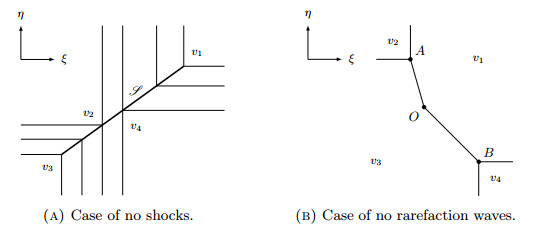
Bioencapsulation in alginate capsules offers an interesting opportunity for the efficient delivery of microbial inoculants for agricultural purposes. The present study evaluated the ionic gelation technique to prepare beads loaded with two plant growth-promoting bacteria (PGPB), Bacillus thuringiensis strain B25 and Pantoea agglomerans strain Pa in 1% alginate supplemented with 5mM proline as an osmoprotectant. Capsule morphology, survival rate, encapsulation efficiency, and viability during 24 months of storage as well as the stability of PGP activities were studied. Our results indicate that more than 99% of bacteria were effectively trapped in the alginate beads, which successfully released live bacteria after 60 days of storage at room temperature. A considerable survival of B. thuringiensis B25 throughout the storage period was detected, while the inoculated concentration of 8.72 × 109 (±0.04 ×109) CFU/mL was reduced to 99.9% for P. agglomerans Pa after 24 months of storage. Notably, a higher survival of individually encapsulated bacteria was observed compared to their co-inoculation. The colonization capacity of model plant Arabidopsis thaliana roots by free and encapsulated bacteria was detected by the triphenyltetrazolium chloride test. Moreover, both strains effectively colonized the rhizosphere, rhizoplane, and endosphere of durum wheat plants and exerted a remarkable improvement in plant growth, estimated as a significant increase in the quantities of total proteins, sugars, and chlorophyll pigments, besides roots and shoots length. This study demonstrated that alginate-encapsulated B. thuringiensis B25 and P. agglomerans Pa could be used as inoculants in agriculture, as their encapsulation ensures robust protection, maintenance of viability and PGP activity, and controlled bacterial biostimulant release into the rhizosphere.
Citation: Amel Balla, Allaoua Silini, Hafsa Cherif-Silini, Francesca Mapelli, Sara Borin. Root colonization dynamics of alginate encapsulated rhizobacteria: implications for Arabidopsis thaliana root growth and durum wheat performance[J]. AIMS Microbiology, 2025, 11(1): 87-125. doi: 10.3934/microbiol.2025006
[1] | Caterina Balzotti, Simone Göttlich . A two-dimensional multi-class traffic flow model. Networks and Heterogeneous Media, 2021, 16(1): 69-90. doi: 10.3934/nhm.2020034 |
[2] | Mohamed Benyahia, Massimiliano D. Rosini . A macroscopic traffic model with phase transitions and local point constraints on the flow. Networks and Heterogeneous Media, 2017, 12(2): 297-317. doi: 10.3934/nhm.2017013 |
[3] | Michael Herty, Adrian Fazekas, Giuseppe Visconti . A two-dimensional data-driven model for traffic flow on highways. Networks and Heterogeneous Media, 2018, 13(2): 217-240. doi: 10.3934/nhm.2018010 |
[4] | Benjamin Seibold, Morris R. Flynn, Aslan R. Kasimov, Rodolfo R. Rosales . Constructing set-valued fundamental diagrams from Jamiton solutions in second order traffic models. Networks and Heterogeneous Media, 2013, 8(3): 745-772. doi: 10.3934/nhm.2013.8.745 |
[5] | Felisia Angela Chiarello, Paola Goatin . Non-local multi-class traffic flow models. Networks and Heterogeneous Media, 2019, 14(2): 371-387. doi: 10.3934/nhm.2019015 |
[6] | Fabio Della Rossa, Carlo D’Angelo, Alfio Quarteroni . A distributed model of traffic flows on extended regions. Networks and Heterogeneous Media, 2010, 5(3): 525-544. doi: 10.3934/nhm.2010.5.525 |
[7] | Cécile Appert-Rolland, Pierre Degond, Sébastien Motsch . Two-way multi-lane traffic model for pedestrians in corridors. Networks and Heterogeneous Media, 2011, 6(3): 351-381. doi: 10.3934/nhm.2011.6.351 |
[8] | Paola Goatin, Chiara Daini, Maria Laura Delle Monache, Antonella Ferrara . Interacting moving bottlenecks in traffic flow. Networks and Heterogeneous Media, 2023, 18(2): 930-945. doi: 10.3934/nhm.2023040 |
[9] | Tibye Saumtally, Jean-Patrick Lebacque, Habib Haj-Salem . A dynamical two-dimensional traffic model in an anisotropic network. Networks and Heterogeneous Media, 2013, 8(3): 663-684. doi: 10.3934/nhm.2013.8.663 |
[10] | Shimao Fan, Michael Herty, Benjamin Seibold . Comparative model accuracy of a data-fitted generalized Aw-Rascle-Zhang model. Networks and Heterogeneous Media, 2014, 9(2): 239-268. doi: 10.3934/nhm.2014.9.239 |
Bioencapsulation in alginate capsules offers an interesting opportunity for the efficient delivery of microbial inoculants for agricultural purposes. The present study evaluated the ionic gelation technique to prepare beads loaded with two plant growth-promoting bacteria (PGPB), Bacillus thuringiensis strain B25 and Pantoea agglomerans strain Pa in 1% alginate supplemented with 5mM proline as an osmoprotectant. Capsule morphology, survival rate, encapsulation efficiency, and viability during 24 months of storage as well as the stability of PGP activities were studied. Our results indicate that more than 99% of bacteria were effectively trapped in the alginate beads, which successfully released live bacteria after 60 days of storage at room temperature. A considerable survival of B. thuringiensis B25 throughout the storage period was detected, while the inoculated concentration of 8.72 × 109 (±0.04 ×109) CFU/mL was reduced to 99.9% for P. agglomerans Pa after 24 months of storage. Notably, a higher survival of individually encapsulated bacteria was observed compared to their co-inoculation. The colonization capacity of model plant Arabidopsis thaliana roots by free and encapsulated bacteria was detected by the triphenyltetrazolium chloride test. Moreover, both strains effectively colonized the rhizosphere, rhizoplane, and endosphere of durum wheat plants and exerted a remarkable improvement in plant growth, estimated as a significant increase in the quantities of total proteins, sugars, and chlorophyll pigments, besides roots and shoots length. This study demonstrated that alginate-encapsulated B. thuringiensis B25 and P. agglomerans Pa could be used as inoculants in agriculture, as their encapsulation ensures robust protection, maintenance of viability and PGP activity, and controlled bacterial biostimulant release into the rhizosphere.
In this paper, we are concerned with the study of a two-dimensional multi-class traffic model. This work is placed in the constantly evolving framework of mathematical models for traffic flow. The goal of traffic models is to provide tools capable of helping traffic management, in order to optimize transport and obtain economic and environmental benefits, such as the reduction of vehicles queues and pollution.
Traffic models are divided into three main categories, which depend on the scale of observation: microscopic, macroscopic and kinetic models. Microscopic models follow the dynamics of each vehicle and are described by ordinary differential equations (ODEs), see e. g. [2,16,19,33,34,38]. Macroscopic models, based on fluid dynamics, consider aggregated quantities such as the density of vehicles and are governed by partial differential equations (PDEs), see e. g. [3,7,12,17,32,41,46]. Kinetic models [24,28,33,36,39,40] are between the previous two classes since they can be derived by microscopic models while macroscopic models can be derived by kinetic descriptions. We refer to [1,15] and references therein for a more complete review on traffic models.
In recent years, the ever-increasing amount of real data, due to new technologies, has widely influenced the research on mathematical models for traffic flow. The common goal of researchers is to exploit the available real data to build ad hoc traffic models, capable of simulating increasingly realistic scenarios. We refer to [8,9,13,37] for some inspiring examples of data-fitted traffic models. The common feature of these models is the application of vehicles trajectory data collected in datasets such as [25,44]. Datasets of this type generally contain data on multi-lane highways and are able to distinguish the type of vehicle.
The focus in this work is on macroscopic traffic models. In particular, we propose a multi-class generalization in two space dimensions of the well-known first order Lighthill-Whitham-Richards (LWR) model [32,41]. First order models such as the LWR are described by a single conservation law
As we have already mentioned, traffic datasets contain information related to multi-lane highways with different types of vehicles. Most traffic models refer to dynamics of single-lane traffic and therefore do not consider the movements related to lane changes. Our aim is to exploit now all the available data, including the line-changing behavior and the different vehicles classes. To this end, we propose an extension of the work by Herty, Fazekas and Visconti for a single-class traffic model [21] to a multi-class traffic model in two space dimensions. The most common approaches which include lane-changing are the two-dimensional models and the multi-lane models. The first approach is an emerging topic, and we refer to [6,21,22] for some examples. The second approach has been used for instance in [26,27], where the authors propose a microscopic, a kinetic and a fluid dynamic model with lane changing. Here, we stick to the two-dimensional approach and incorporate two types of vehicles interacting through the flux functions. The proposed model is then defined by the coupling of LWR-type models for two classes of vehicles in the
The paper is organized as follows. In Section 2 we introduce the traffic model and study the Riemann problems. In Section 3, we describe the numerical scheme and validate the model via numerical tests. In Section 4, we calibrate the model with a German dataset and compare the results with the real trajectories of vehicles. In Section 5, we propose a modified version of the model calibrated with real data, and finally we investigate on the ability of the model to simulate vehicles overtaking compared to a multi-lane model.
In this section, we introduce the traffic model used throughout the paper. Let us consider two classes of vehicles, whose densities are denoted by
{ρt+(qxρ(ρ,μ))x+(qyρ(ρ,μ))y=0μt+(qxμ(ρ,μ))x+(qyμ(ρ,μ))y=0, | (1) |
where
qxρ(ρ,μ)=ρcx(1−(ρ+μrmax))qyρ(ρ,μ)=ρcy(1−(ρ+μrmax))qxμ(ρ,μ)=μcx(1−(ρ+μrmax))qyρ(ρ,μ)=μcy(1−(ρ+μrmax)), | (2) |
where
ux=cx(1−(ρ+μrmax)),uy=cy(1−(ρ+μrmax)). |
Hence,
First of all, we present the properties of model (1). To simplify the notation, we normalize
U=(ρμ),f(U)=(ρcx(1−(ρ+μ))μcx(1−(ρ+μ))),g(U)=(ρcy(1−(ρ+μ))μcy(1−(ρ+μ))) |
and matrices
A(U)=Df(U),B(U)=Dg(U). |
Therefore, we can rewrite system (1) as
Ut+AUx+BUy=0. | (3) |
System (3) is hyperbolic if any linear combination of
λ1=(κ1cx+κ2cy)(1−(ρ+μ)),λ2=(κ1cx+κ2cy)(1−2(ρ+μ)) |
which are real for any couple
γ1=(−11),γ2=(ρ/μ1). |
The first eigenvalue is linearly degenerate, i.e.,
z1=ρ+μ,z2=log(ρ/μ). |
Next, we describe the two-dimensional Riemann problem [10,47] associated with (1). To simplify the computations, we introduce the variable
{rt+(rcx(1−r))x+(rcy(1−r))y=0r(0,x,y)=r0(x,y). | (4) |
The Riemann problem in two dimensions is given by (4) with constant initial data in the four quadrants, i.e.
r0(x,y)={v10<x<∞,0<y<∞v2−∞<x<0,0<y<∞v3−∞<x<0,−∞<y<0v40<x<∞,−∞<y<0. | (5) |
For convenience, we define
Remark 1. The treatment of the two-dimensional Riemann problem proposed in [10] assumes convex flux functions
We look for self-similar solutions
(cx(1−2v)−ξ)vξ+(cy(1−2v)−η)vη=0 | (6) |
which leads us to
(cx(1−2v)−ξ)dη+(cy(1−2v)−η)dξ=0, |
where
The set of singular points parametrized by
S={(ξ,η)|ξ=cx(1−2v),η=cy(1−2v)}. |
Defining
γ(v−,v+)=ˆf(v+)−ˆf(v−)v+−v−=cx(1−v+−v−)ν(v−,v+)=ˆg(v+)−ˆg(v−)v+−v−=cy(1−v+−v−), | (7) |
the Rankine-Hugoniot jump condition is
dηdξ=−ν(v−,v+)−ηγ(v−,v+)−ξ=−cy(1−v+−v−)−ηcx(1−v+−v−)−ξ. | (8) |
Assuming that the normal vector
(γ(v−,v0)−γ(v−,v+))dη+(ν(v−,v0)−ν(v−,v+))dξ=cx(1−v+−v0)dη+cy(1−v+−v0)dξ≥0 | (9) |
for
The initial data in (5) for problem (4) in the variables
limξ/η=const,ξ2+η2→∞v(ξ,η)={v1ξ>0,η>0v2ξ<0,η>0v3ξ<0,η<0v4ξ>0,η<0. | (10) |
The solution of problem (6) with initial data (10) is composed of elementary waves. There are five possible cases: (1) no shocks, (2) no rarefaction waves, (3) exactly one shock, (4) exactly one rarefaction wave, (5) two rarefaction waves and two shocks. In this work we skip the full details of the possible cases and refer to [47] for a detailed discussion. Let us highlight the five cases now:
(1) No shocks: This case is verified when
(2) No rarefaction waves: This case is verified when
(3) Exactly one shock: This case is verified when
v4>v1≥v2≥v3orv2<v3≤v4≤v1. |
The first sub-case is represented in Figure 2(A). Using the Rankine-Hugoniot condition (8) it can be shown that the shock curve is concave, monotonically increasing in
(4) Exactly one rarefaction wave: This case is verified when
v1≤v2≤v3<v4orv2<v1≤v4≤v3. |
The first possibility of initial data gives results similar to the previous case of exactly one shock wave. For the second initial datum there exist several sub-cases but we omit the details. In Figure 3 we show two examples for the two initial data configurations.
(5) Two shocks and two rarefaction waves: This case is verified when
v4>v1≥v3>v2orv4>v3>v1>v2. |
The main difference between the two options of initial data is that in the first case the shock curves are not neighbors while in the second case they are neighbors. There are again several sub-cases, we only show an example of the two possible initial data sets in Figure 4.
In the next section, we introduce a suitable discretization for the multi-class model. For validation purposes of the proposed scheme, we aim to recover the theoretical results from above.
The numerical analysis of system (3) can be done using the dimensional splitting approach [31] which means that the two-dimensional problem is split into two one-dimensional problems. Thus, equation (3) is split into
Ut+AUx=0,Ut+BUy=0. |
We uniformly divide the two-dimensional space
ΔtΔx≤12(maxi,j=1,2{|λi|,|γj|})−1. | (11) |
Then, the time interval
Starting from a given initial datum
Uij∗=Uijn−Δt2Δx(Fni+1/2,j−Fni−1/2,j)Uij∗∗=Uij∗−ΔtΔy(G∗i,j+1/2−G∗i,j−1/2)Uijn+1=Uijn−Δt2Δx(F∗∗i+1/2,j−F∗∗i−1/2,j). |
We use the Local Lax-Friedrichs flux (also known as Rusanov flux) [5,Chapter 3] for
Fi+1/2,j=12(f(Ui+1,j)+f(Ui,j)−αi+1/2,j(Ui+1,j−Ui,j)), |
where
We now test the discretization method for the two-dimensional multi-class model (3) while comparing the numerical results to the theoretical solutions of the Riemann problems introduced in Section 2.1.
Our test setting is given by
ρ0(x,y)={ρ1(x,y)∈(0,5)×(0,5)ρ2(x,y)∈(−5,0)×(0,5)ρ3(x,y)∈(−5,0)×(−5,0)ρ4(x,y)∈(0,5)×(−5,0). |
and
Since we aim to recover the results of the analysis done in Section 2.1, where the plots are defined for the plane
As we have explained in Section 2.1, there are only five possible configurations of the solution, which are determined by the initial values
(1) No shocks: We fix
(2) No rarefaction waves: We fix
(3) Exactly one shock: We fix
(4) Exactly one rarefaction wave: We fix
(5) Two shocks and two rarefaction waves: We fix
In this section, we calibrate the two-dimensional multi-class model with a dataset of real trajectories data. We employ the public German dataset [25] which contains vehicle trajectories data recorded on the German motorway A3, nearby Frankfurt am Main. The analyzed area is a three lanes highway of about 900 meters in length and 12 meters in width, depicted in Figure 6. A system of five video cameras recorded the vehicles passing through the study area, collecting trajectory data for 20 minutes with a sampling period of about 0.2 seconds. We refer to [25] for a detailed description of the dataset and of the data collection method. We observe that the dataset distinguishes several types of vehicles, and particularly in this work we focus on the dynamics of cars and trucks.
In order to calibrate the two-dimensional model with the German dataset, we need first to derive macroscopic quantities from the microscopic information provided by the dataset. Following [21], we describe how we derive the density of cars and trucks,
We consider the data from the second camera from the right of Figure 6, thus we work with 20 minutes of real data. We introduce the time interval (
˜ρ(tk)=Nρ(tk)Lx,˜μ(tk)=Nμ(tk)Lx. | (12) |
The German dataset only provides the position of vehicles with respect to the two directions, thus we need to derive the speed of vehicles from their positions. We assume that each vehicle travels at constant speed which corresponds to the slope of a linear approximation in the least square sense of the vehicle positions. We denote by
˜ux(tk)=1Nρ(tk)Nρ(tk)∑i=1vxi+1Nμ(tk)Nμ(tk)∑i=1wxi˜uy(tk)=1Nρ(tk)Nρ(tk)∑i=1vyi+1Nμ(tk)Nμ(tk)∑i=1wyi. | (13) |
Finally, we combine equations (12) and (13) to define the flux functions as
˜qxρ(tk)=˜ρ(tk)˜ux(tk),˜qyρ(tk)=˜ρ(tk)˜uy(tk)˜qxμ(tk)=˜μ(tk)˜ux(tk),˜qyμ(tk)=˜μ(tk)˜uy(tk). | (14) |
Once we have the density, speed and flux data as functions of time, we aggregate them with respect to a certain time period
The graphs show that the main direction of the flow is along the
rmax=# laneslength of vehicles + safety distance=37.5 m=400vehkm. | (15) |
However, it should be noted that the dataset only contains data in free-flow regimes without capturing congested traffic phase.
Now we need to compute the parameters
mincx(‖ |
The computation is performed using the MATLAB
We observe that the advantage of the multi-class model is that we can cover quite well the clouds of real data by means of the family of flux and velocity functions. However, we note that, since the German dataset contains data which refer only to the not congested phase of traffic, we do not have enough data to better calibrate congested traffic situations. In particular, the choice of
In this section we describe how to treat the microscopic data to define the initial density for the numerical scheme and the reference solution for the comparison of the results. The German dataset gives information about the position of vehicles every 0.2 seconds, thus we work with pointwise data. In order to define a density function
Let
In order to recover the smooth function
and then define
(16) |
We follow a similar procedure to estimate the density of trucks. The parameters
For each video camera of the German dataset we work with records data for about
Now, we compare the numerical simulations of model (1) with the real data computed from equation (16). The simulation refers to the data recorded by the second video camera of the German dataset.
Let us consider the domain
for cars and trucks respectively, with
for cars and trucks, respectively. The parameters used in the following test are
The dotted lines divide the road into three lanes. After
In order to better compare the numerical results with the ground-truth data, we introduce the following errors
(17) |
(18) |
The errors at time
In the previous section we have seen that the two dimensional multi-class LWR (1) with the flux functions defined in (2) and calibrated with real data is able to simulate the dynamics of vehicles. However, our main assumption on the flux functions is that the coefficients
We consider again the German dataset [25]. Our aim is to consider different maximum density of cars and trucks, due to the different length of vehicles, and different parameters
(19) |
Note that in (19) the different maximum densities between cars and trucks is expressed by the term
With the introduction of different coefficients
Next, we repeat a procedure analogous to the one proposed in Section 4.1 to estimate the velocity functions and the fundamental diagrams. In particular, we define different velocity functions for
from which we recover the flux functions as
(20) |
We estimate the parameters
using again the
In Figure 11, we show the family of speed and flux functions obtained with the above described procedure. Note that the speed and flux functions related to trucks, shown Figures 11(B), 11(D), 11(F) and 11(H), are defined for
We repeat the same numerical test proposed in Section 4.3 with the new flux functions (19), estimating again the resulting errors with (17) and (18). The density plots we obtain are similar to the plots shown in Figure 9, thus we omit the picture. However, as shown in Figure 12, we obtain a better estimate of the errors compared to the test done in Section 4.3.
A further investigation of our model is the testing of the ability of capturing vehicles overtaking. We consider the following flux functions
where we have different parameters
We consider a numerical grid
As we can see in Figure 13, we consider a road with two lanes with two cars and a truck. At the beginning of the simulation there is a car in the top lane and a truck in front of the other car in the bottom lane. Since the truck does not move and the cars are free to move along the
Finally, we intend to compare the proposed 2D multi-class approach to a first order multi-class multi-lane model. Indeed, the inclusion of lane change dynamics well fits with the simulation of vehicle overtaking and allows us to compare the results obtained with the 2D multi-class model. Specifically, we extend the multi-lane LWR model proposed in [23] to a multi-class model. Hence, let us consider a road with two lanes and two classes of vehicles
(21) |
where
(22) |
where
The flux functions are chosen similar to the ones used for the two-dimensional multi-class model. Therefore, we define them as
where
In Figure 14, we show the density of cars and trucks on the two lanes at different times. The plots in the first row show Lane 1, with a car and no trucks, the plots in the second row show Lane 2, with a car and a truck which does not move at all during the simulation since its velocity is 0. The source terms in (21) allow lane changing even if the traffic is not congested and vehicles are free to move along their lane. Hence, in Figure 14(E) we see that the density of cars increases at the end of Lane 2, due to the source term, while in Figures 14(B) and 14(C) the density of cars related to Lane 1 increases since the truck does not move and the cars change lane. At the end of the simulation the density of cars is higher on Lane 1 while it is close to 0 on Lane 2.
From the two tests proposed in Sections 5.2 and 5.2.1 we observe that the 2D multi-class model seems to be more suitable for capturing the overtaking of vehicles. Indeed, the two-dimensional description seem to fit better to such a dynamics, as shown in Figures 13 and 14.
In this work we have introduced a two-dimensional multi-class traffic model. We have analyzed the two-dimensional Riemann problems related to our model and provided numerical validations with a numerical scheme based on dimensional splitting. Then, we have analyzed the model with a dataset of real trajectories data, focusing on the dynamics of cars and trucks. The dataset has been used to calibrate the flux and velocity functions and to compare the numerical results with ground-truth data. The numerical tests have shown the good approximation of the trajectories with our model, obtaining a numerical error of
Future investigations will aim at improving the proposed approach with second order models and deriving the corresponding microscopic model as a system of ordinary differential equations.
[1] |
Ehrlich PR, Harte J (2015) To feed the world in 2050 will require a global revolution. Proc Natl Acad Sci 112: 14743-14744. https://doi.org/10.1073/pnas.1519841112 ![]() |
[2] | Ali M, Cybulska J, Frąc M, et al. (2023) Application of polysaccharides for the encapsulation of beneficial microorganisms for agricultural purposes: A review. Int J Biol Macromol 125366. https://doi.org/10.1016/j.ijbiomac.2023.125366 |
[3] | FAO.Pesticides use, pesticides trade and pesticides indicators. Global, regional and country trends, 1990–2019. FAOSTAT Anal Brief Ser No 29 Rome (2021) . |
[4] |
Rani L, Thapa K, Kanojia N, et al. (2021) An extensive review on the consequences of chemical pesticides on human health and environment. J Cleaner Prod 283: 124657. https://doi.org/10.1016/j.jclepro.2020.124657 ![]() |
[5] |
Intisar A, Ramzan A, Sawaira T, et al. (2022) Occurrence, toxic effects, and mitigation of pesticides as emerging environmental pollutants using robust nanomaterials–A review. Chemosphere 293: 133538. https://doi.org/10.1016/j.chemosphere.2022.133538 ![]() |
[6] |
Sharma N, Singhvi R (2017) Effects of chemical fertilizers and pesticides on human health and environment: a review. Int J Agric Environ Biotechnol 10: 675-680. https://doi.org/10.5958/2230-732X.2017.00083.3 ![]() |
[7] | Kaur R, Kaur S (2018) Biological alternates to synthetic fertilizers: efficiency and future scopes. Indian J Agric Res 52: 587-595. https://doi.org/10.18805/IJARe.A-5117 |
[8] | Hossain MA, Hossain MS, Akter M (2023) Challenges faced by plant growth-promoting bacteria in field-level applications and suggestions to overcome the barriers. Physiol Mol Plant Pathol 102029. https://doi.org/10.1016/j.pmpp.2023.102029 |
[9] | Singh K, Guleria V, Kaushal S (2023) Utilization of Biofertilizers and plant growth promoters in hydroponic production system. Curr J Appl Sci Technol 42: 13-23. https://doi.org/10.9734/cjast/2023/v42i374243 |
[10] |
Orozco-Mosqueda MDC, Santoyo G, Glick BR (2023) Recent advances in the bacterial phytohormone modulation of plant growth. Plants 12: 606. https://doi.org/10.3390/plants12030606 ![]() |
[11] | Etesami H, Jeong BR, Glick BR (2023) Biocontrol of plant diseases by Bacillus spp. Physiol Mol Plant Pathol 102048. https://doi.org/10.1016/j.pmpp.2023.102048 |
[12] |
Remus R, Ruppel S, Jacob HJ, et al. (2000) Colonization behaviour of two enterobacterial strains on cereals. Biol Fertil Soils 30: 550-557. https://doi.org/10.1007/s003740050035 ![]() |
[13] |
Mishra A, Chauhan PS, Chaudhry V, et al. (2011) Rhizosphere competent Pantoea agglomerans enhances maize (Zea mays) and chickpea (Cicer arietinum L.) growth, without altering the rhizosphere functional diversity. Antonie Van Leeuwenhoek 100: 405-413. https://doi.org/10.1007/s10482-011-9596-8 ![]() |
[14] |
Cherif-Silini H, Thissera B, Bouket AC, et al. (2019) Durum wheat stress tolerance induced by endophyte Pantoea agglomerans with genes contributing to plant functions and secondary metabolite arsenal. Int J Mol Sci 20: 3989. https://doi.org/10.3390/ijms20163989 ![]() |
[15] |
Lorenzi AS, Bonatelli ML, Chia MA, et al. (2022) Opposite sides of Pantoea agglomerans and its associated commercial outlook. Microorganisms 10: 2072. https://doi.org/10.3390/microorganisms10102072 ![]() |
[16] |
Torres AR, Araújo WL, Cursino L, et al. (2013) Colonization of Madagascar periwinkle (Catharanthus roseus), by endophytes encoding gfp marker. Arch Microbiol 195: 483-489. https://doi.org/10.1007/s00203-013-0897-3 ![]() |
[17] |
Herrera SD, Grossi C, Zawoznik M, et al. (2016) Wheat seeds harbour bacterial endophytes with potential as plant growth promoters and biocontrol agents of Fusarium graminearum. Microbiol Res 186: 37-43. https://doi.org/10.1016/j.micres.2016.03.002 ![]() |
[18] |
Gomis-Cebolla J, Berry C (2023) Bacillus thuringiensis as a biofertilizer in crops and their implications in the control of phytopathogens and insect pests. Pest Manage Sci 79: 2992-3001. https://doi.org/10.1002/ps.7560 ![]() |
[19] |
Azizoglu U (2019) Bacillus thuringiensis as a biofertilizer and biostimulator: a mini-review of the little-known plant growth-promoting properties of Bt. Curr Microbiol 76: 1379-1385. https://doi.org/10.1007/s00284-019-01705-9 ![]() |
[20] |
Mącik M, Gryta A, Frąc M (2020) Biofertilizers in agriculture: An overview on concepts, strategies and effects on soil microorganisms. Adv Agron 162: 31-87. https://doi.org/10.1016/bs.agron.2020.02.001 ![]() |
[21] | Rai S, Mago Y, Aggarwal G, et al. (2023) Liquid bioformulation: a trending approach towards achieving sustainable agriculture. Mol Biotechnol 1–26. https://doi.org/10.1007/s12033-023-00901-0 |
[22] |
Balla A, Silini A, Cherif-Silini H, et al. (2022) Recent advances in encapsulation techniques of plant growth-promoting microorganisms and their prospects in the sustainable agriculture. Appl Sci 12: 9020. https://doi.org/10.3390/app12189020 ![]() |
[23] |
Song S, Liu Y, Wang NR, et al. (2021) Mechanisms in plant–microbiome interactions: lessons from model systems. Curr Opin Plant Biol 62: 102003. https://doi.org/10.1016/j.pbi.2021.102003 ![]() |
[24] | Grosse-Heilmann M, Cristiano E, Deidda R, et al. (2024) Durum wheat productivity today and tomorrow: A review of influencing factors and climate change effects. Resour Environ Sustainability 100170. https://doi.org/10.1016/j.resenv.2024.100170 |
[25] |
Saadaoui N, Silini A, Cherif-Silini H, et al. (2022) Semi-arid-habitat-adapted plant-growth-promoting rhizobacteria allows efficient wheat growth promotion. Agronomy 12: 2221. https://doi.org/10.3390/agronomy12092221 ![]() |
[26] |
Wu Z, Zhao Y, Kaleem I, et al. (2011) Preparation of calcium–alginate microcapsuled microbial fertilizer coating Klebsiella oxytoca Rs-5 and its performance under salinity stress. Eur J Soil Biol 47: 152-159. https://doi.org/10.1016/j.ejsobi.2010.11.008 ![]() |
[27] |
Panichikkal J, Prathap G, Nair RA, et al. (2021) Evaluation of plant probiotic performance of Pseudomonas sp. encapsulated in alginate supplemented with salicylic acid and zinc oxide nanoparticles. Int J Biol Macromol 166: 138-143. https://doi.org/10.1016/j.ijbiomac.2020.10.110 ![]() |
[28] |
Chi Y, Wang D, Jiang M, et al. (2020) Microencapsulation of Bacillus megaterium NCT-2 and its effect on remediation of secondary salinization soil. J Microencapsulation 37: 134-143. https://doi.org/10.1080/02652048.2019.1705409 ![]() |
[29] |
Kerbab S, Silini A, Chenari Bouket A, et al. (2021) Mitigation of NaCl stress in wheat by rhizosphere engineering using salt habitat adapted PGPR halotolerant bacteria. Appl Sci 11: 1034. https://doi.org/10.3390/app11031034 ![]() |
[30] |
Saidi S, Cherif-Silini H, Chenari Bouket A, et al. (2021) Improvement of Medicago sativa crops productivity by the co-inoculation of Sinorhizobium meliloti–actinobacteria under salt stress. Curr Microbiol 78: 1344-1357. https://doi.org/10.1007/s00284-021-02394-z ![]() |
[31] |
Slama HB, Cherif-Silini H, Chenari Bouket A, et al. (2019) Screening for Fusarium antagonistic bacteria from contrasting niches designated the endophyte Bacillus halotolerans as plant warden against Fusarium. Front Microbiol 9: 3236. https://doi.org/10.3389/fmicb.2018.03236 ![]() |
[32] |
Tsavkelova EA, Cherdyntseva TA, Netrusov AI (2004) Bacteria associated with the roots of epiphytic orchids. Microbiology 73: 710-715. https://doi.org/10.1007/s11021-005-0013-z ![]() |
[33] |
Silini A, Cherif-Silini H, Yahiaoui B (2016) Growing varieties durum wheat (Triticum durum) in response to the effect of osmolytes and inoculation by Azotobacter chroococcum under salt stress. Afr J Microbiol Res 10: 387-399. https://doi.org/10.5897/AJMR2015.7723 ![]() |
[34] |
DuBois M, Gilles KA, Hamilton JK, et al. (1956) Colorimetric method for determination of sugars and related substances. Anal Chem 28: 350-356. https://doi.org/10.1021/ac60111a017 ![]() |
[35] |
Lowry OH, Rosebrough NJ, Farr AL, et al. (1951) Protein measurement with the Folin phenol reagent. J Biol Chem 193: 265-275. https://doi.org/10.1016/S0021-9258(19)52451-6 ![]() |
[36] |
Berninger T, González López Ó, Bejarano A, et al. (2018) Maintenance and assessment of cell viability in formulation of non-sporulating bacterial inoculants. Microb Biotechnol 11: 277-301. https://doi.org/10.1111/1751-7915.12880 ![]() |
[37] |
Daffonchio D, Hirt H, Berg G (2015) Plant-microbe interactions and water management in arid and saline soils. Principles of Plant-Microbe Interactions: Microbes for Sustainable Agriculture. Cham: Springer 265-276. https://doi.org/10.1007/978-3-319-08575-3_28 ![]() |
[38] |
Hayat S, Hayat Q, Alyemeni MN, et al. (2012) Role of proline under changing environments: a review. Plant Signaling Behav 7: 1456-1466. https://doi.org/10.4161/psb.21949 ![]() |
[39] |
Wu Z, Li X, Liu X, et al. (2020) Membrane shell permeability of Rs-198 microcapsules and their ability for growth promoting bioactivity compound releasing. RSC Adv 10: 1159-1171. https://doi.org/10.1039/C9RA06935F ![]() |
[40] |
Kaur R, Kaur S, Dwibedi V, et al. (2023) Development and characterization of rice bran-gum Arabic based encapsulated biofertilizer for enhanced shelf life and controlled bacterial release. Front Microbiol 14: 1267730. https://doi.org/10.3389/fmicb.2023.1267730 ![]() |
[41] |
García AH (2011) Anhydrobiosis in bacteria: from physiology to applications. J Biosci 36: 939-950. https://doi.org/10.1007/s12038-011-9107-0 ![]() |
[42] |
Schoebitz M, Simonin H, Poncelet D (2012) Starch filler and osmoprotectants improve the survival of rhizobacteria in dried alginate beads. J Microencapsulation 29: 532-538. https://doi.org/10.3109/02652048.2012.665090 ![]() |
[43] |
Liffourrena AS, Lucchesi GI (2018) Alginate-perlite encapsulated Pseudomonas putida A (ATCC 12633) cells: Preparation, characterization and potential use as plant inoculants. J Biotechnol 278: 28-33. https://doi.org/10.1016/j.jbiotec.2018.04.019 ![]() |
[44] |
Timmusk S, Behers L, Muthoni J, et al. (2017) Perspectives and challenges of microbial application for crop improvement. Front Plant Sci 8: 49. https://doi.org/10.3389/fpls.2017.00049 ![]() |
[45] |
Guo L, Wu Z, Rasool A, et al. (2012) Effects of free and encapsulated co-culture bacteria on cotton growth and soil bacterial communities. Eur J Soil Biol 53: 16-22. https://doi.org/10.1016/j.ejsobi.2012.08.003 ![]() |
[46] |
Anwar MS, Paliwal A, Firdous N, et al. (2019) Co-culture development and bioformulation efficacy of psychrotrophic PGPRs to promote growth and development of pea (Pisum sativum) plant. J Gen Appl Microbiol 65: 88-95. https://doi.org/10.2323/jgam.2018.05.007 ![]() |
[47] |
Santos MS, Nogueira MA, Hungria M (2019) Microbial inoculants: reviewing the past, discussing the present and previewing an outstanding future for the use of beneficial bacteria in agriculture. Amb Express 9: 1-22. https://doi.org/10.1186/s13568-019-0932-0 ![]() |
[48] | Ozdal M, Ozdal OG, Sezen A, et al. (2017) Continuous production of indole-3-acetic acid by immobilized cells of Arthrobacter agilis. 3 Biotech 7: 1-6. https://doi.org/10.1007/s13205-017-0605-0 |
[49] |
Sandhya VZAS, SK ZA, Grover M, et al. (2009) Alleviation of drought stress effects in sunflower seedlings by the exopolysaccharides producing Pseudomonas putida strain GAP-P45. Biol Fertil Soils 46: 17-26. https://doi.org/10.1007/s00374-009-0401-z ![]() |
[50] |
Duca DR, Glick BR (2020) Indole-3-acetic acid biosynthesis and its regulation in plant-associated bacteria. Appl Microbiol Biotechnol 104: 8607-8619. https://doi.org/10.1007/s00253-020-10869-5 ![]() |
[51] |
Höper D, Bernhardt J, Hecker M (2006) Salt stress adaptation of Bacillus subtilis: a physiological proteomics approach. Proteomics 6: 1550-1562. https://doi.org/10.1002/pmic.200500197 ![]() |
[52] |
Dunyashev TP, Laptev GY, Yildirim EA, et al. (2021) Identification of genes associated with the synthesis of siderophores by the Bacillus subtilis. J Livestock Sci 12: 287-291. https://doi.org/10.33259/JLivestSci.2021.287-291 ![]() |
[53] | Yadav AN, Kumar V, Dhaliwal HS, et al. (2018) Microbiome in crops: diversity, distribution, and potential role in crop improvement. Crop improvement through microbial biotechnology.Elsevier 305-332. https://doi.org/10.1016/B978-0-444-63987-5.00015-3 |
[54] |
Pan L, Cai B (2023) Phosphate-solubilizing bacteria: advances in their physiology, molecular mechanisms and microbial community effects. Microorganisms 11: 2904. https://doi.org/10.3390/microorganisms11122904 ![]() |
[55] | Wang JJ, Yan AH, Wang W, et al. (2016) Screening, identification and phosphate-solubilizing characteristics of phosphate-solubilizing bacteria strain D2 (Pantoea sp.) in rhizosphere of Pinus tabuliformis in iron tailings yard. Ying Yong Sheng tai xue bao=The Journal of Applied Ecology 27: 3705-3711. |
[56] | Kalayu G (2019) Phosphate solubilizing microorganisms: promising approach as biofertilizers. Int J Agron 4917256. https://doi.org/10.1155/2019/4917256 |
[57] |
Khourchi S, Elhaissoufi W, Loum M, et al. (2022) Phosphate solubilizing bacteria can significantly contribute to enhance P availability from polyphosphates and their use efficiency in wheat. Microbiol Res 262: 127094. https://doi.org/10.1016/j.micres.2022.127094 ![]() |
[58] |
Suleimanova AD, Beinhauer A, Valeeva LR, et al. (2015) Novel glucose-1-phosphatase with high phytase activity and unusual metal ion activation from soil bacterium Pantoea sp. strain 3.5. 1. Appl Environ Microbiol 81: 6790-6799. https://doi.org/10.1128/AEM.01384-15 ![]() |
[59] |
Park JH, Lee HH, Han CH, et al. (2016) Synergistic effect of co-inoculation with phosphate-solubilizing bacteria. Korean J Agric Sci 43: 401-414. https://doi.org/10.7744/kjoas.20160043 ![]() |
[60] | Tu L, He Y, Shan C, et al. (2016) Preparation of microencapsulated Bacillus subtilis SL-13 seed coating agents and their effects on the growth of cotton seedlings. BioMed Res Int 16. https://doi.org/10.1155/2016/3251357 |
[61] |
Liu B, Luo J, Wang X, et al. (2013) Alginate/quaternized carboxymethyl chitosan/clay nanocomposite microspheres: preparation and drug-controlled release behavior. J Biomater Sci Polym Ed 4: 589-605. https://doi.org/10.1080/09205063.2012.701160 ![]() |
[62] |
He Y, Wu Z, Tu L, et al. (2015) Encapsulation and characterization of slow-release microbial fertilizer from the composites of bentonite and alginate. Appl Clay Sci 109: 68-75. https://doi.org/10.1016/j.clay.2015.02.001 ![]() |
[63] |
Pour MM, Saberi-Riseh R, Mohammadinejad R, et al. (2019) Investigating the formulation of alginate-gelatin encapsulated Pseudomonas fluorescens (VUPF5 and T17-4 strains) for controlling Fusarium solani on potato. Int J Biol Macromol 133: 603-613. https://doi.org/10.1016/j.ijbiomac.2019.04.071 ![]() |
[64] |
Al-Qaysi SA, Al-Haideri H, Al-Shimmary SM, et al. (2021) Bioactive levan-Type exopolysaccharide produced by Pantoea agglomerans ZMR7: Characterization and optimization for enhanced production. J Microbiol Biotechnol 31: 696. https://doi.org/10.4014/jmb.2101.01025 ![]() |
[65] |
Wang M, Geng L, Xue B, et al. (2021) Structure characteristics and function of a novel extracellular polysaccharide from Bacillus thuringiensis strain 4D19. Int J Biol Macromol 189: 956-964. https://doi.org/10.1016/j.ijbiomac.2021.08.193 ![]() |
[66] |
Donati I, Holtan S, Mørch YA, et al. (2005) New hypothesis on the role of alternating sequences in calcium– alginate gels. Biomacromolecules 6: 1031-1040. https://doi.org/10.1021/bm049306e ![]() |
[67] |
Liew CV, Chan LW, Ching AL, et al. (2006) Evaluation of sodium alginate as drug release modifier in matrix tablets. Int J Pharm 309: 25-37. https://doi.org/10.1016/j.ijpharm.2005.10.040 ![]() |
[68] |
Allard-Massicotte R, Tessier L, Lécuyer F, et al. (2016) Bacillus subtilis early colonization of Arabidopsis thaliana roots involves multiple chemotaxis receptors. MBio 7: 10-1128. https://doi.org/10.1128/mBio.01664-16 ![]() |
[69] |
Doornbos RF, Geraats BP, Kuramae EE, et al. (2011) Effects of jasmonic acid, ethylene, and salicylic acid signaling on the rhizosphere bacterial community of Arabidopsis thaliana. Mol Plant Microbe Interact 24: 395-407. https://doi.org/10.1094/MPMI-05-10-0115 ![]() |
[70] |
Polisetti S, Bible AN, Morrell-Falvey JL, et al. (2016) Raman chemical imaging of the rhizosphere bacterium Pantoea sp. YR343 and its co-culture with Arabidopsis thaliana. Analyst 141: 2175-2182. https://doi.org/10.1039/C6AN00080K ![]() |
[71] | Beauregard PB, Chai Y, Vlamakis H, et al. (2013) Bacillus subtilis biofilm induction by plant polysaccharides. Proce Natl Acad Sci 110: 1621-1630. https://doi.org/10.1073/pnas.1218984110 |
[72] |
Fan D, Subramanian S, Smith DL (2020) Plant endophytes promote growth and alleviate salt stress in Arabidopsis thaliana. Sci Rep 10: 12740. https://doi.org/10.1038/s41598-020-69713-5 ![]() |
[73] |
Graça AT, Hall M, Persson K, et al. (2021) High-resolution model of Arabidopsis photosystem II reveals the structural consequences of digitonin-extraction. Sci Rep 11: 15534. https://doi.org/10.1038/s41598-021-94914-x ![]() |
[74] |
Mirskaya GV, Khomyakov YV, Rushina NA, et al. (2022) Plant development of early-maturing spring wheat (Triticum aestivum L.) under inoculation with Bacillus sp. V2026. Plants 11: 1817. https://doi.org/10.3390/plants11141817 ![]() |
[75] |
Sood G, Kaushal R, Sharma M (2020) Significance of inoculation with Bacillus subtilis to alleviate drought stress in wheat (Triticum aestivum L.). Vegetos 33: 782-792. https://doi.org/10.1007/s42535-020-00149-y ![]() |
[76] |
Rojas-Padilla J, de-Bashan LE, Parra-Cota FI, et al. (2022) Microencapsulation of Bacillus Strains for Improving Wheat (Triticum turgidum Subsp. durum) Growth and Development. Plants 11: 2920. https://doi.org/10.3390/plants11212920 ![]() |
[77] |
Suman A, Shukla L, Marag PS, et al. (2020) Potential use of plant colonizing Pantoea as generic plant growth promoting bacteria for cereal crops. J Environ Biol 41: 987-994. https://doi.org/10.22438/jeb/41/5/MRN-1250 ![]() |
[78] |
Hussain A, Hasnain S (2011) Interactions of bacterial cytokinins and IAA in the rhizosphere may alter phytostimulatory efficiency of rhizobacteria. World J Microbiol Biotechnol 27: 2645-2654. https://doi.org/10.1007/s11274-011-0738-y ![]() |
[79] |
Yaghoubi Khanghahi M, Leoni B, Crecchio C (2021) Photosynthetic responses of durum wheat to chemical/microbiological fertilization management under salt and drought stresses. Acta Physiol Plant 43: 1-14. https://doi.org/10.1007/s11738-021-03289-z ![]() |
[80] |
Yong JWH, Ng YF, Tan SN, et al. (2010) Effect of fertilizer application on photosynthesis and oil yield of jatropha curcas L. Photosynthetica 48: 208-218. https://doi.org/10.1007/s11099-010-0026-3 ![]() |
[81] |
Kanagendran A, Chatterjee P, Liu B, et al. (2019) Foliage inoculation by Burkholderia vietnamiensis CBMB40 antagonizes methyl jasmonate-mediated stress in Eucalyptus grandis. J Plant Physiol 242: 153032. https://doi.org/10.1016/j.jplph.2019.153032 ![]() |
[82] |
Acosta-Motos JR, Ortuño MF, Bernal-Vicente A, et al. (2017) Plant responses to salt stress: adaptive mechanisms. Agronomy 7: 18. https://doi.org/10.3390/agronomy7010018 ![]() |
[83] |
Singh BK, Trivedi P, Egidi E, et al. (2020) Crop microbiome and sustainable agriculture. Nat Rev Microbiol 18: 601-602. https://doi.org/10.1038/s41579-020-00446-y ![]() |
[84] |
Bhise KK, Dandge PB (2019) Alleviation of salinity stress in rice plant by encapsulated salt tolerant plant growth promoting bacteria Pantoea agglomerans strain KL and its root colonization ability. Arch Agron Soil Sci 65: 1955-1968. https://doi.org/10.1080/03650340.2019.1584395 ![]() |
[85] |
He Y, Wu Z, Ye BC, et al. (2016) Viability evaluation of alginate-encapsulated Pseudomonas putida Rs-198 under simulated salt-stress conditions and its effect on cotton growth. Eur J Soil Biol 75: 135-141. https://doi.org/10.1016/j.ejsobi.2016.05.002 ![]() |
[86] |
Soluch R, Hülter NF, Romero Picazo D, et al. (2021) Colonization dynamics of Pantoea agglomerans in the wheat root habitat. Environ Microbiol 23: 2260-2273. https://doi.org/10.1111/1462-2920.15430 ![]() |
[87] |
Compant S, Clément C, Sessitsch A (2010) Plant growth-promoting bacteria in the rhizo-and endosphere of plants: their role, colonization, mechanisms involved and prospects for utilization. Soil Biol Biochem 42: 669-678. https://doi.org/10.1016/j.soilbio.2009.11.024 ![]() |
[88] |
Kumar A, Prakash A, Johri BN (2011) Bacillus as PGPR in crop ecosystem. Bacteria in agrobiology: crop ecosystems. Berlin Heidelberg: Springer 37-59. https://doi.org/10.1007/978-3-642-18357-7_2 ![]() |
![]() |
![]() |
1. | Maya Briani, Emiliano Cristiani, Paolo Ranut, Macroscopic and Multi-Scale Models for Multi-Class Vehicular Dynamics with Uneven Space Occupancy: A Case Study, 2021, 10, 2075-1680, 102, 10.3390/axioms10020102 | |
2. | Durgesh Vikram, Sanjay Mittal, Partha Chakroborty, Stabilized finite element computations with a two-dimensional continuum model for disorderly traffic flow, 2022, 232, 00457930, 105205, 10.1016/j.compfluid.2021.105205 | |
3. | Shrey Agrawal, Venkatesan Kanagaraj, Martin Treiber, Two-dimensional LWR model for lane-free traffic, 2023, 625, 03784371, 128990, 10.1016/j.physa.2023.128990 | |
4. | Anamika Pandey, Rahul Barthwal, T. Raja Sekhar, Construction of solutions of the Riemann problem for a two-dimensional Keyfitz-Kranzer type model governing a thin film flow, 2025, 498, 00963003, 129378, 10.1016/j.amc.2025.129378 |