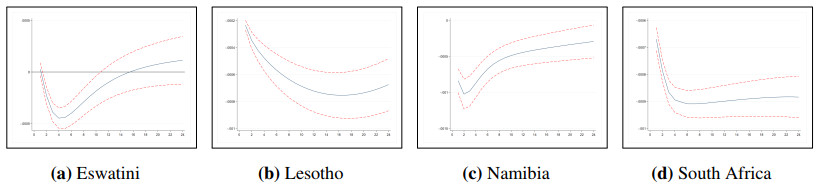
Academic interest in understanding the role of financial technology (FinTech) in sustainable development has grown exponentially in recent years. Many studies have highlighted the context, yet no reviews have explored the integration of FinTech and sustainability through the lens of the banking aspect. Therefore, this study sheds light on the literature trends associated with FinTech and sustainable banking using an integrated bibliometric and systematic literature review (SLR). The bibliometric analysis explored publication trends, keyword analysis, top publisher, and author analysis. With the SLR approach, we pondered the theory-context-characteristics-methods (TCCM) framework with 44 articles published from 2002 to 2023. The findings presented a substantial nexus between FinTech and sustainable banking, showing an incremental interest among global scholars. We also provided a comprehensive finding regarding the dominant theories (i.e., technology acceptance model and autoregressive distributed lag model), specific contexts (i.e., industries and countries), characteristics (i.e., independent, dependent, moderating, and mediating variables), and methods (i.e., research approaches and tools). This review is the first to identify the less explored tie between FinTech and sustainable banking. The findings may help policymakers, banking service providers, and academicians understand the necessity of FinTech in sustainable banking. The future research agenda of this review will also facilitate future researchers to explore the research domain to find new insights.
Citation: Md. Shahinur Rahman, Iqbal Hossain Moral, Md. Abdul Kaium, Gertrude Arpa Sarker, Israt Zahan, Gazi Md. Shakhawat Hossain, Md Abdul Mannan Khan. FinTech in sustainable banking: An integrated systematic literature review and future research agenda with a TCCM framework[J]. Green Finance, 2024, 6(1): 92-116. doi: 10.3934/GF.2024005
[1] | Patrick Mumbi Chileshe . Banking structure and the bank lending channel of monetary policy transmission: Evidence from panel data methods. Quantitative Finance and Economics, 2018, 2(2): 497-524. doi: 10.3934/QFE.2018.2.497 |
[2] | Richard J. Cebula, Robert Boylan . Uncertainty regarding the effectiveness of Federal Reserve monetary policies over time in the U.S.: an exploratory empirical assessment. Quantitative Finance and Economics, 2019, 3(2): 244-256. doi: 10.3934/QFE.2019.2.244 |
[3] | Haryo Kuncoro, Fafurida Fafurida, Izaan Azyan Bin Abdul Jamil . Growth volatility in the inflation-targeting regime: Evidence from Indonesia. Quantitative Finance and Economics, 2024, 8(2): 235-254. doi: 10.3934/QFE.2024009 |
[4] | Cunyi Yang, Li Chen, Bin Mo . The spillover effect of international monetary policy on China's financial market. Quantitative Finance and Economics, 2023, 7(4): 508-537. doi: 10.3934/QFE.2023026 |
[5] | Simiso Msomi, Damien Kunjal . Industry-specific effects of economic policy uncertainty on stock market volatility: A GARCH-MIDAS approach. Quantitative Finance and Economics, 2024, 8(3): 532-545. doi: 10.3934/QFE.2024020 |
[6] | Serçin ŞAHİN, Serkan ÇİÇEK . Interest rate pass-through in Turkey during the period of unconventional interest rate corridor. Quantitative Finance and Economics, 2018, 2(4): 837-859. doi: 10.3934/QFE.2018.4.837 |
[7] | Gülserim Özcan . The amplification of the New Keynesian models and robust optimal monetary policy. Quantitative Finance and Economics, 2020, 4(1): 36-65. doi: 10.3934/QFE.2020003 |
[8] | Thomas C. Chiang . Economic policy uncertainty and stock returns—evidence from the Japanese market. Quantitative Finance and Economics, 2020, 4(3): 430-458. doi: 10.3934/QFE.2020020 |
[9] | David Kuo Chuen Lee, Chia Mei Shih, Jincheng Zheng . Asian CBDCs on the rise: An in-depth analysis of developments and implications. Quantitative Finance and Economics, 2023, 7(4): 665-696. doi: 10.3934/QFE.2023032 |
[10] | Victor J. Valcarcel . Yield curve rotations, monetary shocks, and Greenspan’s Conundrum. Quantitative Finance and Economics, 2019, 3(1): 1-21. doi: 10.3934/QFE.2019.1.1 |
Academic interest in understanding the role of financial technology (FinTech) in sustainable development has grown exponentially in recent years. Many studies have highlighted the context, yet no reviews have explored the integration of FinTech and sustainability through the lens of the banking aspect. Therefore, this study sheds light on the literature trends associated with FinTech and sustainable banking using an integrated bibliometric and systematic literature review (SLR). The bibliometric analysis explored publication trends, keyword analysis, top publisher, and author analysis. With the SLR approach, we pondered the theory-context-characteristics-methods (TCCM) framework with 44 articles published from 2002 to 2023. The findings presented a substantial nexus between FinTech and sustainable banking, showing an incremental interest among global scholars. We also provided a comprehensive finding regarding the dominant theories (i.e., technology acceptance model and autoregressive distributed lag model), specific contexts (i.e., industries and countries), characteristics (i.e., independent, dependent, moderating, and mediating variables), and methods (i.e., research approaches and tools). This review is the first to identify the less explored tie between FinTech and sustainable banking. The findings may help policymakers, banking service providers, and academicians understand the necessity of FinTech in sustainable banking. The future research agenda of this review will also facilitate future researchers to explore the research domain to find new insights.
The CMA is a multilateral agreement that provides a framework for a fixed exchange rate regime between the South African rand (ZAR) and the currencies of Lesotho, Eswatini1, and Namibia (herein referred to as the LEN countries). The main objective of this currency union is to foster the sustained economic development and advancement of the less developed members (Wang et al., 2007). The Agreement gives member countries the power to issue their local currencies with the South-African bilateral agreements dictating the areas where the currencies are legal tenders. A crucial concern about the structure of the CMA is the absence of a joint central bank that is responsible for conducting monetary policy interventions and is accountable to all member countries. The CMA arrangement constrains the LEN countries from exercising independent discretionary monetary policy. As a result, South Africa's economic superiority over the LEN countries is exerted through its sole discretion over monetary policy decisions in the region. The South African Reserve Bank (SARB) is responsible for monetary policy formulation and implementation with its local economy as its primary target. The core assumption of this policy arrangement is that as long as the LEN currencies are fixed to the ZAR and the SARB pursues a domestic policy of low and stable inflation, policy effects will be transmitted from the South African economy to the rest of the LEN countries without delay (Seleteng, 2016). However, experiences from developed countries and other monetary unions suggests that the transmission of monetary policy depends on multiple factors that should be carefully studied. The Optimal Currency Area (OCA) literature highlights the benefits of monetary integration if there is a high degree labour mobility, price and wage flexibility, inflation differentials, effectiveness of monetary policy, and correlation of shocks among member countries (Corden, 1972; Mundell, 1973; 1961).
1Before 2018, Eswatini was known as Swaziland.
Following SARB's adoption of an inflation rate targeting (IT) regime in 2000, there was rise in research that focused on the efficacy of this policy instrument in South Africa. Several studies in South Africa suggest that SARB's inflation-targeting framework is ineffective in restraining inflationary pressures within the target range (Ikhide and Uanguta, 2010; Ajilore and Ikhide, 2013; Bonga-Bonga and Kabundi, 2015; Seleteng, 2016). For example, during the period from 2014 to 2016, the SARB entered a contractionary phase when forecasts indicated that inflation was expected to rise. These contractionary monetary policy episodes are found to have negatively affected economic growth in South Africa, especially in manufacturing production (Bonga-Bonga and Kabundi, 2015). There is a limited number of research studies that extend this analysis to the LEN countries. Empirically, it is still not clearly understood how South Africa's monetary policy decisions affect the LEN economies and the channels through which these effects are transmitted.
Given the limited scope for monetary policy adjustment by the LEN countries, it is crucial to understand how policy-induced changes are transmitted from South Africa to the LEN countries for the following reasons. Firstly, a clear and functional understanding of the monetary policy transmission mechanism may help authorities and policymakers to precisely ascertain the relative effectiveness of the channels to achieve policy targets. Secondly, since the financial sector in the LEN countries is highly integrated with their South-African counterpart, adequate information about the mechanism may enable appropriate weights and emphasis to be placed on monetary policy targets and goals during monetary policy design and implementation. Finally, in the case where these channels do not optimally function for a member country, an adjustment mechanism such as liquidity management may be implemented to restore the economy to equilibrium, thus correcting domestic liquidity imbalances.
This study addresses the gap in the literature on the CMA by comparatively evaluating the transmission of South Africa's monetary policy shocks in the region. This study empirically attempts to answer the questions: How do monetary policy-induced changes affect macroeconomic indicators in the CMA? How effectively does each member country respond to those changes? This study implements a structural vector autoregressive (SVAR) model with short-run restriction and uses the estimated impulse response functions (IRFs) to address these questions. In particular, this paper assesses how economic output, inflation, broad money supply, domestic credit, lending rate spread across the CMA countries react to shocks to the South African repo rate. Following the recent works by Ikhide and Uanguta (2010) and Seleteng (2016), this study similarly identifies the South African repo rate (henceforth referred to as the repo rate) as the relevant monetary policy instrument of the CMA. Unlike previous studies in the CMA, this study uses monthly data from February 2000 to December 2018 to better reflect the short-run dynamics of monetary policy during the IT period. Monthly GDP data is estimated from quarterly observations using the Chow and Lin (1971) regressions approach. The U.S. federal reserve funds rate is included in the estimated model to control for changes to domestic monetary policy due to external shocks following studies such as Kim and Roubini (2000) and Aslanidi (2007).
The rest of this paper is structured as follows: Section two provides a review of the theoretical and empirical studies on monetary policy transmission mechanisms in developed and emerging market economies. Section three presents an overview of the CMA, the structure of the arrangement, and the unique features of monetary policy for each member country. Section four describes the data used in this study, the theoretical background of short-run SVAR models, and empirical model specifications. Sections five and six presents the results and discussion. Section six provides the results of robustness tests. Lastly, section seven concludes the findings in the study.
Whether or not monetary policy has any effect on prices and output is an issue that has been debated since the Classical Quantity Theory in the 20th century. The literature on monetary policy has identified several channels through which policy effects can be transmitted. Mishkin (1995) specified the four most prominent channels, namely; interest rate, exchange rate, bank credit, and equity price channels. The interest rate channel (which is the prime focus of this paper) is viewed as the main channel of monetary policy transmission (Can et al., 2020; Loayza and Schmidt-Hebbel, 2002; Mishkin, 1995; Taylor, 1995). The interest rate channel theoretically functions under the assumption of nominal rigidities, that is, firms are subject to short-term constraints in their response to monetary policy changes. Under this assumption, a contractionary monetary policy leads to a rise in the real interest rate, which in turn increases the cost of capital. The rising cost of capital leads to a decline in investment spending and aggregate demand, which ultimately causes a decline in output.
For countries considering forming a monetary union, the OCA literature has laid out a set of conditions necessary for achieving desired monetary policy goals without compromising economic outcomes of one member for the other. If these conditions are satisfied, the benefits of a monetary union are derived from the adoption of a single currency and elimination of exchange rate volatility. However, adoption of fixed exchange rate regime and free capital mobility requires member countries to surrender independent monetary policy. This theory was formally introduced by Mundell (1968) who argued that factor mobility and price and wage flexibility were important for coping with economic shocks. In the case where wages/prices are rigid and labor is immobile, countries may find it harder to adjust to asymmetric shocks because the single monetary policy will not be appropriate to respond to idiosyncratic shocks. Research that followed this work has identified several other important factors such as capital mobility, similarity of shocks, and Business cycle synchronisation (Corden, 1972; Mundell, 1973; Tavlas, 2009)2.
2See Kunroo (2016) for a detailed survey of the OCA literature. The main criticism of OCA theory has been its failure to identify an overriding criterion that can be used to judge the desirability and/or viability of a monetary union
Empirically, there is extensive literature on the existence and efficacy of the interest rate channel in developed countries (Arestis and Sawyer, 2003; Bernanke and Gertler, 1995; Dodge, 2002; Iwata and Wu, 2006; Leeper et al., 1996; Peersman and Smets, 2001; Rafiq and Mallick, 2008; Tavlas, 2009), however, similar studies in developing countries (especially in Southern Africa) have only become popular in the last two decades. Most notably, Cheng (2006) applied both recursive and non-recursive SVAR models to monthly data in Kenya for the period 1997 to 2005. Similar to our study, Cheng (2006) obtained monthly GDP estimates by interpolating annual GDP data using low frequency industry production data. The findings showed that a contractionary monetary policy leads to an initial increase in price levels, followed by a statistically significant decline for a period of about two years following the shock. The response to a contractionary monetary policy was an initial rise in output but eventually tapering off. However, this decline is not statistically significant. Shocks to the interest rate were found to explain a much larger fraction of inflation than output. The author concluded that there is evidence of exchange rate pass-through to inflation given that positive shocks to interest rates lead initially to an exchange rate depreciation, but eventually appreciates for about two years.
Buigut (2009) estimated a three-variable recursive VAR for three East African Community (EAC)3 countries using data from the period between 1984 and 2006. The paper explored the importance of the interest channel in the region. The authors found that the interest rate transmission mechanism is weak in all three countries. A shock to the interest rate was found to have no statistically significant effect on either inflation or real output. However, Davoodi et al. (2013) argued that these findings are biased by several factors: (i) The study uses a sample that includes too few observations for empirical analyses, resulting in few degrees of freedom; (ii) it includes periods of substantial changes in monetary policy implementation, financial deepening, and other structural shifts in each economy which may have contributed to large uncertainty surrounding the effectiveness of monetary policy. Davoodi et al. (2013) proposed that using a Bayesian VAR model could resolve these issues because it provides an effective way of dealing with over-parameterization. In contrast, their results found that an expansionary monetary increases prices significantly in Kenya and Uganda, while output increases in Burundi, Kenya, and Rwanda.
3The author focused on Kenya, Tanzania, and Uganda.
Similar to the current study, there are very few studies that compare the effect of South Africa's monetary policy conduct on the economies of the CMA countries. Ikhide and Uanguta (2010) and Seleteng (2016) both examined the impact of SARB's monetary policy on the LEN economies using a VAR framework. Unlike the approach in this study, and Ikhide Uanguta (2010) used monthly data but excluded economic output from the estimated models, while Seleteng (2016) used annually aggregated data. These studies focused on how changes in the SARB's monetary policy instrument (repo rate) affect the money supply, credit, and prices in the CMA and thus evaluate the ability of the LEN economies to undertake independent monetary policy under the prevalent structure. Both studies found statistically significant results that lending rates and price levels were instantaneously sensitive to changes in the repo rate. However, Ikhide and Uanguta (2010) also found that money supply is instantaneously responsive to the repo while rate, Seleteng (2016) did not find any significant relationship. These significant differences may be attributed to several factors such as the difference in the period under study, specification of the model, and structural breaks. Ikhide and Uanguta (2010) confirmed that the repo rate is a relevant policy instrument in the LEN economies. Both studies concluded that the LEN countries are not capable of independent monetary policy given the nature of their agreement with South Africa.
There are also country-specific studies that have investigated the transmission of monetary policy in the CMA. For example, Gumata et al. (2013) investigated the presence of different monetary policy transmission channels in South Africa using a Large Bayesian VAR model with quarterly data from the period 1990Q1 to 2012Q2. Their findings revealed that credit, interest rate, asset prices, exchange rate, and expectations channels are all potent in the South African economy, but differ in magnitudes. The study concluded that the interest rate channel was the most important transmitter of monetary policy shocks. Bonga-Bonga (2010) examined the responses of the short and long-term interest rates to monetary, demand, and supply shocks in South Africa using data from 1986 to 2007. The empirical analysis conducted in this study followed an SVAR methodology with long-run restrictions. The author found that the effects of monetary policy shocks caused the short and long-term interest rates to move in the same direction. However, the short-run and long-run interest rates move in different directions in the presence of positive supply shocks.
The reviewed literature from the CMA shows that most studies examined the monetary policy transmission mechanism in the CMA using VAR or SVAR models. These studies included data from the period before and after the inflation targeting regime was adopted. The selected studies reveal mixed results regarding the effectiveness of monetary policy in the CMA. Some studies found a causal relationship from monetary policy instruments to macroeconomic variables (Ikhide and Uanguta, 2010; Seleteng, 2016; Sheefeni and Ocran, 2012) while others found that monetary policy is ineffective (Mkhonta, 2018; Dlamini and Skosana, 2017). Our study follows the recommendations of Blanchard and Watson (1984), amongst others, to identify monetary policy shocks in the CMA using an SVAR approach. Although the results may not change drastically, this paper will explore where structural identification will help to address the inconsistencies in the literature. Unlike previous studies in the CMA, data used in this study focuses on the period after the inflation targeting regime was adopted, following Davoodi et al. (2013). This approach will ensure that the estimated results are consistent since the monetary policy transmission mechanism is sensitive to structural shifts. Most of the reviewed studies did not incorporate any controls for external changes that may influence the implementation of monetary policy in the CMA. The U.S. federal funds rate will be included in the estimated model to control for exogenous shocks to the repo rate, as recommended by Kim and Roubini (2000).
The origins of the CMA can be traced back to the creation of the Rand Monetary Area (RMA) between governments Eswatini, Lesotho, and South Africa in 1974. Before this period, the South African currency was informally circulated as a medium of exchange and legal tender in Botswana, Eswatini, and Lesotho. These countries were de facto part of South Africa's monetary system since the internal movement of capital within the region was not restricted and all external transactions were executed through South African banks under South African exchange control regulations. The RMA was updated to the Common Monetary Area in 1986 after Eswatini and Lesotho individually negotiated terms with South Africa. The CMA became a multipartite agreement when Namibia formally became a member in 1992, shortly after gaining its independence.
The CMA differs greatly from a typical money union like the Euro Zone. Its unique features reflect the historical dominance of South Africa in the region and the dependence of smaller LEN countries on its economy. The current structure of the CMA can be summarized as follows. Each of the four members has an independent central bank, which is responsible for implementing policies and issuing national currencies. The currencies of Lesotho (LSL), Eswatini (SZL), and Namibia (NAD) have been pegged one to one with the South African Rand (ZAR). The ZAR is the only currency that circulates as legal tender throughout the entire CMA region while currencies of the rest of the members are restricted within their respective borders. Individual LEN currencies and the ZAR are perfect substitutes, with no conversion costs. To support the fixed exchange rate regime, LEN countries are required to back their currency issues with foreign exchange reserves. The reserves are kept in a common pool managed by SARB, and they can be made available upon any member's request. There are no restrictions to intra-CMA funds transfers (capital or current transactions). However, all CMA members apply a common exchange control system that is determined by the South African authorities. The LEN currencies receive annual payments from South Africa as compensation for foregone seigniorage.
The institutional framework of the CMA inhibits the LEN from exercising independent discretionary monetary policy. The LEN countries are required to set interest rates within close range to those in South Africa to avoid distorting the fixed exchange rate. In effect, the CMA has granted the South African authorities the power to formulate and implement monetary policy for the whole region. The CMA arrangement provides the SARB with a wider range of monetary policy options relative to the rest of the members. Consequently, monetary policy instruments implemented in South Africa have implications for the rest of the CMA. Before 2000, the SARB operated under an eclectic approach to monetary policy, where growth in money supply and credit extension was used as intermediate guidelines for short-term interest rates. However, this policy was not transparent, and sometimes questionable actions were taken that were costly to the domestic economic growth (Aron and Muellbauer, 2007). The inflation-targeting approach was adopted to enhance policy transparency, accountability, and predictability. Under the South African IT framework, the appropriate measure of inflation was identified as CPIX (CPI less mortgage interest rates) which is specifically maintained within a target range of 3 to 6 percent. The repo rate remains the main operational tool for maintaining price stability. The adoption of the IT framework marked the SARB's shift to a floating exchange rate regime to ensure the competitiveness of South Africa's exports. However, the IT approach in South Africa has been experiencing a decline in efficiency over time, as evidenced by the failure of high interest rates to keep inflation within the target range (Meyer et al., 2018). Other related studies suggest that SARB's inflation target range is very low for conducive economic growth in the LEN countries. For instance, Mosikari and Eita (2018) estimates that the optimal level of inflation for Eswatini is 12 percent, while Seleteng (2005) estimated 10 percent for Lesotho. This suggests that the SARB's policies that lower inflation to the target band may have a negative impact on the LEN economies. In addition, the CMA countries are also members of the Southern African Customs Union (SACU), which could further distort the efficacy of of monetary policy in the region4. In recent years following the 2008 global financial crisis, the SARB added a complementary mandate to oversee and maintain financial stability, which to some extent may influence the effect of monetary policy not only in South Africa but throughout the rest of the CMA.
4The SACU arrangement allows for free trade among members and a common external tariff on goods from outside the Union.
This study uses monthly data for the period between 2000M2 and 2018M12 for all four member countries of the CMA (there are 227 observations per country). Macroeconomic data for individual countries was sourced from reports from the South African Reserve Bank, Central Bank of Lesotho, Bank of Namibia, and Central Bank of Eswatini. Missing data was complemented with reports from the International Monetary Fund's International Financial Statistics. Following Ikhide and Uanguta (2010), Seleteng (2016), and Cheng (2006), data on the following variables were gathered; economic output (lgdp), inflation5 (lcpi), broad money supply6 (lm2), domestic credit (ldc), and lending rate spread (lrs). All of these variables, except economic output, were obtained at a monthly frequency. A statistical approach described in Appendix A was implemented by interpolating monthly GDP from observed quarterly GDP data. This technique is particularly useful in this analysis since estimating SVAR models requires all variables to have the same frequency. Consistent with past studies, (Bernanke, 1986: Ikhide and Uanguta, 2010; Davoodi et al., 2013) for example, the following variables have been log-transformed: money supply, inflation, domestic credit, economic output.
5Indexed such that CPI2010M06=100.
6For the LEN countries, M2 does not include the estimated amount of ZAR circulating their respective countries.
This paper implements a short-run SVAR model to trace the impact of shocks to the repo rate on selected macroeconomic variables in the CMA. The strength of this model lies in the use of forecast error variance decomposition to quantify the average contribution of a given structural shock to the variability of the data over time. Structural VAR model is a multivariate, linear representation of a vector of observable variables lagged on itself. Let n be the number of endogenous variables in the model, following (Bernanke, 1986; Kim and Roubini, 2000; Aslanidi, 2007), the structure of each economy in the CMA can be described in a reduced form as
Yt=p∑i=1AiYt−i+BXt+εtYt=A1Yt−1+⋯+ApYt−p+BXt+εt | (1) |
where Yt is an (n×1) vector of endogenous variables observed at time t. Xt is a vector of exogenous variables. Ai is an (n×n) vector of coefficient estimates, εt is an (n×1) vector of of serially uncorrelated reduced form disturbances, and p is the optimal lag length of each variable. The variance-covariance matrix is, E(εtε′t)=φ.
Equation 1 can be reparameterized into its structural form as
Γ0Yt=p∑i=1ΓiYt−i+ΠXt+μt=Γ1Yt−1+⋯+ΓpYt−p+ΠXt+μt | (2) |
where Γ0 is the contemporaneous coefficients matrix with the diagonal elements normalized to equal one but the off-diagonal elements may be arbitrary. Γi represents the matrices of the parameters of the economic variables, and μt represents the structure of the economic shock, with variance and covariance matrices denoted as, E(μtμ′t)=ϑ.
Therefore, the link between the reduced (Eq. 1) and structural (Eq. 2) forms is,
Ai=Γ−10Γi,B=Γ−10Π,andμt=Γ0εt | (3) |
Likewise, the variance-covariance matrix relationship between reduced and structural forms can be written as
φ=Γ0ϑ(Γ−10) | (4) |
The reduced form can be estimated using OLS, and point estimates of the parameters (Ai) and variance-covariance matrix (φ) can be found. However, identifying restrictions have to be imposed to recover the structural form parameters.
The estimates for ϑ and the parameters in the structural form representation can be obtained only through the estimates of φ. The matrix ϑ has n(n+1) parameters to be estimated, while φ contains only n(n+1)/2. Thus, at least n(n−1)/2 restrictions must be imposed on the contemporaneous matrix Γ0 to recover the structural form parameters.
This section describes the set-up of the short-run SVAR model for the economies of Eswatini, Lesotho, Namibia, and South Africa. A six-variable SVAR model was estimated independently for each CMA country following Kim and Roubini (2000) and Aslanidi (2007). The estimated SVAR model for country i, in time period t has the following endogenous variables,
Yit=[sarrt,lrsit,ldcit,lm2it,lcpiit,lgdpit]′ | (5) |
The U.S. Federal Funds rate (ffrt) is included exogenously in models as a control for foreign monetary policy shocks in setting domestic monetary policy (Kim and Roubini, 2000; Aslanidi, 2007). The repo-rate (sarrit) is used as a measure of domestic monetary policy stance in the CMA. The real GDP (lgdpit) and inflation (lcpiit) represent the economic activity in each country and help to characterize the market in the economy. The short-term interest rates are represented by the lending rate spread (lrsit). This enables an investigation into the interaction of monetary policy and the interest rate channel. Finally, domestic credit extension (ldcit) and broad money supply (lm2it) are important macroeconomic indicators that capture the level of economic activity. These variables are included to identify their dynamic effects on the real sector of the economy.
The focus of this paper is centered on the analysis of the resulting IRFs and FEVDs which estimate the responses of given variables to innovations in another variable in the system, ceteris paribus. As discussed earlier in the previous section, the estimation of the SVAR requires an identification scheme where a set of theoretically valid restrictions are imposed on the elements of the contemporaneous matrix Γ0. However, specifying the model restrictions is a daunting task. This is because it is not clear which aspects of the model arise due to the assumptions imposed on the model and which arise from the data. Aslanidi (2007) argues that the method of imposing restrictions is not different from other specification methods, although the results are likely to be sensitive to model specification and imposed restrictions. They point that this approach makes the results more transparent because it can be supported by economic theory.
The econometric identification of monetary policy shocks is crucial to the model specification. Consequently, this study specifically follows the identification scheme adopted by Kim and Roubini (2000) and Aslanidi (2007). However, the model is modified to take into account the context of monetary policy conduct in the CMA. The structural shocks are identified from their reduced form (εt) by imposing restrictions on the contemporaneous matrix Γ0 based on equation 3:
[μlgdpμlcpiμlm2μldcμlrsμsarr]=[100000Γ2110000Γ31Γ321000Γ41Γ42Γ431Γ45Γ460Γ520Γ541Γ560Γ620001][εlgdpεlcpiεlm2εldcεlrsεsarr] | (6) |
The structural disturbance in the model are represented by the μit matrix where; μlgdp→ domestic output shocks, μlcpi→ domestic inflation shocks, μlm2→ money supply shocks, μldc→ domestic credit shocks, μlrs→ lending rate spread shocks, and μsarr→ repo rate shocks. The residuals from reduced form equation (εit) show the unexpected changes in each variable given the information available.
The non-zero coefficients indicate that a variable i affects contemporaneously variable j. The restrictions imposed on the Γ0 matrix are based, firstly, on the timing of information. A zero restriction is imposed based on the fact that some variables are available to monetary authorities after a lag (e.g. GDP, inflation). Secondly, behavioral restrictions are imposed on variables that respond slowly to movements in financial and policy variables. The following restrictions are imposed on the contemporaneous matrix based on theoretical economic expectations;
1. The first and second equations represent the goods market. Economic output does not respond to price and financial signals (interest rate and credit) contemporaneously. This restriction reflects the assumption of nominal rigidities. Producers do not instantaneously alter output and prices in response to innovations in financial variables and monetary policy within a short time horizon. The price equation is assumed to be contemporaneously affected by changes in output (Aslanidi, 2007).
2. The money supply equation is assumed to be the reaction function of the monetary authority, which sets the level of money after observing the current values of output and the price levels (Kim and Roubini, 2000).
3. Domestic credit is assumed to contemporaneously respond to domestic output, price levels, money supply, and interest rates shocks. This is because the real activity and domestic interest rates give households expectations of future economic activity (Kim and Roubini, 2000). Similarly, the lending rate spread is assumed to contemporaneously change with all variables except GDP and money supply due to information lag. Borrowers will quickly respond to the changes in the real cost of borrowing.
4. Finally, the repo is the main monetary policy instrument in the CMA; hence we assume that it is the SARB's monetary reaction function. Given the inflation-targeting framework, the repo rate contemporaneously responds only to changes in inflation, but not to any other variables. This means that the reserve bank sets the repo rate only after observing the current level of inflation (Ikhide and Uanguta, 2010; Seleteng, 2016).
In the case of a six-variable SVAR model, a just-identified model requires at least 15 restrictions. This study imposed 18 restrictions on the contemporaneous matrix. That is, the estimated model is over-identified. The over-identifying restrictions can be validated/rejected by computing a likelihood ratio (LR) test that compares the log-likelihood of the structural form and the log-likelihood of the reduced form (Lütkepohl and Krätzig, 2004). Given the restrictions on Γ0, the structural system of contemporaneous variables can be expressed as
lgdpt=μlgdpΓ21lgdpt+lcpit=μlcpiΓ31lgdpt+Γ32lcpit+lm2t=μlm2Γ41lgdpt+Γ42lcpit+Γ43lm2t+ldct=μldcΓ52lcpit+Γ54ldct+lrst+Γ56sarrt=μlrsΓ62lcpit+sarrt=μsarr | (7) |
This section presents the estimated impact of monetary policy shocks on selected macroeconomic indicators in each CMA member country. To ensure correct model estimation, the data is tested for stationarity. The Augmented Dickey Fuller (ADF) test results presented in Appendix B reveals that all variables are stationary at first differences. Despite these results, our approach is to estimate the SVAR models at levels. Our strategy follows recent empirical studies such as Herrera and Pesavento (2013) among many others who argue that estimating VAR models in levels and identifying the structural impulse responses through short-run restrictions provides the most robust strategy. Several studies have drawn attention to the restrictive nature of the SVAR methodology, however, we agree with Bernanke and Gertler (1995) and many others who the impulse response functions provide more understanding of the impact of monetary policy than the statistical results.
The impulse response of economic output, inflation, broad money supply, domestic credit, and lending rate spread are generated from the estimated short-run SVAR model within a 24-month period after impact. Figures 1 through 5 provide impulse responses of selected variables to a one percent positive shock to the South African repo rate (monetary policy tightening). Impulse Response graphs contain 90 percent confidence bands about the impulse response function. A response is statistically significant if its computed error bands do not include zero. Figure 1 reveals that economic output reacts negatively to a rise in the short-term interest rates, with the impact immediately responsive and statistically significant for all CMA countries. For Eswatini, economic output reaches its minimum within 4 months, recovers and becomes insignificant after 10 months. The intensity of the shock is more pronounced in Namibia, with economic output declining by 0.01 percent, but quickly beginning recovery after 3 months. Lesotho's response is similar but reaches a 0.009 percent minimum within 16 months. For South Africa, economic output falls but does not show any signs of recovery within 24 months following a policy shock. These findings are in contrast to Seleteng (2016) who found that the response of economic growth in the CMA region to a positive shock in the repo rate is positive and statistically significant for one year only. However, the pattern and speed of adjustment of our results corresponds with findings from most studies in the CMA literature (Buigut (2009) in EAC; Sheefeni and Ocran (2012) in Namibia; Kabundi and Ngwenya (2011) in South Africa).
The response of inflation to a monetary policy shock shows a significant and contemporaneous change for all countries, as shown in figure 2. These results are particularly crucial, given that inflation is a primary policy target in South Africa's monetary policy regime. Following a one-time shock to the repo rate, inflation in South Africa rapidly increases by about 0.1 percent within five months, tapering off thereafter. In the LEN countries, price levels respond similar to South Africa, with the recovery much faster for Namibia. These results contradict theoretical expectations from a contractionary monetary policy. These unconventional responses have been extensively investigated in the literature and have become known as the price puzzle7 (Kim and Roubini, 2000; Leeper et al., 1996). To address price puzzles, studies such as Ncube and Ndou (2013) and Kabundi and Ngwenya (2011) have recommended including additional measures which can better reflect changes in inflation expectations, such as the U.S. federal funds rate, and business and consumer confidence measures. However, including both the U.S. federal reserve funds rate or world oil price index did not solve the price puzzle in our estimated models. Nonetheless, findings similar to ours have been reported in other studies in the CMA and other developing countries (Buigut, 2009; Ikhide and Uanguta, 2010; Gumata et al. (2013); Seleteng, 2016; Mkhonta, 2018).
7The price puzzle is described as an effect of the central bank having superior information regarding future economic conditions which leads it to raise interest rates because it expects inflation to rise in the future (Ncube and Ndou, 2013).
Figure 3 shows that the impact of monetary policy on broad money supply is asymmetric across countries in the CMA. Theoretically, money supply is expected to fall in response to contractionary monetary policy due to increased cost of borrowing. At impact, the response for South Africa is negative and insignificant for the first 4 months after impact, and become significant thereafter. Similarly, money supply in Eswatini initially falls, reaching its minimum within 7 months, followed by a modest recovery. In contrast, the initial impact on money supply is positive for Namibia and Lesotho, with the effects more persistent for Lesotho. These findings suggest that monetary policy interventions in the region could have unintended consequences on the supply of money for Lesotho and Namibia. Our studies in the literature such as Seleteng (2016) and Dlamini and Skosana (2017) found that money supply in the CMA is not responsive to shocks to the repo rate, while Ikhide and Uanguta (2010) and Gumata et al. (2013) found a negative relationship.
In the case of domestic credit, the CMA countries also display asymmetric behaviour. The response of domestic credit to a positive change in the repo rate is shown in figure 4. The impact of a rise in monetary policy on domestic credit in South Africa is negative and only becomes significant after 8 months. For Eswatini, there is a statistically significant contemporaneous response that shows a sharp decline within 4 months, followed by a rapid rebound. Lesotho and Namibia's responses are opposite, domestic credit in both countries initially increases on impact before rebounding a few months later. The results presented in figures 3 and 4 are be indicative of some of the key challenges facing policymakers in the LEN countries. The banking sector in the LEN countries is characterized by high commercial bank excess reserves. This excess liquidity renders monetary policy ineffective since banks can simply draw on these funds to continue extending credit to borrowers.
Finally, figure 5 shows the response of lending rate spread to a one percent rise in the repo rate. Lending rate spread responses to policy innovations are also asymmetric across the CMA countries. In Eswatini, the lending rate spread continues to increase by more than 0.04 percent following policy impact. For Namibia, there is a sharp increase by about 0.04 percent but declines after the second month. There is an instantaneous rise in the lending rate spread in South Africa by approximately 0.07 percent, which becomes insignificant within 12 months. In line with our findings, Ikhide and Uanguta (2010), and Seleteng (2016) found that the response of lending rates to a positive shock on the repo rate is positive and statistically significant. In South Africa Gumata et al. (2013) and Bonga-Bonga (2010) also found similar responses.
Since the identification of SVAR models is based on theoretical economic expectations, their results alone are considered inadequate. According to Maturu and Ndirangu (2010), the variance decomposition results show the relative importance of a given endogenous variable in explaining the out-of-sample forecast error in another endogenous variable of interest under analysis. For instance, FEVDs will enable us to characterize the relative importance of the repo rate in explaining the out-of-sample forecast errors in GDP. If a larger proportion of out-of-sample forecast errors in GDP are explained by the repo rate, then there is a greater possibility that the monetary policy instrument is a useful tool. In addition, it is most likely that the corresponding impulse responses will as well be statistically significant and large in magnitude. This implies that the results from variance decomposition are useful in validating the estimated impulse response function.
Appendix C presents the forecast error variance decomposition results for all variables in each CMA country. We have presented the results on a graph instead of the usual table because they are much easier to compare across countries. The shocks have been decomposed into a 24-month time horizon. The results can be summarised as follows: Firstly, apart from own shocks, the second most important variable in explaining the forecast errors in economic output during the short time horizon is the repo rate. The repo rate's importance in explaining fluctuations in real economic output increases steadily over time but differs slightly across countries. Secondly, the repo rate is the third most important variable which explains the variance errors in inflation, after itself and economic output. The importance of the repo rate generally remains steady over time, with an exception in Namibia. Lastly, inflation is more important in explaining the fluctuations in the repo rate for South Africa than in the LEN countries. These findings reveal the fact South African authorities do not consider prevalent economic situations in the LEN countries when implementing monetary policy in the CMA.
The forecast error variance decomposition results may also be useful to explain the sources of asymmetric responses observed in money supply, lending rate spread, and domestic credit. The variance decomposition of money supply shows that after a period of 12 months, shocks to the repo rate explain over 20 percent of fluctuations in Lesotho. In Eswatini, Namibia, and South Africa, this is less than 10 percent. This suggests that the money supply in Lesotho is more sensitive to changes in the repo rate compared to the other LEN countries. Shocks to money supply explain over 10 percent of fluctuations in the lending rate spread in South Africa. This is greater compared to other countries and may explain the observed initial decline in South Africa. The presence of a large informal sector may also be attributed to the observed asymmetrical monetary policy responses. Davoodi et al. (2013) argued developing countries in sub-Saharan Africa are characterized by an underdeveloped formal financial market that puts constraints on the monetary policy transmission mechanism. Future research could look further into the sources of asymmetrical shocks in order to determine proper policy interventions.
To ensure the robustness of our results, further econometric checks have to be performed on the estimated models. One of the standard tests for robustness in SVAR models is the sensitivity test. This test is performed by re-estimating the model under a different lag structure. The same method of identification discussed in section 4 was used. The number of lags is also chosen according to the lag length criterion tests. We used four lags for Eswatini, Namibia, and South Africa's economies while only two lags were used in Lesotho's model. The test results presented in Appendix D are in line with findings in section 5.1, with differences observed only in money supply and domestic credit. Contrary to prior findings where money supply responses were significant for Eswatini and Lesotho, the re-estimated model suggests that money supply in the CMA is insignificantly responsive to innovations to the repo rate at the 90 percent confidence level. The observed inconsistencies may be caused by several factors such as structural breaks, wrong selection of variables, etc. However, the fact that all other variables have consistent responses to monetary policy shocks in both models suggests that further robustness tests may be necessary.
Another test for the robustness of the econometric results is the break-point test, where the model over the whole sample period is compared with the model estimated over various sub-samples. In this case, the data series is divided into two parts; the first sub-sample is from the period 2000M2 to 2009M6, the second sub-sample is from the period 2009M7 to 2018M12. Under the same identification regime, Appendix E shows the results of the test for each country. The results show that the estimated impulse response patterns of the sub-sample are consistent with the whole sample. This suggests that the estimated results are robust to the sample size.
In addition, the VAR residuals were tested for heteroskedasticity and normality. The results presented in table 2 indicate that the null hypothesis that the residuals are multivariate normal cannot be rejected for all countries. There is no heteroskedasticity in the residuals. Lastly, table 3 shows that the null hypothesis of no correlation at lag order one cannot be rejected at the 5 percent significance level.
The main conclusion drawn from the findings in section 5 is that macroeconomic variables in the CMA are responsive to changes in the monetary policy instrument. There is also evidence of asymmetrical responses amongst member countries. Specifically, the responses of money supply, domestic credit and lending rate spread in some of the LEN countries are asymmetric to those in South Africa. This implies that during periods of economic instability, policy actions taken by the SARB may exacerbate conditions in the LEN countries, exposing them further to the risk of economic turmoil. To address the issue of asymmetric policy shocks, one possible option for the LEN countries could be to embark on independent monetary policy. However, majority of researchers agree that this option may not be viable given the unilateral dependence of the LEN countries on South Africa (Houssa, 2008; Ikhide and Uanguta, 2010; Seleteng, 2016). Alternatively, policy makers may focus their efforts in identifying the nature of shocks faced by each CMA country into supply shocks, terms of trade shocks, demand shocks, and nominal shocks. A clear understanding of nature of shocks in the CMA will enable the identification of the sources of shocks which are crucial to implement successful stabilization policies.
The objective of this paper is to examine the effect of monetary policy shocks on the Common Monetary Area. The LEN currencies are fixed to the South African rand, and capital flows between countries are tariff-free. However, the CMA cannot be characterized as a fully-fledged monetary union since monetary policy is set independently in South Africa. Interest rates in the LEN countries cannot deviate too far from those in South Africa. As a result, the South African repo rate is the relevant monetary policy instrument in the region. The effect of unexpected monetary policy shocks has remained largely unresolved, especially in developing countries. There is a limited number of studies on the monetary policy transmission mechanism in developing countries compared to industrialized countries. The current literature on monetary policy transmission mechanisms in the CMA differs in their results depending on various aspects like model specification, the econometric technique used, variable selection, and the period under study.
This study comparatively evaluates the effect of shocks to the South African repo rate on the LEN economies. Our analysis is based on a six-variable structural VAR model estimated using monthly data for the period 2000 to 2018. Due to data limitations, the study applied a Chow-Lin temporal disaggregation method to interpolate monthly economic output. Unlike previous studies in the CMA, the U.S. federal funds rate was used to control for changes due to external factors. In addition, the study focused primarily on the period following the implementation of the inflation targeting regime to better reflect the short-run dynamics of monetary policy.
Our findings suggest that an exogenous and temporary increase in the South African repo rate tends to be followed by a statistically significant decline in output and a rise in price levels for all countries in the CMA. The magnitude of the impact varies by country, with South Africa experiencing a significant decrease in output and an increase in price levels. These results seem to be stable over different sample periods. The findings also showed that the effect of monetary policy shocks on domestic credit, lending rate spread, and money supply is asymmetric across the CMA region. These findings suggest that monetary policy interventions employed in South Africa may have a negative effect on the financial sector in the LEN countries. If further policy strategies are not implemented in the LEN countries to offset these effects, economic conditions in the LEN countries may continue to worsen. The empirical literature suggests several factors that might have contributed to the observed asymmetric responses in the CMA. One of the causes might be the underdevelopment of the financial markets and, hence, the weak transmission of financial signals to commercial banks, investors, and consumers. The presence of informal markets in the CMA is also a source of concern in the transmission of monetary policy. Given the large size of informal economies, national account statistics may not fully reflect the dynamics of monetary policy. The paucity of data on the total volume of the South African currency circulating in the LEN countries may have also affected our results, particularly the response of broad money supply.
Our results suggest that it is important for the South African authorities to pay close attention to the economic conditions in the LEN countries when implementing monetary policy in order to avoid exacerbating economic instability in the LEN countries. The LEN countries are also recommended to improve measures to estimate the volume of the ZAR circulation in their respective economies through regular monetary surveys. This will provide a more accurate estimation of the dynamics of monetary policy in the LEN countries. Improving the efficacy of monetary policy in the CMA may require addressing these issues and, among others, ensuring that monetary targets and interest rate policy are consistent among all members.
This study suffers from several limitations that could be addressed in future research. Firstly, our model does not include any measures of fiscal policy and exchange rate, which may have an effect on the monetary policy shocks in the CMA. Secondly, our research focused only on monetary policy shocks. It could also be interesting to explore the effects of output shocks, demand shocks and supply shocks in the CMA. Lastly, Gumata et al. (2013) have argued that the transmission of monetary policy shocks in South Africa has changed since the 2008 global financial crisis. Although our results showed robustness to the break-point test, future research could estimate the transmission of South Africa's monetary policy shocks to the LEN countries using alternative strategies.
I would like to express my appreciation to Dr. Samia Islam, Dr. Michail Fragkias, Dr. Jingxian Hu, Dr. Leming Qu, Bahlakoana Mabetha, and Makamohelo Shiloh Seoela for their assistance and guidance during the earlier versions of the manuscript. I am also grateful to the anonymous reviewers for their insightful feedback and recommendations. Any remaining errors are my own.
The author declares no conflict of interest in this paper.
[1] |
Abdul-Rahim R, Bohari SA, Aman A, et al. (2022) Benefit–risk perceptions of FinTech adoption for sustainability from bank consumers' perspective: The moderating role of fear of COVID-19. Sustainability 14: 8357. https://doi.org/10.3390/su14148357 doi: 10.3390/su14148357
![]() |
[2] |
Aduba JJ (2021) On the determinants, gains and challenges of electronic banking adoption in Nigeria. Int J Soc Econ 48: 1021–1043. https://doi.org/10.1108/IJSE-07-2020-0452 doi: 10.1108/IJSE-07-2020-0452
![]() |
[3] |
Alaabed A, Masih M, Mirakhor A (2016) Investigating risk shifting in Islamic banks in the dual banking systems of OIC member countries: an application of two-step dynamic GMM. Risk Manage 18: 236–263. https://doi.org/10.1057/s41283-016-0007-3 doi: 10.1057/s41283-016-0007-3
![]() |
[4] |
Aracil E, Nájera-Sánchez JJ, Forcadell FJ (2021) Sustainable banking: A literature review and integrative framework. Financ Res Lett 42: 101932. https://doi.org/10.1016/j.frl.2021.101932 doi: 10.1016/j.frl.2021.101932
![]() |
[5] | Ashrafi DM, Dovash RH, Kabir MR (2022) Determinants of fintech service continuance behavior: moderating role of transaction security and trust. J Global Bus Technol 18. Available from: https://www.proquest.com/docview/2766511260?pq-origsite = gscholar & fromopenview = true & sourcetype = Scholarly%20Journals. |
[6] |
Ashta A, Herrmann H (2021) Artificial intelligence and fintech: An overview of opportunities and risks for banking, investments, and microfinance. Strateg Change 30: 211–222. https://doi.org/10.1002/jsc.2404 doi: 10.1002/jsc.2404
![]() |
[7] |
Banna H, Hassan MK, Ahmad R, et al. (2022) Islamic banking stability amidst the COVID-19 pandemic: the role of digital financial inclusion. Int J Islamic Middle 15: 310–330. https://doi.org/10.1108/IMEFM-08-2020-0389 doi: 10.1108/IMEFM-08-2020-0389
![]() |
[8] |
Boratyńska K (2019) Impact of digital transformation on value creation in Fintech services: an innovative approach. J Promot Manage 25: 631–639. https://doi.org/10.1080/10496491.2019.1585543 doi: 10.1080/10496491.2019.1585543
![]() |
[9] |
Bose S, Khan HZ, Rashid A, et al. (2018) What drives green banking disclosure? An institutional and corporate governance perspective. Asia Pac J Manage 35: 501–527. https://doi.org/10.1007/s10490-017-9528-x doi: 10.1007/s10490-017-9528-x
![]() |
[10] |
Brahmi M, Esposito L, Parziale A, et al. (2023) The role of greener innovations in promoting financial inclusion to achieve carbon neutrality: an integrative review. Economies 11: 194. https://doi.org/10.3390/economies11070194 doi: 10.3390/economies11070194
![]() |
[11] |
Çera G, Phan QPT, Androniceanu A, et al. (2020) Financial capability and technology implications for online shopping. EaM Ekon Manag. https://doi.org/10.15240/tul/001/2020-2-011 doi: 10.15240/tul/001/2020-2-011
![]() |
[12] |
Chang HY, Liang LW, Liu YL (2021) Using environmental, social, governance (ESG) and financial indicators to measure bank cost efficiency in Asia. Sustainability 13: 11139. https://doi.org/10.3390/su132011139 doi: 10.3390/su132011139
![]() |
[13] |
Coffie CPK, Zhao H, Adjei Mensah I (2020) Panel econometric analysis on mobile payment transactions and traditional banks effort toward financial accessibility in Sub-Sahara Africa. Sustainability 12: 895. https://doi.org/10.3390/su12030895 doi: 10.3390/su12030895
![]() |
[14] |
Cumming D, Johan S, Reardon R (2023) Global fintech trends and their impact on international business: a review. Multinatl Bus Rev 31: 413–436. https://doi.org/10.1108/MBR-05-2023-0077 doi: 10.1108/MBR-05-2023-0077
![]() |
[15] |
Danladi S, Prasad M, Modibbo UM, et al. (2023) Attaining Sustainable Development Goals through Financial Inclusion: Exploring Collaborative Approaches to Fintech Adoption in Developing Economies. Sustainability 15: 13039. https://doi.org/10.3390/su151713039 doi: 10.3390/su151713039
![]() |
[16] |
Davis FD, Bagozzi RP, Warshaw PR (1989) User acceptance of computer technology: A comparison of two theoretical models. Manage Sci 35: 982–1003. https://doi.org/10.1287/mnsc.35.8.982 doi: 10.1287/mnsc.35.8.982
![]() |
[17] |
Dewi IGAAO, Dewi IGAAP (2017) Corporate social responsibility, green banking, and going concern on banking company in Indonesia stock exchange. Int J Soc Sci Hum 1: 118–134. https://doi.org/10.29332/ijssh.v1n3.65 doi: 10.29332/ijssh.v1n3.65
![]() |
[18] |
Diep NTN, Canh TQ (2022) Impact analysis of peer-to-peer Fintech in Vietnam's banking industry. J Int Stud 15. https://doi.org/10.14254/2071-8330.2022/15-3/12 doi: 10.14254/2071-8330.2022/15-3/12
![]() |
[19] |
Dong Y, Chung M, Zhou C, et al. (2018) Banking on "mobile money": The implications of mobile money services on the value chain. Manuf Serv Oper Manag. https://doi.org/10.1287/msom.2018.0717 doi: 10.1287/msom.2018.0717
![]() |
[20] |
Eisingerich AB, Bell SJ (2008) Managing networks of interorganizational linkages and sustainable firm performance in business‐to‐business service contexts. J Serv Mark 22: 494–504. https://doi.org/10.1108/08876040810909631 doi: 10.1108/08876040810909631
![]() |
[21] |
Ellili NOD (2022) Is there any association between FinTech and sustainability? Evidence from bibliometric review and content analysis. J Financ Serv Mark 28: 748–762. https://doi.org/10.1057/s41264-022-00200-w doi: 10.1057/s41264-022-00200-w
![]() |
[22] |
Fenwick M, Vermeulen EP (2020) Banking and regulatory responses to FinTech revisited-building the sustainable financial service'ecosystems' of tomorrow. Singap J Legal Stud 2020: 165–189. https://doi.org/10.2139/ssrn.3446273 doi: 10.2139/ssrn.3446273
![]() |
[23] |
Gangi F, Meles A, Daniele LM, et al. (2021) Socially responsible investment (SRI): from niche to mainstream. The Evolution of Sustainable Investments and Finance: Theoretical Perspectives and New Challenges, 1–58. https://doi.org/10.1007/978-3-030-70350-9_1 doi: 10.1007/978-3-030-70350-9_1
![]() |
[24] |
Gbongli K, Xu Y, Amedjonekou KM, et al. (2020) Evaluation and classification of mobile financial services sustainability using structural equation modeling and multiple criteria decision-making methods. Sustainability 12: 1288. https://doi.org/10.3390/su12041288 doi: 10.3390/su12041288
![]() |
[25] |
Goodell JW, Kumar S, Lim WM, et al. (2021) Artificial intelligence and machine learning in finance: Identifying foundations, themes, and research clusters from bibliometric analysis. J Behav Exp Financ 32: 100577. https://doi.org/10.1016/j.jbef.2021.100577 doi: 10.1016/j.jbef.2021.100577
![]() |
[26] |
Gozman D, Willcocks L (2019) The emerging Cloud Dilemma: Balancing innovation with cross-border privacy and outsourcing regulations. J Bus Res 97: 235–256. https://doi.org/10.1016/j.jbusres.2018.06.006 doi: 10.1016/j.jbusres.2018.06.006
![]() |
[27] |
Gruin J, Knaack P (2020) Not just another shadow bank: Chinese authoritarian capitalism and the 'developmental'promise of digital financial innovation. New Polit Econ 25: 370–387. https://doi.org/10.1080/13563467.2018.1562437 doi: 10.1080/13563467.2018.1562437
![]() |
[28] |
Guang-Wen Z, Siddik AB (2023) The effect of Fintech adoption on green finance and environmental performance of banking institutions during the COVID-19 pandemic: the role of green innovation. Environ Sci Pollut Res 30: 25959–25971. https://doi.org/10.1007/s11356-022-23956-z doi: 10.1007/s11356-022-23956-z
![]() |
[29] |
Guo Y, Holland J, Kreander N (2014) An exploration of the value creation process in bank-corporate communications. J Commun manage 18: 254–270. https://doi.org/10.1108/JCOM-10-2012-0079 doi: 10.1108/JCOM-10-2012-0079
![]() |
[30] | Hassan MK, Rabbani MR, Ali MAM (2020) Challenges for the Islamic Finance and banking in post COVID era and the role of Fintech. J Econ Coop Dev 41: 93–116. Available from: https://www.proquest.com/docview/2503186452?pq-origsite = gscholar & fromopenview = true & sourcetype = Scholarly%20Journals. |
[31] | Hassan SM, Rahman Z, Paul J (2022) Consumer ethics: A review and research agenda. Psychol Market 39: 111–130. |
[32] |
He J, Zhang S (2022) How digitalized interactive platforms create new value for customers by integrating B2B and B2C models? An empirical study in China. J Bus Res 142: 694–706. https://doi.org/10.1016/j.jbusres.2022.01.004 doi: 10.1016/j.jbusres.2022.01.004
![]() |
[33] |
Hommel K, Bican PM (2020) Digital entrepreneurship in finance: Fintechs and funding decision criteria. Sustainability 12: 8035. https://doi.org/10.3390/su12198035 doi: 10.3390/su12198035
![]() |
[34] |
Hyun S (2022) Current Status and Challenges of Green Digital Finance in Korea. Green Digital Finance and Sustainable Development Goals, 243–261. https://doi.org/10.1007/978-981-19-2662-4_12 doi: 10.1007/978-981-19-2662-4_12
![]() |
[35] |
Ⅱ WWC, Demrig I (2002) Investment and capitalisation of firms in the USA. Int J Technol Manage 24: 391–418. https://doi.org/10.1504/IJTM.2002.003062 doi: 10.1504/IJTM.2002.003062
![]() |
[36] |
Iman N (2018) Is mobile payment still relevant in the fintech era? Electron Commer Res Appl 30: 72–82. https://doi.org/10.1016/j.elerap.2018.05.009 doi: 10.1016/j.elerap.2018.05.009
![]() |
[37] |
Ji F, Tia A (2022) The effect of blockchain on business intelligence efficiency of banks. Kybernetes 51: 2652–2668. https://doi.org/10.1108/K-10-2020-0668 doi: 10.1108/K-10-2020-0668
![]() |
[38] |
Jibril AB, Kwarteng MA, Botchway RK, et al. (2020) The impact of online identity theft on customers' willingness to engage in e-banking transaction in Ghana: A technology threat avoidance theory. Cogent Bus Manag 7: 1832825. https://doi.org/10.1080/23311975.2020.1832825 doi: 10.1080/23311975.2020.1832825
![]() |
[39] |
Khan A, Goodell JW, Hassan MK, et al. (2022) A bibliometric review of finance bibliometric papers. Financ Res Lett 47: 102520. https://doi.org/10.1016/j.frl.2021.102520 doi: 10.1016/j.frl.2021.102520
![]() |
[40] |
Khan HU, Sohail M, Nazir S, et al. (2023) Role of authentication factors in Fin-tech mobile transaction security. J Big Data 10: 138. https://doi.org/10.1186/s40537-023-00807-3 doi: 10.1186/s40537-023-00807-3
![]() |
[41] |
Kumar S, Lim WM, Sivarajah U, et al. (2023) Artificial intelligence and blockchain integration in business: trends from a bibliometric-content analysis. Inform Syst Front 25: 871–896. https://doi.org/10.1007/s10796-022-10279-0 doi: 10.1007/s10796-022-10279-0
![]() |
[42] |
Kumari A, Devi NC (2022) The Impact of FinTech and Blockchain Technologies on Banking and Financial Services. Technol Innov Manage Rev 12. https://doi.org/10.22215/timreview/1481 doi: 10.22215/timreview/1481
![]() |
[43] |
Lai X, Yue S, Guo C, et al. (2023) Does FinTech reduce corporate excess leverage? Evidence from China. Econ Anal Policy 77: 281–299. https://doi.org/10.1016/j.eap.2022.11.017 doi: 10.1016/j.eap.2022.11.017
![]() |
[44] |
Lee WS, Sohn SY (2017) Identifying emerging trends of financial business method patents. Sustainability 9: 1670. https://doi.org/10.3390/su9091670 doi: 10.3390/su9091670
![]() |
[45] |
Lekakos G, Vlachos P, Koritos C (2014) Green is good but is usability better? Consumer reactions to environmental initiatives in e-banking services. Ethics Inf Technol 16: 103–117. https://doi.org/10.1007/s10676-014-9337-6 doi: 10.1007/s10676-014-9337-6
![]() |
[46] | Mądra-Sawicka M (2020) Financial management in the big data era. In Management in the Era of Big Data, 71–81, Auerbach Publications. https://doi.org/10.1201/9781003057291-6 |
[47] |
Mejia-Escobar JC, González-Ruiz JD, Duque-Grisales E (2020) Sustainable financial products in the Latin America banking industry: Current status and insights. Sustainability 12: 5648. https://doi.org/10.3390/su12145648 doi: 10.3390/su12145648
![]() |
[48] | Mhlanga D (2023) FinTech for Sustainable Development in Emerging Markets with Case Studies. In FinTech and Artificial Intelligence for Sustainable Development: The Role of Smart Technologies in Achieving Development Goals, 337–363, Springer. https://doi.org/10.1007/978-3-031-37776-1_15 |
[49] |
Mohr I, Fuxman L, Mahmoud AB (2022) A triple-trickle theory for sustainable fashion adoption: the rise of a luxury trend. J Fash Mark Manag Int J 26: 640–660. https://doi.org/10.1108/JFMM-03-2021-0060 doi: 10.1108/JFMM-03-2021-0060
![]() |
[50] |
Muniz Jr AM, Schau HJ (2005) Religiosity in the abandoned Apple Newton brand community. J Consum Res 31: 737–747. https://doi.org/10.1086/426607 doi: 10.1086/426607
![]() |
[51] |
Naruetharadhol P, Ketkaew C, Hongkanchanapong N, et al. (2021) Factors affecting sustainable intention to use mobile banking services. Sage Open 11: 21582440211029925. https://doi.org/10.1177/21582440211029925 doi: 10.1177/21582440211029925
![]() |
[52] |
Nenavath S (2022) Impact of fintech and green finance on environmental quality protection in India: By applying the semi-parametric difference-in-differences (SDID). Renew Energ 193: 913–919. https://doi.org/10.1016/j.renene.2022.05.020 doi: 10.1016/j.renene.2022.05.020
![]() |
[53] |
Nosratabadi S, Pinter G, Mosavi A, et al. (2020) Sustainable banking; evaluation of the European business models. Sustainability 12: 2314. https://doi.org/10.3390/su12062314 doi: 10.3390/su12062314
![]() |
[54] |
Ortas E, Burritt RL, Moneva JM (2013) Socially Responsible Investment and cleaner production in the Asia Pacific: does it pay to be good? J Cleaner Prod 52: 272–280. https://doi.org/10.1016/j.jclepro.2013.02.024 doi: 10.1016/j.jclepro.2013.02.024
![]() |
[55] |
Oseni UA, Adewale AA, Omoola SO (2018) The feasibility of online dispute resolution in the Islamic banking industry in Malaysia: An empirical legal analysis. Int J Law Manag 60: 34–54. https://doi.org/10.1108/IJLMA-06-2016-0057 doi: 10.1108/IJLMA-06-2016-0057
![]() |
[56] |
Paiva BM, Ferreira FA, Carayannis EG, et al. (2021) Strategizing sustainability in the banking industry using fuzzy cognitive maps and system dynamics. Int J Sustain Dev World Ecol 28: 93–108. https://doi.org/10.1080/13504509.2020.1782284 doi: 10.1080/13504509.2020.1782284
![]() |
[57] |
Parmentola A, Petrillo A, Tutore I, et al. (2022) Is blockchain able to enhance environmental sustainability? A systematic review and research agenda from the perspective of Sustainable Development Goals (SDGs). Bus Strateg Environ 31: 194–217. https://doi.org/10.1002/bse.2882 doi: 10.1002/bse.2882
![]() |
[58] |
Paul J, Lim WM, O'Cass A, et al. (2021) Scientific procedures and rationales for systematic literature reviews (SPAR‐4‐SLR). Int J Consum Stud 45: O1–O16. https://doi.org/10.1111/ijcs.12695 doi: 10.1111/ijcs.12695
![]() |
[59] |
Puschmann T, Hoffmann CH, Khmarskyi V (2020) How green FinTech can alleviate the impact of climate change—the case of Switzerland. Sustainability 12: 10691. https://doi.org/10.3390/su122410691 doi: 10.3390/su122410691
![]() |
[60] |
Rahman S, Moral IH, Hassan M, et al. (2022) A systematic review of green finance in the banking industry: perspectives from a developing country. Green Financ 4: 347–363. https://doi.org/10.3934/GF.2022017 doi: 10.3934/GF.2022017
![]() |
[61] |
Ryu HS, Ko KS (2020) Sustainable development of Fintech: Focused on uncertainty and perceived quality issues. Sustainability 12: 7669. https://doi.org/10.3390/su12187669 doi: 10.3390/su12187669
![]() |
[62] |
Sagnier C, Loup-Escande E, Lourdeaux D, et al. (2020) User acceptance of virtual reality: an extended technology acceptance model. Int J Hum–Comput Interact 36: 993–1007. https://doi.org/10.1080/10447318.2019.1708612 doi: 10.1080/10447318.2019.1708612
![]() |
[63] |
Sethi P, Chakrabarti D, Bhattacharjee S (2020) Globalization, financial development and economic growth: Perils on the environmental sustainability of an emerging economy. J Policy Model 42: 520–535. https://doi.org/10.1016/j.jpolmod.2020.01.007 doi: 10.1016/j.jpolmod.2020.01.007
![]() |
[64] |
Singh RK, Mishra R, Gupta S, et al. (2023) Blockchain applications for secured and resilient supply chains: A systematic literature review and future research agenda. Comput Ind Eng 175: 108854. https://doi.org/10.1016/j.cie.2022.108854 doi: 10.1016/j.cie.2022.108854
![]() |
[65] |
Sun Y, Luo B, Wang S, et al. (2021) What you see is meaningful: Does green advertising change the intentions of consumers to purchase eco‐labeled products? Bus Strateg Environ 30: 694–704. https://doi.org/10.1002/bse.2648 doi: 10.1002/bse.2648
![]() |
[66] |
Talom FSG, Tengeh RK (2019) The impact of mobile money on the financial performance of the SMEs in Douala, Cameroon. Sustainability 12: 183. https://doi.org/10.3390/su12010183 doi: 10.3390/su12010183
![]() |
[67] |
Taneja S, Siraj A, Ali L, et al. (2023) Is fintech implementation a strategic step for sustainability in today's changing landscape? An empirical investigation. IEEE T Eng Manage. https://doi.org/10.3390/su12010183 doi: 10.3390/su12010183
![]() |
[68] |
Tara K, Singh S, Kumar R, et al. (2019) Geographical locations of banks as an influencer for green banking adoption. Prabandhan: Indian J Manag 12: 21–35. https://doi.org/10.17010/pijom/2019/v12i1/141425 doi: 10.17010/pijom/2019/v12i1/141425
![]() |
[69] |
Tchamyou VS, Erreygers G, Cassimon D (2019) Inequality, ICT and financial access in Africa. Technol Forecast Soc Change 139: 169–184. https://doi.org/10.1016/j.techfore.2018.11.004 doi: 10.1016/j.techfore.2018.11.004
![]() |
[70] |
Tripathi R (2023) Framework of green finance to attain sustainable development goals: an empirical evidence from the TCCM approach. Benchmarking. https://doi.org/10.1108/BIJ-05-2023-0311 doi: 10.1108/BIJ-05-2023-0311
![]() |
[71] |
Truby J, Brown R, Dahdal A (2020) Banking on AI: mandating a proactive approach to AI regulation in the financial sector. Law Financ Mark Rev 14: 110–120. https://doi.org/10.1080/17521440.2020.1760454 doi: 10.1080/17521440.2020.1760454
![]() |
[72] |
Tsindeliani IA, Proshunin MM, Sadovskaya TD, et al. (2022) Digital transformation of the banking system in the context of sustainable development. J Money Laund Contro 25: 165–180. https://doi.org/10.1108/JMLC-02-2021-0011 doi: 10.1108/JMLC-02-2021-0011
![]() |
[73] |
Ullah A, Pinglu C, Ullah S, et al. (2023) Impact of intellectual capital efficiency on financial stability in banks: Insights from an emerging economy. Int J Financ Econ 28: 1858–1871. https://doi.org/10.1002/ijfe.2512 doi: 10.1002/ijfe.2512
![]() |
[74] |
Yadav MS (2010) The decline of conceptual articles and implications for knowledge development. J Mark 74: 1–19. https://doi.org/10.1509/jmkg.74.1.1 doi: 10.1509/jmkg.74.1.1
![]() |
[75] |
Yan C, Siddik AB, Yong L, et al. (2022) A two-staged SEM-artificial neural network approach to analyze the impact of FinTech adoption on the sustainability performance of banking firms: The mediating effect of green finance and innovation. Systems 10: 148. https://doi.org/10.3390/systems10050148 doi: 10.3390/systems10050148
![]() |
[76] |
Yang C, Masron TA (2022) Impact of digital finance on energy efficiency in the context of green sustainable development. Sustainability 14: 11250. https://doi.org/10.3390/su14181125 doi: 10.3390/su14181125
![]() |
[77] |
Yigitcanlar T, Cugurullo F (2020) The sustainability of artificial intelligence: An urbanistic viewpoint from the lens of smart and sustainable cities. Sustainability 12: 8548. https://doi.org/10.3390/su1220854 doi: 10.3390/su1220854
![]() |
[78] |
Zhang Y (2023) Impact of green finance and environmental protection on green economic recovery in South Asian economies: mediating role of FinTech. Econ Chang Restruct 56: 2069–2086. https://doi.org/10.1007/s10644-023-09500-0 doi: 10.1007/s10644-023-09500-0
![]() |
[79] | Zhao D, Strotmann A (2015) Analysis and visualization of citation networks. Morgan & Claypool Publishers. |
[80] |
Zhao Q, Tsai PH, Wang JL (2019) Improving financial service innovation strategies for enhancing china's banking industry competitive advantage during the fintech revolution: A Hybrid MCDM model. Sustainability 11: 1419. https://doi.org/10.3390/su11051419 doi: 10.3390/su11051419
![]() |
[81] |
Zuo L, Strauss J, Zuo L (2021) The digitalization transformation of commercial banks and its impact on sustainable efficiency improvements through investment in science and technology. Sustainability 13: 11028. https://doi.org/10.3390/su131911028 doi: 10.3390/su131911028
![]() |
1. | Tapiwa Victor Warikandwa, 2023, Chapter 10, 978-3-031-23862-8, 207, 10.1007/978-3-031-23863-5_10 | |
2. | Yixiang Li, Fusheng Wang, The corporate path to green innovation: does the digital economy matter?, 2023, 30, 1614-7499, 79149, 10.1007/s11356-023-27818-0 | |
3. | Teboho Mashao, Ireen Choga, The asymmetric effect of the stabilisation policies on the general price level in the Southern African Common Monetary Area, 2024, 0972-5792, 10.1007/s40847-024-00383-z | |
4. | Usman Adamu Bello, Auwal Isah, Does difference in monetary policy framework matter for interest rate Pass-through? Evidence from TVP-VAR with stochastic volatility, 2025, 25, 13030701, 100201, 10.1016/j.cbrev.2025.100201 |