoir under a potent antiretroviral therapy [27]. In 2016, Wang et al. developed an HIV latent model with two modes of transmission and they analyzed the local and global dynamics of the system [34].
It is crucial to study the treatment from the control perspective. First of all, the virus can become drug resistant overtime. Secondly, the drugs used in the therapy can be dangerous to patients' health if the dosage is not well proportioned. For example, one of such drugs is the reverse transcriptase inhibitors (RTIs)†. These types of drugs can lead to a harmful side effect in patients such as cardiovascular problems, lactic acidosis and mitochondrial damage due to uncontrolled dosage overtime [5,33]. A number of mathematical models have been developed to study optimal control of HIV infection [12,13,15,16,30]. In 1997, Kirschner et al. used an existing ODE model from [24] to investigate an optimal chemotherapy strategy for HIV infection, and their objective function was based on the number of T-cell counts and the systemic cost in the optimality system [17]. In 2015, Ana-Maria Croicu considered an objective function that aims to minimize both the viral load in the blood stream and the control cost [9]. To the best of our knowledge, few works have taken the termination viral load and latent reservoir into account in the control of HIV infection.
†They are used to interfere with the process of the HIV, an RNA virus, to be reverse transcribed from RNA to DNA with the help of the enzyme reverse transcriptase.
In this paper, we incorporate the termination level and the running cost of viral load, latently and actively infected T cells over the treatments into the objective function. Our goal is to decrease the infected T cells (both latently and actively infected T cells), the viral load in the blood stream (which will cause an increase in the number of CD4+ T cells) and to reduce the cost of treatment. Specifically, we consider two drug classes, the RTIs and the protease inhibitor (PIs)‡.
‡These drugs act on infected cells by inhibiting the cleavage of protein precursors with the help of enzyme protease, which is required to produce and package new viruses that emerge from an infected cell and can infect other susceptible T cells.
The rest of the paper is organized as follows. Section 2 introduces the model we used. Section 3 presents the basic reproduction number and summarizes the local and global dynamics of the system obtained in [21]. Section 4 defines the objective function, verifies the necessary condition of the optimal system and presents the existence and uniqueness of the optimal solution. Section 5 is devoted to our numerical results. Final concluding remark and discussions are provided in Section 6.
The model we will study in the paper can be written as a system of ODEs:
dTdt=λ−dTT−(1−ϵRT)kVIT,dLdt=αL(1−ϵRT)kVIT−dLL−aL,dT∗dt=(1−αL)(1−ϵRT)kVIT−δT∗+aL,dVIdt=(1−ϵPI)NδT∗−cVI. | (1) |
The variable T represents the number the susceptible CD4+ T cells, L (resp. T∗) measures the number of the latently (resp. productively) infected CD4+ T cells, and VI is the concentration of free virus in the blood stream. ϵRT(t) and ϵPI(t) capture the time-dependent efficacy of of RT and protease inhibitors, respectively. The definition of model parameters is provided in Appendix A. For simplicity, let X=(T,L,T∗,VI)T and F denote the right hand of (1). Hence, system (1) is of the form dX/dt=F(X,ϵRT,ϵPI).
With constant controls, the dynamics of this system have been analyzed by Pankavish in [21] based on the basic reproduction number R0, which is defined as the expected number of secondary cases produced by one primary case in an otherwise susceptible population.
It follows from [21] that the basic reproduction number associated with system (1) is given by
R0=dL(1−ϵ)kλN(1−αL)+a(1−ϵ)kλNcdT(dL+a), |
where (1−ϵ)=(1−ϵRT)(1−ϵPI). Moreover, system (1) has at most two biologically feasible equilibria which depend on the value of R0. The non infectious equilibrium (NIE) (T,L,T∗,VI)=(λdT,0,0,0) always exists, and the endemic equilibrium (EE)
(T,L,T∗,VI)=(λdTR0,αLR0(dL+a)λ(R0−1),dTc(1−ϵ)kNδ(R0−1),(1−ϵPI)dTk(1−ϵ)(R0−1)) |
is the only feasible and nontrivial equilibrium when R0>1. The following summarizes Pankavich's results in [21], which establish the local and global dynamics of system (1).
Theorem 3.1. If R0≤1, then the NIE is locally asymptotically stable. If R0>1 then the NIE is an unstable saddle point, and the EE is locally asymptotically stable.
Theorem 3.2. If R0≤1, then the NIE is globally asymptotically stable. If R0>1, then the EE is globally asymptotically stable.
These results show that R0 serves as a sharp infection threshold; specifically, if the basic reproduction number is less than or equal to the unity, the infection dies out, whereas if the basic reproduction number is above the unity, the infection will persist and become established.
The disease threshold dynamics are numerically verified in terms of the basic reproduction number R0. The result is illustrated in Figure 1.
In this section, we introduce and analyze an optimal control problem for HIV infection. Our goal is to find an effective control treatment. Let tf denote the termination time of investigation. We consider the model on the time interval [0,tf]. The objective functional we consider is given by
J=∫tft0A1VI(t)dt+∫tft0A2L(t)dt+∫tft0A3T∗(t)dt+A4VI(tf)+A5L(tf)+A6T∗(tf)+∫tft0(B1ϵRT(t)+B2ϵ2RT(t))dt+∫tft0(C1ϵPI(t)+C2ϵ2PI(t))dt. | (2) |
The first three integrals measure the total number of The first three integrals measure the total number of viral load, latently and productively infected cells, respectively, over the entire investigation time. VI(tf),L(tf) and T∗(tf) are the corresponding termination level of each population. The last two integrals consist of the overall cost of two types of antiretroviral drugs, where the quadratic terms are introduced to account for the nonlinear cost potentially arising at high treatment levels [4]. The constants A1,A2,A3,A4,A5,A6,B1,B2,C1, and C2 define the weights associated with the corresponding controls.
Our major goal is to minimize the total viral load, the termination viral load and latent reservoir, and the control cost over the investigation time. To that end, we consider
min(ϵRT,ϵPI)∈UJ(ϵRT,ϵPI), |
subject to
dXdt=F(X,ϵRT,ϵPI), |
where U={ϵ(t)=(ϵRT(t),ϵPI(t)):ϵ is measurable,aRT≤ϵRT(t)≤bRT,aPI≤ϵPI(t)≤bPI,t∈[0,tf]} is the control set. Here aRT,bRT, aPI and bPI are nonnegative constants with aRT<bRT and aPI<bPI.
In this section, we will focus on the necessary condition for the optimality system. To proceed, we will use the Pontryagin's Maximum Principle [26] to seek the optimal control solution. This method introduces the adjoint variables and represents the optimal control solution in terms of state and adjoint variables, which transfers the problem of minimizing the objective functional into a problem that minimizes the Hamiltonian in terms of controls.
Theorem 4.1. Assume that B2>0 and C2>0. Given the optimal control ϵ∗RT and ϵ∗PI, and the solution X∗ of the corresponding state system in (1), there exists the adjoint variable Ψ =(ψ1,ψ2,ψ3,ψ4)T satisfying:
ψ′1=ψ1(dT+(1−ϵRT)kVI)−ψ2(αL(1−ϵRT)kVI)−ψ3((1−αL)(1−ϵRT)kVI),ψ′2=−A2+ψ2(dL+a)−ψ3a,ψ′3=−A3+ψ3δ−ψ4(1−ϵPI)Nδ,ψ′4=ψ1(dT+(1−ϵRT)kT)−ψ2(αL(1−ϵRT)kT)−ψ3((1−αL)(1−ϵRT)kT)+ψ4c, |
with
Ψ(tf)=(0,A5,A6,A4). |
Moreover, the optimal control is given by
ϵ∗RT=min{max{aRT,((ψ2−ψ3)αLkVIT+(ψ3−ψ1)kVIT−B1)2B2},bRT} |
and
ϵ∗PI=min{max{aPI,(ψ4(NδT∗)−C1)2C2},bPI}. |
Proof. Based on the result from Pontryagrin's Maximum principle, we will construct an Hamiltonian. Let H be the Hamiltonian of the optimality system given by
H=H(t,T,L,T∗,VI,ψ1,ψ2,ψ3,ψ4,ϵRT,ϵPI)=A1VI+A2L+A3T∗+B1ϵRT+B2ϵ2RT+C1ϵPI+C2ϵ2PI+ψ1[λ−dTT−(1−ϵRT)kVIT]+ψ2[αL(1−ϵRT)kVIT−dLL−aL]+ψ3[(1−αL)(1−ϵRT)kVIT−δT∗+aL]+ψ4[(1−ϵPI)NδT∗−cVI]. |
To solve the optimal control, the adjoint variable must satisfy the following system, Ψ′=−∇XH; that is,
ψ′1=−∂H∂T=ψ1(dT+(1−ϵRT)kVI)−ψ2(αL(1−ϵRT)kVI)−ψ3((1−αL(1−ϵRT)kVI),ψ′2=−∂H∂L=−A2+ψ2(dL+a)−ψ3(a),ψ′3=−∂H∂T∗=−A3+ψ3(δ)−ψ4((1−ϵPI)Nδ),ψ′4=−∂H∂VI=−A1+ψ1((1−ϵRT)kT)−ψ2(αL(1−ϵRT)kT)−ψ3((1−αL)(1−ϵRT)kT)+ψ4(c). |
Using the transversality condition, we have
(T(tf),L(tf),T∗(tf),VI(tf))=(0,A5,A6,A4). |
The optimal solution in the interior of the control set U is determined by
∂H∂ϵRT=B1+2B2ϵRT+ψ1kVIT−ψ2αLkVIT−ψ3(1−αL)kVIT=0,∂H∂ϵPI=C1+2C2ϵPI−ψ4(NδT∗)=0. |
Thus in the interior of U, we get
ϵ∗RT=(ψ2−ψ3)αLkVIT+(ψ3−ψ1)kVIT−B12B2, | (3) |
ϵ∗PI=ψ4NδT∗−C12C2. | (4) |
Furthermore, by the classical control argument that involves bounds on controls, we conclude that,
ϵ∗RT={(ψ2−ψ3)αLkVIT+(ψ3−ψ1)kVIT−B12B2=:vRT ifaRT<vRT<bRT,aRT ifvRT≤aRT,bRT ifvRT≥bRT. |
Thus,
ϵ∗RT=min{max{aRT,((ψ2−ψ3)αLkVIT+(ψ3−ψ1)kVIT−B1)2B2},bRT}. |
Similarly for the second control we have
ϵ∗PI={ψ4NδT∗−C12C2=:vPI ifaPI<vPI<bPI,aPI ifvPI≤aPI,bPI ifvPI≥bPI, |
and hence
ϵ∗PI=min{max{aPI,(ψ4(NδT∗)−C1)2C2},bPI}. |
In addition, the Hessian matrix of Hamiltonian in term of ϵRT and ϵPI is positively definite, which ensures that (3) is a minimizer. It completes the proof.
In this section, our investigation will be focused on the existence and uniqueness of our optimal control problem.
Theorem 4.2. Consider the control problem with the state equations (1), there exists an ϵ∗=(ϵ∗RT,ϵ∗PI)∈U such that
min(ϵRT,ϵPI)∈UJ(ϵRT,ϵPI)=J(ϵ∗RT,ϵ∗PI), |
where U={ϵ(t)=(ϵRT(t),ϵPI(t)):ϵ is measurable,aRT≤ϵRT(t)≤bRT,aPI≤ϵPI(t)≤bPI,t∈[0,tf]} is the control set.
Proof. By Theorem 4.1 [13], our assertion on the existence of the optimality system holds if the following conditions are satisfied:
C1. {(X(0),ϵ∗):ϵ(t)∈U}≠∅;
C2. The admissible control set U is closed and convex;
C3. The right hand side of the state system is bounded by a linear function in the state and control variable.
C4. The integrand of the objective functional J (i.e., A1VI+A2L+A3T∗+B1ϵRT+B2ϵ2RT+C1ϵPI+C2ϵ2PI) is convex on U.
C5. There exist constants K1 and K2 such that the integrand of the objective function is bounded below by K1(|ϵRT|β+|ϵPI|β)−K2 where K1>0 and β>1.
The verification of C1 and C3 for the state equation follows directly from the result of [21,Theorem 3.1] for the existence, positivity and the boundedness of the ODE solutions. The verification of C2 follows directly from the construction and definition of the optimal control, which ensures the compactness required for the optimal control. C4 is satisfied since the integrand is convex on the control set. C5 follows immediately by taking K1=min{(B1+B2),(C1+C2)}, K2=0 and β=2. Hence, the existence of the optimal control pair is proved.
To prove the uniqueness of the system we shall invoke the lemma below.
Lemma 4.3. Let the function ϵ∗(s) be defined as ϵ∗(s)=min(max(s,a),b) for some positive constants a<b. Then ϵ∗(s) is Liptschitz continuous in s.
Proof. Let s1,s2∈R and a,b be positive constant. To prove the assertion for min(max(s,a),b), it suffices to show that Liptchitz continuity holds for max(s,a).
1. s1≥a,s2≥a;|max(s1,a)−max(s2,a)|=|s1−s2|.
2. s1≥a,s2≤a;|max(s1,a)−max(s2,a)|=|s1−a|≤|s1−s2|.
3. s1≤a,s2≥a;|max(s1,a)−max(s2,a)|=|a−s2|≤|s1−s2|.
4. s1≤a,s2≤a;|max(s1,a)−max(s2,a)|=|a−a|=0≤|s1−s2|.
Since this is true for all cases, hence the Lipschitz continuity is satisfied for ϵ∗ in s.
Theorem 4.4. Given that tf is sufficiently small, the solution to the bounded optimality system is unique.
This theorem can be proved by the classical energy method [12,15].
The standard forward-backward sweep method is used to solve the optimality system in an iterative manner [14]. In this paper we adopt the Runge-Kutta 4 for the sweep method. This method solves the state system with an initial guess forward in time and then solves the adjoint system backward in time using the transversality condition as the initial guess, the controls are then updated after each iteration using the formulas for the optimal control derived in Theorem 4.1. This iteration is continued until convergence is achieved.
In what follows, we compare the results by using two objective functionals. The first objective function has been introduced in previous research [9] in the optimal control of HIV infection. Note that the model in [9] is a basic viral dynamic model without consideration of latently infected CD4+ T cells, and it uses a different objective functional. We want to compare the optimal control with and without including the termination level of viral load and latent reservoir.
J∗=∫tft0˜A1VI(t)dt+∫tft0(˜B1ϵRT(t)+˜B2ϵ2RT(t))dt+∫tft0(˜C1ϵPI(t)+˜C2ϵ2PI(t))dt. | (5) |
The parameter values used in the numerical simulations are provided in Table 1. The time unit is the day. The weight factors are set as follows: ˜A1=A1=0.02,A2=10,A3=10,A4=10,A5=10,A6=20 with ˜B1=B1=8,˜B2=B2=9,˜C1=C1=11,˜C2=C2=13. These values are chosen to balance the weights of variables in the objective function to be minimized. For example, because the magnitude of the viral load in the objective function is significantly greater than the magnitude of the drug efficacy, the choice of weights is chosen to balance the difference. Weights for the termination terms are chosen as a result of emphasis made for the viral load and the level of latently infected cells in the termination. The weights for the running cost and productively infected cells are based on the size of infected cells (both latent and active) [1,9]. Here the weight parameters with and without tilde are for the objective functionals (5) and (2), respectively.
Variable | Units | Discription | Value | Ref. |
λ | mL−1 day−1 | recruitment rate of T from the thymus | 10000 | [6] |
dT | day−1 | death rate of T | 0.01 | [18] |
dL | day−1 | death rate of L | 0.004 | [6] |
δ | day−1 | death rate of T∗ | 1 | [35] |
c | day−1 | clearance rate of V | 23 | [36] |
N | RNA copies cell−1 | burst rate of T∗ | 3000 | [24,29] |
a | day−1 | activation rate of L | 0.1 | [28] |
αL | day−1 | proportion of L | 0.001 | [32] |
k | mL day−1 | rate of infection of T | 2.4×10−8 | [24] |
T0 | cell mL−1 | initial value of T | 600000 | [37] |
T∗0 | cell mL−1 | initial value of T∗ | 0.3 | [28] |
L0 | cell mL−1 | initial value of L | 2 | [28] |
VI0 | RNA copies mL−1 | initial value of VI | 50 | [28] |
Unlike the objective functional J∗ defined in (5), the objective function J presented in this paper defined in (2) includes the termination level. The viral load at the termination time is of great interest because suppressing the viral load to below the detection limit is a major objective of current antiretroviral treatment. The pool size of latently infected cells at the termination time may also play a vital role in predicting a rapid viral rebound to the pretreatment level or post-treatment control [8], i.e., patients can maintain undetectable viral load for a prolonged time without a therapeutic treatment. As seen in Figure 2, for the first 40 days of treatment period, both objective functionals were able to increase the number of T cells. However, using objective functional J, the T-cell count keeps increasing, whereas the number of T cells using the objective functional J∗ fluctuated through the rest of the treatment period. Regarding the viral load in the blood stream, the objective functional J resulted in a very low level of virus by the end of the treatment period and the decrease was consistent throughout the treatment period, while with J∗, the level of viral load fluctuated and between 55th day and the 70th day behaved almost the same as no treatment. The dynamics of the latently and productively infected cells had the similar results to the viral load for the two objective functions (see Figure 2). The corresponding controls are displayed in Figure 3. It shows that the amount of drug used for J is higher than that of J∗ from day 1 till day 59, but between the 60th day and 118th day of the regimen, the amount of drug given for J was less than that of J∗. Overall, the effort on the control for J∗ is more than that of J.
Using the objective functional presented in this paper and the combination weight factor A1=0.02,A2=10,A3=10,A4=10,A5=10,A6=20,B1=8,B2=9,C1=11,C2=13. we study the optimal control problem for tf=30 days, 60 days, and 120 days. The obtained results are plotted in Figures 4–9.
In what follows, for simplicity, the first (resp. second) control is referred to as the control using the RTIs (resp. PIs). In the 30-day period of treatment, it is observed from Figure 4 that the susceptible T cells increased, whereas the latently infected cells, the actively infected cells and the viral load decreased throughout the treatment. This is consistent with the result of the controls. The first control started out with an efficacy averaging 70 percent for the first 5 days, while the second control was not administered for that period of time. Then, over the course of the treatment, the efficacy of the first control decreased averaging 55 percent till the end of the regimen, while the efficacy of the second control increased. The highest efficacy for the second control over this treatment period was about 35 percent. The increase in the second control agrees with the fact that it was needed to incapacitate the virus in the blood stream so that the virions will not be able to successfully infect cells in the body. This treatment was able to consistently increase the number of T cells in the body with the viral load in the blood stream at 29 RNA copies/mL which is below the detection level.
We compare this with the 60-day treatment (see Figures 6 and 7) and the following were observed. The number of T cells consistently increased throughout the treatment period, the number of the latently infected cells decreased, the actively infected cells and the viral load all decreased till the end of the treatment period. The level of actively infected cells was very low with the viral load in the bloodstream at about 0.15 RNA copies/mL by the end of the treatment, which is much less than that of the 30-day treatment period. In the control used in the 60-day treatment period, the first control started out by averaging 70 percent in the first 5 days at this time and the second drug was again not administered. Between the 5th and the 10th day, the first control averaged about 65 percent and the second control averaged about 10 percent. From the 10th day till the end of the treatment period, the first control averaged about 40 percent while the second control increased and averaged about 35 percent till the end of the treatment period.
Lastly, we considered a longer period of time 120 days (see Figures 8 and 9). In this case, the number of T cells increased throughout the treatment period, while the latently infected cells, actively infected cells, viral load all decreased throughout the treatment period. By the end of the treatment, the viral load was about 0.00083 RNA copies/mL. In the control for the 120 days, the first control started out by averaging 55 percent in the first 10 days, and the second control averaging out 30 percent. By the 30th day of the treatment, both controls were maintained at about 36 percent till the end of the treatment period.
In this paper, we formulate an optimal control problem for the chemotherapy treatment in HIV patients by deriving the necessary condition of the optimal control pair, using the Pontryagin's maximum principle. Our result shows that including the terminal state of virus and infected cells in the objective functional, particularly the viral load and latent reservoir at the termination time, leads to an effective optimal control in two folds: (1) It causes the number of uninfected T cells in the blood stream to increase consistently; (2) it also decreases the level of free virus in the bloodstream and keeps the viral load below the detection level (50 RNA copies/mL). Moreover, the obtained optimal control indicates that the RT inhibitor should begin with strong dosing scheme while the protease inhibitor can start with little or no dosage for the first few days of the treatment period. In the days following, the dosage for RI inhibitor can decrease while that of the protease inhibitor should increase, and they should be averaged about the same level till the end of the treatment period.
There are a couple of limitations in our work. Firstly, the control in this study is continuous over time and thus it not very practical since the implementation cost will be high, which we did not incorporate in our study. A way to handle this is to introduce a piece-wise linear control for the model. Secondly, the model we consider in this paper is a basic model with HIV latency. For instance, taking multi-scale (within host and between host) and mutation of the virus into account in the model formulation will better reflect HIV dynamics over a longer time interval or when drug resistance emerges in patients. It would be interesting to compare the corresponding difference in optimal controls.
L. Rong's research is supported in part by the National Science Foundation (DMS-1758290). This work is partially supported by a grant from the Simons Foundation (No. 317407, X. Wang). The authors thank the editor and anonymous referees for their valuable comments that improved this paper.
The authors declare there is no conflict of intetest.
[1] | B. M. Adams, H. T. Banks, M. Davidian, H. D. Kwon, H. T. Tran, S. N. Wynne and E. S. Rosenberg, HIV dynamics: modeling, data analysis, and optimal treatment protocols, J. Comput. Appl. Math., 185 (2005), 10–49. |
[2] | S. Alizon and C. Magnus, Modeling the course of HIV infection: Insight from ecology and evolution, Viruses, 4 (2012), 1984–2013. |
[3] | E. J. Arts and D. J. Hazuda, HIV-1 antiretroviral drug therapy, Cold Spring Harb. Perspect. Med., 2 (2012), a007161. |
[4] | E. Asano, L. J. Gross, S. Lenhart and L. A. Real, Optimal control of vaccine distribution in a rabies metapopulation model, Math. Biosci. Eng., 5 (2008), 219–238. |
[5] | K. Brinkman, H. J. M.Ter Hofstede, D. M. Burger, J. A. M. Smeitink and P. P. Koopmans, Adverse effects of reverse transcriptase inhibitors: mitochondrial toxicity as common pathway, AIDS, 12 (1998), 1735–1744. |
[6] | D. S. Callaway and A. S. Perelson, HIV-1 infection and low steady state viral loads, Bull. Math. Biol., 64 (2002), 29–64. |
[7] | T. W. Chun, L. Stuyver, S. B. Mizell, L. A. Ehler, J. A. Mican, M. Baseler, A. L. Lloyd, M. A. Nowak and A. S. Fauci, Presence of an inducible HIV-1 latent reservoir during highly active antiretroviral therapy, Proc. Natl. Acad. Sci. USA, 94 (1997), 13193–13197. |
[8] | J. M. Conway and A. S. Perelson, Post-treatment control of HIV infection, Proc. Natl. Acad. Sci. USA, 112 (2015), 5467–5472. |
[9] | A. M. Croicu, Short and long term optimal control of a mathematical model for HIV infection of CD4+ T cells, Bull. Math. Biol., 77 (2015), 2035–2071. |
[10] | N. Dorratoltaj, R. Nikin-Beers, S. M. Ciupe, S. G. Eubank and K. M. Abbas, Multi-scale immunoepidemiological modeling of within-host and between-host HIV dynamics: systematic review of math model, Peer J, 5 (2017), e3877. |
[11] | D. Finzi, M. Hermankova, T. Pierson, L. M. Carruth, C. Buck, R. E. Chaisson, T. C. Quinn, K. Chadwick, J. Margolick, R. Brookmeyer, J. Gallant, M. Markowitz, D. D. Ho, D. D. Richman and R. F. Siliciano, Identification of a reservoir for HIV-1 in patients on highly active antiretroviral therapy, Science, 278 (1997), 1295–1300. |
[12] | K. R. Fister, S. Lenhart and J. McNally, Optimizing chemotherapy in an HIV model, Electron. J. Differ. Equ., 32 (1998), 1–12. |
[13] | W. H. Fleming and R. W. Rishel, Deterministic and stochastic optimal control, 1st edition, Springer-Verlag, New York, 1975. |
[14] | W. K. Hackbush, A numerical method for solving parabolic equations in opposite orientations, Computing, 20 (1978), 229–240. |
[15] | H. R. Joshi, Optimal control of an HIV immunology model, Optim. Control Appl. Methods, 23 (2002), 199–213. |
[16] | J. Karrakchou, M. Rachik and S. Gourari, Optimal control and infectiology: Application to an HIV/AIDS model, Appl. Math. Comput., 177 (2006), 807–818. |
[17] | D. Kirshner, S. Lenhart and S. Serbin, Optimal control of the chemotherapy of HIV, J. Math. Biol., 35 (1997), 775–792. |
[18] | H. Mohri, S. Bonhoeffer, S. Monard, A. S. Perelson and D. D. Ho, Rapid turnover of T lymphocytes in SIV-infected rhesus macaques, Science 279 (1998), 1223–1227. |
[19] | D. Morgan, C. Mahe, B. Mayanja, J. Okongo and R. Lubega, HIV-1 infection in rural Africa: Is there a difference in median time to aids and survival compared with that in industrialized countries?, AIDS, 16 (2002), 597–632. |
[20] | M. J. Pace, L. Agosto, E. H. Graf and U. O'Doherty, HIV reservoirs and latency models, Virology, 411 (2011), 344–354. |
[21] | S. Pankavish, The effects of latent Infection on the dynamics of HIV, Differ. Equ. Dyn. Syst., 24 (2016), 281–303. |
[22] | A. Pegu, M. Asokan, L. Wu, K. Wang, Activation and lysis of Human CD4 cells latently infected with HIV-1, Nat. Commun., 6 (2015). |
[23] | A. S. Perelson and P. Nelson, Mathematical analysis of HIV–1 in vivo, SIAM Rev., 41 (1998), 3–44. |
[24] | A. S. Perelson, D. E. Kirschner and R. D. Boer, Dynamics of HIV infection CD4 T cells, Math. Biosci., 114 (1993), 81–125. |
[25] | N. Perera, Deterministic and stochastic models of virus dynamics, Ph.D. Thesis, Texas Tech University, 2003. |
[26] | L. S. Pontryagin, V. G. Boltyanskii, R. V. Gamkrelidze and E. F. Mishchenko, The mathematical theory of optimal processes, 1st edition, John Wiley, 1962. |
[27] | L. Rong and A. S. Perelson, Modeling latently infected cell activation: Viral and latent reservoir persistence, and viral blips in HIV-infected patients on potent therapy, PLoS Comput. Biol., 5(10) (2009), 1000533. |
[28] | L. Rong and A. S. Perelson, Modeling HIV persistence, the latent reservoir, and viral blips, J. Theort. Biol., 260 (2009), 308–331. |
[29] | L. Rong and A. S. Perelson, Asymmetric division of activated latently infected cells may explain the decay kinetics of the HIV-1 latent reservoir and intermittent viral blip, Math. Biosci., 217 (2009), 77–87. |
[30] | M. Roshanfekr, M. H. Farahi and R. Rahbarian, A different approach of optimal control on an HIV immunology model, Ain. Shams. Eng. J., 5 (2014), 213–219. |
[31] | R. F. Siliciano and W. C. Greene, HIV latency, Cold Spring Harb. Perspect. Med., 1 (2011), a007096. |
[32] | L. E. Jones, A. S. Perelson, Transient viremia, plasma viral load, and reservoir replenishment in HIV-infected patients on antiretroviral therapy. J Acquir. Immune. Defic. Syndr., 45 (2007), 483–493. |
[33] | U.S. Department of health and human services AIDSinfo, Antiretroviral Therapy-Associated Common and/or Severe Adverse Effects, Available from: https://aidsinfo.nih.gov/ guidelines/htmltables/1/5551 |
[34] | X. Wang, S. Tang, X. Song and L. Rong, Mathematical analysis of an HIV latent infection model including both virus-to-cell infection and cell-to-cell transmission, J. Biol. Dynam., 11(sup2) (2016), 455–483. |
[35] | M. Markowitz, M. Louie, A. Hurley, E. Sun, M. Di Mascio, A. S. Perelson and D. D. Ho, A novel antiviral intervention results in more accurate assessment of human immunodeficiency virus type 1 replication dynamics and T-cell decay in vivo, J. Virol., 77 (2003), 5037–5038. |
[36] | B. Ramratnam, S. Bonhoeffer, J. Binley, A. Hurley, L. Zhang, J. E. Mittler, M. Markowitz, J. P. Moore, A. S. Perelson and D. D. Ho, Rapid production and clearance of HIV-1 and hepatitis C virus assessed by large volume plasma apheresis. Lancet, 354 (1999), 1782–1785. |
[37] | H. Kim and A. S. Perelson. Viral and latent reservoir persistence in HIV-1- infected patients on therapy, PLoS Comput. Biol., 2 (2006), e135. |
[38] | J. K. Wong, M. Hezareh, H. F. Gunthard, D. V. Havlir, C. C. Ignacio, C. A. Spina and D. D. Richman, Recovery of replication-competent HIV despite prolonged suppression of plasma viremia, Science, 278 (1997), 1291–1295. |
1. | Pooya Aavani, Linda J.S. Allen, The role of CD4 T cells in immune system activation and viral reproduction in a simple model for HIV infection, 2019, 75, 0307904X, 210, 10.1016/j.apm.2019.05.028 | |
2. | Arezoo Vafamand, Navid Vafamand, Jafar Zarei, Roozbeh Razavi-Far, Mehrdad Saif, Multi-objective NSBGA-II control of HIV therapy with monthly output measurement, 2021, 68, 17468094, 102561, 10.1016/j.bspc.2021.102561 | |
3. | Amedeo De Nicolò, Alessandra Manca, Alice Ianniello, Alice Palermiti, Andrea Calcagno, Micol Ferrara, Miriam Antonucci, Jessica Cusato, Valeria Avataneo, Elisa De Vivo, Stefano Bonora, Francesco Giuseppe De Rosa, Giovanni Di Perri, Antonio D’Avolio, Development and Validation of an Up-to-Date Highly Sensitive UHPLC-MS/MS Method for the Simultaneous Quantification of Current Anti-HIV Nucleoside Analogues in Human Plasma, 2021, 14, 1424-8247, 460, 10.3390/ph14050460 | |
4. | Sha He, Sanyi Tang, Qimin Zhang, Libin Rong, Robert A. Cheke, Modelling optimal control of air pollution to reduce respiratory diseases, 2023, 458, 00963003, 128223, 10.1016/j.amc.2023.128223 |
Variable | Units | Discription | Value | Ref. |
λ | mL−1 day−1 | recruitment rate of T from the thymus | 10000 | [6] |
dT | day−1 | death rate of T | 0.01 | [18] |
dL | day−1 | death rate of L | 0.004 | [6] |
δ | day−1 | death rate of T∗ | 1 | [35] |
c | day−1 | clearance rate of V | 23 | [36] |
N | RNA copies cell−1 | burst rate of T∗ | 3000 | [24,29] |
a | day−1 | activation rate of L | 0.1 | [28] |
αL | day−1 | proportion of L | 0.001 | [32] |
k | mL day−1 | rate of infection of T | 2.4×10−8 | [24] |
T0 | cell mL−1 | initial value of T | 600000 | [37] |
T∗0 | cell mL−1 | initial value of T∗ | 0.3 | [28] |
L0 | cell mL−1 | initial value of L | 2 | [28] |
VI0 | RNA copies mL−1 | initial value of VI | 50 | [28] |
Variable | Units | Discription | Value | Ref. |
λ | mL−1 day−1 | recruitment rate of T from the thymus | 10000 | [6] |
dT | day−1 | death rate of T | 0.01 | [18] |
dL | day−1 | death rate of L | 0.004 | [6] |
δ | day−1 | death rate of T∗ | 1 | [35] |
c | day−1 | clearance rate of V | 23 | [36] |
N | RNA copies cell−1 | burst rate of T∗ | 3000 | [24,29] |
a | day−1 | activation rate of L | 0.1 | [28] |
αL | day−1 | proportion of L | 0.001 | [32] |
k | mL day−1 | rate of infection of T | 2.4×10−8 | [24] |
T0 | cell mL−1 | initial value of T | 600000 | [37] |
T∗0 | cell mL−1 | initial value of T∗ | 0.3 | [28] |
L0 | cell mL−1 | initial value of L | 2 | [28] |
VI0 | RNA copies mL−1 | initial value of VI | 50 | [28] |