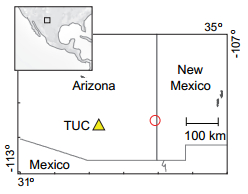
Nowadays, traditional machine learning methods for building predictive models of credit card customer churn are no longer sufficient for effective customer management. Additionally, interpreting these models has become essential. This study aims to balance the data using sampling techniques to forecast whether a customer will churn, combine machine learning methods to build a comprehensive customer churn prediction model, and select the model with the best performance. The optimal model is then interpreted using the Shapley Additive exPlanations (SHAP) values method to analyze the correlation between each independent variable and customer churn. Finally, the causal impacts of these variables on customer churn are explored using the R-learner causal inference method. The results show that the complete customer churn prediction model using Extreme Gradient Boosting (XGBoost) achieved significant performance, with accuracy, precision, recall, F1 score, and area under the curve (AUC) all reaching 97%. The SHAP values method and causal inference method demonstrate that several variables, such as the customer's total number of transactions, the total transaction amount, the total number of bank products, and the changes in both the amount and the number of transactions from the fourth quarter to the first quarter, have an impact on customer churn, providing a theoretical foundation for customer management.
Citation: Ying Li, Keyue Yan. Prediction of bank credit customers churn based on machine learning and interpretability analysis[J]. Data Science in Finance and Economics, 2025, 5(1): 19-34. doi: 10.3934/DSFE.2025002
[1] | Hongwei Zheng, Yujuan Tian . Exponential stability of time-delay systems with highly nonlinear impulses involving delays. Mathematical Modelling and Control, 2025, 5(1): 103-120. doi: 10.3934/mmc.2025008 |
[2] | Bangxin Jiang, Yijun Lou, Jianquan Lu . Input-to-state stability of delayed systems with bounded-delay impulses. Mathematical Modelling and Control, 2022, 2(2): 44-54. doi: 10.3934/mmc.2022006 |
[3] | Gani Stamov, Ekaterina Gospodinova, Ivanka Stamova . Practical exponential stability with respect to h−manifolds of discontinuous delayed Cohen–Grossberg neural networks with variable impulsive perturbations. Mathematical Modelling and Control, 2021, 1(1): 26-34. doi: 10.3934/mmc.2021003 |
[4] | Hongyu Ma, Dadong Tian, Mei Li, Chao Zhang . Reachable set estimation for 2-D switched nonlinear positive systems with impulsive effects and bounded disturbances described by the Roesser model. Mathematical Modelling and Control, 2024, 4(2): 152-162. doi: 10.3934/mmc.2024014 |
[5] | Yanchao He, Yuzhen Bai . Finite-time stability and applications of positive switched linear delayed impulsive systems. Mathematical Modelling and Control, 2024, 4(2): 178-194. doi: 10.3934/mmc.2024016 |
[6] | Yangtao Wang, Kelin Li . Exponential synchronization of fractional order fuzzy memristor neural networks with time-varying delays and impulses. Mathematical Modelling and Control, 2025, 5(2): 164-179. doi: 10.3934/mmc.2025012 |
[7] | Weisong Zhou, Kaihe Wang, Wei Zhu . Synchronization for discrete coupled fuzzy neural networks with uncertain information via observer-based impulsive control. Mathematical Modelling and Control, 2024, 4(1): 17-31. doi: 10.3934/mmc.2024003 |
[8] | Biresh Kumar Dakua, Bibhuti Bhusan Pati . A frequency domain-based loop shaping procedure for the parameter estimation of the fractional-order tilt integral derivative controller. Mathematical Modelling and Control, 2024, 4(4): 374-389. doi: 10.3934/mmc.2024030 |
[9] | Tengda Wei, Xiang Xie, Xiaodi Li . Persistence and periodicity of survival red blood cells model with time-varying delays and impulses. Mathematical Modelling and Control, 2021, 1(1): 12-25. doi: 10.3934/mmc.2021002 |
[10] | Yunhao Chu, Yansheng Liu . Approximate controllability for a class of fractional semilinear system with instantaneous and non-instantaneous impulses. Mathematical Modelling and Control, 2024, 4(3): 273-285. doi: 10.3934/mmc.2024022 |
Nowadays, traditional machine learning methods for building predictive models of credit card customer churn are no longer sufficient for effective customer management. Additionally, interpreting these models has become essential. This study aims to balance the data using sampling techniques to forecast whether a customer will churn, combine machine learning methods to build a comprehensive customer churn prediction model, and select the model with the best performance. The optimal model is then interpreted using the Shapley Additive exPlanations (SHAP) values method to analyze the correlation between each independent variable and customer churn. Finally, the causal impacts of these variables on customer churn are explored using the R-learner causal inference method. The results show that the complete customer churn prediction model using Extreme Gradient Boosting (XGBoost) achieved significant performance, with accuracy, precision, recall, F1 score, and area under the curve (AUC) all reaching 97%. The SHAP values method and causal inference method demonstrate that several variables, such as the customer's total number of transactions, the total transaction amount, the total number of bank products, and the changes in both the amount and the number of transactions from the fourth quarter to the first quarter, have an impact on customer churn, providing a theoretical foundation for customer management.
Earthquakes are monitored by using seismic stations that record digitally the motion of the ground and represent it as a function of time in seismograms. However, the seismic signals generated by some anthropogenic activities, including explosions, are frequently misinterpreted as natural earthquakes. Several methods to discriminate between earthquakes and explosions have been proposed. Commonly used methods compare in the time-domain the seismic phases recorded in seismograms. For example, in [1] the authors compared the phases Pg and Lg and found that in the tectonically stable eastern USA, the mean Pg/Lg ratios are 0.5 for earthquakes and 1.25 for explosions. In southern Russia, the Pg/Lg ratio for earthquakes was found to be about 1.3, and for explosions 3.2. However, current discriminating methods are not always successful. A data from the Powder River Basin in the western USA and the Altai-Sayan region in Russia suggest that a simple time-domain Lg/P amplitude ratio analysis does not separate signals originating from earthquakes and explosions consistently enough to provide a reliable discrimination [2]. Also, the S/P amplitude ratio does not reliably separate explosions from locally recorded natural seismicity in Southern California [3].
In 2014, a 5.2 magnitude earthquake struck in Arizona USA, triggering a large number of aftershocks. A few years before, a sequence of mining explosions were carried out in the same region, where subsequently the 5.2 magnitude earthquake and its aftershocks occurred. A nearby seismic station located about 160 km away, recorded all these events, thus providing an important data set of earthquake and explosion seismograms that makes it possible to apply and investigate different seismic discriminating techniques (see e.g. [7]). In this study, we use a data set originating from these explosions and the aftershocks of the 5.2 magnitude earthquake, in order to investigate the Dynamic Fourier Analysis (DFA) as a discriminating method.
The paper is organized as follows. In section 2, we present the background of the analyzed data. Section 3 is devoted to an introduction of the Dynamic Fourier Analysis and its application for discriminating signals originating from natural earthquakes and mining explosions. Results and a discussion on the suitability of the Dynamic Fourier Analysis technique are presented in section 4. Conclusions are given in section 5.
The earthquakes in this study represent a set of aftershocks of the June 26,2014, 5.2 magnitude intraplate earthquake. That is, this earthquake located between the sates of Arizona and New Mexico in the USA (Figure 1) occurred far from active tectonic boundaries. The explosions analyzed were carried out in a large surface copper mine, as part of quarry blasts activities. We selected earthquakes and explosions with similar local magnitude, in the range 3.0−3.3, and occurring close to each other, within a 10 km radius. The magnitude used in this study is measured by the Richter scale (ML) that assigns a number to quantify the size of an earthquake or an explosion. We collected seismograms of earthquakes and explosions recorded at seismic station TUC of the IU network. We also downloaded broadband seismograms from the Incorporated Research Institutions for Seismology Data Management Centers (IRIS DMC). The instrument response was removed and windows of data were selected using the Seismic Analysis Code (SAC) software. We analyzed the seismograms representing the displacement of the ground (in nm) in the vertical direction (Z-component). Information about the seismic station and the selected events is presented in Tables 1 and 2, respectively. Figure 2 shows examples of seismograms recorded at the TUC stations from one earthquake and one explosion.
Station | Network | Latitude | Longitude | Average distance to events | Average Azimuth |
TUC | IU | 32.3∘ | −110.8∘ | 161 km | 76∘ |
Events | Magnitude (ML) | Date | Time (UTC) | Latitude | Longitude |
Explosion 1 | 3.1 | 12/27/99 | 20:58:33 | 32.59∘ | −109.05∘ |
Explosion 2 | 3.0 | 01/24/00 | 22:10:15 | 32.67∘ | −109.08∘ |
Explosion 3 | 3.1 | 04/12/00 | 19:39:04 | 32.65∘ | −109.09∘ |
Explosion 4 | 3.2 | 03/19/01 | 21:29:02 | 32.64∘ | −109.15∘ |
Earthquake 1 | 3.3 | 06/29/14 | 15:40:10 | 32.59∘ | −109.12∘ |
Earthquake 2 | 3.2 | 07/11/14 | 6:15:55 | 32.64∘ | −109.11∘ |
Earthquake 3 | 3.0 | 07/12/14 | 7:12:53 | 32.58∘ | −109.08∘ |
Earthquake 4 | 3.0 | 07/17/14 | 9:12:27 | 32.53∘ | −109.06∘ |
A physical process can be described either in the time domain, by the values of some quantity h as a function of time t, e.g., h(t), or in the frequency domain, where the process is specified by giving its amplitude H (generally a complex number) as a function of frequency f, that is H(f), with −∞<f<∞. For many purposes it is useful to consider h(t) and H(f) as two different representations of the same function. It is possible to go back and forth between these two representations by means of the Fourier transform formulas:
h(t)=∫∞−∞H(f)e−2πiftdf |
H(f)=∫∞−∞h(t)e2πiftdt |
The Fourier transform H(f) converts waveform data in the time domain into the frequency domain. The Inverse Fourier transform h(t) converts the frequency domain components back into the original time-domain signal. A frequency-domain plot shows how much of the signal lies within each given frequency band over a range of frequencies.
In order to analyze a statistical time series, it must be assumed that the structure of the statistical or stochastic process generating the observations is essentially invariant through time. The conventional assumptions are summarized in the condition of stationarity as follows, if a time series xt is stationary, its second-order behavior remains the same, regardless of the time t. Based on the Spectral Representation Theorem [8,9], a stationary series is represented through the superposition of sines and cosines that oscillate at various frequencies. Therefore, a stationary time series can be represented with combination of sine and cosine series.
Next, we introduce definitions that will be used when applying the DFA.
A Discrete Fourier Transform (DFT) is used for discrete time series with a finite number of samples from a process that is continuous in time. When discontinuity occurs, the signal value abruptly jumps, yielding spectral leakage i.e. the input signal does not complete a whole number of cycles within the DFT time window. Consequently, it is required to multiply finite sampled time series by a windowing function, or a taper that decreases and approaches zero. Tapering is a process that reduces spectral leakage by multiplying the original time sequence by a function of time, such that the time series decreases and approaches zero, thus avoiding sudden discontinuities at the first and last point of the time series.
A stationary process Xt can be defined by using linear combinations of the form:
Xt=m∑j=1(Ajcos(2πλjt)+Bjsin(2πλjt)), | (3.1) |
where 0≤λ≤12 is a fixed constant and Aj, Bj for (j=1,m) are all uncorrelated random variables with a mean =0 and
var(Aj)=var(Bj)=σ2j. |
We assume ∑mj=1σ2j=σ2, so that the variance of the process Xt is σ2, where the spectral density f(λ) satisfies:
∫12−12f(λ)dλ=σ2. |
Then for large values of m the process given in (3.1) approximates the stationary process with spectral density f.
To estimate the spectral density, we define the estimators as weighted averages of periodogram values (I) for frequencies in the range (j−m)/n to (j+m)/n. In particular:
ˆf(j/n)=m∑k=−mWm(k)I(j+kn), |
where the Daniell kernel Wm(k) with parameter m is a centered moving average which creates a smoothed value at time t by averaging all values between times t−m and t+m (inclusive). We define:
Wm(k)=12m+1 for −m≤k≤m, ∑kWm(k)=1, and ∑kkWm(k)=0. |
The smoothing formula {ut} for a Daniell kernel with m=1 corresponds to the three weights (13,13,13) and is given by:
ˆut=13(ut−1+ut+ut+1). |
Applying the Daniell kernel again the smoothed values {ˆut} results in more extensive smoothing, averaging over a wider time interval
^^ut=ˆut−1+ˆut+ˆut+13=19ut−2+19ut−2+39ut+29ut+1+19ut+2. | (3.2) |
Consequently, applying the Daniell kernel transforms the spectral windows into a form of Gaussian probability density function; for more details see [10].
In this subsection we discuss a unit root methodology that is often used to test long memory behavior, the so-called Augmented Dickey Fuller Test (ADF).
The geophysical time series discussed in this study has a complicated dynamic structure that is not easily captured by traditional methods, such as a simple first-order linear autoregressive (AR(1)) models (see [4]). Therefore, there is a need for models that can capture such a unique dynamic structure. It is possible to augment the basic autoregressive unit root test to accommodate general autoregressive moving-average (ARMA(p,q)) models with unknown orders [5] and their test is referred to as the augmented Dickey Fuller (ADF) test (see [6]). The ADF is a very powerful test that can handle more complex models. It tests the null hypothesis that a time series yt is a unit root against the alternative that it is stationary, assuming that the data dynamics has an ARMA structure. The ADF test is based on estimating the test regression:
yt=βDt+ϕyt−1+p∑j=1ψjΔyt−j+ϵt, | (3.3) |
where Dt is a vector of deterministic term. The p lagged difference terms, Δyt−j, are used to approximate the ARMA structure of the errors, and the value of p is set so that the error ϵt is serially uncorrelated. An alternative formulation of (3.3) is
Δyt=βDt+πϕyt−1+p∑j=1ψjΔyt−j+ϵt, | (3.4) |
where π=ϕ−1. In practice, the test regression (3.4) is used since the ADF t-statistic is the usual t-statistic reported for testing the significance of the coefficient yt−1. An important practical issue for the implementation of the ADF test is the specification of the lag length p. If p is too small then the remaining serial correlation in the errors will bias the test. If p is too large, the power of the test will suffer. For the Augmented Dickey Fuller t-statistic test, small p-values suggest the data is stationary. Usually, small p-values are those that are smaller than a 5% level of significance.
We begin this subsection by using the ADF tests to test for a unit root in the seismic waves generated by the earthquake and explosion time series. To illustrate the ADF test procedure, we consider the seismograms of earthquake and the explosion time series as recorded by the seismic station TUC of the IU network. Figure 2 shows the seismograms recorded for earthquake 1 and explosion 1 from Table 2.
The ADF test was performed for all events (explosions and earthquakes) listed in Table 2. The summary statistics of the ADF results are shown in Table 3.
Events | Test statistic | p-value |
Earthquake 1 | -42.018 | 0.01 |
Earthquake 2 | -41.400 | 0.01 |
Earthquake 3 | -37.389 | 0.01 |
Earthquake 4 | -39.088 | 0.01 |
Explosion 1 | -40.298 | 0.01 |
Explosion 2 | -36.349 | 0.01 |
Explosion 3 | -40.830 | 0.01 |
Explosion 4 | -41.936 | 0.01 |
The ADF tests the following:
H0: There is a unit root for the time series, or
Ha: There is no unit root for the time series and the series is stationary.
Because the computed p-values are lower than the significance level α=0.05, we reject the null hypothesis H0 for all 8 events, and accept the alternative hypothesis Ha. Thus all studied events represent stationary time series.
As observed from the ADF test (Table 3) and the analyzed seismograms, the seismic signals are stationary. Thus the recorded seismograms represent a stationary process and therefore a dynamic stationary Fourier analysis is needed.
The purpose of the dynamic series analysis is to depict the spectral behavior of the seismic signals as they evolve over time. The Fourier Transform is used to extract this information by calculating a base 2 logarithm (log2); in that case it is required that the length or range of the time series to be evaluated contains a total number of data points equal to 2m, where m is a positive integer. If the total number of data points is not a power of two, it is also possible to express the Fourier transform of length n in terms of several shorter Discrete Fourier Transforms (DFT). The Fast Fourier Transforms (FFT), an algorithm for calculation of the DFT, is applied in the present analysis. We obtain the spectral series from a short segment of the data using the DFT. After characterization of the first segment, the spectral analysis is performed on a new segment that is shifted from the first segment. This process is repeated until the end of the data. We assume that xt represents the series of interest, where t=1,......,6016. The analyzed data segments are {xtk+1,......,xtk+256}, for tk=128k, where k=0,1,......,45. Therefore, the first segment analyzed is {x1,.....,x256}, the second segment analyzed is {x129,.......,x384}, and so on. Each segment of 256 observations was tapered using a cosine bell, and spectral estimation is performed using a repeated Daniell kernel with weights 19{1,2,3,2,1}. These coefficients are obtained with the R software by applying the kernel option.
One interpretation of the results of the time-frequency analysis presented above is to consider it based on local transforms of the data xt, given by:
dj,k=n−1/2n∑t=1xtψj,k(t), | (3.5) |
where
ψj,k(t)={(n/m)1/2hte−2πitj/mift∈[tk+1,tk+m],0ifotherwise. | (3.6) |
In the above formula ht is a taper and m is a fraction of n. In this study, the number of observations is n=6016, window size m=256, overlap tk=128k for k=0,1,....,45, and ht is a cosine bell taper over 256 points. In equations (1) and (2) above, j indexes the frequency ωj=j/m, for j=1,2.......[m/2] and k indexes the location, or time shift of the transform. We recall that the transforms are based on tapered cosines and sines that are zeroed out over various time intervals.
The DFA technique was applied to the recorded seismograms of the events listed in Table 2, and the results are presented in Figures 3 and 4 for the natural earthquakes and the explosions, respectively. The results are also presented numerically as percentages of the total power spectra for 50− seconds time windows, and 2− Hz frequency windows in Table 4.
Time (seconds) |
Frequency (Hz) |
Earthquakes | Explosions | ||||||
1 (%) |
2 (%) |
3 (%) |
4 (%) |
1 (%) |
2 (%) |
3 (%) |
4 (%) |
||
0-50 | 0-2 | 0.7 | 3.0 | 1.6 | 3.0 | 1.7 | 0.9 | 0.9 | 0.3 |
2-4 | 7.0 | 37.8 | 29.2 | 36.5 | 67.0 | 60.5 | 55.2 | 17.3 | |
4-6 | 5.2 | 7.8 | 13.0 | 7.9 | 5.8 | 3.7 | 7.5 | 4.8 | |
6-8 | 2.5 | 16.4 | 14.1 | 19.1 | 3.0 | 8.7 | 4.7 | 2.0 | |
8-10 | 3.5 | 6.0 | 11.4 | 8.7 | 1.0 | 1.3 | 0.7 | 1.7 |
The power spectra were evaluated by first estimating the periodograms [10], which indicate large and small periodic components of seismic signals in the respective frequencies. This estimation was performed using DFT with a 256 window size. In order to obtain a stable estimation, we computed the smoothed periodograms by using the Daniell kernel window (see subsection 3.2). Furthermore, we used the tapering process to avoid the spectral leakage of the power spectrum for earthquakes and explosions used in this study [11].
There is a considerable difference in the percentage of total power for the time series from earthquakes and explosions; see Figures 3, 4, 5 and Table 4. The results are shown in terms of estimated spectra for frequencies up to 10 Hz (the folding frequency is 20 Hz) for starting (time) tk=128k with k=0,1...........45. The spectral analyses were performed using R and Python.
The power spectrum of the earthquake S-waves starts from low frequencies and may remain strong for a long time window. On the other hand, the explosion power spectrum is strong at lower frequencies and gradually tapers off at high frequencies, especially at those greater than about 2 to 4 Hz; see Figures 3, 4, 5 and Table 4.
Specifically, for both earthquakes and explosions, P-waves and S-waves are identifiable from the power spectra (see Figures 3 and 4) as sequences of picks occurring at around 25 seconds for the P-waves and 50 seconds for the S-waves. For both type of events, the P-waves are with very-low amplitudes at all frequencies. There is no evidence that is possible to discriminate between earthquakes and explosions based on P-wave spectra. On the other hand, the S-waves exhibit a high-amplitude pick between 2 and 4 Hz for both type of events. More interestingly, the S-waves show characteristic peak frequencies (∼ 6-8 Hz) that is present for earthquakes but not for explosions. In order to further explore this characteristic peak in the S-wave frequency content, the values corresponding to the percentages of power in 2−Hz frequency windows were plotted in Figure 5. It can be noticed that for frequencies between 2 and 4 Hz explosions present higher values than earthquakes (Figure 5), and that tendency is reversed for frequencies between 6 and 8 Hz, where earthquakes present higher values than explosions.
Identifying the physical mechanisms that cause this notable difference in the frequency content of the S-waves of earthquakes and explosions is beyond the scope of this paper. However, we observe that the 6−8 Hz S-wave's peak detected for earthquakes but not for explosions, could be the a direct result from different source mechanisms. In general, it is still not well understood how explosions generate S-waves; while natural earthquakes simply shear slip on a fault plane. Explosions are modeled as pressure pulses on a sphere, which in theory should generate only P-waves [12]. The source depth could also play for this difference; while earthquakes occur deep underground, explosions are generally carried out at much shallower depths, which has an effect on how seismic waves propagate and interfere.
A simple, but very effective discriminant is the power spectrum spike. Figures 3, 4 and 5 illustrate that the typical earthquake series always has more than one spikes in the power spectrum, whereas the explosion series do not have consistently for more than one spike.
The algorithm implemented in this paper is different from "spectogram" and "fft" functions used with the SAC seismological program and MATLAB software. SAC computes a spectrogram using all of the data in memory. The spectra are calculated from a truncated autocorrelation function by using the maximum likelihood, maximum entropy, or Power Density Spectral statistical methodologies. The fft (in MATLAB) is a faster implementation of the DFT which reduces an n-point Fourier transform to about (n/2)log2n complex multiplications from n2 multiplications, providing an increase of speed for large data sets. However, the fft is not suitable for non periodic time series or non commensurate frequency (see subsection 3.1). The Dynamic Fourier methodology is a more reliable approach, since tapering is utilized to fix the spectral leakage by minimizing the effect of the discontinuity between the beginning and the end of the time series.
In this study we investigate the use of the Dynamic Fourier method as a tool to discriminate between seismic signals generated by natural earthquakes and mining explosions. The earthquakes analyzed correspond to a group of small-magnitude aftershocks generated by a 5.2 magnitude intraplate earthquake in Arizona. The mining explosions were selected such that they occurred very close to the earthquakes and were cataloged with similar magnitude as the earthquakes. We used seimograms from earthquakes and explosions recorded by a seismic station located about 160 km west from the epicenters of these events. Our results suggest that S-waves frequency content can be used as a discriminant tool. In particular, a high-amplitude peak in the S-waves spectra for frequencies ∼ 6-8 Hz is present for the earthquakes but not for the explosions. Further analysis is suggested in order to investigate how epicentral distance and the magnitude of the events affect these results.
Fourier transform is widely used in digital signal processing for frequency analysis of both stationary and non stationary signals. In the case of transient signals, the characteristics of the signals to be analyzed are strongly time-dependent. This imposes restrictions on the use of the Fourier transform, since the recorded earthquakes and mining explosions do not necessarily fulfill the stationary condition. To overcome this issue we used a short-time Fourier transform where the input signal is a Fourier transform analyzed in a short time window which shifts along the input signal length with some overlap. In addition to dynamic Fourier analysis as a method to overcome the restriction of stationarity, researchers have also sought wavelet methods to discriminate seismic events [7].
The earthquake data used in this study were provided by the Incorporated Research Institutions for Seismology Data Management Center (IRIS DMC).
The authors would like to thank the reviewers for the careful reading of the manuscript and the fruitful suggestions that helped to improve this work.
All authors declare no conflicts of interest in this paper.
[1] |
Akın P (2023) A new hybrid approach based on genetic algorithm and support vector machine methods for hyperparameter optimization in synthetic minority over-sampling technique (SMOTE). AIMS Math 8: 9400–9415. https://doi.org/10.3934/math.2023473 doi: 10.3934/math.2023473
![]() |
[2] |
Alam TM, Shaukat K, Hameed IA, et al. (2020) An Investigation of Credit Card Default Prediction in the Imbalanced Datasets. IEEE Access 8: 201173–201198. https://doi.org/10.1109/access.2020.3033784 doi: 10.1109/access.2020.3033784
![]() |
[3] |
Alfaiz NS, Fati SM (2022) Enhanced Credit Card Fraud Detection Model Using Machine Learning. Electronics 11: 662. https://doi.org/10.3390/electronics11040662 doi: 10.3390/electronics11040662
![]() |
[4] |
AL-Najjar D, Al-Rousan N, AL-Najjar H (2022) Machine learning to develop credit card customer churn prediction. J Theor Appl El Comm 17: 1529–1542. https://doi.org/10.3390/jtaer17040077 doi: 10.3390/jtaer17040077
![]() |
[5] | Bank Churners (2022) Kaggle. Available from: http://www.kaggle.com/competitions/bank-churners/overview. |
[6] |
Butaru F, Chen Q, Clark B, et al. (2016) Risk and Risk Management in the Credit Card Industry. J Bank Financ 72: 218–239. https://doi.org/10.1016/j.jbankfin.2016.07.015 doi: 10.1016/j.jbankfin.2016.07.015
![]() |
[7] |
Chang V, Hall K, Xu Qa, et al. (2024) Prediction of Customer Churn Behavior in the Telecommunication Industry Using Machine Learning Models. Algorithms 17: 231. https://doi.org/10.3390/a17060231 doi: 10.3390/a17060231
![]() |
[8] |
Chen K, Meng X (2020) Interpretation and Understanding in Machine Learning. J Comput Res Dev 57: 1971–1986. https://doi.org/10.7544/issn1000-1239.2020.20190456 doi: 10.7544/issn1000-1239.2020.20190456
![]() |
[9] |
de Lima Lemos RA, Silva TC, Tabak BM (2022) Propension to customer churn in a financial institution: a machine learning approach. Neural Comput Appl 34: 11751–11768. https://doi.org/10.1007/s00521-022-07067-x doi: 10.1007/s00521-022-07067-x
![]() |
[10] |
Dube L, Verster T (2023) Enhancing classification performance in imbalanced datasets: A comparative analysis of machine learning models. Data Sci Financ Econ 3: 354–379. https://doi.org/10.3934/dsfe.2023021 doi: 10.3934/dsfe.2023021
![]() |
[11] |
Erfanian S, Zhou Y, Razzaq A, et al. (2022) Predicting Bitcoin (BTC) Price in the Context of Economic Theories: A Machine Learning Approach. Entropy 24: 1487. https://doi.org/10.3390/e24101487 doi: 10.3390/e24101487
![]() |
[12] | Facure M (2023) Causal Inference in Python. O'Reilly Media, Inc. |
[13] |
Feuerriegel S, Frauen D, Melnychuk V, et al. (2024) Causal machine learning for predicting treatment outcomes. Nat Med 30: 958–968. https://doi.org/10.1038/s41591-024-02902-1 doi: 10.1038/s41591-024-02902-1
![]() |
[14] |
Gebreyesus Y, Dalton D, Nixon S, et al. (2023) Machine Learning for Data Center Optimizations: Feature Selection Using Shapley Additive exPlanation (SHAP). Future Internet 15: 88. https://doi.org/10.3390/fi15030088 doi: 10.3390/fi15030088
![]() |
[15] |
Gu Q, Song S, Zhang X, et al. (2023) Personal Credit Risk Assessment Based on Improved BS-Stacking. Oper Res Manage Sci 32: 137–144. https://doi.org/10.12005/orms.2023.0262 doi: 10.12005/orms.2023.0262
![]() |
[16] |
Guo K, Fan H (2024) Research on AdaFocal-XGBoost Integrated Credit Scoring Model Based on Unbalanced Data. Stat Appl 13: 2204–2214. https://doi.org/10.12677/sa.2024.136214 doi: 10.12677/sa.2024.136214
![]() |
[17] |
Janiesch C, Zschech P, Heinrich K (2021) Machine learning and deep learning. Electron Mark 31: 685–695. https://doi.org/10.1007/s12525-021-00475-2 doi: 10.1007/s12525-021-00475-2
![]() |
[18] |
Jiang T (2022) Mediating Effects and Moderating Effects in Causal Inference. China Ind Econ 5: 100–120. https://doi.org/10.19581/j.cnki.ciejournal.2022.05.005 doi: 10.19581/j.cnki.ciejournal.2022.05.005
![]() |
[19] |
Künzel SR, Sekhon JS, Bickel PJ, et al. (2019) Metalearners for estimating heterogeneous treatment effects using machine learning. P Natl Acad Sci 116: 4156–4165. https://doi.org/10.1073/pnas.1804597116 doi: 10.1073/pnas.1804597116
![]() |
[20] |
Lalwani P, Mishra MK, Chadha JS, et al. (2022) Customer churn prediction system: a machine learning approach. Computing 104: 271–294. https://doi.org/10.1007/s00607-021-00908-y doi: 10.1007/s00607-021-00908-y
![]() |
[21] |
Li J, Xiong R, Lan Y, et al. (2023) Overview of the Frontier Progress of Causal Machine Learning. J Comput Res Dev 60: 59–84. https://doi.org/10.7544/issn1000-1239.202110780 doi: 10.7544/issn1000-1239.202110780
![]() |
[22] |
Li Y, Yan K (2023) Prediction of Barrier Option Price Based on Antithetic Monte Carlo and Machine Learning Methods. Cloud Comput Data Sci 4: 77–86. https://doi.org/10.37256/ccds.4120232110 doi: 10.37256/ccds.4120232110
![]() |
[23] | Molak A (2023) Causal Inference and Discovery in Python. Packt Publishing Ltd. |
[24] |
Peng K, Peng Y, Li W (2023) Research on customer churn prediction and model interpretability analysis. PLOS ONE 18: e0289724. https://doi.org/10.1371/journal.pone.0289724 doi: 10.1371/journal.pone.0289724
![]() |
[25] |
Pudjihartono N, Fadason T, Kempa-Liehr AW, et al. (2022) A Review of Feature Selection Methods for Machine Learning-Based Disease Risk Prediction. Front Bioinform 2: 927312. https://doi.org/10.3389/fbinf.2022.927312 doi: 10.3389/fbinf.2022.927312
![]() |
[26] |
Siddiqui N, Haque MA, Khan SMS, et al. (2024) Different ML-based strategies for customer churn prediction in banking sector. J Data Inf Manage 6: 217–234. https://doi.org/10.1007/s42488-024-00126-z doi: 10.1007/s42488-024-00126-z
![]() |
[27] |
Wu M, Mao Z, Wang D (2024) Shapley Value and its Application. Math Model Appl 13: 110–119. https://doi.org/10.19943/j.2095-3070.jmmia.2024.01.13 doi: 10.19943/j.2095-3070.jmmia.2024.01.13
![]() |
[28] |
Yan K, Li Y (2024) Machine learning-based analysis of volatility quantitative investment strategies for American financial stocks. Quant Financ Econ 8: 364–386. https://doi.org/10.3934/QFE.2024014 doi: 10.3934/QFE.2024014
![]() |
[29] |
Yan K, Wang Y, Li Y (2023) Enhanced Bollinger Band Stock Quantitative Trading Strategy Based on Random Forest. Artif Intell Evol 4: 22–33. https://doi.org/10.37256/aie.4120231991 doi: 10.37256/aie.4120231991
![]() |
[30] | Zhang N, Zheng Y, Duan C (2024) Bank Customer Churn Prediction based on Random Forest Algorithm. In Proceedings of the 5th International Conference on Computer Information and Big Data Applications : 1031–1035. https://doi.org/10.1145/3671151.3671331 |
1. | Maria C. Mariani, Md Al Masum Bhuiyan, Osei K. Tweneboah, Hector Gonzalez-Huizar, Ionut Florescu, Volatility models applied to geophysics and high frequency financial market data, 2018, 503, 03784371, 304, 10.1016/j.physa.2018.02.167 | |
2. | Sachin Sawantt, Purva Golegaonkar, Prayas Gondane, Rushikesh Gole, Srushti Gole, Aniruddha Gondkar, Aditya Gorave, Rupali Deshpande, A.C. Sumathi, N. Yuvaraj, N.H. Ghazali, Earthquake prognosis using machine learning, 2023, 56, 2271-2097, 05017, 10.1051/itmconf/20235605017 | |
3. | Sangkyeum Kim, Kyunghyun Lee, Kwanho You, Seismic Discrimination between Earthquakes and Explosions Using Support Vector Machine, 2020, 20, 1424-8220, 1879, 10.3390/s20071879 |
Station | Network | Latitude | Longitude | Average distance to events | Average Azimuth |
TUC | IU | 32.3∘ | −110.8∘ | 161 km | 76∘ |
Events | Magnitude (ML) | Date | Time (UTC) | Latitude | Longitude |
Explosion 1 | 3.1 | 12/27/99 | 20:58:33 | 32.59∘ | −109.05∘ |
Explosion 2 | 3.0 | 01/24/00 | 22:10:15 | 32.67∘ | −109.08∘ |
Explosion 3 | 3.1 | 04/12/00 | 19:39:04 | 32.65∘ | −109.09∘ |
Explosion 4 | 3.2 | 03/19/01 | 21:29:02 | 32.64∘ | −109.15∘ |
Earthquake 1 | 3.3 | 06/29/14 | 15:40:10 | 32.59∘ | −109.12∘ |
Earthquake 2 | 3.2 | 07/11/14 | 6:15:55 | 32.64∘ | −109.11∘ |
Earthquake 3 | 3.0 | 07/12/14 | 7:12:53 | 32.58∘ | −109.08∘ |
Earthquake 4 | 3.0 | 07/17/14 | 9:12:27 | 32.53∘ | −109.06∘ |
Events | Test statistic | p-value |
Earthquake 1 | -42.018 | 0.01 |
Earthquake 2 | -41.400 | 0.01 |
Earthquake 3 | -37.389 | 0.01 |
Earthquake 4 | -39.088 | 0.01 |
Explosion 1 | -40.298 | 0.01 |
Explosion 2 | -36.349 | 0.01 |
Explosion 3 | -40.830 | 0.01 |
Explosion 4 | -41.936 | 0.01 |
Time (seconds) |
Frequency (Hz) |
Earthquakes | Explosions | ||||||
1 (%) |
2 (%) |
3 (%) |
4 (%) |
1 (%) |
2 (%) |
3 (%) |
4 (%) |
||
0-50 | 0-2 | 0.7 | 3.0 | 1.6 | 3.0 | 1.7 | 0.9 | 0.9 | 0.3 |
2-4 | 7.0 | 37.8 | 29.2 | 36.5 | 67.0 | 60.5 | 55.2 | 17.3 | |
4-6 | 5.2 | 7.8 | 13.0 | 7.9 | 5.8 | 3.7 | 7.5 | 4.8 | |
6-8 | 2.5 | 16.4 | 14.1 | 19.1 | 3.0 | 8.7 | 4.7 | 2.0 | |
8-10 | 3.5 | 6.0 | 11.4 | 8.7 | 1.0 | 1.3 | 0.7 | 1.7 |
Station | Network | Latitude | Longitude | Average distance to events | Average Azimuth |
TUC | IU | 32.3∘ | −110.8∘ | 161 km | 76∘ |
Events | Magnitude (ML) | Date | Time (UTC) | Latitude | Longitude |
Explosion 1 | 3.1 | 12/27/99 | 20:58:33 | 32.59∘ | −109.05∘ |
Explosion 2 | 3.0 | 01/24/00 | 22:10:15 | 32.67∘ | −109.08∘ |
Explosion 3 | 3.1 | 04/12/00 | 19:39:04 | 32.65∘ | −109.09∘ |
Explosion 4 | 3.2 | 03/19/01 | 21:29:02 | 32.64∘ | −109.15∘ |
Earthquake 1 | 3.3 | 06/29/14 | 15:40:10 | 32.59∘ | −109.12∘ |
Earthquake 2 | 3.2 | 07/11/14 | 6:15:55 | 32.64∘ | −109.11∘ |
Earthquake 3 | 3.0 | 07/12/14 | 7:12:53 | 32.58∘ | −109.08∘ |
Earthquake 4 | 3.0 | 07/17/14 | 9:12:27 | 32.53∘ | −109.06∘ |
Events | Test statistic | p-value |
Earthquake 1 | -42.018 | 0.01 |
Earthquake 2 | -41.400 | 0.01 |
Earthquake 3 | -37.389 | 0.01 |
Earthquake 4 | -39.088 | 0.01 |
Explosion 1 | -40.298 | 0.01 |
Explosion 2 | -36.349 | 0.01 |
Explosion 3 | -40.830 | 0.01 |
Explosion 4 | -41.936 | 0.01 |
Time (seconds) |
Frequency (Hz) |
Earthquakes | Explosions | ||||||
1 (%) |
2 (%) |
3 (%) |
4 (%) |
1 (%) |
2 (%) |
3 (%) |
4 (%) |
||
0-50 | 0-2 | 0.7 | 3.0 | 1.6 | 3.0 | 1.7 | 0.9 | 0.9 | 0.3 |
2-4 | 7.0 | 37.8 | 29.2 | 36.5 | 67.0 | 60.5 | 55.2 | 17.3 | |
4-6 | 5.2 | 7.8 | 13.0 | 7.9 | 5.8 | 3.7 | 7.5 | 4.8 | |
6-8 | 2.5 | 16.4 | 14.1 | 19.1 | 3.0 | 8.7 | 4.7 | 2.0 | |
8-10 | 3.5 | 6.0 | 11.4 | 8.7 | 1.0 | 1.3 | 0.7 | 1.7 |