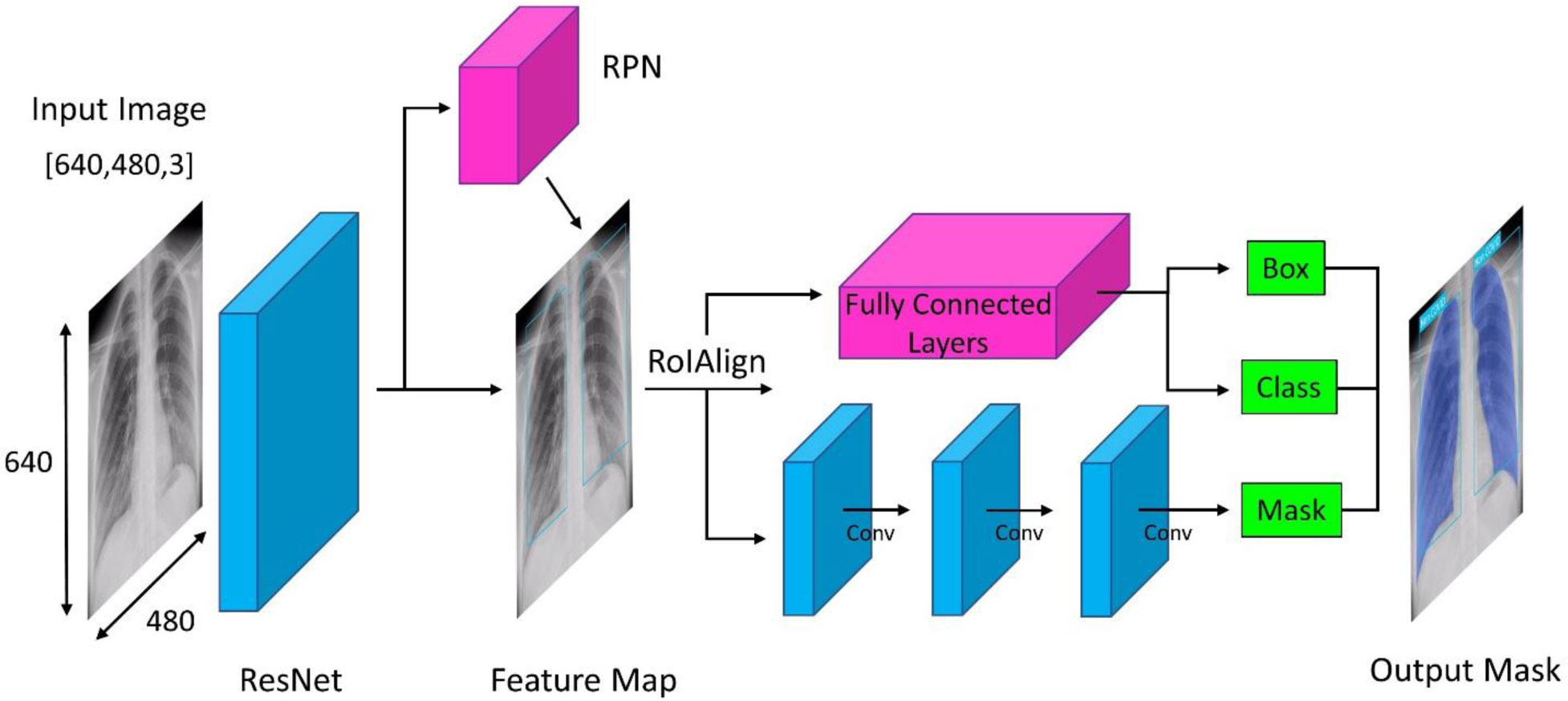
Citation: Carlos Estella, Antonio Baonza. Cell proliferation control by Notch signalling during imaginal discs development in Drosophila[J]. AIMS Genetics, 2015, 2(1): 70-96. doi: 10.3934/genet.2015.1.70
[1] | Swarnava Biswas, Debajit Sen, Dinesh Bhatia, Pranjal Phukan, Moumita Mukherjee . Chest X-Ray image and pathological data based artificial intelligence enabled dual diagnostic method for multi-stage classification of COVID-19 patients. AIMS Biophysics, 2021, 8(4): 346-371. doi: 10.3934/biophy.2021028 |
[2] | Soumyajit Podder, Abhishek Mallick, Sudipta Das, Kartik Sau, Arijit Roy . Accurate diagnosis of liver diseases through the application of deep convolutional neural network on biopsy images. AIMS Biophysics, 2023, 10(4): 453-481. doi: 10.3934/biophy.2023026 |
[3] | Dafina Xhako, Niko Hyka, Elda Spahiu, Suela Hoxhaj . Medical image analysis using deep learning algorithms (DLA). AIMS Biophysics, 2025, 12(2): 121-143. doi: 10.3934/biophy.2025008 |
[4] | Joshua Holcomb, Nicholas Spellmon, Yingxue Zhang, Maysaa Doughan, Chunying Li, Zhe Yang . Protein crystallization: Eluding the bottleneck of X-ray crystallography. AIMS Biophysics, 2017, 4(4): 557-575. doi: 10.3934/biophy.2017.4.557 |
[5] | Vittoria Raimondi, Alessandro Grinzato . A basic introduction to single particles cryo-electron microscopy. AIMS Biophysics, 2022, 9(1): 5-20. doi: 10.3934/biophy.2022002 |
[6] | Haliz Hussein, Hazhmat Ali, Zeki Mohamed, Majeed Mustafa, Khairi Abdullah, Asaad Alasady, Mayada Yalda . Thyroid function and hematological alterations in cardiac catheterization workers: a pre-post observational study on x-ray exposure. AIMS Biophysics, 2025, 12(1): 43-53. doi: 10.3934/biophy.2025004 |
[7] | Jaouhra Cherif, Anis Raddaoui, Ghofrane Ben Fraj, Asma Laabidi, Nada Souissi . Escherichia coli's response to low-dose ionizing radiation stress. AIMS Biophysics, 2024, 11(2): 130-141. doi: 10.3934/biophy.2024009 |
[8] | Mario Lefebvre . A Wiener process with jumps to model the logarithm of new epidemic cases. AIMS Biophysics, 2022, 9(3): 271-281. doi: 10.3934/biophy.2022023 |
[9] | David Kirchenbuechler, Yael Mutsafi, Ben Horowitz, Smadar Levin-Zaidman, Deborah Fass, Sharon G. Wolf, Michael Elbaum . Cryo-STEM Tomography of Intact Vitrified Fibroblasts. AIMS Biophysics, 2015, 2(3): 259-273. doi: 10.3934/biophy.2015.3.259 |
[10] | Adam Redzej, Gabriel Waksman, Elena V Orlova . Structural studies of T4S systems by electron microscopy. AIMS Biophysics, 2015, 2(2): 184-199. doi: 10.3934/biophy.2015.2.184 |
The novel coronavirus was detected for the first time in Wuhan, China in December 2019 and the epidemic has been spreading ever since. The number of people infected all over the world is 21,040,712 (11 March 2021) and the number of confirmed cases all over the world is 119,107,115 (11 March 2021). COVID-19 gets transmitted from respiratory droplets when someone with the virus coughs, sneezes or talks. Due to severity of the pandemic, it is indeed needed for quick and cost-effective detection of COVID-19.
Recently it is reported that artificial intelligence and machine learning have tremendous potential in health care sector [1]. The SARS-CoV-2 virus in the respiratory tract was usually diagnosed using the reverse transcription-polymerase chain reaction (RT-PCR) by taking naso-pharyngeal samples from the patient [2].
The RT-PCR has a lower potential for contamination and the severity of infection can also be estimated. The RT-PCR has a specificity of 46% and a recall of 87% [3]. Though the test usually takes a few hours, the process of collecting and processing the samples in bulk takes days before the results from a single sample are known from the report.
Although RT-PCR is widely used, it is less sensitive than computed tomography [4]. Chest X-ray is an imaging method to detect COVID-19 done in wards, exclusive to patients with suspected infections. Portable radiology equipments are available for suspected patients. Chest imaging plays an important role in early diagnosis and treatment of patients with COVID-19.
Chest radiography is a faster and comparatively inexpensive imaging method and the previous studies have demonstrated its role in predicting COVID-19 in patients infected with COVID-19 [5]–[7]. The sudden and massive increase in workloads, due to the spread of the COVID-19 pandemic has required the development of additional tools to help manage patients. The algorithm established here is also beneficial in cases where there is shortage of expertise in medical teams.
Mask R-CNN is an important AI-based scheme which has been used before in automatic nucleus segmentation [8], lung nodules detection and segmentation [9],[10], liver segmentation [11], automated blood cell counting [12] and multiorgan segmentation [13]. Face detection and segmentation [14], detection of oral disease [15], hand segmentation [16], segmenting the optic nerve [17], segmentation of early gastric cancer [18],[19] and detection and classification of breast tumors [20] is also performed using Mask R-CNN. However, the Mask R-CNN method for the detection of COVID-19 from chest X-ray images has not been explored to the best of our knowledge.
Imaging in COVID-19 is done by expert radiologists whose role is to screen the images through visual observation and report the findings. Chest X-ray is one of the most commonly and widely applied imaging modality. Healthcare centers and hospitals can be highly benefited in applying our proposed method in practice. It would ease the workflow and the test results can be obtained faster than the RT-PCR and so the spread of the disease can be readily controlled.
Detection methods also include the rapid antigen test which provides immediate results although they have lower sensitivity than RT-PCR. Various medical imaging techniques like X-rays, computed tomography (CT) scans, and magnetic resonance imaging (MRI) are used all over the world for diagnosis of diseases. Deep-learning based AI techniques are used to ensure that the diagnosis is accurate [21],[22]. In this study, we assess the performance of an artificial intelligence (AI) system for the detection of COVID-19.
The dataset of images utilized here in this study was collected from COVID-19 image data compiled by Cohen and et al. [23],[24]. The entire dataset of chest X-ray images is a publicly available collection of data and can be obtained from their GitHub repository for further use. The dataset was first made public in February 2020 and is continuously growing ever since. As of now, it contains a total of 542 frontal chest X-ray images from 262 people worldwide of which 408 are standard frontal PA/AP and 134 are AP Supine images [24]. The dataset also contains numerous clinical use cases and tools. Based on these chest X-ray images the deep learning model is evaluated.
The Mask R-CNN is a deep neural network aimed to solve instance segmentation problem in machine learning or computer vision. Mask R-CNN is of enormous importance in medical imaging analysis. Mask R-CNN model is commonly used for object detection and segmentation. Not only it puts a bounding box on the target, but also it creates a mask and classifies the boxes depending on the pixels inside it. It is an extension over the Faster R-CNN model.
The Mask R-CNN model consists of three primary components which are the backbone, the Region Proposal Network (RPN) and RoIAlign. The architecture of the proposed deep learning model is shown in Figure 1. Backbone is a Feature Pyramid Network style deep neural network which can extract multi-level image features. The ResNet forms the backbone of the Mask R-CNN model. The CNN used here is ResNet41, ResNet50, ResNet65 and ResNet101. The CNN ResNet50 consists of 48 convolution layers along with 1 MaxPool and 1 Average Pool layer. Further, it has 3.8 × 109 floating point operations. The RPN uses a sliding window to scan the input image and detects the infected regions in this study. The RoIAlign then examines the RoIs obtained from the RPN and extends the feature maps from the backbone at various locations. The RoIAlign is responsible for the formation of the precise segmentation masks on the images. The RoIPooling in Faster R-CNN is replaced by a more precise and accurate segmentation using the RoIAlign.
The entire model was buildup using the above deep learning mechanism. The various features of the model are described below. The model is capable of classifying objects into different classes, surround them with bounding boxes and create a mask for the detected objects. The multi-mask loss function for each case is given by:
where Lcls, Lbbx and Lmask are classification loss, bounding box regression loss and mask prediction loss respectively. To minimize the loss function the three components are composed in a specific manner. The classification loss is defined using the following equation:
The classification loss Lcls of each anchor is the log loss of whether the area is a lung is calculated from:
The bounding box regression loss is given by the following equation:
In the above mathematical expressions, i is the index of an anchor and pi indicates the prediction probability that the anchor is one of the lungs. Ground truth label is denoted by
where, the label value of a cell (i, j) of a region of m × m size is given by yij.
At first, the AI-based model was required to train on known results. The chest X-ray images with the best quality were selected for training. The images used were in jpg format. For training, testing and validation dataset, images of different age, gender and orientation were considered.
The system was trained on a pneumonia dataset as well as a COVID-19 dataset [23]. This dataset includes 931 images of which 534 were COVID infected according to the RT-PCR test, 134 were pneumonia and the rest were not specified. The validation set consisted of 150 images (75 per label, equally divided between PA and AP images) that were used to compute the performance during the training process [23],[24]. All of these images were obtained from an open source database as mentioned earlier and the patient anonymity was maintained.
The annotations were done using VGG Image Annotator software. VGG Image Annotator is an open-source image annotation tool used to indicate regions in an image and create text that defines them. These images were annotated by skilled pathologists.
The whole setup was implemented in Windows environment using NVIDIA GTX 1080 4 GB GPU on a system with 8 GB RAM and having Intel Core-i5 7th generation @2.20GHz processor. The entire algorithm was implemented using Python programming language.
The experiments on the deep learning model were performed to detect COVID-19 in chest X-ray images. The Mask R-CNN was trained for 100 epochs. The performance assessment of the methods is tabulated in Table 1. The Accuracy, Specificity, Precision and Recall were compared for the different methods as well as for the method used in this study [25]. The trained neural network was used to classify X-ray images. The parameters used to evaluate the performance are:
The TP, TN, FP and FN are the True Positive, True Negative, False Positive and False Negative respectively. The accuracy, specificity, recall, precision and F1-score for the proposed models are shown in Table 1. These metrics evaluate the performance of the deep learning model on the chest X-ray image dataset.
Method | AC | SP | PR | RC | F1 |
ResNet41 | 93.16% | 89.03% | 94.63% | 87.26% | 94.54% |
ResNet50 | 96.98% | 97.36% | 96.60% | 97.32% | 96.93% |
ResNet65 | 94.35% | 91.79% | 96.48% | 89.35% | 95.74% |
ResNet101 | 95.23% | 92.64% | 94.35% | 87.62% | 97.63% |
In order to evaluate the Mask R-CNN model, the 5-fold cross-validation was performed. The first fold was used to test the model while the rest of the dataset was used to train the model. Then the performance was noted from the perspective of a binary classification problem.
The 5-fold cross-validation was performed on all the models of which the ResNet50 proved to be superior than other models. The Confusion Matrix (CM) was computed for the task of binary classification between COVID-19 and Not COVID-19. The results of the CM for all the 5 folds are shown in Figure 2. The performance of the metrics for all 5 folds and their average is illustrated in Table 2. From the Table 2, it is observed that the ResNet50 model has obtained an average accuracy, specificity, precision, recall and F1-score of 96.98%, 97.36%, 96.60%, 97.32% and 96.93% respectively.
Fold Number | AC | SP | PR | RC | F1 |
Fold-1 | 97.55% | 97.10% | 98.0% | 97.12% | 97.55% |
Fold-2 | 98.0% | 99.0% | 97.0% | 98.97% | 97.97% |
Fold-3 | 98.70% | 97.40% | 100% | 97.46% | 98.71% |
Fold-4 | 93.75% | 96.50% | 91.0% | 96.92% | 93.57% |
Fold-5 | 96.90% | 96.80% | 97.0% | 96.80% | 96.89% |
Average | 96.98% | 97.36% | 96.60% | 97.32% | 96.93% |
Among the proposed models the ResNet50 demonstrates a promising amount of accuracy in COVID-19 detection on chest X-ray images. The evaluations indicate that the model is highly capable of accurate detection of the disease. Figure 3 (a) shows the chest X-ray of patients (b) shows the deep learning model prediction with mask.
The biomedical images used are of 640 × 480 for training the model. Our proposed method shows that the infected lungs are obtained with red masks and confined within the bounding boxes. Using our method, the lungs are correctly detected with no false alarms. To obtain better accuracy, experiments are performed extensively on superior quality images of the dataset.
The comparison of the different AI-based COVID-19 detection models is presented in Table 3. From Table 3, it can be seen that our method delivers an accuracy of 96.98% and specificity of 97.36% in COVID-19 detection, which is superior compared to the accuracy of 94.92% and specificity of 92% using SSD proposed by Saiz et al. [21]. Our model is also found to be better in comparison to DeTraC-ResNet18 proposed by Abbas et al. [22], COVIDX-Net proposed by Hemdan et al. [26], COVID-Net proposed by Wang et al. [27] and VGG-19 proposed by Ioannis et al. [28]. Note that VGG-19 has a slightly better specificity than our Mask R-CNN model. In addition to these, our model is also found to be better when it compared with the ResNet50 + SVM model proposed by Sethy and Behera [29] and the shallow Convolutional Neural Network (which contains four layers and lesser number of parameters for more efficient computation) proposed by Mukherjee et al. [30].
The proposed model can also be applied to distinguish COVID-19 pneumonia from other pulmonary diseases such as asthma and bronchitis. The proposed method can also be modified for detecting other abnormalities in the lungs. The advantage of this model is that it provides both bounding box and instance segmentation while the limitation is that larger datasets are required for accurate detection.
Model used | Number of cases taken to train model | AC | SP | Reference |
Mask R-CNN | 534 COVID-19 positive and 134 COVID-19 negative | 96.98% | 97.36% | This work |
DeTraC-ResNet18 | 116 COVID-19 positive and 80 COVID-19 negative | 95.12% | 91.87% | Abbas et al. [22] |
SSD | 100 COVID-19 positive and 887 COVID-19 negative | 94.92% | 92% | Saiz et al. [21] |
COVIDX-Net | 25 COVID-19 positive | 90% | - | Hemdan et al. [26] |
VGG-19 | 224 COVID-19 positive | 93.48% | 98.75% | Ioannis et al. [28] |
COVID-Net | 53 COVID-19 positive and 5526 COVID-19 negative | 92.4% | - | Wang and Wong [27] |
ResNet50 + SVM | 25 COVID-19 positive and 25 COVID-19 negative | 95.38% | 93.47% | Sethy and Behera [29] |
Shallow CNN | 130 COVID-19 positive and 51 COVID-19 negative | 96.92% | - | Mukherjee et al. [30] |
The AI-based method, Mask R-CNN for detection of COVID-19 using chest X-ray image as primary dataset is presented in detail. The method, Mask R-CNN is found to be superior in comparison to other AI-based methods for detecting COVID-19. The deep learning model proposed in this study is not only capable to detect but also able to classify COVID-19 infections from chest X-ray study. The method, Mask R-CNN presented here delivers specificity of 97.36% and accuracy of 96.98% and therefore would be a very effective tool in healthcare. Also, it is noticed that the Mask R-CNN method has potential applications in detecting chest related diseases.
[1] |
Hori K, Sen A, Artavanis-Tsakonas S (2013) Notch signaling at a glance. J Cell Sci 126: 2135-2140. doi: 10.1242/jcs.127308
![]() |
[2] |
ArtavanisTsakonas S, Rand MD, Lake RJ (1999) Notch signaling: Cell fate control and signal itegration in development. Science 284: 770-776. doi: 10.1126/science.284.5415.770
![]() |
[3] |
Kopan R, Ilagan MX (2009) The canonical Notch signaling pathway: unfolding the activation mchanism. Cell 137: 216-233. doi: 10.1016/j.cell.2009.03.045
![]() |
[4] |
Andersson ER, Sandberg R, Lendahl U (2011) Notch signaling: simplicity in design, versatility i function. Development 138: 3593-3612. doi: 10.1242/dev.063610
![]() |
[5] |
Louvi A, Artavanis-Tsakonas S (2012) Notch and disease: a growing field. Semin Cell Dev Biol 23: 473-480. doi: 10.1016/j.semcdb.2012.02.005
![]() |
[6] |
Ntziachristos P, Lim JS, Sage J, et al. (2014) From fly wings to targeted cancer therapies: a cntennial for notch signaling. Cancer Cell 25: 318-334. doi: 10.1016/j.ccr.2014.02.018
![]() |
[7] |
Radtke F, Raj K (2003) The role of Notch in tumorigenesis: oncogene or tumour suppressor? Nat Rv Cancer 3: 756-767. doi: 10.1038/nrc1186
![]() |
[8] |
Ranganathan P, Weaver KL, Capobianco AJ (2011) Notch signalling in solid tumours: a little bit o everything but not all the time. Nat Rev Cancer 11: 338-351. doi: 10.1038/nrc3035
![]() |
[9] | Morgan T (1917) The theory of the gene. Am Nat 51: 513-544. |
[10] |
Dominguez M (2014) Oncogenic programmes and Notch activity: an 'organized crime'. Semin Cell Dev Biol 28: 78-85. doi: 10.1016/j.semcdb.2014.04.012
![]() |
[11] |
Blaumueller CM, Qi H, Zagouras P, et al. (1997) Intracellular cleavage of Notch leads to a hterodimeric receptor on the plasma membrane. Cell 90: 281-291. doi: 10.1016/S0092-8674(00)80336-0
![]() |
[12] |
Bray SJ (2006) Notch signalling: a simple pathway becomes complex. Nat Rev Mol Cell Biol 7: 678-689. doi: 10.1038/nrm2009
![]() |
[13] |
Fortini ME (2009) Notch signaling: the core pathway and its posttranslational regulation. Dev Cll 16: 633-647. doi: 10.1016/j.devcel.2009.03.010
![]() |
[14] |
Bray S, Bernard F (2010) Notch targets and their regulation. Curr Top Dev Biol 92: 253-275. doi: 10.1016/S0070-2153(10)92008-5
![]() |
[15] |
Kandachar V, Roegiers F (2012) Endocytosis and control of Notch signaling. Curr Opin Cell Bol 24: 534-540. doi: 10.1016/j.ceb.2012.06.006
![]() |
[16] |
Baron M (2012) Endocytic routes to Notch activation. Semin Cell Dev Biol 23: 437-442. doi: 10.1016/j.semcdb.2012.01.008
![]() |
[17] |
Vaccari T, Bilder D (2009) At the crossroads of polarity, proliferation and apoptosis: the use of Dosophila to unravel the multifaceted role of endocytosis in tumor suppression. Mol Oncol 3: 354-365. doi: 10.1016/j.molonc.2009.05.005
![]() |
[18] |
Vaccari T, Lu H, Kanwar R, et al. (2008) Endosomal entry regulates Notch receptor activation in Dosophila melanogaster. J Cell Biol 180: 755-762. doi: 10.1083/jcb.200708127
![]() |
[19] |
Fortini ME, Bilder D (2009) Endocytic regulation of Notch signaling. Curr Opin Genet Dev 19: 323-328. doi: 10.1016/j.gde.2009.04.005
![]() |
[20] |
Lu H, Bilder D (2005) Endocytic control of epithelial polarity and proliferation in Drosophila. Nt Cell Biol 7: 1232-1239. doi: 10.1038/ncb1324
![]() |
[21] |
Morrison HA, Dionne H, Rusten TE, et al. (2008) Regulation of early endosomal entry by the Dosophila tumor suppressors Rabenosyn and Vps45. Mol Biol Cell 19: 4167-4176. doi: 10.1091/mbc.E08-07-0716
![]() |
[22] |
Herz HM, Chen Z, Scherr H, et al. (2006) vps25 mosaics display non-autonomous cell survival ad overgrowth, and autonomous apoptosis. Development 133: 1871-1880. doi: 10.1242/dev.02356
![]() |
[23] |
Herz HM, Bergmann A (2009) Genetic analysis of ESCRT function in Drosophila: a tumour mdel for human Tsg101. Biochem Soc Trans 37: 204-207. doi: 10.1042/BST0370204
![]() |
[24] |
Vaccari T, Rusten TE, Menut L, et al. (2009) Comparative analysis of ESCRT-I, ESCRT-II and ECRT-III function in Drosophila by efficient isolation of ESCRT mutants. J Cell Sci 122: 2413-2423. doi: 10.1242/jcs.046391
![]() |
[25] |
Thompson BJ, Mathieu J, Sung HH, et al. (2005) Tumor suppressor properties of the ESCRT-II cmplex component Vps25 in Drosophila. Dev Cell 9: 711-720. doi: 10.1016/j.devcel.2005.09.020
![]() |
[26] |
Moberg KH, Schelble S, Burdick SK, et al. (2005) Mutations in erupted, the Drosophila ortholog o mammalian tumor susceptibility gene 101, elicit non- cell-autonomous overgrowth. Dev Cell 9: 699-710. doi: 10.1016/j.devcel.2005.09.018
![]() |
[27] |
Vaccari T, Bilder D (2005) The Drosophila tumor suppressor vps25 prevents nonautonomous oerproliferation by regulating notch trafficking. Dev Cell 9:687-698. doi: 10.1016/j.devcel.2005.09.019
![]() |
[28] |
Parsons LM, Portela M, Grzeschik NA, et al. (2014) Lgl regulates Notch signaling via edocytosis, independently of the apical aPKC-Par6-Baz polarity complex. Curr Biol 24:2073-2084. doi: 10.1016/j.cub.2014.07.075
![]() |
[29] |
Haynie JL, Bryant PJ (1986) Development of the eye-antenna imaginal disc and morphogenesis o the adult head in Drosophila melanogaster. J Exp Zool 237: 293-308. doi: 10.1002/jez.1402370302
![]() |
[30] | Jurgens G, Hartenstein V (1993) The terminal region of the body pattern Cold Spring Harbor, N: Cold Spring Harbor Laboratory Press: 687-746. |
[31] | Wolff T, Ready DF (1993) Pattern formation in the Drosophila retina. In: Bate M, Mrtinez-Arias A, editors. The development of Drosophila melanogaster. Cold Spring Harbor, Nw York: Cold Spring Harbor Laboratory Press. |
[32] | Garcia-Bellido A (1975) Genetic control of wing disc development in Drosophila. In: Porter R, Rvers J, editors. Cell patterning. Boston: CIBA Symposium. pp. 161-183. |
[33] |
Baker WK (1978) A clonal analysis reveals early developmental restrictions in the Drosophila had. Dev Biol 62: 447-463. doi: 10.1016/0012-1606(78)90227-0
![]() |
[34] |
Blair SS (1995) Compartments and appendage development in Drosophila. BioEssays 17: 299-309. doi: 10.1002/bies.950170406
![]() |
[35] |
Irvine KD, Rauskolb C (2001) Boundaries in development: formation and function. Annu Rev Cell Dev Biol 17: 189-214. doi: 10.1146/annurev.cellbio.17.1.189
![]() |
[36] |
Kumar JP, Moses K (2001) EGF receptor and Notch signaling act upstream of Eyeless/Pax6 to cntrol eye specification. Cell 104: 687-697. doi: 10.1016/S0092-8674(01)00265-3
![]() |
[37] |
Kenyon KL, Ranade SS, Curtiss J, et al. (2003) Coordinating proliferation and tissue secification to promote regional identity in the Drosophila head. Dev Cell 5: 403-414. doi: 10.1016/S1534-5807(03)00243-0
![]() |
[38] | Singh A, Tare M, Puli OR, et al. (2011) A glimpse into dorso-ventral patterning of the Dosophila eye. Dev Dyn 241: 69-84. |
[39] |
Cho KO, Choi KW (1998) Fringe is essential for mirror symmetry and morphogenesis in the Dosophila eye. Nature 396: 272-276. doi: 10.1038/24394
![]() |
[40] |
Dominguez M, de Celis JF (1998) A dorsal/ventral boundary established by Notch controls gowth and polarity in the Drosophila eye. Nature 396: 276-278. doi: 10.1038/24402
![]() |
[41] | Papayannopoulos V, Tomlinson A, Panin VM, et al. (1998) Dorsal-ventral signaling in the Dosophila eye. Science 281: 2031-2034. |
[42] | Cavodeassi F, Diez Del Corral R, Campuzano S, et al. (1999) Compartments and organising bundaries in the Drosophila eye: the role of the homeodomain Iroquois proteins. Development 126: 4933-4942. |
[43] | Maurel-Zaffran C, Treisman JE (2000) pannier acts upstream of wingless to direct dorsal eye dsc development in Drosophila. Development 127: 1007-1016. |
[44] |
Haines N, Irvine KD (2003) Glycosylation regulates Notch signalling. Nat Rev Mol Cell Biol 4: 786-797. doi: 10.1038/nrm1228
![]() |
[45] |
Munro S, Freeman M (2000) The Notch signalling regulator Fringe acts in the Golgi apparatus ad requires the glycosyltransferase signature motif DxD. Curr Biol 10: 813-820. doi: 10.1016/S0960-9822(00)00578-9
![]() |
[46] |
Bruckner K, Perez L, Clausen H, et al. (2000) Glycosyltransferase activity of Fringe modulates Ntch-Delta interactions. Nature 406: 411-415. doi: 10.1038/35019075
![]() |
[47] |
Roignant JY, Treisman JE (2009) Pattern formation in the Drosophila eye disc. Int J Dev Biol 53: 795-804. doi: 10.1387/ijdb.072483jr
![]() |
[48] |
Chao JL, Tsai YC, Chiu SJ, et al. (2004) Localized Notch signal acts through eyg and upd to pomote global growth in Drosophila eye. Development 131: 3839-3847. doi: 10.1242/dev.01258
![]() |
[49] |
Dominguez M, Ferres-Marco D, Gutierrez-Avino FJ, et al. (2004) Growth and specification of te eye are controlled independently by Eyegone and Eyeless in Drosophila melanogaster. Nat Gnet 36: 31-39. doi: 10.1038/ng1281
![]() |
[50] | Hombria JC, Brown S (2002) The fertile field of Drosophila Jak/STAT signalling. Curr Biol 12: R69-575. |
[51] |
Tsai YC, Sun YH (2004) Long-range effect of upd, a ligand for Jak/STAT pathway, on cell cycle i Drosophila eye development. Genesis 39: 141-153. doi: 10.1002/gene.20035
![]() |
[52] |
Reynolds-Kenneally J, Mlodzik M (2005) Notch signaling controls proliferation through cll-autonomous and non-autonomous mechanisms in the Drosophila eye. Dev Biol 285: 38-48. doi: 10.1016/j.ydbio.2005.05.038
![]() |
[53] |
Gutierrez-Avino FJ, Ferres-Marco D, Dominguez M (2009) The position and function of the Ntch-mediated eye growth organizer: the roles of JAK/STAT and four-jointed. EMBO Rep 10: 1051-1058. doi: 10.1038/embor.2009.140
![]() |
[54] |
Ishikawa HO, Takeuchi H, Haltiwanger RS, et al. (2008) Four-jointed is a Golgi kinase that posphorylates a subset of cadherin domains. Science 321: 401-404. doi: 10.1126/science.1158159
![]() |
[55] |
Edgar BA (2006) From cell structure to transcription: Hippo forges a new path. Cell 124: 267-273. doi: 10.1016/j.cell.2006.01.005
![]() |
[56] |
Halder G, Johnson RL (2011) Hippo signaling: growth control and beyond. Development 138: 9-22. doi: 10.1242/dev.045500
![]() |
[57] | Da Ros VG, Gutierrez-Perez I, Ferres-Marco D, et al. (2013) Dampening the signals transduced trough hedgehog via microRNA miR-7 facilitates notch- induced tumourigenesis. PLoS Biol 11: e001554. |
[58] |
Yao S, Lum L, Beachy P (2006) The ihog cell-surface proteins bind Hedgehog and mediate pthway activation. Cell 125: 343-357. doi: 10.1016/j.cell.2006.02.040
![]() |
[59] |
McLellan JS, Yao S, Zheng X, et al. (2006) Structure of a heparin-dependent complex of Hdgehog and Ihog. Proc Natl Acad Sci U S A 103: 17208-17213. doi: 10.1073/pnas.0606738103
![]() |
[60] |
Camp D, Currie K, Labbe A, et al. (2010) Ihog and Boi are essential for Hedgehog signaling in Dosophila. Neural Dev 5: 28. doi: 10.1186/1749-8104-5-28
![]() |
[61] |
Yan D, Wu Y, Yang Y, et al. (2010) The cell-surface proteins Dally-like and Ihog differentially rgulate Hedgehog signaling strength and range during development. Development 137:2033-2044. doi: 10.1242/dev.045740
![]() |
[62] |
Heberlein U, Singh CM, Luk AY, et al. (1995) Growth and differentiation in the Drosophila eye coordinated by hedgehog. Nature 373: 709-711. doi: 10.1038/373709a0
![]() |
[63] |
Christiansen AE, Ding T, Bergmann A (2012) Ligand-independent activation of the Hedgehog pthway displays non-cell autonomous proliferation during eye development in Drosophila. Mch Dev 129: 98-108. doi: 10.1016/j.mod.2012.05.009
![]() |
[64] |
Jones S, Zhang X, Parsons DW, et al. (2008) Core signaling pathways in human pancreatic cncers revealed by global genomic analyses. Science 321: 1801-1806. doi: 10.1126/science.1164368
![]() |
[65] |
Treisman JE, Heberlein U (1998) Eye development in Drosophila: formation of the eye field and cntrol of differentiation. Curr Top Dev Biol 39: 119-158. doi: 10.1016/S0070-2153(08)60454-8
![]() |
[66] | Wolff T, Ready DF (1991) The beginning of pattern formation in the Drosophila compound eye: te morphogenetic furrow and the second mitotic wave. Development 113: 841-850. |
[67] |
Heberlein U, Moses K (1995) Mechanisms of Drosophila retinal morphogenesis: The virtues of bing progressive. Cell 81: 987-990. doi: 10.1016/S0092-8674(05)80003-0
![]() |
[68] | Horsfield J, Penton A, Secombe J, et al. (1998) decapentaplegic is required for arrest in G1 phase dring Drosophila eye development. Development 125: 5069-5078. |
[69] |
Penton A, Selleck SB, Hoffmann FM (1997) Regulation of cell cycle synchronization by dcapentaplegic during Drosophila eye development. Science 275: 203-206. doi: 10.1126/science.275.5297.203
![]() |
[70] |
Thomas BJ, Gunning DA, Cho J, et al. (1994) Cell cycle progression in the developing Dosophila eye: Roughex encodes a novel protein required for the establishment of G1. Cell 77:1003-1014. doi: 10.1016/0092-8674(94)90440-5
![]() |
[71] |
De Nooij JC, Hariharan IK (1995) Uncoupling cell fate determination from patterned cell dvision in the Drosophila eye. Science 270: 983-985. doi: 10.1126/science.270.5238.983
![]() |
[72] |
Baonza A, Freeman M (2005) Control of cell proliferation in the Drosophila eye by Notch sgnaling. Dev Cell 8: 529-539. doi: 10.1016/j.devcel.2005.01.019
![]() |
[73] |
Firth LC, Baker NE (2005) Extracellular signals responsible for spatially regulated proliferation i the differentiating Drosophila eye. Dev Cell 8: 541-551. doi: 10.1016/j.devcel.2005.01.017
![]() |
[74] |
Sukhanova MJ, Du W (2008) Control of cell cycle entry and exiting from the second mitotic wve in the Drosophila developing eye. BMC Dev Biol 8: 7. doi: 10.1186/1471-213X-8-7
![]() |
[75] |
Knoblich JA, Sauer K, Jones L, et al. (1994) Cyclin E controls S phase progression and its down rgulation during Drosophila embryogenesis is required for the arrest of cell proliferation. Cell77: 107-120. doi: 10.1016/0092-8674(94)90239-9
![]() |
[76] |
Lane ME, Sauer K, Wallace K, et al. (1996) Dacapo, a cyclin-dependent kinase inhibitor, stops cll proliferation during Drosophila development. Cell 87: 1225-1235. doi: 10.1016/S0092-8674(00)81818-8
![]() |
[77] |
Reis T, Edgar BA (2004) Negative regulation of dE2F1 by cyclin-dependent kinases controls cell ccle timing. Cell 117: 253-264. doi: 10.1016/S0092-8674(04)00247-8
![]() |
[78] | Ma C, Moses K (1995) wingless and patched are negative regulators of the morphogenetic frrow and can affect tissue polarity in the developing Drosophila compound eye. Development121: 2279-2289. |
[79] |
Heberlein U, Hariharan IK, Rubin GM (1993) Star is required for neuronal differentiation in the Dosophila retina and displays dosage-sensitive interactions with Ras1. Devl Biol 160: 51-63. doi: 10.1006/dbio.1993.1285
![]() |
[80] |
Domínguez M, Hafen E (1997) Hedgehog directly controls initiation and propagation of retinal dfferentiation in the Drosophila eye. Genes Dev 11: 3254-3264. doi: 10.1101/gad.11.23.3254
![]() |
[81] | Greenwood S, Struhl G (1999) Progression of the morphogenetic furrow in the Drosophila eye: te roles of Hedgehog, Decapentaplegic and the Raf pathway. Development 126: 5795-5808. |
[82] |
Masucci JD, Miltenberger RJ, Hoffmann FM (1990) Pattern-specific expression of the Drosophila decapentaplegic gene in inaginal disks is regulated by 3' cis- regulatory elements. Gnes Dev 4: 2011-2023. doi: 10.1101/gad.4.11.2011
![]() |
[83] |
Baker NE, Yu SY (2001) The EGF receptor defines domains of cell cycle progression and srvival to regulate cell number in the developing Drosophila eye. Cell 104: 699-708. doi: 10.1016/S0092-8674(01)00266-5
![]() |
[84] |
Duman-Scheel M, Weng L, Xin S, et al. (2002) Hedgehog regulates cell growth and proliferation b inducing Cyclin D and Cyclin E. Nature 417: 299-304. doi: 10.1038/417299a
![]() |
[85] |
Dominguez M, Brunner M, Hafen E, et al. (1996) Sending and receiving the hedgehog signal: Cntrol by the Drosophila Gli protein Cubitus interruptus. Science 272: 1621-1625. doi: 10.1126/science.272.5268.1621
![]() |
[86] | Domínguez M (1999) Dual role for Hedgehog in the regulation of the proneural gene atonal dring ommatidia development. Development 126: 2345-2353. |
[87] |
Jarman AP, Grell EH, Ackerman L, et al. (1994) atonal is the proneural gene for Drosophila potoreceptors. Nature 369: 398-400. doi: 10.1038/369398a0
![]() |
[88] |
Bossuyt W, De Geest N, Aerts S, et al. (2009) The atonal proneural transcription factor links dfferentiation and tumor formation in Drosophila. PLoS Biol 7: e40. doi: 10.1371/journal.pbio.1000040
![]() |
[89] | Lim J, Jafar-Nejad H, Hsu YC, et al. (2008) Novel function of the class I bHLH protein Dughterless in the negative regulation of proneural gene expression in the Drosophila eye. EBO Rep 9: 1128-1133. |
[90] |
Sukhanova MJ, Deb DK, Gordon GM, et al. (2007) Proneural basic helix-loop- helix proteins ad epidermal growth factor receptor signaling coordinately regulate cell type specification and ck inhibitor expression during development. Mol Cell Biol 27: 2987-2996. doi: 10.1128/MCB.01685-06
![]() |
[91] |
Singh A, Choi KW (2003) Initial state of the Drosophila eye before dorsoventral specification is euivalent to ventral. Development 130: 6351-6360. doi: 10.1242/dev.00864
![]() |
[92] | Chern JJ, Choi KW (2002) Lobe mediates Notch signaling to control domain- specific growth in te Drosophila eye disc. Development 129: 4005-4013. |
[93] | Sun X, Artavanis-Tsakonas S (1996) The intracellular deletions of Delta and Serrate define dminant negative forms of the Drosophila Notch ligands. Development 122: 2465-2474. |
[94] |
Furriols M, Bray S (2001) A model Notch response element detects Suppressor of Hirless-dependent molecular switch. Curr Biol 11: 60-64. doi: 10.1016/S0960-9822(00)00044-0
![]() |
[95] | de Celis JF, Tyler DM, de Celis J, et al. (1998) Notch signalling mediates segmentation of the Dosophila leg. Development 125: 4617-4626. |
[96] |
Rafel N, Milan M (2008) Notch signalling coordinates tissue growth and wing fate specification i Drosophila. Development 135: 3995-4001. doi: 10.1242/dev.027789
![]() |
[97] |
Diaz-Benjumea F, Cohen SM (1993) Interactions between dorsal and ventral cells in the iaginal disc directs wing development in Drosophila. Cell 75: 741-752. doi: 10.1016/0092-8674(93)90494-B
![]() |
[98] | Tabata T, Schwartz C, Gustavson E, et al. (1995) Creating a Drosophila wing de novo, the role o engrailed and the compartment hypotesis. Development 121: 3359-3369. |
[99] | Zecca M, Basler K, Struhl G (1995) Sequential organizing activities of engrailed, hedgehog and dcapentaplegic in the Drosophila wing. Development 121: 2265-2278. |
[100] |
Lawrence PA, Morata G (1976) Compartments in the wing of Drosophila: a study of the egrailed gene. Dev Biol 50: 321-337. doi: 10.1016/0012-1606(76)90155-X
![]() |
[101] | Blair SS, Brower DL, Thomas JB, et al. (1994) The role of apterous in the control of drsoventral compartmentalization and PS integrin gene expression in the developing wing of Dosophila. Development 120: 1805-1815. |
[102] | Diaz-Benjumea FJ, Cohen SM (1995) Serrate signals through Notch to establish a Wingless-dependent organizer at the dorsal/ventral compartment boundary of the Drosophila wng. Development 121: 4215-4225. |
[103] |
Milan M, Cohen SM (2000) Subdividing cell populations in the developing limbs of Drosophila: d wing veins and leg segments define units of growth control? Dev Biol 217: 1-9. doi: 10.1006/dbio.1999.9493
![]() |
[104] | de Celis JF, García-Bellido A, Bray SJ (1996a) Activation and function of Notch at the drso-ventral boundary of the wing imaginal disc. Development 122: 359-369. |
[105] |
Doherty D, Feger G, Younger-Shepherd S, et al. (1996) Delta is a ventral to dorsal signal cmplementary to Serrate, another Notch ligand, in Drosophila wing formation. Genes Dev 10:421-434. doi: 10.1101/gad.10.4.421
![]() |
[106] | Fleming RJ, Gu Y, Hukriede NA (1997) Serrate-mediated activaction of Notch is specifically bocked by the product of the gene fringe in the dorsal compartment of the Drosophila wing iaginal disc. Development 124: 2973-2981. |
[107] |
Panin VM, Papayannopoulos V, Wilson R, et al. (1997) fringe modulates Notch-ligand iteractions. Nature 387: 908-912. doi: 10.1038/43191
![]() |
[108] |
Kim J, Irvine KD, Carroll SB (1995) Cell recognition, signal induction, and symmetrical gene ativation at the dorsal-ventral boundary of the developing Drosophila wing. Cell 82: 795-802. doi: 10.1016/0092-8674(95)90476-X
![]() |
[109] |
Couso JP, Knust E, Martínez Arias A (1995) Serrate and wingless cooperate to induce vestigial gne expression and wing formation in Drosophila. Curr Biol 5: 1437-1448. doi: 10.1016/S0960-9822(95)00281-8
![]() |
[110] |
Irvine KD, Wieschaus E (1994) fringe, a boundary-specific signalling molecule, mediates iteractions between dorsal and ventral cells during Drosophila wing development. Cell 79:595-606. doi: 10.1016/0092-8674(94)90545-2
![]() |
[111] |
Kim J, Sebring A, Esch JJ, et al. (1996) Integration of positional signals and regulation of wing frmation and identity by Drosophila vestigial gene. Nature 382: 133-138. doi: 10.1038/382133a0
![]() |
[112] |
Baena-Lopez LA, Garcia-Bellido A (2006) Control of growth and positional information by the gaded vestigial expression pattern in the wing of Drosophila melanogaster. Proc Natl Acad Sci US A 103: 13734-13739. doi: 10.1073/pnas.0606092103
![]() |
[113] |
Baena-Lopez LA, Nojima H, Vincent JP (2012) Integration of morphogen signalling within the gowth regulatory network. Curr Opin Cell Biol 24: 166-172. doi: 10.1016/j.ceb.2011.12.010
![]() |
[114] | Neumann CJ, Cohen SM (1996) A hierarchy of cross-regulation involving Notch, wingless, vstigial and cut organizes the dorsal/ventral axis of the Drosophila wing. Development 122:3477-3485. |
[115] |
Zecca M, Struhl G (2007) Recruitment of cells into the Drosophila wing primordium by a fed-forward circuit of vestigial autoregulation. Development 134: 3001-3010. doi: 10.1242/dev.006411
![]() |
[116] | Klein T, Martinez-Arias A (1999) The Vestigial gene product provides a molecular context for te interpretation of signals during the development of the wing in Drosophila. Development 126:913-925. |
[117] | Klein T, Martinez Arias A (1998) Different spatial and temporal interactions between Notch, wngless, and vestigial specify proximal and distal pattern elements of the wing in Drosophila. Dv Biol 194: 196-212. |
[118] |
Williams JA, Bell JB, Carroll SB (1991) Control of Drosophila wing and haltere development by te nuclear vestigial gene product. Genes Dev 5: 2481-2495. doi: 10.1101/gad.5.12b.2481
![]() |
[119] |
Baonza A, Garcia-Bellido A (2000) Notch signaling directly controls cell proliferation in the Dosophila wing disc. Proc Natl Acad Sci U S A 97: 2609-2614. doi: 10.1073/pnas.040576497
![]() |
[120] | Go JM, Eastman DS, Artavanis-Tsakonas S (1998) Cell proliferation control by Notch signaling in Drosophila development. Development 125: 2031-2040. |
[121] |
Giraldez AJ, Cohen SM (2003) Wingless and Notch signaling provide cell survival cues and cntrol cell proliferation during wing development. Development 130: 6533-6543. doi: 10.1242/dev.00904
![]() |
[122] |
de Celis JF, Garcia-Bellido A (1994) Roles of the Notch gene in Drosophila wing morphogenesis. Mch Dev 46: 109-122. doi: 10.1016/0925-4773(94)90080-9
![]() |
[123] |
Baonza A, Roch F, Martin-Blanco E (2000) DER signaling restricts the boundaries of the wing feld during Drosophila development. Proc Natl Acad Sci U S A 97: 7331-7335. doi: 10.1073/pnas.97.13.7331
![]() |
[124] | Zecca M, Struhl G (2002) Subdivision of the Drosophila wing imaginal disc by EGFR-mediated sgnaling. Development 129: 1357-1368. |
[125] |
Wang SH, Simcox A, Campbell G (2000) Dual role for Drosophila epidermal growth factor rceptor signaling in early wing disc development. Genes Dev 14: 2271-2276. doi: 10.1101/gad.827000
![]() |
[126] |
Simcox AA, Grumbling G, Schnepp B, et al. (1996) Molecular, phenotypic, and expression aalysis of vein, a gene required for growth of the Drosophila wing disc. Devl Biol 177:475-489. doi: 10.1006/dbio.1996.0179
![]() |
[127] |
Paul L, Wang SH, Manivannan SN, et al. (2013) Dpp-induced Egfr signaling triggers pstembryonic wing development in Drosophila. Proc Natl Acad Sci U S A 110: 5058-5063. doi: 10.1073/pnas.1217538110
![]() |
[128] |
O'Brochta D, Bryant PJ (1985) A zone of non-proliferating cells at a lineage restriction boundary i Drosophila. Nature 313: 138-141. doi: 10.1038/313138a0
![]() |
[129] |
Johnston LA, Edgar BA (1998) Wingless and Notch regulate cell-cycle arrest in the developing Dosophila wing. Nature 394: 82-84. doi: 10.1038/27925
![]() |
[130] | Buceta J, Herranz H, Canela-Xandri O, et al. (2007) Robustness and stability of the gene rgulatory network involved in DV boundary formation in the Drosophila wing. PLoS One 2: e02. |
[131] |
Herranz H, Perez L, Martin FA, et al. (2008) A Wingless and Notch double- repression mchanism regulates G1-S transition in the Drosophila wing. Embo J 27: 1633-1645. doi: 10.1038/emboj.2008.84
![]() |
[132] |
Andrade-Zapata I, Baonza A (2014) The bHLH factors extramacrochaetae and daughterless cntrol cell cycle in Drosophila imaginal discs through the transcriptional regulation of the Cc25 phosphatase string. PLoS Genet 10: e1004233. doi: 10.1371/journal.pgen.1004233
![]() |
[133] | de Celis JF, de Celis J, Ligoxiars P, et al. (1996) Functional relationships between Notch and bLH genes of the E(spl) complex: the E(spl) genes mediate only a subset of Notch activies dring imaginal development. Development 122: 2719-2728. |
[134] |
Massari ME, Murre C (2000) Helix-loop-helix proteins: regulators of transcription in eucaryotic oganisms. Mol Cell Biol 20: 429-440. doi: 10.1128/MCB.20.2.429-440.2000
![]() |
[135] |
San Juan BP, Andrade-Zapata I, Baonza A (2012) The bHLH factors Dpn and members of the Espl) complex mediate the function of Notch signaling regulating cell proliferation during wing dsc development. Biol Open 1: 667-676. doi: 10.1242/bio.20121172
![]() |
[136] | Djiane A, Krejci A, Bernard F, et al. (2013) Dissecting the mechanisms of Notch induced hperplasia. Embo J 32: 60-71. |
[137] | Rulifson EJ, Blair SS (1995) Notch regulates wingless expression and is not required for the rception of paracrine wingless signals during wing margin development in Drosophila. Dvelopment 121: 2813-2824. |
[138] |
Klein T, Martinez-Arias A (1997) An intrisic dominant negative activity of Serrate that is mdulated during wing development in Drosophila. Dev Biol 189: 123-134. doi: 10.1006/dbio.1997.8564
![]() |
[139] | Alexandre C, Baena-Lopez A, Vincent JP (2014) Patterning and growth control by membrane-tethered Wingless. Nature 505: 180-185. |
[140] |
Johnston LA, Sanders AL (2003) Wingless promotes cell survival but constrains growth during Dosophila wing development. Nat Cell Biol 5: 827-833. doi: 10.1038/ncb1041
![]() |
[141] |
Halder G, Polaczyk P, Kraus ME, et al. (1998) The Vestigial and Scalloped proteins act together t directly regulate wing-specific gene expression in Drosophila. Genes Dev 12: 3900-3909. doi: 10.1101/gad.12.24.3900
![]() |
[142] |
Simmonds AJ, Liu X, Soanes KH, et al. (1998) Molecular interactions between Vestigial and Salloped promote wing formation in Drosophila. Genes Dev 12: 3815-3820. doi: 10.1101/gad.12.24.3815
![]() |
[143] |
Zhao B, Tumaneng K, Guan KL (2011) The Hippo pathway in organ size control, tissue rgeneration and stem cell self-renewal. Nat Cell Biol 13: 877-883. doi: 10.1038/ncb2303
![]() |
[144] |
Djiane A, Zaessinger S, Babaoglan AB, et al. (2014) Notch inhibits Yorkie activity in Drosophila wng discs. PLoS One 9: e106211. doi: 10.1371/journal.pone.0106211
![]() |
[145] |
Graves HK, Woodfield SE, Yang CC, et al. (2012) Notch signaling activates Yorkie non-cell atonomously in Drosophila. PLoS One 7: e37615. doi: 10.1371/journal.pone.0037615
![]() |
[146] |
Bach EA, Ekas LA, Ayala-Camargo A, et al. (2007) GFP reporters detect the activation of the Dosophila JAK/STAT pathway in vivo. Gene Expr Patterns 7: 323-331. doi: 10.1016/j.modgep.2006.08.003
![]() |
[147] |
Rauskolb C, Irvine KD (1999) Notch-mediated segmentation and growth control of the Dosophila leg. Dev Biol 210: 339-350. doi: 10.1006/dbio.1999.9273
![]() |
[148] | Bishop SA, Klein T, Arias AM, et al. (1999) Composite signalling from Serrate and Delta etablishes leg segments in Drosophila through Notch. Development 126: 2993-3003. |
[149] |
Estella C, Voutev R, Mann RS (2012) A dynamic network of morphogens and transcription fctors patterns the fly leg. Curr Top Dev Biol 98: 173-198. doi: 10.1016/B978-0-12-386499-4.00007-0
![]() |
[150] | Kerber B, Monge I, Mueller M, et al. (2001) The AP-2 transcription factor is required for joint frmation and cell survival in Drosophila leg development. Development 128: 1231-1238. |
[151] |
Saj A, Arziman Z, Stempfle D, et al. (2010) A combined ex vivo and in vivo RNAi screen for ntch regulators in Drosophila reveals an extensive notch interaction network. Dev Cell 18:862-876. doi: 10.1016/j.devcel.2010.03.013
![]() |
[152] |
Ferres-Marco D, Gutierrez-Garcia I, Vallejo DM, et al. (2006) Epigenetic silencers and Notch cllaborate to promote malignant tumours by Rb silencing. Nature 439: 430-436. doi: 10.1038/nature04376
![]() |
[153] |
Brumby AM, Richardson HE (2003) scribble mutants cooperate with oncogenic Ras or Notch to cuse neoplastic overgrowth in Drosophila. Embo J 22: 5769-5779. doi: 10.1093/emboj/cdg548
![]() |
[154] |
Brumby AM, Richardson HE (2005) Using Drosophila melanogaster to map human cancer pthways. Nat Rev Cancer 5: 626-639. doi: 10.1038/nrc1671
![]() |
[155] |
Pagliarini RA, Xu T (2003) A genetic screen in Drosophila for metastatic behavior. Science 302:1227-1231. doi: 10.1126/science.1088474
![]() |
[156] |
Miles WO, Dyson NJ, Walker JA (2011) Modeling tumor invasion and etastasis in Drosophila. Ds Model Mech 4: 753-761. doi: 10.1242/dmm.006908
![]() |
[157] |
Elsum I, Yates L, Humbert PO, et al. (2012) The Scribble-Dlg-Lgl polarity module in dvelopment and cancer: from flies to man. Essays Biochem 53: 141-168. doi: 10.1042/bse0530141
![]() |
[158] |
Leong GR, Goulding KR, Amin N, et al. (2009) Scribble mutants promote aPKC and JK-dependent epithelial neoplasia independently of Crumbs. BMC Biol 7: 62. doi: 10.1186/1741-7007-7-62
![]() |
[159] |
Zeitler J, Hsu CP, Dionne H, et al. (2004) Domains controlling cell polarity and proliferation in te Drosophila tumor suppressor Scribble. J Cell Biol 167: 1137-1146. doi: 10.1083/jcb.200407158
![]() |
[160] |
Parsons LM, Grzeschik NA, Allott ML, et al. (2010) Lgl/aPKC and Crb regulate the Slvador/Warts/Hippo pathway. Fly (Austin) 4: 288-293. doi: 10.4161/fly.4.4.13116
![]() |
[161] |
Robinson BS, Huang J, Hong Y, et al. (2010) Crumbs regulates Salvador/Warts/Hippo signaling i Drosophila via the FERM-domain protein Expanded. Curr Biol 20: 582-590. doi: 10.1016/j.cub.2010.03.019
![]() |
[162] |
Grzeschik NA, Amin N, Secombe J, et al. (2007) Abnormalities in cell proliferation and aico-basal cell polarity are separable in Drosophila lgl mutant clones in the developing eye. Dev Bol 311: 106-123. doi: 10.1016/j.ydbio.2007.08.025
![]() |
[163] |
Cohen B, Shimizu M, Izrailit J, et al. (2010) Cyclin D1 is a direct target of JAG1-mediated Ntch signaling in breast cancer. Breast Cancer Res Treat 123: 113-124. doi: 10.1007/s10549-009-0621-9
![]() |
[164] |
Ling H, Sylvestre JR, Jolicoeur P (2010) Notch1-induced mammary tumor development is cyclin D-dependent and correlates with expansion of pre- malignant multipotent duct-limited pogenitors. Oncogene 29: 4543-4554. doi: 10.1038/onc.2010.186
![]() |
[165] |
Sharma VM, Calvo JA, Draheim KM, et al. (2006) Notch1 contributes to mouse T-cell leukemia b directly inducing the expression of c-myc. Mol Cell Biol 26: 8022-8031. doi: 10.1128/MCB.01091-06
![]() |
[166] |
Weng AP, Millholland JM, Yashiro-Ohtani Y, et al. (2006) c-Myc is an important direct target of Ntch1 in T-cell acute lymphoblastic leukemia/lymphoma. Genes Dev 20: 2096-2109. doi: 10.1101/gad.1450406
![]() |
[167] |
Klinakis A, Szabolcs M, Politi K, et al. (2006) Myc is a Notch1 transcriptional target and a rquisite for Notch1-induced mammary tumorigenesis in mice. Proc Natl Acad Sci U S A 103:9262-9267. doi: 10.1073/pnas.0603371103
![]() |
[168] |
Dohda T, Maljukova A, Liu L, et al. (2007) Notch signaling induces SKP2 expression and pomotes reduction of p27Kip1 in T-cell acute lymphoblastic leukemia cell lines. Exp Cell Res 313: 3141-3152. doi: 10.1016/j.yexcr.2007.04.027
![]() |
1. | Shivangi Gupta, Sunanda Gupta, 2023, Chapter 16, 978-981-19-5291-3, 173, 10.1007/978-981-19-5292-0_16 | |
2. | Padma Nyoman Crisnapati, Dechrit Maneetham, RIFIS: A Novel Rice Field Sidewalk Detection Dataset for Walk-Behind Hand Tractor, 2022, 7, 2306-5729, 135, 10.3390/data7100135 | |
3. | F. M. Javed Mehedi Shamrat, Sami Azam, Asif Karim, Rakibul Islam, Zarrin Tasnim, Pronab Ghosh, Friso De Boer, LungNet22: A Fine-Tuned Model for Multiclass Classification and Prediction of Lung Disease Using X-ray Images, 2022, 12, 2075-4426, 680, 10.3390/jpm12050680 | |
4. | Durjoy Majumder, Development of a fast fourier transform-based analytical method for COVID-19 diagnosis from chest X-ray images using GNU octave, 2022, 47, 0971-6203, 279, 10.4103/jmp.jmp_26_22 | |
5. | Megha Agarwal, Vinti Gupta, Abhinav Goel, Aditya Raghav, Neeraj Dhiman, 2022, 2767, 0094-243X, 030001, 10.1063/5.0104372 | |
6. | Eugen Antal, Pavol Marák, Automated Transcription of Historical Encrypted Manuscripts, 2022, 82, 1338-9750, 65, 10.2478/tmmp-2022-0019 | |
7. | Anandbabu Gopatoti, P. Vijayalakshmi, Optimized chest X-ray image semantic segmentation networks for COVID-19 early detection, 2022, 30, 08953996, 491, 10.3233/XST-211113 | |
8. | Suganya D., Kalpana R., Prognosticating various acute covid lung disorders from COVID-19 patient using chest CT Images, 2023, 119, 09521976, 105820, 10.1016/j.engappai.2023.105820 | |
9. | Anusua Basu, Pradip Senapati, Mainak Deb, Rebika Rai, Krishna Gopal Dhal, A survey on recent trends in deep learning for nucleus segmentation from histopathology images, 2023, 1868-6478, 10.1007/s12530-023-09491-3 | |
10. | M. Emin Sahin, Hasan Ulutas, Esra Yuce, Mustafa Fatih Erkoc, Detection and classification of COVID-19 by using faster R-CNN and mask R-CNN on CT images, 2023, 0941-0643, 10.1007/s00521-023-08450-y | |
11. | Hsieh-Fu Tsai, Soumyajit Podder, Pin-Yuan Chen, Microsystem Advances through Integration with Artificial Intelligence, 2023, 14, 2072-666X, 826, 10.3390/mi14040826 | |
12. | G. Prabakaran, K. Jayanthi, An efficient Covid-19 detection and severity analysis using optimized mask region-based convolution neural network, 2023, 45, 10641246, 11679, 10.3233/JIFS-230312 | |
13. | Pradip Senapati, Anusua Basu, Mainak Deb, Krishna Gopal Dhal, Sharp dense U-Net: an enhanced dense U-Net architecture for nucleus segmentation, 2024, 15, 1868-8071, 2079, 10.1007/s13042-023-02017-y | |
14. | Om Ramakisan Varma, Mala Kalra, Sheeraz Kirmani, COVID‐19: A systematic review of prediction and classification techniques, 2023, 33, 0899-9457, 1829, 10.1002/ima.22905 | |
15. | Adetokunbo MacGregor John-Otumu, Charles Ikerionwu, Oluwaseun Oladeji Olaniyi, Oyewole Dokun, Udoka Felista Eze, Obi Chukwuemeka Nwokonkwo, 2024, Advancing COVID-19 Prediction with Deep Learning Models: A Review, 979-8-3503-5815-5, 1, 10.1109/SEB4SDG60871.2024.10630186 | |
16. | Ritu Agarwal, Tanupriya Choudhury, Neelu J. Ahuja, Tanmay Sarkar, Adadi Parise, Hybrid Deep Learning Algorithm-Based Food Recognition and Calorie Estimation, 2023, 2023, 1745-4549, 1, 10.1155/2023/6612302 | |
17. | S. Cheraghi, S. Amiri, F. Abdolali, A. Janati Esfahani, A. Amiri Tehrani Zade, R. Ahadi, F. Ansari, E. Raiesi Nafchi, Z. Hormozi-Moghaddam, A modified deep learning model in the classification of post-COVID-19 lung disease and a comparative study on Iranian and international databases, 2024, 22, 1605-0258, 55, 10.61186/ijrr.22.1.55 | |
18. | Oyewole Dokun, Adetokunbo John-Otumu, Udoka Eze, Charles Ikerionwu, Chukwuemeka Etus, Emeka Nwanga, Ogadimma Okonkwo, Deep Learning Model for COVID-19 Classification Using Fine Tuned ResNet50 on Chest X-Ray Images , 2024, 9, 2637-5680, 10, 10.11648/j.mlr.20240901.12 | |
19. | Dyala Aljagoub, Ri Na, Chongsheng Cheng, Delamination detection in concrete decks using numerical simulation and UAV-based infrared thermography with deep learning, 2025, 170, 09265805, 105940, 10.1016/j.autcon.2024.105940 |
Method | AC | SP | PR | RC | F1 |
ResNet41 | 93.16% | 89.03% | 94.63% | 87.26% | 94.54% |
ResNet50 | 96.98% | 97.36% | 96.60% | 97.32% | 96.93% |
ResNet65 | 94.35% | 91.79% | 96.48% | 89.35% | 95.74% |
ResNet101 | 95.23% | 92.64% | 94.35% | 87.62% | 97.63% |
Fold Number | AC | SP | PR | RC | F1 |
Fold-1 | 97.55% | 97.10% | 98.0% | 97.12% | 97.55% |
Fold-2 | 98.0% | 99.0% | 97.0% | 98.97% | 97.97% |
Fold-3 | 98.70% | 97.40% | 100% | 97.46% | 98.71% |
Fold-4 | 93.75% | 96.50% | 91.0% | 96.92% | 93.57% |
Fold-5 | 96.90% | 96.80% | 97.0% | 96.80% | 96.89% |
Average | 96.98% | 97.36% | 96.60% | 97.32% | 96.93% |
Model used | Number of cases taken to train model | AC | SP | Reference |
Mask R-CNN | 534 COVID-19 positive and 134 COVID-19 negative | 96.98% | 97.36% | This work |
DeTraC-ResNet18 | 116 COVID-19 positive and 80 COVID-19 negative | 95.12% | 91.87% | Abbas et al. [22] |
SSD | 100 COVID-19 positive and 887 COVID-19 negative | 94.92% | 92% | Saiz et al. [21] |
COVIDX-Net | 25 COVID-19 positive | 90% | - | Hemdan et al. [26] |
VGG-19 | 224 COVID-19 positive | 93.48% | 98.75% | Ioannis et al. [28] |
COVID-Net | 53 COVID-19 positive and 5526 COVID-19 negative | 92.4% | - | Wang and Wong [27] |
ResNet50 + SVM | 25 COVID-19 positive and 25 COVID-19 negative | 95.38% | 93.47% | Sethy and Behera [29] |
Shallow CNN | 130 COVID-19 positive and 51 COVID-19 negative | 96.92% | - | Mukherjee et al. [30] |
Method | AC | SP | PR | RC | F1 |
ResNet41 | 93.16% | 89.03% | 94.63% | 87.26% | 94.54% |
ResNet50 | 96.98% | 97.36% | 96.60% | 97.32% | 96.93% |
ResNet65 | 94.35% | 91.79% | 96.48% | 89.35% | 95.74% |
ResNet101 | 95.23% | 92.64% | 94.35% | 87.62% | 97.63% |
Fold Number | AC | SP | PR | RC | F1 |
Fold-1 | 97.55% | 97.10% | 98.0% | 97.12% | 97.55% |
Fold-2 | 98.0% | 99.0% | 97.0% | 98.97% | 97.97% |
Fold-3 | 98.70% | 97.40% | 100% | 97.46% | 98.71% |
Fold-4 | 93.75% | 96.50% | 91.0% | 96.92% | 93.57% |
Fold-5 | 96.90% | 96.80% | 97.0% | 96.80% | 96.89% |
Average | 96.98% | 97.36% | 96.60% | 97.32% | 96.93% |
Model used | Number of cases taken to train model | AC | SP | Reference |
Mask R-CNN | 534 COVID-19 positive and 134 COVID-19 negative | 96.98% | 97.36% | This work |
DeTraC-ResNet18 | 116 COVID-19 positive and 80 COVID-19 negative | 95.12% | 91.87% | Abbas et al. [22] |
SSD | 100 COVID-19 positive and 887 COVID-19 negative | 94.92% | 92% | Saiz et al. [21] |
COVIDX-Net | 25 COVID-19 positive | 90% | - | Hemdan et al. [26] |
VGG-19 | 224 COVID-19 positive | 93.48% | 98.75% | Ioannis et al. [28] |
COVID-Net | 53 COVID-19 positive and 5526 COVID-19 negative | 92.4% | - | Wang and Wong [27] |
ResNet50 + SVM | 25 COVID-19 positive and 25 COVID-19 negative | 95.38% | 93.47% | Sethy and Behera [29] |
Shallow CNN | 130 COVID-19 positive and 51 COVID-19 negative | 96.92% | - | Mukherjee et al. [30] |