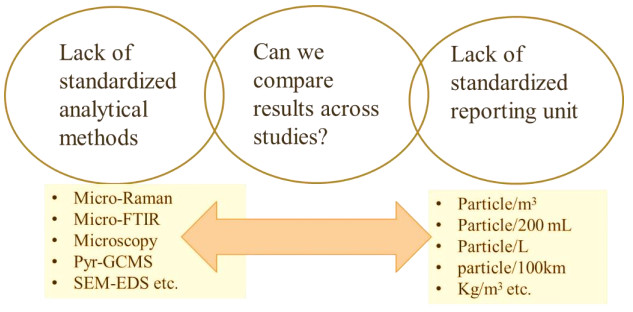
Citation: Chun Wen, Qian-Ying Hu, Bing-Yuan Pu, Yu-Yun Huang. Acceleration of an adaptive generalized Arnoldi method for computing PageRank[J]. AIMS Mathematics, 2021, 6(1): 893-907. doi: 10.3934/math.2021053
[1] | Hongyan Guo . Automorphism group and twisted modules of the twisted Heisenberg-Virasoro vertex operator algebra. Electronic Research Archive, 2021, 29(4): 2673-2685. doi: 10.3934/era.2021008 |
[2] | Pengliang Xu, Xiaomin Tang . Graded post-Lie algebra structures and homogeneous Rota-Baxter operators on the Schrödinger-Virasoro algebra. Electronic Research Archive, 2021, 29(4): 2771-2789. doi: 10.3934/era.2021013 |
[3] | Daniel Sanchez, David Alfaya, Jaime Pizarroso . Motives meet SymPy: studying λ-ring expressions in Python. Electronic Research Archive, 2025, 33(4): 2118-2147. doi: 10.3934/era.2025093 |
[4] |
Kengo Matsumoto .
|
[5] | Yanni Yang, Quanguo Chen . The criteria for automorphisms on finite-dimensional algebras. Electronic Research Archive, 2024, 32(11): 6140-6152. doi: 10.3934/era.2024285 |
[6] | Xue Yu . Orientable vertex imprimitive complete maps. Electronic Research Archive, 2024, 32(4): 2466-2477. doi: 10.3934/era.2024113 |
[7] | Peigen Cao, Fang Li, Siyang Liu, Jie Pan . A conjecture on cluster automorphisms of cluster algebras. Electronic Research Archive, 2019, 27(0): 1-6. doi: 10.3934/era.2019006 |
[8] | Jingjing Hai, Xian Ling . Normalizer property of finite groups with almost simple subgroups. Electronic Research Archive, 2022, 30(11): 4232-4237. doi: 10.3934/era.2022215 |
[9] | Agustín Moreno Cañadas, Robinson-Julian Serna, Isaías David Marín Gaviria . Zavadskij modules over cluster-tilted algebras of type A. Electronic Research Archive, 2022, 30(9): 3435-3451. doi: 10.3934/era.2022175 |
[10] | Lin Shen, Shu Wang, Yongxin Wang . The well-posedness and regularity of a rotating blades equation. Electronic Research Archive, 2020, 28(2): 691-719. doi: 10.3934/era.2020036 |
Plastics (i.e., synthetic organic polymers) have seen significant growth in recent decades, with global output increasing tenfold from 1950 to 2013. While the quantity of plastics produced in Europe has remained relatively stable over the past ten years, the amount of plastics produced in the rest of the globe has continued to grow [1]. Given the high number of plastics produced and the long shelf life of plastics, it should come as no surprise that plastics may be discovered in the natural environment. Initially, scientists and the general public focused their attention on bigger pieces of plastic litter. Small plastic particles have been found in marine environments for many years, even though they were initially discovered in the early 1970s [1,2].
Environmental experts across the globe consider plastic waste contamination of ecosystems to be one of the most severe environmental problems facing the planet. Small-sized particles, which make up a significant portion of this debris, have gotten more attention lately and are causing special worry. They are referred to as nano- (NPs) and microplastics (MPs), and their largest diameters are generally defined as being 0.001–0.1 µm (for NPs) and 0.1 µm –5 mm (MPs) respectively [3,4]. A broad definition of small particles is that they can either be produced directly for use in a variety of consumer and industrial applications, acting as primary sources of these particles, or they can be generated through the fragmentation of larger plastic particles, acting as secondary sources of these particles.
The probability of ecosystem exposure increases as ambient NPs and MPs concentrations rise, and the likelihood of interaction, ingestion, and harmful consequences throughout food webs increases as well. Nano- and micro- plastics have the potential to have a negative effect on biota in a number of ways, according to the researchers [5,6]. Nano- and micro- plastics, like macroplastics and macrofauna, may cause entanglement and physical impediment in creatures such as zooplankton, which has a proportionately smaller size than macroplastics and macrofauna [7,8]. If MPs are eaten, they may cause intestinal obstructions, which can result in hunger or decreased energy budgets. Plastics are also composed of a mix of various compounds, including polymers, dyes, and plasticisers, all of which have the potential to be hazardous in certain circumstances [9]. Oxidative stress is a frequent reaction to microplastic exposure that has been documented [5]. Plastics, in addition to chemicals, have the potential to serve as a vector for organism movement, which may result in the introduction of non-native species and diseases [10]. While these potential consequences should be taken into consideration, with a few exceptions [11], the vast majority of laboratory investigations have not shown MPs to be intrinsically harmful when they have been subjected to them at high concentrations over extended periods of time. The potential of chronic sub-lethal harm to lower trophic species exposed over longer, more realistic environmental timescales has, on the other hand, been brought to attention. This may have ramifications for ecosystems, possibly resulting in trophic cascades [12]. Even more importantly, such studies are often conducted on species and ecosystems that are deemed adaptable or regionally widespread, such as those found along temperate coastlines. However, numerous studies have shown and addressed the detrimental impacts of MPs and NPs to marine creatures and sea animals as well as terrestrial animals and human health [13].
As a result of the ubiquity of MPs/NPs in ecosystems, studies on the quantification and identification of MPs/NPs are on rise yearly. With the proliferation of research, there have been significant restrictions in terms of reporting units and technique, which creates difficulties when comparing data across studies (Figure 1). As a result, reaching a judgment or conclusion about the existence of MPs/NPs in a specific area or matrix may be very challenging and when quantified may be difficult to interpret.
The aforementioned constraint may be overcome via the use of index models (IMs). The use of pollution and risk indices is critical for an accurate evaluation of MPs/NPs contamination of various media. Model-generated data are seen as simplified representations of environmental problems, which enables policymakers to better understand environmental difficulties and, as a consequence, make more timely and efficient environmental decisions. The aim of this study is to present index models that can be adopted in the study of environmental MPs and NPs globally.
Although the development of methods for evaluating environmental quality may be traced back many decades, the issue is still relevant and far from being resolved. A large number of different national and international scientific and political organizations are paying close attention to the issue of various kinds of environmental indices and indicators, which is a subject that has received particular attention recently. Following Ott's [14] definition, an indicator is a method developed to reduce a vast amount of data to its simplest form while maintaining important significance for the questions that are being asked of the data. Furthermore, Malkina-Pykh and Pyk [15] described environmental indicators as measurements of change in the state of an environmental system or in human activities that have an impact on the state of an environmental system, most often in connection to a standard or value as well as an aim or goal. The modeling process is summarized in Figure 1.
An index is a kind of indication that does not have a unit of measurement. When multiple indicators are combined to represent the overall effect on a certain element within a wider context, such as the "status of the environment, " the result is known as an index (or composite index) (though it could, of course, attempt to describe the broader state-of-the-environment at any scale). The technique for aggregating indicators into an index is critical for the issue of index creation since it increases the credibility of indices used in practice.
The index is a mathematical formula that produces a single number as the end result of the calculation of measurable variables, thus providing a measure of the qualitative condition of a media medium. Government agencies and authorities use IMs to convey to the public how filthy the air, soil, water or the environment is currently or will become in the future. IMs are used for both pollution and risk assessment and are among the most widely used tools in many fields.
Models are now currently being developed for marine litter monitoring and assessment [16,17] while concerning the smaller particle sizes have been overlooked. Generally, constant monitoring of pollutant concentration is required for calculation of an IMs, such as for MPs/NPs. The MPs/NPs dosage is represented by the product of its concentration and exposure duration. The health consequences associated with a particular dosage are determined via epidemiological study, which varies depending on the substance. Because environmental MPs/NPs vary in their potency (due to the different polymer composition), it is bests to translate pollutant concentrations into index values differently. IMs values are usually divided into ranges based on a specified class that describes the degree of the effect on the quality or health.
IMs definitions vary from state to state depending on local governments' views on respect for national thresholds and quality requirements. The calculating technique and the descriptor categories are often used to establish an index. The majority of environmental contaminants indices, using linear or non-linear computation techniques, provide all variables separately. The IMs presented in this study are linear and will facilitate the estimation of MPs and NPs exposure experienced by ecosystems and humans. This study can serve as a foundation for the process of developing body burden benchmarks for effects MPs and NPs in different environment compartments. The summary of these models is presented in Table 1. Detailed interpretation or description of the index models is done in sections 2.1 to 2.3.
Type/purpose | Matrix | Model name | Formulae | Classification |
Contamination or accumulation and pollution load assessment | Water, soil, air or food item | Plastic particle contamination factors (pCf) polymer-based particle contamination factors (ppCf) |
pCfi=pipb | pPCf < 1: low contamination, 1≤pCf/ppCf < 3: moderately contaminated, 3≤ pCf/ppCf ≤ 6: considerably contaminated pCf/ppCf ≥ 6 very highly contaminated while for surface water, soil and air (deposited MPs/NPs), the contamination factors can be categorized as values with pPCf < 100: low contamination, 100≤pCf/ppCf < 300: moderately contaminated, 300≤pCf/ppCf ≤ 600: considerably contaminated and pCf/ppCf ≥ 600: very highly contaminated. |
ppCfi=pmipmb | ||||
Plastic/polymer-based pollution load index (pPLI) | pPLI=(ppCf1×ppCf2×ppCf3…ppCfn)1n | pPLI > 1: polluted pPLI < 1: not polluted |
||
Marine organism | plastic- bioconcentration or accumulation factors (pBCf or pBAf) | pBCfi=pi−organismorbiotapb−water | pBCf/pBSAf/ ppBCf/ppBSAf < 1: low accumulation 1 ≤ pBCf/pBSAf/ ppBCf/ppBSAf < 3: moderate accumulation |
|
plastic-biota-sediment accumulation factor (pBSAf) | pBSAfi=pi−organismorbiotapb−sediment | 3≤ pBCf/pBSAf/ ppBCf/ppBSAf ≤ 6: considerable pBCf/pBSAf/ ppBCf/ppBSAf > 6: very highly accumulated. |
||
plastic polymer biota- bioconcentration or accumulation factors (ppBCf) | ppBCfi=pi−organismorbiotapb−water | |||
plastic polymer biota-sediment accumulation factor (ppBSAf) | pBSAfi=pi−organismorbiotapb−sediment | |||
Biota accumulation load index (BALI) | BALI=(ppBCf1×ppBCf2×ppBCf3………ppBCfn)1n | BALI > 1: polluted BALI < 1: not polluted |
||
BALI=(ppBSAf1×ppBSAf2×ppBSAf3………ppBSAfn)1n | ||||
Polymeric risks assessment (ecological risks of MPs/NPs) | Ecological systems | polymer risks indices (pRi) | pRi=∑(pmipT×SJ) | pRi < 150: low risks, pRi of 150 to < 300: medium risks pRi of 300 to < 600: considerable risks pRi of 600 to 1200: high risks pRi > 1200: very high risks |
polymer ecological risks index (pERI) | pERIi=pRi×ppCfi pERIi=pRi×ppBCfiorppBSAfi |
For pERI: Class I: < 10, class II:10–100, Class III: 101–1,000, Class IV: 1,001–10,000 Class V: > 10,000 The classes correspond to low, medium, considerable, high and very high polymeric risks to ecological systems. |
||
Health risks assessment | Plastic Estimated daily intake (pEDI) | Exposure from oral ingestion | pEDIoral=piorpmi×RIBw | For pEDI, when > 1, it indicates high intake of MPs or NPs low when < 1. |
Exposure from dermal routes | pEDIDermal=pmi×SA×dp×ABS×ET×FE×DE×CFBw×AT | |||
Exposure from inhalation routes | pEDIinhalation=pi×PM10×DAIR×PIAF×ET×FSPO×CF×ED×EFBw×AT | |||
Cancer risks | Plastic carcinogenic risks (pCR) | pCRoralordermalorinhalation=EDIoralordermalorinhalation×CSForalordermalorinhalationpCRt=pCRoral+pCRdermal+pCRinhalation |
The extent of contamination and pollution load of water (including drinking water), soil, air and food items by MPs and NPs can be estimated using contamination models such as plastic particle contamination factors (pCf) and pollution load index (pPLI) in the media presented in Eqs 1 and 3.
pCfi=pipb | (1) |
The "pi" refers to the quantity of MPs/NPs in the studied matrix (water, soil, air and food) while "pb" is the quantity of MPs/NPs in background or reference matrix. Background values from samples taken previous to the fast growth of the plastics sector would be the best candidates [17].
The pCf can be computed based on the type of polymer identified in the media. Therefore, Eq1 can be written as Eq 2.
ppCfi=pmipmb | (2) |
Where "ppCf" is the polymer based plastic contamination factors, "pmi" is the quantity of a specific polymer (e.g., PET, PE, PVC etc) identified in the matrix and "pmb" is quantity of a specific polymer (e.g., PET, PE, PVC etc) identified in the reference or background matrix.
For drinking water and food products, contamination factors are classified as follows: pPCf less than 1 indicates minimal contamination, pCf/ppCf between 1 and 3 indicates moderate contamination, between 3 and 6 indicates considerable pollution, and when greater than 6 indicates very high contamination while For surface water, soil, and air (deposited MPs/NPs), contamination factors are classified as follows: pPCf less than 100 indicates low contamination, when ranged from100 to 300 indicates moderate contamination, between 301 to 600 indicates considerable pollution, and pCf/ppCf greater than 600 indicates very high contamination.
The polymer based pollution load index (pPLI) can then be computed as the nth root of the product of individual ppCf, presented in Eq 3. The matrix can be said to be polluted when pPLI > 1 and not polluted when pPLI < 1.
pPLI=(ppCf1×ppCf2×ppCf3………ppCfn)1n | (3) |
Plants, animals, and other creatures that live in saltwater or the brackish water of coastal estuaries are referred to as marine life. MPs and NPs are present in all ecosystem's components.
Biota may and will interact with them from the soil, water, or air. Many MP studies have reported the presence of MPs/NPs in more than 220 diverse marine organism including marine mammals, fish, invertebrates and fish-eating birds etc [10]. The type, size, density, abundance, shape, and color of MPs/NPs all influence their bioavailability and therefore their toxicological impact on biota. Models such as the bioconcentration or accumulation factors (BCf/BAf) and biota-sediment accumulation factor (BSAf) can be applied to evaluate the level of accumulation of MPs/NPs in biota.
In surface water, the plastic- bioconcentration or accumulation factors (or simply pBCf or pBAf) can be defined as the ratio of a plastic particle quantity or concentration in an organism to the plastic particle aqueous concentration or quantity. "pBCf" can be expressed in units of liter per kilogram (ratio of mg of MPs/NPs per kg of organism to mg of MPs/NPs per liter of water). The expression for this model is presented in Eq 4, where pi is quantity or concentrations of MPs or NPs counted in organism or biota and water.
pBCfi=pi−organismorbiotapb−water | (4) |
However, some organisms exist or live in the benthic. Deposited sediments are often a local sink for tiny plastic particles, increasing the exposure for sediment related biota that indiscriminately forage. Bioavailable plastic particle concentrations in sediment are required to assess food chain transmission and possible toxicity. The bioavailable particle may be determined directly by collecting and studying benthic invertebrates or approximated using the plastic-biota-sediment accumulation factor (pBSAf) described in Eq 5.
pBSAfi=pi−organismorbiotapb−sediment | (5) |
The pBCf and pBSAf can be calculated based on the different types of polymer identified in the organism and water or sediment. Therefore, it can be considered plastic polymer bioconcentration or accumulation factor (ppBCf/BAf) and plastic polymer biota-sediment accumulation factor (ppBSAf) presented in Eqs 6 and 7, Where; "pmi-organism/biota" is quantity of a specific polymer (e.g., PET, PE, PVC etc) identified in the organism and "pmi-water or sediment" is quantity of a specific polymer (e.g., PET, PE, PVC etc) identified in the water or sediment.
ppBCfi=pi−organismorbiotapb−water | (6) |
pBSAfi=pi−organismorbiotapb−sediment | (7) |
These models may be considered a simple observed ratio or the predicted value of a partitioning model. A partitioning model is based on the assumptions that particles divide between water and aquatic organisms, as well as the notion that the organisms and the aquatic environment in which they are located are in equilibrium.
However, the pBCf/pBSAf/ ppBCf/ppBSAf can be categorized low accumulation when < 1, moderate when 1 ≤ pBCf/pBSAf/ ppBCf/ppBSAf < 3, considerable when 3≤ pBCf/pBSAf/ ppBCf/ppBSAf ≤ 6 and pBCf/pBSAf/ ppBCf/ppBSAf > 6 very highly accumulated.
The overall accumulation load of polymer in the organism can be estimated using biota accumulation load index (BALI) presented in Eq 8 for water and Eq 9 for sediment. This is computed as the nth root of the product of individual accumulaton factors. The biota can be said to be polluted when BALI > 1 and not polluted when BALI < 1.
BALI=(ppBCf1×ppBCf2×ppBCf3………ppBCfn)1n | (8) |
BALI=(ppBSAf1×ppBSAf2×ppBSAf3………ppBSAfn)1n | (9) |
Due to the large sub-atomic scale of the plastic polymer, it is regarded as biochemically inert, and therefore is not seen as hazardous to the environment or human health. However, polymerisation reactions are seldom complete, and therefore unreacted residual monomers may be discovered in the polymeric material, some of which are hazardous to human health and the environment or may have an effect on the polymer's characteristics [18]. The remaining monomer content varies considerably depending on the kind of polymer, the polymerization process, and the methods used to reduce residual monomer content [19]. Arajo [19] reported in their review that residual monomer substance levels for various polymers varied from none or very low levels (100 ppm; for example, 0.0001 percent) to up to 40,000 ppm during an audit (for example 4 percent). Apart from the residual monomers, additional polymerisation pollutants may be present in a plastic object. These include oligomers, low-atomic-weight polymer segments, catalyst residues, and polymerisation solvents, as well as a broad range of plastic additives, such as handling aids and final product additives [20]. Due to the fact that the majority of these non-polymeric segments have a low atomic weight, they may migrate from the plastic item to the environment and then into humans, where they may cause damage.
The ecological risks of MPs/NPs can be determined based on polymer types and their chemical toxicity coefficient or risk scores. The formulae for calculating the polymer risks indices ("pRi") and for computing the polymer risks indices for overall samples (pRoverall) are given in Eqs 10 and 11. Kabir and colleagues [21] recently presented and utilized these models. "pRi" is a tool for assessing the polymeric hazards posed by MPs/NPs to environmental systems. "pRoverall", which assesses the overall polymeric risks for all samples, is calculated as the nth root of the polymer risks indices products, which is the root of the number of samples.
pRi=∑(pmipT×SJ) | (10) |
pRoverall=(pR1×pR2×pR3………pRn)1n | (11) |
The "pmi" column represents the number of distinct MPs/NPs polymers discovered in sample i whereas the "pT" column represents the total number of distinct MPs/NPs polymers identified in sample i. The "Sj" is the chemical toxicity coefficient or risk score, which is derived from hazard ratings for various polymers. These ratings are given based on their toxicity to ecosystems and may be found in ref [20].
The polymeric risk indices (pRi) are classified as low when they are less than 150, medium when they are between 150 and 300, significant when they are between 300 and 600, high when they are more than 600 and very high when they are greater than 1200 [21].
The polymer ecological risks index (pERI) can be calculated to estimates the risks of MPs/NPs to ecological systems including biota in relation to the extent of contamination. The pERI are presented in the Eq 12 for other matrix and Eq 13 for biota while for overall samples Eq 14 can be applied.
pERIi=pRi×ppCfi | (12) |
pERIi=pRi×ppBCfiorppBSAfi | (13) |
pERIoverall=(pERI1×pEPRI2×pEPRI3×…….pERIn)1/n | (14) |
The MPs/NPs pollution risk index (pERI) is categorized as follows: Class I when it is less than 10, Class II when it is between 10 and 100, Class III when it is between 101 and 1,000, Class IV when it is between 1,000 and 10,000, and Class V when it is more than 10,000 [21]. The classifications correlate to polymeric hazards to ecological systems that are modest, medium, substantial, high, and very high.
However, higher MPs pollution risks and levels have been shown to be highly dependent on the presence of hazardous MPs polymers, just as the high MPs pollution loading index. Studies by [21,22,23] have recently described and applied these models in evaluating real-world data.
An individual risk pathway as a result of human exposure to MPs/NPs contamination of water/food/soil/dusts/air/biota could be through either oral ingestion, dermal or inhalation or collectively. All "pEDI" can be calculated for different age groups (i.e adults and children). It is worthy to note that the different values presented for the different parameters might vary with geological area. "pEDI" are interpreted based values greater or lesser than 1. When > 1, it indicates high intake of MPs or NPs and low when < 1.
It is also possible that humans will be exposed to MPs or NPs directly if they consume these particles in drinking water or other foods like honey, beer, or table salt [10]. One more direct method of consuming MPs is via atmospheric fallout from indoor airborne MPs when eating in a public place. This route of exposure was exposed by [24] in their study, and they predicted that individuals would inject MPs ranging from 13,731 to 68,415 particles each year in this scenario. In a similar vein, [25] calculated that people may absorb about 80 g of MPs per day via plant consumption.
To evaluate the daily intake of MPs or NPs via oral ingestion can be achieved by applying Eq 15, which can be calculated on number of particle ("pi") basis as well as type of polymer ("pmi"). pEDIoral can be computed for water (in particle/kg-day), and soil.
pEDIoral=piorpmi×RIBw | (15) |
Where "pi" or "pmi" remains as described previously, RI: ingestion rate for water is (2.2 L/day for adults; 1.8 L/day for children) while for soil (100 mg/day); BW: average body weight [26].
Study of [23] evaluated the risks from oral ingestion of MPs from bottled water and reported pEDI of less than 1 for both adult and children. This study showed that this IMs can be applied for evaluating actual data from environmental process.
There is no evidence to support the notion that MPs or NPs may be ingested via dermal or inhalation, although some investigations have indicated that this is possible. It is possible that dermal routes of exposure will need to be calculated since skin pores, although they vary by race and age, have been found to range from 40 to 500 microns in size on average [27,28]. As a result, MPs/NPs may possibly fit into these pores with ease, which can occur as a result of atmospheric deposition on the skin, recreational activities such as swimming [22], or via the use of personal care products (face wash, scrubs, and soap), among other sources [10].
To evaluate the daily intake of MPs or NPs via dermal routes can be achieved by applying Eq 16,
pEDIDermal=pmi×SA×dp×ABS×ET×FE×DE×CFBw×AT | (16) |
where, "FE" (365 days/year) is the exposure frequency;
"DE" (70 years for adults; and 6 years for children) is the exposure duration
"AT" (365 days/year × 70 years for an adult; 365 days/year × 6 years for a child) is the averaging time,
"SA" (18000 cm2) is the skin area available for contact,
:dp" (cm/hour) is the pore diffusion coefficient which is 0.001 if the polymer particle is in the soils/dusts and for water, it can be calculated using Eqs17 and 18;
"ABS" (unitless) is the dermal absorption factor (0.001);
"ET" (0.58 h/event) is the exposure time while CF (0.001 L/cm3) is the unit conversion factor [29].
dp=D/τ | (17) |
The tortuosity factor ("τ") is a relationship between the diffusivity of adsorbate in the pore and the diffusivity of free solution. Considering that the pore diffusion coefficient ("D") is directly proportional to the molecular diffusivity of the adsorbate ("D") [30], it may be treated as a unit (1). Wilke and Chang [31] provided Eq 18 as an example of how to calculate the pore diffusion coefficient.
dp=D=7.4×10−8(ϕM)0.5TηbV0.6mi | (18) |
Where, ϕ: is a relationship between the effective molecular weight of water and the diffusion process; it has the value 2.6.,
"M": solvent molecular weight (water: 18.01528 g/mol),
"T": temperature (K),
"ηb": solution viscosity (cp),
"Vmi": polymer molecular volume (cm3 /mol), although it varies according to polymer and temperature, it may be calculated as the ratio of molar mass to density of the polymer.
When all parameter is obtained and fitted in Eq 18, "dp" can then be determined.
MPs in the air are inhaled at varying rates according on their size, which also influences their capacity to penetrate the respiratory system. Thus, in certain instances, the particle may be both inhalable (capable of depositing in the upper airways after entering via the nose) and respirable (capable of reaching and depositing in the deep lung) [32,33]. MPs that are fibrous and inhalable do not satisfy the World Health Organization's definition of fiber, which requires a length more than 5 microns, a diameter less than 3 microns, and a length-to-diameter ratio higher than 3:1. The World Health Organization (WHO) published a report on this subject in 1997 [34].
As a consequence, the estimated daily intake (EDI) associated with exposure to MPs/NPs through inhalation routes may be calculated using Eq 19.
pEDIinhalation=pi×PM10×DAIR×PIAF×ET×FSPO×CF×ED×EFBw×AT | (19) |
Where;
"CF" is the conversion factor (10−6 kg/mg),
"PM10" is the content of inhalable particulates in ambient air (0.15 mg/m3),
"DAIR" is Daily air inhalation rate (14.5 m3 /day),
"PIAF" is the Retention fraction of inhaled particulates in body (0.75) and
"FSPO" is the fraction of soil-borne particulates in air (0.5).
It is possible to calculate the incremental risk of getting cancer over the course of a person's lifetime by consuming MPs/NPs polymers. Eq 20 show how to do this by multiplying the anticipated daily intake by a cancer slope factor (CSF), whereas Eq 21 show how to calculate the overall risks from all routes (21). In the context of quantitative risk assessment of chemicals or substances being assessed as carcinogens, the cancer slope factor (CSF) is a metric that emerges. It is a measure of the chance of developing cancer as a result of a lifetime exposure to an agent. U.S. Environmental Protection Agency provides CSF for several monomers including vinyl chloride (PVC), ethylene oxide (e.g., PE and PET), and styrene (PS), among others [35,36].
pCRoralordermalorinhalation=EDIoralordermalorinhalation×CSForalordermalorinhalation | (20) |
pCRt=pCRoral+pCRdermal+pCRinhalation | (21) |
Recently, Enyoh [22] used the pCR model on data of MPs collected in five rivers in Southeastern, Nigeria. They compared the data obtained with the U.S Environmental Protection Agency regulatory the acceptable cancer risk range is between 1×10−6 to 1×10−4. Results from their study showed that PCR via oral pathway was high for all polymer types (PE, PP, PVC, PS and PET) for both adult and children. They concluded that both adult and children population were at risk of carcinogenic effects from oral of the different MP polymers. However, via dermal pathway, they found low risks since the values were comparable with the acceptable range. This study demonstrated the applicability of this model.
The index models described in the paper can be applied for studies on MPs/NPs in different matrix. The models are can be easily applied when all parameters have been determined. It is recommended that future studies on MPs/NPs should apply models. When it comes to environmental problems, information from models is seen as simplified ideas. To make things easier for those making choices, environmental problems are simply expressed in easy-to understand terms.
There are no conflicts of interest to declare.
This paper was presented at the International Symposium on Plastic Pollution held on 28th and 29th October 2021 with the theme of Removal, Analysis, and Risk Assessment of Microplastics. The symposium was organized by the University of Seoul & Hanyang University, South Korea with the support of the Ministry of Environment & KEITI, South Korea.
This study was partially supported by the Special Funds for Innovative Area Research (No.20120015, FY 2008-FY2012) and Basic Research (B) (No. 24310005, FY2012-FY2014; No.18H03384, FY2017 FY2020) of Grant-in-Aid for Scientific Research of Japanese Ministry of Education, Culture, Sports, Science and Technology (MEXT) and the Steel Foundation for Environmental Protection Technology of Japan (No. C-33, FY 2015-FY 2017).
[1] | L. Page, S. Brin, R. Motwami, T. Winograd, The PageRank citation ranking: Bringing order to the web, Technical report, Computer Science Department, Stanford University, Stanford, CA, 1999. |
[2] | S. Kamvar, T. Haveliwala, C. Manning, G. H. Golub, Exploiting the block structure of the web for computing PageRank, Technical Report, SCCM-03-02, Stanford University, 2003. |
[3] | G. H. Golub, C. F. Van Loan, Matrix Computations, third ed., The Johns Hopkins University Press, Baltimore, London, 1996. |
[4] |
D. Gleich, A. Gray, C. Greif, T. Lau, An inner-outer iteration for computing PageRank, SIAM J. Sci. Comput., 32 (2010), 349-371. doi: 10.1137/080727397
![]() |
[5] | Z. L. Tian, Y. Liu, Y. Zhang, Z. Y. Liu, M. Y. Tian, The general inner-outer iteration method based on regular splittings for the PageRank problem, Appl. Math. Comput., 356 (2019), 479-501. |
[6] |
X. Y. Tan, A new extrapolation method for pagerank computations, J. Comput. Appl. Math., 313 (2017), 383-392. doi: 10.1016/j.cam.2016.08.034
![]() |
[7] |
G. H. Golub, C. Greif, An Arnoldi-type algorithm for computing PageRank, BIT., 46 (2006), 759-771. doi: 10.1007/s10543-006-0091-y
![]() |
[8] |
G. Wu, Y. Wei, A Power-Arnoldi algorithm for computing pagerank, Numer. Linear Algebra Appl., 14 (2007), 521-546. doi: 10.1002/nla.531
![]() |
[9] |
R. Morgan, M. Zheng, A harmonic restarted Arnoldi algorithm for calculating eigenvalues and determining multiplicity, Linear Algebra Appl., 415 (2006), 96-113. doi: 10.1016/j.laa.2005.07.024
![]() |
[10] |
Q. Y. Hu, C. Wen, T. Z. Huang, Z. L. Shen, X. M. Gu, A variant of the Power-Arnoldi algorithm for computing PageRank, J. Comput. Appl. Math., 381 (2021), 113034. doi: 10.1016/j.cam.2020.113034
![]() |
[11] |
A. Essai, Weighted FOM and GMRES for solving nonsymmetric linear systems, Numer. Algorithms, 18 (1998), 277-292. doi: 10.1023/A:1019177600806
![]() |
[12] | H. S. Najafi, H. Ghazvini, Weighted restarting method in the weighted Arnoldi algorithm for computing the eigenvalues of a nonsymmetric matrix, Appl. Math. Comput., 175 (2006), 1276-1287. |
[13] |
J. F. Yin, G. J. Yin, M. Ng, On adaptively accelerated Arnoldi method for computing PageRank, Numer. Linear Algebra Appl., 19 (2012), 73-85. doi: 10.1002/nla.789
![]() |
[14] |
C. Wen, Q. Y. Hu, G. J. Yin, X. M. Gu, Z. L. Shen, An adaptive Power-GArnoldi algorithm for computing PageRank, J. Comput. Appl. Math., 386 (2021), 113209. doi: 10.1016/j.cam.2020.113209
![]() |
[15] |
Z. X. Jia, Refined iterative algorithms based on Arnoldi's process for large unsymmetric eigenproblems, Linear Algebra Appl., 259 (1997), 1-23. doi: 10.1016/S0024-3795(96)00238-8
![]() |
[16] | A. Langville, C. Meyer, Googles PageRank and Beyond: The Science of the Search Engine Rankings, Princeton University Press, 2006. |
[17] | T. Haveliwala, S. Kamvar, The second eigenvalue of the google matrix, in: Proceedings of the Twelfth International World Wide Web of Conference, 2003. |
[18] | F. Tudisco, C. Di Fiore, A preconditioning approach to the PageRank computation problem, Linear Algebra Appl., 435 (2011), 2222-2246. |
[19] |
S. Cipolla, C. Di Fiore, F. Tudisco, Euler-Richardson method preconditioned by weakly stochastic matrix algebras: A potential contribution to Pagerank computation, Electronic J. Linear Al., 32 (2017), 254-272. doi: 10.13001/1081-3810.3343
![]() |
[20] |
G. Wu, Y. C. Wang, X. Q. Jin, A preconditioned and shifted GMRES algorithm for the PageRank problem with multiple damping factors, SIAM J. Sci. Comput., 34 (2012), 2558-2575. doi: 10.1137/110834585
![]() |
1. | Hongyan Guo, Chengkang Xu, Restricted modules for gap-p Virasoro algebra and twisted modules for certain vertex algebras, 2023, 227, 00224049, 107322, 10.1016/j.jpaa.2023.107322 | |
2. | Hongyan Guo, Huaimin Li, Restricted modules and associated vertex algebras of extended Heisenberg-Virasoro algebra, 2023, 635, 00218693, 463, 10.1016/j.jalgebra.2023.07.044 |
Type/purpose | Matrix | Model name | Formulae | Classification |
Contamination or accumulation and pollution load assessment | Water, soil, air or food item | Plastic particle contamination factors (pCf) polymer-based particle contamination factors (ppCf) |
pCfi=pipb | pPCf < 1: low contamination, 1≤pCf/ppCf < 3: moderately contaminated, 3≤ pCf/ppCf ≤ 6: considerably contaminated pCf/ppCf ≥ 6 very highly contaminated while for surface water, soil and air (deposited MPs/NPs), the contamination factors can be categorized as values with pPCf < 100: low contamination, 100≤pCf/ppCf < 300: moderately contaminated, 300≤pCf/ppCf ≤ 600: considerably contaminated and pCf/ppCf ≥ 600: very highly contaminated. |
ppCfi=pmipmb | ||||
Plastic/polymer-based pollution load index (pPLI) | pPLI=(ppCf1×ppCf2×ppCf3…ppCfn)1n | pPLI > 1: polluted pPLI < 1: not polluted |
||
Marine organism | plastic- bioconcentration or accumulation factors (pBCf or pBAf) | pBCfi=pi−organismorbiotapb−water | pBCf/pBSAf/ ppBCf/ppBSAf < 1: low accumulation 1 ≤ pBCf/pBSAf/ ppBCf/ppBSAf < 3: moderate accumulation |
|
plastic-biota-sediment accumulation factor (pBSAf) | pBSAfi=pi−organismorbiotapb−sediment | 3≤ pBCf/pBSAf/ ppBCf/ppBSAf ≤ 6: considerable pBCf/pBSAf/ ppBCf/ppBSAf > 6: very highly accumulated. |
||
plastic polymer biota- bioconcentration or accumulation factors (ppBCf) | ppBCfi=pi−organismorbiotapb−water | |||
plastic polymer biota-sediment accumulation factor (ppBSAf) | pBSAfi=pi−organismorbiotapb−sediment | |||
Biota accumulation load index (BALI) | BALI=(ppBCf1×ppBCf2×ppBCf3………ppBCfn)1n | BALI > 1: polluted BALI < 1: not polluted |
||
BALI=(ppBSAf1×ppBSAf2×ppBSAf3………ppBSAfn)1n | ||||
Polymeric risks assessment (ecological risks of MPs/NPs) | Ecological systems | polymer risks indices (pRi) | pRi=∑(pmipT×SJ) | pRi < 150: low risks, pRi of 150 to < 300: medium risks pRi of 300 to < 600: considerable risks pRi of 600 to 1200: high risks pRi > 1200: very high risks |
polymer ecological risks index (pERI) | pERIi=pRi×ppCfi pERIi=pRi×ppBCfiorppBSAfi |
For pERI: Class I: < 10, class II:10–100, Class III: 101–1,000, Class IV: 1,001–10,000 Class V: > 10,000 The classes correspond to low, medium, considerable, high and very high polymeric risks to ecological systems. |
||
Health risks assessment | Plastic Estimated daily intake (pEDI) | Exposure from oral ingestion | pEDIoral=piorpmi×RIBw | For pEDI, when > 1, it indicates high intake of MPs or NPs low when < 1. |
Exposure from dermal routes | pEDIDermal=pmi×SA×dp×ABS×ET×FE×DE×CFBw×AT | |||
Exposure from inhalation routes | pEDIinhalation=pi×PM10×DAIR×PIAF×ET×FSPO×CF×ED×EFBw×AT | |||
Cancer risks | Plastic carcinogenic risks (pCR) | pCRoralordermalorinhalation=EDIoralordermalorinhalation×CSForalordermalorinhalationpCRt=pCRoral+pCRdermal+pCRinhalation |
Type/purpose | Matrix | Model name | Formulae | Classification |
Contamination or accumulation and pollution load assessment | Water, soil, air or food item | Plastic particle contamination factors (pCf) polymer-based particle contamination factors (ppCf) |
pCfi=pipb | pPCf < 1: low contamination, 1≤pCf/ppCf < 3: moderately contaminated, 3≤ pCf/ppCf ≤ 6: considerably contaminated pCf/ppCf ≥ 6 very highly contaminated while for surface water, soil and air (deposited MPs/NPs), the contamination factors can be categorized as values with pPCf < 100: low contamination, 100≤pCf/ppCf < 300: moderately contaminated, 300≤pCf/ppCf ≤ 600: considerably contaminated and pCf/ppCf ≥ 600: very highly contaminated. |
ppCfi=pmipmb | ||||
Plastic/polymer-based pollution load index (pPLI) | pPLI=(ppCf1×ppCf2×ppCf3…ppCfn)1n | pPLI > 1: polluted pPLI < 1: not polluted |
||
Marine organism | plastic- bioconcentration or accumulation factors (pBCf or pBAf) | pBCfi=pi−organismorbiotapb−water | pBCf/pBSAf/ ppBCf/ppBSAf < 1: low accumulation 1 ≤ pBCf/pBSAf/ ppBCf/ppBSAf < 3: moderate accumulation |
|
plastic-biota-sediment accumulation factor (pBSAf) | pBSAfi=pi−organismorbiotapb−sediment | 3≤ pBCf/pBSAf/ ppBCf/ppBSAf ≤ 6: considerable pBCf/pBSAf/ ppBCf/ppBSAf > 6: very highly accumulated. |
||
plastic polymer biota- bioconcentration or accumulation factors (ppBCf) | ppBCfi=pi−organismorbiotapb−water | |||
plastic polymer biota-sediment accumulation factor (ppBSAf) | pBSAfi=pi−organismorbiotapb−sediment | |||
Biota accumulation load index (BALI) | BALI=(ppBCf1×ppBCf2×ppBCf3………ppBCfn)1n | BALI > 1: polluted BALI < 1: not polluted |
||
BALI=(ppBSAf1×ppBSAf2×ppBSAf3………ppBSAfn)1n | ||||
Polymeric risks assessment (ecological risks of MPs/NPs) | Ecological systems | polymer risks indices (pRi) | pRi=∑(pmipT×SJ) | pRi < 150: low risks, pRi of 150 to < 300: medium risks pRi of 300 to < 600: considerable risks pRi of 600 to 1200: high risks pRi > 1200: very high risks |
polymer ecological risks index (pERI) | pERIi=pRi×ppCfi pERIi=pRi×ppBCfiorppBSAfi |
For pERI: Class I: < 10, class II:10–100, Class III: 101–1,000, Class IV: 1,001–10,000 Class V: > 10,000 The classes correspond to low, medium, considerable, high and very high polymeric risks to ecological systems. |
||
Health risks assessment | Plastic Estimated daily intake (pEDI) | Exposure from oral ingestion | pEDIoral=piorpmi×RIBw | For pEDI, when > 1, it indicates high intake of MPs or NPs low when < 1. |
Exposure from dermal routes | pEDIDermal=pmi×SA×dp×ABS×ET×FE×DE×CFBw×AT | |||
Exposure from inhalation routes | pEDIinhalation=pi×PM10×DAIR×PIAF×ET×FSPO×CF×ED×EFBw×AT | |||
Cancer risks | Plastic carcinogenic risks (pCR) | pCRoralordermalorinhalation=EDIoralordermalorinhalation×CSForalordermalorinhalationpCRt=pCRoral+pCRdermal+pCRinhalation |