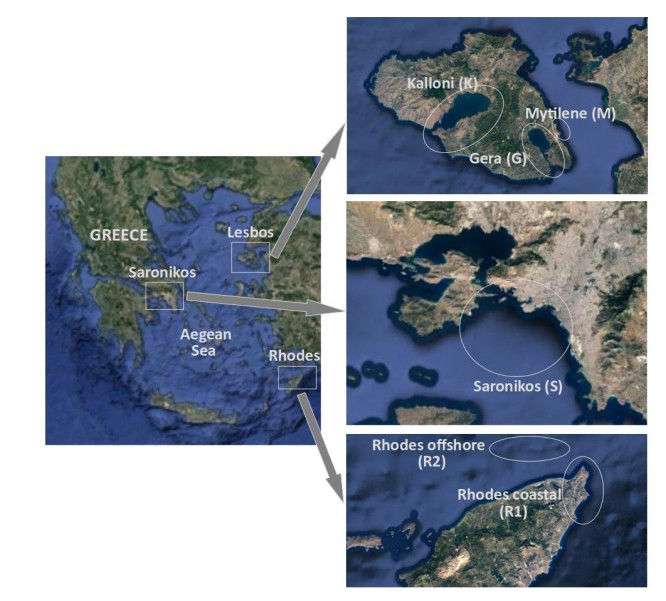
Citation: Cristiane Sá Roriz Fonteles, Richard H. Finnell, Timothy M. George, Raymond J. Harshbarger. Craniosynostosis: current conceptions and misconceptions[J]. AIMS Genetics, 2016, 3(1): 99-129. doi: 10.3934/genet.2016.1.99
[1] | Jianquan Li, Yiqun Li, Yali Yang . Epidemic characteristics of two classic models and the dependence on the initial conditions. Mathematical Biosciences and Engineering, 2016, 13(5): 999-1010. doi: 10.3934/mbe.2016027 |
[2] | Qianqian Cui . Global stability of multi-group SIR epidemic model with group mixing and human movement. Mathematical Biosciences and Engineering, 2019, 16(4): 1798-1814. doi: 10.3934/mbe.2019087 |
[3] | Sherry Towers, Katia Vogt Geisse, Chia-Chun Tsai, Qing Han, Zhilan Feng . The impact of school closures on pandemic influenza: Assessing potential repercussions using a seasonal SIR model. Mathematical Biosciences and Engineering, 2012, 9(2): 413-430. doi: 10.3934/mbe.2012.9.413 |
[4] | Yoichi Enatsu, Yukihiko Nakata, Yoshiaki Muroya . Global stability for a class of discrete SIR epidemic models. Mathematical Biosciences and Engineering, 2010, 7(2): 347-361. doi: 10.3934/mbe.2010.7.347 |
[5] | Yu Tsubouchi, Yasuhiro Takeuchi, Shinji Nakaoka . Calculation of final size for vector-transmitted epidemic model. Mathematical Biosciences and Engineering, 2019, 16(4): 2219-2232. doi: 10.3934/mbe.2019109 |
[6] | Julien Arino, Fred Brauer, P. van den Driessche, James Watmough, Jianhong Wu . A final size relation for epidemic models. Mathematical Biosciences and Engineering, 2007, 4(2): 159-175. doi: 10.3934/mbe.2007.4.159 |
[7] | Kento Okuwa, Hisashi Inaba, Toshikazu Kuniya . Mathematical analysis for an age-structured SIRS epidemic model. Mathematical Biosciences and Engineering, 2019, 16(5): 6071-6102. doi: 10.3934/mbe.2019304 |
[8] | Takeshi Miyama, Sung-mok Jung, Katsuma Hayashi, Asami Anzai, Ryo Kinoshita, Tetsuro Kobayashi, Natalie M. Linton, Ayako Suzuki, Yichi Yang, Baoyin Yuan, Taishi Kayano, Andrei R. Akhmetzhanov, Hiroshi Nishiura . Phenomenological and mechanistic models for predicting early transmission data of COVID-19. Mathematical Biosciences and Engineering, 2022, 19(2): 2043-2055. doi: 10.3934/mbe.2022096 |
[9] | Z. Feng . Final and peak epidemic sizes for SEIR models with quarantine and isolation. Mathematical Biosciences and Engineering, 2007, 4(4): 675-686. doi: 10.3934/mbe.2007.4.675 |
[10] | Haijun Hu, Xupu Yuan, Lihong Huang, Chuangxia Huang . Global dynamics of an SIRS model with demographics and transfer from infectious to susceptible on heterogeneous networks. Mathematical Biosciences and Engineering, 2019, 16(5): 5729-5749. doi: 10.3934/mbe.2019286 |
Machine Learning (ML) algorithms are considered as powerful and reliable tools for prediction, applied so far in many scientific disciplines [1]. ML is a collection of algorithms of different types that improve prediction by gaining knowledge from data [2]. The main advantage of ML techniques is their ability to model multidimensional interactions between nonlinear, noisy, correlated or partly missing data. The field of marine ecology, characterized by complex interactions among a large number of variables, is a specific field counting several applications of ML algorithms [3].
Phytoplankton communities usually follow a rather typical annual pattern on biomass variations and species succession [4]. However, under a combination of forcing factors, it is possible that a regime shift in the system may be established. These factors can be the hydrodynamic conditions in the area [5], the buffering capacity of the system [6], salinity, temperature, nutrients, organic compounds [7], the presence of zooplankton and bacterial populations as well as species interactions [8]. However, work on those aspects [9], has shown that regime shifts are closely connected with the presence of nutrients; high sensitivity was also found in temperature fluctuations. These regime shifts in the case of phytoplankton are expressed as algal blooms. In many cases these blooms are due to exponential growth and dominance of toxic species of phytoplankton [10]. As the shift from the pristine conditions to an undesirable bloom does not follow a linear pattern, is of utmost importance to develop a probabilistic approach so that possible harmful effects on shellfish and fish culture as well as on human health can be indicated.
Algal blooms seem to be a problem of great concern among scientists due to the considerable economic, health and ecological effects on the neighboring coastal zones [11,12]. Τhe various causes of blooms, often linked with eutrophication in coastal ecosystems, is a matter under consideration [13,14]. Many methods have therefore been applied aiming at associating different parameters expressing land use [15], human activities, nutrient loadings, climate changes or other environmental processes with excessive algal growth [13,16,17]. These include the use of ML algorithms. In most cases, ML was applied to model and predict primary production (in terms of chlorophyll-α or biomass) using physical and chemical variables, as temperature, salinity, inorganic nitrogen and phosphorus concentrations [18,19,20]. In addition, there are studies that have modelled different dimensions of primary production such as diversity [21,22] or algal growth time [23]. A recent attempt has been made by Yu et al. (2021) [24] to predict phytoplankton blooms, using Machine Learning algorithms. Their argument is that "when planktonic algae proliferate over certain limits, harmful algal blooms (HABs) will occur". This is not necessarily the case [25]; increased concentrations of toxic algae can occur without a bloom and vice versa: not all blooms end to be toxic. Although algal blooms are entirely natural phenomena and have appeared throughout historical times [10], the frequency of occurrence has increased at an alarming level, over the last few decades. Land based sources of eutrophication, even when they are not the main drivers for triggering mechanisms for bloom formation, it is beyond any doubt that input of nutrients into the marine environment supports bloom formation. However, the principal question remains: will toxic microalgae grow? The answer can be given only if any predictions based on Machine Learning techniques, are targeted towards the exponential growth of toxic species, already recorded in the areas under study.
HABs are being considered as a specific form of coastal eutrophication, causing serious impact including death of organisms, toxins production, hypoxia and often human poisoning due to the consumption of infected fish or shellfish [14,26,27]. ML algorithms have been used to assess the formation of HABs in both fresh and marine waters [28]. Abundance as well as presence/absence of specific harmful algal species have been studied aiming to reveal possible associations with several biotic and abiotic variables. HAB forming species recently studied with selected ML techniques include Karenia brevis [29], Planktothrix rubescens [30] and Dinophysis acuminata [31], while Bourel et al. [32] have used ensembles i.e., combinations of classifiers to predict the presence-absence of 8 harmful marine phytoplankton species. Considering the various ML algorithms, special attention has been given to Neural Networks (NNs) and Random Forests (RFs), two commonly used algorithms with great performance even when dealing with complex ecological processes as HAB formation [33,34,35]. Some applications of NNs include the assessment of algal population dynamics [36,37,38], the classification of different algal types [39,40] and detection of HABs from satellite images [41,42]. On the other hand, the increased effectiveness of RFs has been denoted on the assessment of the distribution of phytoplankton cell size [43] and on the efficient prediction of chlorophyll-a concentration [44,45], of the abundance of some toxic genotypes [46] and of the presence of some toxic algal species [47].
Variables used as drivers in the application of ML algorithms for the prediction of the abundance or presence/absence of HAB forming microalgae, include both biotic and abiotic variables. Abiotic variables used so far include site, season, calendar day, distance from the coast, meteorological variables (air temperature, wind speed and direction, cloud cover), physical variables of seawater (temperature, salinity, conductivity, turbidity, oxygen saturation, pH, Secchi depth, photosynthetic radiation, remote sensing reflectance) and nutrients in both dissolved and particulate forms [29,32,38,46]. Considering biotic variables, chl-a is often used [24,29,47], as well as the abundances of microcrustaceans, ciliates, tintinids, microheterotrophs, cladocerans or copepodes [29,36]. It seems that the selection of cause variables in the existing literature mainly aims to improving the prediction of HABs, although not necessarily exploring a cause-and-effect relationship from the ecological point of view.
In the present study, a screening of several ML algorithms and ensembles is performed aiming to detect the presence/absence of 18 harmful or potentially harmful microalgae at genus level, using existing data collected from six coastal areas in the Aegean Sea, Greece, representing different productivity levels. The overall performance of each algorithm is assessed considering all the 18 studied microalgae. The cause variables include physical variables (temperature and salinity) as well as nutrients, already established in previous studies [16,20] as drivers of algal growth. The objectives of the study are: (a) to identify the most efficient algorithms or ensembles based on the overall prediction of presence/absence of the 18 microalgae, (b) to quantify the effect of cause variables on microalgal growth and (c) to attempt possible grouping of the studied HAB forming microalgae, considering their response to the abiotic drivers. Since all cause variables are easily measured on a routine basis, the results of this study can form the basis of an operational system for the prediction of HABs in coastal waters, eliminating possible adverse effects on ecosystem and human health.
The data set used in the present work was set up by compilation of six sets collected from 42 sampling sites in three different areas of the Aegean Sea, Greece. Five out of six sets originate from coastal waters whereas one set (Rhodes offshore) is characteristic of pelagic waters (Figure 1). The sampling areas are: (a) Kalloni Gulf (Island of Lesbos): eight sampling stations K1-K8 [48] (b) Gulf of Gera (Island of Lesbos): Sampling sites G1-G8 [49] (c) the coastal area of the city of Mytilini: sampling sites M1 and M2 [50] (d) Saronikos Gulf (near Athens): sampling sites S1-S9 [51] (e) offshore waters NW of the city of Rhodes R1-R5 [52] and (f) coastal waters of the city of Rhodes: ten sampling sites RH1-RH10 [51].
The variables included in the dataset are: (a) temperature (T) and salinity (S) [20], also expressing seasonality and (b) nutrient concentrations, namely dissolved inorganic nitrogen (DIN), phosphates ($ {\mathrm{P}\mathrm{O}}_{4}^{3-} $) and silicates ($ {\mathrm{S}\mathrm{i}\mathrm{O}}_{2} $), often recognized as drivers of algal growth and increased primary productivity in coastal waters [53]. These variables are used as inputs (cause variables) in the ML algorithms. The target variable trying to estimate is the presence or absence of 18 genera of harmful or potentially harmful algae estimated by the list of toxic microalgae of IOC-UNESCO [54], shown in Table 1. The number of occurrences of each genus in the 889 samples, the sites where found and summary statistics of the abiotic conditions under which each genus appeared, are shown in Table 1. Redfield' s ratio, i.e., nitrogen to phosphorus ratio (N:P), a characteristic proxy of the nutrient limiting primary productivity, is also shown in Table 1.
Genus | Occurrences | Sampling Areas | T (℃) | S (psu) | DIN (μM) | PO43- (μΜ) | SiO2 (μΜ) | N:P |
Alexandrium | 25 | K, R1 | 14.76 (9.8-23.9) |
37.81 (34.0-40.1) |
5.78 (0.5-39.8) |
0.12 (0.0-1.5) |
15.97 (3.4-86.6) |
196.64 (7.8-1217.5) |
Amphidinium | 71 | K, S, R1, R2 | 17.04 (9.8-23.9) |
38.26 (34.0-40.2) |
4.78 (0.5-39.8) |
0.13 (0.0-1.5) |
14.34 (2.4-86.6) |
232.41 (1.7-6020.0) |
Cryptomonas | 168 | K | 17.10 (9.5-27.7) |
38.57 (34.0-41.1) |
4.13 (0.5-45.2) |
0.09 (0.0-1.6) |
14.36 (1.7-94.0) |
180.16 (6.81-6020.0) |
Dictyocha | 48 | K | 13.93 (9.4-23.3) |
38.29 (34.8-40.7) |
4.46 (0.6-33.2) |
0.10 (0.0-1.3) |
16.22 (4.3-70.9) |
102.69 (7.4-692.2) |
Dinophysis | 228 | K, G, M, S, R1, R2 | 18.27 (9.7-28.2) |
38.29 (28.3-40.6) |
2.70 (0.1-38.0) |
0.16 (0.0-1.8) |
8.85 (0.4-80.7) |
43.76 (2.8-1712.0) |
Goniodoma | 21 | G, M, S, R1, R2 | 19.20 (13.4-27.4) |
38.35 (37.3-39.1) |
2.84 (0.4-11.7) |
0.45 (0.0-6.0) |
9.20 (0.8-15.1) |
25.41 (1.5-116.7) |
Gonyaulax | 37 | K, G, M, S, R1, R2 | 18.41 (14.37-22.11) |
38.82 (36.4-40.6) |
1.66 (0.3-4.1) |
0.19 (0.0-0.85) |
7.19 (2.1-20.9) |
30.05 (2.7-379.0) |
Gymnodinium | 279 | K, M, S, R1, R2 | 19.58 (9.6-27.7) |
38.83 (28.0-40.2) |
1.76 (0.1-27.8) |
0.07 (0.0-4.1) |
9.66 (1.6-53.9) |
68.13 (0.2-1660.0) |
Gyrodinium | 177 | K, G, M, S, R1, R2 | 18.23 (9.7-27.7) |
38.51 (34.7-40.3) |
2.78 (0.4-45.2) |
0.16 (0.0-1.8) |
8.63 (1.5-94.0) |
70.01 (1.7-1660.0) |
Karenia | 394 | S, R1, R2 | 20.00 (13.1-27.6) |
38.55 (28.0-39.7) |
2.01 (0.1-38.0) |
0.15 (0.0-6.0) |
8.02 (0.4-53.9) |
33.04 (1.4-1643.0) |
Karlodinium | 133 | K | 17.20 (9.9-27.7) |
38.68 (34.8-40.7) |
3.51 (0.5-33.2) |
0.07 (0.0-1.3) |
12.64 (1.7-70.9) |
180.74 (6.8-6020.0) |
Lingulodinium | 23 | K, R1 | 14.81 (9.9-25.6) |
37.67 (34.8-40.0) |
6.00 (1.4-33.2) |
0.10 (0.0-1.3) |
11.81 (2.4-68.7) |
282.78 (9.6-1660.0) |
Navicula | 165 | G, M, S, R1, R2 | 19.27 (10.0-26.5) |
39.00 (37.1-40.3) |
2.22 (0.3-23.8) |
0.14 (0.0-4.1) |
9.22 (1.1-53.9) |
44.78 (0.2-1643.0) |
Peridinium | 301 | K, G, M, S, R1, R2 | 18.92 (9.6-27.7) |
38.62 (28.0-40.3) |
2.60 (0.1-39.8) |
0.11 (0.0-2.3) |
9.87 (1.2-86.6) |
71.58 (1.7-1712.0) |
Phaeocystis | 71 | S, R1 | 16.4 (13.1-26.7) |
38.27 (37.6-39.1) |
2.98 (0.5-14.1) |
0.36 (0.0-6.0) |
5.44 (0.4-17.2) |
16.24 (1.4-72.5) |
Polykrikos | 38 | K, M, R1 | 14.88 (10.0-25.6) |
38.7 (34.9-40.4) |
3.77 (0.6-22.8) |
0.06 (0.0-0.9) |
9.56 (1.1-70.9) |
346.81 (11.9-6020.0) |
Prorocentrum | 777 | K, G, M, S, R1, R2 | 19.10 (9.4-28.2) |
38.61 (28.0-41.1) |
2.26 (0.1-38.0) |
0.13 (0.0-6.0) |
8.43 (0.4-83.0) |
51.69 (1.4-1660.0) |
Pseudo-nitzschia | 491 | K, G, M, S, R1, R2 | 17.39 (9.4-27.7) |
38.5 (28.3-41.1) |
2.89 (0.4-45.2) |
0.14 (0.0-6.0) |
8.92 (0.4-94.0) |
78.51 (1.4-1712.0) |
A variety of ML algorithms was used to classify the presence or absence of the 18 genera of HAB forming microalgae (Table 2). The selected classifiers cover all basic supervised ML categories i.e., rules, trees, lazy, functions and bayes, whereas classic ensemble methods, already used in marine ecology [3], were also applied. In order to optimize the performance of the basic classifiers, different values of the crucial (hyper) parameters of each algorithm were tested during training. Thus, the training included at least four different versions of each basic algorithm (Table 2, last column) eliminating the relation of the parameters' selection to the final algorithm performance. Random Forest (RF) was considered as an ensemble, since it uses a set of decision trees to provide classification. For some ensembles (e.g., Voting), which use techniques trained by the combination of the results of basic classifiers, the number of combined classifiers was limited to three in order to avoid ties. The selected three classifiers were the three best performing basic classifiers, as previously defined.
Category | Abbreviation | Algorithm Description | Hyper parameter values |
Rules | Jrip |
Repeated incremental pruning to produce error reduction (RIPPER) | Batch size = 50,100 Min total weight = 2, 5 |
Part | PART decision list | Batch size = 50,100 Min number of instances per rule = 2, 5 |
|
Trees | J48 | C4 pruned decision tree | Batch size = 50,100 Min number of instances per leaf = 2, 5 Number of folds = 3, 5 |
Rep | Decision tree using reduced error pruning with backfiting | Batch size = 50,100 Min number of instances per leaf = 2, 5 |
|
Lazy | IBk | The k-nearest neighbors using Euclidean distance | Number of neighbors = 1, 5, 10, 20 |
KStar | Nearest neighbor with entropic distance | Global blend = 5, 10, 20, 50 | |
Functions | Log | Multinomial logistic regression | |
MLP | Multilayer Perceptron using backpropagation | Number of neurons = 2, 5, 7, 10 | |
Bayes | NB | Naïve Bayes using estimator classes | Batch size = 50,100 |
BN | Bayes network | Batch size = 50,100 | |
Ensembles | RF | Forest of random decision trees | Batch size = 50,100 Number of iterations = 20, 50,100 |
Bagging | Bagging classifiers to reduce variance | Classifiers: The best basic one Number of iterations = 10 |
|
Boosting | Boosting classifiers using Adaboost M1 method | Classifier: The best basic one | |
CVR | Classification using regression methods | Classifier: M5 model tree | |
RC | Randomizabling classifiers (Random Committee) | Classifier: Random tree | |
Stacking | Combining classifiers using stacking method | Classifiers: The 3 best basic ones Number of folds = 10 |
|
Voting | Combining classifiers using votes | Classifiers: The 3 best basic ones Combination rule: Average of Probabilities |
The efficiency of algorithms was evaluated using the 10-fold cross validation (10-fold CV) procedure [55]. The RWeka interface written in R [56], of Weka ML techniques [57], was used to run and test the algorithms. The number of correctly classified instances of either the presence or absence of each genus as labels of the target class over the total number of water samples (i.e., accuracy), was used to determine the performance effectiveness of each algorithm. Additionally, some other measures of predictive performance were used in order to better evaluate the algorithms' predictions [58]. More precisely, sensitivity (or recall) expressing algorithm completeness, is the fraction of the correct genus presences over the total number of predicted presences (true presences plus false absences) in the total samples. A similar measure for the prediction of absences was used as a second measure of completeness, since the conditions related to the absence of a genus in a sample are also considered crucial [59]. This measure is specificity which is the same fraction calculated on absences (number of correct predicted absences over the sum of true absences plus false presences). Moreover, precision was used, expressing the power of algorithm's correctness as it measures in how many instances that the algorithm predicted as "genus present", the genus was actually present. The classic measure of kappa statistic that represents the degree of accuracy and reliability in classification problems, was also included [60]. Kappa statistic ranges from -1 (total disagreement) through 0 (random classification) to 1 (perfect agreement). Finally, due to the existence of imbalanced data, i.e., low number of appearances for eight genera (i.e., Alexandrium, Amphidinium, Dictyocha, Goniodoma, Gonyaulax, Lingulodinium, Phaeocystis and Polykrikos) in the dataset (see Table 1), one more specialized evaluation measure was used; the discriminant power is a measure that combines sensitivity and specificity and evaluates how well an algorithm distinguishes the presences and absences of a rare genus in case of imbalanced data [59]. The thresholds for this measure are: "poor" for values less than 1, "limited" for values between 1 and 2, "fair" for values between 2 and 3 and "good" for values higher than 3 [61].
The performance of an algorithm can be crucially affected by the variables included in the input dataset [24]. In order to select the optimal subset of the input abiotic variables offering the higher predictive performance to each trained algorithm, an exhaustive search was implemented. All possible subsets of the five available abiotic variables containing 5 (all input variables), 4 out of 5 (5 subsets), 3 out of 5 (10 subsets) and 2 out of 5 (10 subsets) variables were used to find the best combination.
Some ML algorithms estimate directly the importance of the input variables (e.g., most rules or trees). Especially for tree algorithms, applying the split-criterion in each node, the relative importance of each input variable can be easily determined. The most common method to measure a node's effectiveness in trees is the Gini Impurity which measures the probability of misclassification for a new instance (i.e., sample) by its specific tree node. A related measure for RFs is the Mean Decrease of Impurity (MDI) which weighs the impact of each input variable to the final prediction (presence of a genus) by measuring the effectiveness of each variable at reducing the uncertainty during tree induction [62]. In this study the MDI measure was used to assess the importance of each abiotic variable to the presence or absence of the potentially harmful algae genera. Furthermore, this measure was also used for grouping genera affected by similar abiotic conditions. Hierarchical clustering was applied using Euclidean distance and Ward's minimum variance method.
The performance of the ML algorithms measured by the accuracy of correctly classified presences/absences in the 889 seawater samples is presented in Table 3 while Table 4 contains the best performing algorithm along with the optimal values of hyper parameters and the optimal subset of the abiotic variables. The predictive performance for all genera is above 70%, being over 90% for 10 out of 18 genera. The higher percentage of correctly classified instances was for Lingulodinium (98.1%) and the lowest for Peridinium (72.2%). The performance of the various algorithms did not vary significantly within each genus, but mainly among genera. Indeed, the range of predictive performance among different algorithms for the same genus ranged from 32.2% for Gymnodinium to 1.4% for Goniodoma (9.1% the mean difference for all genera), while the corresponding range among genera for the same algorithm ranged from 44.2% for Naïve Bayes to 25.8% for RF technique (30.6% mean difference for all algorithms).
Rules | Trees | Lazy | Functions | Bayes | Ensembles | ||||||||||||
HA genus | Jrip | Part | J48 | Rep | IBK | KStar | Logistic | MLP | NB | BN | RF | Bagging | Boosting | CVR | RC | Stacking | Voting |
Alexandrium | 0.970 | 0.973 | 0.972 | 0.972 | 0.976 | 0.968 | 0.971 | 0.972 | 0.957 | 0.965 | 0.973 | 0.972 | 0.968 | 0.972 | 0.972 | 0.972 | 0.972 |
Amphidinium | 0.918 | 0.919 | 0.916 | 0.917 | 0.916 | 0.917 | 0.919 | 0.916 | 0.907 | 0.910 | 0.925 | 0.921 | 0.917 | 0.919 | 0.922 | 0.920 | 0.917 |
Cryptomonas | 0.925 | 0.885 | 0.899 | 0.873 | 0.749 | 0.907 | 0.858 | 0.894 | 0.838 | 0.910 | 0.936 | 0.936 | 0.942 | 0.882 | 0.904 | 0.811 | 0.940 |
Dictyocha | 0.954 | 0.943 | 0.947 | 0.943 | 0.955 | 0.953 | 0.943 | 0.943 | 0.936 | 0.948 | 0.965 | 0.946 | 0.946 | 0.940 | 0.943 | 0.946 | 0.937 |
Dinophysis | 0.759 | 0.771 | 0.756 | 0.748 | 0.741 | 0.727 | 0.727 | 0.744 | 0.731 | 0.780 | 0.769 | 0.760 | 0.780 | 0.767 | 0.736 | 0.744 | 0.770 |
Goniodoma | 0.976 | 0.976 | 0.976 | 0.976 | 0.979 | 0.970 | 0.975 | 0.975 | 0.965 | 0.976 | 0.976 | 0.978 | 0.979 | 0.977 | 0.970 | 0.976 | 0.976 |
Gonyaulax | 0.957 | 0.955 | 0.958 | 0.958 | 0.955 | 0.954 | 0.956 | 0.958 | 0.926 | 0.926 | 0.961 | 0.960 | 0.961 | 0.958 | 0.953 | 0.958 | 0.958 |
Gymnodinium | 0.772 | 0.780 | 0.793 | 0.772 | 0.692 | 0.803 | 0.680 | 0.742 | 0.524 | 0.753 | 0.846 | 0.811 | 0.773 | 0.812 | 0.802 | 0.686 | 0.827 |
Gyrodinium | 0.793 | 0.802 | 0.802 | 0.800 | 0.729 | 0.801 | 0.800 | 0.798 | 0.775 | 0.799 | 0.827 | 0.812 | 0.790 | 0.799 | 0.790 | 0.801 | 0.808 |
Karenia | 0.813 | 0.762 | 0.757 | 0.765 | 0.779 | 0.791 | 0.588 | 0.729 | 0.650 | 0.666 | 0.848 | 0.791 | 0.794 | 0.772 | 0.799 | 0.683 | 0.805 |
Karlodinium | 0.901 | 0.879 | 0.880 | 0.889 | 0.748 | 0.898 | 0.857 | 0.884 | 0.852 | 0.916 | 0.917 | 0.915 | 0.916 | 0.899 | 0.911 | 0.850 | 0.916 |
Lingulodinium | 0.980 | 0.981 | 0.981 | 0.978 | 0.974 | 0.972 | 0.973 | 0.974 | 0.966 | 0.974 | 0.980 | 0.976 | 0.975 | 0.974 | 0.981 | 0.980 | 0.981 |
Navicula | 0.812 | 0.818 | 0.814 | 0.805 | 0.803 | 0.792 | 0.814 | 0.832 | 0.791 | 0.814 | 0.822 | 0.814 | 0.800 | 0.838 | 0.799 | 0.814 | 0.814 |
Peridinium | 0.714 | 0.706 | 0.687 | 0.685 | 0.586 | 0.679 | 0.658 | 0.674 | 0.654 | 0.650 | 0.722 | 0.719 | 0.717 | 0.708 | 0.690 | 0.661 | 0.717 |
Phaeocystis | 0.947 | 0.944 | 0.945 | 0.952 | 0.951 | 0.934 | 0.918 | 0.961 | 0.916 | 0.951 | 0.958 | 0.961 | 0.956 | 0.947 | 0.954 | 0.920 | 0.961 |
Polykrikos | 0.957 | 0.962 | 0.961 | 0.961 | 0.964 | 0.955 | 0.958 | 0.958 | 0.945 | 0.958 | 0.962 | 0.961 | 0.958 | 0.962 | 0.958 | 0.960 | 0.963 |
Prorocentrum | 0.876 | 0.875 | 0.874 | 0.875 | 0.876 | 0.880 | 0.874 | 0.874 | 0.862 | 0.874 | 0.877 | 0.877 | 0.872 | 0.875 | 0.854 | 0.874 | 0.874 |
Pseudo-nitzschia | 0.757 | 0.756 | 0.761 | 0.754 | 0.771 | 0.765 | 0.665 | 0.699 | 0.565 | 0.737 | 0.791 | 0.768 | 0.771 | 0.762 | 0.750 | 0.653 | 0.786 |
Mean | 0.877 | 0.872 | 0.871 | 0.868 | 0.841 | 0.870 | 0.841 | 0.863 | 0.820 | 0.862 | 0.892 | 0.882 | 0.879 | 0.876 | 0.872 | 0.845 | 0.885 |
HA genus | Best Algorithm | Hyper Paremeters values | Best Parameter Combination |
Alexandrium | IBk | Number of neighbors=5 | T+S+PO43- |
Amphidinium | RF | Batch size=50 Number of iterations=50 |
T+S+DIN+ PO43-+SiO2 |
Cryptomonas | Boosting | Classifier: Jrip (Batch size=100 Min total weight=5) |
T+S+DIN+ PO43-+SiO2 |
Dictyocha | RF | Batch size = 100 Number of iterations = 50 |
T + S + PO4 + SiO2 |
Dinophysis | BN/ Boosting |
Batch size = 100/ Classifier: IBk (Number of neighbors = 10) |
T + S + DIN + PO43- + SiO2 |
Goniodoma | IBk | Number of neighbors = 10 | T + S + DIN + PO43- + SiO2 |
Gonyaulax | RF | Batch size = 100 Number of iterations = 100 |
T + S + DIN + PO43- + SiO2 |
Gymnodinium | RF | Batch size = 50 Number of iterations = 50 |
T + S + DIN + PO43- + SiO2 |
Gyrodinium | RF | Batch size = 50 Number of iterations = 100 |
T + S + DIN + PO43- +SiO2 |
Karenia | RF | Batch size = 50 Number of iterations = 100 |
T + S + PO43- + SiO2 |
Karlodinium | RF | Batch size = 50 Number of iterations = 100 |
T + S + DIN+ PO43- +SiO2 |
Lingulodinium | Part/ RC/ Voting |
Batch size = 50, Min number of instances per rule =2/ Classifier: Random tree/ Classifiers: Jrip (Batch size = 100, Min total weight = 5), Part (Batch size = 100, Min number of instances per rule = 2), J48 (Batch size = 100, Min number of instances per leaf = 5) |
T + S + DIN + PO43- +SiO2 |
Navicula | CVR | Classifier: M5 model tree | T + S + DIN + PO43- + SiO2 |
Peridinium | RF | Batch size = 50 Number of iterations = 100 |
T + S + DIN + PO43- + SiO2 |
Phaeocystis | MLP/Bagging/Voting | Number of neurons = 7/ Classifier:MLP/ Classifiers: MLP, Rep (Batch size = 50, Min number of instances per leaf = 2), BN (Batch size = 100) |
T + S + DIN + PO43- + SiO2 |
Polykrikos | IBk | Number of neighbors = 10 | T + S + PO43- |
Prorocentrum | KStar | Global blend = 50 | T + S + PO43- |
Pseudo-nitzschia | RF | Batch size = 50 Number of iterations = 100 |
T + S + DIN + PO43- + SiO2 |
Among the basic algorithms, Jrip showed the best performance with mean predictive percentage for all genera equal to 87.7%. Part rule algorithm, both trees, KStar, MLP and BN also showed high performance exceeding 86%. Although IBK and MLP are the best performing algorithms regarding 6, and 3 genera respectively, they achieve lower overall performance compared to other basic algorithms. Finally, the classical statistical procedure of Logistic function and NB give predictions of rather low quality.
As expected, the ensembles generally exceed the performance of single classifiers, with four ensembles (RF, Bagging, Boosting, and Voting) achieving higher performances than the best basic, when considering means for all genera. The most efficient ensemble is RF with 89.2% mean predictive performance (1.5% higher than Jrip), providing the highest prediction for 9 genera (Amphidinium, Dictyocha, Gonyaulax, Gymnodinium, Gyrodinium, Karenia, Karlodinium, Peridinium and Pseudo-nitzschia). The second-best performing ensemble is voting with almost 0.7% lower mean predictive percentage (88.5%) compared to RF, offering the most successful prediction for Phaeocystis. Both Bagging and Boosting also performed sufficiently well, the former achieving an overall performance above 88.2% and the latter providing the best prediction for 4 genera. CVR, RC and Stacking show the same or lower performance compared with the basic algorithms and thus are considered as inefficient. For most genera (13 out of 18), the performance of the algorithms was optimal when all variables were included in the input data set. For Dictyocha and Karenia the best performance was achieved when removing DIN, whereas for the two Lazy algorithms (IBk and KStar) the prediction for Alexandrium, Polykrikos and Prorocentrum was optimal when T, S and $ {\mathrm{P}\mathrm{O}}_{4}^{3-} $ were only included in the input variables. For RF in particular, the use of all five abiotic variables optimized the prediction of 7 genera (Ampidinium, Gonyaulax, Gymnodinium, Gyrodinium, Karlodinium, Peridinium and Pseudo-nitzschia). Therefore in the rest of the analysis aiming to propose a single algorithm for all genera and to assess the relative importance of the input variables for prediction, RF the best performing ensemble was applied on the initial input data set of all five abiotic variables.
Since RF seems to be the overall best performing algorithm, more details based on RF classification results using the initial dataset (optimal parameter combination) are shown in Table 5. The mean precision is 0.723, meaning that in 72.3% of the cases in which RF predicted the presence of a genus, the genus is actually present. For some genera this measure is rather high, being 91.5% for Karlodinium and 88.1% for Prorocentrum, showing an upward trend for genera with high number of occurrences in the whole database. On the other hand, the rate of correct classifications of presences that is sensitivity is rather moderate since only 43.1% of the total genus presences is classified correctly by the algorithm. The predictions are even worse for genera present in less than 10% of the samples (rare species), as Alexandrium, Goniodoma, Gonyaulax or Polykrikos. The specificity of the RF classification (correctly predicted absences) is generally high with a mean of 92.1%. This finding implies that absences are more correctly classified than presences, especially for rare genera. On the other hand, the mean value of Kappa statistic is equal to 0.469 showing a rather moderate predictive performance of the RF algorithm. The value of Kappa statistic is lower for the rare genera and varies from 0.287 (fair agreement) for Gonyaulax to 0.723 (substantial agreement) for Cryptomonas. However, the discriminant power, a special measure for the predictive performance for rare genera, is greater than 2 for 6 rare genera, showing that the prediction by RF is "fair" in these cases. Considering each specific genus, the prediction is "good" being above 3 for Lingulodinium and "limited" being 1.632 for Amphidinium.
Algae genus | N# presences | Precision | Sensitivity | Specificity | Kappa statistic | Discriminant power |
Alexandrium | 25 | 0.556 | 0.200 | 0.995 | 0.312 | 2.154 |
Amphidinium | 71 | 0.567 | 0.239 | 0.984 | 0.386 | 1.632 |
Cryptomonas | 168 | 0.878 | 0.774 | 0.974 | 0.723 | |
Dictyocha | 48 | 0.615 | 0.333 | 0.988 | 0.503 | 2.049 |
Dinophysis | 228 | 0.647 | 0.407 | 0.912 | 0.500 | |
Goniodoma | 21 | 0.667 | 0.095 | 0.999 | 0.324 | 2.565 |
Gonyaulax | 37 | 0.667 | 0.108 | 0.998 | 0.287 | 2.261 |
Gymnodinium | 279 | 0.814 | 0.659 | 0.931 | 0.655 | |
Gyrodinium | 177 | 0.682 | 0.353 | 0.970 | 0.509 | |
Karenia | 394 | 0.758 | 0.787 | 0.800 | 0.604 | |
Karlodinium | 133 | 0.915 | 0.489 | 0.992 | 0.595 | |
Lingulodinium | 23 | 0.833 | 0.217 | 0.999 | 0.291 | 3.100 |
Navicula | 165 | 0.550 | 0.200 | 0.963 | 0.480 | |
Peridinium | 301 | 0.642 | 0.405 | 0.884 | 0.518 | |
Phaeocystis | 71 | 0.867 | 0.549 | 0.993 | 0.633 | 2.840 |
Polykrikos | 38 | 0.667 | 0.158 | 0.996 | 0.349 | 2.119 |
Prorocentrum | 777 | 0.881 | 0.985 | 0.429 | 0.375 | |
Pseudo-nitzschia | 490 | 0.811 | 0.808 | 0.769 | 0.668 | |
Mean values | 0.723 | 0.431 | 0.921 | 0.469 | 2.340 |
According to our findings, RF seems to be the most efficient algorithm among single classifiers and ensembles. Since RF is also showing a general immediacy in weighing the effects of input variables based on the composition of the constructed trees, it is selected for providing further insights on the role of the abiotic drivers for the presence/absence of potentially harmful microalgae.
The relative importance of each abiotic variable in the trees constructed for each genus by the RF algorithm, is assessed with the MDI measure (Figure 2). Temperature (T) has the most powerful effect with an MDI median of 0.325, whereas its importance varies among genera (minimum of 0.12 for Pseudo-nitzschia and maximum of 0.40 for Prorocentrum). On the other hand, salinity (S) seems to have the lowest effect, with an MDI median equal to 0.169 (minimum of 0.11 for Amfidinium and maximum of 0.19 for Lingulodinium). $ {\mathrm{P}\mathrm{O}}_{4}^{3-} $is the most important variable among nutrients, with a median of 0.26, against 0.21 and 0.22 for DIN and $ {\mathrm{S}\mathrm{i}\mathrm{O}}_{2} $, respectively. Moreover, the importance of nutrients did not vary considerably among genera (standard deviation equal to 0.39, 0.39 and 0.34 for DIN, $ {\mathrm{P}\mathrm{O}}_{4}^{3-} $and $ {\mathrm{S}\mathrm{i}\mathrm{O}}_{2} $, respectively), implying the similar effect of each nutrient on all species considered.
Possible similarity in the importance of the effects of abiotic variables among the various genera in terms of MDI is assessed with hierarchical clustering (Figure 3). It seems that two groups are formed when considering the effect of the abiotic variables on trees' construction. The first cluster is formed off 8 genera, being Pseudo-nitzschia, Dictyocha, Peridinium, Phaeoystis Gymnodinium, Cryptomonas, and two genera belonging to the Brachidiniales (BR) order (Karenia and Karlodinium). The second cluster includes 10 genera, i.e., Dinophysis, Navicula, Prorocentrum, genera of the Gonyaulacales (GO) order (Alexandrium, Goniodoma, Gonyaulax and Lingulodinium) and of the Gymnodiniales (GY) order (Amphidinium, Gyrodinium and Polykrikos). The members of the first cluster are generally less affected by the abiotic variables compared to the members of the second cluster. Nutrients seem to have the main role for the occurrence of the members of the first cluster, compared to the physical variables (temperature and salinity). On the other hand, the members of the second cluster are mostly affected by temperature and nutrients, whereas salinity has the lowest effect.
In this study, we assessed the overall performance of various ML techniques (both single and ensemble) for the prediction of the presence/absence of 18 harmful or potentially harmful marine microalgae at genus level. We further optimized the performance of the algorithms using different values of initial parameters using 10-fold CV. Jrip was the best performing basic classifier implying that single rules involving the five input variables can rather effectively model the response variable. The effectiveness of Jrip single rules has been also identified in previous studies [63,64]. The best ensemble method was RF achieving an overall mean accuracy of 89.2% and outperforming the rest algorithms by at least 1.5% in accuracy. This is in accordance with many previous studies that revealed the exceptional predictive power of RFs [65,33], including marine ecological studies [66,44]. A possible explanation for the successful performance of RFs is that they retain the benefits of the embedded decision trees, while they also combine tree results exploiting the great effectiveness of the majority of voting schema [67]. Thus, the recurring discrimination of samples based on the abiotic variables during the tree induction process crucially supports the detection of genera presences. The bootstrapping methods used for the construction of different trees protect RFs from overfitting issues while the majority of voting schema combines the predictions by taking into advantage the overlapped conditions that drive genus's occurrence in each tree [68]. Results on accuracy were remarkably high (89.2%), although the detailed analysis of the RF results revealed some predicting weaknesses. The sensitivity of the model was found rather moderate, implying that some real presences were not correctly identified by the algorithm. On the other hand, the specificity was above 90% indicating that absences were successfully determined. The precision of the predictions was found relatively high (72.3%), implying that the predicted presences are in a high percentage real presences. High specificity combined with moderate sensitivity and low kappa statistic is a weakness of RFs, usually arising when occurrences are rare [69,70]. Data bootstrapping is biased towards the major class (absences) leading RF technique to over-predict the major and under-predict the minor class [71]. This imbalance was observed in the present study since 8 genera (i.e., Alexandrium, Amphidinium, Dictyocha, Goniodoma, Gonyaulax, Lingulodinium, Phaeocystis and Polykrikos) were only present in less than 8% of the total samples. Discriminant power for these genera showed at least a "fair" prediction for RFs with the exception of Amphidinium for which prediction was characterized as "limited". This finding combined with the higher mean sensitivity for the other more common genera (being 58.7%), which improves as the balance between presences and absences increases (80.8% and 78.7% for the genera with the most presences Pseudo-nitzschia and Karenia, respectively) render RF results as satisfying. Therefore, special attention should be paid to the prediction of occurrences of rare genera using RFs or other ML techniques. This can be improved by using specific performance measures or by appropriate manipulations on the dataset, as oversampling or repeated random sampling [72]. The exhaustive search for the optimal subset of abiotic variables to be used for input in the ML algorithms, showed that the best performance was achieved when all five variables were used for most genera. As it was found in a similar study [24], a low number of input variables improves prediction, however it seems that in our case the number of five variables is already low and the removal of variables results to information loss. Aiming to exploit the advantages of RFs, we weighted the relative importance of the input variables (i.e., T, S, DIN, $ {\mathrm{P}\mathrm{O}}_{4}^{3-} $ and $ {\mathrm{S}\mathrm{i}\mathrm{O}}_{2} $) using the initial dataset, in terms of MDI, for the presence/absence of the 18 harmful microalgal genera. Temperature was the most important driver of occurrence, although its power varied among genera. The key-role of temperature for the appearance and abundance of phytoplankton species has been recognized by various studies in Mediterranean Sea [20,73,48] and its effect is considered as mainly indirect, associated with seasonal changes and stratification which are identified as drivers of primary production in both ocean and coastal waters [74]. Due to the recent climate changes and the tendency of seawater temperature to increase, the effect of temperature is currently under thorough investigation [75]. It has been reported since a long time [76] that higher temperatures favor the growth of flagellates, whereas diatoms are well adapted at lower temperatures. According to Wyatt [77] "diatoms have seasonal standing-crop maxima in spring and autumn in middle latitudes while lower numbers occur during the intervening months". On the contrary blooms of dinoflagellates are more characteristic during summer time, especially in temperate and subtropical seas. The latter favors dinoflagellate dominance over diatoms during summer period. It is obvious that algal species succession is highly depended on temperature changes and this fact is clearly reflected in the results of the present work.
Although temperature is an important driver of HABs, salinity had the less significant role (lower mean MDI value) for the studied microalgae. There are two possible explanations for this lack of significance. Salinity acts on marine organisms through the osmotic pressure exercised on their cellular fluids. In the present work the range of salinity values was fairly limited (around 38 psu) and therefore it cannot be concluded whether this variable is not of importance to phytoplankton or the effects were not shown due to the narrow range of the salinity values used in this work.
Nutrients were also found to affect the presence of the studied microalgae, phosphates having a principal effect. The mean N:P values referring to specific genera are much higher than 16 with only one exception: the microalga Phaeocystis (with a corresponding N:P value 16:24). This value indicates that the conditions were phosphorus limited (Table 1). Nutrient concentrations in the surface waters in the Eastern Mediterranean are low due to mixing processes with nutrient poorer basin water and biological activity [78]. In addition to this shortcoming that limits phytoplankton abundance, the Eastern Mediterranean Sea seems to be phosphorus limited [79]. This is a rather peculiar characteristic, since the oceans, including the Atlantic, are nitrogen limited and the Atlantic Ocean supplies the Mediterranean with water masses through the Strait of Gibraltar. The interpretation of the results in the present work is also complicated because there is an additional problem regarding phosphates. There are some algal species that given a supply of phosphates, they can store it within their cells in the form of polyphosphate (volutin) granules [7]. This "luxury phosphorus" as it is known in the literature, can be consumed by the algae and support algal growth in the absence of an external supply source. This way any relationship between phosphorus concentration dissolved in the marine environment and algal standing stock is weakened due to the presence of stored phosphorus in some species.
According to the MDI values, expressing the effect of abiotic drivers for the appearance of the studied harmful microalgae, a possible grouping of those organisms was attempted. Two clusters were formed, the first of eight genera (Pseudo-nitzschia, Dictyocha, Peridinium, Phaeoystis Gymnodinium, Cryptomonas, Karenia and Karlodinium) and the second of ten genera (Dinophysis, Navicula, Prorocentrum, Alexandrium, Goniodoma, Gonyaulax, Lingulodinium, Amphidinium, Gyrodinium and Polykrikos). The first group is rather diverse, including members from several phyla, as Bacillariophyta (Pseudo-nitzschia), Ochrophyta (Dictyocha), Miozoa (Peridinium, Gymnodinium, Karenia, Karlodinium), Haptophyta (Phaeocystis) and Cryptophyta (Cryptomonas). The second group mainly includes members of the Miozoa phylum, except of Navicula belonging to Bacillariophyta. Therefore, common functional characteristics of genera belonging to the same phyla seem to play a role for their appearance [80], however this role is not so clear-cut. Members of both clusters are influenced by nutrients, and mainly by phosphorus, which is possibly related to the phosphorus limitation often observed in Eastern Mediterranean waters. For some of the studied genera, the role of phosphorus as a driver for presence of some genera can be found in the existing literature, as Karlodinium in Li et al. [81] and Cryptomonas in Gasol et al. (1993) [82]. Considering the physical drivers, salinity plays a minor role for both groups, although its role is important for specific microalgae as Pseudo-nitzchia [83,84] and Karenia [85]. Temperature is the main driver for the presence of the members of the second group, mainly involving Miozoa. Changes of temperature express seasonality, so related processes as stratification or factors as light availability [86] may be also important for the proliferation of the members of the second group.
Results from this study showed that it is possible to anticipate harmful algal blooms and design management practices to mitigate their effects on marine life and humans. This could be done by monitoring abiotic drivers that were identified in this work and issue appropriate warnings that may suggest some sort of action on behalf of appropriate management authorities. This study and the proposed methodology may form the basis for an effort to improve predictability of these occasionally devastating events.
In the present study various ML techniques were assessed for their efficiency to predict the appearance of 18 potentially harmful marine microalgae using data from 6 coastal sites in Eastern Mediterranean. RF algorithm using five abiotic drivers was the most efficient algorithm for prediction when considering overall the 18 studied microalgae. Moreover RF results were useful to enlighten the role of the various abiotic drivers in an ecological context. Although the overall performance of RF was satisfying in terms of predictability, a more exhaustive training of the algorithm with large number of samples is always desirable. Since the five abiotic variable are easily measured in a routine basis, the proposed methodology may form the basis of an operational system to be used for the prediction of HABs and therefore eliminate effects on marine life and human health.
This research is funded in the context of the project "Predicting harmful algae blooms using machine learning techniques" (MIS 5047187) under the call for proposals "Supporting researchers with an emphasis on new researchers" (EDULLL 103). The project is co-financed by Greece and the European Union (European Social Fund- ESF) by the Operational Programme Human Resources Development, Education and Lifelong Learning 2014–2020.
The authors wish to thank two anonymous reviewers for their insightful comments on a previous version of the manuscript.
There is no conflict of interests.
[1] |
Slater BJ, Lenton KA, Kwan MD, et al. (2008) Cranial sutures: a brief review. Plast Reconstr Surg 121: 170e-178e. doi: 10.1097/01.prs.0000304441.99483.97
![]() |
[2] |
Twigg SR, Wilkie AO (2015) A genetic-pathophysiological framework for craniosynostosis. Am J Hum Genet 97: 359-377. doi: 10.1016/j.ajhg.2015.07.006
![]() |
[3] | Hamm JA, Robin NH (2015) Newborn craniofacial malformations. Orofacial clefting and craniosynostosis. Clin Perinotol 42: 321-336. |
[4] |
Persing JA, Jane JA, Shaffrey M (1989) Virchow and the pathogenesis of craniosynostosis: a translation of his work. Plast Reconstr Surg 83: 738-742. doi: 10.1097/00006534-198904000-00025
![]() |
[5] |
Patel A, Terner J, Travieso R, et al. (2012) On Bernard Sarnat's 100th birthday: pathology and management of craniosynostosis. J Craniofac Surg 23: 105-112. doi: 10.1097/SCS.0b013e318240fb0d
![]() |
[6] |
Cohen MM Jr (2005) Editorial: perspectives on craniosynostosis. Am J Med Genet A 136A: 313-326. doi: 10.1002/ajmg.a.30757
![]() |
[7] |
Boulet SL, Rasmussen SA, Honein MA (2008) A population-based study of craniosynostosis in metropolitan Atlanta, 1989-2003. Am J Med Genet A 146A: 984-991. doi: 10.1002/ajmg.a.32208
![]() |
[8] |
Lee HQ, Hutson JM, Wray AC, et al. (2012) Changing epidemiology of nonsyndromic craniosynostosis and revisiting the risk factors. J Craniofac Surg 23: 1245-1251. doi: 10.1097/SCS.0b013e318252d893
![]() |
[9] | Chim H, Manjila S, Cohen AR, et al. (2011) Molecular signaling in pathogenesis of craniosynostosis: the role of fibroblast growth factor and transforming growth factor-b. Neurosurg Focus 31: E7. |
[10] | Kabbani H, Raghuveer TS (2004) Craniosynostosis. Am Fam Physician 69: 2863-2870. |
[11] |
Kimonis V, Gold JA, Hoffman TL, et al. (2007) Genetics of craniosynostosis. Semin Pediatr Neurol 14: 150-161. doi: 10.1016/j.spen.2007.08.008
![]() |
[12] |
French LR, Jackson IT, Melton LJ (1990) A population-based study of craniosynostosis. J Clin Epidemiol 43: 69-73. doi: 10.1016/0895-4356(90)90058-W
![]() |
[13] |
Alazami AM, Seidahmed MZ, Alzahrani F, et al. (2014) Novel IFT122 mutation associated with impaired ciliogenesis and cranioectodermal dysplasia. Mol Genet Genomic Med 2: 103-106. doi: 10.1002/mgg3.44
![]() |
[14] |
Currarino G (2007) Sagittal synostosis in X-linked hypophosphatemic rickets and related diseases. Pediatr Radiol 37: 805-812. doi: 10.1007/s00247-007-0503-4
![]() |
[15] | Adès LC, Sullivan K, Biggin A, et al. (2006) FBN1, TGFBR1, and the Marfan-craniosynostosis/mental retardation disorders revisited. Am J Med Genet A 140: 1047-1058. |
[16] |
Iqbal Z, Cejudo-Martin P, de Brouwer A, et al. (2010) Disruption of the podosome adaptor protein TKS4 (SH3PXD2B) causes the skeletal dysplasia, eye, and cardiac abnormalities of Frank-Ter Haar Syndrome. Am J Hum Genet 86: 254-261. doi: 10.1016/j.ajhg.2010.01.009
![]() |
[17] |
Agrawal D, Steinbok P, Cochrane DD (2006) Diagnosis of isolated sagittal synostosis: are radiographic studies necessary? Childs Nerv Syst 22: 375-378. doi: 10.1007/s00381-005-1243-0
![]() |
[18] |
Levi B, Wan DC, Wong VW, et al. (2012) Cranial suture biology: from pathways to patient care. J Craniofac Surg 23: 13-19. doi: 10.1097/SCS.0b013e318240c6c0
![]() |
[19] |
Van der Meulen J (2012) Metopic synostosis. Childs Nerv Syst 28: 1359-1367. doi: 10.1007/s00381-012-1803-z
![]() |
[20] |
Yee ST, Fearon JA, Gosain AK, et al. (2015) Classification and Management of Metopic Craniosynostosis. J Craniofac Surg 26: 1812-1817. doi: 10.1097/SCS.0000000000001866
![]() |
[21] |
Senarath-Yapa K, Chung MT, McArdle A, et al. (2012) Craniosynostosis: molecular pathways and future pharmacologic therapy. Organogenesis 8: 103-113. doi: 10.4161/org.23307
![]() |
[22] |
Jehee FS, Johnson D, Alonso LG, et al. (2005) Molecular screening for microdeletions at 9p22-p24 and 11q23-q24 in a large cohort of patients with trigonocephaly. Clin Genet 67: 503-510. doi: 10.1111/j.1399-0004.2005.00438.x
![]() |
[23] | Hoischen A, van Bon BW, Rodriguez-Santiago B, et al. (2011) De novo nonsense mutations in ASXL1 cause Bohring-Opitz syndrome. Nat Genet43: 729-731. |
[24] |
Malpuech G, Demeocq F, Palcoux JB, et al. (1983) A previously undescribed autosomal recessive multiple congenital anomalies/mental retardation (MCA/MR) syndrome with growth failure, lip/palate cleft(s), and urogenital anomalies. Am J Med Genet 16: 475-480. doi: 10.1002/ajmg.1320160405
![]() |
[25] |
Vissers LE, Cox TC, Maga AM, et al. (2011) Heterozygous mutations of FREM1 are associated with an increased risk of isolated metopic craniosynostosis in humans and mice. PLoS Genet 7: e1002278. doi: 10.1371/journal.pgen.1002278
![]() |
[26] | McDonald-McGinn DM, Feret H, Nah HD, et al. (2010) Metopic craniosynostosis due to mutations in GLI3: A novel association. Am J Med GenetA 152A: 1654-1660. |
[27] | Twigg SR, Lloyd D, Jenkins D, et al. (2012) Mutations in multidomain protein MEGF8 identify a Carpenter syndrome subtype associated with defective lateralization. Am J Hum Genet91: 897-905. |
[28] |
Wilkie AO, Byren JC, Hurst JA, et al. (2010) Prevalence and complications of single-gene and chromosomal disorders in craniosynostosis. Pediatrics 126: e391-400. doi: 10.1542/peds.2009-3491
![]() |
[29] |
Lajeunie E, Le Merrer M, Bonaïti-Pellie C, et al. (1995) Genetic study of nonsyndromic coronal craniosynostosis. Am J Med Genet 55: 500-504. doi: 10.1002/ajmg.1320550422
![]() |
[30] |
Ziyadeh J, Le Merrer M, Robert M, et al. (2013) Mucopolysaccharidosis type I and craniosynostosis. Acta Neurochir (Wien) 155: 1973-1976. doi: 10.1007/s00701-013-1831-9
![]() |
[31] | Villarreal DD, Villarreal H, Paez AM, et al. (2013) A patient with a unique frameshift mutation in GPC3, causing Simpson-Golabi-Behmel syndrome, presenting with craniosynostosis, penoscrotal hypospadias, and a large prostatic utricle. Am J Med Genet A 161A: 3121-3125. |
[32] |
Shahinian HK, Jaekle R, Suh RH, et al. (1998) Obstetrical factors governing the etiopathogenesis of lambdoid synostosis. Am J Perinatol 15: 281-286. doi: 10.1055/s-2007-993943
![]() |
[33] |
Czerwinski M, Kolar JC, Fearon JA (2011) Complex craniosynostosis. Plast Reconstr Surg 128: 955-961. doi: 10.1097/PRS.0b013e3182268ca6
![]() |
[34] |
Chai Y, Maxson RE Jr (2006) Recent advances in craniofacial morphogenesis. Dev Dyn 235: 2353-2375. doi: 10.1002/dvdy.20833
![]() |
[35] |
Schweizer G, Ayer-LeLièvre C, Le Douarin NM (1983) Restrictions in developmental capacities in the dorsal root ganglia during the course of development. Cell Differ 13:191-200. doi: 10.1016/0045-6039(83)90089-1
![]() |
[36] |
Spears R, Svoboda KKH (2005) Growth factors and signaling proteins in craniofacial development. Semin Orthod 11: 184-198. doi: 10.1053/j.sodo.2005.07.003
![]() |
[37] | Delannet M, Duband JL (1992) Transforming growth factor-beta control of cell-substratum adhesion during avian neural crest cell emigration in vitro. Development 116: 275-287. |
[38] |
Baird A (1994) Fibroblast growth factors: activities and significance of nonneurotrophin neurotrophic growth factors. Curr Opin Neurobiol 4: 78-86. doi: 10.1016/0959-4388(94)90035-3
![]() |
[39] | Morrison-Graham K, Schatteman GC, Bork T, et al. (1992) A PDGF receptor mutation in the mouse (Patch) perturbs the development of a non-neuronal subset of neural crest-derived cells. Development 115: 133-142. |
[40] |
Bodine PV (2008) Wnt signalling controlo f bone cell apoptosis. Cell Res 18: 248-253. doi: 10.1038/cr.2008.13
![]() |
[41] |
Noden DM (1983) The role of the neural crest in patterning of avian cranial skeletal, connective, and muscle tissues. Dev Biol 96: 144-165. doi: 10.1016/0012-1606(83)90318-4
![]() |
[42] |
Deckelbaum RA, Holmes G, Zhao Z, et al. (2012) Regulation of cranial morphogenesis and cell fate at the neural crest-mesoderm boundary by engrailed 1. Development 139: 1346-1358. doi: 10.1242/dev.076729
![]() |
[43] |
Twigg SR, Kan R, Babbs C, et al. (2004) Mutations of ephrin-B1 (EFNB1), a marker of tissue boundary formation, cause craniofrontonasal syndrome. Proc Natl Acad Sci U S A 101: 8652 - 8657. doi: 10.1073/pnas.0402819101
![]() |
[44] |
Merrill AE, Bochukova EG, Brugger SM, et al. (2006) Cell mixing at a neural crest-mesoderm boundary and deficient ephrin-Eph signaling in the pathogenesis of craniosynostosis. Hum Mol Genet 15: 1319-1328. doi: 10.1093/hmg/ddl052
![]() |
[45] | Noden DM (1978) The control of avian cephalic neural crest cytodifferentiation. I. Skeletal and connective tissues. Dev Biol 67: 296-312. |
[46] | Noden DM (1988) Interactions and fates of avian craniofacial mesenchyme. Development 103: 121-140. |
[47] | Le Lievre CS (1978) Participation of neural crest-derived cells in the genesis of the skull in birds. J Embryol Exp Morphol 47: 17-37. |
[48] | Couly GF, Coltey PM, Le Douarin NM (1993) The triple origin of skull in higher vertebrates: a study in quail-chick chimeras. Development 117: 409-429. |
[49] |
Jiang X, Iseki S, Maxson RE, et al. (2002) Tissue origins and interactions in the mammalian skull vault. Dev Biol 241: 106-116. doi: 10.1006/dbio.2001.0487
![]() |
[50] |
Nie X (2005) Cranial base in craniofacial development: developmental features, influence on facial growth, anomaly, and molecular basis. Acta Odontol Scand 63:127-135. doi: 10.1080/00016350510019847
![]() |
[51] | Sasaki T, Ito Y, Bringas P Jr, et al. (2006) TGF (beta)-mediated FGF signaling is crucial for regulating cranial neural crest cell proliferation during frontal bone development. Development 133: 371-381. |
[52] |
Bernard S, Loukas M, Rizk E, et al. (2015) The human occipital bone: review and update on its embryology and molecular development. Childs Nerv Syst 31: 2217-2223. doi: 10.1007/s00381-015-2870-8
![]() |
[53] |
Mishina Y, Snider TN (2014) Neural crest cell signaling pathways critical to cranial bone development and pathology. Exp Cell Res 325: 138-147. doi: 10.1016/j.yexcr.2014.01.019
![]() |
[54] |
Zhao H, Feng J, Ho TV, et al. (2015) The suture provides a niche for mesenchymal stem cells of craniofacial bones. Nat Cell Biol 17: 386-396. doi: 10.1038/ncb3139
![]() |
[55] |
Ogle RC, Tholpady SS, McGlynn KA, et al. (2004) Regulation of cranial suture morphogenesis. Cells Tissues Organs 176: 54-66. doi: 10.1159/000075027
![]() |
[56] |
Opperman LA, Rawlins JT (2005) The extracellular matrix environment in suture morphogenesis and growth. Cells Tissues Organs 181: 127-135. doi: 10.1159/000091374
![]() |
[57] | Manzanares MC, Goret-Nicaise M, Dhem A (1988) Metopic sutural closure in the human skull. J Anat 161: 203-215. |
[58] |
Sahar DE, Longaker MT, Quarto N (2005) Sox9 neural crest determinant gene controls patterning and closure of the posterior frontal cranial suture. Dev Biol 280: 344-361. doi: 10.1016/j.ydbio.2005.01.022
![]() |
[59] | Opperman LA (2000) Cranial sutures as intramembranous bone growth sites. Dev Dyn 219: 472-485. |
[60] |
Gagan JR, Tholpady SS, Ogle RC (2007) Cellular dynamics and tissue interactions of the dura mater during head development. Birth Defects Res C Embryo Today 81: 297-304. doi: 10.1002/bdrc.20104
![]() |
[61] |
Richtsmeier JT, Flaherty K (2013) Hand in glove: brain and skull in development and dysmorphogenesis. Acta Neuropathol 125: 469-489. doi: 10.1007/s00401-013-1104-y
![]() |
[62] |
Mao JJ, Nah HD (2004) Growth and development: hereditary and mechanical modulations. Am J Orthod Dentofacial Orthop 125: 676-689. doi: 10.1016/j.ajodo.2003.08.024
![]() |
[63] | Todd TW, Lyon DW (1925) Cranial suture closure: Its progress and age relationship. Part II. Ectocranial closure in adult males of white stock. Am J Phys Anthropol 8: 23-45. |
[64] | Ting MC, Wu NL, Roybal PG, et al. (2009) EphA4 as an effector of Twist1 in the guidance of osteogenic precursor cells during calvarial bone growth and in craniosynostosis. Development 136: 855-864. |
[65] |
Greenwald JA, Mehrara BJ, Spector JA, et al. (2000) Regional differentiation of cranial suture-associated dura mater in vivo and in vitro: implications for suture fusion and patency. J Bone Miner Res 15: 2413-2430. doi: 10.1359/jbmr.2000.15.12.2413
![]() |
[66] |
Zimmerman B, Moegelin A, de Souza P, et al. (1998) Morphology of the development of the sagittal suture of mice. Anat Embryol (Berl) 197:155-165. doi: 10.1007/s004290050127
![]() |
[67] | Senarath-Yapa K, Li S, Meyer NP, et al. (2013) Integration of multiple signaling pathways determines differences in the osteogenic potential and tissue regeneration of neural crest-derived and mesoderm-derived calvarial bones. Int J Mol Sci 14: 5978-5997. |
[68] | Quarto N, Wan DC, Kwan MD, et al. (2010) Origin matters: differences in embryonic tissue origin and Wnt signaling determine the osteogenic potential and healing capacity of frontal and parietal calvarial bones. J Bone Miner Res 25: 1680-1694. |
[69] | Cohen Jr MM (1993) Sutural biology and the correlates of craniosynostosis. Am J Med Genet 47: 581-616. |
[70] | Pritchard JJ, Scott JH, Girgis FG (1956) The structure and development of cranial and facial sutures. J Anat 90: 73 - 86. |
[71] | Koskinen-Moffett LK, Moffett BC Jr, Graham JM Jr (1982) Cranial synostosis and intra-uterine compression: a developmental study of human sutures. Prog Clin Biol Res 101: 365-378. |
[72] |
Kokich VG (1976) Age changes in the human frontozygomatic suture from 20 to 95 years. Am J Orthod 69: 411-430. doi: 10.1016/0002-9416(76)90209-8
![]() |
[73] |
Morriss-Kay GM, Wilkie AO (2005) Growth of the normal skull vault and its alteration in craniosynostosis: insights from human genetics and experimental studies. J Anat 207: 637-653. doi: 10.1111/j.1469-7580.2005.00475.x
![]() |
[74] |
Nayak SR, Krishnamurthy A, Kumar SJM, et al. (2007) The mendosal suture of the occipital bone: occurrence in Indian population, embryology and clinical significance. Surg Radiol Anat 29: 329-332. doi: 10.1007/s00276-007-0216-2
![]() |
[75] |
Kirmi O, Lo SJ, Johnson D, et al. (2009) Craniosynostosis: a radiological and surgical perspective. Semin Ultrasound CT MR 30: 492-512. doi: 10.1053/j.sult.2009.08.002
![]() |
[76] |
Vu HL, Panchal J, Parker EE, et al. (2001) The timing of physiologic closure of the metopic suture: a review of 159 patients using reconstructed 3D CT scans of the craniofacial region. J Craniofac Surg 12: 527-532. doi: 10.1097/00001665-200111000-00005
![]() |
[77] |
Park SS, Beyer RP, Smyth MD, et al. (2015) Osteoblast differentiation profiles define sex specific gene expression patterns in craniosynostosis. Bone 76: 169-176. doi: 10.1016/j.bone.2015.03.001
![]() |
[78] | Kim HJ, Rice DP, Kettunen PJ, et al. (1998) FGF-, BMP- and Shh-mediated signalling pathways in the regulation of cranial suture morphogenesis and calvarial bone development. Development 125: 1241-1251. |
[79] |
Mansukhani A, Ambrosetti D, Holmes G, et al. (2005) Sox2 induction by FGF and FGFR2 activating mutations inhibits Wnt signaling and osteoblast differentiation. J Cell Biol 168: 1065-1076. doi: 10.1083/jcb.200409182
![]() |
[80] |
Liu B, Yu HM, Hsu W (2007) Craniosynostosis caused by Axin2 deficiency is mediated through distinct functions of beta-catenin in proliferation and differentiation. Dev Biol 301: 298-308. doi: 10.1016/j.ydbio.2006.10.018
![]() |
[81] |
McMahon JA, Takada S, Zimmerman LB, et al. (1998) Noggin-mediated antagonism of BMP signaling is required for growth and patterning of the neural tube and somite. Genes Dev 12: 1438-1452. doi: 10.1101/gad.12.10.1438
![]() |
[82] |
Rosen V (2006) BMP and BMP inhibitors in bone. Ann N Y Acad Sci 1068: 19-25. doi: 10.1196/annals.1346.005
![]() |
[83] |
Warren SM, Brunet LJ, Harland RM, et al. (2003) The BMP antagonist noggin regulates cranial suture fusion. Nature 422: 625-629. doi: 10.1038/nature01545
![]() |
[84] |
Rice DP, Kim HJ, Thesleff I (1999) Apoptosis in murine calvarial bone and suture development. Eur J Oral Sci 107: 265-275. doi: 10.1046/j.0909-8836.1999.eos107406.x
![]() |
[85] |
Agresti M, Gosain AK (2005) Detection of apoptosis in fusing versus nonfusing mouse cranial sutures. J Craniofac Surg 16: 572-578. doi: 10.1097/01.scs.0000168759.61947.7f
![]() |
[86] |
Opperman LA, Sweeney TM, Redmon J, et al. (1993) Tissue interactions with underlying dura mater inhibit osseous obliteration of developing cranial sutures. Dev Dyn 198: 312-322. doi: 10.1002/aja.1001980408
![]() |
[87] | Opperman LA, Passarelli RW, Morgan EP, et al. (1995) Cranial sutures require tissue interactions with dura mater to resist osseous obliteration in vitro. J Bone Miner Res 10: 1978-1987. |
[88] |
Tholpady SS, Freyman TF, Chachra D, et al. (2007) Tensional forces influence gene expression and sutural state of rat calvariae in vitro. Plast Reconstr Surg 120: 601-611. doi: 10.1097/01.prs.0000270284.69632.6b
![]() |
[89] |
Mao JJ (2002) Mechanobiology of craniofacial sutures. J Dent Res 81: 810-816. doi: 10.1177/154405910208101203
![]() |
[90] |
Kopher RA, Mao JJ (2003) Suture growth modulated by the oscillatory component of micromechanical strain. J Bone Min Res 18: 521-528. doi: 10.1359/jbmr.2003.18.3.521
![]() |
[91] |
Mao JJ, Wang X, Kopher RA, et al. (2003) Strain induced osteogenesis in the cranial suture upon controlled delivery of low-frequency cyclic forces. Front Biosci 8: A10-A17. doi: 10.2741/917
![]() |
[92] |
Ingber DE, Tensegrity I (2003) Cell structure and hierarchical systems biology. J Cell Sci 116: 1157-1173. doi: 10.1242/jcs.00359
![]() |
[93] | Carinci P, Becchetti E, Bodo M (2000) Role of the extracellular matrix and growth factors in skull morphogenesis and in the pathogenesis of craniosynostosis. Int J Dev Biol 44: 715-723. |
[94] |
Salmivirta M, Jalkanen M (1995) Syndecan family of cell surface proteoglycans: developmentally regulated receptors for extracellular effector molecules. Experientia 51: 863-872. doi: 10.1007/BF01921737
![]() |
[95] |
Bidwell JP, Pavalko FM (2010) The Load-Bearing Mechanosome Revisited. Clin Rev Bone Miner Metab 8: 213-223. doi: 10.1007/s12018-010-9075-1
![]() |
[96] | Carinci P, Becchetti E, Baroni T, et al. (2007) Extracellular matrix and growth factors in the pathogenesis of some craniofacial malformations. Eur J Histochem 51: 105-116. |
[97] | Bodo M, Carinci F, Baroni T, et al. (1997) Apert's syndrome: differential in vitro production of matrix macromolecules and its regulation by interleukins. Eur J Clin Invest 27: 36-42. |
[98] |
Bodo M, Carinci F, Baroni T, et al. (1998) Interleukin pattern of Apert fibroblasts in vitro. Eur J Cell Biol 75: 383-388. doi: 10.1016/S0171-9335(98)80072-1
![]() |
[99] |
Locci P, Baroni T, Pezzetti F, et al. (1999) Differential in vitro phenotype pattern, transforming growth factorbeta(1) activity and mRNA expression of transforming growth factor-beta(1) in Apert osteoblasts. Cell Tissue Res 297: 475-483. doi: 10.1007/s004410051374
![]() |
[100] |
Bodo M, Baroni T, Carinci F, et al. (1999) A regulatory role of fibroblast growth factor in the expression of decorin, biglycan, betaglycan and syndecan in osteoblasts from patients with Crouzon's syndrome. Eur J Cell Biol 78: 323-330. doi: 10.1016/S0171-9335(99)80066-1
![]() |
[101] |
Su N, Jin M, Chen L (2014) Role of FGF/FGFR signaling in skeletal development and homeostasis: learning from mouse models. Bone Res 2: 14003. doi: 10.1038/boneres.2014.3
![]() |
[102] |
Liu YH, Tang Z, Kundu RK, et al. (1999) Msx2 gene dosage influences the number of proliferative osteogenic cells in growth centers of the developing murine skull: a possible mechanism for MSX2-mediated craniosynostosis in humans. Dev Biol 205: 260-274. doi: 10.1006/dbio.1998.9114
![]() |
[103] |
Cohen MM Jr (1997) Transforming growth factor beta s and fibroblast growth factors and their receptors: role in sutural biology and craniosynostosis. J Bone Miner Res 12: 322-331. doi: 10.1359/jbmr.1997.12.3.322
![]() |
[104] |
Jabs EW, Müller U, Li X, et al. (1993) A mutation in the homeodomain of the human MSX2 gene in a family affected with autosomal dominant craniosynostosis. Cell 75: 443-450. doi: 10.1016/0092-8674(93)90379-5
![]() |
[105] |
Warman ML, Mulliken JB, Hayward PG, et al. (1993) Newly recognized autosomal dominant craniosynostotic syndrome. Am J Med Genet 46: 444-449. doi: 10.1002/ajmg.1320460420
![]() |
[106] |
Ma L, Golden S, Wu L, et al. (1996) The molecular basis of Boston-type craniosynostosis: the Pro148-->His mutation in the N-terminal arm of the MSX2 homeodomain stabilizes DNA binding without altering nucleotide sequence preferences. Hum Mol Genet 5: 1915-1920. doi: 10.1093/hmg/5.12.1915
![]() |
[107] | Wilkie AO, Tang Z, Elanko N, et al. (2000) Functional haploinsufficiency of the human homeobox gene MSX2 causes defects in skull ossification. Nat Genet 24: 387-390. |
[108] |
Alappat S, Zhang ZY, Chen YP (2003) Msx homeobox gene family and craniofacial development. Cell Res 13: 429-442. doi: 10.1038/sj.cr.7290185
![]() |
[109] | MacKenzie A, Ferguson MW, Sharpe PT (1992) Expression patterns of the homeobox gene, Hox-8, in the mouse embryo suggest a role in specifying tooth initiation and shape. Development 115: 403-420. |
[110] | Jowett AK, Vainio S, Ferguson MW, et al. (1993) Epithelial-mesenchymal interactions are required for msx 1 and msx 2 gene expression in the developing murine molar tooth. Development 117: 461-470. |
[111] |
Catron KM, Wang H, Hu G, et al. (1996) Comparison of MSX-1 and MSX-2 suggests a molecular basis for functional redundancy. Mech Dev 55: 185-199. doi: 10.1016/0925-4773(96)00503-5
![]() |
[112] |
Zhang H, Catron KM, Abate-Shen C (1996) A role for the Msx-1 homeodomain in transcriptional regulation: residues in the N-terminal arm mediate TATA binding protein interaction and transcriptional repression. Proc Natl Acad Sci U S A 93: 1764-1769. doi: 10.1073/pnas.93.5.1764
![]() |
[113] |
Newberry EP, Latifi T, Battaile JT, et al. (1997) Structure-function analysis of Msx2-mediated transcriptional suppression. Biochemistry 36: 10451-10462. doi: 10.1021/bi971008x
![]() |
[114] |
Catron KM, Zhang H, Marshall SC, et al. (1995) Transcriptional repression by Msx-1 does not require homeodomain DNA-binding sites. Mol Cell Biol 15: 861-871. doi: 10.1128/MCB.15.2.861
![]() |
[115] |
Wang W, Chen X, Xu H, et al. (1996) Msx3: a novel murine homologue of the Drosophila msh homeobox gene restricted to the dorsal embryonic central nervous system. Mech Dev 58: 203-215. doi: 10.1016/S0925-4773(96)00562-X
![]() |
[116] | MacKenzie A, Ferguson MW, Sharpe PT (1991) Hox-7 expression during murine craniofacial development. Development 113: 601-611. |
[117] |
Satokata I, Maas R (1994) Msx1 deficient mice exhibit cleft palate and abnormalities of craniofacial and tooth development. Nat Genet 6: 348-356. doi: 10.1038/ng0494-348
![]() |
[118] |
Turner N, Grose R (2010) Fibroblast growth factor signalling: from development to cancer. Nat Rev Cancer 10: 116-129. doi: 10.1038/nrc2780
![]() |
[119] |
Isacchi A, Bergonzoni L, Sarmientos P (1990) Complete sequence of a human receptor for acidic and basic fibroblast growth factors. Nucleic Acids Res 18: 1906. doi: 10.1093/nar/18.7.1906
![]() |
[120] |
Pasquale EB, Singer SJ (1989) Identification of a developmentally regulated protein-tyrosine kinase by using anti-phosphotyrosine antibodies to screen a cDNA expression library. Proc Natl Acad Sci USA 86: 5449-5453. doi: 10.1073/pnas.86.14.5449
![]() |
[121] |
Pasquale EB (1990) A distinctive family of embryonic protein-tyrosine kinase receptors. Proc Natl Acad Sci USA 87: 5812-5816. doi: 10.1073/pnas.87.15.5812
![]() |
[122] | Partanen J, Makela TP, Eerola E, et al. (1991) FGFR-4, a novel acidic fibroblast growth factor receptor with a distinct expression pattern. EMBO J 10: 1347-1354. |
[123] |
Wiedemann M, Trueb B (2000) Characterization of a novel protein (FGFRL1) from human cartilage related to FGF receptors. Genomics 69: 275-279. doi: 10.1006/geno.2000.6332
![]() |
[124] |
Sleeman M, Fraser J, McDonald M, et al. (2001) Identification of a new fibroblast growth factor receptor, FGFR5. Gene 271: 171-182. doi: 10.1016/S0378-1119(01)00518-2
![]() |
[125] |
Trueb B (2011) Biology of FGFRL1, the fifth fibroblast growth factor receptor. Cell Mol Life Sci 68: 951-964. doi: 10.1007/s00018-010-0576-3
![]() |
[126] |
Teven CM, Farina EM, Rivas J, et al. (2014) Fibroblast growth factor (FGF) signaling in development and skeletal diseases. Genes Dis 1: 199-213. doi: 10.1016/j.gendis.2014.09.005
![]() |
[127] |
Beenken A, Mohammadi M (2009) The FGF family: biology, pathophysiology and therapy. Nat Rev Drug Discov 8: 235-253. doi: 10.1038/nrd2792
![]() |
[128] |
Green PJ, Walsh FS, Doherty P (1996) Promiscuity of fibroblast growth factor receptors. Bioessays 18: 639-646. doi: 10.1002/bies.950180807
![]() |
[129] | Iseki S, Wilkie AO, Heath JK, et al. (1997) Fgfr2 and osteopontin domains in the developing skull vault are mutually exclusive and can be altered by locally applied FGF2. Development 124: 3375-3384. |
[130] |
Delezoide AL, Benoist-Lasselin C, Legeai-Mallet L, et al. (1998) Spatio-temporal expression of FGFR 1, 2 and 3 genes during human embryo-fetal ossification. Mech Dev 77: 19-30. doi: 10.1016/S0925-4773(98)00133-6
![]() |
[131] |
Gosain AK, Recinos RF, Agresti M, et al. (2004) TGF-beta1, FGF-2, and receptor mRNA expression in suture mesenchyme and dura versus underlying brain in fusing and nonfusing mouse cranial sutures. Plast Reconstr Surg 113: 1675-1684. doi: 10.1097/01.PRS.0000117362.33347.43
![]() |
[132] |
Crossley PH, Minowada G, McArthur CA, et al. (1996) Roles for FGF8 in the induction, initiation, and maintenance of chick limb development. Cell 84: 127-136. doi: 10.1016/S0092-8674(00)80999-X
![]() |
[133] | Sarkar S, Petiot A, Copp A, et al. (2001) FGF2 promotes skeletogenic differentiation of cranial neural crest cells. Development 128: 2143-2152. |
[134] |
Jabs EW, Li X, Scott AF, et al. (1994) Jackson-Weiss and Crouzon syndromes are allelic with mutations in fibroblast growth factor receptor 2. Nat Genet 8: 275-279. doi: 10.1038/ng1194-275
![]() |
[135] |
Muenke M, Schell U, Hehr A, et al. (1994) A common mutation in the fibroblast growth factor receptor 1 gene in Pfeiffer syndrome. Nat Genet 8: 269-274. doi: 10.1038/ng1194-269
![]() |
[136] |
Reardon W, Winter RM, Rutland P, et al. (1994) Mutations in the fibroblast growth factor receptor 2 gene cause Crouzon syndrome. Nat Genet 8: 98-103. doi: 10.1038/ng0994-98
![]() |
[137] |
Shiang R, Thompson LM, Zhu YZ, et al. (1994) Mutations in the transmembrane domain of FGFR3 cause the most common genetic form of dwarfism, achondroplasia. Cell 78: 335-342. doi: 10.1016/0092-8674(94)90302-6
![]() |
[138] | Iseki S, Wilkie AO, Morriss-Kay GM (1999) Fgfr1 and Fgfr2 have distinct differentiation- and proliferation-related roles in the developing mouse skull vault. Development 126: 5611-5620. |
[139] | Kan SH, Elanko N, Johnson D, et al. (2002) Genomic screening of fibroblast growth-factor receptor 2 reveals a wide spectrum of mutations in patients with syndromic craniosynostosis. Am J Hum Genet 70:472-86. |
[140] |
Anderson J, Burns HD, Enriquez-Harris P, et al. (1998) Apert syndrome mutations in fibroblast growth factor receptor 2 exhibit increased affinity for FGF ligand. Hum Mol Genet 7: 1475-1483. doi: 10.1093/hmg/7.9.1475
![]() |
[141] |
Yu K, Herr AB, Waksman G, et al. (2000) Loss of fibroblast growth factor receptor 2 ligand-binding specificity in Apert syndrome. Proc Natl Acad Sci U S A 97:14536-1441. doi: 10.1073/pnas.97.26.14536
![]() |
[142] |
Neilson KM, Friesel RE (1995) Constitutive activation of fibroblast growth factor receptor-2 by a point mutation associated with Crouzon syndrome. J Biol Chem 270: 26037-26040. doi: 10.1074/jbc.270.44.26037
![]() |
[143] |
Robertson SC, Meyer AN, Hart KC, et al. (1998) Activating mutations in the extracellular domain of the fibroblast growth factor receptor 2 function by disruption of the disulfide bond in the third immunoglobulin-like domain. Proc Natl Acad Sci U S A 95: 4567-4572. doi: 10.1073/pnas.95.8.4567
![]() |
[144] |
Oldridge M, Zackai EH, McDonald-McGinn DM, et al. (1999) De novo alu-element insertions in FGFR2 identify a distinct pathological basis for Apert syndrome. Am J Hum Genet 64: 446-461. doi: 10.1086/302245
![]() |
[145] |
Wilkie AOM, Slaney SF, Oldridge M, et al. (1995) Apert syndrome results from localized mutations of FGFR2 and is allelic with Crouzon syndrome. Nat Genet 9: 165-172. doi: 10.1038/ng0295-165
![]() |
[146] |
Przylepa KA, Paznekas W, Zhang M, et al. (1996) Fibroblast growth factor receptor 2 mutations in Beare-Stevenson cutis gyrata syndrome. Nat Genet 13: 492-494. doi: 10.1038/ng0896-492
![]() |
[147] | Li X, Lewanda AF, Eluma F, et al. (1994) Two craniosynostotic syndrome loci, Crouzon and Jackson-Weiss, map to chromosome 10q23-q26. Genomics 22: 418-424. |
[148] |
Dionne CA, Modi WS, Crumley G, et al. (1992) BEK, a receptor for multiple members of the fibroblast growth factor (FGF) family, maps to human chromosome 10q25.3→q26. Cytogenet Cell Genet 60: 34-36. doi: 10.1159/000133290
![]() |
[149] |
McGillivray G, Savarirayan R, Cox T C, et al. (2005) Familial scaphocephaly syndrome caused by a novel mutation in the FGFR2 tyrosine kinase domain. J Med Genet 42: 656-662. doi: 10.1136/jmg.2004.027888
![]() |
[150] |
Robin NH, Feldman GJ, Mitchell HF, et al. (1994) Linkage of Pfeiffer syndrome to chromosome 8 centromere and evidence for genetic heterogeneity. Hum Mol Genet 3: 2153-2158. doi: 10.1093/hmg/3.12.2153
![]() |
[151] |
Zhou YX, Xu X, Chen L, et al. (2000) A Pro250Arg substitution in mouse Fgfr1 causes increased expression of Cbfa1 and premature fusion of calvarial sutures. Hum Mol Genet 9: 2001-2008. doi: 10.1093/hmg/9.13.2001
![]() |
[152] | Schell U, Hehr A, Feldman GJ, et al. (1995) Mutations in FGFR1 and FGFR2 cause familial and sporadic Pfeiffer syndrome. Hum Mol Genet 4: 323-328. |
[153] |
Lajeunie E, Ma HW, Bonaventure J, et al. (1995) FGFR2 mutations in Pfeiffer syndrome. Nat Genet 9: 108. doi: 10.1038/ng0295-108
![]() |
[154] |
Rutland P, Pulleyn LJ, Reardon W, et al. (1995) Identical mutations in the FGFR2 gene cause both Pfeiffer and Crouzon syndrome phenotypes. Nat Genet 9: 173-176. doi: 10.1038/ng0295-173
![]() |
[155] |
Cunningham ML, Seto ML, Ratisoontorn C, et al. (2007) Syndromic craniosynostosis: from history to hydrogen bonds. Orthod Craniofacial Res 10: 67-81. doi: 10.1111/j.1601-6343.2007.00389.x
![]() |
[156] |
Merrill AE, Sarukhanov A, Krejci P, et al. (2012) Bent bone dysplasia-FGFR2 type, a distinct skeletal disorder, has deficient canonical FGF signaling. Am J Hum Genet 90: 550-557. doi: 10.1016/j.ajhg.2012.02.005
![]() |
[157] |
White KE, Cabral JM, Davis SI, et al. (2005) Mutations that cause osteoglophonic dysplasia define novel roles for FGFR1 in bone elongation. Am J Hum Genet 76: 361-367. doi: 10.1086/427956
![]() |
[158] | Farrow EG, Davis SI, Mooney SD, et al. (2006) Extended mutational analyses of FGFR1 in osteoglophonic dysplasia. Am J Med Genet A 140: 537-539. |
[159] | Ibrahimi OA, Zhang F, Eliseenkova AV, et al. (2004) Proline to arginine mutations in FGF receptors 1 and 3 result in Pfeiffer and Muenke craniosynostosis syndromes through enhancement of FGF binding affinity. Hum Mol Genet 13: 69-78. |
[160] |
Cunningham ML, Seto ML, Ratisoontorn C, et al. (2007) Syndromic craniosynostosis: from history to hydrogen bonds. Orthod Craniofac Res 10: 67-81. doi: 10.1111/j.1601-6343.2007.00389.x
![]() |
[161] |
El Ghouzzi V, Lajeunie E, Le Merrer M, et al. (1999) Mutations within or upstream of the basic helix-loop-helix domain of the TWIST gene are specific to Saethre-Chotzen syndrome. Europ J Hum Genet 7: 27-33. doi: 10.1038/sj.ejhg.5200240
![]() |
[162] | Gripp KW, Zackai EH, Stolle CA (2000) Mutations in the human TWIST gene. Hum Mutat 15: 150-155, 2000. |
[163] |
Paznekas WA, Cunningham ML, Howard TD, et al. (1998) Genetic heterogeneity of Saethre-Chotzen syndrome, due to TWIST and FGFR mutations. Am J Hum Genet 62: 1370-1380. doi: 10.1086/301855
![]() |
[164] |
Johnson D, Iseki S, Wilkie AO, et al. (2000) Expression patterns of Twist and Fgfr1, -2 and -3 in the developing mouse coronal suture suggest a key role for twist in suture initiation and biogenesis. Mech Dev 91: 341-345. doi: 10.1016/S0925-4773(99)00278-6
![]() |
[165] | Rice DP, Aberg T, Chan Y, et al. (2000) Integration of FGF and TWIST in calvarial bone and suture development. Development 127: 1845-1855. |
[166] | Kress W, Schropp C, Lieb G, et al. (2006) Saethre-Chotzen syndrome caused by TWIST 1 gene mutations: functional differentiation from Muenke coronal synostosis syndrome. Eur J Hum Genet 14: 39-48. |
[167] |
Sharma VP, Fenwick AL, Brockop MS, et al. (2013) Mutations in TCF12, encoding a basic helix-loop-helix partner of TWIST1, are a frequent cause of coronal craniosynostosis. Nat Genet 45: 304-307. doi: 10.1038/ng.2531
![]() |
[168] | Di Rocco F, Baujat G, Arnaud E, et al. (2014) Clinical spectrum and outcomes in families with coronal synostosis and TCF12 mutations. Eur J Hum Genet 22: 1413-1416. |
[169] | Quintero-Rivera F, Robson CD, Reiss RE, et al. (2006) Intracranial anomalies detected by imaging studies in 30 patients with Apert syndrome. Am J Med Genet A 140: 1337-1338. |
[170] |
Meyers GA, Orlow SJ, Munro IR, et al. (1995) Fibroblast growth factor receptor 3 (FGFR3) transmembrane mutation in Crouzon syndrome with acanthosis nigricans. Nat Genet 11: 462-464. doi: 10.1038/ng1295-462
![]() |
[171] |
Arnaud-Lopez L, Fragoso R, Mantilla-Capacho J, et al. (2007) Crouzon with acanthosis nigricans: further delineation of the syndrome. Clin Genet 72: 405-410. doi: 10.1111/j.1399-0004.2007.00884.x
![]() |
[172] | Cohen MM Jr (1999) Let's call it 'Crouzonodermoskeletal syndrome' so we won't be prisoners of our own conventional terminology. Am J Med Genet 84: 74. |
[173] | Cohen MM Jr, Kreiborg S (1992) Birth prevalence studies of the Crouzon syndrome: comparison of direct and indirect methods. Clin Genet 41: 12-15. |
[174] | Fernandes MB, Maximino LP, Perosa GB, et al. (2016) Apert and Crouzon syndromes-cognitive development, brain abnormalities, and molecular aspects. Am J Med Genet Ain press. |
[175] |
Cohen MM Jr (1993) Pfeiffer syndrome update, clinical subtypes, and guidelines for differential diagnosis. Am J Med Genet 45: 300-307. doi: 10.1002/ajmg.1320450305
![]() |
[176] |
Vogels A, Fryns JP (2006) Pfeiffer syndrome. Orphanet J Rare Dis 1: 19. doi: 10.1186/1750-1172-1-19
![]() |
[177] |
Jenkins D, Seelow D, Jehee FS, et al. (2007) RAB23 mutations in Carpenter syndrome imply an unexpected role for hedgehog signaling in cranial-suture development and obesity. Am J Hum Genet 80: 1162-1170. doi: 10.1086/518047
![]() |
[178] |
Bellus GA, Gaudenz K, Zackai EH, et al. (1996) Identical mutations in three diferente fibroblast growth fator receptor genes in autosomal dominant craniosynostosis syndromes. Nat Genet 14: 174-176. doi: 10.1038/ng1096-174
![]() |
[179] | Muenke M, Gripp KW, McDonald-McGinn DM, et al. (1997) A unique point mutation in the fibroblast growth factor receptor 3 gene (FGFR3) defines a new craniosynostosis syndrome. Am J Hum Genet 60: 555-564. |
[180] | Kruszka P, Addissie YA, Yarnell CM, et al. (2016) Muenke syndrome: An international multicenter natural history study. Am J Med Genet A in press. |
[181] | Czeizel AE, Elek C, Susanszky E (1993) Birth prevalence study of Apert syndrome. Am J Med Genet 45: 392. |
[182] | Orphanet report series, Prevalence of rare diseases: Bibliographic data. July 2015, Number 1. Available from:http://www.orpha.net/orphacom/cahiers/docs/GB/Prevalence_of_rare_diseases_by_alphabetical_list.pdf |
[183] | Carpenter syndrome. Available from: http://www.orpha.net/consor/cgi-bin/OC_Exp.php?Lng=GB&Expert=65759 |
1. | Zeynep Gökçe İşlier, Refik Güllü, Wolfgang Hörmann, An exact and implementable computation of the final outbreak size distribution under Erlang distributed infectious period, 2020, 325, 00255564, 108363, 10.1016/j.mbs.2020.108363 | |
2. | Elena Almaraz, Antonio Gómez‐Corral, Number of infections suffered by a focal individual in a two‐strain SIS model with partial cross‐immunity, 2019, 42, 0170-4214, 4318, 10.1002/mma.5652 | |
3. | Antonio Gómez-Corral, Martín López-García, Maria Jesus Lopez-Herrero, Diana Taipe, On First-Passage Times and Sojourn Times in Finite QBD Processes and Their Applications in Epidemics, 2020, 8, 2227-7390, 1718, 10.3390/math8101718 | |
4. | R. Fernández-Peralta, A. Gómez-Corral, A structured Markov chain model to investigate the effects of pre-exposure vaccines in tuberculosis control, 2021, 509, 00225193, 110490, 10.1016/j.jtbi.2020.110490 | |
5. | J. Amador, A. Gómez-Corral, A stochastic epidemic model with two quarantine states and limited carrying capacity for quarantine, 2020, 544, 03784371, 121899, 10.1016/j.physa.2019.121899 | |
6. | Kaiyan Zhao, Shaojuan Ma, Qualitative analysis of a two-group SVIR epidemic model with random effect, 2021, 2021, 1687-1847, 10.1186/s13662-021-03332-w | |
7. | A. Gómez-Corral, M. López-García, M. T. Rodríguez-Bernal, On time-discretized versions of the stochastic SIS epidemic model: a comparative analysis, 2021, 82, 0303-6812, 10.1007/s00285-021-01598-y | |
8. | Gilberto González-Parra, Abraham J. Arenas, Mathematical Modeling of SARS-CoV-2 Omicron Wave under Vaccination Effects, 2023, 11, 2079-3197, 36, 10.3390/computation11020036 | |
9. | Gilberto Gonzalez-Parra, Abraham J. Arenas, Nonlinear Dynamics of the Introduction of a New SARS-CoV-2 Variant with Different Infectiousness, 2021, 9, 2227-7390, 1564, 10.3390/math9131564 | |
10. | Lubna Pinky, Hana M. Dobrovolny, Epidemiological Consequences of Viral Interference: A Mathematical Modeling Study of Two Interacting Viruses, 2022, 13, 1664-302X, 10.3389/fmicb.2022.830423 | |
11. | Taye Samuel Faniran, Aatif Ali, Nawal E. Al-Hazmi, Joshua Kiddy K. Asamoah, Taher A. Nofal, Matthew O. Adewole, Dan Selişteanu, New Variant of SARS-CoV-2 Dynamics with Imperfect Vaccine, 2022, 2022, 1099-0526, 1, 10.1155/2022/1062180 | |
12. | Robin N. Thompson, Emma Southall, Yair Daon, Francesca A. Lovell-Read, Shingo Iwami, Craig P. Thompson, Uri Obolski, The impact of cross-reactive immunity on the emergence of SARS-CoV-2 variants, 2023, 13, 1664-3224, 10.3389/fimmu.2022.1049458 | |
13. | Gilberto González-Parra, Abraham J. Arenas, Qualitative analysis of a mathematical model with presymptomatic individuals and two SARS-CoV-2 variants, 2021, 40, 2238-3603, 10.1007/s40314-021-01592-6 | |
14. | Fabio A. C. C. Chalub, Antonio Gómez‐Corral, Martín López‐García, Fátima Palacios‐Rodríguez, A Markov chain model to investigate the spread of antibiotic‐resistant bacteria in hospitals, 2023, 151, 0022-2526, 1498, 10.1111/sapm.12637 | |
15. | Suman Kumari, Partha Sarathi Mandal, Moitri Sen, First passage time and peak size probability distributions for a complex epidemic model, 2024, 139, 2190-5444, 10.1140/epjp/s13360-024-05108-z | |
16. | Rukhsar Ikram, Amir Khan, Aeshah A. Raezah, Impact of supervise neural network on a stochastic epidemic model with Levy noise, 2024, 9, 2473-6988, 21273, 10.3934/math.20241033 | |
17. | Md. Mamun-Ur-Rashid Khan, Md. Rajib Arefin, Jun Tanimoto, Time delay of the appearance of a new strain can affect vaccination behavior and disease dynamics: An evolutionary explanation, 2023, 8, 24680427, 656, 10.1016/j.idm.2023.06.001 | |
18. | A. Di Crescenzo, A. Gómez-Corral, D. Taipe, A computational approach to extreme values and related hitting probabilities in level-dependent quasi-birth–death processes, 2025, 228, 03784754, 211, 10.1016/j.matcom.2024.08.019 | |
19. | Jing Yang, Shaojuan Ma, Juan Ma, Jinhua Ran, Xinyu Bai, Stochastic Analysis for the Dual Virus Parallel Transmission Model with Immunity Delay, 2024, 1557-8666, 10.1089/cmb.2024.0662 | |
20. | Shirali Kadyrov, Farkhod Haydarov, Khudoyor Mamayusupov, Komil Mustayev, Endemic coexistence and competition of virus variants under partial cross-immunity, 2025, 33, 2688-1594, 1120, 10.3934/era.2025050 |
Genus | Occurrences | Sampling Areas | T (℃) | S (psu) | DIN (μM) | PO43- (μΜ) | SiO2 (μΜ) | N:P |
Alexandrium | 25 | K, R1 | 14.76 (9.8-23.9) |
37.81 (34.0-40.1) |
5.78 (0.5-39.8) |
0.12 (0.0-1.5) |
15.97 (3.4-86.6) |
196.64 (7.8-1217.5) |
Amphidinium | 71 | K, S, R1, R2 | 17.04 (9.8-23.9) |
38.26 (34.0-40.2) |
4.78 (0.5-39.8) |
0.13 (0.0-1.5) |
14.34 (2.4-86.6) |
232.41 (1.7-6020.0) |
Cryptomonas | 168 | K | 17.10 (9.5-27.7) |
38.57 (34.0-41.1) |
4.13 (0.5-45.2) |
0.09 (0.0-1.6) |
14.36 (1.7-94.0) |
180.16 (6.81-6020.0) |
Dictyocha | 48 | K | 13.93 (9.4-23.3) |
38.29 (34.8-40.7) |
4.46 (0.6-33.2) |
0.10 (0.0-1.3) |
16.22 (4.3-70.9) |
102.69 (7.4-692.2) |
Dinophysis | 228 | K, G, M, S, R1, R2 | 18.27 (9.7-28.2) |
38.29 (28.3-40.6) |
2.70 (0.1-38.0) |
0.16 (0.0-1.8) |
8.85 (0.4-80.7) |
43.76 (2.8-1712.0) |
Goniodoma | 21 | G, M, S, R1, R2 | 19.20 (13.4-27.4) |
38.35 (37.3-39.1) |
2.84 (0.4-11.7) |
0.45 (0.0-6.0) |
9.20 (0.8-15.1) |
25.41 (1.5-116.7) |
Gonyaulax | 37 | K, G, M, S, R1, R2 | 18.41 (14.37-22.11) |
38.82 (36.4-40.6) |
1.66 (0.3-4.1) |
0.19 (0.0-0.85) |
7.19 (2.1-20.9) |
30.05 (2.7-379.0) |
Gymnodinium | 279 | K, M, S, R1, R2 | 19.58 (9.6-27.7) |
38.83 (28.0-40.2) |
1.76 (0.1-27.8) |
0.07 (0.0-4.1) |
9.66 (1.6-53.9) |
68.13 (0.2-1660.0) |
Gyrodinium | 177 | K, G, M, S, R1, R2 | 18.23 (9.7-27.7) |
38.51 (34.7-40.3) |
2.78 (0.4-45.2) |
0.16 (0.0-1.8) |
8.63 (1.5-94.0) |
70.01 (1.7-1660.0) |
Karenia | 394 | S, R1, R2 | 20.00 (13.1-27.6) |
38.55 (28.0-39.7) |
2.01 (0.1-38.0) |
0.15 (0.0-6.0) |
8.02 (0.4-53.9) |
33.04 (1.4-1643.0) |
Karlodinium | 133 | K | 17.20 (9.9-27.7) |
38.68 (34.8-40.7) |
3.51 (0.5-33.2) |
0.07 (0.0-1.3) |
12.64 (1.7-70.9) |
180.74 (6.8-6020.0) |
Lingulodinium | 23 | K, R1 | 14.81 (9.9-25.6) |
37.67 (34.8-40.0) |
6.00 (1.4-33.2) |
0.10 (0.0-1.3) |
11.81 (2.4-68.7) |
282.78 (9.6-1660.0) |
Navicula | 165 | G, M, S, R1, R2 | 19.27 (10.0-26.5) |
39.00 (37.1-40.3) |
2.22 (0.3-23.8) |
0.14 (0.0-4.1) |
9.22 (1.1-53.9) |
44.78 (0.2-1643.0) |
Peridinium | 301 | K, G, M, S, R1, R2 | 18.92 (9.6-27.7) |
38.62 (28.0-40.3) |
2.60 (0.1-39.8) |
0.11 (0.0-2.3) |
9.87 (1.2-86.6) |
71.58 (1.7-1712.0) |
Phaeocystis | 71 | S, R1 | 16.4 (13.1-26.7) |
38.27 (37.6-39.1) |
2.98 (0.5-14.1) |
0.36 (0.0-6.0) |
5.44 (0.4-17.2) |
16.24 (1.4-72.5) |
Polykrikos | 38 | K, M, R1 | 14.88 (10.0-25.6) |
38.7 (34.9-40.4) |
3.77 (0.6-22.8) |
0.06 (0.0-0.9) |
9.56 (1.1-70.9) |
346.81 (11.9-6020.0) |
Prorocentrum | 777 | K, G, M, S, R1, R2 | 19.10 (9.4-28.2) |
38.61 (28.0-41.1) |
2.26 (0.1-38.0) |
0.13 (0.0-6.0) |
8.43 (0.4-83.0) |
51.69 (1.4-1660.0) |
Pseudo-nitzschia | 491 | K, G, M, S, R1, R2 | 17.39 (9.4-27.7) |
38.5 (28.3-41.1) |
2.89 (0.4-45.2) |
0.14 (0.0-6.0) |
8.92 (0.4-94.0) |
78.51 (1.4-1712.0) |
Category | Abbreviation | Algorithm Description | Hyper parameter values |
Rules | Jrip |
Repeated incremental pruning to produce error reduction (RIPPER) | Batch size = 50,100 Min total weight = 2, 5 |
Part | PART decision list | Batch size = 50,100 Min number of instances per rule = 2, 5 |
|
Trees | J48 | C4 pruned decision tree | Batch size = 50,100 Min number of instances per leaf = 2, 5 Number of folds = 3, 5 |
Rep | Decision tree using reduced error pruning with backfiting | Batch size = 50,100 Min number of instances per leaf = 2, 5 |
|
Lazy | IBk | The k-nearest neighbors using Euclidean distance | Number of neighbors = 1, 5, 10, 20 |
KStar | Nearest neighbor with entropic distance | Global blend = 5, 10, 20, 50 | |
Functions | Log | Multinomial logistic regression | |
MLP | Multilayer Perceptron using backpropagation | Number of neurons = 2, 5, 7, 10 | |
Bayes | NB | Naïve Bayes using estimator classes | Batch size = 50,100 |
BN | Bayes network | Batch size = 50,100 | |
Ensembles | RF | Forest of random decision trees | Batch size = 50,100 Number of iterations = 20, 50,100 |
Bagging | Bagging classifiers to reduce variance | Classifiers: The best basic one Number of iterations = 10 |
|
Boosting | Boosting classifiers using Adaboost M1 method | Classifier: The best basic one | |
CVR | Classification using regression methods | Classifier: M5 model tree | |
RC | Randomizabling classifiers (Random Committee) | Classifier: Random tree | |
Stacking | Combining classifiers using stacking method | Classifiers: The 3 best basic ones Number of folds = 10 |
|
Voting | Combining classifiers using votes | Classifiers: The 3 best basic ones Combination rule: Average of Probabilities |
Rules | Trees | Lazy | Functions | Bayes | Ensembles | ||||||||||||
HA genus | Jrip | Part | J48 | Rep | IBK | KStar | Logistic | MLP | NB | BN | RF | Bagging | Boosting | CVR | RC | Stacking | Voting |
Alexandrium | 0.970 | 0.973 | 0.972 | 0.972 | 0.976 | 0.968 | 0.971 | 0.972 | 0.957 | 0.965 | 0.973 | 0.972 | 0.968 | 0.972 | 0.972 | 0.972 | 0.972 |
Amphidinium | 0.918 | 0.919 | 0.916 | 0.917 | 0.916 | 0.917 | 0.919 | 0.916 | 0.907 | 0.910 | 0.925 | 0.921 | 0.917 | 0.919 | 0.922 | 0.920 | 0.917 |
Cryptomonas | 0.925 | 0.885 | 0.899 | 0.873 | 0.749 | 0.907 | 0.858 | 0.894 | 0.838 | 0.910 | 0.936 | 0.936 | 0.942 | 0.882 | 0.904 | 0.811 | 0.940 |
Dictyocha | 0.954 | 0.943 | 0.947 | 0.943 | 0.955 | 0.953 | 0.943 | 0.943 | 0.936 | 0.948 | 0.965 | 0.946 | 0.946 | 0.940 | 0.943 | 0.946 | 0.937 |
Dinophysis | 0.759 | 0.771 | 0.756 | 0.748 | 0.741 | 0.727 | 0.727 | 0.744 | 0.731 | 0.780 | 0.769 | 0.760 | 0.780 | 0.767 | 0.736 | 0.744 | 0.770 |
Goniodoma | 0.976 | 0.976 | 0.976 | 0.976 | 0.979 | 0.970 | 0.975 | 0.975 | 0.965 | 0.976 | 0.976 | 0.978 | 0.979 | 0.977 | 0.970 | 0.976 | 0.976 |
Gonyaulax | 0.957 | 0.955 | 0.958 | 0.958 | 0.955 | 0.954 | 0.956 | 0.958 | 0.926 | 0.926 | 0.961 | 0.960 | 0.961 | 0.958 | 0.953 | 0.958 | 0.958 |
Gymnodinium | 0.772 | 0.780 | 0.793 | 0.772 | 0.692 | 0.803 | 0.680 | 0.742 | 0.524 | 0.753 | 0.846 | 0.811 | 0.773 | 0.812 | 0.802 | 0.686 | 0.827 |
Gyrodinium | 0.793 | 0.802 | 0.802 | 0.800 | 0.729 | 0.801 | 0.800 | 0.798 | 0.775 | 0.799 | 0.827 | 0.812 | 0.790 | 0.799 | 0.790 | 0.801 | 0.808 |
Karenia | 0.813 | 0.762 | 0.757 | 0.765 | 0.779 | 0.791 | 0.588 | 0.729 | 0.650 | 0.666 | 0.848 | 0.791 | 0.794 | 0.772 | 0.799 | 0.683 | 0.805 |
Karlodinium | 0.901 | 0.879 | 0.880 | 0.889 | 0.748 | 0.898 | 0.857 | 0.884 | 0.852 | 0.916 | 0.917 | 0.915 | 0.916 | 0.899 | 0.911 | 0.850 | 0.916 |
Lingulodinium | 0.980 | 0.981 | 0.981 | 0.978 | 0.974 | 0.972 | 0.973 | 0.974 | 0.966 | 0.974 | 0.980 | 0.976 | 0.975 | 0.974 | 0.981 | 0.980 | 0.981 |
Navicula | 0.812 | 0.818 | 0.814 | 0.805 | 0.803 | 0.792 | 0.814 | 0.832 | 0.791 | 0.814 | 0.822 | 0.814 | 0.800 | 0.838 | 0.799 | 0.814 | 0.814 |
Peridinium | 0.714 | 0.706 | 0.687 | 0.685 | 0.586 | 0.679 | 0.658 | 0.674 | 0.654 | 0.650 | 0.722 | 0.719 | 0.717 | 0.708 | 0.690 | 0.661 | 0.717 |
Phaeocystis | 0.947 | 0.944 | 0.945 | 0.952 | 0.951 | 0.934 | 0.918 | 0.961 | 0.916 | 0.951 | 0.958 | 0.961 | 0.956 | 0.947 | 0.954 | 0.920 | 0.961 |
Polykrikos | 0.957 | 0.962 | 0.961 | 0.961 | 0.964 | 0.955 | 0.958 | 0.958 | 0.945 | 0.958 | 0.962 | 0.961 | 0.958 | 0.962 | 0.958 | 0.960 | 0.963 |
Prorocentrum | 0.876 | 0.875 | 0.874 | 0.875 | 0.876 | 0.880 | 0.874 | 0.874 | 0.862 | 0.874 | 0.877 | 0.877 | 0.872 | 0.875 | 0.854 | 0.874 | 0.874 |
Pseudo-nitzschia | 0.757 | 0.756 | 0.761 | 0.754 | 0.771 | 0.765 | 0.665 | 0.699 | 0.565 | 0.737 | 0.791 | 0.768 | 0.771 | 0.762 | 0.750 | 0.653 | 0.786 |
Mean | 0.877 | 0.872 | 0.871 | 0.868 | 0.841 | 0.870 | 0.841 | 0.863 | 0.820 | 0.862 | 0.892 | 0.882 | 0.879 | 0.876 | 0.872 | 0.845 | 0.885 |
HA genus | Best Algorithm | Hyper Paremeters values | Best Parameter Combination |
Alexandrium | IBk | Number of neighbors=5 | T+S+PO43- |
Amphidinium | RF | Batch size=50 Number of iterations=50 |
T+S+DIN+ PO43-+SiO2 |
Cryptomonas | Boosting | Classifier: Jrip (Batch size=100 Min total weight=5) |
T+S+DIN+ PO43-+SiO2 |
Dictyocha | RF | Batch size = 100 Number of iterations = 50 |
T + S + PO4 + SiO2 |
Dinophysis | BN/ Boosting |
Batch size = 100/ Classifier: IBk (Number of neighbors = 10) |
T + S + DIN + PO43- + SiO2 |
Goniodoma | IBk | Number of neighbors = 10 | T + S + DIN + PO43- + SiO2 |
Gonyaulax | RF | Batch size = 100 Number of iterations = 100 |
T + S + DIN + PO43- + SiO2 |
Gymnodinium | RF | Batch size = 50 Number of iterations = 50 |
T + S + DIN + PO43- + SiO2 |
Gyrodinium | RF | Batch size = 50 Number of iterations = 100 |
T + S + DIN + PO43- +SiO2 |
Karenia | RF | Batch size = 50 Number of iterations = 100 |
T + S + PO43- + SiO2 |
Karlodinium | RF | Batch size = 50 Number of iterations = 100 |
T + S + DIN+ PO43- +SiO2 |
Lingulodinium | Part/ RC/ Voting |
Batch size = 50, Min number of instances per rule =2/ Classifier: Random tree/ Classifiers: Jrip (Batch size = 100, Min total weight = 5), Part (Batch size = 100, Min number of instances per rule = 2), J48 (Batch size = 100, Min number of instances per leaf = 5) |
T + S + DIN + PO43- +SiO2 |
Navicula | CVR | Classifier: M5 model tree | T + S + DIN + PO43- + SiO2 |
Peridinium | RF | Batch size = 50 Number of iterations = 100 |
T + S + DIN + PO43- + SiO2 |
Phaeocystis | MLP/Bagging/Voting | Number of neurons = 7/ Classifier:MLP/ Classifiers: MLP, Rep (Batch size = 50, Min number of instances per leaf = 2), BN (Batch size = 100) |
T + S + DIN + PO43- + SiO2 |
Polykrikos | IBk | Number of neighbors = 10 | T + S + PO43- |
Prorocentrum | KStar | Global blend = 50 | T + S + PO43- |
Pseudo-nitzschia | RF | Batch size = 50 Number of iterations = 100 |
T + S + DIN + PO43- + SiO2 |
Algae genus | N# presences | Precision | Sensitivity | Specificity | Kappa statistic | Discriminant power |
Alexandrium | 25 | 0.556 | 0.200 | 0.995 | 0.312 | 2.154 |
Amphidinium | 71 | 0.567 | 0.239 | 0.984 | 0.386 | 1.632 |
Cryptomonas | 168 | 0.878 | 0.774 | 0.974 | 0.723 | |
Dictyocha | 48 | 0.615 | 0.333 | 0.988 | 0.503 | 2.049 |
Dinophysis | 228 | 0.647 | 0.407 | 0.912 | 0.500 | |
Goniodoma | 21 | 0.667 | 0.095 | 0.999 | 0.324 | 2.565 |
Gonyaulax | 37 | 0.667 | 0.108 | 0.998 | 0.287 | 2.261 |
Gymnodinium | 279 | 0.814 | 0.659 | 0.931 | 0.655 | |
Gyrodinium | 177 | 0.682 | 0.353 | 0.970 | 0.509 | |
Karenia | 394 | 0.758 | 0.787 | 0.800 | 0.604 | |
Karlodinium | 133 | 0.915 | 0.489 | 0.992 | 0.595 | |
Lingulodinium | 23 | 0.833 | 0.217 | 0.999 | 0.291 | 3.100 |
Navicula | 165 | 0.550 | 0.200 | 0.963 | 0.480 | |
Peridinium | 301 | 0.642 | 0.405 | 0.884 | 0.518 | |
Phaeocystis | 71 | 0.867 | 0.549 | 0.993 | 0.633 | 2.840 |
Polykrikos | 38 | 0.667 | 0.158 | 0.996 | 0.349 | 2.119 |
Prorocentrum | 777 | 0.881 | 0.985 | 0.429 | 0.375 | |
Pseudo-nitzschia | 490 | 0.811 | 0.808 | 0.769 | 0.668 | |
Mean values | 0.723 | 0.431 | 0.921 | 0.469 | 2.340 |
Genus | Occurrences | Sampling Areas | T (℃) | S (psu) | DIN (μM) | PO43- (μΜ) | SiO2 (μΜ) | N:P |
Alexandrium | 25 | K, R1 | 14.76 (9.8-23.9) |
37.81 (34.0-40.1) |
5.78 (0.5-39.8) |
0.12 (0.0-1.5) |
15.97 (3.4-86.6) |
196.64 (7.8-1217.5) |
Amphidinium | 71 | K, S, R1, R2 | 17.04 (9.8-23.9) |
38.26 (34.0-40.2) |
4.78 (0.5-39.8) |
0.13 (0.0-1.5) |
14.34 (2.4-86.6) |
232.41 (1.7-6020.0) |
Cryptomonas | 168 | K | 17.10 (9.5-27.7) |
38.57 (34.0-41.1) |
4.13 (0.5-45.2) |
0.09 (0.0-1.6) |
14.36 (1.7-94.0) |
180.16 (6.81-6020.0) |
Dictyocha | 48 | K | 13.93 (9.4-23.3) |
38.29 (34.8-40.7) |
4.46 (0.6-33.2) |
0.10 (0.0-1.3) |
16.22 (4.3-70.9) |
102.69 (7.4-692.2) |
Dinophysis | 228 | K, G, M, S, R1, R2 | 18.27 (9.7-28.2) |
38.29 (28.3-40.6) |
2.70 (0.1-38.0) |
0.16 (0.0-1.8) |
8.85 (0.4-80.7) |
43.76 (2.8-1712.0) |
Goniodoma | 21 | G, M, S, R1, R2 | 19.20 (13.4-27.4) |
38.35 (37.3-39.1) |
2.84 (0.4-11.7) |
0.45 (0.0-6.0) |
9.20 (0.8-15.1) |
25.41 (1.5-116.7) |
Gonyaulax | 37 | K, G, M, S, R1, R2 | 18.41 (14.37-22.11) |
38.82 (36.4-40.6) |
1.66 (0.3-4.1) |
0.19 (0.0-0.85) |
7.19 (2.1-20.9) |
30.05 (2.7-379.0) |
Gymnodinium | 279 | K, M, S, R1, R2 | 19.58 (9.6-27.7) |
38.83 (28.0-40.2) |
1.76 (0.1-27.8) |
0.07 (0.0-4.1) |
9.66 (1.6-53.9) |
68.13 (0.2-1660.0) |
Gyrodinium | 177 | K, G, M, S, R1, R2 | 18.23 (9.7-27.7) |
38.51 (34.7-40.3) |
2.78 (0.4-45.2) |
0.16 (0.0-1.8) |
8.63 (1.5-94.0) |
70.01 (1.7-1660.0) |
Karenia | 394 | S, R1, R2 | 20.00 (13.1-27.6) |
38.55 (28.0-39.7) |
2.01 (0.1-38.0) |
0.15 (0.0-6.0) |
8.02 (0.4-53.9) |
33.04 (1.4-1643.0) |
Karlodinium | 133 | K | 17.20 (9.9-27.7) |
38.68 (34.8-40.7) |
3.51 (0.5-33.2) |
0.07 (0.0-1.3) |
12.64 (1.7-70.9) |
180.74 (6.8-6020.0) |
Lingulodinium | 23 | K, R1 | 14.81 (9.9-25.6) |
37.67 (34.8-40.0) |
6.00 (1.4-33.2) |
0.10 (0.0-1.3) |
11.81 (2.4-68.7) |
282.78 (9.6-1660.0) |
Navicula | 165 | G, M, S, R1, R2 | 19.27 (10.0-26.5) |
39.00 (37.1-40.3) |
2.22 (0.3-23.8) |
0.14 (0.0-4.1) |
9.22 (1.1-53.9) |
44.78 (0.2-1643.0) |
Peridinium | 301 | K, G, M, S, R1, R2 | 18.92 (9.6-27.7) |
38.62 (28.0-40.3) |
2.60 (0.1-39.8) |
0.11 (0.0-2.3) |
9.87 (1.2-86.6) |
71.58 (1.7-1712.0) |
Phaeocystis | 71 | S, R1 | 16.4 (13.1-26.7) |
38.27 (37.6-39.1) |
2.98 (0.5-14.1) |
0.36 (0.0-6.0) |
5.44 (0.4-17.2) |
16.24 (1.4-72.5) |
Polykrikos | 38 | K, M, R1 | 14.88 (10.0-25.6) |
38.7 (34.9-40.4) |
3.77 (0.6-22.8) |
0.06 (0.0-0.9) |
9.56 (1.1-70.9) |
346.81 (11.9-6020.0) |
Prorocentrum | 777 | K, G, M, S, R1, R2 | 19.10 (9.4-28.2) |
38.61 (28.0-41.1) |
2.26 (0.1-38.0) |
0.13 (0.0-6.0) |
8.43 (0.4-83.0) |
51.69 (1.4-1660.0) |
Pseudo-nitzschia | 491 | K, G, M, S, R1, R2 | 17.39 (9.4-27.7) |
38.5 (28.3-41.1) |
2.89 (0.4-45.2) |
0.14 (0.0-6.0) |
8.92 (0.4-94.0) |
78.51 (1.4-1712.0) |
Category | Abbreviation | Algorithm Description | Hyper parameter values |
Rules | Jrip |
Repeated incremental pruning to produce error reduction (RIPPER) | Batch size = 50,100 Min total weight = 2, 5 |
Part | PART decision list | Batch size = 50,100 Min number of instances per rule = 2, 5 |
|
Trees | J48 | C4 pruned decision tree | Batch size = 50,100 Min number of instances per leaf = 2, 5 Number of folds = 3, 5 |
Rep | Decision tree using reduced error pruning with backfiting | Batch size = 50,100 Min number of instances per leaf = 2, 5 |
|
Lazy | IBk | The k-nearest neighbors using Euclidean distance | Number of neighbors = 1, 5, 10, 20 |
KStar | Nearest neighbor with entropic distance | Global blend = 5, 10, 20, 50 | |
Functions | Log | Multinomial logistic regression | |
MLP | Multilayer Perceptron using backpropagation | Number of neurons = 2, 5, 7, 10 | |
Bayes | NB | Naïve Bayes using estimator classes | Batch size = 50,100 |
BN | Bayes network | Batch size = 50,100 | |
Ensembles | RF | Forest of random decision trees | Batch size = 50,100 Number of iterations = 20, 50,100 |
Bagging | Bagging classifiers to reduce variance | Classifiers: The best basic one Number of iterations = 10 |
|
Boosting | Boosting classifiers using Adaboost M1 method | Classifier: The best basic one | |
CVR | Classification using regression methods | Classifier: M5 model tree | |
RC | Randomizabling classifiers (Random Committee) | Classifier: Random tree | |
Stacking | Combining classifiers using stacking method | Classifiers: The 3 best basic ones Number of folds = 10 |
|
Voting | Combining classifiers using votes | Classifiers: The 3 best basic ones Combination rule: Average of Probabilities |
Rules | Trees | Lazy | Functions | Bayes | Ensembles | ||||||||||||
HA genus | Jrip | Part | J48 | Rep | IBK | KStar | Logistic | MLP | NB | BN | RF | Bagging | Boosting | CVR | RC | Stacking | Voting |
Alexandrium | 0.970 | 0.973 | 0.972 | 0.972 | 0.976 | 0.968 | 0.971 | 0.972 | 0.957 | 0.965 | 0.973 | 0.972 | 0.968 | 0.972 | 0.972 | 0.972 | 0.972 |
Amphidinium | 0.918 | 0.919 | 0.916 | 0.917 | 0.916 | 0.917 | 0.919 | 0.916 | 0.907 | 0.910 | 0.925 | 0.921 | 0.917 | 0.919 | 0.922 | 0.920 | 0.917 |
Cryptomonas | 0.925 | 0.885 | 0.899 | 0.873 | 0.749 | 0.907 | 0.858 | 0.894 | 0.838 | 0.910 | 0.936 | 0.936 | 0.942 | 0.882 | 0.904 | 0.811 | 0.940 |
Dictyocha | 0.954 | 0.943 | 0.947 | 0.943 | 0.955 | 0.953 | 0.943 | 0.943 | 0.936 | 0.948 | 0.965 | 0.946 | 0.946 | 0.940 | 0.943 | 0.946 | 0.937 |
Dinophysis | 0.759 | 0.771 | 0.756 | 0.748 | 0.741 | 0.727 | 0.727 | 0.744 | 0.731 | 0.780 | 0.769 | 0.760 | 0.780 | 0.767 | 0.736 | 0.744 | 0.770 |
Goniodoma | 0.976 | 0.976 | 0.976 | 0.976 | 0.979 | 0.970 | 0.975 | 0.975 | 0.965 | 0.976 | 0.976 | 0.978 | 0.979 | 0.977 | 0.970 | 0.976 | 0.976 |
Gonyaulax | 0.957 | 0.955 | 0.958 | 0.958 | 0.955 | 0.954 | 0.956 | 0.958 | 0.926 | 0.926 | 0.961 | 0.960 | 0.961 | 0.958 | 0.953 | 0.958 | 0.958 |
Gymnodinium | 0.772 | 0.780 | 0.793 | 0.772 | 0.692 | 0.803 | 0.680 | 0.742 | 0.524 | 0.753 | 0.846 | 0.811 | 0.773 | 0.812 | 0.802 | 0.686 | 0.827 |
Gyrodinium | 0.793 | 0.802 | 0.802 | 0.800 | 0.729 | 0.801 | 0.800 | 0.798 | 0.775 | 0.799 | 0.827 | 0.812 | 0.790 | 0.799 | 0.790 | 0.801 | 0.808 |
Karenia | 0.813 | 0.762 | 0.757 | 0.765 | 0.779 | 0.791 | 0.588 | 0.729 | 0.650 | 0.666 | 0.848 | 0.791 | 0.794 | 0.772 | 0.799 | 0.683 | 0.805 |
Karlodinium | 0.901 | 0.879 | 0.880 | 0.889 | 0.748 | 0.898 | 0.857 | 0.884 | 0.852 | 0.916 | 0.917 | 0.915 | 0.916 | 0.899 | 0.911 | 0.850 | 0.916 |
Lingulodinium | 0.980 | 0.981 | 0.981 | 0.978 | 0.974 | 0.972 | 0.973 | 0.974 | 0.966 | 0.974 | 0.980 | 0.976 | 0.975 | 0.974 | 0.981 | 0.980 | 0.981 |
Navicula | 0.812 | 0.818 | 0.814 | 0.805 | 0.803 | 0.792 | 0.814 | 0.832 | 0.791 | 0.814 | 0.822 | 0.814 | 0.800 | 0.838 | 0.799 | 0.814 | 0.814 |
Peridinium | 0.714 | 0.706 | 0.687 | 0.685 | 0.586 | 0.679 | 0.658 | 0.674 | 0.654 | 0.650 | 0.722 | 0.719 | 0.717 | 0.708 | 0.690 | 0.661 | 0.717 |
Phaeocystis | 0.947 | 0.944 | 0.945 | 0.952 | 0.951 | 0.934 | 0.918 | 0.961 | 0.916 | 0.951 | 0.958 | 0.961 | 0.956 | 0.947 | 0.954 | 0.920 | 0.961 |
Polykrikos | 0.957 | 0.962 | 0.961 | 0.961 | 0.964 | 0.955 | 0.958 | 0.958 | 0.945 | 0.958 | 0.962 | 0.961 | 0.958 | 0.962 | 0.958 | 0.960 | 0.963 |
Prorocentrum | 0.876 | 0.875 | 0.874 | 0.875 | 0.876 | 0.880 | 0.874 | 0.874 | 0.862 | 0.874 | 0.877 | 0.877 | 0.872 | 0.875 | 0.854 | 0.874 | 0.874 |
Pseudo-nitzschia | 0.757 | 0.756 | 0.761 | 0.754 | 0.771 | 0.765 | 0.665 | 0.699 | 0.565 | 0.737 | 0.791 | 0.768 | 0.771 | 0.762 | 0.750 | 0.653 | 0.786 |
Mean | 0.877 | 0.872 | 0.871 | 0.868 | 0.841 | 0.870 | 0.841 | 0.863 | 0.820 | 0.862 | 0.892 | 0.882 | 0.879 | 0.876 | 0.872 | 0.845 | 0.885 |
HA genus | Best Algorithm | Hyper Paremeters values | Best Parameter Combination |
Alexandrium | IBk | Number of neighbors=5 | T+S+PO43- |
Amphidinium | RF | Batch size=50 Number of iterations=50 |
T+S+DIN+ PO43-+SiO2 |
Cryptomonas | Boosting | Classifier: Jrip (Batch size=100 Min total weight=5) |
T+S+DIN+ PO43-+SiO2 |
Dictyocha | RF | Batch size = 100 Number of iterations = 50 |
T + S + PO4 + SiO2 |
Dinophysis | BN/ Boosting |
Batch size = 100/ Classifier: IBk (Number of neighbors = 10) |
T + S + DIN + PO43- + SiO2 |
Goniodoma | IBk | Number of neighbors = 10 | T + S + DIN + PO43- + SiO2 |
Gonyaulax | RF | Batch size = 100 Number of iterations = 100 |
T + S + DIN + PO43- + SiO2 |
Gymnodinium | RF | Batch size = 50 Number of iterations = 50 |
T + S + DIN + PO43- + SiO2 |
Gyrodinium | RF | Batch size = 50 Number of iterations = 100 |
T + S + DIN + PO43- +SiO2 |
Karenia | RF | Batch size = 50 Number of iterations = 100 |
T + S + PO43- + SiO2 |
Karlodinium | RF | Batch size = 50 Number of iterations = 100 |
T + S + DIN+ PO43- +SiO2 |
Lingulodinium | Part/ RC/ Voting |
Batch size = 50, Min number of instances per rule =2/ Classifier: Random tree/ Classifiers: Jrip (Batch size = 100, Min total weight = 5), Part (Batch size = 100, Min number of instances per rule = 2), J48 (Batch size = 100, Min number of instances per leaf = 5) |
T + S + DIN + PO43- +SiO2 |
Navicula | CVR | Classifier: M5 model tree | T + S + DIN + PO43- + SiO2 |
Peridinium | RF | Batch size = 50 Number of iterations = 100 |
T + S + DIN + PO43- + SiO2 |
Phaeocystis | MLP/Bagging/Voting | Number of neurons = 7/ Classifier:MLP/ Classifiers: MLP, Rep (Batch size = 50, Min number of instances per leaf = 2), BN (Batch size = 100) |
T + S + DIN + PO43- + SiO2 |
Polykrikos | IBk | Number of neighbors = 10 | T + S + PO43- |
Prorocentrum | KStar | Global blend = 50 | T + S + PO43- |
Pseudo-nitzschia | RF | Batch size = 50 Number of iterations = 100 |
T + S + DIN + PO43- + SiO2 |
Algae genus | N# presences | Precision | Sensitivity | Specificity | Kappa statistic | Discriminant power |
Alexandrium | 25 | 0.556 | 0.200 | 0.995 | 0.312 | 2.154 |
Amphidinium | 71 | 0.567 | 0.239 | 0.984 | 0.386 | 1.632 |
Cryptomonas | 168 | 0.878 | 0.774 | 0.974 | 0.723 | |
Dictyocha | 48 | 0.615 | 0.333 | 0.988 | 0.503 | 2.049 |
Dinophysis | 228 | 0.647 | 0.407 | 0.912 | 0.500 | |
Goniodoma | 21 | 0.667 | 0.095 | 0.999 | 0.324 | 2.565 |
Gonyaulax | 37 | 0.667 | 0.108 | 0.998 | 0.287 | 2.261 |
Gymnodinium | 279 | 0.814 | 0.659 | 0.931 | 0.655 | |
Gyrodinium | 177 | 0.682 | 0.353 | 0.970 | 0.509 | |
Karenia | 394 | 0.758 | 0.787 | 0.800 | 0.604 | |
Karlodinium | 133 | 0.915 | 0.489 | 0.992 | 0.595 | |
Lingulodinium | 23 | 0.833 | 0.217 | 0.999 | 0.291 | 3.100 |
Navicula | 165 | 0.550 | 0.200 | 0.963 | 0.480 | |
Peridinium | 301 | 0.642 | 0.405 | 0.884 | 0.518 | |
Phaeocystis | 71 | 0.867 | 0.549 | 0.993 | 0.633 | 2.840 |
Polykrikos | 38 | 0.667 | 0.158 | 0.996 | 0.349 | 2.119 |
Prorocentrum | 777 | 0.881 | 0.985 | 0.429 | 0.375 | |
Pseudo-nitzschia | 490 | 0.811 | 0.808 | 0.769 | 0.668 | |
Mean values | 0.723 | 0.431 | 0.921 | 0.469 | 2.340 |