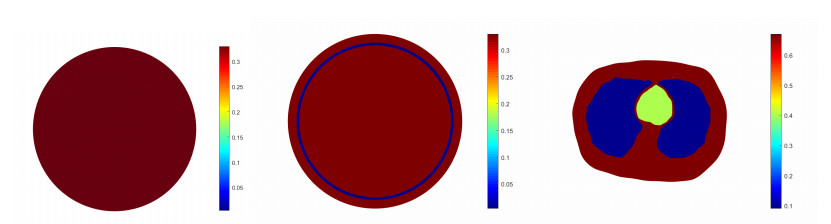
Electrical impedance tomography (EIT) is an imaging technique that reconstructs the conductivity distribution in the interior of an object using electrical measurements from the electrodes that are attached around the boundary. The Complete Electrode Model (CEM) accurately incorporates the electrode size, shape, and effective contact impedance into the forward problem for EIT. In this work, the effect of the conductivity distribution and the electrode contact impedance on the solution of the forward problem is addressed. In particular, the sensitivity of the electric potential with respect to a small-amplitude perturbation in the conductivity, and with respect to some defective electrodes is studied. The Gâteaux derivative is introduced as a tool for the sensitivity analysis and the Gâteaux differentiability of the electric potential with respect to the conductivity and to the contact impedance of the electrodes is proved. The derivative is then expressed as the unique solution to a variational problem and the discretization is performed with Finite Elements of type P1. Numerical simulations for different 2D and 3D configurations are presented. This study illustrates the impact of the presence of perturbations in the parameters of CEM on EIT measurements. Finally, the 2D inverse conductivity problem for EIT is numerically solved for some configurations and the results confirm the conclusions of the numerical sensitivity analysis.
Citation: Marion Darbas, Jérémy Heleine, Renier Mendoza, Arrianne Crystal Velasco. Sensitivity analysis of the complete electrode model for electrical impedance tomography[J]. AIMS Mathematics, 2021, 6(7): 7333-7366. doi: 10.3934/math.2021431
[1] | Saeed Althubiti . Second-order nonlinear neutral differential equations with delay term: Novel oscillation theorems. AIMS Mathematics, 2025, 10(3): 7223-7237. doi: 10.3934/math.2025330 |
[2] | Salvatore Mancha Gonzales, Hasnain Iftikhar, Javier Linkolk López-Gonzales . Analysis and forecasting of electricity prices using an improved time series ensemble approach: an application to the Peruvian electricity market. AIMS Mathematics, 2024, 9(8): 21952-21971. doi: 10.3934/math.20241067 |
[3] | Kai Zhang, Yunpeng Ji, Qiuwei Pan, Yumei Wei, Yong Ye, Hua Liu . Sensitivity analysis and optimal treatment control for a mathematical model of Human Papillomavirus infection. AIMS Mathematics, 2020, 5(3): 2646-2670. doi: 10.3934/math.2020172 |
[4] | Binaya Tuladhar, Hana M. Dobrovolny . Testing the limits of cardiac electrophysiology models through systematic variation of current. AIMS Mathematics, 2020, 5(1): 140-157. doi: 10.3934/math.2020009 |
[5] | Adil Jhangeer, Ali R Ansari, Mudassar Imran, Beenish, Muhammad Bilal Riaz . Lie symmetry analysis, and traveling wave patterns arising the model of transmission lines. AIMS Mathematics, 2024, 9(7): 18013-18033. doi: 10.3934/math.2024878 |
[6] | Mahmoud M. Abdelwahab, Khamis A. Al-Karawi, H. E. Semary . Integrating gene selection and deep learning for enhanced Autisms' disease prediction: a comparative study using microarray data. AIMS Mathematics, 2024, 9(7): 17827-17846. doi: 10.3934/math.2024867 |
[7] | Sireepatch Sangsawang, Usa Wannasingha Humphries, Amir Khan, Puntani Pongsumpun . Sensitivity analysis of cassava mosaic disease with saturation incidence rate model. AIMS Mathematics, 2023, 8(3): 6233-6254. doi: 10.3934/math.2023315 |
[8] | Turki D. Alharbi, Md Rifat Hasan . Global stability and sensitivity analysis of vector-host dengue mathematical model. AIMS Mathematics, 2024, 9(11): 32797-32818. doi: 10.3934/math.20241569 |
[9] | Xia Chang, Haixia Zhao, Zhenxia Xue . MRI image enhancement based on feature clustering in the NSCT domain. AIMS Mathematics, 2022, 7(8): 15633-15658. doi: 10.3934/math.2022856 |
[10] | Luis X. Vivas-Cruz, Celia Martínez-Lázaro, Cruz Vargas-De-León . A mathematical and numerical study of an age-dependent transactivation model for dynamics of HIV infection. AIMS Mathematics, 2025, 10(6): 14560-14595. doi: 10.3934/math.2025656 |
Electrical impedance tomography (EIT) is an imaging technique that reconstructs the conductivity distribution in the interior of an object using electrical measurements from the electrodes that are attached around the boundary. The Complete Electrode Model (CEM) accurately incorporates the electrode size, shape, and effective contact impedance into the forward problem for EIT. In this work, the effect of the conductivity distribution and the electrode contact impedance on the solution of the forward problem is addressed. In particular, the sensitivity of the electric potential with respect to a small-amplitude perturbation in the conductivity, and with respect to some defective electrodes is studied. The Gâteaux derivative is introduced as a tool for the sensitivity analysis and the Gâteaux differentiability of the electric potential with respect to the conductivity and to the contact impedance of the electrodes is proved. The derivative is then expressed as the unique solution to a variational problem and the discretization is performed with Finite Elements of type P1. Numerical simulations for different 2D and 3D configurations are presented. This study illustrates the impact of the presence of perturbations in the parameters of CEM on EIT measurements. Finally, the 2D inverse conductivity problem for EIT is numerically solved for some configurations and the results confirm the conclusions of the numerical sensitivity analysis.
Electrical properties such as the conductivity and the permittivity determine the behaviour of materials under the influence of external electric fields. Electrical Impedance Tomography (EIT) is a non invasive, radiation-free imaging technique which reconstructs the conductivity distribution inside an observation region. In practice, currents of prescribed magnitudes (typically in a frequency range from several hundred Hz to several MHz) are injected at electrodes placed on the boundary of the region. The current passes through the object and the resulting voltages are recorded. These measurements are the data needed to estimate the conductivity within the object. EIT is known to be a low cost and portable imaging modality. With these advantages, EIT is a thriving area of research due to the variety of possible applications (e.g. geophysical [47,65], medical [3,23,26,45,54], and industrial [43,49]).
In EIT systems, the quality and the accuracy of the measurements depend on many factors such as the configuration of the electrodes and the injected current distribution. The size, geometry, and location of the electrodes must be considered because they can affect the data needed for the reconstruction. The voltage loss due to the effective contact impedance, which is the thin resistive layer between the electrode and object, should also be taken into account to accurately measure the boundary voltages. In medical applications, the skin surface is prepared using a certain gel to lower and stabilize the contact impedance between the skin and the electrode. Moreover, current shunting effect through the pickup electrodes is a well-known problem in EIT systems. This phenomenon can be efficiently reduced by the geometry of the electrodes or by their separation. Also, boundary current distribution or current patterns affect the data since they are linearly related, given a fixed conductivity distribution [9]. It is essential to account all these parameters in order to produce more accurate data for the imaging [50].
Mathematically, EIT is divided into two parts: the forward problem and the inverse problem. The forward problem consists of finding the potential distribution inside the domain under examination and on its boundary from the knowledge of the electrical conductivity distribution in the domain and the injected surface current pattern. Solving the forward problem corresponds to the data acquisition. Thus, it is important to correctly model the electrodes on the boundary, their effects, and also the injected currents. For instance, we refer to the review article [4] and the references therein. The simplest forward model for EIT is the continuum model but it does not take into account the electrodes. The gap model attempts to represent the electrodes by points and approximates the current density by a nonzero constant at the surface of each electrode and zero in the gaps between the electrodes. The shunt model accounts the correct configuration of the electrodes but not the effective contact impedance. The Complete Electrode Model (CEM) successfully considers the geometry and location of the electrodes, and their shunting effects [11,53]. Consequently, CEM is the most accurate and commonly used model for EIT. Many theoretical and numerical works have been devoted to the study of the CEM for EIT (see e.g. [6,12,16,24,25,32,33,56]).
The EIT inverse problem is the recovery of the electrical conductivity distribution from the surface voltages and current density [4]. It is also known as Calderón's problem [8] which is a very ill-posed problem. An existence and uniqueness result is obtained by Kohn and Vogelius in [39], and they proved that the conductivity can be uniquely determined by the knowledge of the entire corresponding voltage-to-current or Dirichlet-to-Neumann map. However, only partial information on the Dirichlet-to-Neumann map is available in practical applications. In [34,35,38], the identifiability is proved if only partial information is known. There is an extensive literature on the numerical resolution of the EIT inverse problem. Most of the methods are iterative and are based on deterministic or stochastic least squares reconstruction algorithms (e.g [15,36,42]). Several approaches have been considered for dealing with inaccuracy on the known boundary shape, and in particular the position uncertainty of the electrodes [15,17,18,40,41,46,52]. Simultaneous reconstruction of the conductivity distribution and the contact impedances has been proposed in [30,59]. We can also cite direct inversion methods for EIT, in particular Calderón and D-bar (e.g [19,28]). The use of neural networks and metaheuristic algorithms has been explored too (e.g. [27,57,60,61,63,64]).
In this paper, we focus on the study of the CEM forward problem. The accuracy of the EIT reconstruction relies heavily on one of the associated forward model. We are interested in the sensitivity of the electric potential, and thus of the measurements, with respect to the conductivity or with respect to the contact impedance of the electrodes. This allows to measure the effect of uncertainty in both conductivity and contact impedance values on the CEM forward model. This also permits to understand the impact of possible perturbations of the conductivity (e.g. tumors or strokes in medical applications, or uncertainties in tissue conductivities) or of small changes in the impedance due to skin-to-electrode contact on the potential measurements. It can be viewed as a preliminary step for improving (direct or iterative) inversion methods. For the definition of sensitivity, several definitions exist in the literature. One can find works which are addressed by different communities (applied mathematics, bioengineering, physics, physiology, …). The most simple and used is the numerical computation of the difference between measurements before and after a conductivity change in a known homogeneous medium. In [37], the authors observe numerically the sensitivity distribution of the measured impedance signal with respect to the conductivity distribution. Other works define the sensitivity as the fractional change of transfer impedance (ratio of the measured potential and the applied current) with respect to a change of a conductivity inside a region [2,51]. In [2], the impact of the tissue conductivities and of the size of the electrodes on the performance of the EIT system in the thorax imaging is numerically analyzed. In [62], the authors consider absolute and relative sensitivities (defined by the difference between measured data with and without an anomaly in the conductivity) in a Frobenius-type norm. Furthermore, in [62], conformal maps are employed to introduce an analytic method to determine the sensitivity of boundary measurements to perturbations in conductivity for the CEM on circular domains. Based on this information, well-adapted discretizations of the conductivity space are proposed for the numerical solution of the inverse conductivity problem. The effect of the characteristics of the electrodes (contact impedance, area, boundary shape under the electrode) on the reconstructed EIT images has been studied in [7]. In the present work, an additional analysis tool to investigate the sensitivity of the solution of the CEM to small variations in conductivity or contact impedance is developed. We define in a rigorous way the mathematical setting of the sensitivity equations and we address both theoretical and numerical aspects.
The paper is organized as follows. In Section 2, we introduce the CEM. In Section 3, we present a sensitivity analysis of the CEM. In Section 4, we address the discretization of the CEM and the resulting variational problems for the sensitivity equations using Lagrange finite elements. Section 5 is devoted to the numerical part of the sensitivity analysis. Various two- and three-dimensional numerical simulations, and discussions are provided. The end of Section 5 deals with the numerical solution of the 2D EIT inverse conductivity problem for several configurations. Finally, we give some conclusions and perspectives in the last section.
Let Ω be a bounded simply connected domain in Rd, d=2,3, with a smooth boundary ∂Ω. In the low frequency range under consideration in EIT experiments, the electromagnetic field satisfies the quasi-static Maxwell equations where the time derivatives are neglected [4,10]. This yields the following form of Maxwell's equations in terms of the electric field E and the magnetic field H,
∇⋅(εE)=ρ, | (2.1a) |
∇⋅(μH)=0, | (2.1b) |
∇×E=0, | (2.1c) |
∇×H=J. | (2.1d) |
Here, ρ is the charge density, ε and μ are the electric permittivity and magnetic permeability, respectively, and J is the electric current density. Moreover, given the conductivity distribution σ in the domain Ω, Ohm's law gives
J=σE. | (2.2) |
It follows from (2.1c) that the electric field E derives from an electric (scalar) potential u, i.e.
E=−∇u. | (2.3) |
Taking the divergence of (2.1d), and using (2.2) and (2.3) yields the following elliptic equation for the electrical potential u in Ω
∇⋅(σ∇u)=0. | (2.4) |
The conductivity σ∈L∞(Ω) is assumed to satisfy
σmin≤σ(x)≤σmax,x∈Ω, | (2.5) |
for some constants 0<σmin≤σmax<+∞. Let n:=n(x) be the exterior unit normal of ∂Ω. For a known conductivity distribution σ in Ω and boundary data U (voltage) or I (current density), the equation (2.4) together with either a Dirichlet boundary condition
u=U,onΓ |
or a Neumann boundary condition
σ∂nu=I,on∂Ω,with∫∂ΩI ds=0, |
is called the continuum model for EIT.
In experiments, a finite number of surface electrodes, on which currents of prescribed magnitudes are injected and voltages are recorded, are attached to the boundary. Different electrode models have been proposed and studied but the most accurate mathematical model for real-life EIT is the Complete Electrode Model (CEM) [11,53]. The electrodes are modeled by L subdomains {eℓ}Lℓ=1 on ∂Ω at which we inject current patterns I=(Iℓ)Lℓ=1∈RL and measure the resulting potential vectors U=(Uℓ)Lℓ=1∈RL. The electrodes are also assumed to be well-separated, i.e., ¯em∩¯eℓ=∅, if m≠ℓ, and we set Γe:=L⋃ℓ=1eℓ. The current pattern I belongs to the mean-free subspace
RL⋄:={W=(Wℓ)Lℓ=1∈RL|L∑ℓ=1Wℓ=0} |
due to conservation of electric charge. Each electrode eℓ is considered to be an open subset of the boundary ∂Ω with positive surface measure. Electrodes are assumed to be perfect conductors (i.e. the potential is constant on each electrode). This is the so-called shunting effect. Furthermore, effective contact impedance, which is the thin, highly-resistive layer at the contact of eℓ with ∂Ω, is accounted and denoted by Z=(zℓ)Lℓ=1∈RL that is assumed to satisfy
zℓ>zmin, | (2.6) |
with zmin a positive constant. According to Ohm's law, this effect causes a voltage drop zℓσ∂nu and is modeled by a Robin-type boundary condition [53]
u+zℓσ∂nu=Uℓ, oneℓ,ℓ=1,…,L. | (2.7) |
Now, assuming that the current flowing on each electrode is equal to the current injected and that there is no current flow on the parts of the boundary where there is no electrode, we have
∫eℓσ∂nu ds=Iℓ,ℓ=1,…,L, | (2.8) |
σ∂nu=0,on∂Ω∖Γe. | (2.9) |
The CEM is the following forward problem for EIT: given a current pattern I=(Iℓ)Lℓ=1∈RL⋄, a conductivity distribution σ satisfying (2.5) and contact impedances Z=(zℓ)Lℓ=1∈RL, find the pair (u,U)∈H:=H1(Ω)⊕RL⋄ solution to the boundary-value problem (2.4)-(2.7)-(2.8)-(2.9). Existence of solution is ensured by the condition
L∑ℓ=1Iℓ=0 | (2.10) |
whereas the uniqueness is proved by assuming
L∑ℓ=1Uℓ=0. | (2.11) |
The variational formulation of the CEM forward problem reads: Find (u,U)∈H such that
Bσ((u,U),(w,W))=L∑ℓ=1IℓWℓ, | (2.12) |
for all (w,W)∈H, where the bilinear form Bσ:H×H⟶R is defined by
Bσ((u,U),(w,W)):=∫Ωσ∇u⋅∇w dx+L∑ℓ=11zℓ∫eℓ(u−Uℓ)(w−Wℓ) ds. | (2.13) |
Existence and uniqueness of solution (u,U)∈H has been proved using the Lax-Milgram theorem in [53]. Indeed, the bilinear form Bσ is bounded and coercive on H with respect to norm ‖⋅‖H given by ‖(u,U)‖2H:=‖u‖2H1(Ω)+L∑ℓ=1U2ℓ.
Remark 1. The proof is based on the fact that the space (H,‖⋅‖H) and the quotient space (H1(Ω)⊕RL)/R equipped with the following norm
‖(u,U)‖∗=(‖∇u‖2L2(Ω)+L∑ℓ=1∫eℓ|u−Uℓ|2 dS)1/2 | (2.14) |
are norm-equivalent [53].
In the medical applications that we have in mind, the computational domain Ω may represent a head model or a torso and is composed of different tissues or organs (see Section 5). In this context, we consider a partition of Ω into M open disjoint subdomains (Ωi)i=1,…,M, Ωi⊂Ω, with smooth surfaces such that ¯Ω=M⋃i=1¯Ωi. We require that σ|Ωi∈C(¯Ωi), 1≤i≤M, and assumption (2.5) to prove the existence and uniqueness of a weak solution (u,U)∈H for the CEM problem. The result is again a consequence of the Lax–Milgram theorem [53].
Sensitivity indicates the behavior of the potential when there is a slight variation of physical parameters. Here, we are interested in the sensitivity with respect to the conductivity and with respect to the contact impedance of the electrodes, in order to understand the effect of uncertainty in their values on the CEM forward model. This permits to understand the impact of possible perturbations of the conductivity or small electrode defects on the potential measurements. Mathematically, a rigorous way to describe sensitivity is given by Gâteaux differentiability which expresses a weak concept of derivative.
Definition 1. Let w:X→Y be an application between two Banach spaces X and Y. Let O⊂X be an open set. The directional derivative Dμw(p) of w at p∈O in the direction μ∈X is defined by
Dμw(p)=limh→0w(p+μh)−w(p)h |
if the limit exists. If Dμw(p) exists for any direction μ∈X and if the map μ↦Dμw(p) is linear continuous from X to Y, w is said to be Gâteaux differentiable at p.
We introduce the (open) space of admissible conductivities
Padm:={σ∈L∞(Ω)|σmin<σ<σmax}. |
We set (u,U):=(u(⋅,σ),U(σ)) as the solution of the variational formulation (2.12). Problem (2.12) with conductivity σ∈Padm admits a unique solution (u,U)∈H. The aim is to prove differentiability of (u,U) with respect to σ and to identify its derivative in a given direction μ. We consider a direction μ∈L∞(Ω) with ‖μ‖∞=1 such that σ+hμ∈Padm for any h∈[−h0,h0], h0>0. The solution of the perturbed CEM forward problem with conductivity σ+hμ is (uh,Uh):=(u(⋅,σ+hμ),U(σ+hμ))∈H. The associated variational formulation reads: find (uh,Uh)∈H such that
Bσ+hμ((uh,Uh),(w,W))=L∑ℓ=1IℓWℓ, | (3.1) |
for all (w,W)∈H, where the bilinear form is defined by
Bσ+hμ((uh,Uh),(w,W)):=∫Ω(σ+hμ)∇uh⋅∇w dx+L∑ℓ=11zℓ∫eℓ(uh−Uhℓ)(w−Wℓ) ds. | (3.2) |
In the sequel, we write a≲b if there is a constant C>0 independent from the quantities a and b such that a≤Cb. We have the following preliminary lemma.
Lemma 1. Let σ∈Padm and h0>0 such that σ+hμ∈Padm for any h∈[−h0,h0] and any μ∈L∞(Ω) with ‖μ‖L∞(Ω)=1. Let (u,U) and (uh,Uh) be the respective solutions in H of the variational problems (2.12) and (3.1) for all (w,W)∈H. Then, we have the following estimate
‖∇(uh−u)‖L2(Ω)≲h‖μ‖L∞(Ω). |
Proof. Subtracting (2.12) from (3.1) leads to
Bσ((uh−u,Uh−U),(w,W))=−h∫Ωμ∇uh⋅∇w dx,∀(w,W)∈H. | (3.3) |
Taking (w,W)=(uh−u,Uh−U) and using the Cauchy-Schwarz inequality, we get
|Bσ((uh−u,Uh−U),(uh−u,Uh−U))|≤h‖μ‖L∞(Ω)‖∇uh‖L2(Ω)‖∇(uh−u)‖L2(Ω). | (3.4) |
Considering both the coercivity of Bσ on H and the equivalence of the norms ‖⋅‖H and ‖⋅‖∗ (see Remark 1) in (3.4) leads to
‖(uh−u,Uh−U)‖2∗≲h‖μ‖L∞(Ω)‖∇uh‖L2(Ω)‖∇(uh−u)‖L2(Ω). |
Furthermore, the definition of the ‖⋅‖∗-norm gives
‖∇(uh−u)‖L2(Ω)≲‖(uh−u,Uh−U)‖∗. |
Thus we have
‖(uh−u,Uh−U)‖2∗≲h‖μ‖L∞(Ω)‖∇uh‖L2(Ω)‖(uh−u,Uh−U)‖∗. | (3.5) |
We study in the same way the variational problem (3.1), that is, we again use the coercivity of Bσ+hμ, the definition of the ‖⋅‖∗-norm, and the equivalence of the norms ‖⋅‖H and ‖⋅‖∗ to prove that
‖∇uh‖L2(Ω)≤C(σmin) |
with C(σmin) a positive constant independent from h. Hence, (3.5) reads
‖(uh−u,Uh−U)‖∗≲h‖μ‖L∞(Ω) |
and finally, applying again the definition of the norm ‖⋅‖∗, we conclude
‖∇(uh−u)‖L2(Ω)≲h‖μ‖L∞(Ω). |
Proposition 1. Let σ∈Padm and h0>0 such that σ+hμ∈Padm for any h∈[−h0,h0] and μ∈L∞(Ω) with ‖μ‖L∞(Ω)=1. Then the solution (u(⋅,σ),U(σ)) of (2.12) is Gâteaux differentiable with respect to σ. Moreover, the Gâteaux derivative of (u,U) in the direction μ∈L∞(Ω) is the unique solution of the following variational problem: find (u1,U1)∈H such that
Bσ((u1,U1),(w,W))=−∫Ωμ∇u⋅∇w dx. | (3.6) |
for all (w,W)∈H.
Proof. Let us introduce the differential quotients
u1,h:=uh−uhandU1,h:=Uh−Uh. |
Subtracting (2.12) from (3.1) and dividing by h leads to
Bσ((u1,h,U1,h),(w,W))=−∫Ωμ∇uh⋅∇w dx,∀(w,W)∈H. | (3.7) |
We compare the previous formulation (3.7) with the variational formulation (3.6)
∫Ω∇(u1,h−u1)⋅∇w dx+L∑ℓ=11zℓ∫eℓ((u1,h−u1)−(U1,hℓ−U1ℓ))(w−Wℓ) ds=−∫Ωμ∇(uh−u)⋅∇w dx. |
We take (w,W)=(u1,h−u1,U1,h−U1) and get
Bσ((u1,h−u1,U1,h−U1),(u1,h−u1,U1,h−U1))≤‖μ‖L∞(Ω)‖∇(uh−u)‖L2(Ω)‖∇(u1,h−uh)‖L2(Ω). |
The coercivity of Bσ on H and the equivalence of the norms ‖⋅‖H and ‖⋅‖∗ (see (2.14)) give
‖(u1,h−u1,U1,h−U1)‖2∗≲‖μ‖L∞(Ω)‖∇(uh−u)‖L2(Ω)‖∇(u1,h−uh)‖L2(Ω) |
and the definition of the ‖⋅‖∗-norm leads to
‖(u1,h−u1,U1,h−U1)‖2∗≲‖μ‖L∞(Ω)‖∇(uh−u)‖L2(Ω)‖(u1,h−u1,U1,h−U1)‖∗. |
Hence, we obtain
‖(u1,h−u1,U1,h−U1)‖∗≲‖μ‖L∞(Ω)‖∇(uh−u)‖L2(Ω). | (3.8) |
Finally, from Lemma 1, (3.8) reads
‖(u1,h−u1,U1,h−U1)‖∗≲h‖μ‖2L∞(Ω). |
This proves the strong convergence of the sequence (u1,h,U1,h)h to (u1,U1) in H.
Now it remains to show that the map μ↦(u1,U1) is linear continuous from L∞(Ω) to H. For fixed μ, the derivative is defined by the solution of (3.6) and the right-hand side of (3.6) is linear in μ. The continuity of the linear application μ↦(u1,U1) follows from the following estimate: taking (w,W)=(u1,U1) in (3.6), we get
‖(u1,U1)‖∗≲‖μ‖L∞(Ω). |
This yields the continuity of the directional derivative with respect to μ and proves that (u(⋅,σ),U(σ)) is Gâteaux differentiable with respect to the conductivity σ.
The derivative (u1,U1) of the potential (u,U) with respect to the conductivity σ in the direction μ is solution of the following boundary value problem
{−∇⋅(σ∇u1)=∇⋅(μ∇u)inΩ,u1+zℓσ∂nu1=−zℓμ∂nu+U1ℓoneℓ, ℓ=1,…,L,∫eℓσ∂nu1 ds=−∫eℓμ∂nu dsℓ=1,…,L,σ∂nu1=−μ∂nuon∂Ω∖Γe, | (3.9) |
where u is the solution of the unperturbed problem, i.e., it satisfies ∇⋅(σ∇u)=0. Formally, consider a perturbation of σ of the form σ+μh for fixed μ. The potential uh is the solution of the perturbed equation ∇⋅((σ+μh)∇u)=0. Subtracting the above equations and dividing by h yields
−∇⋅(σ∇(uh−uh))=∇⋅(μ∇u). |
At the limit h→0, we get that the sensitivity u1 satisfies the first equation of (3.9). The different boundary equations can be obtained in a similar way. The variational formulation of the problem (3.9) is given by (3.6).
Remark 2. The Fréchet differentiability of the map (σ,y)↦(u,U), where y:=1/Z, has been proved by Kaipio et al (see [36] Theorem 2.3). Indeed, it implies the Gâteaux differentiability of the potential with respect to both the conductivity σ and the inverse 1/Z of contact impedance. Nevertheless, the sensitivity analysis of the forward CEM needs only the existence of all the directional derivatives of (u,U). We have detailed the proof of Proposition 1 for readers who want to deepen the concept of sensitivity analysis for PDEs. Furthermore, we propose a proof for the Gâteaux differentiability of the potential with respect to Z (and not to 1/Z as in [36]) and give an expression of the directional derivatives of (u,U) in that case (see Section 3.2 Proposition 2 and Appendix).
We introduce the set of admissible contact impedances
Zadm:={Z∈RL|zmin<zℓ<zmax}, |
with 0<zmin≤zmax<+∞. Similar arguments to those in the proof of Proposition 1 lead to the following result.
Proposition 2. Let I be a fixed current pattern and σ a known conductivity distribution satisfying (2.5). Let Z∈Zadm such that Z+ηh∈Zadm for any h∈[−h0,h0] and η∈RL. Then the solution (u,U) of (2.12) is Gâteaux differentiable with respect to Z. Furthermore, its Gâteaux derivative in the direction η is the unique solution of the following variational problem: find (u2,U2)∈H such that
Bσ((u2,U2),(w,W))=L∑ℓ=1ηℓz2ℓ∫eℓ(u−Uℓ)(w−Wℓ) ds | (3.10) |
for all (w,W)∈H.
The full proof of Proposition 2 is given in the Appendix. The derivative (u2,U2) of the potential (u,U) with respect to the contact impedance Z in the direction η is solution of the following boundary value problem
{∇⋅(σ∇u2)=0inΩu2+zℓσ∂nu2=−ηℓσ∂nu+U2ℓoneℓ, ℓ=1,…,L,∫eℓσ∂nu2 ds=0 ℓ=1,…,L,σ∂nu2=0on∂Ω∖Γe. | (3.11) |
The variational formulation of the problem (3.11) is given by (3.10) subject to the above boundary conditions.
Remark 3. Using the same arguments as in the proofs of Propositions 1 and 2, we can show that (u,U) is Gâteaux differentiable with respect to both σ and Z, simultaneously. The derivative (u3,U3) is the unique element in H satisfying
Bσ((u3,U3),(w,W))=−∫Ωμ∇u⋅∇w dx+L∑ℓ=1ηℓz2ℓ∫eℓ(u−Uℓ)(w−Wℓ) ds |
for all (w,W)∈H. We recognize that
(u3,U3)=(u1,U1)+(u2,U2) |
where (u1,U1) and (u2,U2) are the respective unique solutions of problems (3.7) and (3.10). It seems that it is more interesting to study the sensitivity with respect to σ and Z separately to better understand the influences of perturbations in each parameter on the CEM.
In this section, we address the discretization of the different variational problems. We use the finite element method (FEM) to compute an approximate solution of the CEM and the sensitivity equations (3.6) and (3.10).
We consider a triangular (respectively tetrahedral) mesh Th for 2D (respectively 3D) geometries Ω. For any mesh parameter h, we denote by Ωh the discrete domain and by N the number of nodes. On Th, we introduce Xh the standard vector space of Lagrange finite elements of type P1. The approximation uh∈Xh of the electric potential u is of the form
uh(x)=N∑j=1vjφj(x), x∈Ωh, | (4.1) |
where the functions φj are linear basis functions. We focus on the numerical implementation proposed in [36]. We explain here the main lines of the approach.
To impose the uniqueness condition (2.11), a different representation of the voltage U is introduced. Define a set of vectors ϕℓ∈RL, ℓ=1,…,L−1, by ϕ1=(1,−1,0,…,0)T, ϕ2=(1,0,−1,…,0)T, …,ϕL−1=(1,0,…,−1)T. The approximation Uh of U is expressed as
Uh=L−1∑k=1βkϕk, | (4.2) |
where βk∈R, k=1,…,L−1. Let β=(βk)T∈RL−1 and define the matrix P=(ϕ1|…|ϕL−1)∈RL×L−1. Thus, the voltages Uh are determined by
Uh=Pβ. | (4.3) |
Consider the test functions (w,W)=(φi,0). Substituting (4.1)-(4.2) to (2.12), we get for each i=1,…,N
N∑j=1vj∫Ωhσ∇φj⋅∇φi dx+L∑ℓ=11zℓ∫eℓ(N∑j=1vjφj−L−1∑k=1βkϕk)φi ds=0. |
We get the following linear system
[S+MC][vβ]=[0RN], | (4.4) |
with v=(vj)Nj=1∈RN, S=(Sij),M=(Mij)∈RN×N of respective coefficients Sij=∫Ωhσ∇φj⋅∇φi dx and Mij=∑Lℓ=11zℓ∫eℓφjφi ds, and C=(Ciℓ)∈RN×(L−1) with Ciℓ=−(1z1∫e1φi ds−1zℓ+1∫eℓ+1φi ds). Furthermore, the conditions (2.7) and (2.8) give
1zℓ∫eℓ(Uℓ−u) ds=Iℓ,ℓ=1,2,…,L. | (4.5) |
Then, the approximations (4.1) and (4.2) are used in (4.5). In order to obtain a symmetric system of equations in the end, the number of equations above should be reduced to L−1 which is done by subtracting from I1 all the remaining current values Iℓ, ℓ=2,3,…,L. We have
I1−I2=N∑j=1(−1z1∫e1φj ds+1z2∫e2φj ds)uhj+(1z1∫e1 ds+1z2∫e2 ds)β1+L−1∑ℓ=1,ℓ≠1(1z1∫e1 ds)βℓI1−I3=N∑j=1(−1z1∫e1φj ds+1z3∫e3φj ds)uhj+(1z1∫e1 ds+1z3∫e3 ds)β2+L−1∑ℓ=1,ℓ≠2(1z1∫e1 ds)βℓ⋮ |
I1−IL=N∑j=1(−1z1∫e1φj ds+1zL∫eLφj ds)uhj+(1z1∫e1 ds+1zL∫eL ds)βL−1+L−1∑ℓ=1,ℓ≠L−1(1z1∫e1 ds)βℓ. |
Note that ∫eℓ ds=|eℓ|, where |eℓ| is the length (respectively the area) of the electrode in the two-dimensional (respectively in the three-dimensional) case and that PTI=(I1−I2,I1−I3,…,I1−IL)T. Therefore, we have the following matrix form of the L−1 equations equivalent to (4.5)
[CTG][vβ]=[PTI], | (4.6) |
where G∈R(L−1)×(L−1) is given by
Gik={|e1|z1,i≠k|e1|z1+|ek+1|zk+1,i=k. |
Finally, an approximate solution of the CEM is obtained by solving the following linear system of size N+L−1
[S+MCCTG][vβ]=[0RNPTI]. | (4.7) |
The vector v=(vj)j∈RN gives the approximation uh of the potential u, and β leads to the calculation of the voltages Uh at the electrodes using (4.3).
We again apply the approach of Kaipio et al [36] described previously. The approximation u1h of the sensitivity u1 is defined by
u1h(x)=N∑j=1v1jφj(x) | (4.8) |
where v1j is the approximated value of u1 at the jth node. To ensure that the potential U1 satisfy the condition (2.11), we search the approximation U1h of U1 under the form
U1h=L−1∑k=1β1kϕk, | (4.9) |
with U1h=Pβ1 and β1=(β1k)T∈RL−1 (see (4.3)).
We set the test functions (w,W)=(φi,0). We substitute (4.1), (4.8) and (4.9) to (3.6), and we get for i=1,…,N
N∑j=1v1j∫Ωhσ∇φj⋅∇φi dx+L∑ℓ=11zℓ∫eℓ(N∑j=1v1jφj−L−1∑k=1β1kϕk)φi ds=−N∑j=1vj∫Ωhμ∇φj⋅∇φi dx, | (4.10) |
where v=(vj)Nj=1 represents the approximation of the solution u of the unperturbed CEM (2.4)-(2.7)-(2.8)-(2.9). Furthermore, from the boundary conditions in (3.9), we deduce
1zℓ∫eℓ(U1ℓ−u1) ds=0ℓ=1,2,…,L. | (4.11) |
We use the same method which is presented previously to treat (4.5). Finally, we obtain the following linear system of size N+L−1
[S+MCCTG][v1β1]=[Fv0RL−1], | (4.12) |
where v1=(v1j)Nj=1, F=(Fij)∈RN×N with coefficients Fij=−∫Ωhμ∇φj⋅∇φi dx. The vector v is computed using the resolution of the linear system (4.7).
The approximation of the potential (u2,U2) is given respectively by
u2h(x)=N∑j=1v2jφj(x), | (4.13) |
where v2j is the approximation of the value of u2 at the jth node, and
U2h=L−1∑k=1β2kϕk, | (4.14) |
with U2h=Pβ2 and β2=(β2k)T∈RL−1. The approximations (4.13) and (4.14), and the choice of test functions (w,W)=(φi,0) in (3.10) give for i=1,…,N
N∑j=1v2j∫Ωhσ∇φj⋅∇φi dx+L∑ℓ=11zℓ∫eℓ(N∑j=1v2jφj−L−1∑k=1β2kϕk)φi ds=N∑j=1vj(L∑ℓ=1ηℓz2ℓ∫eℓφiφj ds)−L∑ℓ=1Uℓh(∫eℓηℓz2ℓφi ds), | (4.15) |
where v=(vj)Nj=1∈RN is the approximation of the potential u of the unperturbed CEM (2.4)-(2.7)-(2.8)-(2.9) and Uh=(Uℓh)∈RL is obtained from (4.2). The boundary conditions in (3.11) lead to the relation
1zℓ∫eℓ(U2ℓ−u2) ds=ηℓIℓzℓ,ℓ=1,2,…,L. | (4.16) |
We get the following linear system of size N+L−1
[S+MCCTG][v2β2]=[Qv+DUhPTI], | (4.17) |
where v2=(v2j)Nj=1∈RN, Q=(Qij)∈RN×N with Qij=L∑ℓ=1ηℓz2ℓ∫eℓφiφj ds, D=(Diℓ)∈RN×L with Dij=−ηℓz2ℓ∫eℓφi ds, and I=(ηℓIℓzℓ)ℓ∈RL.
We study numerically how a slight variation of the conductivity or of the contact impedance of the electrodes affect the electric potential u and the EIT measurements U∈RL for several two- and three-dimensional configurations. To this end, we compute the numerical sensitivity u1h of the electric potential in the domain and the sensitivity U1h of the measured voltages at electrodes (see (4.8) and (4.9) respectively) with respect to a small-amplitude perturbation in the conductivity. And we compute the numerical sensitivity u2h of the electric potential in the domain and the sensitivity U2h of the measured voltages at electrodes (see (4.13) and (4.14) respectively) with respect to a small perturbation in the contact impedance of some electrodes. This provides dimensionless qualitative indicators. The electric potential and the voltages are more impacted if the values of their sensitivity are high.
We consider three geometries: the unit disk, a head model, and a thorax (see Figure 1). The background conductivity in the unit disk is equal to 0.33S.m−1. The head model consists of three concentric disks with respective (dimensionless) radii r1=0.87, r2=0.9, and r3=1 representing the three main tissues of the head: brain, skull, and scalp. Each layer is assumed to be homogeneous and isotropic. The adopted conductivity values are σ1=σ3=0.33S.m−1 for the brain and the scalp, respectively, and σ2=0.004S.m−1 for the skull. A CT scan of the thorax domain is obtained from [58]. Solving the CEM by means of finite elements requires the parametrization of the boundaries of the lungs, the heart, and the chest. These parametric curves are approximated using Fourier series. The coefficients of the Fourier series are estimated by finding the parametric curve that fits the data points on the boundary curve. With this, any practical domain or object may be studied for real-life applications of EIT. The background conductivity modeling the blood is set to 0.67S.m−1. The conductivities of the lungs and the heart are, respectively, fixed to 0.09S.m−1 and 0.4S.m−1 [44].
For the numerical simulations, L=32 electrodes are attached around the boundary of the studied domain Ω (see Figure 2). The current pattern I=(Iℓ)ℓ is defined by
Iℓ=sin(2πℓL),ℓ=0,1,2,…,L−1. |
In order to impose the conservation of charge in real-life systems, when a current is injected through one electrode, its corresponding negative measure is injected through the opposite electrode. The sine function satisfies this requirement in 2D set-up and [53] discussed in detail this choice of current pattern. All simulations are executed with the software FreeFem++ [29]. The different linear systems are solved with the default direct sparse solver in FreeFem++.
The sensitivity (u1h,U1h) of the potential in the given direction μ is computed as the solution of the linear system (4.12) presented in Section 4. The contact impedance is set to be constant across all electrodes on the boundary, that is, zℓ=0.1, ∀ℓ=0,…,L−1.
Unit disk. We consider a small-amplitude perturbation in the conductivity. This perturbation is modeled by a disk D=Dr(x0) of radius r centred at x0. The direction of the computed derivative is μ=1D. A FEM mesh structure with 17 662 triangular elements and 8 992 nodes is used with mesh size h=0.038. At the top of Figure 3, the perturbation of radius r=0.1 is placed at two different positions, namely x0=(0.4,0) (called configuration Ⅰ) and x0=(0.7,0) (called configuration Ⅱ). In the bottom left of Figure 3, we report the sensitivity corresponding to the circular inhomogeneity centered at x0=(0.4,0) for a bigger radius r=0.3 (called configuration Ⅲ). For each test-case, we report the map of the numerical sensitivity u1h. The simulations indicate how the position of the inhomogeneity in the conductivity affects sensitivity. In particular, it shows that the largest values of the sensitivity are observed around the inhomogeneity and the sensitivity decreases away from the defect. Also, increasing the inhomogeneity's size increases the amplitude of the sensitivity significantly. Finally, in the bottom right of Figure 3, we present the sensitivity corresponding to two circular perturbations (called configuration Ⅳ): one centered at x0=(0.4,0) of radius r=0.1 and the other centered at x0=(−0.4,−0.5) of radius r=0.2.
To complete observations of Figure 3, Table 1 reports the sensitivity values U1h of the voltages (i.e. of the EIT measurements) at some chosen electrodes. For configurations Ⅰ and Ⅱ, we have selected the electrodes e4 and e30 which are located on the side of the conductivity perturbation, and the electrodes e13 and e17 which are on the opposite side. The sensitivity U1h recorded at the electrodes near the inclusion, namely the components U1h(4) and U1h(30) at electrodes e4 and e30, has bigger values than at the ones far from it (i.e. the components U1h(13) and U1h(17) at electrodes e13 and e17). A factor of 10 is observed. Thus the electrodes e4 and e30 retain more information. Furthermore, as expected, the sensitivity U1h becomes larger when the inclusion is very close to the boundary (Configurations Ⅱ and Ⅳ) and when the inclusion is bigger in size (Configurations Ⅲ and Ⅳ). Same conclusions are obtained in [62] using another definition of the sensitivity.
![]() |
Unit disk | ||||
Electrode | Ⅰ | Ⅱ | Ⅲ | Ⅳ |
4 | -0.0444 | -0.0720 | -0.4054 | -0.0926 |
13 | 0.0003 | 0.0013 | 0.0036 | 0.0354 |
17 | -0.0004 | 0.0023 | -0.0046 | -0.1593 |
30 | 0.0409 | 0.0737 | 0.3779 | 0.0040 |
Head model. First, we study the effect of a small perturbation in the conductivity of the region Ω1 modeling the brain. The mesh characteristics are h=0.0368, 17 952 triangular elements, and 9 137 nodes. Figure 4 compares the sensitivity map of the potential with respect to a perturbation of different locations and areas. We perform the same test-cases as for the unit disk (see Figure 3). We observe a similar behaviour of the sensitivity, with respect to the location, size, and number of perturbations. However, we note that there is a significant decrease in the sensitivity values for all the test-cases. The values of the sensitivity U1h of the voltages on the boundary are close to zero (see Table 2). Nevertheless, here again the values are higher (factor of 10) at the electrodes which are on the side of the perturbation. The skull with a very small conductivity plays an important role in EIT. The adult skull is extremely resistive compared to the other tissues and acts as an electrical shield between the scalp and brain.
Head model | |||||
Electrode | Ⅰ | Ⅱ | Ⅲ | Ⅳ | skull |
4 | -0.0040 | -0.0063 | -0.0368 | -0.0102 | -45.80 |
13 | 0.0001 | 0.0002 | 0.0010 | 0.0025 | 0.6728 |
17 | -0.0001 | 7.7E-05 | -0.0012 | -0.0139 | -14.24 |
30 | 0.0034 | 0.0062 | 0.0314 | 0.0011 | 26.24 |
Now, we consider the configuration when only the skull conductivity is perturbed (i.e μ=1Ω2) and there is no perturbation in the brain. Figure 5 shows the sensitivity map in this case. Furthermore, the sensitivity values of the voltages at some electrodes for this simulation are given in Table 2 (last column). They are notably larger than the ones obtained from Configurations Ⅰ-Ⅳ. It confirms that EIT measurements are highly sensitive to uncertainties in the value of the skull conductivity [20,21].
Thorax. The numerical sensitivity is computed on a mesh with characteristics: h=0.0428, 18 450 triangular elements, and 9 386 nodes. Figure 6 shows the effect of a perturbation in the heart and the lungs conductivity on the sensitivity values. This perturbation may model a congenital cardiac defect or a pulmonary anomaly in the lungs, respectively. We observe that the interior electric potential is less sensitive to a presence of an anomaly (of the same size) in the heart than in the lungs. Furthermore, the largest values of the sensitivity of the boundary voltages are recorded at the electrodes which are close to the perturbation (see Table 3).
![]() |
Thorax | ||
Electrode | Ⅰ | Ⅱ |
2 | -0.0020 | -0.0103 |
19 | -0.0001 | -0.0004 |
25 | 0.0101 | 0.0046 |
29 | 0.0017 | 0.0139 |
We consider two geometries: the unit ball and a spherical head model. In the unit ball, the background conductivity is fixed to 0.33S.m−1. The mesh size used for the unit ball is h=0.074, for a total of 501 044 tetrahedrons and 87 817 nodes. As in 2D, the spherical head model consists of three concentric balls B1, B2 and B3, and of respective radii r1=0.87, r2=0.9 and r3=1 (see Figure 7). The regions Ω1=B1, Ω2=B2∖Ω1 and Ω3=B3∖Ω2 represent the brain, the skull, and the scalp, respectively. The values chosen for the conductivity of these tissues are the same as the 2D ones: 0.33S.m−1 for the brain and the scalp layers, and 0.004S.m−1 for the bone layer. The mesh uses 572 497 tetrahedrons and 99 546 nodes, ending up with a mesh size of h=0.074.
We consider standard positioning for the electrodes and use the 10-10 system [22] (see Figure 7). This system gives us the spherical coordinates of the centers of L=71 electrodes which are represented by small patches: the intersection of ∂Ω with a ball of radius 0.1. The contact impedance is the same on all electrodes and fixed to 0.1. For ℓ=0,1,…,L−1, the electrode eℓ is defined by the longitude θℓ∈[0,2π[ and latitude φℓ∈[−π/2,π/2] of its center. The current pattern Iℓ on this electrode is set to
Iℓ=sinθℓcosφℓ, ℓ=0,1,…,L−1. |
We compute the numerical sensitivity (u1h,U1h) by solving the linear system (3.6) with direction μ set as the indicator function of the considered perturbation.
Unit ball. We first consider a spherical perturbation of center (0.45,0,0.45) and of radius 0.2. We give on the top left of Figure 8 the sensitivity u1h with respect to the conductivity in the direction corresponding to the indicator function of the ball. Top right and bottom of Figure 8 show the sensitivity in the direction of a perturbation centered at (0,0.3,0.35). This perturbation is of radius 0.2 on the top right and of radius 0.35 on the bottom. As in 2D, we observe the largest values of the sensitivity around the support of the perturbation. Increasing the volume of the inhomogeneity increases the amplitude of the sensitivity.
Spherical head model. We run the same test-cases as for the unit ball. These three perturbations are all contained in the brain layer. The corresponding sensitivities are given in Figure 9. We observe the same behavior as with the unit ball if we look at the location of the biggest sensitivity values. However, the amplitude of these values is significantly lower here than in the unit ball because of the presence of the skull.
Surface measurements. The inverse problem we are interested in consists of the reconstruction of the conductivity inside the domain from the knowledge of the electrical potential values on the electrodes, which represent here the measurements. Assuming that the conductivity in healthy domains is known, this problem is equivalent to looking for inhomogeneities in this background parameter. Then, a similar argument to the one involved in [14] can be invoked: the Gâteaux derivative can be used to better understand the difference between the measurements on healthy and unknown domains.
In Figure 10, we show on the left the values of the numerical sensitivity on the boundary of the domain. On the right, we can observe the resulting values on the electrodes. Top of the figure is the case of a spherical perturbation centered at (0,0.3,0.35) with radius 0.2. On the bottom, the perturbation is of same radius and centered at (0,−0.3,0.35). It appears that the highest absolute values of the surface measurements are located on the electrodes that are the nearest from the perturbation. The sign of these values seems to be governed by the sign of the injected current, which is the same as the sign of the y-coordinate. In fact, we observe that the positive part of the sensitivity is oriented to the part of the boundary where a negative current is injected. This behavior matches the one we observed in the 2D simulations.
Remark 4. We can see that the conclusions are qualitatively similar for 2D and 3D configurations. Nevertheless, it seems easier to observe with eye the impact on the measurements in 3D. Thus, we report the numerical sensitivity of the potential with respect to contact impedance in 3D only, but keep the results in function of the conductivity in 2D and 3D for comparative purposes.
We compute the numerical sensitivity (u2h,U2h) by solving the linear system (3.10) with direction η∈{0,1}L: for 1≤ℓ≤L, ηℓ is set to 1 if we consider a perturbation on the ℓ-th electrode, and to 0 otherwise.
We model a small perturbation in the contact impedance of the 6th electrode, i.e. ηℓ=0 for all 1≤ℓ≤L except for η6=1. We show on the top left of Figure 11 the values of the sensitivity on the electrodes, i.e. the values of the vector U2h. On the top right, we give the values of U2h in the case of a perturbation on the 31st electrode. The bottom of this figure reports the values in the case of both the 6th and the 31st electrodes are perturbed.
We first notice that a peak in the values of U2h is easily visible: the highest amplitude is reached at the perturbed electrode. This behavior has been observed in all cases where a single electrode is perturbed. Moreover, the case of two perturbed electrodes indicate two peaks, each located at a perturbed electrode. It is in fact an illustration of the linearity of the sensitivity equation (3.10) with respect to the direction of differentiation: the vector η in the case of two perturbed electrodes is the sum of the two vectors corresponding to each electrode perturbed independently. Then, the sensitivities can also be summed to obtain the sensitivity corresponding to a perturbation of both electrodes.
The previous numerical sensitivity analysis shows the effect of a perturbation in the conductivity or in the contact impedance of electrodes on the measured voltages U=(Uℓ)ℓ∈RL which are the data of the EIT inverse problem. In particular, we have observed that a few number of electrodes only are sensitive with respect to a small-amplitude perturbation in the conductivity medium and the impact depends on both the size and location of the inclusion. This section gives some first numerical simulations that confirm that the reconstruction process can be affected if the information recorded by those electrodes is missing.
To this end, we consider a domain Ω containing one inclusion. The background conductivity of the medium and the geometric characteristics of the inclusion are assumed to be known. We would like to estimate the conductivity value σp inside the perturbation. To solve this inverse problem, we reformulate it into a minimization problem in R. Then we minimize numerically the cost functional using the Broyden-Fletcher-Goldfarb-Shanno (BFGS) method, which is a gradient-based optimization algorithm. No regularization is applied. It was shown numerically in [57] that the BFGS algorithm is effective in solving the inverse EIT problem if the supports of the inclusions are known. We compare the numerical approximation of σp for different configurations: measurements taken on all the electrodes or on some of them only, perturbation in the contact impedance of some electrodes.
Let Ω be the unit disk with a (fixed) circular inclusion Ωp centered at (0.7,0) with radius r=0.1. This is Configuration Ⅱ tested in Section 5.1.2. We work with synthetic data that are generated setting a background conductivity σb=0.33S.m−1 and a conductivity σp=0.4S.m−1 inside the inclusion. The contact impedance is constant across L electrodes and it is equal to zℓ=0.1, ℓ=0,…,L−1 (unless indicated otherwise). To obtain the voltage data Ujobs∈RL, j=1,…,16, sixteen current patterns are applied on the electrodes and the first current has the form
I1={I1ℓ}L−1ℓ=0=sinθℓ. |
The remaining fifteen current patterns are obtained by 'rotating' the values of the first current pattern, that is, to get the second current pattern I2, we have I2(0)=I1(L−1), I2(1:L/2)=I1(0:L/2−1), and I2(L/2+1:L−1)=I1(L/2:L−2). This is repeated until we obtain the fifteen additional current patterns. A 1% additive noise is applied to the data (compare with [31,57]). For the numerical resolution of the forward CEM, an FEM mesh structure with 25 858 triangular elements, 13 122 nodes, and mesh size 0.0115 was used, while a different mesh system with 17 882 triangular elements, 9 102 nodes, and mesh size 0.0138 was adopted for the inversion method. We implemented the numerical solver for the forward problem (4.7) with FreeFem++ [29]. The inverse conductivity problem is reformulated as the minimization of the following cost functional J:R→R
J(˜σ)=1216∑j=1‖Uj(σ)−Ujobs‖22, | (5.1) |
where the voltages Uj(σ)∈RL are obtained by solving the forward CEM (2.4)-(2.7)-(2.8)-(2.9) with the conductivity distribution σ (σb in the background Ω∖¯Ωp and ˜σ inside the inclusion Ωp) and the current pattern Ij. The minimization is done in MATLAB using the built-in command for BFGS algorithm, fminunc. Tolerance is set to be the default (ϵ= 1E-06). Initial guess is set to ˜σ0=0.6S.m−1.
Recall that we have observed in Section 5.1.2 that the sensitivity U1h of the voltages with respect to a small-amplitude perturbation in the conductivity has higher values on the electrodes 1-8 and 25-32 (see Configuration Ⅱ Figure 3 and Table 1). Supposedly, these electrodes contain the main information for the reconstruction process. On the contrary, the sensitivity values U1h on electrodes 9-24 are small or almost zero. This inspires us three first set-ups for the numerical resolution of the inverse problem:
A. The voltages are recorded on the L=32 electrodes (see Figure 1 left).
B. We keep only the sixteen values of the voltages on electrodes 1-8 and 25-32 (see Figure 12 left) which are located on the side of the inclusion.
C. We keep only the sixteen values of the voltages on electrodes 9-24 (see Figure 12 right) which are located on the opposite side of the inclusion.
Furthermore, we have seen that the voltages are sensitive to a small change in the contact impedance of electrodes and the impact is localized on the electrodes which are perturbed. It is interesting to observe the effect of such a small variation in the contact impedance of some electrodes on the numerical resolution of the inverse problem. We add two set-ups. The data are recorded on all the L=32 electrodes.
D. We set z=0.07 for electrodes 1-8 and 25-32 and z=0.1 for the rest.
E. We set z=0.07 for electrodes 9-24 and z=0.1 for the rest.
We report on Figure 13 the numerical reconstruction of the conductivity for the different set-ups, and in Table 4 the corresponding approximations of σp and relative errors. In agreement with the numerical sensitivity analysis, the accuracy deteriorates when the EIT measurements on the electrodes located on the side of the inclusion are missing. Set-up C leads to an error of 35% against 8% for Set-up B which considers only the electrodes on the side of the inclusion. This confirms that those electrodes contain the essential data for the reconstruction process. Furthermore, Set-ups D and E illustrate that the accuracy of the reconstruction depends on the contact impedance of the electrodes too. This first study attests that the sensitivity analysis for the CEM leads to a very good understanding of the link between the parameters of the model and the EIT measurements. We will give in the last part the conclusions and the perspectives these numerical results suggest.
Unit disk | ||||||
True | A. | B. | C. | D. | E. | |
Approximation | 0.4 | 0.3626 | 0.3671 | 0.5426 | 0.3731 | 0.3743 |
Relative error | 0.0934 | 0.0822 | 0.3566 | 0.0670 | 0.0640 |
In this work, we proposed a sensitivity analysis of both the interior electric potential and the EIT measurements with respect to small changes in conductivity inside an object or in contact impedance of the surface electrodes. We thoroughly studied the sensitivity of the electric potential using its Gâteaux differentiability in the theoretical and numerical point of view. We conclude that the useful information for the inversion process in EIT is essentially contained on a small part of the boundary, that is, at the electrodes close to the perturbations in the conductivity. The boundary measurements, which are the data for the inverse conductivity problem, are more sensitive to anomalies that are located near the boundary and to those that are larger in size. This study is insightful to know the critical parameter values (size, location) of a small-amplitude perturbation in the conductivity under which the measurements are not sensitive, and hence under which inhomogeneities could not be detectable. We have also observed in the head model that an uncertainty of a tissue conductivity value could have a great impact on the measurements, and by consequence on the EIT reconstruction.
Furthermore, we have shown that the sensitivity of the measurements to a small perturbation in the electrode-skin interface is localized on the faulty electrode. By linearity, when the contact impedance of another electrode is a little bit perturbed, the total sensitivity is the sum of the corresponding sensitivities. This could be damageable for the EIT conductivity reconstruction if those electrodes are located on the side of the sought inclusions. This observation is in agreement with other works which propose to reconstruct the electrode contact impedance as part of the EIT inverse problem [7,17,30,59].
Sensitivity analysis is very instructive to understand how EIT detects changes in inhomogeneous media with and without anomaly. It would be interesting to explicitly define the relations between the sensitivity values and the characteristics of the perturbations as in [14,62]. More precisely, one of our objectives is to find explicit relations between the EIT measurements and the geometric parameters (center, volume, …) of the inclusions. To know the supports of the inclusions could give good initial guesses for an iterative reconstruction process. For instance, this would allow to retrieve with a good accuracy the value of the conductivity inside the inclusions as we have seen in Section 5.3. The first reconstruction tests presented in this work are promising but this is a full-fledged research project which needs more time and investigation. This is beyond the scope of this paper. Although shape derivative has been used in several studies to reconstruct conductivity distribution in EIT [1,31,48,55] but to our knowledge, a rigourous sensitivity analysis with respect to the shape of the electrode has not been done. Finally, another conclusion of our study is that the essential data for the inverse conductivity problem are contained in a few number of electrodes. The accuracy deteriorates if those electrodes (and thus this part of the boundary) are not taken into account. To be sure not to miss this main information, data completion methods [5,13] could be a possible way to get the values of the voltages on all the boundary of the object under inspection. These are interesting future research directions which require comprehensive separate studies.
This work was funded by the UP System Enhanced Creative Work and Research Grant (ECWRG-2019-2-11-R).
The authors declare there is no conflict of interest in this paper.
Proof. Let (u,U) be the solution in H of the variational problem (2.12). Suppose that Z=(zℓ)Lℓ=1∈Zadm, h>0, and η∈RL such that ˜Z:=Z+ηh∈Zadm. Let (˜uh,˜Uh) the solution in H of the perturbed CEM forward problem with contact impedance ˜Z. The corresponding variational problem reads as follows: find (˜uh,˜Uh)∈H such that
B˜Z((˜uh,˜Uh),(w,W))=L∑ℓ=1IℓWℓ | (6.1) |
for all (w,W)∈H, where the bilinear form is defined by
B˜Z((˜uh,˜Uh),(w,W)):=∫Ωσ∇˜uh⋅∇w dx+L∑ℓ=11zℓ+ηℓh∫eℓ(˜uh−˜Uhℓ)(w−Wℓ) ds. | (6.2) |
Subtracting (2.12) from (6.1) and dividing by h, we have
∫Ωσ∇(˜uh−uh)⋅∇w dx+L∑ℓ=1∫eℓ(1h(˜uhzℓ+hηℓ−uzℓ)−1h(˜Uhℓzℓ+hηℓ−Uℓzℓ))(w−Wℓ) ds=0. | (6.3) |
A Taylor expansion yields
1zℓ+hηℓ=1zℓ−hηℓz2ℓ+O(h2), |
from which we deduce
1h(˜uhzℓ+hηℓ−uzℓ)=1zℓ˜uh−uh−ηℓz2ℓ˜uh+O(h). |
Now, the differential quotients defined by
uh,2:=˜uh−uhandUh,2:=˜Uh−Uh |
satisfy the variational formulation
Bσ((uh,2,Uh,2),(w,W))=L∑ℓ=1ηℓz2ℓ∫eℓ(˜uh−˜Uhℓ)(w−Wℓ) ds+O(h) | (6.4) |
for all (w,W)∈H. Furthermore, suppose (u2,U2)∈H is the solution to the variational problem (3.10) i.e.
Bσ((u2,U2),(w,W))=L∑ℓ=1ηℓz2ℓ∫eℓ(u−Uℓ)(w−Wℓ) ds |
for all (w,W)∈H. Next, subtracting (3.10) from (6.4), we obtain
Bσ((uh,2−u2,Uh,2−U2),(w,W))=L∑ℓ=1ηℓz2ℓ∫eℓ((˜uh−u)−(˜Uhℓ−Uℓ))(w−Wℓ) ds+O(h). |
Take (w,W)=(uh,2−u2,Uh,2−U2). From the continuity and coercivity of the bilinear form Bσ, and using the equivalence of norms, we get
‖(uh,2−u2,Uh,2−U2)‖∗≲‖η‖∞‖(˜uh−u,˜Uh−U)‖∗+O(h) | (6.5) |
where ‖η‖∞:=max1≤ℓ≤L|ηℓ|. In the same way, we set (w,W)=(˜uh−u,˜Uh−U) in (6.4) and we get
‖(˜uh−u,˜Uh−U)‖∗≲h‖η‖∞‖(˜u,˜U)‖∗+O(h2) |
and hence
‖(uh,2−u2,Uh,2−U2)‖∗≲h‖η‖2∞+O(h2). |
This proves the strong convergence of (uh,2,Uh,2)h to (u2,U2).
Lastly we prove that the map η↦(u2,U2) is linear continuous from RL to H. Since (u2,U2) is defined by (3.10) and the right-hand side of (3.10) is linear in η, the map is also linear in η. Taking (w,W)=(u2,U2) in (3.10), the continuity of the bilinear form gives us the following estimate
‖(u2,U2)‖∗≲‖η‖∞. |
This ends the proof of the Gâteaux differentiability of the CEM forward solution with respect to the contact impedance.
[1] |
Y. F. Albuquerque, A. Laurain, K. Sturm, A shape optimization approach for electrical impedance tomography with point measurements, Inverse Probl., 36 (2020), 095006. doi: 10.1088/1361-6420/ab9f87
![]() |
[2] |
L. Andiani, A. Rubiyanto, Endarko, Sensitivity analysis of thorax imaging using two-dimensional electrical impedance tomography (EIT), Journal of Physics: Conference Series, 1248 (2019), 012009. doi: 10.1088/1742-6596/1248/1/012009
![]() |
[3] |
A. P. Bagshaw, A. D. Liston, R. H. Bayford, A. Tizzard, A. P. Gibson, A. T. Tidswell, et al., Electrical impedance tomography of human brain function using reconstruction algorithms based on the finite element method, Neuroimage, 20 (2003), 752–764. doi: 10.1016/S1053-8119(03)00301-X
![]() |
[4] |
L. Borcea, Electrical impedance tomography, Inverse Probl., 19 (2003), 997–998. doi: 10.1088/0266-5611/19/4/501
![]() |
[5] |
L. Bourgeois, A. Recoquillay, A mixed formulation of the Tikhonov regularization and its application to inverse pde problems, ESAIM-Math. Model. Num., 52 (2018), 123–145. doi: 10.1051/m2an/2018008
![]() |
[6] | G. Boverman, B. S. Kim, D. Isaacson, J. C. Newell, The complete electrode model for imaging and electrode contact compensation in electrical impedance tomography, in 2007 29th Annual International Conference of the IEEE Engineering in Medicine and Biology Society, (2007), 3462–3465. |
[7] |
A. Boyle, A. Adler, The impact of electrode area, contact impedance and boundary shape on EIT images, Physiol. Meas., 32 (2011), 745–754. doi: 10.1088/0967-3334/32/7/S02
![]() |
[8] | A. Calderón, On an inverse boundary value problem, Seminar on Numerical Analysis and its Applications to Continuum Physics (Río de Janeiro, 1980), 65–73. |
[9] | Z. Chen, Reconstruction algorithms for electrical impedance tomography, PhD thesis, University of Wollongong, New South Wales, Australia, 1990. |
[10] |
M. Cheney, D. Isaacson, J. Newell, Electrical impedance tomography, SIAM REVIEW, 41 (1999), 85–101. doi: 10.1137/S0036144598333613
![]() |
[11] |
K.-S. Cheng, D. Isaacson, J. Newell, D. Gisser, Electrode models for electric current computed tomography, IEEE T. Biomed. Eng., 36 (1989), 918–924. doi: 10.1109/10.35300
![]() |
[12] |
M. Crabb, Convergence study of 2D forward problem of electrical impedance tomography with high order finite elements, Inverse Probl. Sci. En., 25 (2017), 1397–1422. doi: 10.1080/17415977.2016.1255739
![]() |
[13] |
M. Darbas, J. Heleine, S. Lohrengel, Numerical resolution by the quasi-reversibility method of a data completion problem for Maxwell's equations, Inverse Probl. Imag., 14 (2020), 1107–1133. doi: 10.3934/ipi.2020056
![]() |
[14] |
M. Darbas, J. Heleine, S. Lohrengel, Sensitivity analysis for 3D Maxwell's equations and its use in the resolution of an inverse medium problem at fixed frequency, Inverse Probl. Sci. En., 28 (2020), 459–496. doi: 10.1080/17415977.2019.1588896
![]() |
[15] |
J. Dardé, N. Hyvönen, A. Seppänen, S. Staboulis, Simultaneous recovery of admittivity and body shape in electrical impedance tomography: An experimental evaluation, Inverse Probl., 29 (2013), 085004. doi: 10.1088/0266-5611/29/8/085004
![]() |
[16] |
J. Dardé, S. Staboulis, Electrode modelling: The effect of contact impedance, ESAIM: M2AN, 50 (2016), 415–431. doi: 10.1051/m2an/2015049
![]() |
[17] |
J. Dardé, H. Hakula, N. Hyvönen, S. Staboulis, Fine-tuning electrode information in electrical impedance tomography, Inverse Probl. Imag., 6 (2012), 399–421. doi: 10.3934/ipi.2012.6.399
![]() |
[18] |
J. Dardé, N. Hyvönen, A. Seppänen, S. Staboulis, Simultaneous reconstruction of outer boundary shape and admittivity distribution in electrical impedance tomography, SIAM J. Imaging Sci., 6 (2013), 176–198. doi: 10.1137/120877301
![]() |
[19] |
M. Dodd, J. Mueller, A real-time D-bar algorithm for 2-D electrical impedance tomography data, Inverse Probl. Imag., 8 (2014), 1013–1031. doi: 10.3934/ipi.2014.8.1013
![]() |
[20] | M. Fernández-Corazza, S. Turovets, P. Govyadinov, C. Muravchik, D. Tucker, Effects of head hodel inaccuracies on regional scalp and skull conductivity estimation using real EIT measurements, in II Latin American Conference on Bioimpedance, Springer, 2016, 5–8. |
[21] |
M. Fernández-Corazza, S. Turovets, P. Luu, N. Price, C. Muravchik, D. Tucker, Skull modeling effects in conductivity estimates using parametric electrical impedance tomography, IEEE T. Biomed. Eng., 65 (2018), 1785–1797. doi: 10.1109/TBME.2017.2777143
![]() |
[22] | M. Fernández-Corazza, N. von Ellenrieder, C. H. Muravchik, Estimation of electrical conductivity of a layered spherical head model using electrical impedance tomography, Journal of Physics: Conference Series, 332 (2011), 12–22. |
[23] |
L. G. Grassi, R. Santiago, G. Florio, L. Berra, Bedside Evaluation of Pulmonary Embolism by Electrical Impedance Tomography, Anesthesiology, 132 (2020), 896–896. doi: 10.1097/ALN.0000000000003059
![]() |
[24] |
H. Hakula, N. Hyvönen, T. Tuominen, On the hp-adaptive solution of complete electrode model forward problems of electrical impedance tomography, J. Comput. Appl. Math., 236 (2012), 4645–4659. doi: 10.1016/j.cam.2012.04.005
![]() |
[25] |
H. Hakula, N. Hyvönen, T. Tuominen, On the hp-adaptive solution of complete electrode model forward problems of electrical impedance tomography, J. Comput. Appl. Math., 236 (2012), 4645–4659. doi: 10.1016/j.cam.2012.04.005
![]() |
[26] |
R. J. Halter, A. Hartov, K. D. Paulsen, A broadband high-frequency electrical impedance tomography system for breast imaging, IEEE T. Biomed. Eng., 55 (2008), 650–659. doi: 10.1109/TBME.2007.903516
![]() |
[27] |
S. J. Hamilton, A. Hauptmann, Deep D-bar: Real-time Electrical Impedance Tomography imaging with deep neural networks, IEEE T. Med. Imaging, 37 (2018), 2367–2377. doi: 10.1109/TMI.2018.2828303
![]() |
[28] | S. Hamilton, D. Isaacson, V. Kolehmainen, P. Muller, J. Toivanen, P. Bray, 3d EIT reconstructions from electrode data using direct inversion D-bar and Calderón methods, arXiv preprint arXiv: 2007.03018. |
[29] | F. Hecht, New development in FreeFem++, J. Numer. Math., 20 (2012), 251–265. |
[30] |
L. Heikkinen, T. Vilhunen, R. West, M. Vauhkonen, Simultaneous reconstruction of electrode contact impedances and internal electrical properties: Ii. laboratory experiments, Meas. Sci. Technol., 13 (2002), 1855–1861. doi: 10.1088/0957-0233/13/12/308
![]() |
[31] | M. Hintermüller, A. Laurain, Electrical impedance tomography: from topology to shape, Control Cybern., 37 (2008), 913–933. |
[32] |
N. Hyvönen, Complete electrode model of electrical impedance tomography: Approximation properties and characterization of inclusions, SIAM J. Appl. Math., 64 (2004), 902–931. doi: 10.1137/S0036139903423303
![]() |
[33] |
N. Hyvönen, L. Mustonen, Smoothened complete electrode model, SIAM J. Appl. Math., 77 (2017), 2250–2271. doi: 10.1137/17M1124292
![]() |
[34] |
N. Hyvönen, P. Piiroinen, O. Seiskari, Point measurements for a Neumann-to-Dirichlet map and the Calderón problem in the plane, SIAM J. Math. Anal., 44 (2012), 3526–3536. doi: 10.1137/120872164
![]() |
[35] |
O. Y. Imanuvilov, G. Uhlmann, M. Yamamoto, The Calderón problem with partial data in two dimensions, J. Am. Math. Soc., 23 (2010), 655–691. doi: 10.1090/S0894-0347-10-00656-9
![]() |
[36] |
J. P. Kaipio, V. Kolehmainen, E. Somersalo, M. Vauhkonen, Statistical inversion and Monte Carlo sampling methods in electrical impedance tomography, Inverse Probl., 16 (2000), 1487–1522. doi: 10.1088/0266-5611/16/5/321
![]() |
[37] | P. Kauppinen, J. Hyttinen, J. Malmivuo, Sensitivity distribution visualizations of impedance tomography measurement strategies, International Journal of Bioelectromagnetism, 8 (2006), 1–9. |
[38] |
C. Kenig, J. Sjostraa, G. Uhlmann, The Calderón problem with partial data, Ann. Math., 165 (2007), 567–591. doi: 10.4007/annals.2007.165.567
![]() |
[39] | R. Kohn, M. Vogelius, Determining conductivity by boundary measurements, Commun. Pure Appl. Math., 37 (1984), 113–123. |
[40] |
V. Kolehmainen, M. Lassas, P. Ola, The inverse conductivity problem with an imperfectly known boundary in three dimensions, SIAM J. Appl. Math., 67 (2007), 1440–1452. doi: 10.1137/060666986
![]() |
[41] |
V. Kolehmainen, M. Lassas, P. Ola, Electrical impedance tomography problem with inaccurately known boundary and contact impedances, IEEE T. Med. Imaging, 27 (2008), 1404–1414. doi: 10.1109/TMI.2008.920600
![]() |
[42] |
A. Lechleiter, A. Rieder, Newton regularizations for impedance tomography: A numerical study, Inverse Probl., 22 (2006), 1967–1987. doi: 10.1088/0266-5611/22/6/004
![]() |
[43] |
X. Li, F. Yang, J. Ming, A. Jadoon, S. Han, Imaging the corrosion in grounding grid branch with inner-source electrical impedance tomography, Energies, 11 (2018), 1739. doi: 10.3390/en11071739
![]() |
[44] | D. Miklavcic, N. Pavselj, F. Hart, Electric Properties of Tissues, vol. 6, 2006. |
[45] |
C. C. A. Morais, B. S. Fakhr, R. R. de Santis Santiago, R. D. Fenza, E. Marutani, S. Gianni, et al., Bedside electrical impedance tomography unveils respiratory chimera in covid-19, Am. J. Resp. Crit. Care, 203 (2021), 120–121. doi: 10.1164/rccm.202005-1801IM
![]() |
[46] |
A. Nissinen, V. Kolehmainen, J. Kaipio, Compensation of modelling errors due to unknown boundary in electrical impedance tomography, IEEE T. Med. Imaging, 30 (2011), 231–242. doi: 10.1109/TMI.2010.2073716
![]() |
[47] | R. Parker, The inverse problem of resistivity sounding, Geophysics, 142 (1984), 2143–2158. |
[48] |
S. Ren, M. Soleimani, Y. Xu, F. Dong, Inclusion boundary reconstruction and sensitivity analysis in electrical impedance tomography, Inverse Probl. Sci. Eng., 26 (2018), 1037–1061. doi: 10.1080/17415977.2017.1378195
![]() |
[49] |
F. Santosa, M. Vogelius, A computational algorithm to determine cracks from electrostatic boundary measurements, Int. J. Eng. Sci., 29 (1991), 917–937. doi: 10.1016/0020-7225(91)90166-Z
![]() |
[50] |
G. Saulnier, A. Ross, N. Liu, A high-precision voltage source for EIT, Physiol. meas., 27 (2006), S221–S236. doi: 10.1088/0967-3334/27/5/S19
![]() |
[51] | O. Shuvo, M. Islam, Sensitivity analysis of the tetrapolar electrical impedance measurement systems using comsol multiphysics for the non-uniform and inhomogeneous medium, Dhaka University Journal of Science, 1 (2016), 7–12. |
[52] |
M. Soleimani, C. Gómez-Laberge, A. Adler, Imaging of conductivity changes and electrode movement in eit, Physiol. Meas., 27 (2006), S103–S113. doi: 10.1088/0967-3334/27/5/S09
![]() |
[53] |
E. Somersalo, M. Cheney, D. Isaacson, Existence and uniqueness for electrode models for electric current computed tomography, SIAM J. Appl. Math., 52 (1992), 1023–1040. doi: 10.1137/0152055
![]() |
[54] |
V. Tomicic, R. Cornejo, Lung monitoring with electrical impedance tomography: technical considerations and clinical applications, J. Thorac. Dis., 11 (2019), 3122. doi: 10.21037/jtd.2019.06.27
![]() |
[55] |
O.-P. Tossavainen, M. Vauhkonen, L. M. Heikkinen, T. Savolainen, Estimating shapes and free surfaces with electrical impedance tomography, Meas. Sci. Technol., 15 (2004), 1402–1411. doi: 10.1088/0957-0233/15/7/024
![]() |
[56] |
P. J. Vauhkonen, M. Vauhkonen, T. Savolainen, J. P. Kaipio, Three-dimensional electrical impedance tomography based on the complete electrode model, IEEE T. Biomed. Eng., 46 (1999), 1150–1160. doi: 10.1109/10.784147
![]() |
[57] | A. Velasco, M. Darbas, R. Mendoza, M. Bacon, J. de Leon, Comparative study of heuristic algorithms for electrical impedance tomography, Philippine Journal of Science, 149 (2020), 747–761. |
[58] |
C. Venkatratnam, F. Nagi, Spatial resolution in electrical impedance tomography: A topical review, Journal of Electrical Bioimpedance, 8 (2017), 66–78. doi: 10.5617/jeb.3350
![]() |
[59] |
T. Vilhunen, J. Kaipio, P. Vauhkonen, T. Savolainen, M. Vauhkonen, Simultaneous reconstruction of electrode contact impedances and internal electrical properties, part i: theory, Meas. Sci. Technol., 13 (2002), 1848–1854. doi: 10.1088/0957-0233/13/12/307
![]() |
[60] |
H. Wang, K. Liu, Y. Wu, S. Wang, Z. Zhang, F. Li, et al., Image reconstruction for electrical impedance tomography using radial basis function neural network based on hybrid particle swarm optimization algorithm, IEEE Sens. J., 21 (2021), 1926–1934. doi: 10.1109/JSEN.2020.3019309
![]() |
[61] |
Z. Wei, D. Liu, X. Chen, Dominant-current deep learning scheme for electrical impedance tomography, IEEE T. Biomed. Eng., 66 (2019), 2546–2555. doi: 10.1109/TBME.2019.2891676
![]() |
[62] |
R. Winkler, A. Rieder, Resolution-controlled conductivity discretization in electrical impedance tomography, SIAM J. Imaging Sci., 7 (2014), 2048–2077. doi: 10.1137/140958955
![]() |
[63] |
Y. Wu, B. Chen, K. Liu, C. Zhu, H. Pan, J. Jia, et al., Shape reconstruction with multiphase conductivity for electrical impedance tomography using improved convolutional neural network method, IEEE Sens. J., 21 (2021), 9277–9287. doi: 10.1109/JSEN.2021.3050845
![]() |
[64] |
Y. Zhang, H. Chen, L. Yang, K. Liu, F. Li, C. Bai, et al., A proportional genetic algorithm for image reconstruction of static electrical impedance tomography, IEEE Sens. J., 20 (2020), 15026–15033. doi: 10.1109/JSEN.2020.3012544
![]() |
[65] |
T. Zhu, R. Feng, J.-Q. Hao, J.-G. Zhou, H.-L. Wang, S.-Q. Wang, The application of electrical resistivity tomography to detecting a buried fault: A case study, J. Environ. Eng. Geoph., 14 (2009), 145–151. doi: 10.2113/JEEG14.3.145
![]() |
1. | Erika Antonette T. Enriquez, Renier G. Mendoza, Arrianne Crystal T. Velasco, Philippine Eagle Optimization Algorithm, 2022, 10, 2169-3536, 29089, 10.1109/ACCESS.2022.3158357 | |
2. | C A Romero-Beltrán, A M González-Vargas, J J Cabrera-López, Non-invasive blood pressure as an application of electrical impedance: a short review, 2021, 2008, 1742-6588, 012013, 10.1088/1742-6596/2008/1/012013 | |
3. | Christos Dimas, Vassilis Alimisis, Nikolaos Uzunoglu, Paul P. Sotiriadis, A Point-Matching Method of Moment with Sparse Bayesian Learning Applied and Evaluated in Dynamic Lung Electrical Impedance Tomography, 2021, 8, 2306-5354, 191, 10.3390/bioengineering8120191 | |
4. | Anil Kumar Khambampati, Sunam Kumar Sharma, Kyung Youn Kim, Estimation of electrode contact impedance and conductivity of single-layer graphene using electrical impedance tomography, 2022, 33, 0957-0233, 084007, 10.1088/1361-6501/ac6c76 | |
5. | Olivia Brabant, Sarah Loroesch, Andy Adler, Andreas D. Waldmann, Anthea Raisis, Martina Mosing, Performance evaluation of electrode design and material for a large animal electrical impedance tomography belt, 2022, 191, 0042-4900, 10.1002/vetr.2184 | |
6. | Zhiwei Tian, Yanyan Shi, Can Wang, Meng Wang, Ke Shen, Classification of Hemorrhage Using Priori Information of Electrode Arrangement With Electrical Impedance Tomography, 2023, 11, 2169-3536, 31355, 10.1109/ACCESS.2023.3262575 | |
7. | Rose Anne D. Alas, Arrianne Crystal T. Velasco, A Sensitivity-Based Algorithm Approach in Reconstructing Images in Electrical Impedance Tomography, 2024, 12, 2169-3536, 146560, 10.1109/ACCESS.2024.3473616 | |
8. | Xiang Tian, Jian’an Ye, Tao Zhang, Liangliang Zhang, Xuechao Liu, Feng Fu, Xuetao Shi, Canhua Xu, Multi-Path Fusion in SFCF-Net for Enhanced Multi-Frequency Electrical Impedance Tomography, 2024, 43, 0278-0062, 2814, 10.1109/TMI.2024.3382338 | |
9. | Teemu Tyni, Adam R. Stinchcombe, Spyros Alexakis, A Boundary Integral Equation Method for the Complete Electrode Model in Electrical Impedance Tomography with Tests on Experimental Data, 2024, 17, 1936-4954, 672, 10.1137/23M1585696 | |
10. | Ting Zhang, Yan Liu, Erfang Ma, Bo Peng, Ardalan Aarabi, Siqi Zhang, Ying Hu, Jing Xiang, Yakang Dai, Flexible-Center Hat Complete Electrode Model for EEG Forward Problem, 2024, 71, 0018-9294, 2287, 10.1109/TBME.2024.3365803 | |
11. | Bruna Gabriela Pedro, John Alexander Gomez Sanchez, Pedro Bertemes-Filho, Sensitivity Approach for Blood Glucose Impedance Data Using the Geselowitz Theorem, 2024, 46, 22141804, 100698, 10.1016/j.sbsr.2024.100698 | |
12. | Ting Zhang, Yan Liu, Liantao Cheng, Erfang Ma, Siqi Zhang, Xiaolin Li, Dezhong Yao, Yakang Dai, Three-Dimensional Electrode Model for EEG Forward Problem, 2025, 72, 0018-9294, 1473, 10.1109/TBME.2024.3509539 | |
13. | Ibrar Amin, Shuaikai Shi, Hasan AlMarzouqi, Zeyar Aung, Waqar Ahmed, Panos Liatsis, Kolmogorov-Arnold Vision Transformer for Image Reconstruction in Lung Electrical Impedance Tomography, 2025, 6, 2644-1268, 519, 10.1109/OJCS.2025.3559390 |
Unit disk | ||||
Electrode | Ⅰ | Ⅱ | Ⅲ | Ⅳ |
4 | -0.0444 | -0.0720 | -0.4054 | -0.0926 |
13 | 0.0003 | 0.0013 | 0.0036 | 0.0354 |
17 | -0.0004 | 0.0023 | -0.0046 | -0.1593 |
30 | 0.0409 | 0.0737 | 0.3779 | 0.0040 |
Head model | |||||
Electrode | Ⅰ | Ⅱ | Ⅲ | Ⅳ | skull |
4 | -0.0040 | -0.0063 | -0.0368 | -0.0102 | -45.80 |
13 | 0.0001 | 0.0002 | 0.0010 | 0.0025 | 0.6728 |
17 | -0.0001 | 7.7E-05 | -0.0012 | -0.0139 | -14.24 |
30 | 0.0034 | 0.0062 | 0.0314 | 0.0011 | 26.24 |
Thorax | ||
Electrode | Ⅰ | Ⅱ |
2 | -0.0020 | -0.0103 |
19 | -0.0001 | -0.0004 |
25 | 0.0101 | 0.0046 |
29 | 0.0017 | 0.0139 |
Unit disk | ||||||
True | A. | B. | C. | D. | E. | |
Approximation | 0.4 | 0.3626 | 0.3671 | 0.5426 | 0.3731 | 0.3743 |
Relative error | 0.0934 | 0.0822 | 0.3566 | 0.0670 | 0.0640 |
Unit disk | ||||
Electrode | Ⅰ | Ⅱ | Ⅲ | Ⅳ |
4 | -0.0444 | -0.0720 | -0.4054 | -0.0926 |
13 | 0.0003 | 0.0013 | 0.0036 | 0.0354 |
17 | -0.0004 | 0.0023 | -0.0046 | -0.1593 |
30 | 0.0409 | 0.0737 | 0.3779 | 0.0040 |
Head model | |||||
Electrode | Ⅰ | Ⅱ | Ⅲ | Ⅳ | skull |
4 | -0.0040 | -0.0063 | -0.0368 | -0.0102 | -45.80 |
13 | 0.0001 | 0.0002 | 0.0010 | 0.0025 | 0.6728 |
17 | -0.0001 | 7.7E-05 | -0.0012 | -0.0139 | -14.24 |
30 | 0.0034 | 0.0062 | 0.0314 | 0.0011 | 26.24 |
Thorax | ||
Electrode | Ⅰ | Ⅱ |
2 | -0.0020 | -0.0103 |
19 | -0.0001 | -0.0004 |
25 | 0.0101 | 0.0046 |
29 | 0.0017 | 0.0139 |
Unit disk | ||||||
True | A. | B. | C. | D. | E. | |
Approximation | 0.4 | 0.3626 | 0.3671 | 0.5426 | 0.3731 | 0.3743 |
Relative error | 0.0934 | 0.0822 | 0.3566 | 0.0670 | 0.0640 |