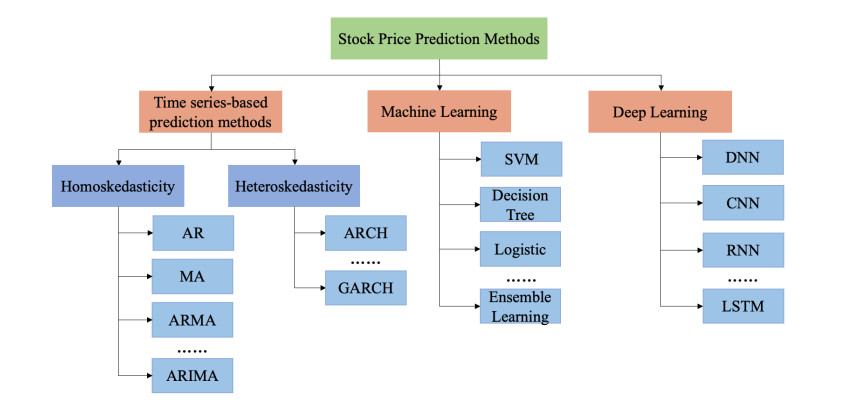
Text-based chatbots are implemented in the financial sector to enhance the relationship between the customer and services provided by the sector, and also to address external challenges and customer requirements. The chatbot technology in the financial sector serves to examine customers' frequently asked questions and the representation of the process using machine learning. In light of this, this study presents a comprehensive systematic literature review of articles focused on text-based chatbots in the financial sector. It describes the understanding of chatbots in the financial sector in terms of implementation, adoption intention, attitude toward use and acceptance; it also describes how people experience chatbots, specifically in terms of perception, expectation and trust, as well as how they are engaging and emotionally motivated; management of the security and privacy vulnerabilities of the chatbots; and identifies the potential strategies that can hinder the efficient, successful evolution of chatbots in the financial sector. Finally, the main findings regarding the use of text chatbots in the financial sector are presented; additionally, the open issues in current research are highlighted and a number of research opportunities that can be pursued in the future are suggested.
Citation: Hana Demma Wube, Sintayehu Zekarias Esubalew, Firesew Fayiso Weldesellasie, Taye Girma Debelee. Text-Based Chatbot in Financial Sector: A Systematic Literature Review[J]. Data Science in Finance and Economics, 2022, 2(3): 232-259. doi: 10.3934/DSFE.2022011
[1] | Fatima Tfaily, Mohamad M. Fouad . Multi-level stacking of LSTM recurrent models for predicting stock-market indices. Data Science in Finance and Economics, 2022, 2(2): 147-162. doi: 10.3934/DSFE.2022007 |
[2] | Angelica Mcwera, Jules Clement Mba . Predicting stock market direction in South African banking sector using ensemble machine learning techniques. Data Science in Finance and Economics, 2023, 3(4): 401-426. doi: 10.3934/DSFE.2023023 |
[3] | Zimei Huang, Zhenghui Li . What reflects investor sentiment? Empirical evidence from China. Data Science in Finance and Economics, 2021, 1(3): 235-252. doi: 10.3934/DSFE.2021013 |
[4] | Qian Shen, Yifan Zhang, Jiale Xiao, Xuhua Dong, Zifei Lin . Research of daily stock closing price prediction for new energy companies in China. Data Science in Finance and Economics, 2023, 3(1): 14-29. doi: 10.3934/DSFE.2023002 |
[5] | Jiawei He, Roman N. Makarov, Jake Tuero, Zilin Wang . Performance evaluation metric for statistical learning trading strategies. Data Science in Finance and Economics, 2024, 4(4): 570-600. doi: 10.3934/DSFE.2024024 |
[6] | Chen Chang, Hongwei Lin . A topological based feature extraction method for the stock market. Data Science in Finance and Economics, 2023, 3(3): 208-229. doi: 10.3934/DSFE.2023013 |
[7] | Tahir Afzal, Muhammad Asim Afridi, Muhammad Naveed Jan . Integrating LSTM with Fama-French six factor model for predicting portfolio returns: Evidence from Shenzhen stock market China. Data Science in Finance and Economics, 2025, 5(2): 177-204. doi: 10.3934/DSFE.2025009 |
[8] | Fangzhou Huang, Jiao Song, Nick J. Taylor . The impact of time-varying risk on stock returns: an experiment of cubic piecewise polynomial function model and the Fourier Flexible Form model. Data Science in Finance and Economics, 2021, 1(2): 141-164. doi: 10.3934/DSFE.2021008 |
[9] | Sangjae Lee, Joon Yeon Choeh . Exploring the influence of online word-of-mouth on hotel booking prices: insights from regression and ensemble-based machine learning methods. Data Science in Finance and Economics, 2024, 4(1): 65-82. doi: 10.3934/DSFE.2024003 |
[10] | Kazuo Sano . Intelligence and global bias in the stock market. Data Science in Finance and Economics, 2023, 3(2): 184-195. doi: 10.3934/DSFE.2023011 |
Text-based chatbots are implemented in the financial sector to enhance the relationship between the customer and services provided by the sector, and also to address external challenges and customer requirements. The chatbot technology in the financial sector serves to examine customers' frequently asked questions and the representation of the process using machine learning. In light of this, this study presents a comprehensive systematic literature review of articles focused on text-based chatbots in the financial sector. It describes the understanding of chatbots in the financial sector in terms of implementation, adoption intention, attitude toward use and acceptance; it also describes how people experience chatbots, specifically in terms of perception, expectation and trust, as well as how they are engaging and emotionally motivated; management of the security and privacy vulnerabilities of the chatbots; and identifies the potential strategies that can hinder the efficient, successful evolution of chatbots in the financial sector. Finally, the main findings regarding the use of text chatbots in the financial sector are presented; additionally, the open issues in current research are highlighted and a number of research opportunities that can be pursued in the future are suggested.
The stock market, regarded as the economy's barometer, has been a key research area of interest for academics and industry. On the one hand, the stock market creates a favorable financing environment for companies. On the other hand, investors could earn potential returns from the stock market by executing investment decisions such as capital allocation, stock selection and timing (Keller and Siegrist, 2006). Correspondingly, investors have to be exposed to the investment risks associated with the investment behavior. As a result, stock price prediction is significant for the investment decision of investors to balance their return and risk of investment. Stock prices are highly volatile, non-linear and stochastic due to external factors such as political events and corporate development (Nti et al., 2021), making it difficult to achieve accurate stock price prediction by relying on the previous stock price data. Thus, it is critical for stock price prediction to capture both the previous stock price and other factors (Zhang et al., 2022b).
In stock price prediction research, the raw data can be divided into quantitative and qualitative data, which are characterized by multiple sources of heterogeneity, uncertainty and large scale (Zhang and Zhang, 2022). Before using these data for analysis, certain methods need to be used to process, integrate and utilize them to form a data warehouse for subsequent prediction studies (Evans et al., 2018).
Existing studies have used single algorithms to mine the stock price-related information and make single predictions to make the investment decision (Ariyo et al., 2014; Brogaard and Zareei, 2022). However, the generalization ability of investment decisions derived from a single model is weak and unreliable (Zhang et al., 2018). Therefore, the introduction of multiple models with different structures or the same structure but different initializations for stock price prediction and the integration of multiple predictions as the basis for the final investment decision are significant for improving the interpretability and reliability of stock price prediction models (Gandhmal and Kumar, 2019). Given the aforementioned difficulties, the data fusion (DF) method has been applied to stock price prediction (Thakkar and Chaudhari, 2021). Data fusion refers to the process of integrating the data, features and knowledge with multiple sources and/or different structures and modalities to obtain fused data, fused features and fused knowledge for the further analysis (Li et al., 2022a, 2022b). Previous studies have shown that fusing heterogeneous data from multiple sources and using multiple models for stock price prediction are more accurate and have better generalization performance compared to using single-source data and a single model (Thakkar and Chaudhari, 2021).
Although data fusion has been emphasized in stock price prediction, previous studies only focused on one aspect of the stock price prediction using data fusion. Furthermore, there is a lack of a complete and systematic summary of studies on the application of data fusion in stock price prediction. Thus, this study reviews the relevant research on data fusion in stock price prediction from three main levels of data fusion (data-level, feature-level and decision-level fusion), presenting a complete understanding of the research problem (Guo et al., 2014; Lee et al., 2023; Zhang et al., 2022). It is worth noting that only all types of stock price prediction problems are addressed, and no distinction is made between timeframes.
The article unfolds as follows. Section 2 briefly introduces the stock price prediction methods applied in the existing studies. Section 3 provides a statistical overview of the literature on existing studies. Sections 4, 5 and 6 provide an overview on stock price prediction using data fusion at the data, feature and decision levels, respectively. Finally, this study concludes and highlights the directions for future studies.
In recent years, extensive traditional time series-based prediction methods have been widely used in stock market prediction (Figure 1). The autoregressive (AR), moving average (MA) and autoregressive moving average (ARMA) models are basic models for stock price time-series prediction. It should be noted that the stock prices are supposed to be pre-processed and reach a stationary state (Shi et al., 2012). The autoregressive integrated moving average (ARIMA) model, developed based on the ARMA model, is one of the most representative statistical models for time-series data analysis and can be used to fit models to non-stationary stock price time series data (Ariyo et al., 2014). However, the above four models are only suitable for predicting stationary time-series data under certain conditions, and their variances are supposed to be constant, while the stock market is non-stationary. Autoregressive conditional heteroskedasticity (ARCH) and generalized autoregressive conditional heteroskedasticity (GARCH) could address the problems caused by the traditional econometric second assumption (constant variance) for time-series variables and are more widely used in stock price prediction (Jeantheau, 2004; Liu et al., 2021b). Traditional stock price prediction methods mainly use the linear regression to predict stock trends based on stationary linear historical data. However, stock prices are non-stationary, influenced not only by historical stock trading data but also by non-linear factors such as political factors, investment sentiment and specific events.
Machine learning algorithms with excellent non-linear regression performance are becoming popular methods for stock market prediction, including logistic regression, support vector machine (SVM), decision tree and ensemble learning (Brogaard and Zareei, 2022; Cheng et al., 2021; Xiao et al., 2019; Yang et al., 2022). Recently, machine learning algorithms have also been gradually applied to quantitative stock trading, and research results have shown that machine learning-based quantitative stock trading strategies are better than traditional simple trading strategies (Wang and Yan, 2023; Yan et al., 2023). In addition, deep learning algorithms, such as deep neural network (DNN), convolution neural network (CNN) and recurrent neural network (RNN) can extract potential features of highly unstructured data and explore complex intrinsic patterns of stock price movements based on time series data, and they have been used to predict stock market trends (Hu et al., 2021; Liu et al., 2021a; Lu and Lu, 2021). Among all RNN-based models, long short-term memory (LSTM) could be the most effective model for time series prediction. LSTM uses a set of memory cells with gate structure to replace hidden neurons of RNN. As such, through the gate structure feature, the information is retained and persistently updated in the following training iterations. So, it has the advantage of solving the gradient explosion and gradient disappearance problems in neural network algorithms. Several articles have shown that it outperforms RNNs and traditional machine learning algorithms in stock predictions based on time series data (Liu et al., 2021; Brogaard and Zareei, 2022).
Although machine learning and deep learning have the advantage of non-linear prediction performance in stock price prediction, most of the studies only utilize a single historical stock data and a single prediction model to realize stock price prediction, which has limitations in terms of interpretability and generalization performance (Zhang et al., 2018). Extending stock-related data sources, fusing multiple sources of heterogeneous stock data and fusing multi-algorithm prediction results thus become the key to improving prediction performance.
In subsequent studies, external factors such as macroeconomic indicators, financial network news and specific events have been gradually combined with stock price data and incorporated into stock price prediction models (Lee et al., 2023). Additionally, among the factors affecting stock prices, market and investor sentiment offers important information. Some studies have emphasized the explanation of sentiment information of stock prices, and the results showed a positive correlation between sentiment information and stock market trading volume (Long et al., 2020; Shields et al., 2021; Zhang et al., 2017a). With the development of graph mining techniques, graph data such as candlestick charts have also been considered as one of the information sources for stock price prediction models (Kim and Kim, 2019; Liu et al., 2022; Wang et al., 2019).
The stock market has a huge variety of data sources, including the internet, databases, emails, social networking sites, news media, etc. These sources generate vast amounts of stock-related data daily, typically in terabytes or gigabytes (Nti et al., 2021). However, the ubiquity of such data and the impact of factors such as public sentiment and economic indicators on stock prices make the integration and utilization of data from multiple sources challenging in stock price prediction. Within this context, data fusion is emerging as a critical area of research in stock price prediction. The primary objective is to combine data from various sources into a new dataset or feature set that provides comprehensive knowledge of the factors influencing stock price movement. The resulting dataset is then used as input for prediction models to generate more accurate predictions and facilitate better investment decisions. Previous research has shown that the application of data fusion methods in predicting stock prices results in models with higher accuracy and better generalization performance (Stoean et al., 2019; Thakkar and Chaudhari, 2021; Zhou et al., 2022). Thus, data fusion has become a crucial subject of exploration for researchers, with the potential to provide investors with more reliable insights to inform their investment decisions.
To provide a clear overview of data fusion development in stock price prediction, we extensively searched the SCI literature database within the Web of Science platform. We used advanced search parameters, including the first-level subject term "Data Fusion" and the second-level subject term "Stock". The search was limited to articles published between 2003 and 2023 and was subjected to the "Web of Science Core Collection" inclusion criteria. Our search returned a total of 379 records.
Using the search results, we created Figure 2 to display the trend in the number of articles published over time. As observed from the graph, the number of relevant studies generally increased over time and significantly spiked between 2015 and 2022.
Research interest in applying data fusion to stock price prediction varies globally. Figure 3 displays the distribution of relevant literature by country/region using the results of our literature search, indicating that China and the USA have the most relevant studies.
We further counted the research directions of the screened literature to construct the top ten research directions based on the number of publications, as displayed in Figure 4. As observed in the figure, most of the relevant literature is published in the fields of computer science, mathematics, engineering and business economics.
The above literature statistical visualizations demonstrate that the number of studies on stock price prediction through data fusion is increasing, indicating potential future research implications in this field.
Additionally, analysis of the existing literature reveals that research on stock price prediction with data fusion is primarily conducted at three levels: data-level, feature-level and decision-level fusion.
Figure 5 illustrates that stock-related data can be categorized into two main types: qualitative and quantitative data. Quantitative data mainly comprises numerical information such as historical stock data, corporate financial data, macroeconomic data and other stock-related index data. Traditional stock price prediction models primarily utilize historical stock data to predict trends, as described in Section 2 of this paper. However, the efficient market hypothesis (EMH) and the stochastic wandering hypothesis (SWH) suggest that using historical stock data alone may not predict future stock price trends effectively (Malkiel and Fama, 1970; Malkiel, 2015). Recent studies, such as Stoean et al. (2019), used LSTM-based prediction models to predict the closing price of 25 stocks in the Bucharest Stock Exchange using only historical stock prices and achieved significant results. They also suggested that combining multi-indicator data can further improve the predictive power of their models.
Apart from quantitative data, qualitative factors that impact stock price are also gaining attention in recent research, as indicated in (García-Medina et al., 2018). One such factor is event-specific information from online media or Web news which has high correlations with stock price, with studies showing that various events, including financial network news and firm-specific announcements, can impact stock price trends (Shi et al., 2022; Thakkar and Chaudhari, 2021). However, information on stock price-related events collected from the Web is very sparse. Although Web news is incrementally available, events are usually presented as unstructured text, so the number of events that can be extracted from Web news remains limited. In addition, the same event may be described differently on different websites in different ways and thus be treated as different events, leading to event sparsity. So, using event information solely for stock price prediction is not enough (Zhang et al., 2017). Additionally, from behavioral finance theory, emotions also play a significant role in decision making, and investors' own investment emotions may influence their investment decisions (Zhou et al., 2018). For example, the collective level of optimism or pessimism in society can affect investor decisions (Nofsinger, 2005). Due to the recent advances in natural language processing (NLP) techniques, sentiment-driven stock prediction techniques have also been proposed by extracting indicators of public mood from social media, where positive mood for a stock will probably indicate a rising trend in the price, and negative mood will more likely mean a decreasing trend (Bollen et al., 2011). Therefore, sentiment information from social media, such as investor sentiment information in financial forum discussions and tweets, can also be used as a complement to quantitative data.
Several studies have added sentiment information to their prediction models, with improved predictive power as a result (Li et al., 2020). For example, Chiong et al. (2018) developed a sentiment analysis method based on financial news disclosure, extracting sentiment-related features as input for the stock price prediction model. Compared with the prediction models without the inclusion of sentiment-related features, their proposed SVM and particle swarm optimization (PSO)-based model with sentiment feature extraction performed well in terms of accuracy and time. Their results showed a positive correlation between public sentiment and future stock prices. However, relying on the sentiments alone is not sufficient for prediction either. For example, during holidays, people's mood tends to be positive, yet it may not really reflect their investment opinions.
To tackle the above problems, data-level fusion and feature-level fusion are being introduced in stock price prediction research to achieve integration of data from different sources and provide more informative inputs for the subsequent prediction model training.
Data-level fusion and feature-level fusion can solve the challenge of fusing multi-source stock data, but they differ in their approaches. Multi-source stock data includes two types of data: multi-source homogeneous data and multi-source heterogeneous data. Data-level fusion can be applied to both types of data, while feature-level fusion is more suitable for heterogeneous data.
In data-level fusion, raw stock-related data from various sources are merged before being further processed. Homogeneous stock data fusion involves the straightforward merger of stock data with the same structure, such as the direct integration of different dimensions of stock trading data provided by Bloomberg and Wind (Zhang et al., 2017b). However, the small number of homogeneous stock data categories and the simple fusion process limit the interpretability of the final fused data on stock prices. Therefore, some scholars performed data-level fusion on heterogeneous data. For instance, Nti et al. (2021) proposed a novel multisource information-fusion stock price movement prediction framework based on a hybrid deep neural network architecture (CNN and LSTM) named IKN-ConvLSTM and fused stock-related information from six heterogeneous data sources using data preprocessing and record matching approaches. The empirical evaluation of their model was carried out with stock data (January 3, 2017, to January 31, 2020) from the Ghana Stock Exchange (GSE), and the results showed good prediction accuracy (98.31%), specificity (0.9975), sensitivity (0.8939%) and F-score (0.9672) of the amalgamated dataset compared with the distinct dataset. Considering the limitation of using only stock price data to predict stock prices, Lee et al. (2023) introduced two types of modal data, macroeconomic indicators and month/week, into a stock direction classification model based on historical stock price data and achieved data-level fusion by modally linking the three types of data. The test results showed better performance for the fusion model compared with the comparison models and achieved statistically significant results. Specifically, 27 out of 50 stocks achieved higher classification accuracy than the comparative model. However, the manual merging required to integrate heterogeneous data can be time-consuming and labor-intensive.
Reviewing the data-level fusion for multi-source stock data, it can be found that data fusion in this level has certain shortcomings in terms of efficiency, effectiveness and stock price interpretability. More research is therefore distributed in the areas of feature-level fusion and decision-level fusion.
While the raw data contains rich information, it also has a significant amount of noise. Direct data-level fusion may not yield effective information. Therefore, feature extraction, feature selection and feature fusion of the raw data are required before training prediction models with the data. In feature-level fusion, the input data can be heterogeneous, and it is usual to extract statistical features or signals from the heterogeneous raw data and select or connect these features before further analysis.
Regarding heterogeneous stock-related data, historical stock data provides fundamental stock trading information, and technical indicators derived from it can serve as features for learning specific interpretations. Specific event attributes or market behavior based on a particular context can also be considered for potential features since stock markets are affected by numerous specific events. Moreover, investor or market sentiment can significantly impact stock market volatility, making sentiment features obtained using sentiment analysis another useful input for stock price prediction models. While individual features provide valuable information about the stock market, combining different aspects of these features through feature fusion is crucial to extract the intrinsic characteristics of the stock market. Feature fusion is performed in a manner that can be viewed as feature-level abstraction or object refinement of the processed data (Chiong et al., 2018). The fused features can be applied to all levels of models for subsequent stock market analysis.
Through analysis, it has been discovered that obtaining information related to stock price fluctuations and considering the influencing features related to stock price comprehensively are crucial to improve the accuracy of prediction models. Generally speaking, there are four types of features that may affect stock prices: quantitative features (stock price features, macroeconomic features, financial status features), sentiment features (financial forum features, tweet features, social media features), specific event features (network news features, corporate announcement features) and chart features (candlestick chart features). Different feature extraction and fusion methods are required for different features.
Regarding fusion paths, there are two types of paths: serial fusion and parallel fusion. The specific paths for feature-level fusion are shown in Figure 6. The former path has only one fusion step, which is "raw data - data merging - feature extraction - feature fusion - model training". The latter path has multiple parallel processing steps, which are "raw data - feature extraction - feature fusion - model training".
In the serial fusion path, feature selection and fusion are based on the merged dataset. Nti et al. (2021) combined six heterogeneous data sources containing quantitative features, sentiment features and specific event features into a data warehouse using record matching, followed by training a CNN and stacked LSTM model on the resulting data warehouse to achieve feature selection and fusion. Lee et al. (2023) used modal linking to merge stock price data, macroeconomic indicators and date data in quantitative features before feature-level fusion to generate merged data and use them as input to the multi-headed attention layer for feature fusion. Due to differences in dimensionality and structure among the raw stock-related data, direct merging of raw data is time and operationally expensive. Moreover, serial fusion is prone to sparsity issues, so its application in stock price prediction problems has been limited.
Unlike serial feature fusion, the parallel fusion path employs various models to perform feature extraction and fusion for data with different categories of features, solving the dimensional inconsistency issue that results from fusing features with variable dimensions. The most fundamental feature in stock price prediction is the quantitative feature represented by historical stock data, containing rich stock-related information. Considering the non-stationary, stochastic and non-linear characteristics of stock price time-series data, some scholars utilize a combination of multiscale convolutional feature fusion (MCFF) and LSTM to achieve the fusion of different representation features. They employ the wavelet transform and normalization to denoise the financial time series data and achieve the fusion of eight features, including close, open, volume, etc. In this way, the stock price history data are represented as the form of an eight-dimensional time series and further used as the input for an LSTM-based model. This method successfully extracts and merges diverse features from stock price time-series data, thus improving the generalization performance of the prediction model (Zhang and Zhang, 2022). Furthermore, independent component analysis (ICA) can be adopted to extract stock price features and technical indicator features from historical prices, fusing both features using canonical correlation analysis (CCA) based feature fusion methods (Guo et al., 2014).
However, using only stock price features or technical indicator features for stock price prediction has its limitations; therefore, incorporating stock price related features such as event-specific features, sentiment features and graph features should be considered to establish a multi-class feature fusion model. Zhang et al. (2017b) explored the joint effect of event-specific features and investor sentiment on stock price prediction in their study. They accomplished the fusion of quantitative stock features, sentiment features and event-specific features through the framework of coupled matrix and tensor factorization and introduced a stock correlation matrix to tackle the data sparsity issue. The analytical frameworks of Sun et al. (2021) and Daradkeh (2022) were based on CNN and bidirectional long short term memory (Bi-LSTM) networks and using two algorithms to extract event features from text data and emotional polarity features based on backward and forward contextual information, respectively. They then implemented feature fusion through a fully connected network. Differently, Daradkeh further incorporated another quantitative feature, i.e., macroeconomic features, into their stock trend prediction framework. Both studies suggested that combining quantitative stock data with non-quantitative stock-related information from event-specific features, sentiment polarity, etc. can enhance stock price prediction performance. Moreover, the candlestick chart, strongly associated with stock price features, is considered to be the optimal chart feature for stock price prediction, and thus it was also incorporated in the studies of Kim and Kim (2019) and Liu et al. (2022). In the feature extraction and fusion framework of the candlestick chart, the most prominent algorithm used is CNN+Bi-LSTM, which enables extraction of graph features and transforms them into time-series features for further fusion with stock price features and technical features as inputs to the final prediction model.
Although the aforementioned studies used feature-level fusion to unveil more intrinsic information about stocks and achieved robust prediction results, most of them used only a single prediction model such as SVM, LSTM or reinforcement learning (RL) to obtain a single prediction result as an investment basis for decision making (Daradkeh, 2022; Guo et al., 2014; Liu et al., 2022). However, the prediction results of a single prediction model are vulnerable to interference from different factors, resulting in unstable investment decisions. Consequently, it is indispensable to enhance the reliability of the prediction outcomes by considering the prediction results from multiple prediction models at the final decision level.
Generally, if the prediction models have different structures or the same structure but with random initialization, they may generate distinct stock price prediction outcomes. Additionally, different prediction models vary in their capabilities to learn different types of data or features, which in turn affects the final prediction outcomes. In this case, better prediction can often be obtained by considering the results of multiple prediction models rather than relying on a single prediction outcome from a single model (Sun et al., 2021). The learning result of a model can be deemed the probability of belonging to a specific category under the influence of a certain feature, and the fusion of outcomes can improve the correlation between features to a certain extent (Lai et al., 2021). This strategy of aggregating "group intelligence" is also known as ensemble learning in the data mining field, which aims to balance the limitations of a single model by the strengths of each base learner. The ensemble learning embodies the idea of decision-level fusion, which means that the decision output from multiple base learners is combined into a single prediction result about the stock price to obtain a more stable investment decision than a single model. Hence, this combination of multiple predictions provided by multiple learners is also known as decision-level fusion (Ho et al., 1994; Tulyakov et al., 2008).
In terms of structural similarities and differences between the base learners, decision-level fusion can be classified into decision-level fusion of homogeneous base learners and decision-level fusion of heterogeneous base learners. The variance lies in the application of prediction models with distinct parameters of the same algorithm or different algorithms.
Prediction studies based on homogeneous algorithms utilize the same algorithm for training and predicting stock price related data. Among these algorithms that are listed in Table 1 in terms of popularity, the artificial neural network (ANN) is the most frequently used base learner for stock price prediction, followed by LSTM, decision tree and SVM. Precisely, ANN enables us to design useful nonlinear systems accepting large numbers of inputs, with the design based solely on instances of input-output relationships. However, ANNs are based on the empirical risk minimization principle, which may run the risk of model over-fitting and local minimums (Giacomel et al., 2015; Lahmiri, 2018). The LSTM model is one kind of recurrent neural network which can theoretically store an infinite amount of time information and avoids the negative influence of vanishing gradient and exploding gradient through the control of input gate, output gate and forget gate (Xie et al., 2018; Yang et al., 2020). Additionally, probabilistic neural network (PNN), extreme learning machine (ELM) and deep belief network (DBN) have also been applied as base learners in a few studies. In contrast, heterogeneous algorithms for stock price prediction combine several different algorithms for model training and prediction, ensuring the diversity of model prediction.
Base Learner | Source | Algorithm |
Homogeneous | Giacomel et al., 2015; Lahmiri, 2018; Lahmiri and Boukadoum, 2015; Nezhad and Bidgoli, 2019 |
ANN |
Xie et al., 2018; Yang et al., 2020 | LSTM | |
Zhou et al., 2022 | Decision Tree | |
Qiu et al., 2017 | SVM | |
Chandrasekara et al., 2019 | PNN | |
Khuwaja et al., 2020 | ELM | |
Wang et al., 2018 | DBN | |
Zhong et al., 2017 | Random Forest | |
Lin et al., 2021 | KNN | |
Melin et al., 2012 | ANFIS1 |
1 Adaptive Neuro Fuzzy Inference System
Table 2 summarizes the stock price prediction literature based on heterogeneous algorithms. Most of these studies use integration between different machine algorithms. For instance, Abraham and Auyeung (2009) investigated how the seemingly chaotic behavior of stock markets could be well-represented using an ensemble of intelligent paradigms which includes ANN, SVM, neuro-fuzzy system (NFS) and a difference boosting neural network (DBNN). Experimental results reveal that the ensemble techniques performed better than the individual methods. Similarly, some other scholars have used different combinations of algorithms for stock price prediction studies, such as Back Propagation Neural Network (BPNN)-RNN-SVR, CNN-LSTM and ANN-DecisionTree-KNN. Non-machine learning algorithms such as user knowledge, expert knowledge, speech and text encoder, and Delphi method is also used as part of ensemble algorithms for stock price prediction.
Base Learner | Source | Algorithms |
Heterogeneous | Abraham and Auyeung, 2009 | ANN, DBNN, NFS, SVM |
Alhnaity and Abbod, 2020 | BPNN, RNN, SVR | |
Chong et al., 2020 | CNN, CNN-LSTM, LSTM | |
Qian and Rasheed, 2007 | ANN, Decision Tree, KNN | |
Barak et al., 2017 | ANN, Decision Tree, Rule-Based Algorithm, SVM | |
Dash et al., 2019 | MLP2, Random Forest, Naïve Bayes, SVM | |
Lee and Kim, 1995 | ID3, Expert Knowledge, User Knowledge | |
Sawhney et al., 2020 | MTL3, Speech and Text Encoder, SVM | |
Kuo et al., 1996 | ANN, Delphi Method | |
Kristjanpoller and Michell, 2018 | ANFIS, GARCH |
2 Multi-Layer Perceptron
3 Multi-Task Learning
After summarizing the characteristics of the base learners in the study, we analyze the model structure of the existing literature with reference to Zhang's (2022a) study and can conclude that the decision fusion model design can be divided into three categories, which are traditional decision-level fusion, auxiliary model-based decision-level fusion and two-stage decision-level fusion.
Traditional decision-level fusion involves multiple base learners (e.g., Figure 7), which fuse the prediction results of each base learner to provide a combined prediction of the corresponding analysis variables. Stock price prediction using stock time-series data is one of these cases. It decomposes the stock time-series features into multiple parts according to the slide window and trains the time-series features of each part independently to provide partial predictions of the corresponding analysis variables. The partial predictions are then fused. Carta et al. (2021) proposed a multi-level and multi-integrated stock trading model based on deep learning and deep reinforcement learning to address the low performance of a single supervised classifier in predicting future market behavior. Their base learners were selected based on a deep double-Q network (DQN) that used raw stock price data to generate multiple stock trading signals through different iterations. Finally, given such outputs from the previous meta-learners, they proposed that the final agent works in an ensemble fashion, which considers majority voting of decisions to generate the final trading signal with respect to long, idle or short positions. Similarly, Liu et al. (2021a) segmented the stock time-series data into datasets based on a 20-day time interval. They proposed three-branch structure based on CNN and LSTM with different parameter settings to complete feature fusion and stock price prediction for each segmented dataset, respectively. The prediction results of the three-branch model were then fused in the LSTM's cell model as input to the dense layer used for denormalization to achieve the final decision fusion. Precisely, the final output of their designed model is the predicted value of the (t+1)-th day's closing stock price using the previous t days' feature data.
In contrast, the idea of Shi et al. (2022) used two different algorithms, multiple linear regression (MLR) and LightBoost, to train the stock price dataset separately. They then introduced the LSTM as the fusion algorithm to fuse the prediction results and formed the final prediction. Similar studies have also used a combination of logistic regression, random forest and XGBoost as the base learners for the first level of the basic prediction model, followed by a new XGBoost as the second level of the prediction model to achieve decision-level fusion (Zhang and Lu, 2021).
Auxiliary model-based decision-level fusion consists of two parallel prediction paths (e.g., Figure 8), the original prediction model based on stock data and the prediction model based on the effects of external factors. The latter usually predicts the effects of external factors using machine learning algorithms or non-machine learning algorithms, and the former fine-tunes the prediction results by combining the latter in the decision-level fusion to form the final fusion decision (Kuo et al., 1996; Kristjanpoller and Michell, 2018). Lai et al. (2021) considered the effect of news features on stock prices in their stock price prediction model. They built two models, the original stock price prediction model and the news feature prediction model, using two algorithms, Bi-GRU and TextCNN, to learn news text data and time-series data, respectively. The prediction results of the two models were then fused into a final single prediction by assigning different weights to different inputs well, so as to tell the discriminant network which inputs are more important using the weighted sum method and attention mechanism.
In the two-stage decision-level fusion, the prediction in the second stage is based on the fusion prediction result of the first stage as input (e.g., Figure 9). To improve on the traditional method of using the technical indicators on day t as the input to the model to predict the closing price of stocks on day t+n, Zhang et al. (2021) proposed a two-stage ensemble learning algorithm based on a support vector regression – ensemble adaptive neuro fuzzy inference system (SVR-ENANFIS). The SVR was used in the first stage of the model to predict the day t+n values of several technical indicators such as moving average (MA) and exponential moving average (EMA), which were used as inputs for the second stage of the model. In the second stage, the ENANFIS ensemble algorithm, which contains 10 basic ANFIS models, was used to train and obtain predictions for the input data obtained in the previous stage. Finally, a weighted average method was used to fuse the 10 outputs to obtain the stock closing price prediction for day t+n. Comparison results indicated that this two-stage model performed better than the single-stage ENANFIS, two-stage SVR-linear and two-stage SVR-SVR models.
As seen from the summary of the three types of decision fusion model design, traditional decision fusion is a more applied decision fusion framework with certain advantages in terms of comprehensibility and operability. However, this framework only considers a single stock data source and overlooks external factors such as sentiment information and news events that may impact stock prices. Most prediction models use the relevant features on day t to predict the stock price on day t+n. Hence, there is research value and significance in exploring auxiliary model-based decision-level fusion and two-stage decision-level fusion as alternative approaches.
Again, from the point of view of the techniques fused at the decision level, the main methods applied in stock price prediction research are Voting, Weighted sum, Averaging, Stacking, LSTM, and XGBoost, with the Majority voting being the most applied Voting method, and the Weighted averaging being the one that is applied more in Averaging method. In addition, tree-based algorithms, fuzzy algorithms, sorting and selection methods are also applied (Zhang et al., 2021a).
This paper presented a systematic review of research on stock price prediction from a data fusion perspective, summarizing three important data fusion levels in the field of stock price prediction: stock price prediction based on data-level fusion, stock price prediction based on feature-level fusion and stock price prediction based on decision-level fusion.
The results highlight that data fusion has been widely used and has achieved some success in the field of stock price prediction. Meanwhile, the application of data fusion in the field of stock price prediction provides some insights. The types of stock-related data available in the study vary, including mainly historical stock data, macroeconomic data, specific events, user sentiment information, chart information, etc. To improve the accuracy of prediction models, future studies could focus on broadening the scope of data collection and obtaining stock-related information as much as possible. For instance, as a new type of additional event information, the domain knowledge graph contains some stock-related knowledge. Introducing knowledge graph information can well enrich event descriptions and reduce sparsity, thus making better use of the event information and improving the accuracy of stock price prediction. In addition, considering the specificity of text information for the collection and processing of text information, natural language processing (NLP) techniques such as generative adversarial network (GAN) and autoencoder can be used to accomplish text classification, text information extraction and other tasks to improve the ability of acquiring stock-related text information.
This paper has certain content limitations that can be addressed in future work. One limitation is that the summary of the paper is not extensive enough and lacks necessary detail. For example, the stock price prediction problem can be further categorized into short-term prediction and long-term classification. Future studies can broaden the scope and quantity of literature collection to gain a better understanding of research progress in this field over various periods. Another limitation is that the issues addressed in this paper only cover a small portion of the finance field, and the stock price prediction field has relatively mature research. Future studies can focus on cutting-edge research topics in the current financial market, such as anomaly detection, quantitative trading and other emerging research domains.
The authors acknowledge the financial support of the National Natural Science Foundation (71932008, 71401188), the Engineering Research Center of National Financial Security of the Ministry of Education and CUFE Postgraduate students support program for the integration of research and teaching (202320).
The authors declare they have not used artificial intelligence (AI) tools in the creation of this article.
The authors declare that there is no conflict of interest.
[1] |
Abdulquadri A, Mogaji E, Kieu TA, et al. (2021) Digital transformation in financial services provision: a Nigerian perspective to the adoption of chatbot. J Enterp Communities 15: 258-281. https://doi.org/10.1108/JEC-06-2020-0126 doi: 10.1108/JEC-06-2020-0126
![]() |
[2] |
Adam M, Wessel M, Benlian A (2021) AI-based chatbots in customer service and their effects on user compliance. Electron Mark 31: 427-445. https://doi.org/10.1007/s12525-020-00414-7 doi: 10.1007/s12525-020-00414-7
![]() |
[3] |
Ahmad NA, Che MH, Zainal A, et al. (2018) Review of chatbots design techniques. Int J Comput Appl 181: 7-10. https://doi.org/10.5120/ijca2018917606 doi: 10.5120/ijca2018917606
![]() |
[4] |
Al Nasseri A, Tucker A, de Cesare S (2015) Quantifying Stock Twits semantic terms' trading behavior in financial markets: An effective application of decision tree algorithms. Expert Syst Appl 42: 9192-9210. https://doi.org/10.1016/j.eswa.2015.08.008 doi: 10.1016/j.eswa.2015.08.008
![]() |
[5] |
Allal-Chérif O, Simón-Moya V, Ballester ACC (2021) Intelligent purchasing: How artificial intelligence can redefine the purchasing function. J Bus Res 124: 69-76, https://doi.org/10.1016/j.jbusres.2020.11.050 doi: 10.1016/j.jbusres.2020.11.050
![]() |
[6] |
Alt MA, Vizeli I, Săplăcan Z (2021) Banking with a Chatbot - A Study on Technology Acceptance. Stud U Babes-Bol Oecon 66: 13-35. https://doi.org/10.2478/subboec-2021-0002 doi: 10.2478/subboec-2021-0002
![]() |
[7] |
Araujo T (2018) Living up to the chatbot hype: The influence of anthropomorphic design cues and communicative agency framing on conversational agent and company perceptions. Comput Human Behav 85: 183-189. https://doi.org/10.1016/j.chb.2018.03.051 doi: 10.1016/j.chb.2018.03.051
![]() |
[8] | Attfield S, Kazai G, Lalmas M, et al. (2011) Towards a science of user engagement. Expert WSDM Workshop on User Modelling for Web Applications: 9-12. |
[9] |
Aznoli F, Navimipour NJ (2017) Cloud services recommendation: Reviewing the recent advances and suggesting the future research directions. J Netw Comput Appl 77: 73-86. https://doi.org/10.1016/j.jnca.2016.10.009 doi: 10.1016/j.jnca.2016.10.009
![]() |
[10] |
Bajdor P (2021) Simulations of the relationship between the experience level of e-commerce customers and the adopted variables - Implications for management in the area of online shopping. Procedia Comput Sci 192: 2576-2585. https://doi.org/10.1016/j.procs.2021.09.027 doi: 10.1016/j.procs.2021.09.027
![]() |
[11] | Banu S, Patil SD (2020) An Intelligent Web App Chatbot. International Conference on Smart Technologies in Computing, Electrical and Electronics 309-315. https://doi.org/10.1109/icstcee49637.2020.9276948 |
[12] | Bavaresco R, Silveira D, Reis E, et al. (2020) Conversational agents in business: A systematic literature review and future research directions. Comput Sci Rev 36. https://doi.org/10.1016/j.cosrev.2020.100239 |
[13] | Bhuiyan MSI, Razzak A, Ferdous MS, et al. (2020) BONIK: A Blockchain Empowered Chatbot for Financial Transactions. IEEE 19th International Conference on Trust, Security and Privacy in Computing and Communications 1079-1088. https://doi.org/10.1109/trustcom50675.2020.00143 |
[14] |
Boyle EA, Connolly TM, Hainey T, et al. (2012) Engagement in digital entertainment games: A systematic review. Comput Hum Behav 28: 771-780. https://doi.org/10.1016/j.chb.2011.11.020 doi: 10.1016/j.chb.2011.11.020
![]() |
[15] | Brachten F, Kissmer T, Stieglitz S (2021) The acceptance of chatbots in an enterprise context - A survey study. Int J Inf Manage 60. https://doi.org/10.1016/j.ijinfomgt.2021.102375 |
[16] | Brave S, Nass C (2007) Emotion in human-computer interaction. Human-computer interaction handbook 103-118, CRC Press, 103-118. |
[17] | Brüggemeier B, Lalone P (2022) Perceptions and reactions to conversational privacy initiated by a conversational user interface. Comput Speech Lang 71. https://doi.org/10.1016/j.csl.2021.101269 |
[18] |
Cheng Y, Jiang H (2021) Customer-brand relationship in the era of artificial intelligence: understanding the role of chatbot marketing efforts. J Prod Brand Manage 31: 252-264 https://doi.org/10.1108/JPBM-05-2020-2907 doi: 10.1108/JPBM-05-2020-2907
![]() |
[19] |
Chung M, Ko E, Joung H, et al. (2020) Chatbot e-service and customer satisfaction regarding luxury brands. J Bus Res 117: 587-595. https://doi.org/10.1016/j.jbusres.2018.10.004 doi: 10.1016/j.jbusres.2018.10.004
![]() |
[20] | Ciechanowski L, Przegalinska A, Wegner K (2017) The necessity of new paradigms in measuring human-chatbot interaction. International Conference on Applied Human Factors and Ergonomics 205-214. https://doi.org/10.1007/978-3-319-60747-4_19 |
[21] | Debeauvais T (2016) Challenge and retention in games. University of California, Irvine. |
[22] | Distler V, Lallemand C, Bellet T (2018) Acceptability and acceptance of autonomous mobility on demand: The impact of an immersive experience. Proceedings of the 2018 CHI Conference on Human Factors in Computing Systems 1-10. https://doi.org/10.1145/3173574.3174186 |
[23] |
Doherty K, Doherty G (2018) Engagement in HCI: conception, theory and measurement. ACM Comput Surv 51: 1-39. https://doi.org/10.1145/3234149 doi: 10.1145/3234149
![]() |
[24] |
Eren BA (2021) Determinants of customer satisfaction in chatbot use: evidence from a banking application in Turkey. Int J Bank Mark: 39: 294-311. https://doi.org/10.1108/IJBM-02-2020-0056 doi: 10.1108/IJBM-02-2020-0056
![]() |
[25] | Fessl A, Rivera-Pelayo V, Müller L, et al. (2011) Motivation and user acceptance of using physiological data to support individual reflection. 2nd International Workshop on Motivation and Affective Aspects in Technology Enhanced Learning. |
[26] |
Følstad A, Taylor C (2021) Investigating the user experience of customer service chatbot interaction: a framework for qualitative analysis of chatbot dialogues. Qual User Exper 6: 1-17. https://doi.org/10.1007/s41233-021-00046-5 doi: 10.1007/s41233-021-00046-5
![]() |
[27] | Frison AK, Wintersberger P, Riener A, et al. (2019) In UX we trust investigation of aesthetics and usability of driver-vehicle interfaces and their impact on the perception of automated driving. Conference on Human Factors in Computing Systems-Proceedings, https://doi.org/10.1145/3290605.3300374 |
[28] |
Go E, Sundar SS (2019) Humanizing chatbots: The effects of visual, identity and conversational cues on humanness perceptions. Comput Hum Behav 97: 304-316. https://doi.org/10.1016/j.chb.2019.01.020 doi: 10.1016/j.chb.2019.01.020
![]() |
[29] | Goethe O, Niksirat KS, Hirskyj-Douglas I, et al. (2019) From UX to engagement: connecting theory and practice, addressing ethics and diversity. Expert Syst Appl 91-99. https://doi.org/10.1007/978-3-030-23560-4_7 |
[30] | Gondaliya K, Butakov S, Zavarsky P (2020) SLA as a mechanism to manage risks related to chatbot services. IEEE Intl Conference on High Performance and Smart Computing, HPSC and 2020 IEEE Intl Conference on Intelligent Data and Security IDS 2020. 235-240. https://doi.org/10.1109/BigDataSecurity-HPSC-IDS49724.2020.00050 |
[31] | Hentzen JK, Hoffmann A, Dolan R, et al. (2021) Artificial intelligence in customer-facing financial services: aystematic literature review and agenda for future research. Int J Bank Mark https://doi.org/10.1108/IJBM-09-2021-0417 |
[32] |
Hildebrand C, Bergner A (2021) Conversational robo advisors as surrogates of trust: onboarding experience, firm perception, and consumer financial decision making. J Acad Mark Sci 49: 659-676. https://doi.org/10.1007/s11747-020-00753-z doi: 10.1007/s11747-020-00753-z
![]() |
[33] | Hsu PF, Nguyen TK, Huang JY (2021) Value co-creation and co-destruction in self-service technology: A customer's perspective Electron Commer Res Appl 46 https://doi.org/10.1016/j.elerap.2021.101029 |
[34] | Huang SYB, Lee CJ, Lee SC (2021) Toward a Unified Theory of Customer Continuance Model for Financial Technology Chatbots. Sensors 21. https://doi.org/10.3390/s21175687 |
[35] | Hwang S, Kim J (2021) Toward a chatbot for financial sustainability. Sustainability 13: 3173. https://doi.org/10.3390/su13063173 |
[36] | Illescas-Manzano MD, López NV, González NA, et al. (2021) Implementation of chatbot in online commerce, and open innovation. J Open Innovation: Technol Mark Complexity 7: 125. https://doi.org/10.3390/joitmc7020125 |
[37] | Jang M, Jung Y, Kim S (2021) Investigating managers' understanding of chatbots in the Korean financial industry. Comput Hum Behav 120: 106747. https://doi.org/10.1016/j.chb.2021.106747 |
[38] | Kasilingam DL (2020) Understanding the attitude and intention to use smartphone chatbots for shopping. Technology in Society Technology in Society 62. https://doi.org/10.1016/j.techsoc.2020.101280 |
[39] | Khan MM (2020) Development of an e-commerce Sales Chatbot. International Conference on Smart Communities: Improving Quality of Life Using ICT, IoT and AI 173-176. https://doi.org/10.1109/HONET50430.2020.9322667 |
[40] | Khan S, Rabbani MR (2020) Chatbot as Islamic Finance Expert (CaIFE) When Finance Meets Artificial Intelligence. Proceedings of the 2020 4th International Symposium on Computer Science and Intelligent Control 2020: 1-5. https://doi.org/10.1145/3440084.3441213 |
[41] |
Kumar V, Ramachandran D, Kumar B (2021) Influence of new-age technologies on marketing: A research agenda. Expert Syst Appl 125: 864-877. https://doi.org/10.1016/j.jbusres.2020.01.007 doi: 10.1016/j.jbusres.2020.01.007
![]() |
[42] |
Kushwaha AK, Kumar P, Kar AK (2021) What impacts customer experience for B2B enterprises on using AI-enabled chatbots? Insights from Big data analytics. Ind Market Manag 98: 207-221. https://doi.org/10.1016/j.indmarman.2021.08.011 doi: 10.1016/j.indmarman.2021.08.011
![]() |
[43] | Lai ST, Leu FY, Lin JW (2018) A banking chatbot security control procedure for protecting user data security and privacy. International Conference on Broadband and Wireless Computing, Communication and Applications 561-571. https://doi.org/10.1007/978-3-030-02613-4_50 |
[44] |
Lee M, Frank L, IJsselsteijn W, et al. (2021) A Cryptocurrency Chatbot in the Social-technical Gap of Trust. Comput Supp Coop Work 30: 79-117. https://doi.org/10.1007/s10606-021-09392-6 doi: 10.1007/s10606-021-09392-6
![]() |
[45] | Li CH, Chen K, Chang YJ (2019) When there is no progress with a task-oriented chatbot: A conversation analysis. Proceedings of the 21st International Conference on Human-Computer Interaction with Mobile Devices and Services. https://doi.org/10.1145/3338286.3344407 |
[46] | Li L, Li C, Ji D (2021) Deep context modeling for multi-turn response selection in dialogue systems. Information Processing and Management 58. https://doi.org/10.1016/j.ipm.2020.102415. |
[47] |
Liu D, Santhanam R, Webster (2017) Toward Meaningful Engagement: a framework for design and research of Gamified information systems. MIS Quarterly 41: 1011-1034 https://doi.org/10.25300/MISQ/2017/41.4.01 doi: 10.25300/MISQ/2017/41.4.01
![]() |
[48] |
Lo Presti L, Maggiore G, Marino V (2021) The role of the chatbot on customer purchase intention: towards digital relational sales. Italian J Market 2021: 165-188, https://doi.org/10.1007/s43039-021-00029-6 doi: 10.1007/s43039-021-00029-6
![]() |
[49] | Lukoff K, Yu C, Kientz J, et al. (2018) What makes smartphone use meaningful or meaningless? Proceedings of the ACM on Interactive, Mobile, Wearable and Ubiquitous Technologies 2: 1-26. https://doi.org/10.1145/3191754 |
[50] | Mayer RC, Davis JH (1999) The effect of the performance appraisal system on trust for management: A field quasi-experiment. J Appl Psychol 84: 123-136. |
[51] |
Miklosik A, Evans N, Qureshi AMA (2021) The Use of Chatbots in Digital Business Transformation: A Systematic Literature Review. IEEE Access 9: 106530-106539. https://doi.org/10.1109/ACCESS.2021.3100885 doi: 10.1109/ACCESS.2021.3100885
![]() |
[52] | Milian EZ, Spinola MM, Carvalho MM (2019) Fintechs: A literature review and research agenda. Electron Commer Res Appl 34. https://doi.org/10.1016/j.elerap.2019.100833 |
[53] |
Mondal A, Dey M, Das D, er al. (2018) An automated conversation system for the educational domain. International Joint Symposium on Artificial Intelligence and Natural Language Processing (ISAI-NLP) 2018: 1-5. https://doi.org/10.1109/iSAI-NLP.2018.8692927 doi: 10.1109/iSAI-NLP.2018.8692927
![]() |
[54] |
Muniasamy A, Alasiry A (2020) Deep Learning: The Impact on Future eLearning. Int J Emerg Technol 15: 188-199. https://doi.org/10.3991/ijet.v15i01.11435 doi: 10.3991/ijet.v15i01.11435
![]() |
[55] | Nayak B, Bhattacharyya SS, Krishnamoorthy B (2021) Explicating the role of emerging technologies and firm capabilities towards attainment of competitive advantage in health insurance service firms. Technol Forecast Soc Change 170: 120892. https://doi.org/10.1016/j.techfore.2021.120892 |
[56] |
Ng M, Kovila PLC, Ehsan T, et al. (2021) Simulating the effects of social presence on trust, privacy concerns & usage intentions in automated bots for finance. 2020 IEEE European Symposium on Security and Privacy Workshops 2021: 190-199. https://doi.org/10.1109/EuroSPW51379.2020.00034 doi: 10.1109/EuroSPW51379.2020.00034
![]() |
[57] | Nguyen D, M Chiu, YTH, et al. (2021) Determinants of continuance intention towards banks' chatbot services in vietnam: A necessity for sustainable development. Sustainability 13: 7625 https://doi.org/10.3390/su13147625 |
[58] |
Nordheim CB, Følstad A, Bjørkli CA (2019) An Initial Model of Trust in Chatbots for Customer Service-Findings from a Questionnaire Study. Interact Comput 31: 317-335. https://doi.org/10.1093/iwc/iwz022 doi: 10.1093/iwc/iwz022
![]() |
[59] | Nuruzzaman M, Hussain (2020) IntelliBot: A Dialogue-based chatbot for the insurance industry. Knowl-Based Syst 196: 105810. https://doi.org/10.1016/j.knosys.2020.105810 |
[60] | Poncette AS, Rojas PD, Hofferbert J, et al. (2020) Hackathons as stepping stones in health care innovation: case study with systematic recommendations. J Med Internet Res 22: e17004. https://doi.org/10.2196/17004 |
[61] |
Quah JTS, Chua YW (2019) Chatbot assisted marketing in financial service industry. Lecture Notes in Computer Science, 11515 LNCS : 107-114. https://doi.org/10.1007/978-3-030-23554-3_8 doi: 10.1007/978-3-030-23554-3_8
![]() |
[62] |
Rajaobelina L, Brun I, Kilani N, et al. (2021) Examining emotions linked to live chat services: The role of e-service quality and impact on word of mouth. J Financ Serv Market 2021: 1-8. https://doi.org/10.1057/s41264-021-00119-8 doi: 10.1057/s41264-021-00119-8
![]() |
[63] | Rodríguez Cardona D, Werth O, Schönborn S, et al. (2019) A mixed methods analysis of the adoption and diffusion of Chatbot Technology in the German insurance sector. Twenty-fifth Americas Conference on Information Systems, Cancun. |
[64] |
Sowa K, Przegalinska A, Ciechanowski L (2021) Cobots in knowledge work: Human-AI collaboration in managerial professions. J Bus Res 125: 135-142. https://doi.org/10.1016/j.jbusres.2020.11.038 doi: 10.1016/j.jbusres.2020.11.038
![]() |
[65] |
Stoeckli E, Dremel C, Uebernickel F, et al. (2020) How affordances of chatbots cross the chasm between social and traditional enterprise systems. Electron Mark 30: 369-403. https://doi.org/10.1007/s12525-019-00359-6 doi: 10.1007/s12525-019-00359-6
![]() |
[66] |
Suhel SF, Shukla VK, Vyas S, et al. (2020) Conversation to Automation in Banking through Chatbot Using Artificial Machine Intelligence Language. IEEE 8th International Conference on Reliability, Infocom Technologies and Optimization (Trends and Future Directions)2020: 611-618. https://doi.org/10.1109/ICRITO48877.2020.9197825 doi: 10.1109/ICRITO48877.2020.9197825
![]() |
[67] | Thompson C (2018) Assessing chatbot interaction as a means of driving customer engagement. Bachelor's Thesis. |
[68] | Thusi P, Maduku DK (2020) South African millennials' acceptance and use of retail mobile banking apps: An integrated perspective. Comput Hum Behav 111. https://doi.org/10.1016/j.chb.2020.106405 |
[69] | Toader DC, Boca G, Toader R, et al. (2020) The effect of social presence and chatbot errors on trust. Sustainability 12. https://doi.org/10.3390/SU12010256 |
[70] | Tran OT, Luong TC (2020) Understanding what the users say in chatbots: A case study for the Vietnamese language. Eng Appl Artif Intell 87: 103322. https://doi.org/10.1016/j.engappai.2019.103322 |
[71] |
van den Broeck E, Zarouali B, Poels K (2019) Chatbot advertising effectiveness: When does the message get through? Comput Hum Behav 98: 150-157. https://doi.org/10.1016/j.chb.2019.04.009 doi: 10.1016/j.chb.2019.04.009
![]() |
[72] | Vikar M, Honmane V, Rao S (2019) Humanizing the Chatbot with Semantics based Natural Language Generation. Proceedings of the International Conference on Intelligent Computing and Control Systems 891-894. https://doi.org/10.1109/ICCS45141.2019.9065723 |
[73] |
Vlačić, Božidar, Leonardo Corbo, et al. (2021) The evolving role of artificial intelligence in marketing: A review and research agenda. J Busi Res 128: 187-203. https://doi.org/10.1016/j.jbusres.2021.01.055 doi: 10.1016/j.jbusres.2021.01.055
![]() |
[74] | Weerabahu D, Gamage A, Dulakshi C, et al. (2019) Digital Assistant for Supporting Bank Customer Service. International Conference of the Sri Lanka Association for Artificial Intelligence 177-186. Springer, Singapore. https://doi.org/10.1007/978-981-13-9129-3_13 |
[75] |
Yamada M, Goda Y, Matsukawa H (2015) A computer-supported collaborative learning design for quality interaction. IEEE Multimedia 23: 48-59. https://doi.org/10.1109/MMUL.2015.95 doi: 10.1109/MMUL.2015.95
![]() |
[76] |
Ye W, Li Q (2020) Chatbot Security and Privacy in the Age of Personal Assistants. 2020 IEEE/ACM Symposium on Edge Computing (SEC)2020:388-393. https://doi.org/10.1109/SEC50012.2020.00057 doi: 10.1109/SEC50012.2020.00057
![]() |
[77] |
Zhang JZ, Watson IV GF (2020) Marketing ecosystem: An outside-in view for sustainable advantage. Ind Market Manag 88: 287-304. https://doi.org/10.1016/j.indmarman.2020.04.023 doi: 10.1016/j.indmarman.2020.04.023
![]() |
[78] |
Zhou L, Gao J, Li D, et al. (2020) The design and implementation of xiaoice, an empathetic social chatbot. Comput Linguist 46: 53-93. https://doi.org/10.1162/coli_a_00368 doi: 10.1162/coli_a_00368
![]() |
1. | Markus Haas, The Cowles–Jones test with unspecified upward market probability, 2023, 3, 2769-2140, 324, 10.3934/DSFE.2023019 | |
2. | Katakam Bhavana, Indukuri Raghu Varma, Kalluri Rohitha, G Suryanarayana, Kachi Anvesh, Sama Kruthika, 2024, Predicting and Forecasting Metal Stock Prices Using Machine Learning, 979-8-3315-0579-0, 102, 10.1109/ICCCMLA63077.2024.10871315 |
Base Learner | Source | Algorithm |
Homogeneous | Giacomel et al., 2015; Lahmiri, 2018; Lahmiri and Boukadoum, 2015; Nezhad and Bidgoli, 2019 |
ANN |
Xie et al., 2018; Yang et al., 2020 | LSTM | |
Zhou et al., 2022 | Decision Tree | |
Qiu et al., 2017 | SVM | |
Chandrasekara et al., 2019 | PNN | |
Khuwaja et al., 2020 | ELM | |
Wang et al., 2018 | DBN | |
Zhong et al., 2017 | Random Forest | |
Lin et al., 2021 | KNN | |
Melin et al., 2012 | ANFIS1 |
Base Learner | Source | Algorithms |
Heterogeneous | Abraham and Auyeung, 2009 | ANN, DBNN, NFS, SVM |
Alhnaity and Abbod, 2020 | BPNN, RNN, SVR | |
Chong et al., 2020 | CNN, CNN-LSTM, LSTM | |
Qian and Rasheed, 2007 | ANN, Decision Tree, KNN | |
Barak et al., 2017 | ANN, Decision Tree, Rule-Based Algorithm, SVM | |
Dash et al., 2019 | MLP2, Random Forest, Naïve Bayes, SVM | |
Lee and Kim, 1995 | ID3, Expert Knowledge, User Knowledge | |
Sawhney et al., 2020 | MTL3, Speech and Text Encoder, SVM | |
Kuo et al., 1996 | ANN, Delphi Method | |
Kristjanpoller and Michell, 2018 | ANFIS, GARCH |
Base Learner | Source | Algorithm |
Homogeneous | Giacomel et al., 2015; Lahmiri, 2018; Lahmiri and Boukadoum, 2015; Nezhad and Bidgoli, 2019 |
ANN |
Xie et al., 2018; Yang et al., 2020 | LSTM | |
Zhou et al., 2022 | Decision Tree | |
Qiu et al., 2017 | SVM | |
Chandrasekara et al., 2019 | PNN | |
Khuwaja et al., 2020 | ELM | |
Wang et al., 2018 | DBN | |
Zhong et al., 2017 | Random Forest | |
Lin et al., 2021 | KNN | |
Melin et al., 2012 | ANFIS1 |
Base Learner | Source | Algorithms |
Heterogeneous | Abraham and Auyeung, 2009 | ANN, DBNN, NFS, SVM |
Alhnaity and Abbod, 2020 | BPNN, RNN, SVR | |
Chong et al., 2020 | CNN, CNN-LSTM, LSTM | |
Qian and Rasheed, 2007 | ANN, Decision Tree, KNN | |
Barak et al., 2017 | ANN, Decision Tree, Rule-Based Algorithm, SVM | |
Dash et al., 2019 | MLP2, Random Forest, Naïve Bayes, SVM | |
Lee and Kim, 1995 | ID3, Expert Knowledge, User Knowledge | |
Sawhney et al., 2020 | MTL3, Speech and Text Encoder, SVM | |
Kuo et al., 1996 | ANN, Delphi Method | |
Kristjanpoller and Michell, 2018 | ANFIS, GARCH |