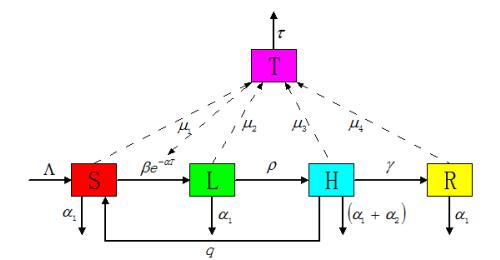
Citation: Xiaoke Li, Fuhong Yan, Jun Ma, Zhenzhong Chen, Xiaoyu Wen, Yang Cao. RBF and NSGA-II based EDM process parameters optimization with multiple constraints[J]. Mathematical Biosciences and Engineering, 2019, 16(5): 5788-5803. doi: 10.3934/mbe.2019289
[1] | Baojun Song, Wen Du, Jie Lou . Different types of backward bifurcations due to density-dependent treatments. Mathematical Biosciences and Engineering, 2013, 10(5&6): 1651-1668. doi: 10.3934/mbe.2013.10.1651 |
[2] | Jiaxin Nan, Wanbiao Ma . Stability and persistence analysis of a microorganism flocculation model with infinite delay. Mathematical Biosciences and Engineering, 2023, 20(6): 10815-10827. doi: 10.3934/mbe.2023480 |
[3] | Longxing Qi, Shoujing Tian, Jing-an Cui, Tianping Wang . Multiple infection leads to backward bifurcation for a schistosomiasis model. Mathematical Biosciences and Engineering, 2019, 16(2): 701-712. doi: 10.3934/mbe.2019033 |
[4] | Kazeem Oare Okosun, Robert Smith? . Optimal control analysis of malaria-schistosomiasis co-infection dynamics. Mathematical Biosciences and Engineering, 2017, 14(2): 377-405. doi: 10.3934/mbe.2017024 |
[5] | Guangming Qiu, Zhizhong Yang, Bo Deng . Backward bifurcation of a plant virus dynamics model with nonlinear continuous and impulsive control. Mathematical Biosciences and Engineering, 2024, 21(3): 4056-4084. doi: 10.3934/mbe.2024179 |
[6] | Zehan Liu, Daoxin Qiu, Shengqiang Liu . A two-group epidemic model with heterogeneity in cognitive effects. Mathematical Biosciences and Engineering, 2025, 22(5): 1109-1139. doi: 10.3934/mbe.2025040 |
[7] | Marek Bodnar, Monika Joanna Piotrowska, Urszula Foryś . Gompertz model with delays and treatment: Mathematical analysis. Mathematical Biosciences and Engineering, 2013, 10(3): 551-563. doi: 10.3934/mbe.2013.10.551 |
[8] | Kasia A. Pawelek, Anne Oeldorf-Hirsch, Libin Rong . Modeling the impact of twitter on influenza epidemics. Mathematical Biosciences and Engineering, 2014, 11(6): 1337-1356. doi: 10.3934/mbe.2014.11.1337 |
[9] | Ya-Dong Zhang, Hai-Feng Huo, Hong Xiang . Dynamics of tuberculosis with fast and slow progression and media coverage. Mathematical Biosciences and Engineering, 2019, 16(3): 1150-1170. doi: 10.3934/mbe.2019055 |
[10] | Haifeng Zhang, Jinzhi Lei . Optimal treatment strategy of cancers with intratumor heterogeneity. Mathematical Biosciences and Engineering, 2022, 19(12): 13337-13373. doi: 10.3934/mbe.2022625 |
The harmful use of alcohol causes a large disease, social and economic burden in societies. In 2012, about 3.3 million deaths, or 5.9% of all global deaths, were attributable to alcohol consumption. Alcohol consumption can have an impact not only on the incidence of diseases, injuries and other health conditions, but also on the course of disorders and their outcomes in individuals [1]. According to a research report by the Shanghai Institute of Environmental Economics, the number of patients due to alcoholism has increased by 28.5 times, and the number of deaths has increased by 30.6 times in the past seven years [2]. Thus, it is very important to study drinking behavior.
Recently, many authors have studied mathematical models of drinking [3,4,5,6,7,8,9]. Bani et al. [3] studied the influence of environmental factors on college alcohol drinking patterns. Mulone et al. [4] developed a two-stage (four compartments) model for youths with serious drinking problems and their treatment, and the stability of all the equilibria was obtained. Mushayabasa et al. [5] formulated a deterministic model for evaluating the impact of heavy alcohol drinking on the reemerging gonorrhea epidemic. Lee et al. [6] studied the optimal control intervention strategies in low- and high-risk problem drinking populations. Mubayi et al. [7] studied the impact of relative residence times on the distribution of heavy drinkers in highly distinct environments and found that alcohol consumption is a function of social dynamics, environmental contexts, individuals' preferences and family history. Huo, Chen and Xiang [8] introduced a more realistic binge drinking model with time delay, in which time delay is used to represent the time lag of the immunity against drinking. Xiang, Liu and Huo [9] proposed a new SAIRS alcoholism model with birth and death on complex heterogeneous networks.
Media coverage is one of the effective ways to control alcoholism or infectious diseases, many authors have studied alcoholism or epidemic models with media coverage [10,11,12,13,14]. Cui et al. [10] developed a three dimensional compartmental model to investigate the impact of media coverage to the spread and control of infectious diseases. Pawelek et al. [11] studied the impact of twitter on influenza epidemics. Huo and Zhang [12] introduced a more realistic mathematical influenza model including dynamics of Twitter, which might reduce and increase the spread of influenza. Huo and Zhang [13] formulated a novel alcoholism model which involved impact of Twitter and investigated the occurrence of backward, forward bifurcation and Hopf bifurcation. Huo and Yang [14] introduced a novel SEIS epidemic model with the impact of media. Above results show that media coverage can regard as a good indicator in controlling the emergence and spread of the epidemic disease or alcoholism. Many scholars have done a lot of researches on drinking or infectious diseases with or without media coverage [15,16,17,18,19,20,21,22].
Alcoholism can be defined as a pattern of alcohol use that compromises the health and safety of oneself and others. There are a variety of treatment methods currently available, such as behavioral treatments, medications and mutual-support Groups [23]. The goal of a person pursuing treatment is to abstain from alcohol or to cut back on drinking. Many people have studied the epidemic or alcoholism models with treatment [24,25,26].
Motivated by the above [13,14], we set up a new alcoholism model with treatment and effect of Twitter in this paper. We derive the basic reproductive number of the model and study the stability of our model. Furthermore, we investigate the occurrence of backward and forward bifurcation.
The organization of this paper is as follows: In Section 2, we present a new alcoholism model with treatment and effect of Twitter. In Section 3, we derive the basic reproductive number and study the stability of all equilibria. We also study the occurrence of backward and forward bifurcation. In Section 4, we perform some numerical simulations to illustrate and extend our main results. Sensitivity analysis and some discussion are given in last section.
The total population in this model is divided into four compartments: S(t), L(t), H(t), R(t). S(t) represents the number of moderate drinkers, that is, the people who do not drink or drink within daily and weekly limits [13]. L(t) represents the number of light problem drinkers, that is, the drinkers who drink beyond daily or weekly ceiling [13]. H(t) represents the number of heavy problem drinkers, that is, the drinkers who drink far more than daily and weekly limits [13]. R(t) represents the number of quitting drinkers, that is, the people who quit problem drinking by treatment permanently. T(t) represents the number of messages that Twitter provide about alcoholism at time t. The total number of population at time t is given by
N(t)=S(t)+L(t)+H(t)+R(t). |
The population flowing among those compartments is shown in the following diagram (Figure 1).
The diagram leads to the following system of ordinary differential equations:
{dSdt=Λ+qH−βSHe−αT−α1S,dLdt=βSHe−αT−ρL−α1L,dHdt=ρL−γH−qH−(α1+α2)H,dRdt=γH−α1R,dTdt=μ1S+μ2L+μ3H+μ4R−τT. | (2.1) |
Where all the parameters are positive constants and Λ is the recruitment rate of the population. α1 is the natural death rate. α2 is the alcoholism-related death rate. β is the rate of transmission between moderate drinkers and heavy problem drinkers, and it is reduced by a factor e−αT due to the behavior change of the public after reading information about alcoholism. α is the coefficient that determines how effective the drinking information can reduce the transmission rate. τ is outdated-rate of tweets. ρ is the transmission rate from the light problem drinkers to the heavy problem drinkers. After treatment, the transfer rate of the heavy problem drinkers to the moderate drinkers is q, the transfer rate of the heavy problem drinkers to the quitting drinkers is γ. μi(i=1,2,3,4) are the rates that moderate drinkers, light problem drinkers, heavy problem drinkers and quitting drinkers may tweet about alcoholism during an alcoholism occasion, respectively.
Adding the first four equations of system (2.1), we have
dNdt=dSdt+dLdt+dHdt+dRdt=Λ−α1N−α2H≤Λ−α1N. |
Then it follows that limt→∞supN(t)≤Λα1.
According to the fifth equation of system (2.1), we obtain
dTdt=μ1S+μ2L+μ3H+μ4R−τT≤Λ(μ1+μ2+μ3+μ4)α1−τT, |
then it follows that limt→∞supT(t)≤Λ(μ1+μ2+μ3+μ4)α1τ, so the set is
Ω={(S,L,H,R,T)∈R5+:0≤S,L,H,R≤N≤Λα1,0≤T≤Λ(μ1+μ2+μ3+μ4)α1τ}. | (2.2) |
Therefore, we will consider the global stability of system (2.1) on the set Ω.
It is easy to see that system (2.1) always has a alcohol free equilibrium P0=(S0,L0,H0,R0,T0), where
S0=Λα1,L0=0,H0=0,R0=0,T0=Λμ1α1τ. |
By applying the method of the next generation matrix in [27], we obtain the basic reproduction number R0. System (2.1) can be written as
dxdt=F(x)−V(x), |
where x=(L,S,H,R,T)T,
F(x)=(βSHe−αT0000),and V(x)=(ρL+α1L−Λ−qH+βSHe−αT+α1S−ρL+qH+γH+(α1+α2)H−γH+α1R−μ1S−μ2L−μ3H−μ4R+τT). |
The Jacobian matrices of F(x) and V(x) at the alcohol free equilibrium P0 are
DF(P0)=(00Λβα1e−αμ1Λα1τ0000000000000000000000), |
and
DV(P0)=(ρ+α100000α1Λβα1e−αμ1Λα1τ−q00−ρ0α1+α2+q+γ0000−γα10−μ2−μ1−μ3−μ4τ). |
Therefore, the basic reproduction number R0 is
R0=Λβρe−αμ1Λα1τα1(α1+ρ)(α1+α2+q+γ). | (3.1) |
Theorem 1. When R0<1 and T(t)≥Λμ1α1τ, the alcohol free equilibrium P0 of system (2.1) is globally asymptotically stable; When R0<1 and T(t)<Λμ1α1τ, the alcohol free equilibrium P0 of system (2.1) is locally asymptotically stable; When R0>1, the alcohol free equilibrium P0 of system (2.1) is unstable.
Proof. The characteristic equation of the system (2.1) at the alcohol free equilibrium P0 is
|λ+α10Λβα1e−αμ1Λα1τ−q000λ+(α1+ρ)−Λβα1e−αμ1Λα1τ000−ρλ+(α1+α2+q+γ)0000−γλ+α10−μ1−μ2−μ3−μ4λ+τ|=0. | (3.2) |
Therefore, Eq.(3.2) can be written as
(λ+τ)(λ+α1)2[(λ+(α1+ρ))(λ+(α1+α2+q+γ))−Λβρα1e−αμ1Λα1τ]=0. | (3.3) |
Therefore, the three eigenvalues of the Eq.(3.2) are λ1=−τ, λ2=−α1, λ3=−α1, and the other eigenvalues are determined by the equation
(λ+(α1+ρ))(λ+(α1+α2+q+γ))−Λβρα1e−αμ1Λα1τ=0. | (3.4) |
Therefore, the Eq.(3.4) can be written as
λ2+λ(2α1+α2+q+γ+ρ)+(α1+ρ)(α1+α2+q+γ)(1−R0)=0. | (3.5) |
By Viete theorem, we have
λ4+λ5=−(2α1+α2+q+γ+ρ)<0, |
and
λ4λ5=(α1+ρ)(α1+α2+q+γ)(1−R0). |
Thus, when R0<1, the alcohol free equilibrium P0 is locally asymptotically stable; when R0>1, the alcohol free equilibrium P0 is unstable.
Define the Lyapunov function
M(S,L,H,R,T)=ρL(t)+(α1+ρ)H(t). |
It is clear that M(t)≥0 and the equality holds if and only if L(t)=H(t)=0. Differentiating M(S,L,H,R,T) and from the Eq.(2.2), we obtain S(t)≤Λα1. Therefore, when T(t)≥Λμ1α1τ, we have
dM(S,L,H,R,T)dt=ρdL(t)dt+(α1+ρ)dH(t)dt=ρ(βSHe−αT−(α1+ρ)L)+(α1+ρ)(ρL−(α1+α2+γ+q)H)=[ρβSe−αT−(α1+ρ)(α1+α2+γ+q)]H≤[Λβρα1e−αμ1Λα1τ−(α1+ρ)(α1+α2+γ+q)]H=(α1+ρ)(α1+α2+γ+q)H[Λβρe−αμ1Λα1τα1(α1+ρ)(α1+α2+γ+q)−1]=(α1+ρ)(α1+α2+γ+q)H[R0−1]. | (3.6) |
It follows that M(S,L,H,R,T) is bounded and non-increasing. Thus, limt→∞M(S,L,H,R,T) exists. Note that R0<1 guarantees that dM(S,L,H,R,T)dt≤0 for all t≥0. Consequently, for system (2.1) there holds
limt→∞L(t)=0,limt→∞H(t)=0. |
Hence, by LaSalle's Invariance Principle [28], the alcohol free equilibrium is globally attractive. We show that the alcohol free equilibrium P0 is globally asymptotic stability when R0<1.
Theorem 2. (Ⅰ) When θ=0 and R0>1, the system (2.1) has a unique positive alcoholism equilibrium P∗0;
(Ⅱ)When θ≠0 and R0>max{R01,1}, the system (2.1) has a unique positive alcoholism equilibrium P∗1;
(Ⅲ)When R02=R0<min{R01,1}, the system (2.1) has a unique positive alcoholism equilibrium P∗2;
(Ⅳ)When R02<R0<min{R01,1}, the system (2.1) has two different positive alcoholism equilibria P∗3 and P∗4.
Proof. Assuming the right-hand sides of system (2.1) is 0, we have
{Λ+qH−βSHe−αT−α1S=0,βSHe−αT−ρL−α1L=0,ρL−γH−qH−(α1+α2)H=0,γH−α1R=0,μ1S+μ2L+μ3H+μ4R−τT=0. | (3.7) |
Let (S,L,H,R,T)=(S∗,L∗,H∗,R∗,T∗) be the solution of Eq.(3.7), we have
{Λ+qH∗−βS∗H∗e−αT∗−α1S∗=0,βS∗H∗e−αT∗−ρL∗−α1L∗=0,ρL∗−γH∗−qH∗−(α1+α2)H∗=0,γH∗−α1R∗=0,μ1S∗+μ2L∗+μ3H∗+μ4R∗−τT∗=0. | (3.8) |
By Eq.(3.8), we obtain
S∗=Λα1+[ρq−(α1+ρ)(α1+α2+γ+q)]H∗α1ρ, | (3.9) |
L∗=(α1+α2+γ+q)H∗ρ, | (3.10) |
R∗=γH∗α1, | (3.11) |
T∗=Λμ1α1τ+H∗α1ρτ[μ1qρ−μ1(α1+ρ)(α1+α2+q+γ)+α1μ2(α1+α2+q+γ)+α1μ3ρ+γμ4ρ]. | (3.12) |
Combine the above Eqs.(3.9)-(3.12) and the first equation of Eq.(3.8), we have
[1−θH∗R01]R0=e−θH∗, | (3.13) |
where
R01=Λρθ(α1+ρ)(α1+α2+q+γ)−ρq, | (3.14) |
and
θ=−α[μ1qρ−μ1(α1+ρ)(α1+α2+q+γ)+α1μ2(α1+α2+q+γ)+α1μ3ρ+γμ4ρ]α1ρτ. | (3.15) |
For the sake of simplicity, we define
R02=R01e1−R01. | (3.16) |
In what follows, we assume
F(H∗)=R0−R0R01θH∗−e−θH∗. | (3.17) |
Thus
F′(H∗)=θe−θH∗−R0R01θ, | (3.18) |
F″(H∗)=−θ2e−θH∗. | (3.19) |
The following work is to discuss the properties of Eq.(3.17).
(Ⅰ) When θ=0 and R0>1, the existence of the unique alcoholism equilibrium P∗0 of system (2.1) can be obtained by Eq.(3.13), as shown in line L4 of Figure 2, where
H∗0=Λρ(α1+ρ)(α1+α2+q+γ)−ρq(1−1R0),S∗0=Λα1−Λα1(1−1R0),L∗0=Λ(α1+α2+q+γ)(α1+ρ)(α1+α2+q+γ)−ρq(1−1R0),R∗0=Λγρα1[(α1+ρ)(α1+α2+q+γ)−ρq](1−1R0),T∗0=Λμ1α1τ+Λα1τ[(α1+ρ)(α1+α2+q+γ)−ρq][μ1qρ+α1μ3ρ+γμ4ρ+α1μ2(α1+α2+q+γ)−μ1(α1+ρ)(α1+α2+q+γ)](1−1R0). |
(Ⅱ) When θ≠0 and R0>1, we have F(0)=R0−1>0 and F(∞)=−∞<0. Assume that F′(H∗)<θ(1−R0R01). Thus, when θ>0 and R0>R01 or θ<0, we obtain F′(H∗)<0. Therefore, there is a unique positive solution for Eq.(3.17). Thus, the alcoholism equilibrium P∗1=(S∗1,L∗1,H∗1,R∗1,T∗1) can be obtained, as shown in regions ΩA and ΩB of Figure 2.
(Ⅲ) When θ>0 and R0<1, we have F(0)=R0−1<0, F(∞)=−∞<0 and F″(H∗)<0. Assume that F′(H∗)=θe−θH∗−R0R01θ=θ(e−θH∗−R0R01). If F′(H∗)=0, Eq.(3.17) has the unique positive solution H∗2=1θln(R01R0) when R0<R01. Meanwhile, we also have
F(H∗2)=R0−R0R01θH∗2−e−θH∗2=0. |
Therefore, we obtain R0=R02=R01e(1−R01). Thus, the alcoholism equilibrium P∗2=(S∗2,L∗2,H∗2,R∗2,T∗2) can be obtained, as shown in line L2 of Figure 2.
(Ⅳ) When R02<R0<1, we have F(H∗2)>0. Eq.(3.17) has two different positive solutions H∗3 and H∗4, where H∗3 and H∗4 satisfy the following condition H∗3<H∗2<H∗4. Thus, the alcoholism equilibria P∗3=(S∗3,L∗3,H∗3,R∗3,T∗3) and P∗4=(S∗4,L∗4,H∗4,R∗4,T∗4) can be obtained, as shown in region ΩE of Figure 2.
Remark 1. For simplicity, the six curves (Li,i=1,2,3,4,5,6) divide the space in which R0 and θ are located into seven regions as shown in Figure 2.
L1:R0=R01(θ),withR0>1,L2:R0=R01(θ)e1−R01(θ),withR0<min{R01(θ),1},L3:θ=0,withR0<1,L4:θ=0,withR0>1,L5:R0=R01(θ)e1−R01(θ),withR01(θ)<R0<1,L6:R0=1. |
In this section, we study the local stability of the alcoholism equilibria P∗i(i=0,1,2,3,4). First we obtain the characteristic matrix of system (2.1) at the alcoholism equilibria P∗i(i=0,1,2,3,4), as follows
|λ+βH∗ie−αT∗i+α10βS∗ie−αT∗i−q0−αβS∗iH∗ie−αT∗i−βH∗ie−αT∗iλ+(α1+ρ)−βS∗ie−αT∗i0αβS∗iH∗ie−αT∗i0−ρλ+(α1+α2+q+γ)0000−γλ+α10−μ1−μ2−μ3−μ4λ+τ|=0. | (3.20) |
In order to simplify Eq.(3.20), we have
Φ=βe−αT∗i=α1(α1+ρ)(α1+α2+γ+q)e−αT∗iΛρe−Λαμ1α1τR0=α1(α1+ρ)(α1+α2+γ+q)eθH∗iΛρR0, |
ΦS∗i=(α1+ρ)(α1+α2+γ+q)ρ=ΛθR01+q. |
Then the characteristic equation can be rewritten as:
(λ+α1)G(λ)=0, | (3.21) |
G(λ)=λ4+a1(H∗i)λ3+a2(H∗i)λ2+a3(H∗i)λ+a4(H∗i)=0, | (3.22) |
where
a1(H∗i)=3α1+α2+q+γ+ρ+τ+H∗iΦ, | (3.23) |
a2(H∗i)=(2α1+α2+q+γ+ρ+τ)(α1+H∗iΦ)+(2α1+α2+q+γ+ρ)τ+αH∗i(ΛθR01+q)(μ2−μ1), | (3.24) |
a3(H∗i)=(2α1+α2+q+γ+ρ)(α1+H∗iΦ)τ+(α1+α2+q+γ)α1H∗iΦ+(α1+α2+γ)ρH∗iΦ+αH∗i(ΛθR01+q)[(2α1+α2+q+γ)(μ2−μ1)+ρ(μ3−μ1)], | (3.25) |
a4(H∗i)=τ(α1+ρ)(α1+α2+q+γ)[(α1+H∗iΦ)−α1(1+H∗iθ)]. | (3.26) |
Theorem 3. For system (2.1), we assume that μ1=μ2=μ3=μ4.
(Ⅰ) When θ=0,α2=0 and R0>1(i.e.,L4), the alcoholism equilibrium P∗0 is locally asymptotically stable;
(Ⅱ)When θ≠0, R0>max{1,R01}(i.e.,ΩA, and ΩB), Φ>α1θ and τ>ρ, the alcoholism equilibrium P∗1 is locally asymptotically stable;
(Ⅲ)When R02=R0<min{1,R01}(i.e.,L2), the alcoholism equilibrium P∗2 may be locally stable or not;
(Ⅳ)(ⅰ)When R02<R0<min{1,R01}(i.e.,ΩE), the alcoholism equilibrium P∗3 is unstable,
(ⅱ)When R02<R0<min{1,R01}(i.e.,ΩE) and τ>ρ, the alcoholism equilibrium P∗4 is locally asymptotically stable.
Proof. (Ⅰ)When θ=0, applying to the proof of (Ⅰ) of Theorem 2. We linearize the system (2.1) and evaluate the characteristic equation at the alcoholism equilibrium P∗0, and get
|λ+βH∗0e−αT∗0+α10βS∗0e−αT∗0−q0−αβS∗0H∗0e−αT∗0−βH∗0e−αT∗0λ+(α1+ρ)−βS∗0e−αT∗00αβS∗0H∗0e−αT∗00−ρλ+(α1+q+γ)0000−γλ+α10−μ1−μ1−μ1−μ1λ+τ|=0. |
Thus, the characteristic equation can be rewritten as:
(λ+α1)(λ+τ)G1(λ)=0, |
G1(λ)=λ3+b1λ2+b2λ+b3=0, |
where
b1=3α1+q+γ+ρ+H∗0Φ,b2=(2α1+q+γ+ρ)(α1+H∗0Φ),b3=[(α1+ρ)(α1+γ)+α1q]H∗0Φ. |
It is clear that b1>0, b2>0 and b3>0. Applying Routh−Hurwitz [13], by assuming that B=b1b2−b3. Then, we obtain
B=(2α1+q+γ+ρ)(H∗0Φ)2+(7α12+5α1q+5α1γ+5α1ρ+q2+2qγ+2qρ+γ2+γρ+ρ2)(H∗0Φ)+6α13+5α12q+5α12γ+5α12ρ+α1q2+2α1qγ+2α1qρ+α1γ2+2α1γρ+α1ρ2>0 |
Hence, the alcoholism equilibrium P∗0 is locally asymptotically stable.
(Ⅱ)When μ1=μ2=μ3=μ4 and Φ>α1θ, by Eqs.(3.23)-(3.26), we have
a1(H∗1)=3α1+α2+q+γ+ρ+τ+H∗1Φ>0,a2(H∗1)=(2α1+α2+q+γ+ρ+τ)(α1+H∗1Φ)+(2α1+α2+q+γ+ρ)τ>0,a3(H∗1)=(2α1+α2+q+γ+ρ)(α1+H∗1Φ)τ+(α1+α2+q+γ)α1H∗1Φ+(α1+α2+γ)ρH∗1Φ>0,a4(H∗1)=τ(α1+ρ)(α1+α2+q+γ)[(α1+H∗1Φ)−α1(1+H∗1θ)]>0. |
Applying Routh−Hurwitz [13], let C=a1a2−a3. Then, we obtain
C=c1H∗12+c2H∗1+c3>0, |
where
c1=2Φ2α1+Φ2α2+Φ2γ+Φ2q+Φ2ρ+Φ2τ>0,c2=7Φα12+Φα22+Φγ2+Φq2+Φρ2+Φτ2+5Φα1α2+5Φα1γ+2Φα2γ+5Φα1q+2Φα2q+5Φα1ρ+Φα2ρ+6Φα1τ+2Φqρ+2Φqτ+2Φρτ+Φγρ+2Φγq+2Φα2τ+2Φγτ>0,c3=α1α22+5α12α2+α1γ2+5α12γ+α1q2+5α12q+α1ρ2+5α12ρ+9α12τ+α2τ2+α22τ+γτ2+γ2τ+qτ2+q2τ+ρτ2+ρ2τ+2α1α2q+2α1α2ρ+6α1α2τ+2α1γq+2α1γρ+6α1γτ+6α1qτ+2α2qτ+6α1ρτ+2α2ρτ+2γqτ+2γρτ+2qρτ+2α1qρ+3α1τ2+6α13+2α1α2γ+2α2γτ>0. |
Then, let D=a3[a1a2−a3]−a21a4, and get
D=d1H∗12+d2H∗1+d3, |
It is clear that D>0 and di>0(i=1,2,3), when τ>ρ. Because the expression of di(i=1,2,3) are too long, we list them in Appendix. Hence, the alcoholism equilibrium P∗1 is locally asymptotically stable.
(Ⅲ)Applying to the proof of (Ⅲ) of Theorem 2, when H∗2=1θln(R01R0), we obtain Φ=α1θ. Thus, by Eq.(3.26), we have
a4(H∗2)=τ(α1+ρ)(α1+α2+q+γ)[(α1+H∗2Φ)−α1(1+H∗2θ)]=0, |
and by Eq.(3.23), we have
a1(H∗2)=3α1+α2+q+γ+ρ+τ+H∗2Φ>0. |
Thus, we know that Eq.(3.21) has negative and zero eigenvalues. Therefore, the alcoholism equilibrium P∗2 may be locally stable or not.
(Ⅳ)(ⅰ)Applying to the proof of (Ⅳ) of Theorem 2, when H∗3<H∗2=1θln(R01R0), we obtain Φ<α1θ. Thus, by Eq.(3.26), we have
a4(H∗3)=τ(α1+ρ)(α1+α2+q+γ)[(α1+H∗3Φ)−α1(1+H∗3θ)]<0, |
and by Eq.(3.23), we have
a1(H∗3)=3α1+α2+q+γ+ρ+τ+H∗3Φ>0. |
Assuming g1(H∗3),g2(H∗3),g3(H∗3),g4(H∗3) is the root of Eq.(3.22), and we assume that the real parts satisfying Re(g1(H∗3))≤Re(g2(H∗3))≤Re(g3(H∗3))≤Re(g4(H∗3)), so we obtain
g1(H∗3)+g2(H∗3)+g3(H∗3)+g4(H∗3)=−a1(H∗3)<0, |
and
g1(H∗3)g2(H∗3)g3(H∗3)g4(H∗3)=a4(H∗3)<0. |
There are Re(g1(H∗3))<0 and Re(g4(H∗3))>0, thus, the alcoholism equilibrium P∗3 is unstable.
(ⅱ) When H∗4>H∗2=1θln(R01R0), we obtain Φ>α1θ. Thus, by Eq.(3.26), we have
a4(H∗4)=τ(α1+ρ)(α1+α2+q+γ)[(α1+H∗4Φ)−α1(1+H∗4θ)]>0. |
By μ1=μ2=μ3=μ4, we have
a1(H∗4)=3α1+α2+q+γ+ρ+τ+H∗4Φ>0,a2(H∗4)=(2α1+α2+q+γ+ρ+τ)(α1+H∗4Φ)+(2α1+α2+q+γ+ρ)τ>0,a3(H∗4)=(2α1+α2+q+γ+ρ)(α1+H∗4Φ)τ+(α1+α2+q+γ)α1H∗4Φ+(α1+α2+γ)ρH∗4Φ>0. |
Applying Routh−Hurwitz [13], by assuming that E=a1a2−a3. Then, we obtain
E=e1H∗42+e2H∗4+e3>0, |
where
e1=2Φ2α1+Φ2α2+Φ2γ+Φ2q+Φ2ρ+Φ2τ>0,e2=7Φα12+Φα22+Φγ2+Φq2+Φρ2+Φτ2+5Φα1α2+5Φα1γ+2Φγq+Φγρ+5Φα1q+2Φα2γ+5Φα1ρ+2Φα2q+Φα2ρ+6Φα1τ+2Φα2τ+2Φγτ+2Φqρ+2Φqτ+2Φρτ>0,e3=5α12α2+α1α22+α1γ2+5α12γ+α1q2+5α12q+α1ρ2+5α12ρ+3α1τ2+9α12τ+α2τ2+α22τ+γτ2+γ2τ+qτ2+q2τ+ρτ2+ρ2τ+6α13+2α1α2γ+2α1α2q+2α1α2ρ+6α1α2τ+2α1γq+2α1γρ+6α1γτ+2α2γτ+2α1qρ+2α2qτ+6α1qτ+6α1ρτ+2α2ρτ+2γρτ+2γqτ+2qρτ>0. |
Then, by assuming that F=a3[a1a2−a3]−a21a4, and get
F=f1H∗42+f2H∗4+f3, |
It is clear that D>0, when fi>0(i=1,2,3) and τ>ρ. Because the expression of fi(i=1,2,3) are too long, we do not list it here, and it is placed in Appendix. Hence, the alcoholism equilibrium P∗4 is locally asymptotically stable.
In this section, we study the change of the parameter β causing a forward or a backward bifurcation to occur.
Theorem 4. (Ⅰ) If R01>1, when R0=1, the system (2.1) appears a backward bifurcation.
(Ⅱ) If R01<1, when R0=1, the system (2.1) appears a forward bifurcation.
Proof. Using the central manifold theory described in [29]. Introducing x1=S, x2=L, x3=H, x4=R, x5=T, the system (2.1) becomes
{dx1dt=Λ+qx3−βx1x3e−αx5−α1x1:=f1,dx2dt=βx1x3e−αx5−ρx2−α1x2:=f2,dx3dt=ρx2−γx3−qx3−(α1+α2)x3:=f3,dx4dt=γx3−α1x4:=f4,dx5dt=μ1x1+μ2x2+μ3x3+μ4x4−τx5:=f5. | (3.27) |
Thus, the alcohol free equilibrium P0 is
P0=X0=(Λα1,0,0,0,Λμ1α1τ), |
In view of Theorem 4.1 [29], the Jacobian matrix J(P0) of the system (3.27) in the alcohol free equilibrium is
J(X0)=(−α10q−Λβα1e−αμ1Λα1τ000−(α1+ρ)Λβα1e−αμ1Λα1τ000ρ−(α1+α2+q+γ)0000γ−α10μ1μ2μ3μ4−τ). |
We establish the local stability of alcohol free equilibrium taking β as bifurcation parameter, when R0=1 corresponding to β=β∗=α1(α1+ρ)(α1+α2+q+γ)eΛαμ1α1τΛρ, thus, we obtain
J(X0)=(−α10q−α1(α1+ρ)(α1+α2+q+γ)ρ000−(α1+ρ)α1(α1+ρ)(α1+α2+q+γ)ρ000ρ−(α1+α2+q+γ)0000γ−α10μ1μ2μ3μ4−τ). |
It is clear that 0 is a simple eigenvalue of J(P0). Therefore, there is a right eigenvector associated with 0 eigenvalues that is R=(r1,r2,r3,r4,r5)T, there is a left eigenvector associated with 0 eigenvalues is L=(l1,l2,l3,l4,l5), and it is required to satisfy LR=1.
Therefore, the right eigenvector is
R=(−α1(α1+α2+q+γ)−ρ(α1+α2+γ)α1ρα1+α2+q+γρ1γα1−(μ1−μ2)(α1+α2+q+γ)ρτ−(α1μ1−α1μ3+α2μ1+γμ1−γμ4)α1τ), |
the left eigenvector is
L=(0,ρ2α1+α2+q+γ+ρ,α1+ρ2α1+α2+q+γ+ρ,0,0). |
In view of Theorem 4.1 [29], we know that
a=5∑k,i,j=1lkrirj∂2fk(X0)∂xi∂xj,b=5∑k,i=1lkri∂2fk(X0)∂xi∂β. |
Therefore, we obtain
a=l2r1r3∂2f2(X0)∂x1∂x3+l2r3r1∂2f2(X0)∂x3∂x1+l2r3r5∂2f2(X0)∂x3∂x5+l2r5r3∂2f2(X0)∂x5∂x3=2l2(r1r3∂2f2(X0)∂x1∂x3+r3r5∂2f2(X0)∂x3∂x5)=−2ρΛαβe−Λαμ1α1τα1(2α1+α2+q+γ+ρ)[α1(μ2−μ1)(α1+α2+q+γ)−ρ(α1μ1−α1μ3+α2μ1+γμ1−γu4)α1ρτ]+2ρβe−Λαμ1α1τ2α1+α2+q+γ+ρ[−(α1+ρ)(α1+α2+γ)−α1qα1ρ]=2[α1(α1+ρ)(α1+α2+q+γ)2+ρ(α1+ρ)(α1+α2+γ)(α1+α2+q+γ)Λρ(2α1+α2+q+γ+ρ)](R01−1).b=l2r3∂2f2(X0)∂x3∂β=Λρe−Λαμ1α1τα1(2α1+α2+q+γ+ρ)>0. |
According to Theorem 4.1 of [29], we notice that the coefficient b is always positive. The coefficient a is positive when R01>1. In this case, the direction of the bifurcation of the system (2.1) at R0=1 is backward, as shown in the Figure 9(a). The coefficient a is negative when R01<1. Under this circumstance, the direction of the bifurcation of the system (2.1) at R0=1 is forward, as shown in the Figure 9(b).
The goal of this section is to present some numerical simulations which complement the theoretical results in the previous sections. We choose some parameters based on the Table 1.
Parameter | Description | Value | Source |
Λ | The constant recruitment rate of the population | 0.7−0.8day−1 | [30] |
β | Transmission coefficient from the moderate drinkers | ||
compartment to the light problem drinkers compartment | 0.0099−0.9person−1 | Estimate | |
α | The coefficient that determines how effective the positive | ||
drinking information can reduce the transmission rate | 0.00091−0.8tweet−1 | Estimate | |
ρ | Transmission coefficient from the light problem drinkers | ||
compartment to the heavy problem drinkers compartment | 0.04−0.99day−1 | Estimate | |
μ1 | The rates that the moderate drinkers may tweet | ||
about alcoholism during an alcoholism occasion | 0−1day−1 | [11] | |
μ2 | The rates that the light problem drinkers may tweet | ||
about alcoholism during an alcoholism occasion | 0−1day−1 | [11] | |
μ3 | The rates that the heavy problem drinkers may tweet | ||
about alcoholism during an alcoholism occasion | 0−1day−1 | [11] | |
μ4 | The rates that quitting drinkers may tweet | ||
about alcoholism during an alcoholism occasion | 0−1day−1 | [13] | |
α1 | The natural death rate of the population | 0.009−0.6year−1 | [4,5] |
α2 | The death rate due to heavy alcoholism | 0.02−0.5day−1 | Estimate |
q | Transmission coefficient from the heavy problem drinkers | ||
compartment to the moderate drinkers compartment | 0.006−0.99day−1 | Estimate | |
γ | Transmission coefficient from the heavy problem drinkers | ||
compartment to quitting drinkers compartment | 0.006−0.99day−1 | Estimate | |
τ | The rate that message become outdated | 0.03−0.6year−1 | [11] |
As an example, we choose a set of the following parameters, the parameter values are Λ=0.8,α=0.007,α1=0.009,α2=0.5,μ1=0.04,μ2=0.8,μ3=0.8,μ4=0.8,γ=0.1,q=0.07,ρ=0.09,τ=0.03 and β=0.001. It follows from Theorem 1 that the alcohol free equilibrium P0=(88.89,0,0,0,118.52) of system (2.1) is globally asymptotically stable for any value of time t when R0=0.0519<1 (see Figure 3 (a) and (b)). Furthermore, we can also observe that the value of the equilibrium P∗(t) changes as t increasing and eventually tends to P0=(88.89,0,0,0,118.52) from Figure 3 (a) and (b).
In order to verify the local stability of the alcoholism equilibrium P∗1, we choose a set of the following parameters, the parameter values are Λ=0.8,α=0.007,α1=0.009,α2=0.5,μ1=0.04,μ2=0.04,μ3=0.04,μ4=0.04,γ=0.1,q=0.07,ρ=0.09,τ=0.03 and β=0.004. It follows from Theorem 3 that the alcoholism equilibrium P∗1=(28.16,6.09,0.81,8.97,58.71) of system (2.1) is locally asymptotically stable for any value of time t when R0=2.0765>max{1,R01}, where R01=0.6128 (see Figure 4 (a) and (b)). Furthermore, we can also observe that the value of the equilibrium P∗(t) changes with t increasing and eventually tends to P∗1=(28.16,6.09,0.81,8.97,58.71) from Figure 4 (a) and (b).
In order to verify the local stability of the alcoholism equilibrium P∗4, we choose a set of the following parameters, the parameter values are Λ=8,α=0.07,α1=0.003,α2=0.005,μ1=0.025,μ2=0.025,μ3=0.025,μ4=0.025,γ=0.01,q=0.07,ρ=0.4,τ=0.45 and β=0.9. It follows from Theorem 3 that the alcoholism equilibrium P∗4=(254.1677,85.2877,387.8429,1292.7081,112.2223) of system (2.1) is locally asymptotically stable for any value of time t when R02<R0<max{1,R01}, where R01=2.7788, R0=0.8486 and R02=0.4692 (see Figure 5 (a) and (b)). Furthermore, we can also observe that the value of the equilibrium P∗(t) changes with t increasing and eventually tends to P∗4=(254.1677,85.2877,387.8429,1292.7081,112.2223) from Figure 5 (a) and (b).
Then, we choose a set of the following parameters, the parameter values are Λ=0.8,α=0.007,α1=0.009,α2=0.5,μ1=0,μ2=0.008,μ3=0.8,μ4=0.8,γ=0.1,q=0.99,ρ=0.99,τ=0.03 and β=0.0204. It follows from Theorem 3 that the alcoholism equilibrium P∗1=(49.19,0.92,0.57,6.37,185.30) of system (2.1) is locally asymptotically stable for any value of time t when R0=1.1238>max{1,R01} and β<β∗, where R01=−2.9044 and β∗=0.021 (see Figure 6 (a) and (b)).
If we choose β as 0.076 (see Figure 7 (a) and (b)), we have more intricate dynamic behaviors on system (2.1). As an example, we choose a set of the following parameters, the parameter values are Λ=0.8,α=0.00626,α1=0.009,α2=0.4,μ1=0.009,μ2=0.004,μ3=0.8,μ4=0.8,γ=0.1,q=0.06,ρ=0.9,τ=0.03 and β=0.076. The alcoholism equilibrium P∗1 of system (2.1) occurs a Hopf bifurcation when R0=9.9478>1 and β>β∗, where β∗=0.011 (see Figure 7 (a-d)). In Figure 7 (a-d), we can readily see that the solution curves of system (2.1) perform a sustained periodic oscillation and phase trajectories approaches limit cycle.
In order to demonstrate some results about Hopf bifurcation, we consider β as bifurcation parameter. We know that the alcoholism equilibrium P∗1 is feasible for β∈[0.0099,0.8]. Thus, system (2.1) is stable when 0.0099≤β<0.011, and Hopf bifurcation occurs at the alcoholism equilibrium P∗1 when 0.011≤β<0.08, and system (2.1) becomes stable again when 0.08≤β≤0.2, as depicted in Figure 8(a-e).
The backward and forward bifurcation diagram of system (2.1) is shown in Figure 9, and the direction of bifurcation depends upon the value of R01. As seen in the backward bifurcation diagram of Figure 9(a) when R01=4.4936>1, there is a threshold quantity Rt which is the value of R0. The alcohol free equilibrium is globally asymptotically stable when R0<Rt, where Rt=0.1350. There are two alcoholism equilibria and a alcohol free equilibrium when Rt<R0<1, the upper ones are stable, the middle ones are unstable and the lower ones is globally asymptotically stable. There are a stable alcoholism equilibria and an unstable alcohol free equilibrium when R0>1. As seen in the forward bifurcation diagram of Figure 9(b) when R01=0.5357<1, the alcohol free equilibrium is globally asymptotically stable when R0<1. There are a stable alcoholism equilibria and an unstable alcohol free equilibrium when R0>1.
In this section, we examine the effects of changes in some parameters on the number of heavy problem drinkers. Therefore, we carry out the sensitivity analysis of heavy problem drinkers H.
Figure 10 shows a comparison between the parameters of system (2.1) versus the heavy problem drinkers, we main consider the effect of μ1,q,γ,τ on the dynamics of heavy problem drinkers. Firstly, we choose the effect of parameter μ1 on the dynamics of heavy problem drinkers, the other parameter values are Λ=0.8,α=0.07,α1=0.009,α2=0.5,μ2=0.8,μ3=0.8,μ4=0.8,γ=0.1,q=0.09,ρ=0.09,τ=0.03 and β=0.04, as depicted in Figure 10(a). We know that the number of heavy problem drinkers will decrease when μ1 increase from Figure 10(a). The simulation shows that more Twitter messages can result in the lower alcoholism cases, and changing the number of Twitter messages posted per day does affect the time when the alcoholism reaches the peak. Secondly, we choose the effect of parameter q on the dynamics of heavy problem drinkers, the other parameter values are Λ=0.8,α=0.007,α1=0.009,α2=0.5,μ1=0.04,μ2=0.8,μ3=0.8,μ4=0.8,γ=0.1,ρ=0.09,τ=0.03 and β=0.15, as depicted in Figure 10(b). We know that the number of heavy problem drinkers will decrease when q increase from Figure 10(b). Thirdly, we choose the effect of parameter γ on the dynamics of heavy problem drinkers, the other parameter values are Λ=0.8,α=0.007,α1=0.009,α2=0.5,μ1=0.04,μ2=0.8,μ3=0.8,μ4=0.8,q=0.07,τ=0.03,ρ=0.01 and β=0.15, as depicted in Figure 10(c). We know that the number of heavy problem drinkers will decrease when γ increase from Figure 10(c). The simulation results in Figure 10(b) and 10(c) show that treatment significantly reduces the number of alcoholism cases. Finally, we choose the effect of parameter τ on the dynamics of heavy problem drinkers, the other parameter values are Λ=0.8,α=0.007,α1=0.009,α2=0.5,μ1=0.04,μ2=0.8,μ3=0.8,μ4=0.8,γ=0.1,q=0.07,ρ=0.09 and β=0.15, as depicted in Figure 10(d). We know that the number of heavy problem drinkers will increase when τ increase from Figure 10(d). This indicates that the rate of upper outdated Twitter messages result in the upper alcoholism cases.
We construct a new alcoholism model with treatment and effect of Twitter in this paper. We study the stability of all equilibria and derive the basic reproductive number R0. We also investigate the occurrence of backward and forward bifurcation for a certain defined range of R0 by the center manifold theory. Furthermore, we give some numerical results and sensitivity analysis to extend and illustrate our results. Our results show that Twitter may be a good indicator of alcoholism model and affect the emergence and spread of drinking behavior. How to prove existence of Hopf bifurcation analytically is interesting and still open. We will leave this work in future.
We are grateful to the anonymous referees and the editors for their valuable comments and suggestions which improved the quality of the paper. This work is supported by the National Natural Science Foundation of China (11861044 and 11661050), and the HongLiu first-class disciplines Development Program of Lanzhou University of Technology.
The authors declare there is no conflict of interest.
The formula of d1, d2, d3 in the proof of (Ⅱ) of Theorem 3.
d1=7Φ2α14+12Φ2α13α2+12Φ2α13γ+12Φ2α13q+12Φ2α13ρ+18Φ2α13τ+6Φ2α12α22+12Φ2α12α2γ+12Φ2α12α2q+18Φ2α12α2ρ+21Φ2α12α2τ+6Φ2α12γ2+12Φ2α12γq+18Φ2α12γρ+21Φ2α12γτ+6Φ2α12q2+12Φ2α12qρ+21Φ2α12qτ+6Φ2α12ρ2+21Φ2α12ρτ+13Φ2α12τ2+Φ2α1α23+3Φ2α1α22γ+3Φ2α1α22q+7Φ2α1α22ρ+8Φ2α1α22τ+3Φ2α1α2γ2+6Φ2α1α2γq+14Φ2α1α2γρ+16Φ2α1α2γτ+3Φ2α1α2q2+10Φ2α1α2qρ+16Φ2α1α2qτ+7Φ2α1α2ρ2+14Φ2α1α2ρτ+10Φ2α1α2τ2+Φ2α1γ3+3Φ2α1γ2q+7Φ2α1γ2ρ+8Φ2α1γ2τ+3Φ2α1γq2+10Φ2α1γqρ+16Φ2α1γqτ+14Φ2α1γρτ+10Φ2α1γτ2+Φ2α1q3+3Φ2α1q2ρ+8Φ2α1q2τ+3Φ2α1qρ2+10Φ2α1qτ2+Φ2α1ρ3+8Φ2α1ρ2τ+10Φ2α1ρτ2+2Φ2α1τ3+Φ2α23ρ+Φ2α23τ+3Φ2α22γτ+2Φ2α22qρ+3Φ2α22qτ+Φ2α22ρ2+2Φ2α22ρτ+2Φ2α22τ2+3Φ2α2γ2ρ+3Φ2α2γ2τ+4Φ2α2γqρ+6Φ2α2γqτ+2Φ2α2γρ2+4Φ2α2γρτ+4Φ2α2γτ2+3Φ2α2q2τ+2Φ2α2qρ2+3Φ2α2qρτ+4Φ2α2qτ2+Φ2α2ρ3+2Φ2α2ρ2τ+3Φ2α2ρτ2+Φ2α2τ3+Φ2γ3ρ+Φ2γ3τ+2Φ2γ2qρ+3Φ2γ2qτ+Φ2γ2ρ2+2Φ2γ2ρτ+2Φ2γ2τ2+Φ2γq2ρ+3Φ2γq2τ+2Φ2γqρ2+3Φ2γqρτ+4Φ2γqτ2+Φ2γρ3+2Φ2γρ2τ+3Φ2γρτ2+Φ2γτ3+Φ2q3τ+Φ2q2ρτ+2Φ2q2τ2+Φ2qρ2τ+2Φ2qρτ2+Φ2qτ3+Φ2ρ3τ+2Φ2ρ2τ2+Φ2ρτ3+6αΦα13α2ρ+8αΦα12α22ρ+8αΦα12α2γρ+8αΦα12α2qρ+8αΦα12α2ρ2+2αΦα12α2ρτ+2αΦα1α23ρ+4αΦα1α22γρ+4αΦα1α22qρ+10αΦα1α22ρ2+2αΦα1α22ρτ+2αΦα1α2γ2ρ+4αΦα1α2γqρ+10αΦα1α2γρ2+2αΦα1α2γρτ+2αΦα1α2q2ρ+10αΦα1α2qρ2+2αΦα1α2qρτ+2αΦα1α2ρ3+2αΦα1α2ρ2τ+2αΦα23ρ2+4αΦα22γρ2+4αΦα22qρ2+2αΦα22ρ3+2αΦα22ρ2τ+2αΦα2γ2ρ2+4αΦα2γqρ2+2αΦα2γρ3+2αΦα2γρ2τ+Φ2α2q2ρ+2αΦα2q2ρ2+2αΦα2qρ3+2αΦα2qρ2τ+3Φ2α22γρ+7Φ2α1γρ2+10Φ2α1qρτ, |
d2=6Φα15+9αα14α2ρ+11Φα14α2+11Φα14γ+11Φα14q+11Φα14ρ+26Φα14τ+15αα13α22ρ+6Φα13α22+15αα13α2γρ+12Φα13α2γ+15αα13α2qρ+15αα13α2ρ2+6αα13α2ρτ+18Φα13α2ρ+33Φα13α2τ+6Φα13γ2+12Φα13γq+18Φα13γρ+33Φα13γτ+6Φα13q2+12Φα13qρ+33Φα13qτ+6Φα13ρ2+27Φα13τ2+7αα12α23ρ+Φα12α23+14αα12α22γρ+3Φα12α22γ+14αα12α22qρ+3Φα12α22q+23αα12α22ρ2+8αα12α22ρτ+8Φα12α22ρ+14Φα12α22τ+3Φα12α2γ2+14αα12α2γqρ+6Φα12α2γq+23αα12α2γρ2+8αα12α2γρτ+16Φα12α2γρ+28Φα12α2γτ+7αα12α2q2ρ+3Φα12α2q2+23αα12α2qρ2+8αα12α2qρτ+11Φα12α2qρ+28Φα12α2qτ+7αα12α2ρ3+8αα12α2ρ2τ+8Φα12α2ρ2+αα12α2ρτ2+26Φα12α2ρτ+27Φα12α2τ2+Φα12γ3+3Φα12γ2q+8Φα12γ2ρ+14Φα12γ2τ+3Φα12γq2+11Φα12γqρ+28Φα12γqτ+8Φα12γρ2+26Φα12γρτ+27Φα12γτ2+Φα12q3+3Φα12q2ρ+14Φα12q2τ+3Φα12qρ2+19Φα12qρτ+27Φα12qτ2+Φα12ρ3+14Φα12ρ2τ+27Φα12ρτ2+7Φα12τ3+αα1α24ρ+3αα1α23γρ+3αα1α23qρ+9αα1α23ρ2+2αα1α23ρτ+Φα1α23ρ+2Φα1α23τ+3αα1α22γ2ρ+6αα1α22γqρ+18αα1α22γρ2+4αα1α22γρτ+6Φα1α22γτ+3αα1α22q2ρ+18αα1α22qρ2+4αα1α22qρτ+2Φα1α22qρ+9αα1α22ρ3+10αα1α22ρ2τ+2Φα1α22ρ2+αα1α22ρτ2+5Φα1α22ρτ+9Φα1α22τ2+αα1α2γ3ρ+3αα1α2γ2qρ+9αα1α2γ2ρ2+2αα1α2γ2ρτ+3Φα1α2γ2ρ+3αα1α2γq2ρ+18αα1α2γqρ2+4αα1α2γqρτ+4Φα1α2γqρ+12Φα1α2γqτ+9αα1α2γρ3+10αα1α2γρ2τ+4Φα1α2γρ2+αα1α2γρτ2+10Φα1α2γρτ+18Φα1α2γτ2+αα1α2q3ρ+9αα1α2q2ρ2+2αα1α2q2ρτ+Φα1α2q2ρ+6Φα1α2q2τ+9αα1α2qρ3+10αα1α2qρ2τ+2Φα1α2qρ2+αα1α2qρτ2+5Φα1α2qρτ+αα1α2ρ4+2αα1α2ρ3τ+Φα1α2ρ3+αα1α2ρ2τ2+5Φα1α2ρ2τ+15Φα1α2ρτ2+5Φα1α2τ3+Φα1γ3ρ+2Φα1γ3τ+2Φα1γ2qρ+6Φα1γ2qτ+2Φα1γ2ρ2+9Φα1γ2τ2+Φα1γq2ρ+6Φα1γq2τ+2Φα1γqρ2+5Φα1γqρτ+18Φα1γqτ2+Φα1γρ3+5Φα1γρ2τ+15Φα1γρτ2+5Φα1γτ3+2Φα1q3τ+9Φα1q2τ2+5Φα1qτ3+2Φα1ρ3τ+9Φα1ρ2τ2+5Φα1ρτ3+αα24ρ2+3αα23γρ2+3αα23qρ2+2αα23ρ3+2αα23ρ2τ+Φα23τ2+3αα22γ2ρ2+6αα22γqρ2+4αα22γρ3+3Φα22γτ2+3αα22q2ρ2+4αα22qρ3+4αα22qρ2τ+Φα22qτ(3τ−ρ)+αα22ρ4+2αα22ρ3τ+αα22ρ2τ2+2Φα22ρτ2+Φα22τ3+αα2γ3ρ2+3αα2γ2qρ2+2αα2γ2ρ3+2αα2γ2ρ2τ+3Φα2γ2τ2+3αα2γq2ρ2+4αα2γqρ3+4αα2γqρ2τ+αα2γρ4+2αα2γρ3τ+αα2γρ2τ2+4Φα2γρτ2+2Φα2γτ3+αα2q3ρ2+2αα2q2ρ3+2αα2q2ρ2τ+Φα2q2τ(3τ−2ρ)+αα2qρ4+2αα2qρ3τ+αα2qρ2τ2+Φα2qρτ(3τ−2ρ)+2Φα2qτ3+2Φα2ρ2τ2+Φα2ρτ3+Φγ3τ2+Φρ2τ3+Φγ2qτ(3τ−ρ)+2Φγ2ρτ2+Φγ2τ3+Φγq2τ(3τ−2ρ)+Φγqρτ(3τ−2ρ)+2Φγqτ3+2Φγρ2τ2+Φγρτ3+Φq3τ(τ−ρ)+Φq2ρτ(τ−ρ)+Φq2τ(τ2−ρ2)+Φqρ2τ(τ−ρ)+Φqρτ3+Φρ3τ2+7αα12α2γ2ρ+3Φα1α22γρ+6Φα1α22qτ+6Φα1α2γ2τ+18Φα1α2qτ2+5Φα1γ2ρτ+12Φα1qρτ2+4αα22γρ2τ+Φα2γqτ(6τ−2ρ)+33Φα13ρτ+12Φα13α2q, |
d3=12α15τ+16α14α2τ+16α14γτ+16α14qτ+16α14ρτ+18α14τ2+7α13α22τ+14α13α2qτ+14α13α2ρτ+21α13α2τ2+7α13γ2τ+14α13γqτ+14α13γρτ+7α13q2τ+14α13qρτ+21α13qτ2+7α13ρ2τ+21α13ρτ2+6α13τ3+α12α23τ+3α12α22qτ+3α12α22ρτ+8α12α22τ2+3α12α2γ2τ+6α12α2γqτ+6α12α2γρτ+16α12α2γτ2+3α12α2q2τ+6α12α2qρτ+16α12α2qτ2+3α12α2ρ2τ+16α12α2ρτ2+5α12α2τ3+α12γ3τ+3α12γ2qτ+3α12γ2ρτ+8α12γ2τ2+3α12γq2τ+6α12γqρτ+16α12γqτ2+3α12γρ2τ+16α12γρτ2+5α12γτ3+α12q3τ+3α12q2ρτ+8α12q2τ2+3α12qρ2τ+16α12qρτ2+5α12qτ3+α12ρ3τ+8α12ρ2τ2+5α12ρτ3+α1α23τ2+3α1α22qτ2+3α1α22ρτ2+α1α22τ3+3α1α2γ2τ2+6α1α2γqτ2+6α1α2γρτ2+3α1α2q2τ2+6α1α2qρτ2+2α1α2qτ3+3α1α2ρ2τ2+2α1α2ρτ3+α1γ3τ2+3α1γ2ρτ2+α1γ2τ3+3α1γq2τ2+6α1γqρτ2+2α1γqτ3+3α1γρ2τ2+2α1γρτ3+α1q3τ2+3α1q2ρτ2+α1q2τ3+3α1qρ2τ2+2α1qρτ3+α1ρ3τ2+α1ρ2τ3+14α13α2γτ+21α13γτ2+3α12α22γτ+3α1α22γτ2+2α1α2γτ3+3α1γ2qτ2. |
The formula of f1, f2, f3.
f1=7Φ2α14+12Φ2α13α2+12Φ2α13γ+12Φ2α13q+12Φ2α13ρ+18Φ2α13τ+6Φ2α12α22+12Φ2α12α2γ+12Φ2α12α2q+18Φ2α12α2ρ+21Φ2α12α2τ+6Φ2α12γ2+12Φ2α12γq+18Φ2α12γρ+21Φ2α12γτ+6Φ2α12q2+12Φ2α12qρ+21Φ2α12qτ+6Φ2α12ρ2+21Φ2α12ρτ+13Φ2α12τ2+Φ2α1α23+3Φ2α1α22γ+3Φ2α1α22q+7Φ2α1α22ρ+8Φ2α1α22τ+3Φ2α1α2γ2+6Φ2α1α2γq+14Φ2α1α2γρ+16Φ2α1α2γτ+3Φ2α1α2q2+10Φ2α1α2qρ+16Φ2α1α2qτ+7Φ2α1α2ρ2+14Φ2α1α2ρτ+10Φ2α1α2τ2+Φ2α1γ3+3Φ2α1γ2q+7Φ2α1γ2ρ+8Φ2α1γ2τ+3Φ2α1γq2+10Φ2α1γqρ+16Φ2α1γqτ+14Φ2α1γρτ+10Φ2α1γτ2+Φ2α1q3+3Φ2α1q2ρ+8Φ2α1q2τ+3Φ2α1qρ2+10Φ2α1qτ2+Φ2α1ρ3+8Φ2α1ρ2τ+10Φ2α1ρτ2+2Φ2α1τ3+Φ2α23ρ+Φ2α23τ+3Φ2α22γτ+2Φ2α22qρ+3Φ2α22qτ+Φ2α22ρ2+2Φ2α22ρτ+2Φ2α22τ2+3Φ2α2γ2ρ+3Φ2α2γ2τ+4Φ2α2γqρ+6Φ2α2γqτ+2Φ2α2γρ2+4Φ2α2γρτ+4Φ2α2γτ2+3Φ2α2q2τ+2Φ2α2qρ2+3Φ2α2qρτ+4Φ2α2qτ2+Φ2α2ρ3+2Φ2α2ρ2τ+3Φ2α2ρτ2+Φ2α2τ3+Φ2γ3ρ+Φ2γ3τ+2Φ2γ2qρ+3Φ2γ2qτ+Φ2γ2ρ2+2Φ2γ2ρτ+2Φ2γ2τ2+Φ2γq2ρ+3Φ2γq2τ+2Φ2γqρ2+3Φ2γqρτ+4Φ2γqτ2+Φ2γρ3+2Φ2γρ2τ+3Φ2γρτ2+Φ2γτ3+Φ2q3τ+Φ2q2ρτ+2Φ2q2τ2+Φ2qρ2τ+2Φ2qρτ2+Φ2qτ3+Φ2ρ3τ+2Φ2ρ2τ2+Φ2ρτ3+6αΦα13α2ρ+8αΦα12α22ρ+8αΦα12α2γρ+8αΦα12α2qρ+8αΦα12α2ρ2+2αΦα12α2ρτ+2αΦα1α23ρ+4αΦα1α22γρ+4αΦα1α22qρ+10αΦα1α22ρ2+2αΦα1α22ρτ+2αΦα1α2γ2ρ+4αΦα1α2γqρ+10αΦα1α2γρ2+2αΦα1α2γρτ+2αΦα1α2q2ρ+10αΦα1α2qρ2+2αΦα1α2qρτ+2αΦα1α2ρ3+2αΦα1α2ρ2τ+2αΦα23ρ2+4αΦα22γρ2+4αΦα22qρ2+2αΦα22ρ3+2αΦα22ρ2τ+2αΦα2γ2ρ2+4αΦα2γqρ2+2αΦα2γρ3+2αΦα2γρ2τ+Φ2α2q2ρ+2αΦα2q2ρ2+2αΦα2qρ3+2αΦα2qρ2τ+3Φ2α22γρ+7Φ2α1γρ2+10Φ2α1qρτ,f2=6Φα15+9αα14α2ρ+11Φα14α2+11Φα14γ+11Φα14q+11Φα14ρ+26Φα14τ+15αα13α22ρ+6Φα13α22+15αα13α2γρ+12Φα13α2γ+15αα13α2qρ+15αα13α2ρ2+6αα13α2ρτ+18Φα13α2ρ+33Φα13α2τ+6Φα13γ2+12Φα13γq+18Φα13γρ+33Φα13γτ+6Φα13q2+12Φα13qρ+33Φα13qτ+6Φα13ρ2+27Φα13τ2+7αα12α23ρ+Φα12α23+14αα12α22γρ+3Φα12α22γ+14αα12α22qρ+3Φα12α22q+23αα12α22ρ2+8αα12α22ρτ+8Φα12α22ρ+14Φα12α22τ+3Φα12α2γ2+14αα12α2γqρ+6Φα12α2γq+23αα12α2γρ2+8αα12α2γρτ+16Φα12α2γρ+28Φα12α2γτ+7αα12α2q2ρ+3Φα12α2q2+23αα12α2qρ2+8αα12α2qρτ+11Φα12α2qρ+28Φα12α2qτ+7αα12α2ρ3+8αα12α2ρ2τ+8Φα12α2ρ2+αα12α2ρτ2+26Φα12α2ρτ+27Φα12α2τ2+Φα12γ3+3Φα12γ2q+8Φα12γ2ρ+14Φα12γ2τ+3Φα12γq2+11Φα12γqρ+28Φα12γqτ+8Φα12γρ2+26Φα12γρτ+27Φα12γτ2+Φα12q3+3Φα12q2ρ+14Φα12q2τ+3Φα12qρ2+19Φα12qρτ+27Φα12qτ2+Φα12ρ3+14Φα12ρ2τ+27Φα12ρτ2+7Φα12τ3+αα1α24ρ+3αα1α23γρ+3αα1α23qρ+9αα1α23ρ2+2αα1α23ρτ+Φα1α23ρ+2Φα1α23τ+3αα1α22γ2ρ+6αα1α22γqρ+18αα1α22γρ2+4αα1α22γρτ+6Φα1α22γτ+3αα1α22q2ρ+18αα1α22qρ2+4αα1α22qρτ+2Φα1α22qρ+9αα1α22ρ3+10αα1α22ρ2τ+2Φα1α22ρ2+αα1α22ρτ2+5Φα1α22ρτ+9Φα1α22τ2+αα1α2γ3ρ+3αα1α2γ2qρ+9αα1α2γ2ρ2+2αα1α2γ2ρτ+3Φα1α2γ2ρ+3αα1α2γq2ρ+18αα1α2γqρ2+4αα1α2γqρτ+4Φα1α2γqρ+12Φα1α2γqτ+9αα1α2γρ3+10αα1α2γρ2τ+4Φα1α2γρ2+αα1α2γρτ2+10Φα1α2γρτ+18Φα1α2γτ2+αα1α2q3ρ+9αα1α2q2ρ2+2αα1α2q2ρτ+Φα1α2q2ρ+6Φα1α2q2τ+9αα1α2qρ3+10αα1α2qρ2τ+2Φα1α2qρ2+αα1α2qρτ2+5Φα1α2qρτ+αα1α2ρ4+2αα1α2ρ3τ+Φα1α2ρ3+αα1α2ρ2τ2+5Φα1α2ρ2τ+15Φα1α2ρτ2+5Φα1α2τ3+Φα1γ3ρ+2Φα1γ3τ+2Φα1γ2qρ+6Φα1γ2qτ+2Φα1γ2ρ2+9Φα1γ2τ2+Φα1γq2ρ+6Φα1γq2τ+2Φα1γqρ2+5Φα1γqρτ+18Φα1γqτ2+Φα1γρ3+5Φα1γρ2τ+15Φα1γρτ2+5Φα1γτ3+2Φα1q3τ+9Φα1q2τ2+5Φα1qτ3+2Φα1ρ3τ+9Φα1ρ2τ2+5Φα1ρτ3+αα24ρ2+3αα23γρ2+3αα23qρ2+2αα23ρ3+2αα23ρ2τ+Φα23τ2+3αα22γ2ρ2+6αα22γqρ2+4αα22γρ3+3Φα22γτ2+3αα22q2ρ2+4αα22qρ3+4αα22qρ2τ+Φα22qτ(3τ−ρ)+αα22ρ4+2αα22ρ3τ+αα22ρ2τ2+2Φα22ρτ2+Φα22τ3+αα2γ3ρ2+3αα2γ2qρ2+2αα2γ2ρ3+2αα2γ2ρ2τ+3Φα2γ2τ2+3αα2γq2ρ2+4αα2γqρ3+4αα2γqρ2τ+αα2γρ4+2αα2γρ3τ+αα2γρ2τ2+4Φα2γρτ2+2Φα2γτ3+αα2q3ρ2+2αα2q2ρ3+2αα2q2ρ2τ+Φα2q2τ(3τ−2ρ)+αα2qρ4+2αα2qρ3τ+αα2qρ2τ2+Φα2qρτ(3τ−2ρ)+2Φα2qτ3+2Φα2ρ2τ2+Φα2ρτ3+Φγ3τ2+Φρ2τ3+Φγ2qτ(3τ−ρ)+2Φγ2ρτ2+Φγ2τ3+Φγq2τ(3τ−2ρ)+Φγqρτ(3τ−2ρ)+2Φγqτ3+2Φγρ2τ2+Φγρτ3+Φq3τ(τ−ρ)+Φq2ρτ(τ−ρ)+Φq2τ(τ2−ρ2)+Φqρ2τ(τ−ρ)+Φqρτ3+Φρ3τ2+7αα12α2γ2ρ+3Φα1α22γρ+6Φα1α22qτ+6Φα1α2γ2τ+18Φα1α2qτ2+5Φα1γ2ρτ+12Φα1qρτ2+4αα22γρ2τ+Φα2γqτ(6τ−2ρ)+33Φα13ρτ+12Φα13α2q,f3=12α15τ+16α14α2τ+16α14γτ+16α14qτ+16α14ρτ+18α14τ2+7α13α22τ+14α13α2qτ+14α13α2ρτ+21α13α2τ2+7α13γ2τ+14α13γqτ+14α13γρτ+7α13q2τ+14α13qρτ+21α13qτ2+7α13ρ2τ+21α13ρτ2+6α13τ3+α12α23τ+3α12α22qτ+3α12α22ρτ+8α12α22τ2+3α12α2γ2τ+6α12α2γqτ+6α12α2γρτ+16α12α2γτ2+3α12α2q2τ+6α12α2qρτ+16α12α2qτ2+3α12α2ρ2τ+16α12α2ρτ2+5α12α2τ3+α12γ3τ+3α12γ2qτ+3α12γ2ρτ+8α12γ2τ2+3α12γq2τ+6α12γqρτ+16α12γqτ2+3α12γρ2τ+16α12γρτ2+5α12γτ3+α12q3τ+3α12q2ρτ+8α12q2τ2+3α12qρ2τ+16α12qρτ2+5α12qτ3+α12ρ3τ+8α12ρ2τ2+5α12ρτ3+α1α23τ2+3α1α22qτ2+3α1α22ρτ2+α1α22τ3+3α1α2γ2τ2+6α1α2γqτ2+6α1α2γρτ2+3α1α2q2τ2+6α1α2qρτ2+2α1α2qτ3+3α1α2ρ2τ2+2α1α2ρτ3+α1γ3τ2+3α1γ2ρτ2+α1γ2τ3+3α1γq2τ2+6α1γqρτ2+2α1γqτ3+3α1γρ2τ2+2α1γρτ3+α1q3τ2+3α1q2ρτ2+α1q2τ3+3α1qρ2τ2+2α1qρτ3+α1ρ3τ2+α1ρ2τ3+14α13α2γτ+21α13γτ2+3α12α22γτ+3α1α22γτ2+2α1α2γτ3+3α1γ2qτ2. |
[1] | A. M. Nikalje, A. Kumar and S. K. V. Sai, Influence of parameters and optimization of EDM performance measures on MDN 300 steel using Taguchi method, Int. J. Adv. Manuf. Tech., 69 (2013), 41–49. |
[2] | X. P. Dang, Constrained multi-objective optimization of EDM process parameters using kriging model and particle swarm algorithm, Mater. Manuf. Process., 33 (2018), 397–404. |
[3] | G. D'Urso, C. Giardini, G. Maccarini, et al., Analysis of the surface quality of steel and ceramic materials machined by micro-EDM, European Society for Precision Engineering and Nanotechnology, Conference Proceedings-18th International Conference and Exhibition, EUSPEN 2018, 431–432. |
[4] | G. D'Urso, C. Giardini and M. Quarto, Characterization of surfaces obtained by micro-EDM milling on steel and ceramic components, Int. J. Adv. Manuf. Tech., 97 (2018), 2077–2085. |
[5] | S. Gopalakannan, T. Senthilvelan and S. Ranganathan, Modeling and Optimization of EDM Process Parameters on Machining of Al 7075-B 4 C MMC Using RSM, Procedia Eng., 38 (2012), 685–690. |
[6] | H. Mohammadjifar, L. Q. Bui and C. T. Ngyen, Experimental investigation of the effects of tool initial surface roughness on the electrical discharge machining (EDM) performance, Int. J. Adv. Manuf. Tech., 95 (2018), 2093–2104. |
[7] | J. L. Lin, K. S. Wang, B. H. Yan, et al., Optimization of the electrical discharge machining process based on the Taguchi method with fuzzy logics, J. Mater. Process. Tech., 102 (2000), 48–55. |
[8] | K. P. Somashekhar, N. Ramachandran and J. Mathew, Optimization of material removal rate in micro-EDM using artificial neural network and genetic algorithms, Mater. Manuf. Process., 25 (2010), 467–475. |
[9] | M. Arindam, Process parameter optimization during EDM of AISI 316 LN stainless steel by using fuzzy based multi-objective PSO, J. Mech. Sci. Tech., 27 (2013), 2143–2151. |
[10] | G. D'Urso, C. Giardini, M. Quarto, et al., Cost index model for the process performance optimization of micro-EDM drilling on tungsten carbide, Micromach., 8 (2017), 251. |
[11] | S. Parsana, N. Radadia, M. Sheth, et al., Machining parameter optimization for EDM machining of Mg–RE–Zn–Zr alloy using multi-objective Passing Vehicle Search algorithm, Arch. Civ. Mech. Eng., 18 (2018), 799–817. |
[12] | G. Rajyalakshmi and P. V. Ramaiah, Multiple process parameter optimization of wire electrical discharge;machining on Inconel 825 using Taguchi grey relational analysis, Int. J. Adv. Manuf. Tech., 69 (2013), 1249–1262. |
[13] | R. Świercz, D. Oniszczuk-Świercz and T. Chmielewski, Multi-Response Optimization of Electrical Discharge Machining Using the Desirability Function, Micromach., 10 (2019), 72. |
[14] | P. S. Bharti, S. Maheshwari and C. Sharma, Multi-objective optimization of electric-discharge machining process using controlled elitist NSGA-II, J. Mech. Sci. Tech., 26 (2012), 1875–1883. |
[15] | D. Kanagarajan, R. Karthikeyan, K. Palanikumar, et al., Optimization of electrical discharge machining characteristics of WC/Co composites using non-dominated sorting genetic algorithm (NSGA-II), Int. J. Adv. Manuf. Tech., 36 (2008), 1124–1132. |
[16] | C. Ulas and H. Ahmet, Modeling and analysis of electrode wear and white layer thickness in die-sinking EDM process through response surface methodology, Int. J. Adv. Manuf. Tech., 38 (2008), 1148–1156. |
[17] | A. Majumder, P. K. Das, A. Majumber, et al., An approach to optimize the EDM process parameters using desirability-based multi-objective PSO, Prod. Manuf. Res., 2 (2014), 228–240. |
[18] | F. G. Cao and D. Y. Yang, The study of high efficiency and intelligent optimization system in EDM sinking process, J. Mater. Process. Tech., 49 (2004), 83–87. |
[19] | G. K. M. Rao, G. Rangajanardhaa, D. H. Rao, et al., Development of hybrid model and optimization of surface roughness in electric discharge machining using artificial neural networks and genetic algorithm, J. Mater. Process. Tech., 209 (2009), 1512–1520. |
[20] | G. Rajyalakshmi and P. V. Ramaiah, Multiple process parameter optimization of wire electrical discharge machining on Inconel 825 using Taguchi grey relational analysis, Int. J. Adv. Manuf. Tech., 69 (2013), 1249–1262. |
[21] | R. Maneswar and P. P. Kumar, Parametric optimization for selective surface modification in EDM using Taguchi analysis, Mater. Manuf. Process., 31 (2016), 422–431. |
[22] | J. L. Lin and C. L. Lin, The use of the orthogonal array with grey relational analysis to optimize the electrical discharge machining process with multiple performance characteristics, Int. J. Mach. Tool. Manuf., 42 (2002), 237–244. |
[23] | P. Sathiya, S. Aravindan and A. N. Haq, Mechanical and metallurgical properties of friction welded AISI 304 austenitic stainless steel, Int. J. Adv. Manuf. Tech., 26 (2005), 505–511. |
[24] | Z. Zhang, X. Q. Yang and H. Ma, Optimization of Enzymatic Hydrolysis of Tilapia Waste by Plackett-Burman Design and Central Composite Design, Food Sci., 32 (2011), 1–5. |
[25] | X. Li, C. Gong, L. Gu, et al., A reliability-based optimization method using sequential surrogate model and Monte Carlo simulation, Struct. Multidiscip. O., (2018), 1–22. |
[26] | F. Biancofiore, M. Busilacchio, M. Verdecchia, et al., Recursive neural network model for analysis and forecast of PM10 and PM2.5, Atmo. Pollut. Res., 8 (2017), 652–659. |
[27] | Q. Zhou, H. Jiang, J. Wang, et al., A hybrid model for PM 2.5 forecasting based on ensemble empirical mode decomposition and a general regression neural network, Sci. Total Environ., 496 (2014), 264–274. |
[28] | K. Deb, A. Pratap, S. Agarwal, et al., A fast and elitist multiobjective genetic algorithm: NSGA-II, IEEE T. Evolut. Comput., 6 (2002), 182–197. |
[29] | H. Li and Q. F. Zhang, Multiobjective optimization problems with complicated Pareto sets, MOEA/D and NSGA-II, IEEE T. Evolut. Comput., 13 (2009), 284–302. |
[30] | S. Mardle and K. M. Miettinen, Nonlinear multiobjective optimization, J. Oper. Res. Soc., 51 (2000), 246. |
[31] | R. Gudolph, On a multi-objective evolutionary algorithm and its convergence to the Pareto set, IEEE International Conference on Evolutionary Computation, IEEE ICEC 1998, 511–516. |
[32] | S. Chaki, N. B. Rani, S. Ghosal, et al., Multi-objective optimisation of pulsed Nd:YAG laser cutting process using integrated ANN–NSGAII model, J. Intell. Manuf., 29 (2018), 175–190. |
[33] | T. Chang, J. Q. Lu, A. X. Shen, et al., Simulation and optimization of the post plasma-catalytic system for toluene degradation by a hybrid ANN and NSGA-II method, Appl. Catal. B-Environ., 244 (2019), 107–119. |
[34] | A. Adel, Y. Kai and M. Alper, ASRSM: A sequential experimental design for response surface optimization, Qual. Reliab. Eng. Int., 29 (2013), 241–258. |
[35] | W. Chen, M. H. Nguyen, W. H. Chiu, et al., Optimization of the plastic injection molding process using the Taguchi method, RSM, and hybrid GA-PSO, Int. J. Adv. Manuf. Tech., 83 (2016), 1873–1886. |
[36] | S. J. An, W. Q. Liu and S. Venkatesh, Fast cross-validation algorithms for least squares support vector machine and kernel ridge regression, Pattern Recogn., 40 (2005), 2154–2162. |
[37] | T. Chen and S. J. Lu, Accurate and efficient traffic sign detection using discriminative adaboost and support vector regression, IEEE T. Veh. Tech., 65 (2016), 4006–4015. |
[38] | X. K. Li, H. B. Qiu, Z. Z. Chen, et al., A local Kriging approximation method using MPP for reliability-based design optimization, Comput. Struct., 162 (2016), 102–115. |
[39] | X. K. Li, H. B. Qiu, Z. Z. Chen, et al., A local sampling method with variable radius for RBDO using Kriging, Eng. Computation., 32 (2015), 1908–1933. |
[40] | G. Shieh, Improved shrinkage estimation of squared multiple correlation coefficient and squared cross-validity coefficient, Organ. Res. Meth., 11 (2008), 387–407. |
[41] | H. Wang, S. Shan, G. G. Wang, et al., Integrating least square support vector regression and mode pursuing sampling optimization for crashworthiness design, J. Mech. Design, 133 (2011), 041002. |
1. | Zhan‐Ping Ma, Hai‐Feng Huo, Hong Xiang, Spatiotemporal patterns induced by delay and cross‐fractional diffusion in a predator‐prey model describing intraguild predation, 2020, 43, 0170-4214, 5179, 10.1002/mma.6259 | |
2. | Hai-Feng Huo, Shuang-Lin Jing, Xun-Yang Wang, Hong Xiang, Modeling and analysis of a H1N1 model with relapse and effect of Twitter, 2020, 560, 03784371, 125136, 10.1016/j.physa.2020.125136 | |
3. | Zizhen Zhang, Fangfang Yang, Wanjun Xia, Influence of time delay on bifurcation of a synthetic drug transmission model with psychological addicts, 2020, 2020, 1687-1847, 10.1186/s13662-020-02607-y | |
4. | Shuang-Lin Jing, Hai-Feng Huo, Hong Xiang, Modeling the Effects of Meteorological Factors and Unreported Cases on Seasonal Influenza Outbreaks in Gansu Province, China, 2020, 82, 0092-8240, 10.1007/s11538-020-00747-6 | |
5. | Zhong-Kai Guo, Hong Xiang, Hai-Feng Huo, Analysis of an age-structured tuberculosis model with treatment and relapse, 2021, 82, 0303-6812, 10.1007/s00285-021-01595-1 | |
6. | Hai-Feng Huo, Li-Na Gu, Hong Xiang, MODELLING AND ANALYSIS OF AN HIV/AIDS MODEL WITH DIFFERENT WINDOW PERIOD AND TREATMENT, 2021, 11, 2156-907X, 1927, 10.11948/20200279 | |
7. | Cheng-Cheng Zhu, Jiang Zhu, Spread trend of COVID-19 epidemic outbreak in China: using exponential attractor method in a spatial heterogeneous SEIQR model, 2020, 17, 1551-0018, 3062, 10.3934/mbe.2020174 | |
8. | Qian Yang, Hai-Feng Huo, Hong Xiang, Analysis of an edge-based SEIR epidemic model with sexual and non-sexual transmission routes, 2023, 609, 03784371, 128340, 10.1016/j.physa.2022.128340 | |
9. | Banan Maayah, Omar Abu Arqub, Hilbert approximate solutions and fractional geometric behaviors of a dynamical fractional model of social media addiction affirmed by the fractional Caputo differential operator, 2023, 25900544, 100092, 10.1016/j.csfx.2023.100092 | |
10. | SHAHER MOMANI, R. P. CHAUHAN, SUNIL KUMAR, SAMIR HADID, ANALYSIS OF SOCIAL MEDIA ADDICTION MODEL WITH SINGULAR OPERATOR, 2023, 31, 0218-348X, 10.1142/S0218348X23400972 | |
11. | Ning Li, Yuequn Gao, Modified fractional order social media addiction modeling and sliding mode control considering a professionally operating population, 2024, 32, 2688-1594, 4043, 10.3934/era.2024182 | |
12. | Yasir Nadeem Anjam, Saira Tabassum, Muhammad Arshad, Mati ur Rahman, Muhammad Ateeq Tahir, Mathematical insights of social media addiction: fractal-fractional perspectives, 2024, 99, 0031-8949, 055230, 10.1088/1402-4896/ad348c | |
13. | Saduri Das, Prashant K. Srivastava, Pankaj Biswas, The role of social media in a tuberculosis compartmental model: Exploring Hopf-bifurcation and nonlinear oscillations, 2024, 03784754, 10.1016/j.matcom.2024.11.015 | |
14. | V. Madhusudanan, L. Guerrini, B. S. N. Murthy, Nhu-Ngoc Dao, Sungrae Cho, Exploring the Dynamics of Social Media Addiction and Depression Models With Discrete and Distributed Delays, 2025, 13, 2169-3536, 55682, 10.1109/ACCESS.2025.3555306 |
Parameter | Description | Value | Source |
\Lambda | The constant recruitment rate of the population | 0.7-0.8 day^{-1} | [30] |
\beta | Transmission coefficient from the moderate drinkers | ||
compartment to the light problem drinkers compartment | 0.0099 - 0.9 person^{-1} | Estimate | |
\alpha | The coefficient that determines how effective the positive | ||
drinking information can reduce the transmission rate | 0.00091 - 0.8 tweet^{-1} | Estimate | |
\rho | Transmission coefficient from the light problem drinkers | ||
compartment to the heavy problem drinkers compartment | 0.04 - 0.99 day^{-1} | Estimate | |
{\mu_1} | The rates that the moderate drinkers may tweet | ||
about alcoholism during an alcoholism occasion | 0 - 1 day^{-1} | [11] | |
{\mu_2} | The rates that the light problem drinkers may tweet | ||
about alcoholism during an alcoholism occasion | 0 - 1 day^{-1} | [11] | |
{\mu_3} | The rates that the heavy problem drinkers may tweet | ||
about alcoholism during an alcoholism occasion | 0 - 1 day^{-1} | [11] | |
{\mu_4} | The rates that quitting drinkers may tweet | ||
about alcoholism during an alcoholism occasion | 0 - 1 day^{-1} | [13] | |
{\alpha_1} | The natural death rate of the population | 0.009 - 0.6year^{-1} | [4,5] |
{\alpha_2} | The death rate due to heavy alcoholism | 0.02 - 0.5day^{-1} | Estimate |
q | Transmission coefficient from the heavy problem drinkers | ||
compartment to the moderate drinkers compartment | 0.006 - 0.99day^{-1} | Estimate | |
\gamma | Transmission coefficient from the heavy problem drinkers | ||
compartment to quitting drinkers compartment | 0.006 - 0.99day^{-1} | Estimate | |
{\tau} | The rate that message become outdated | 0.03 - 0.6year^{-1} | [11] |
Parameter | Description | Value | Source |
\Lambda | The constant recruitment rate of the population | 0.7-0.8 day^{-1} | [30] |
\beta | Transmission coefficient from the moderate drinkers | ||
compartment to the light problem drinkers compartment | 0.0099 - 0.9 person^{-1} | Estimate | |
\alpha | The coefficient that determines how effective the positive | ||
drinking information can reduce the transmission rate | 0.00091 - 0.8 tweet^{-1} | Estimate | |
\rho | Transmission coefficient from the light problem drinkers | ||
compartment to the heavy problem drinkers compartment | 0.04 - 0.99 day^{-1} | Estimate | |
{\mu_1} | The rates that the moderate drinkers may tweet | ||
about alcoholism during an alcoholism occasion | 0 - 1 day^{-1} | [11] | |
{\mu_2} | The rates that the light problem drinkers may tweet | ||
about alcoholism during an alcoholism occasion | 0 - 1 day^{-1} | [11] | |
{\mu_3} | The rates that the heavy problem drinkers may tweet | ||
about alcoholism during an alcoholism occasion | 0 - 1 day^{-1} | [11] | |
{\mu_4} | The rates that quitting drinkers may tweet | ||
about alcoholism during an alcoholism occasion | 0 - 1 day^{-1} | [13] | |
{\alpha_1} | The natural death rate of the population | 0.009 - 0.6year^{-1} | [4,5] |
{\alpha_2} | The death rate due to heavy alcoholism | 0.02 - 0.5day^{-1} | Estimate |
q | Transmission coefficient from the heavy problem drinkers | ||
compartment to the moderate drinkers compartment | 0.006 - 0.99day^{-1} | Estimate | |
\gamma | Transmission coefficient from the heavy problem drinkers | ||
compartment to quitting drinkers compartment | 0.006 - 0.99day^{-1} | Estimate | |
{\tau} | The rate that message become outdated | 0.03 - 0.6year^{-1} | [11] |