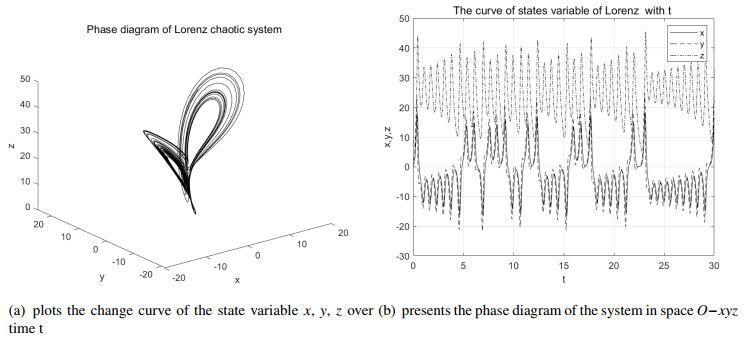
The aim of the present paper is to study pseudoparallel invariant submanifolds of a K-paracontact metric manifold. We consider pseudoparallel, Ricci-generalized pseudoparallel and 2-Ricci generalized pseudo parallel invariant submanifolds of a K-paracontact manifold and we obtain new results. We think contributes to providing some new and interesting results in the area of geometric structures on manifolds geometry.
Citation: Mehmet Atçeken, Tuğba Mert. Characterizations for totally geodesic submanifolds of a K-paracontact manifold[J]. AIMS Mathematics, 2021, 6(7): 7320-7332. doi: 10.3934/math.2021430
[1] | Meng Li, Gulijiamali Maimaitiaili . New results on finite-time projective synchronization for memristor-based hybrid delayed BAM neural networks with applications to DNA image encryption. AIMS Mathematics, 2024, 9(4): 9822-9846. doi: 10.3934/math.2024481 |
[2] | Shamsa Kanwal, Saba Inam, Fahima Hajjej, Ala Saleh Alluhaidan . Securing air defense visual information with hyperchaotic Folded Towel Map-Based encryption. AIMS Mathematics, 2024, 9(11): 31217-31238. doi: 10.3934/math.20241505 |
[3] | Erxi Zhu . Time-delayed feedback control for chaotic systems with coexisting attractors. AIMS Mathematics, 2024, 9(1): 1088-1102. doi: 10.3934/math.2024053 |
[4] | Qinghui Liu, Xin Zhang . Chaos detection in predator-prey dynamics with delayed interactions and Ivlev-type functional response. AIMS Mathematics, 2024, 9(9): 24555-24575. doi: 10.3934/math.20241196 |
[5] | Majid Khan, Hafiz Muhammad Waseem . An efficient confidentiality scheme based on quadratic chaotic map and Fibonacci sequence. AIMS Mathematics, 2024, 9(10): 27220-27246. doi: 10.3934/math.20241323 |
[6] | Erdal Bayram, Gülşah Çelik, Mustafa Gezek . An advanced encryption system based on soft sets. AIMS Mathematics, 2024, 9(11): 32232-32256. doi: 10.3934/math.20241547 |
[7] | Fatma S. Alrayes, Latifah Almuqren, Abdullah Mohamed, Mohammed Rizwanullah . Image encryption with leveraging blockchain-based optimal deep learning for Secure Disease Detection and Classification in a smart healthcare environment. AIMS Mathematics, 2024, 9(6): 16093-16115. doi: 10.3934/math.2024779 |
[8] | Ning Cui, Junhong Li . A new 4D hyperchaotic system and its control. AIMS Mathematics, 2023, 8(1): 905-923. doi: 10.3934/math.2023044 |
[9] | Guilin Tang, Ning Li . Chaotic behavior and controlling chaos in a fast-slow plankton-fish model. AIMS Mathematics, 2024, 9(6): 14376-14404. doi: 10.3934/math.2024699 |
[10] | Abdul Razaq, Louai A. Maghrabi, Musheer Ahmad, Qamar H. Naith . Novel substitution-box generation using group theory for secure medical image encryption in E-healthcare. AIMS Mathematics, 2024, 9(3): 6207-6237. doi: 10.3934/math.2024303 |
The aim of the present paper is to study pseudoparallel invariant submanifolds of a K-paracontact metric manifold. We consider pseudoparallel, Ricci-generalized pseudoparallel and 2-Ricci generalized pseudo parallel invariant submanifolds of a K-paracontact manifold and we obtain new results. We think contributes to providing some new and interesting results in the area of geometric structures on manifolds geometry.
After the relationship between chaos theory [1,2] and cryptography was found. the theory [3,4] has aroused the attention of cryptographers. They have suggested that the properties of quasi-randomness and initial value sensitivity of chaotic systems [5,6] can satisfy the requirements of encryption algorithms [7,8,9]. Subsequently, they have developed a considerable number of excellent encryption algorithms. In encryption algorithms, the pseudo-random sequence generated by the chaotic system is essentially adopted to perform scrambling and diffusion operations on image pixels. This type of algorithm shows the advantages of simple implementation and strong attack resistance, and they fall into three categories, including image encryption algorithms with low-dimensional chaotic systems, image encryption algorithms with high-dimensional chaotic systems, and image encryption algorithms combined with chaotic systems and other methods.
In the algorithm of image encryption with low-dimensional chaotic systems, Guo et al. [10] first proposed the concept of the "chaos password", which draws upon the sequence generated by the Logistic mapping to implement image encryption. The algorithm's security is entirely determined by the generated pseudo-random sequence. Subsequently, a symmetric product cipher was developed using the two-dimensional (2D) Baker map, whereas the operational efficiency of the encryption algorithm cannot be ensured [11]. On the basis of Kolmogoroff flows and shift registers, a block-oriented symmetric cryptosystem was employed to increase image encryption efficiency [12].
The sequence of high-dimensional chaotic systems is recognized to be more irregular and unpredictable as compared with that of low-dimensional chaotic systems. The key space of the algorithm is significantly larger than the key space of the algorithm constructed with low-dimensional chaotic systems [13,14,15]. Accordingly, they are more suitable for image encryption. For instance, the Cat mapping's sequence is employed to perform the scrambling operation; next, the Logistic mapping is adopted for the diffusion operation [16]. The image pixel is scrambled twice by the Henon map and the Lorenz map to increase the efficiency of image encryption [17]. However, the diffusion operation is not implemented on the image pixels, and the algorithm's security cannot be ensured. Based on the problems exposed in [17], the image pixel scrambling operation is performed using the Logistic map, and then the diffusion operation is implemented using the Henon map sequence, so as to improve the algorithm's security [18]. Furthermore, a secure symmetric encryption is developed using the sequence of 3D Cat maps to perform image pixel scrambling operations multiple times [19]. Park proposed a conservative chaotic system [20] with a simple structure, large LEs, continuous chaotic ranges, and high complexity, which is utilized to generate a novel image encryption algorithm exhibiting superior encryption performance. The above algorithms all exhibit a sufficiently large key space to be resistant to brute force attacks.
In the image encryption algorithm combining chaotic systems and other methods, the image pixel scrambling operation is performed using the DNA sequence, and the scrambling operation and the diffusion operation are carried out again using the 2D Logistic chaotic map [24]. The algorithm exhibits strong key sensitivity, while being easy to implement, and even capable of resisting statistical attacks. Furthermore, the plaintext image is first encoded into a DNA sequence, and then the sequence generated by chaos is employed to perform permutation and replacement operations on the encoded image pixels [25].
Since the theory of chaotic cryptography is not well established, the above schemes only demonstrate their security experimentally and cannot provide strict mathematical proofs. Thus, the research of chaotic cryptography is still a challenging frontier subject, which is worth investigating in depth. Existing chaotic encryption algorithms suggest that the security of low-dimensional or hyper-chaotic systems as chaotic passwords cannot be ensured; an attacker can always find a cracking method to predict the sequence came from a chaotic system, as an attempt to decipher the intercepted ciphertext. For instance, the improved CKBA algorithm of image encryption [21] was cracked by Li et al. [22] through selected plaintext and known plaintext attacks. Using the same method, they successfully deciphered the hyperchaotic system-based image encryption algorithm [23]. The chaotic system with time-delay is characterized by its infinite dimension, stronger randomness, more complex dynamic behavior, and being more difficult to predict. Accordingly, the time-delay chaotic system becomes the choice of the next generation of chaotic ciphers. Moreover, the above encryption algorithms always use the sequence generated by a single chaotic system to realize scrambling operations and diffusion operations. This measure can be easily tracked using cryptanalysis techniques, thus causing the lost security of the encryption algorithm and the exposure of the encrypted information. In this paper, the focus is placed on an algorithm of image encryption based on a hybrid heterogeneous chaotic system with time-delay. The main feature of this algorithm refers to using the sequences generated by multiple heterogeneous time-delay chaotic systems to form a sequence set. Three sequences in the set are randomly assigned into image pixel scrambling and diffusion operations during image encryption. This encryption scheme can avoid brute force attacks because it cannot simulate the encryption sequence, and the key space of the encryption algorithm is increased, which improves the security of the algorithm. In addition, the encryption efficiency is improved by adopting non-iterative encryption.
The paper is organized as follows. In this first section, the research status of image encryption technology is briefed. In the second section, the stability and Hopf bifurcation of single-delay chaotic systems are studied. In the third section, image encryption is performed using the heterogeneous single-delay chaotic system. In the fourth section, the algorithm's encryption effect is verified. Lastly, the conclusions of this paper are drawn.
Since chaotic systems with time-delay is characterized by an infinite-dimensional state space and a highly complex dynamic behavior, they are used for serving as a chaotic password [26,27,28,29]. Besides the initial value conditions and parameters of the chaotic system, the chaotic cipher's key space of should also cover the time-delay and the position of the time-delay. Time-delay chaotic systems at different locations exhibit different dynamic behaviors. A chaotic system with time-delays at different positions is termed a heterogeneous time-delay chaotic system. For the state transition of a time-delay chaotic system, the bifurcation criticality of the system should be explored, in which Hopf bifurcation [30,31] has been most commonly investigated.
Over the past few years, there have been rare studies of the Hopf bifurcation of chaotic systems with time-delay. In general, the linearization of the time-delay chaotic system at the singular point is the transcendental equation. Subsequently, the roots' distribution of the transcendental equation determines the Hopf bifurcation condition of the chaotic system with time-delay, which was analyzed by Hale [32]. The above result lays a theoretical basis for the Hopf bifurcation study of time-delay chaotic systems. Wei Junjie et al. [33] proposed the exponential polynomial zero-point distribution theorem based on Rouche's theorem, which has deepened the study of Hopf bifurcation theory. Faria and Magalhães [34,35,36] generalized and applied canonical theory to time-delay differential equations. They proposed a canonical calculation method which significantly contributed to the development of the bifurcation theory. Thus far, the bifurcation study of time-delay chaotic systems has been initiated and gradually deepened. Gamal et al. [37] presented a generalized form of the time-delay Lorenz system. This Lorenz system had 2n+1dimensions. Furthermore, they investigated the stability of trivial and non-trivial fixed points and the existence Hopf bifurcation conditions. Kun et al. [38] adopted the improved undetermined coefficient method to validate the homoclinic orbit of the Chen system with time delay feedback and proposed the helical involute projection method. Lian et al. [39] conducted the Hopf bifurcation study of Lorenz-like systems with time-delay. Additionally, Li et al. [40] conducted a Hopf bifurcation study of interfering Lorenz-like systems with time-delay. The research and analysis of the mentioned literature confirm that different time delay positions significantly affect the dynamic behavior of the system. In a 3D general Lorenz system, multiple heterogeneous single delay chaotic systems can be formed using time delays at different positions and can be generated by the sequence that the image encryption algorithm is dependent on.
The unified system was proposed by Lü et al. in 2002. The system links the Lorenz system, Lü system and Chen system. Its system model is expressed as
{˙x=(25α+10)(y−x)˙y=(28−35α)x−xz+(29α−1)y˙z=xy−(8+α)z/3 | (2.1) |
where x, y, z denote state variables and α∈[0,1] represents the system parameter. When α∈[0,0.8), the system pertains to the Lorenz system; when α∈(0.8,1], the system belongs to the Chen system; and when α=0.8, the system belongs to the Lü system. The unified chaotic system model expresses the basic structure of the Lorenz model well through a single parameter. However, its system parameters are too singular, limiting the parameter range. Subsequently, researchers formed numerous deformations of Lorenz systems based on continuous updating (e.g., Lorenz-like systems [41]), and presented corresponding bifurcation laws and stability conditions [42]. Without loss of generality, this paper elucidates a general Lorenz system and the bifurcation law of its heterogeneous single-delay chaotic system. The system's equation is expressed as:
{˙x=a(y−x)˙y=bx+dy−xz˙z=−cz+xy | (2.2) |
where a, b, c, d denote system parameters. Equation (2.2) contains seven terms, of which there are only two nonlinear terms, compared with other chaotic or hyperchaotic systems. Since the structure of the system is simpler, the circuit implementation can be achieved more easily. Thus, the system has a promising application in secure communication and other fields. Figure 1 illustrates the phase diagram and state vector trend of a general Lorenz system over time when a=10, b=28, c=8/3, and d=−1.
Figure 1 suggests that the general Lorenz system has two chaotic attractors with two wings and the state variable keeps periodic oscillation permanently with time. It is therefore implied that the system has entered a chaotic state.
Numerous researchers have obtained the time-delayed Lorenz chaotic system by imposing time-delay on the state variables. However, different positions of time delays can be introduced to form functional differential dynamical systems with different dynamical behaviors. In Eq (2.2), applying a single time delay to the state variables, nine forms of heterogeneous single time-delay chaotic systems can be established.
{˙x=a(y(A)−x(B))˙y=bx(C)+dy(D)−x(E)z(F)˙z=−cz(G)+x(H)y(I) |
where A−Z represents the imposition of a time delay of the form t−τ and τ(>0) denotes time lag. The nine heterogeneous time-delay chaotic systems consist of three identical equilibrium points:
(0,0,0),(√(b+d)c,√(b+d)c,b+d),(−√(b+d)c,−√(b+d)c,b+d). |
When the parameters a>0, b+d<0, c>0, the system contains a unique equilibrium point O(0,0,0), and the time-delay general Lorenz system shows a better parameter range than other systems. Next, the stability of O(0,0,0) of a single delay Lorenz system with a delay at position A (System A for short) is considered.
The linearization equation of system A at the point O(0,0,0) is expressed as
{˙x=a[y(t−τ)−x]˙y=bx+dy˙z=−cz | (2.3) |
where the value range of a, b, c, and d is a>0, b+d<0, c>0, a+c>d, |d|>|b|. The characteristic equation of Eq (2.3) is expressed as
|−a−λae−λτ0be−λ000−c−λ|=0. | (2.4) |
Equation (2.4) can be reduced to
λ3+(a+c−d)λ2+(ca−cd−ad)λ−acd−ab(λ+c)e−λτ=0. | (2.5) |
Where p1=a+c−d; p2=ca−cd−ad; p3=−acd; p4=−ab; p5=−abc. Thus, the following lemma can be expressed as follows.
Lemma 1. If τ=0, a>0, b+d<0, c>0, a+c>d and |d|>|b|, the zero equilibrium point of system A is locally asymptotically stable.
Proof. When τ=0, the characteristic Eq (2.5) is transformed into
λ3+p1λ2+(p2+p4)λ+p3+p5=0. | (2.6) |
Since a>0, b+d<0, c>0, a+c>d, and |d|>|b|, it is easy to obtain that p1>0, p2+p4>0, and p3+p5>0. According to the Routh-Hurwitz theorem, all roots of Eq (2.6) are common in having negative real parts. Thus, the point O(0,0,0) of system A is asymptotically stable when τ=0.
When τ>0, suppose λ=iω (ω is an undetermined constant greater than zero) is a pure imaginary root of Eq (2.5) so that the imaginary part ω satisfies
−iω3−p1ω2+ip2ω+p3+(ip4ω+p5)(cosωτ−isinωτ)=0. | (2.7) |
According to the equality of plural numbers, it can be obtained that
{p5cosωτ+p4ωsinωτ=p1ω2−p3p4ωcosωτ−p5sinωτ=ω3−p2ω. | (2.8) |
Equation (2.8) can be equivalently transformed into
ω6+(p21−2p2)ω4+(p22−2p1p3−p24)ω2+p23−p25=0. | (2.9) |
A conclusion for Eq (2.9) can be reached as follows.
Lemma 2. If a>0, b+d<0, c>0, a+c>d and |d|>|b|, Eq (9) has at least one positive real root.
Proof. Set u=ω2. Then, Eq (2.9) can be reduced to
u3+(p21−2p2)u2+(p22−2p1p3−p24)u+p23−p25=0. | (2.10) |
Suppose
f(u)=u3+(p21−2p2)u2+(p23−2p1p3−p24)u+p23−p25. | (2.11) |
Equation (2.11) can be converted into
f(u)=1+(p21−2p2)1u+(p23−2p1p3−p24)1u2+(p23−p25)1u31u3. | (2.12) |
It can be derived from Eqs (2.11) and (2.12) that
f(0)=p23−p25<0, limu→+∞f(u)=+∞. |
According to the zeros value theorem, there is at least one real number u0∈(0,+∞) that makes f(u0)=0. Thus, Eq (2.10) has one positive real root at minimum. Since u=ω2, Eq (2.9) has at least one positive real root.
Suppose ω0 is a real root of Eq (2.9). Then, Eq (2.5) has a pure imaginary root iω0. It can be obtained from Eq (2.8) that
cosωτ=p4ω4−(p1p5+p2p4)ω2+p3p5p24ω2+p25. | (2.13) |
By substituting ω=ω0 into Eq (2.13), the time-delay τ can be calculated as:
τk=1ω0arccos(p4ω40−(p2p4−p1p5)ω20−p3p5p24ω20+p25)+2kπω0,k=0,1,2,⋯ | (2.14) |
Thus, (ω0,τk) is the solution of Eq (2.5), suggesting that λ=±iω0 is a pair of conjugate pure imaginary roots of Eq (2.5) when τ=τk.
Suppose τ0=min{τk}. Then, time delay τ=τ0 is the minimum value when the pure imaginary root λ=±iω0 of Eq (2.5) appears. Thus, we have the following lemma.
Lemma 3. If a>0, b+d<0, c>0, a+c>d, |d|>|b|, and τ=τ0, then, Eq (2.5) has a pair of pure imaginary roots λ=±iω0.
Suppose λ(τ)=α(τ)+iω(τ) satisfies α(τk)=0 and ω(τk)=ω0. The following presents the transversal conditions.
Lemma 4. If a>0, b+d<0, c>0, a+c>d, |d|>|b| and f′(ω20)>0, then dReλ(τ)dτ|τ=τk>0.
Proof. The derivation regarding τ of both sides of Eq (2.5) is performed to obtain
[3λ2+2p1λ+p2+p4e−λτ−τ(p4λ+p5)e−λτ]dλdτ=λ(p4λ+p5)e−λτ. | (2.15) |
It can be calculated according to Eq (2.5) that
(p4λ+p5)e−λτ=λ(λ2+p1λ+p2). | (2.16) |
Substituting Eq (2.16) into Eq (2.15) yields
(dλdτ)−1=−3λ2+2p1λ+p2λ2(λ2+p1λ+p2)+p4λ(p4λ+p5)−τλ | (2.17) |
τk=iω0 and therefore
Re[(dλdτ)−1|τ=τk]=−Re[3λ2+2p1λ+p2λ2(λ2+p1λ+p2)|τ=τk]+Re[p4λ(p4λ+p5)|τ=τk]=Re[−3ω20+2ip1ω0+p2ω20(ω20−ip1ω0−p2)]+Re(p4p4ω20−ip5ω0))=(p2−3ω20)(ω20−p2)−2p21ω20ω20[(p2−ω20)2+p21ω20]−p24p24ω20+p25. | (2.18) |
When τ=τk, Eq (2.5) has pure imaginary roots iω0, which are substituted into Eq (2.5) to obtain
−iω30−p1ω20+ip2ω0+p2−(ip4ω0+p5)e−iω0τ=0 | (2.19) |
|e−iω0τ|=1, because e−iω0τ=cosω0τ−isinω0τ. Thus, it can be calculated using Eq (2.19) that
|−p1ω20+p3+i(p2ω0−ω30)|=|−p5−ip4ω0)|. |
Namely,
ω20(p2−ω20)2+(p1ω20−p3)2=(p4ω0)2+p25. | (2.20) |
As obtained by combining Eqs (2.18) and (2.20),
Re[(dλdτ)−1|τ=τk]=3ω40+2(p21−2p2)ω20+(p22−2p1p3−p24)p24ω20+p25=f′(ω20)p24ω20+p25>0. |
Sign[Re(dλdτ|τ=τk)]=Sign{Re[(dλdτ)−1|τ=τk]}. Thus, the lemma is proved.
According to Lemma 4 and Hopf bifurcation theory, we draw the following conclusions.
Theorem 1. If a>0, b+d<0, c>0, a+c>d, |d|>|b|, and f′(ω20)>0, then,
(1) when τ∈[0,τ0), system A is asymptotically stable at the point O(0,0,0)
(2) when τ>τ0, system A is unstable at the point O(0,0,0)
(3) τ=τk(k=0,1,2,⋯) is the Hopf bifurcation value of system A, suggesting that Hopf bifurcation occurs at the point O(0,0,0) in system A.
Considering that the parameters of system A are b+d<0, c>0 and a+c>d, system A is simulated with a=10, c=2.5 and d=2. In this case, system A can be converted into
{˙x=10y(t−τ)−10x˙y=−4x+2y−xz˙z=−2.5z+xy. | (2.21) |
It can be calculated using mathematical software that the positive real root of Eq (2.9) is ω0=3.2376, f′(ω20)=2.0909×103>0, and τ0=0.2173 in Eq (2.14). Thus, Corollary 1 can be simplified into the following corollaries.
Corollary 1. If a>0, b+d<0, c>0, a+c>d and f′(ω20)>0, then,
(1) When τ∈[0,0.2173), system (2.21) is asymptotically stable at the point O(0,0,0).
(2) When τ>0.2173, system (2.21) is unstable at the point O(0,0,0).
(3) τ=0.2173+0.6177kπ(k=0,1,2,3,⋯) is the Hopf bifurcation value of system (2.21), suggesting that a Hopf bifurcation occurs in system (2.21) at the point O(0,0,0), leading to limit cycles.
Mathematical software is applied to draw the state variables' trajectory diagram and phase diagram when the time-delay takes different values, as illustrated in Figures 2–4. The results obtained is verified.
As shown in Figure 2, the value of the state variable of system (2.21) approaches the equilibrium point O(0,0,0) over time and as a result of which the equilibrium point O(0,0,0) of system (2.21) is asymptotically stable.
It can be observed in Figure 3 that when τ=0.2173, the variable x,y,z keeps periodic oscillation, and a limit cycles appear in the O−xyz space, suggesting that a Hopf bifurcation occurs at the point O(0,0,0).
As shown from Figure 4, the value of the state variables x,y,z of system (2.21) shifts away from the equilibrium point progressively with time t, suggesting that the point O(0,0,0) is unstable when τ=0.25.
The system's chaos is further verified in the following and the bifurcation diagram of system (2.21) over τ and the phase diagram of system (2.21) when τ=0.3 are respectively drawn, as shown in Figure 5. Figure 5(a) shows the bifurcation diagram of system (2.21) and system (2.21) enters chaos when τ is close to 0.3, further verifying the inferred results. Figure 5(b) shows the attractive substructure of the system, which can be observed to be complex and not similar to the Lorenz system or other chaotic systems.
Based on the above analysis method, the Hopf bifurcation critical values of the heterogeneous single-delay general Lorenz system are obtained using time delays at different positions under a=10, b=−4, c=2.5, and d=2, as listed in Table 1.
Position | A | B | C | D | E | F | G | H | I |
Hopf bifurcation | |||||||||
critical value | 0.2173 | 0.1850 | 0.3276 | 0.3819 | 0.8107 | 0.2765 | 6265 | 0.4396 | 0.2253 |
A sequence set is constructed from the chaotic sequences generated by nine heterogeneous single-delay chaotic systems, and three sequences are randomly assigned into image pixel scrambling and diffusion operations.
Figure 6 shows the whole process of encrypting and decrypting images by combining the chaotic sequences generated by nine heterogeneous single time-delay chaotic systems. The sequence generated by the chaotic system exhibits pseudo-random properties, and it is employed for scrambling and diffusion operations during encryption, so as to form a chaotic cipher. The chaotic cipher is characterized by a large key space, strong self-adaptation of the key stream and high security. With the theory of chaos having been deeply researched, researchers have revealed that low-dimensional or high-dimensional chaotic systems are subject to problems (e.g., simple structure and periodic windows). It is therefore revealed that the sequence resistance of the low-dimensional chaotic system is weak and easy to crack, and the security of the password cannot be ensured. Moreover, the chaotic cipher adopts the sequence of a single chaotic system for scramble operations and diffusion operations. The sequence is extremely easy to identify, and cryptographic security is not ensured. In accordance with the above problems, a sequence set composed of chaotic sequences came from multiple heterogeneous single-delay chaotic systems as appeared in this paper, and three sequences from the set are randomly selected for image pixel scrambling and diffusion operations. Thus, the cryptanalysis technology cannot capture the properties of the chaotic system, which is conducive to ensuring the password's security.
The sequences from the heterogeneous time-delay chaotic system are employed to form a sequence set, and three sequences are randomly selected from the set for image scrambling and diffusion operations. It is therefore ensured that the randomly selected sequence does not necessarily originate from a chaotic system with a single time-delay, and cryptographic security is achieved.
Chaotic systems with time-delay and non-time-delay are essentially different in a considerable number of ways, and their dynamic behaviors are completely different. Time-delay chaotic systems refer to a class of infinite-dimensional dynamical systems. No matter how small the time delay is, the system's state space is infinite-dimensional and exhibits more complex dynamic behavior. Before encryption, nine heterogeneous single-delay general Lorenz systems are adopted to simultaneously generate 27 pseudo-random sequences for the formation of a sequence set. Subsequently, three sequences corresponding to the images after the color image separation channel are randomly selected from the sequence set for scrambling operations and diffusion operations. Accordingly, the chaotic cipher's key space is larger than that of the previous chaotic cipher. If the image size is M×N×3, the amount of data generated using the time-delay chaotic system is significantly larger than M×N. N and M denote the horizontal and vertical pixel values of the image, respectively.
The scrambling operation is the primary work of the encryption algorithm. To be specific, randomly selected sequences from the sequence set are arranged in an ascending order, and then the pixel positions of the plaintext image are transformed according to the sequence, so as to achieve the location of the obfuscated plaintext image pixels. The diffusion operation randomly selects sequences from the sequence set again to build a matrix and multiplies the matrix by the scrambled pixel matrix to capture the pixel matrix of the encrypted image.
For a color plaintext image P with a size of M×N×3, where 3 denotes the number of channels of the color image, in accordance with the channel, P falls into three layers of PR, PG, and PB, and scrambling and diffusion operations are performed for each layer. With PR as an example, the 2D image matrix PR is expanded into a one-dimensional vector by row or column, expressed as A; the following operations are performed with a sequence X randomly selected from the sequence set:
(1) Truncate the number of M×N at the given position parameter d and X to form {xi,i=1,2,⋯,MN}; each element of {xi} is rounded according to Eq (3.1).
\begin{equation} {x_i} = ({x_i}*{10^4})\mod MN + 1. \end{equation} | (3.1) |
(2) Arrange \{ {x_i}\} in ascending order, remove duplicates, and perform a difference operation with the set \{ 1, 2, 3, \cdots, MN\} . The results are sorted in ascending order and added to the end of \{ {x_i}\} .
(3) Swap the A({x_i}) and A({x_{MN - i + 1}}) positions. After the scrambling operation ends, the vector after scrambling is recorded as {P_{SR}} .
Sequences are selected randomly again from the sequence set to build a matrix, and a diffusion operation is carried out on the matrix of the scrambled image. The diffusion operation can be performed using Eq (3.2), and its inverse operation is Eq (3.3). The diffusion operation and the scrambling operation of the plaintext image are over.
\begin{equation} {C_i} = {C_{i - 1}} * {x_i} * {P_{SRi}} \end{equation} | (3.2) |
\begin{equation} {P_{SRi}} = {C_i} / {C_{i - 1}} / {x_i} \end{equation} | (3.3) |
The decryption algorithm is the inverse encryption algorithm's process, described as follows:
Algorithm: Image encryption algorithm based on heterogeneous time-delay chaotic system. |
Input: Plaintext image; |
Encryption key: chaotic system parameters, time delay position, time delay value, truncation sequence position parameter; |
Output: Ciphertext image; |
Step 1: Read plaintext images, construct matrices {P_R} , {P_G} and {P_B} , and form column matrices {A_R} , {A_G} and {A_B} , respectively. |
Step 2: Establish a sequence set in accordance with the key information; randomly select three sequences (X, Y, Z) from the set; intercept the sequence following the position parameter. |
Step 3: Perform a scrambling operation on {P_R} , {P_G} and {P_B} . According to Eq (3.1), round, sort and append the intercepted sequence \{ {x_i}\} to form a novel sequence \{ {x_i}\} . Then, exchange the pixel positions in {A_R} , {A_G} and {A_B} to form a scrambling matrix {P_{SR}} , {P_{SG}} and {P_{SB}} . |
Step 4: Implement a diffusion operation on {P_{SR}} , {P_{SG}} and {P_{SB}} . Again, three sequences (X, Y, Z) are randomly selected from the set, and the sequences are truncated based on the position parameter. The interception sequence \{ {x_i}\} is adopted for operation according to Eq (3.2) to form diffusion matrices {P_{DR}} , {P_{DG}} and {P_{DB}} . |
Step 5: Combine {P_{DR}} , {P_{DG}} and {P_{DB}} . |
Decryption algorithm is the reverse process of encryption algorithm, as presented below:
Algorithm: Image Decryption algorithm based on heterogeneous time-delay chaotic system.. |
Input: Ciphertext image; |
Encryption key: chaotic system parameters, time delay position, time delay value, truncation sequence position parameter; |
Output: Decrypted image; |
Step 1: Read the ciphertext image and construct the matrix {C_R} , {C_G} and {C_B} . |
Step 2: Form a sequence set according to the key information; select three sequences (X, Y, Z) from the set in the sequence selection manner; intercept the pseudo-random sequence \{ {x_i}\} following the position parameter. |
Step 3: Implement the inverse diffusion operation on {C_R} , {C_G} and {C_B} ; use the intercepted sequence \{ {x_i}\} to operate based on Eq (3.3) to form matrices {C_{DR}} , {C_{DG}} and {C_{DB}} . |
Step 4: Perform the scrambling inverse operation on {C_{DR}} , {C_{DG}} and {C_{DB}} . Again, three sequences (X, Y, Z) are selected from the set by sequence selection. According to Eq (3.3), round, sort and append the intercepted sequence to form a novel sequence \{ {x_i}\} . Subsequently, exchange the pixel positions in {C_{DR}} , {C_{DG}} and {C_{DB}} to establish a matrix {C_{SR}} , {C_{SG}} and {C_{SB}} . |
Step 5: Combine {C_{SR}} , {C_{SG}} and {C_{SB}} . |
The algorithm's effectiveness is verified by taking the Lena color image of size 1 as the test object. Color images' encryption and decryption are performed using the aforementioned heterogeneous single delay Lorenz system to form a sequence set. The number of sequence elements generated by the chaotic system with time-delay is significantly larger than the size of the color image, and is stored in a fixed manner. In the sequence, a certain number of elements are intercepted based on the position parameters to participate in the diffusion and scrambling operations. Table 1 and Figure 4 depict the parameters and initial conditions of the heterogeneous single-delay Lorenz system, with the location parameter of {d_i} \ge 300 . The encryption algorithm's performance is evaluated through histogram analysis, adjacent pixel correlation coefficient analysis, NPCR and UACI analysis.
Figure 7 illustrates the R , G , and B pixel histograms for the plaintext image. Figure 8 exhibits the R , G , and B pixel histograms for the encrypted image. Figure 9 depicts the R , G , and B pixel histograms for the decrypted image.
Figure 7 depicts that the pixel values' distribution of the R, G and B channels of the plaintext image has apparent statistical laws, and the plaintext image's pixel value change curve is significant. After scrambling and expansion operations are completed, the pixel values of the ciphertext image are evenly distributed in the respective gray level interval (Figure 8). In other words, the frequency of the respective encrypted image's pixel appears close. Moreover, the three channels are basically the same, and thus, statistical methods cannot be adopted to attack. As demonstrated in Figure 9, the pixel values' distribution of the R, G and B channels of the plaintext image is basically the same as that of the decrypted image. This suggests that the decryption effect is perfect, and the image can be restored to the plaintext image without being affected by the decryption algorithm. The above results confirm that the information of the plaintext image is effectively diffused, and the encryption algorithm has a good ability to resist statistical attacks.
The Pearson correlation coefficient is capable of effectively measuring the dependence of two adjacent sequences in an image in a certain direction. Next, the correlation coefficient is calculated directly using Eq (3.4) for the images before and after encryption.
\begin{equation} r = \frac{{n(\sum\limits_{i = 1}^n {{x_i}{y_i}} ) - (\sum\limits_{i = 1}^n {{x_i}} )(\sum\limits_{i = 1}^n {{y_i}} )}}{{\sqrt {[n(\sum\limits_{i = 1}^n {x_i^2} ) - {{(\sum\limits_{i = 1}^n {{x_i}} )}^2}][n(\sum\limits_{i = 1}^n {y_i^2} ) - {{(\sum\limits_{i = 1}^n {{y_i}} )}^2}]} }} \end{equation} | (3.4) |
where n(\sum\limits_{i = 1}^n {{x_i}{y_i}}) - (\sum\limits_{i = 1}^n {{x_i}})(\sum\limits_{i = 1}^n {{y_i}}) denotes the sample variance, n(\sum\limits_{i = 1}^n {x_i^2}) - {(\sum\limits_{i = 1}^n {{x_i}})^2} and n(\sum\limits_{i = 1}^n {y_i^2}) - {(\sum\limits_{i = 1}^n {{y_i}})^2} denote the sample standard deviations of the sequences {X_j} and {Y_j} (j = 1, 2, \cdots, m - 1) , respectively. The correlation coefficient is one of the important indicators reflecting the encryption algorithm's performance. In general, the correlation coefficient between adjacent pixels can be calculated from the vertical direction, the horizontal direction, and the diagonal direction according to Eq (3.4). When determined in the horizontal direction, m is the row number of the encrypted image; when obtained in the vertical direction, m is the column number of the encrypted image; when calculated from the diagonal direction, m is the diagonal line number of the encrypted image. Table 2 lists the calculation result.
Image | Horizontal direction | Vertical direction | Diagonal direction | ||||||
R | G | B | R | G | B | R | G | B | |
Original image | 0.9002 | 0.9016 | 0.9675 | 0.9412 | 0.9522 | 0.9213 | 0.9423 | 0.9702 | 0.9772 |
Encrypted image | 0.0122 | 0.0023 | 0.0206 | 0.0012 | 0.0056 | 0.0165 | 0.0025 | 0.0021 | 0.0024 |
Table 2 depicts that the encrypted image's correlation coefficients on the vertical, horizontal and diagonal lines all approach zero for the encryption implemented under the heterogeneous single-delay Lorenz system mapping. However, the above are just some of the overall results. The correlation of the plaintext image and the encrypted image in different directions are depicted one by one using a scatter plot to analyze the correlation between any two adjacent pixels in depth. Figure 10 illustrates the correlation coefficient results for the Lena image in the horizontal, vertical, and diagonal directions. Figure 11 illustrates the correlation coefficient results of the encrypted image in horizontal, vertical, and diagonal directions.
As depicted in Figure 10, the plaintext image shows a high correlation between any two adjacent pixels in different directions. However, the correlation between adjacent pixels corresponding to the encrypted image is close to 0 (Figure 11). To be specific, the correlation coefficients changed from 0.9002, 0.9412 and 0.9423 to 0.0122, 0.0012 and 0.0025, respectively. It is therefore revealed that the encryption algorithm eliminates the correlation between the plaintext image's adjacent pixels, thus making it difficult for attackers to acquire any information of the plaintext image through correlation analysis.
The keyspace elements of the encryption and decryption algorithm consist of the time-delay Lorenz system's parameters a , b , c , initial conditions x(t) , y(t) , z(t) , time-delays {\tau _i} , i = 1 to 9 , time-delay position parameters, as well as intercepting pseudo-random sequences' position parameters. In MATLAB, 96-bits of storage system parameters, initial conditions and double delay are required, and the rest can be stored with 64 bits. The position parameter of time-delays is the position relation of time- delay quantities {\tau _i} in the Lorenz system, and different position relations express a chaotic system with time-delay. Moreover, the pseudo-random sequence interception positions are three random values, respectively representing the value positions on different state vectors. Accordingly, the number of all the different combinations of this cryptosystem is significantly higher than 2256. The above large keyspace and the use at a time make the encryption algorithm sufficiently resistant to brute force attacks.
Key sensitivity is recognized as another significant indicator to measure the image encryption algorithm's performance. Encryption algorithms with prominent performance should generally be sensitive to keys, so cryptanalysts cannot crack them by repeated trials. The sensitivity here reveals that the decryption algorithm cannot recover the plaintext image or acquire the relevant information of the plaintext image even after a small modification of any element in the keyspace. In other words, only when a completely correct key is employed can the plaintext image be recovered by a decryption algorithm. Figure 12 presents the image (12 (b)) obtained by decryption with a correct key, the image (12 (c)) obtained by decryption with a wrong delay parameter {\tau} , and the image (12 (d) obtained by intercepting position parameters in the direction of wrong pseudo-random sequence x under the time-delay quantities of {\tau_A} = 0.1521 .
According to Figure 12(b), (c) when the parameters of the heterogeneous single time-delay chaotic system change slightly and other keys are adopted correctly, the decryption algorithm can neither recover and obtain the plaintext image nor acquire any information of the plaintext image. According to Figure 12(c), (d) the decryption algorithm cannot acquire any plaintext image's information when the position parameters intercepted by the pseudo-random sequence are changed or when other keys remain unchanged. This is because the small changes of system parameters and the changes of sequence interception positions generally cause a completely different chaotic matrix B , so the matrix A determined by A = CB is overall inconsistent with the correct scrambling matrix. Thus, the decryption algorithm cannot recover the plaintext image.
The average intensity of change (UACI) and the average rate of change of pixels (NPCR) represent two indicators to measure the diffusion effect of encryption algorithms. NPCR compares the number of changes of elements in the pixel matrix corresponding to the plaintext image and the encrypted image to ensure that sufficient elements of the pixel matrix are changed. UACI reveals the average change in the pixel value of the corresponding position of the plaintext image and the encrypted image. They are defined as
\begin{equation} NPCR = \frac{{\sum\limits_{i = 0}^{M - 1} {\sum\limits_{j = 0}^{N - 1} {D(i,j)} } }}{{M \times N}} \times 100\% \end{equation} | (3.5) |
\begin{equation} UACI = \frac{1}{{M \times N}}\sum\limits_{i = 0}^{M - 1} {\sum\limits_{j = 0}^{N - 1} {\frac{{\left| {A(i,j) - {A_{SD}}(i,j)} \right|}}{{255}}}. } \end{equation} | (3.6) |
Here,
D(i,j) = \left\{ {\begin{array}{*{20}{c}} {0,A(i,j) = {A_{SD}}(i,j)}\\ {1,A(i,j) \ne {A_{SD}}(i,j)} \end{array}} \right. |
where A denotes the plaintext image pixel matrix; M and N represent its rows and column numbers, respectively; and {A_{SD}} represents the encrypted image's pixel matrix. For two random images, the expected values for UACI and NPCR are 96.6094% and 33.4635%, respectively [43]. The NPCR and UACI of the encrypted image are close to their respective expected values, which indicates that the information of the plaintext image is significantly diffused into the encrypted image. Accordingly, the attacker's attempt to obtain information about the plaintext image through differential attack will be futile.
In accordance with Eqs (3.5) and (3.6), the UACI and NPCR values of the encrypted image can be calculated respectively. Table 3 lists the calculation results.
Image | NPCR(%) | UACI(%) | ||||
R | G | B | R | G | B | |
Encrypted image | 99.6011 | 99.6066 | 99.6200 | 33.1101 | 33.2622 | 33.3230 |
Average | 99.6092 | 33.2384 |
The results in Table 3 reveal that the average NPCR of encrypted images is 99.6092%, and the average UACI is nearly 33.2384%, both close to the expected value. To obtain better NPCR and UACI values, the number of columns should be up-regulated in the permutation matrix A. At this time, the size of the required chaos matrix B will increase. In this way, the information of the plaintext image will be better diffused into the encrypted image. Accordingly, the algorithm can effectively resist differential attacks.
The correlation coefficient's reduction between adjacent pixels of an encrypted image is an essential criterion to measure the encryption algorithm's security. This paper attempts to reflect the proposed algorithm's security by comparing it with other encryption algorithms. The image encryption schemes involved in the comparison consist of image encryption algorithms based on multiple chaotic systems [43], bit-level scrambling and diffusion [21,44], generalized Arnold map [12], DNA sequence operation [24], and cyclic shift and XOR operation [45]. Table 4 lists the correlation coefficients between adjacent encrypted images' pixels under various schemes. To be specific, the results of correlation coefficient in the respective direction can be obtained by averaging the correlation coefficients of the R, G, and B layer pixel matrices.
Encryption algorithm | Our | Huang[43] | Lin[21] | Ye[12] | Zhang[24] | Zahra[45] |
Horizontal direction | 0.0012 | -0.0752 | 0.0315 | 0.0464 | 0.0713 | 0.0048 |
Vertical direction | 0.0122 | -0.0753 | 0.2651 | 0.0562 | -0.3255 | 0.0231 |
Diagonal direction | 0.0361 | 0.0466 | 0.0455 | 0.0397 | -0.0423 | 0.0280 |
Average | 0.0169 | 0.0661 | 0.2119 | 0.0478 | 0.1467 | 0.0190 |
As depicted in Table 4, in the comparison of the correlation coefficients of adjacent pixels, the proposed algorithm has the smallest correlation coefficients in the horizontal and vertical directions. In the diagonal direction, the proposed encryption method can lead to a smaller correlation between adjacent pixels of the encrypted image, besides Zahra's result. In the three directions, the results obtained by all algorithms are averaged by taking the absolute value, and the proposed algorithm is also proven to be optimal.
Various image encryption algorithms with excellent statistical results have been selected/cracked by known plaintext image attacks. In this paper, we consider the following measures to prevent this powerful attack. According to Eq (3.1), the permutation-diffusion process is implemented in a single stage, and thus the algorithm can resist divide-and-conquer attacks. According to Table 1, the encrypted chaotic sequence is obtained from the delay and the position of the delay in the chaotic system. The key to this algorithm is not using a single chaotic system to generate data, but employing multiple chaotic systems to generate data. Therefore, the encrypted chaotic sequences originating from multiple chaotic systems cannot be simulated and recognized by AI tools, thus preventing the secret key from being cracked.
In the selected plaintext image attack, cryptanalysts have temporary access to encryption equipment and can choose a specific image for encryption, attempting to find the used secret key: First, the cryptanalyst selects a black image with all pixel values fixed at zero (Figure 13(a)). Then, the cryptanalyst encrypts the black image (Figure 13(b)), resulting in the chaotic sequence possibly used in the encryption process (secret key). Afterward, the cryptanalyst can implement the known plaintext image attack using the Lena image (Figure 13(c)), but without success (Figure 13(d)). From Table 1, the Z - value of Figure 13(a) is S = 0 , and the Lena image is encrypted using S \neq 0 . Therefore, we obtain different Z - value and chaotic sequences for each plaintext image.
In image encryption, the Z-value is commonly used to represent a chaotic sequence of pixel values. A chaotic sequence is a deterministic sequence that appears to be random and can be used in encryption algorithms to generate pseudo-random keystreams.In the context described, the Z-value may be a specific value from a sequence generated by a chaotic system, used to represent the initial state of pixel values or some form of key information. Cryptanalysts can utilize this Z-value to attempt to break the encryption algorithm by selecting specific plaintext images for encryption and observing the generated chaotic sequence in order to infer the key information used for encryption.In summary, the Z-value in image encryption can be used as part of a chaotic sequence to generate pseudo-random keystreams, thereby facilitating the process of encrypting and decrypting images.
Information entropy is a mathematical property that determines the randomness, unpredictability, or complexity of a message. If the encryption process does not produce enough disorder at the output, the cryptosystem can be the subject of the entropy attack. According to the information entropy calculation method given in the literature [46,47], we calculated the images before and after encryption respectively. In Table 5, the entropy results of both The Lena 256 × 256 RGB plain image and its corresponding encrypted image are presented. Therefore, the entropy value close to eight in the encrypted image means a highly unpredictable message; hence, the encryption algorithm can resist an entropy attack.
Plain Image | Encrypted Image | |||||
R | G | B | R | G | B | |
H | 7.6215 | 7.9425 | 7.7426 | 7.8695 | 7.9526 | 7.9265 |
An excellent encryption system, if its encrypted image can effectively resist cropping and noise attacks, possesses good robustness. Taking the cropping attack for example, when the encrypted result is subjected to pixel cropping attacks, the quality of the reconstructed image is significantly reduced. Figure 14 demonstrates various degrees of pixel cropping and the corresponding decryption results, along with an analysis of the encryption scheme's resistance to cropping attacks. It can be seen from the figure that the algorithm has a good ability to resist cropping attacks.
In this section, we analyze the speed of the encryption system. To verify the significant improvement in encryption efficiency of the proposed scheme, we separately calculate the encryption time of the Lena image and the encryption time is obtained many times and averaged. From Table 6, it can be seen that the efficiency of encrypting images using this scheme is significantly better than that of encrypting plaintext images. Comparative experiments also show that our scheme has obvious advantages. The three algorithms were implemented using MATLAB 2022b and were implemented on an AMD Ryzen 96 900HX with Radeon Graphics, and the execution efficiency of the algorithms on color images was calculated.
In view of the excellent qualities of time-delay chaotic systems, such as infinite dimension, a unique encryption scheme designed, and the proposed image encryption algorithm has the advantages of large key space, high encryption efficiency and good security. The image encryption algorithm based on heterogeneous time-delay chaotic systems consists of adding a time-delay to different state variables of chaotic system to form multiple very complex time-delay chaotic systems. Subsequently, the resulting sequences generate a set of sequences employed for cryptographic operations (e.g., image scrambling and diffusion). In accordance with the location where the time lag is added, an encryption strategy is formulated in this location. In this paper, the designed image encryption scheme is presented from four perspectives, including an encryption algorithm, decryption algorithm, algorithm performance analysis and comparative analysis. The proposed encryption algorithm is characterized by its large key space, low computational complexity, and strong ability to resist statistical and differential attacks, which is simple. Moreover, this algorithm is easy to implement. Heterogeneous time-delay chaotic systems exhibit complex dynamic behavior and are capable of ensuring the security of the algorithm by generating chaotic sequences with strong randomness. However, this sequence generation calculation takes a long time. The sequence generated by the time-delay chaotic system can be stored in advance, and it can then be selected randomly and intercepted to achieve real-time encryption.
The authors declare they have not used Artificial Intelligence (AI) tools in the creation of this article.
Some of the authors of this publication are also working on these related projects: (1) higher vocational education teaching fusion production integration platform construction projects of Jiangsu province under grant no.2019(26), (2) natural science fund of Jiangsu province under grant no.BK20131097, (3) “Qin Lan project” teaching team in colleges and universities of Jiangsu province under grant no.2017(15), (4) high level of Jiangsu province key construction project funding under grant no.2017(17), (5)High Level Specialty Group Construction Project of Jiangsu province Higher Vocational Education under grant no.2021(1), (6) Vocational education teacher and teaching innovation team of Jiangsu province under grant no.2021(23), (7) Jiangsu province education science 14th FiveYear plan under grant no.D/2021/03/88, (8) Jiangsu province higher education teaching reform research project under grant no.2023JSJG593.
The authors declare no conflict of interest.
[1] | S. K. Hui, V. N. Mishra, T. Pal, Vandana, Some Classes of Invariant Submanifolds of (LCS)_{n}-Manifolds, Ital. J. Pure Appl. Math., 39 (2018), 359–372. |
[2] | V. Venkatesha, S. Basavarajappa, Invariant Submanifolds of LP-Sasakian Manifolds, Khayyam J. Math., 6 (2020), 16–26. |
[3] | S. Sular, C. Özgür, C. Murathan, Pseudoparallel Anti-Invaraint Submanifolds of Kenmotsu Manifolds, Hacettepe J. Math. Stat., 39 (2010), 535–543. |
[4] |
B. C. Montano, L. D. Terlizzi, M. M. Tripathi, Invariant Submanifolds of Contact (\kappa, \mu)-Manifolds, Glasgow Math. J., 50 (2008), 499–507. doi: 10.1017/S0017089508004369
![]() |
[5] |
M. S. Siddesha, C. S. Bagewadi, Invariant Submanifolds of (\kappa, \mu)-Contact Manifolds Admitting Quarter Symmetric Metric Connection, Int. J. Math. Trends Technol., 34 (2016), 48–53. doi: 10.14445/22315373/IJMTT-V34P511
![]() |
[6] | S. Kaneyuki, F. L. Williams, Almost paracontact and parahodge Structures on Manifolds, Nagoya Math. J., 90 (1985), 173–187. |
[7] |
S. Zamkovoy, Canonical Connections on Paracontact Manifolds, Ann. Globanal Geom., 36 (2009), 37–60. doi: 10.1007/s10455-008-9147-3
![]() |
[8] | B. C. Montano, I. K. Erken, C. Murathan, Nullity Conditions in Paracontact Geometry, Differ. Geom. Appl., 30 (2010), 79–100. |
[9] | D. G. Prakasha, K. Mirji, On (\kappa, \mu)-Paracontact Metric Manifolds, Gen. Math. Notes., 25 (2014), 68–77. |
[10] | M. Atçeken, Ü. Yildirim, S. Dirik, Semiparallel Submanifolds of a Normal Paracontact Metric Manifold, Hacet. J. Math. Stat., 48 (2019), 501–509. |
[11] |
D. E. Blair, T. Koufogiorgos, B. J. Papatoniou, Contact Metric Manifolds Satisfying a Nullity Condition, Israel J. Math., 91 (1995), 189–214. doi: 10.1007/BF02761646
![]() |
[12] |
Venkatesha, D. M. Naik, Cetain Results on K-Paracontact and ParaSasakian Manifolds, J. Geom., 108 (2017), 939–952. doi: 10.1007/s00022-017-0387-x
![]() |
[13] |
A. A. Shaikh, Y. Matsuyama, S. K. Hui, On invariant submanifolds of (LCS)n-manifolds, J. Egypt. Math. Soc., 24 (2016), 263–269. doi: 10.1016/j.joems.2015.05.008
![]() |
[14] | S. K. Hui, S. Uddin, A. H. Alkhaldi, P. Mandal, Invariant submanifolds of generalized Sasakian-space-forms, Int. J. Geom. Methods Mod. Phys., 15 (2018), 1–21. |
[15] |
S. K. Hui, J. Roy, Invariant and anti-invariant submanifolds of special quasi-Sasakian manifolds, J. Geom., 109 (2018), 37. doi: 10.1007/s00022-018-0442-2
![]() |
[16] | S. K. Hui, L. I. Piscoran, T. Pal, Invariant submanifolds of (LCS)n-manifolds with respect to quarter symmetric metric connection, Acta Math. Univ. Comenianae, 87 (2018), 205–221. |
[17] | S. Büyükkütük, I. Kişi, V. N. Mishra, G. Oztürk, Some Characterizations of Curves in Galilean 3-Space \mathbb{G}_{3}, Facta Univ., Ser.: Math. Inf., 31 (2016), 503–512. |
[18] |
L. I. Pişcoran, V. N. Mishra, Projectively flatness of a new class of \left(\alpha, \beta\right) -metrics, Georgian Math. J., 26 (2019), 133–139. doi: 10.1515/gmj-2017-0034
![]() |
[19] | V. Deepmala, K. Drachal, V. N. Mishra, Some algebro-geometric aspects of spacetime c-boundary, Math. Aeterna, 6 (2016), 561–572. |
[20] |
K. Drachal, K. Vandana, Some algebraic aspects of the gluing of differential spaces, Georgian Math. J., 27 (2020), 355–360. doi: 10.1515/gmj-2018-0039
![]() |
[21] |
L. I. Pişcoran, V. N. Mishra, S-curvature for a new class of (\alpha, \beta)-metrics, Revista de la Real Academia de Ciencias Exactas, Físicas y Naturales. Serie A. Matemáticas, 111 (2017), 1187–1200. doi: 10.1007/s13398-016-0358-3
![]() |
[22] |
L. I. Pişcoran, V. N. Mishra, The variational problem in Lagrange spaces endowed with a special type of \left(\alpha, \beta\right) -metrics, Filomat, 32 (2018), 643–652. doi: 10.2298/FIL1802643P
![]() |
[23] | S. K. Hui, V. N. Mishra, A. Patra, Examples of Gradient Ricci Solitons on 4-Dimensional Riemannian Manifold, Modell. Appl. Theory, 1 (2016), 23–27. |
1. | Fan-Qi Meng, Gang Wu, A color image encryption and decryption scheme based on extended DNA coding and fractional-order 5D hyper-chaotic system, 2024, 254, 09574174, 124413, 10.1016/j.eswa.2024.124413 | |
2. | Xiaogang Feng, Guankai Han, Fengying Yan, Dongkui Shen, Zongbin Pang, Qi Li, Local bit-level image encryption algorithm based on one dimensional zero excluded chaotic map, 2024, 99, 0031-8949, 065214, 10.1088/1402-4896/ad418d | |
3. | Ahmed Kareem Shibeeb, Salah Albermany, Sadiq A. Mehdi, 2024, Construct and Dynamic Analyzing of a Novel Eight-Dimensional Hyperchaotic System, 979-8-3503-9203-6, 47, 10.1109/ICAISD63055.2024.10895894 |
Position | A | B | C | D | E | F | G | H | I |
Hopf bifurcation | |||||||||
critical value | 0.2173 | 0.1850 | 0.3276 | 0.3819 | 0.8107 | 0.2765 | 6265 | 0.4396 | 0.2253 |
Image | Horizontal direction | Vertical direction | Diagonal direction | ||||||
R | G | B | R | G | B | R | G | B | |
Original image | 0.9002 | 0.9016 | 0.9675 | 0.9412 | 0.9522 | 0.9213 | 0.9423 | 0.9702 | 0.9772 |
Encrypted image | 0.0122 | 0.0023 | 0.0206 | 0.0012 | 0.0056 | 0.0165 | 0.0025 | 0.0021 | 0.0024 |
Image | NPCR(%) | UACI(%) | ||||
R | G | B | R | G | B | |
Encrypted image | 99.6011 | 99.6066 | 99.6200 | 33.1101 | 33.2622 | 33.3230 |
Average | 99.6092 | 33.2384 |
Encryption algorithm | Our | Huang[43] | Lin[21] | Ye[12] | Zhang[24] | Zahra[45] |
Horizontal direction | 0.0012 | -0.0752 | 0.0315 | 0.0464 | 0.0713 | 0.0048 |
Vertical direction | 0.0122 | -0.0753 | 0.2651 | 0.0562 | -0.3255 | 0.0231 |
Diagonal direction | 0.0361 | 0.0466 | 0.0455 | 0.0397 | -0.0423 | 0.0280 |
Average | 0.0169 | 0.0661 | 0.2119 | 0.0478 | 0.1467 | 0.0190 |
Plain Image | Encrypted Image | |||||
R | G | B | R | G | B | |
H | 7.6215 | 7.9425 | 7.7426 | 7.8695 | 7.9526 | 7.9265 |
Position | A | B | C | D | E | F | G | H | I |
Hopf bifurcation | |||||||||
critical value | 0.2173 | 0.1850 | 0.3276 | 0.3819 | 0.8107 | 0.2765 | 6265 | 0.4396 | 0.2253 |
Image | Horizontal direction | Vertical direction | Diagonal direction | ||||||
R | G | B | R | G | B | R | G | B | |
Original image | 0.9002 | 0.9016 | 0.9675 | 0.9412 | 0.9522 | 0.9213 | 0.9423 | 0.9702 | 0.9772 |
Encrypted image | 0.0122 | 0.0023 | 0.0206 | 0.0012 | 0.0056 | 0.0165 | 0.0025 | 0.0021 | 0.0024 |
Image | NPCR(%) | UACI(%) | ||||
R | G | B | R | G | B | |
Encrypted image | 99.6011 | 99.6066 | 99.6200 | 33.1101 | 33.2622 | 33.3230 |
Average | 99.6092 | 33.2384 |
Encryption algorithm | Our | Huang[43] | Lin[21] | Ye[12] | Zhang[24] | Zahra[45] |
Horizontal direction | 0.0012 | -0.0752 | 0.0315 | 0.0464 | 0.0713 | 0.0048 |
Vertical direction | 0.0122 | -0.0753 | 0.2651 | 0.0562 | -0.3255 | 0.0231 |
Diagonal direction | 0.0361 | 0.0466 | 0.0455 | 0.0397 | -0.0423 | 0.0280 |
Average | 0.0169 | 0.0661 | 0.2119 | 0.0478 | 0.1467 | 0.0190 |
Plain Image | Encrypted Image | |||||
R | G | B | R | G | B | |
H | 7.6215 | 7.9425 | 7.7426 | 7.8695 | 7.9526 | 7.9265 |
ours | [48] | [47] | |
Average Speed (s) | 1.4126 | 2.0322 | 2.0716 |