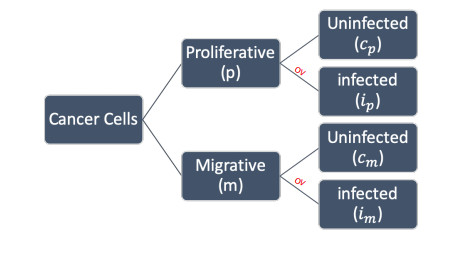
The increasing consumer demands for healthier and more sustainable foods has pushed the food industry in the constant research of new foods, new functional ingredients and bioactive compounds, whose production can be considered as far as sustainable. In this sense, application of the edible mushrooms has attracted the attention of industries because of their good nutritional quality, simple and economically affordable growth, taste, flavor, and textural properties, as well as the presence of bioactive compounds with positive effects on human health. Among edible mushrooms, Pleurotus spp. are considered among the most popular all over the world. Their cultivation is very simple and sustainable, because Pleurotus spp. efficiently grow on several substrates and can degrade various lignocellulosic waste materials. This means that Pleurotus mushrooms can be cultivable all over the world. From the inclusion in food products as extracts to the incorporation as fresh or into powder form, several works have been published in the literature concerning the use of mushrooms as functional ingredients. However, mushroom addiction can modify functional and physicochemical properties of the supplemented foods, hence the main challenge to overcome is to not negatively affect the sensory properties. Although many scientific works have been published on the matter, further research is needed to better understand the role of mushrooms as functional ingredients, due to the different results reported. This review aims for providing the more recent information about Pleurotus incorporation into foods, with a critical vision looking forward to the future, without forgetting an overview of the more recent literature about Pleurotus spp. nutritional value and their healthy promoting compounds.
Citation: Mena Ritota, Pamela Manzi. Edible mushrooms: Functional foods or functional ingredients? A focus on Pleurotus spp.[J]. AIMS Agriculture and Food, 2023, 8(2): 391-439. doi: 10.3934/agrfood.2023022
[1] | Abdulhamed Alsisi, Raluca Eftimie, Dumitru Trucu . Nonlocal multiscale modelling of tumour-oncolytic viruses interactions within a heterogeneous fibrous/non-fibrous extracellular matrix. Mathematical Biosciences and Engineering, 2022, 19(6): 6157-6185. doi: 10.3934/mbe.2022288 |
[2] | Nada Almuallem, Dumitru Trucu, Raluca Eftimie . Oncolytic viral therapies and the delicate balance between virus-macrophage-tumour interactions: A mathematical approach. Mathematical Biosciences and Engineering, 2021, 18(1): 764-799. doi: 10.3934/mbe.2021041 |
[3] | Taeyong Lee, Adrianne L. Jenner, Peter S. Kim, Jeehyun Lee . Application of control theory in a delayed-infection and immune-evading oncolytic virotherapy. Mathematical Biosciences and Engineering, 2020, 17(3): 2361-2383. doi: 10.3934/mbe.2020126 |
[4] | Joseph Malinzi, Rachid Ouifki, Amina Eladdadi, Delfim F. M. Torres, K. A. Jane White . Enhancement of chemotherapy using oncolytic virotherapy: Mathematical and optimal control analysis. Mathematical Biosciences and Engineering, 2018, 15(6): 1435-1463. doi: 10.3934/mbe.2018066 |
[5] | Baba Issa Camara, Houda Mokrani, Evans K. Afenya . Mathematical modeling of glioma therapy using oncolytic viruses. Mathematical Biosciences and Engineering, 2013, 10(3): 565-578. doi: 10.3934/mbe.2013.10.565 |
[6] | Christian Engwer, Markus Knappitsch, Christina Surulescu . A multiscale model for glioma spread including cell-tissue interactions and proliferation. Mathematical Biosciences and Engineering, 2016, 13(2): 443-460. doi: 10.3934/mbe.2015011 |
[7] | Dominik Wodarz . Computational modeling approaches to studying the dynamics of oncolytic viruses. Mathematical Biosciences and Engineering, 2013, 10(3): 939-957. doi: 10.3934/mbe.2013.10.939 |
[8] | Farinaz Forouzannia, Sivabal Sivaloganathan, Mohammad Kohandel . A mathematical study of the impact of cell plasticity on tumour control probability. Mathematical Biosciences and Engineering, 2020, 17(5): 5250-5266. doi: 10.3934/mbe.2020284 |
[9] | Marcello Delitala, Tommaso Lorenzi . Emergence of spatial patterns in a mathematical model for the co-culture dynamics of epithelial-like and mesenchymal-like cells. Mathematical Biosciences and Engineering, 2017, 14(1): 79-93. doi: 10.3934/mbe.2017006 |
[10] | Prathibha Ambegoda, Hsiu-Chuan Wei, Sophia R-J Jang . The role of immune cells in resistance to oncolytic viral therapy. Mathematical Biosciences and Engineering, 2024, 21(5): 5900-5946. doi: 10.3934/mbe.2024261 |
The increasing consumer demands for healthier and more sustainable foods has pushed the food industry in the constant research of new foods, new functional ingredients and bioactive compounds, whose production can be considered as far as sustainable. In this sense, application of the edible mushrooms has attracted the attention of industries because of their good nutritional quality, simple and economically affordable growth, taste, flavor, and textural properties, as well as the presence of bioactive compounds with positive effects on human health. Among edible mushrooms, Pleurotus spp. are considered among the most popular all over the world. Their cultivation is very simple and sustainable, because Pleurotus spp. efficiently grow on several substrates and can degrade various lignocellulosic waste materials. This means that Pleurotus mushrooms can be cultivable all over the world. From the inclusion in food products as extracts to the incorporation as fresh or into powder form, several works have been published in the literature concerning the use of mushrooms as functional ingredients. However, mushroom addiction can modify functional and physicochemical properties of the supplemented foods, hence the main challenge to overcome is to not negatively affect the sensory properties. Although many scientific works have been published on the matter, further research is needed to better understand the role of mushrooms as functional ingredients, due to the different results reported. This review aims for providing the more recent information about Pleurotus incorporation into foods, with a critical vision looking forward to the future, without forgetting an overview of the more recent literature about Pleurotus spp. nutritional value and their healthy promoting compounds.
The go or grow (GOG) hypothesis or the migration-proliferation dichotomy, proposes that cell proliferation and cell migration are two temporally exclusive events: cells either migrate or proliferate and they can periodically switch between proliferative and migratory states [1]. Studies on the GOG hypothesis are conflicting, with some studies supporting and confirming this hypothesis in vitro for different cancer cell types (e.g., glioma, melanoma, or breast cancer) [2,3,4,5,6,7,8,9] and other studies challenging this hypothesis [1,10,11]. For example, Tektonidis et al. [9] presented a computational data-driven study of in vitro glioma invasion based on three experimental papers, and concluded that the GOG mechanism combined with self-repulsion and a density-dependent phenotypic switch is mandatory to duplicate the experimental results [7,12]. On the other hand, Corcoran et al. [1] used time-lapse video-microscopy to monitor directional migration, invasion and mitosis of cancer cells, and concluded that in medulloblastoma cell lines there is no evidence to support the GOG hypothesis. More precisely, their results suggested that migrating and non-migrating cell lines have similar mitotic activities. Similarly, Garay et al. [10] tested this hypothesis on 12 mesothelioma, 13 melanoma and 10 lung cancer cell lines using time-lapse video-microscopy, and their results also contradicted the concept of GOG hypothesis.
Over the last decades, mathematical models have been used in addition to experimental studies to shed some light on this go or grow hypothesis. Many of these models are discrete models of cellular automata (CA) type or lattice gas cellular automata (LGCA) type [7,13,14,15,16,17]. There are also various continuum models described by partial differential equations [11,18,19,20,21,22,23,24,25]. These continuum models can either model separately the migrating and proliferative cancer cell sub-populations [20,21,22,24], or can model them via a single equation for one cancer population that can move for some time instances and proliferate for other time instances [11,18]. Those models that consider separate sub-populations of migrating and proliferative cancer cells incorporate also transition (i.e., switching) rates between these two sub-populations. These transition rates can be either constant [20,26] or density dependent: they can depend for example on the total density of cells [20,22], on the concentration of integrins bound to extracellular matrix (ECM) fibres [23], on the density of ECM fibres [25], on the level of oxygen [19,24].
It should be mentioned here that some GOG models have been reduced to simpler forms; for example, in [20] the authors assumed that proliferating cell sub-population could stop proliferating and thus their go-or-growth model was reduced to a go-or-rest model.
The majority of continuum mathematical models in the literature for the GOG hypothesis focus mainly on local interactions between cells. However, it is known that cells can mechanically sense and react to the presence of other cells up to 100μm away [27], and thus more and more mathematical models have been recently developed to consider such non-local cell-cell and cell-ECM interactions [28,29,30,31]. Nevertheless, these nonlocal models do not usually incorporate the GOG hypothesis.
In this study we plan to investigate (for the first time - to our knowledge) the impact of the GOG hypothesis on oncolytic virotherapies. These oncolytic virotherapies are cancer therapies that use oncolytic viruses (OVs), i.e., viruses that replicate inside and destroy cancer cells. Despite some clinical successes with these oncolytic virotherapies (currently a few such viruses are in the late stages of various clinical trials [32]) there are still many open questions related to the interactions between oncolytic viruses and tumour cells [33]. And, to our knowledge, it is not clear at this moment how the spread of oncolytic viruses through the solid tumours is affected by the GOG hypothesis. In this study, we investigate this particular aspect using a modelling and computational approach, which allows us to test numerically various hypotheses related to cancer-OV interactions.
To this end, we extend our previous non-local multi-scale mathematical model for cancer-oncolytic viruses (OV) interactions [34], by considering also the GOG hypothesis. We consider distinct proliferative and migrating cancer cell subpopulations, and assume that they can become infected with an oncolytic virus (see Figure 1). With the help of this new model, we explore numerically different aspects of the GOG hypothesis, as well as the possibility of having local vs. non-local cell interactions and their impact on cancer invasion in the context of oncolytic virotherapies. We need to emphasise here that with the help of this new model we investigate (for the first time - to our knowledge) the impact of the GOG hypothesis on oncolytic virotherapies.
We describe the new multiscale model in Section 2. The computational approach used to simulate numerically this model is described briefly in Section 3. Then, numerical simulations are presented in Section 4. We conclude in Section 5 with a brief summary and discussion of the results.
Adopting the multiscale moving boundary modelling approach introduced initially in [35], in the following we explore the dynamic interaction between an invading heterotypic tumour and an oncolytic virus. Indeed, considering here the go or grow hypothesis [1], the invading tumour is assumed to consist of two subpopulations of cancer cells, namely migrating and proliferative, which exercise their dynamics within the surrounding ECM and that can become infected by an oncolytic virus over a time interval [0,T]. For t∈[0,T], denoting by Ω(t) the spatial support of the progressing tumour that evolves inside a maximal tissue cube Y (i.e., Ω(t)⊂Y), for any x∈Ω(t), let cm(x,t), cp(x,t), and e(x,t) represent the spatial densities of the migrating cancer cells subpopulation, the proliferating cancer cells subpopulation, and the ECM, respectively. Furthermore, denoting here the oncolytic virus density by v(x,t), ∀x∈Ω(t), as both the migrating and the proliferating cancer cells can become infected by the oncolytic virus v(x,t), let im(x,t) and ip(x,t) represent the densities of infected migrating cancer cells and proliferating cancer cells, respectively (see Figure 1).
By incorporating here the GOG hypothesis, we expand and generalise the modelling framework for tumour-OV interaction proposed in [29,34]. Indeed, building on the multiscale framework introduced in [35], we explore these complex cancer-OV interactions by accounting for the interlinked two-scale dynamics that connects the tissue-scale (macro-scale) tumour macro-dynamics with the cell-scale (micro-scale) proteolytic activity of MDEs that occurs along the invasive edge of the tumour. In the following we describe in detail the macro-dynamics, the micro-dynamics, as well as the double feed-back loop that connects the two scales of activity in our new model.
For each t∈[0,t], and each x∈Ω(t), denoting the total cancer cell population by ctotal(x,t)
ctotal(x,t)=cp(x,t)+ip(x,t)+cm(x,t)+im(x,t), | (2.1) |
and defining the total tumour vector to be u(x,t)=(cp(x,t),ip(x,t),cm(x,t),im(x,t),e(x,t))T, the volume fraction of space occupied by the tumour can therefore be expressed mathematically as
ρ(u)=νee(x,t)+νc(ctotal(x,t)), | (2.2) |
where νe represents the fraction of physical space occupied by the ECM and νc is the fraction of physical space occupied collectively by all cancer subpopulations.
For the tumour dynamics, we assume that the motility of each of the cancer cells subpopulations is due to a combination of random movement (described by linear diffusion term) and a directed migration due to cell-cell and cell-ECM adhesion. The spatial fluxes triggered by cell adhesion that cause the directed cells migration are considered here both from a local and non-local perspective [36,37,38,39,40,41], and in following we will detail their mathematical formulation. For convenience, for each cancer cell subpopulation c∈{cp,cm,ip,im}, we consider a global notation φc(u) describing the effect of the cell adhesion processes either locally, through adhesive interactions between cancer cells and ECM (whereby the tumour cells exercise haptotactic movement [42] towards higher levels of ECM), or non-locally, where both cell-cell and cell-ECM adhesive interactions are accounted for within an appropriate cell sensing region. Thus, φc(u) is mathematically formalised as
φc(u):={ηc∇⋅(c∇e),local {haptotactic interactions between cancer cells and ECM},∇⋅(cAc(⋅,⋅,u(⋅,⋅))),non-local {cell - cells and cell - ECM interactions on a cell sensing region}, | (2.3) |
where, for any given subpopulation c∈{cp,cm,ip,im}, we have that ηc>0 is a constant haptotactic rate associated to c, while Ac(x,t,u(⋅,t)) is a non-local spatial flux term that is detailed as follows. Indeed, following a similar approach as in [28,43,44], ∀c∈{cp,cm,ip,im}, at each spatio-temporal point (x,t) the cell adhesion flux Ac(x,t,u(⋅,t)) cumulates the strengths of the cell-cell and cell-matrix adhesion junctions that cells from cancer subpopulation c that distributed at (x,t) establish with the other cell subpopulations and the ECM distributed within an appropriate maximal sensing region B(x,R) of radius R>0. This is formulated mathematically as
Ac(x,t,u(⋅,t))=1R∫B(0,R)n(y)K(‖y‖2)[Sccc(x+y,t)dy+>Scee(x+y,t)](1−ρ(u))+χΩ(t)(x+y,t)dy. | (2.4) |
here, χΩ(t)(⋅) is the characteristic function of Ω(t), while the term (1−ρ(u))+:=max{(1−ρ(u)),0} enables the avoidance of local overcrowding. Further, for any y∈B(0,R), n(y) denotes the unit radial vector originating from x and pointing to x+y∈B(x,R), which is given by
n(y):={y‖y‖2ify∈B(0,R)∖{(0,0)},(0,0)otherwise. | (2.5) |
with ∥⋅∥2 being the usual Euclidean norm. Moreover, K(⋅):[0,R]→[0,1] is a radially symmetric kernel that explores the dependance of the strengths of the established cell adhesion junctions on the radial distance from the centre of the sensing region x to ζ∈B(x,R). Since these adhesion junction strengths are assumed to decrease as the distance r:=∥x−ζ∥2 increases, K therefore is taken here of the form
K(r):=32πR2(1−r2R),∀r∈[0,R]. | (2.6) |
Furthermore, since in this study we focus only on self-adhesion and we do not consider cross-adhesion bonds between four cancer cells subpopulation, in Eq (2.4) we have that Scc and Sce for any given cancer cell subpopulation c∈{cp,cm,ip,im} represent the adhesive interaction strengths for the self−cell−cell adhesion and cell-ECM, respectively. While the cell-ECM adhesion strength Sce is considered to be a positive constant, the cell-cell adhesion strength Scc explores here the fact that the ability of the cell distributed at x to establish cell-cell adhesive junctions with the cells distributed at the other locations y∈B(x,R) depends on the amount of intercellular Ca2+ ions available within the ECM [45,46]. As a consequence, adopting a similar approach to the one in [47], we assume here that Scc is dependent on the ECM density and it takes the form
Scc(e)=Smaxccexp(1−11−(1−e(x,t))2), | (2.7) |
with Smaxcc representing the maximum strength of cell-cell adhesive junctions established by the cancer cells subpopulation c∈{cp,cm,ip,im} . Therefore, the adhesive strengths for cell-cell and cell-ECM adhesion for all four cancer subpopulation can be compactly expressed via the diagonal matrices
Scell−cell=[Scpcp0000Sipip0000Scmcm0000Simim]andScell−ECM=[Scpe0000Sipe0000Scme0000Sime], | (2.8) |
respectively.
Finally, in the context of the GOG hypothesis, another important aspect that occurs during the tumour dynamics is the transitions between from the proliferative cancer subpopulation and the migrating one. Adopting a similar form to the one proposed in [15,16,26], the transition from proliferative to migrating cancer cells is captured here through the switching term λcp,cm that is given by
λcp,cm=ω2cm−ω1cp, | (2.9) |
where ω1 is the rate of switching from proliferative state cp to migration state cm, and ω2 is the rate of switching from migration to proliferative state. On the other hand, the transition from migrating to proliferative cancer cells is expressed through the switching term λcm,cp defined as
λcm,cp=−λcp,cm. | (2.10) |
Thus, for each of the cancer cells subpopulation c∈{cp,cm,ip,im}, the spatial transport is a combination of random movement (expressed through diffusion) and directed movement due adhesion (explored either locally or non-locally, and represented compactly through φc(u)). Furthermore, for the particular case of the migrating and proliferative cells subpopulation cp, and cm, besides the spatial transport and in addition to their own proliferation (considered here of logistic type [48,49]), their dynamics is also affected by the cell population "exchanges" due to proliferative-migrating transitions (i.e., transitions between proliferative and migrating subpopulations) as well as by the presence of the oncolytic virus that is able to infect cells from both populations. Finally, for their part, the infected cancer cells subpopulations, while exercising a spatial transport of the type described above, they contribute to virus replication and die. Therefore, the dynamics for each cancer cell population can be expressed mathematically as follows.
First, the governing equation for the uninfected proliferative cancer cell subpopulation is given by
∂cp∂t=DcpΔcp−φcp(u)+μpcp(1−ρ(u))−ϱpcpv+λcp,cm, | (2.11) |
where Dcp>0 is a constant diffusion coefficient, the term φcp(u) represents the directed movement triggered by cell-adhesion processes that corresponds to cp and is described in Eq (2.3) for c=cp. Further, μp>0 is an intrinsic constant proliferation rate, ϱp>0 is the rate at which the oncolytic virus infects the proliferative cancer cell population, and λcp,cm is the switching term given in (2.9), representing the process through which migrating cancer cells transition towards proliferative state during the tumour dynamics.
The infected proliferative cancer cell population, ip(t,x), which emerges within this dynamics due to the OV infection of cp, also exercises a spatio-temporal dynamics that is governed by the following equation
∂ip∂t=DipΔip−φip(u)+ϱpcpv−δipip, | (2.12) |
where Dip>0 is a constant random motility coefficient, and φip(u) is the spatial influence of the cell-adhesion processes that is described in Eq (2.3) and corresponds to ip. The cancer cell population increases with at rate ϱp due to the new infections of the proliferative cancer cells, and decreases at rate δip>0 due to infected cell death.
Further, since for the migrating cancer cell population we always take into account not only cell-ECM adhesion but also cell-cell self-adhesion, the directed cell migration term φcm(u) that is defined in Eq (2.3) and corresponds to cm is in this case constantly of the non-local form
φcm(u)=∇⋅(cmAcm(⋅,⋅,u(⋅,⋅))), |
where the spatial flux Acm(⋅,⋅,u(⋅,⋅)) is the one defined in Eq (2.4) for c=cm. As a consequence, the governing equation for the uninfected migrating cell population is
∂cm∂t=DcmΔcm−∇⋅(cmAcm)+μmcm(1−ρ(u))−ϱmcmv+λcm,cp, | (2.13) |
where Dcm>0 is a constant diffusion coefficient, μm>0 is a constant proliferation coefficient, ϱm>0 is a constant rate at which the uninfected migrating population diminishes due infection by the oncolytic virus v. Further, λcm,cp is the switching term given in Eq (2.10) that represents the net transition from the proliferative into the uninfected migrating state that occurs per unit time during the tumour dynamics.
The fourth tumour cell population is the infected migrating cancer cell subpopulation im(t,x) that emerges within this dynamics due to infections by the OV, and its spatio-temporal dynamics is governed by the following equation
∂im∂t=DimΔim−φip(u)+ϱmcmv−δimim. | (2.14) |
where Dim>0 is a constant random motility coefficient, and φim(u) represents the directed migration induced by the cell-adhesion processes that corresponds to im and is described in Eq (2.3) for c=im Further, the infected migrating population expand at a rate ϱm due to new infections occurring among the uninfected migrating cells, and they also die at rate δim>0.
At the same time, the ECM is degraded by both uninfected and infected cancer cell populations and is remodelled within the limit of available space. Thus, its governing dynamics is given mathematically by
∂e∂t=−e(αcpcp+αipip+αcmcm+αimim)+μ2e(1−ρ(u)), | (2.15) |
where αcp>0, αip>0, αcm>0, and αim>0 are the ECM degradation rates caused by cancer cells subpopulation cp, ip, cm, and im, respectively. Further, μ2>0 is a constant ECM remodelling rate.
Concerning the oncolytic virus spatio-temporal dynamics, we adopt here a similar reasoning as in [34], and we assume that the OV motion is described by a random movement that is biased by a "haptotactic-like" spatial transport towards higher ECM levels. Thus, the dynamics of the oncolytic virus that we consider here is governed by
∂v∂t=DvΔv−ηv∇⋅(v∇e)+bmim+bpip−(ϱmcm+ϱpcp)v−δvv, | (2.16) |
where Dv>0 is a constant random motility coefficient, ηv>0 is a constant haptotactic coefficient, bm,bp>0 are a viral replication rates within infected proliferating and infected migrating cancer cells, respectively, and δv>0 is the viral decay rate.
Finally, the coupled interacting tumour − OV macro-dynamics is governed by Eqs (2.11)-(2.16) in the presence of initial conditions
cp(x,0)=c0p(x),ip(x,0)=i0p(x),cm(x,0)=c0m(x),andim(x,0)=i0m(x),∀x∈Ω(0), | (2.17) |
while assuming zero-flux boundary conditions at the moving tumour interface ∂Ω(t).
During their macro-scale dynamics, the four cancer cells subpopulations that get near the tumour interface (i.e., within the outer proliferating rim of the tumour) are able to secrete matrix degrading enzymes (such as the matrix metalloproteinases [50,51]), providing this way a source of MDEs for a cell-scale (micro-scale) proteolytic micro-dynamics that takes place along the invasive edge of the tumour. Indeed, in the presence of this source of MDEs (induced by the tumour macro-dynamics), a cross-interface micro-scale MDEs spatial transport occurs within a micro-scale neighbourhood of the tumour boundary of an appropriate cell-scale thickness ϵ>0, denoted here simply by Nϵ(∂Ω(t)). The areas of significant ECM degradation caused by the pattern of propagation of the advancing front of MDEs within the peritumoural region Nϵ(∂Ω(t))∖Ω(t) will ultimately be explored by the cancer cells that will progress in those regions [51], and so precisely these boundary regions (affected by significant ECM degradation) will shape the pattern of tumour progression. Thus, following the modelling approach introduced in [35] we depict these regions of significant ECM degradations by exploring the MDEs micro-dynamic processes within Nϵ(∂Ω(t)), which enables us ultimately to determine the law of the macro-scale tumour boundary movement.
To formalise these laws of macro-scale boundary movement induced by the boundary MDEs micro-dynamics, we adopt here the approach introduced in [35]. Therefore, the micro-scale neighbourhood Nϵ(∂Ω(t)) is given here as a union of a covering bundle of ϵ−size overlapping micro-domains {ϵY}ϵY∈Pϵ(t), namely,
Nϵ(∂Ω(t)):=⋃ϵY∈P(t)ϵY. |
This enables us to decompose the MDEs micro-dynamics on Nϵ(∂Ω(t)) by exploring this as a union of micro-dynamic processes occurring on each ϵY∈Pϵ(t). At any instance in time t0>0, on each micro-domain ϵY∈Pϵ(t0), a source of MDEs appears at every micro-scale location z∈ϵY∩Ω(t0) as a collective contribution of all the cells (both infected and uninfected) from the tumour outer proliferating rim that arrive during their dynamics within a distance ρ>0 from z. Thus, over any small time interval of length Δt>0, [t0,t0+Δt] and at any micro-scale spatial location z∈ϵY, this MDEs source is therefore given as
fϵY(z,τ)={∫B(z,ρ)∩Ω(t0)(γcpcp+γipip+γcmcm+γimim)(x,t0+τ)dxλ(B(z,ρ)∩Ω(t0)),z∈ϵY∩Ω(t0),0,otherwise, | (2.18) |
where λ(⋅) is the standard Lebesgue measure on RN, the ball B(z,r):={x∈Y:‖z−x‖∞≤ρ} is the maximal outer proliferating rim region from where cells that get to contribute to the formation of MDEs source at (z,τ)∈ϵY×[t0,t0+Δt], and γcp, γip, γcm, γim are all positive constants representing the contributions of the cancer subpopulations of uninfected proliferative cells, infected cancer cells, uninfected migrating cells, and infected migrating cells, respectively.
In the presence of the micro-scale source of MDEs induced from the macro-dynamics on each micro-domain ϵY, these matrix degrading enzymes exhibits a diffusion transport process within the entire ϵY. Thus, denoting the MDEs distribution at (z,τ)∈ϵY×[0,Δt] by m(z,τ), the MDEs micro-dynamics on each ϵY is given by
∂m∂τ=DmΔm+fϵY(z,τ) | (2.19) |
where z∈ϵY, τ∈[0,Δt]. Furthermore, since we assume no pre-existing MDEs within ϵY prior to the initiation of the proteolytic micro-dynamics, the MDEs micro-dynamics Eq (2.25g) takes place in the presence of zero initial conditions. Furthermore, we assume the presence of zero-flux boundary condition, namely
m(z,0)=0,n⋅∇m∣∂Ω=0, | (2.20) |
where nϵY is the outward unit normal on the frontier of the micro-domain ∂ϵY.
During the micro-dynamics Eqs (2.19) and (2.20), the MDEs transported across the interface in the peritumoural region ϵY∖Ω(t0) interact with ECM distribution that they encounter, resulting in degradation of ECM constituents. The advancement of MDEs within the peritumoural region ϵY∖Ω(t) lead to a degradation of the ECM in that cell-scale region, and determines the way the macroscopic tumour boundary evolves, leading to the establishment of a boundary movement law. Indeed, following the derivation in [35], the MDEs micro-dynamics on each micro-domain ϵY enables us to derive the movement characteristics for the relocation of the macro-scale tumour boundary ∂Ω(t)∩ϵY, expressing these through the derivation of a direction of movement ηϵY and a displacement magnitude ξϵY in that direction for the advancement of ∂Ω(t)∩ϵY within the peritumoural region Nϵ(∂Ω(t))∖Ω(t). To simplify the representation, the choreographic movement exercised by the ∂Ω(t)∩ϵY over a given time span [t0,t0+Δt] is represented back at macro-scale through the movement of the associated boundary midpoint x∗ϵY of ϵY (defined topologically with full details in [35], and which can be regarded as "the center of ∂Ω(t)∩ϵY"), as illustrated in Figure 2. For completeness, we briefly outline below the main steps involved in deriving the boundary relocation characteristics that were introduced in [35].
On the cell-scale neighbouring bundle Nϵ(∂Ω(t0)) of the tumour interface, for each of the boundary micro-domains ϵY∈P(t0) at a given a time instance t0>0, we use the regularity property of Lebesgue measure [52] to depict the first dyadic decomposition {Dk}k∈IϵY of ϵY that has the property that the union of those dyadic cubes Dk included in the complement of Ω(t0) approximate to a given global micro-scale accuracy δΩ(⋅)>0. This is schematically illustrated by the small green squares in Figure 2 that are situated outside the black tumour boundary ∂Ω(t0)∩ϵY. Further, denoting by yk the barycenter of Dk, we sub-select a sub-family of dyadic cubes {Dk}k∈I∗ϵY⊂{Dk}k∈IϵY that consists only of those dyadic cubes that are situated furthest away from the boundary midpoint x∗ϵY (corresponding to ϵY) with the property that they carry an amount of MDEs above the mean of MDEs transported within the entire preritumoural region ϵY∖Ω(t0), hence covering precisely the region of significant ECM degradation caused by MDEs within ϵY∖Ω(t0), as illustrated in Figure 2. Thus, by cumulating the contribution to the significant ECM degradation within ϵY∖Ω(t0) of all the dyadic cubes {Dk}k∈I∗ϵY while accounting on both their relative spatial location with respect to x∗ϵY and the amount of MDEs that they get to carry at time τf:=t0+Δt, we obtain the direction of choreographic boundary relocation (exercised by the ∂Ω(t)∩ϵY) due to micro-scale MDEs degradation. Therefore, this boundary movement direction is given by the positive direction of the emerging line defined by the position vectors involved {→ykx∗ϵY}k∈I∗ϵY magnified accordingly by the MDEs mass that each dyadic cube in {Dk}k∈I∗ϵY, carries, namely
ηϵY=x∗ϵY+ν∑k∈I∗ϵY(∫Dkm(z,τf)dz)(yk−x∗ϵY),ν∈[0,∞). | (2.21) |
Furthermore, the magnitude of the actual boundary movement in direction ηϵY is appropriately given as a weighted sum of the Euclidean magnitudes of the position vectors {∥ykx∗ϵY∥2}k∈I∗ϵY, with the weights accounting on the relative contribution brought to the ECM degradation of each of the corresponding dyadic cubes. Thus the movement magnitude in direction ηϵY is given by
ξϵY(x):=∑k∈I∗ϵY∫Dkm(z,τf)dz∑k∈I∗ϵY∫Dkm(z,τf)dz∥→xyk∥2. | (2.22) |
Therefore, as the tumour boundary relocation induced by the micro-dynamics on each ϵY is represented at macro-scale through the movement of the boundary midpoint x∗ϵY, in the context that enough but not complete ECM degradation occurs within ϵY∖Ω(t0) (tissue condition that is detailed and explored in full in [35]), we have that x∗ϵY exercises a relocation to a new position ~x∗ϵY that is given by
~x∗ϵY=x∗ϵY+ξϵY(x)~ηϵY∥~ηϵY∥2, | (2.23) |
where
~ηϵY:=∑k∈I∗ϵY(∫Dkm(z,τf)dz)(yk−x∗ϵY), | (2.24) |
and is illustrated through the dark blue arrow in Figure 2. Thus, a law for macro-scale tumour boundary movement is this way induced by the MDEs micro-dynamics, enabling us to capture the evolution of the tumour boundary over the time interval [t0,t0+Δt] from its state Ω(t0) at t0 to a new spatial configuration at Ω(t0+Δt) at t0+Δt. This relocated domain Ω(t0+Δt) allows the initiation of the dynamics on the next time interval [t0,t0+Δt] where the tumour-oncolytic virus interaction continues its proceedings.
In summary, the multiscale moving boundary model that we obtained for the tumour−OV interaction (schematically illustrated in Figure 2) is structured as follows,
the tumour-OVmacro-dynamics:∂cp∂t=DcpΔcp−φcp(u)+μpcp(1−ρ(u))−ϱpcpv+λcp,cm, | (2.25a) |
∂ip∂t=DipΔip−φip(u)+ϱpcpv−δipip, | (2.25b) |
∂cm∂t=DcmΔcm−∇⋅(cmAcm)+μmcm(1−ρ(u))−ϱmcmv+λcm,cp, | (2.25c) |
∂im∂t=DimΔim−φip(u)+ϱmcmv−δimim, | (2.25d) |
∂e∂t=−e(αcpcp+αipip+αcmcm+αimim)+μ2e(1−ρ(u)), | (2.25e) |
∂v∂t=DvΔv−ηv∇⋅(v∇e)+bmim+bpip−(ϱmcm+ϱpcp)v−δvv, | (2.25f) |
boundary MDEsmicro-dynamics:∂m∂τ=DmΔm+fϵY(z,τ) | (2.25g) |
The macro-dynamics and micro-dynamics are connected through a double feedback loop enabled by:
● a top-down link by which the macro-dynamics induces the source for the micro-dynamics given in Eq (2.18).
● a bottom-up link by which the MDEs micro-dynamics induces and determines the law for the macro-scale tumour boundary movement.
The numerical approach and computational implementation of the novel multiscale moving boundary model require a number of steps that build on the multiscale moving boundary computational framework initially introduced by [35] and further expanded in [34,47].
Macro-scale computations. The maximal macro-scale tissue domain Y⊂R2, where the tumour-OV interacting macro-dynamics Eq 2.25(a)-(f) takes place, is considered here to be Y:=[0,4]×[0,4] and is discretised uniformly using a spatial step size Δx=Δy:=h, with h>0. Let's denote by Yd the discretised Y, i.e., Yd:={(x1i,x2j)}i,j=1…N, with N=[4/h]+1. Further, as the macro-dynamics Eq 2.25(a)-(f) is addressed only on the expanding tumour domain Ω(t)⊂Y, for convenience, for any t>0, we denote the discretised tumour domain by Ωd(t) (i.e., Ωd(t)=Yd∩Ω(t)) and the discretised tumour boundary by ∂Ωd(t) (i.e., the frontier of Ωd(t) is ∂Ωd(t)). To carry out the computations exclusively on the expanding tumour, the numerical scheme that we developed here involves a method of lines-type approach combined with a non-local predictor corrector time-marching method introduced in [47] (and, for completeness, summarised also in Appendices A and B). Finally, as the tumour progresses, Ωd(t) is appropriately expanded by activating and including within tumour domain the new points invaded by cancer within Yd.
Approximating the micro-dynamics and its top-down and bottom-up links with the tumour−OV macro-dynamics. At any instance of time t0, we consider that the cell-scale covering bundle {ϵY}ϵY∈Pϵ(t0) of the discretised tumour interface ∂Ωd(t0) consists of overlapping squares ϵY of micro-scale size ϵ:=2h, which are centred at each of the tumour interface spatial node (x1s,x2p)∈∂Ωd(t0), i.e.,
{ϵY}ϵY∈Pϵ(t0)={B∥⋅∥∞((x1s,x2p),ϵ/2)|(x1s,x2p)∈∂Ωd(t0)} |
where ∥⋅∥∞ is the usual ∞−norm, and B∥⋅∥∞((x1s,x2p),ϵ/2):={(z1,z2)∈R2|∥(x1s,x2p)−(z1,z2)∥∞≤ϵ/2} is the closed ball of radius ϵ/2.} By adopting a similar approach to the one introduced in [35], we use using bilinear shape functions to calculate the MDE source given by Eq (2.18) on each micro-domain ϵY. To solve MDEs micro-dynamics Eq (2.25g), we use backward Euler in time combined with central differences for the spatial discretisation. After finding the MDE distribution m(z,τ), with (z,τ)∈ϵY×[0,Δt], we follow the modelling and computational approach introduced in [35] to determine the direction ηϵY and displacement magnitude ξϵY for the movement of the tumour boundary ∂Ω(t0)∩ϵY that is captured by each micro-domain ϵY:=B∥⋅∥∞ ((x1s,x2p),ϵ) and is represented trough the movement of its midpoint (x1s,x2p)∈∂Ωd(t0). Finally, we use these movement characteristics induced from the micro-dynamics (i.e., ηϵY and ξϵY, ∀ϵY∈Pϵ(t0)) to proceed with the corresponding global relocation of the macro-scale tumour boundary ∂Ωd(t0) to its new spatial configuration ∂Ωd(t0+Δt), which emerges due to the multiscale tumour evolution over the time interval [t0,t0+Δt].
In our numerical experiments, we explore the multiscale model dynamics on three distinct local and non-local scenarios that we consider within the macro-dynamics Eq 2.25(a)-(f) for the directed migration due to cell adhesion for cancer cell subpopulations cp, ip, and im. Specifically, we consider the following cases:
1. The cell-adhesion interactions for both the uninfected proliferative subpopulation cp and for the infected subpopulations ip, and im are considered to be local of haptotactic type, i.e., in the coupled macro-dynamics in Eq 2.25(a)-(f) we have φcp(u)=ηcp∇⋅(cp∇e), φip(u)=ηip∇⋅(ip∇e), and φim(u)=ηim∇⋅(im∇e). Thus, the macro-dynamics Eq 2.25(a)-(f) is in this case of the form:
∂cp∂t=DcpΔcp−ηcp∇⋅(cp∇e)+μpcp(1−ρ(u))−ϱpcpv+λcp,cm, | (3.1a) |
∂ip∂t=DipΔip−ηip∇⋅(ip∇e)+ϱpcpv−δipip, | (3.1b) |
∂cm∂t=DcmΔcm−∇⋅(cmAcm)+μmcm(1−ρ(u))−ϱmcmv+λcm,cp, | (3.1c) |
∂im∂t=DimΔim−ηim∇⋅(im∇e)+ϱmcmv−δimim, | (3.1d) |
∂e∂t=−e(αcpcp+αipip+αcmcm+αimim)+μ2e(1−ρ(u)), | (3.1e) |
∂v∂t=DvΔv−ηv∇⋅(v∇e)+bmim+bpip−(ϱmcm+ϱpcp)v−δvv, | (3.1f) |
with results for this case shown in Figure 4.
2. The cell-adhesion interactions for the uninfected proliferative subpopulation cp are considered now to be non-local, while the infected subpopulations ip, and im are still considered to be local of haptotactic type. Hence, in the coupled macro-dynamics in Eq 2.25(a)-(f) we have φcp(u)=∇⋅(cpAcp(⋅,⋅,u(⋅,⋅))), while φip(u)=ηip∇⋅(ip∇e), and φim(u)=ηim∇⋅(im∇e). Thus, the macro-dynamics Eq 2.25(a)-(f) is in this case of the form:
∂cp∂t=DcpΔcp−∇⋅(cpAcp(⋅,⋅,u(⋅,⋅)))+μpcp(1−ρ(u))−ϱpcpv+λcp,cm, | (3.2a) |
∂ip∂t=DipΔip−ηip∇⋅(ip∇e)+ϱpcpv−δipip, | (3.2b) |
∂cm∂t=DcmΔcm−∇⋅(cmAcm)+μmcm(1−ρ(u))−ϱmcmv+λcm,cp, | (3.2c) |
∂im∂t=DimΔim−ηim∇⋅(im∇e)+ϱmcmv−δimim, | (3.2d) |
∂e∂t=−e(αcpcp+αipip+αcmcm+αimim)+μ2e(1−ρ(u)), | (3.2e) |
∂v∂t=DvΔv−ηv∇⋅(v∇e)+bmim+bpip−(ϱmcm+ϱpcp)v−δvv, | (3.2f) |
with results for this case shown in Figure 5(a).
3. Finally, all the cell-adhesion interactions for both the uninfected proliferative subpopulation cp and for the infected subpopulations ip, and im are considered to be non-local, i.e., in the coupled macro-dynamics in Eq 2.25(a)-(f) we have φcp(u)=∇⋅(cpAcp(⋅,⋅,u(⋅,⋅))), φip(u)=∇⋅(ipAip(⋅,⋅,u(⋅,⋅))), and φim(u)=∇⋅(imAim(⋅,⋅,u(⋅,⋅))). Thus, the macro-dynamics Eq 2.25(a)-(f) is in this case of the form:
∂cp∂t=DcpΔcp−∇⋅(cpAcp)+μpcp(1−ρ(u))−ϱpcpv+λcp,cm, | (3.3a) |
∂ip∂t=DipΔip−∇⋅(ipAip)+ϱpcpv−δipip, | (3.3b) |
∂cm∂t=DcmΔcm−∇⋅(cmAcm)+μmcm(1−ρ(u))−ϱmcmv+λcm,cp, | (3.3c) |
∂im∂t=DimΔim−∇⋅(imAim)+ϱmcmv−δimim, | (3.3d) |
∂e∂t=−e(αcpcp+αipip+αcmcm+αimim)+μ2e(1−ρ(u)), | (3.3e) |
∂v∂t=DvΔv−ηv∇⋅(v∇e)+bmim+bpip−(ϱmcm+ϱpcp)v−δvv, | (3.3f) |
with results for this case shown in Figure 5(b).
The initial conditions for the uninfected proliferative cancer cell population, cp(x,0) is chosen to describe a small localised pre-existing tumour aggregation. This is given by the following equations:
c0p(x)=0.5(exp(−‖x−(2,2)‖222h)−exp(−3.0625))(χB((2,2),0.5−γ)∗ψγ),∀x∈Y, | (3.4) |
whose plot is shown in Figure 3(a). Here ψγ:RN→R+ is the usual standard mollifier of radius γ<<Δx3 given by
ψγ(x):=1γNψ(xγ), | (3.5) |
where ψ is the smooth compact support function given by
ψ(x):={exp1‖x‖22−1if‖x‖2<1,0otherwise. | (3.6) |
Moreover, we assume that the tumour is detected early enough so that migration is not initiated at the start of these simulations, and thus
c0m(x)=0,∀x∈Y. | (3.7) |
Also, since there is no infection at this stage, we assume that both infected proliferative (ip(x,0)) and migrating (im(x,0)) cancer cells are zero:
i0p(x)=0,andi0m(x)=0,∀x∈Y. | (3.8) |
Furthermore, the initial condition for the ECM density, e(x,0), is represented by an arbitrarily chosen heterogeneous pattern described by the following equations (as in [47])
e(x,0)=12min{h(ζ1(x),ζ2(x)),1−c0p(x)}, | (3.9) |
and is shown in Figure 3(b). Here, we have
h(ζ1(x),ζ2(x)):=12+14sin(ξζ1(x)ζ2(x))3⋅sin(ξζ2(x)ζ1(x)),(ζ1(x),ζ2(x)):=13(x+32)∈[0,1]2,∀x∈Y,andξ=7π. | (3.10) |
While other types of heterogeneous ECM patterns could be considered (see [53]), here we focus our attention to explore cancer-viral dynamics on this particular ECM pattern.
Finally, the initial conditions for the OV population, v(x,0) is chosen to describe one single injection in the middle of the tumour aggregation, as in [34]. This is described by the equation
v0(x)=Φ(x)⋅θ(v), | (3.11) |
where
Φ(x)=18(exp(−‖x−(2,2)‖222h)−exp(−1.6625)),andθ(v)={1ifΦ(x)>5×10−5,0otherwise. | (3.12) |
In computations, the initial condition is smoothed out on the frontier of the viral density support Γv:=∂{x∈Y|v0(x)>0} via the averaging
v(x1,x2)=18(−v(x1,x2)+∑i,j∈{−1,0,1}v(x1+ih,x2+jh)),∀(x1,x2)∈Γv. | (3.13) |
The numerical results presented in this Section are obtained with the parameter values described in Table 1 which, for convenience we call them 'baseline parameters'. Whenever we vary these parameters, we state clearly the new values we use for those simulations. Note that these baseline parameters are based on other papers or on our own estimates. For instance, using the GOG hypothesis, we estimated that Dcp is likely much smaller than Dcm. Since we could not find an exact value for Dcp (Dcm was assumed to be 0.00035, as in [43]), we arbitrarily estimated Dcp=10−5.
Param. | Value | Description | Reference |
Dcm | 0.00035 | Uninfected migrating cancer cell diffusion coefficient | [43] |
Dim | 0.0054 | Infected migrating cancer cell diffusion coefficient | [54] |
Dip | 0.00054 | Infected proliferative cancer cell diffusion coefficient | Estimated |
Dcp | 10−5 | Uninfected proliferative cancer cell diffusion coefficient | Estimated |
Dv | 0.0036 | Constant diffusion coefficient for OV | [54] |
ηcp | 0.00285 | Infected proliferative cancer cell haptotaxis coefficient | Estimated |
ηim | 0.0285 | Infected migrating cancer cell haptotaxis coefficient | [29] |
ηip | 0.00285 | Infected proliferative cancer cell haptotaxis coefficient | Estimated |
ηv | 0.0285 | OV haptotaxis coefficient | [29] |
μm | 0.5 | Proliferation rate for uninfected migrating cancer cells | [41] |
μp | 0.75 | Proliferation rate for uninfected proliferative cancer cells | [41] |
Scmcm | 0.1 | Maximum rate of cell-cell adhesion strength | [44] |
Scpcp | 0.05 | Maximum rate of cell-cell adhesion strength | Estimated |
Simim | 0.1 | Maximum rate of cell-cell adhesion strength | Estimated |
Sipip | 0.05 | Maximum rate of cell-cell adhesion strength | Estimated |
Scme | 0.5 | Rate of Cell-ECM adhesion strength | [55] |
Scpe | 0.001 | Rate of Cell-ECM adhesion strength | Estimated |
Sime | 0.5 | Rate of Cell-ECM adhesion strength | Estimated |
Sipe | 0.001 | Rate of Cell-ECM adhesion strength | Estimated |
ω1 | 0.1 | Rate of switching from proliferative state (cp) to migration state (cm) | Estimated |
ω2 | 0.4 | Rate of switching from migration (cm) to proliferative state (cp). | Estimated |
αcm | 0.075 | ECM degradation rate by uninfected cancer cells | Estimated |
αim | αcm2 | ECM degradation rate by infected cancer cells | [29] |
αcp | 0.075 | ECM degradation rate by uninfected cancer cells | Estimated |
αip | αcm2 | ECM degradation rate by infected cancer cells | Estimated |
μ2 | 0.02 | Remodelling term coefficient | [34] |
ϱm | 0.079 | Infection rate of cm cells by OV | [29] |
ϱp | 0.079 | Infection rate of cells by OV | Estimated |
δim | 0.05 | Death rate of infected cancer cells | [54] |
δip | 0.05 | Death rate of infected cancer cells | Estimated |
bm | 40 | Replicating rate of OVs in infected cancer cells cm | Estimated |
bp | 40 | Replicating rate of OVs in infected cancer cells cp | Estimated |
δv | 0.05 | Death rate of OV | [54] |
νe | 1 | The fraction of physical space occupied by the ECM | [47] |
νc | 1 | The fraction of physical space occupied by cancer cells | [47] |
γcm | 1.5 | MDEs secretion rate by uninfected cancer cell | [56] |
γim | 1 | MDEs secretion rate by infected cancer cell | [56] |
γcp | 1 | MDEs secretion rate by uninfected cancer cell | Estimated |
γip | 1.5 | MDEs secretion rate by infected cancer cell | Estimated |
Dm | 0.004 | MDE diffusion coefficient | [57] |
We start in Section 4.1 by investigating numerically the impact of local vs. nonlocal approaches used to describe the cell-cell and cell-matrix adhesion flux. Then, in Section 4.2, we investigate the impact of varying the adhesion strength in the non-local cell flux. Following that we focus on the system of Eq (3.1) without haptotaxis for cp (i.e.,ηcp=0), to investigate the impact of varying different parameters: in Section 4.3 we vary the impact of transition rate between migrating and proliferative cells, in Section 4.4 we vary the impact of OVs infection rate, and in Section 4.5 we vary the impact of OVs replication rate.
First, we focus on model (3.1), described in detail in Section 3.2. In Figure 4(a) we show the dynamics of our multiscale model in the absence of haptotactic terms for the proliferative uninfected cells (ηcp=0), while in Figure 4(b) we show the dynamics of this model in the presence of such haptotactic terms (where ηcp=0.00285, as given in Table 1). We can see that, for the parameter values used in these simulations, there is no difference in the spatial distribution of migrating uninfected or infected cancer cells between panels (a) and (b). However, the addition of haptotactic movement impacts the spatial distribution of proliferative uninfected cancer cells, leading to a more localised cancer cells distribution.
Next, we investigate numerically the dynamics of models (3.2) and (3.3). In Figure 5(a) we show the dynamics of our multi-scale model (3.2) that has two non-local fluxes cm and cp, while in Figure 5(b) we show what happens when we consider non-local fluxes for all cancer cells subpopulations (i.e., model (3.3)). Clearly there is a difference between these results and the previous one. The cancer cells for the case with two non-local fluxes (i.e., for proliferative cp and migrating cm cells) are more invasive, but have lower densities compared to the case where we assume four non-local adhesion fluxes. We can also see that the OV density for the case described by model (3.2) (with two nonlocal fluxes) is almost double compared to the OV density for the case described by model (3.3) (with four nonlocal fluxes).
In this subsection we investigate the effect of cell-cell and cell-matrix adhesion strengths for the two non-local subpopulations (i.e., model (3.2)) versus the four non-local subpopulations (i.e., model (3.3)). In [34] the authors studied the impact of different adhesion strengths in a model with one homogeneous cancer population and showed that when cell-cell adhesion strength was lower than cell-matrix adhesion strength it led to larger tumour spread. Since here we focus on two different cancer cell sub-populations (i.e., migrating and proliferative), we assume that cell-cell adhesion strength is lower than cell-matrix adhesion strength for the migrating cancer cells cm,im (to allow for cell migration), and the other way around for the proliferative cancer cells cp,ip (to reduce cell migration). The results of numerical simulations with these different adhesion strengths are shown in Figure 5. In this case we see relatively similar tumour spread patterns for (a) model (3.2) with two non-local sub-populations and for (b) model (3.3) with four non-local sub-populations. The only difference is a slight increase in the density for uninfected cancer cells and a decrease in the density of infected cancer cells for the model in sub-panels (b).
Since it is difficult to measure the adhesion strengths for cells with different phenotypes, in Figure 6 we also investigate numerically what happens with tumour and virus spread patterns when we assume that all cancer subpopulations have similar cell-cell adhesion strengths that are lower than their cell-matrix adhesion strengths. In this case we see that the cancer cells show less spatial spread compare to the case in Figure 5. Moreover, the OV (which has the highest density in the middle of the tumour mass) cannot destroy the tumour in that region; this is more evident in sub-panels (b) (for model (3.3) with four non-local sub-populations), where the level of the virus is also very reduced. In sub-panels (a) (for model (3.2) with two non-local sub-populations) we still see a bit of reduction in tumour size in the middle of the tumour region where the level of OV is similar as in Figure 5.
We conclude from these two numerical studies that the magnitudes of cell-cell and cell-matrix adhesion strengths for different cancer cell phenotypes (here migrating and proliferative cells), combined with their local/non-local character, influence significantly the spread of the virus through the tumour.
In the next three sub-sections we return to model (3.1) without haptotaxis for cp cells (i.e., ηcp=0), and investigate the impact of transition rates between migrating and proliferative cell sub-populations, as well as the impact of virus infection and replication rates.
We return now to model (3.1) without haptotaxis for cp (i.e., ηcp=0), and investigate numerically the impact of varying the transition rates between migrating and proliferating cancer cells, and the differences from the baseline results shown before in Figure 4(a). In Figure 7 we investigate the spatial spread of tumour and virus populations when (a) ω2=ω1=0.1, and (b) ω2=ω14=0.025. We see that decreasing ω2 leads to an increase in the density of migrating cells (cm,im, in sub-panel (b)) compared to the case in Figure 4(a). Moreover, we see an increase in the spatial spread of the tumour between sub-panel (a) and sub-panel (b) where there are more migrating cancer cells.
In Figure 8 we investigate the impact of the infection rates of cancer cells by the virus particles for the model (3.1) when we ignore the haptotaxis for cp (i.e., ηcp=0); we compare the results with those in Figure 7(b). In Figure 8(a) we assume that the proliferating cells have a faster infection rates compared to the migrating cells: ϱp=3ϱm=0.316. In Figure 8(b) we assume that the migrating cells have a faster infection rates compared to the proliferating cells: ϱm=3ϱp=0.316. We see that by increasing the OV infection rate for any cancer subpopulation it leads to an increase viral density, better viral spread and better killing of cancer cells. This cancer-killing effect is slightly more pronounced in sub-panels (a) where ϱp>ϱm.
In Figure 9 we investigate the impact of varying the OV replication rate for model (3.1) without cp haptotaxis (i.e., ηcp=0), and the results are compared to Figure 7(b). When (a) bp=50≥bm=40, the impact of increasing bp by a small amount is more evident on the proliferative cancer population cp and on the virus density. When (b) bp=bm8=5 and bm=40, it is clear that decreasing bp while keeping bm fixed leads to a very low OV density and a higher density of proliferative cells cp in the middle of the tumour mass compared to the case (a) when bp>bm. Compared to the results in Figure 7(b) we deduce that increasing bp leads to a reduction in cp but not cm and an increase in virus v levels, while decreasing bp leads to an increase in both cp and cm and a drastic reduction in virus v levels.
In this study we proposed a new multiscale moving boundary model that considers the local/non-local interactions between cancer cells and ECM, as well as the infections of cancer cells with oncolytic viruses (OV), all in the context of the go or grow hypothesis. This model generalises the previous studies in [34] (that focused on nonlocal multi-scale moving boundary models for oncolytic virotherapies in the context of a homogeneous cancer population) and [29,58] (that focused on local multiscale moving boundary models for oncolytic virotherapies in the context of a homogeneous cancer population). Here, we consider a heterogeneous cancer cell population formed of two sub-populations: mainly-migrating and mainly-proliferative cells.
Using this new model, we investigated not only the impact of different cell-cell and cell-ECM interaction strengths on the overall spread of cancer cells and OVs (see Figures 4-6), but also the effect of changes in the transition rates between the migrating and proliferative cells (see Figure 7), as well as the effects of varying the infection rates of different cancer cells (Figure 8), and the proliferation rates of viruses inside different cancer cells (Figure 9). First, we have seen that the magnitudes of cell-cell and cell-matrix adhesion strengths for different cancer cell phenotypes (i.e., migrating and proliferative cells), combined with their local/non-local character, influence significantly the spread of the virus through the tumour. Second, we have seen that the killing of cancer cells by the OVs is slightly more pronounced when the proliferating cells have a faster infection rate compared to the migrating cells (i.e., ϱp>ϱm). This suggests that giving the virus during a certain time interval, when the majority of cells in the solid tumour are in a proliferative phase, might eventually lead to better cancer killing. Finally, we have seen that viral replication inside proliferating cells (and viral burst size from these cells) might affect in some cases not only the density of proliferative cells but also the density of migrating cells. For example, when bp>bm, the migrating cells do not seem to be greatly impacted; however, for bp<bm, the migrating cells are impacted.
To conclude, we emphasise that the heterogeneity of solid cancers (formed of sub-populations of cells with different phenotypes; e.g., mainly-migrating and mainly-proliferative cells) might impact the success of oncolytic therapies, where the virus needs to spread throughout the tumour to be able to eliminate it. We would also like to suggest that one possible explanation for the contradictory experimental results in regard to validity of the go or grow hypothesis for various cancer cell lines (see our discussion in the Introduction) might be related to the differences in the heterogeneity of tumours for these different cell lines. However, this hypothesis will have to be tested experimentally in the future.
The first author would like to acknowledge the financial support received from the Saudi Arabian Cultural Bureau in the UK on behalf of Taibah University, Medina, Saudi Arabia.
All authors declare no conflicts of interest in this paper.
In order to carry out the macro-scale computation exclusively on the developing tumour Ω(t0), for any t0>0, we define the spatial cancer indicator function I(⋅,⋅):{1,...,M}×{1,...,M}→{0,1} given by
I(s,p):={1if(xs,xp)∈Ω(t0),0if(xs,xp)∉Ω(t0). | (A.1) |
Further, in order to identify the immediate outside nodes that are neighbours to the tumour boundary (which are needed during the computation), we define the spatially closest outside neighbour indicator functions Hx,+1(⋅,⋅), Hx,−1(⋅,⋅), Hy,+1(⋅,⋅), Hy,−1(⋅,⋅):{2,...,M−1}×{2,...,M−1}→{0,1}, defined by
Hx,±1(s,p):=|I(s,p)−I(s,p±1)|⋅I(s,p),Hy,±1(s,p):=|I(s±1,p)−I(s,p)|⋅I(s,p). | (A.2) |
These enable us to exercise the computations for the cancer cells dynamics only on the expanding cancer region Ω(t0). Indeed, these indicator functions allow us to take advantage of the zero-flux conditions at the moving boundary ∂Ω(t0) and appropriately assign density values that are required in computations (and that are dictated by the boundary conditions) at each of these closest outside points to the tumour boundary (along each spatial direction). Finally, these closest outside points to the tumour boundary are given by the union of pre-images H−1x,−1({1})∪H−1x,+1({1})∪H−1y,−1({1})∪H−1y,+1({1}).
This section explain finite difference-midpoint method used through the paper, into two subsections local flux and non-local flux to accounts for all fluxes considered in this paper.
At any spatial discretised spatial location (x1s,x2p)∈Ω(t0), and any discretised time tt:=t0+lδt, ∀l∈{0,…,[Δt/δt]}, we denote by cls,p the discretised densities at the ((x1s,x2p),tl) for each of the subpopulations c∈{cp,ip,cm,im}. Similar notations we adopt also for the discretised adhesion fluxes at ((x1s,x2p),tl), namely Alc,s,p stands for the discretised adhesion flux for each of the subpopulations c∈{cp,ip,cm,im}. In this context, using the zero-flux conditions across the tumour's moving boundary (accounted here for via the indicators in equations Eqs (A.1) and (A.2)), we are able to cary out computations on the expanding spatial mesh by involving midpoint approximations for the cancer subpopulation densities
cls,p±12:=cls,p+[Hx,±1(s,p)cls,p+I(s,p±1)cls,p±1]2,cls±12,p:=cls,p+[Hy,±1(s,p)cls,p+I(s±1,p)cls±1,p]2, | (B.1) |
as well as for the adhesion fluxes
Alc,s,p±12:=Alc,s,p+[Hx,±1(s,p)Alc,s,p+I(s,p±1)Alc,s,p±1]2,Alc,s±12,p:=Alc,s,p+[Hy,±1(s,p)Alc,s,p+I(s±1,p)Alc,s±1,p]2, | (B.2) |
while the central differences at the virtual midpoint nodes (s,p±12) and (s±12,p) are given by:
● for c:
[cx]ls,p+12:=[Hx,+1(s,p)cls,p+I(s,p+1)cls,p+1]−cls,pΔx,[cx]ls,p−12:=cls,p−[Hx,−1(s,p)cls,p+I(s,p−1)cls,p−1]Δx,[cy]ls+12,p:=[Hy,+1(s,p)cls,p+I(s+1,p)cls+1,p]−cls,pΔy,[cy]ls−12,p:=cls,p−[Hy,−1(s,p)cls,p+I(s−1,p)cls−1,p]Δy, | (B.3) |
Hence, the discretisation of the spatial operator ∇⋅[Dc∇c−cAc(t,x,u(t,⋅))] in Eq (2.25c) is obtained by
(∇⋅[Dc∇c−cA(t,x,u(t,⋅))])ls,p≈Dc([cx]ls,p+12−[cx]ls,p−12)−cls,p+12⋅Alc,s,p+12+cls,p−12⋅Alc,s,p−12Δx+Dc([cy]ls+12,p−[cy]ls−12,p)−cls+12,p⋅Alc,s+12,p+cls−12,p⋅Alc,s−12,pΔy. | (B.4) |
Denoting now by Flc,s,p the discretised value of the flux Fc:=Dc∇c−cAc(t,x,u(t,⋅)) at the spatio-temporal node ((xs,xp),tl), we observe that the discretisation of ∇⋅Fc=∇⋅[Dc∇c−cAc(t,x,u(t,⋅))] given in Eq (2.25c) can therefore be equivalently expressed in a compact form as
(∇⋅Fc)ls,p≃Flc,s,p+12−Flc,s,p−12+Flc,s+12,p+Flc,s−12,ph, | (B.5) |
where
Flc,s,p±12=Dc[cx]ls,p±12−cls,p±12⋅Alc,s,p±12,Flc,s±12,p=Dc[cy]ls±12,p−cls±12,p⋅Alc,s±12,p. |
For the case when the spatial flux is given through the local operator Fc:=DcΔc−ηc∇⋅c∇e) where c∈{cp,ip,im}, we have the discretisation
(Fc)ls,p≈Dc([cx]ls,p+12−[cx]ls,p−12)−ηccls,p+12⋅[ex]ls,p+12+ηccls,p−12⋅[ex]ls,p−12Δx+Dc([cy]ls+12,p−[cy]ls−12,p)−ηccls+12,p⋅[ey]ls+12,p+ηccls−12,p⋅[ey]ls−12,pΔy, | (B.6) |
where
cls,p±12:=cls,p+[Hx,±1(s,p)cls,p+I(s,p±1)cls,p±1]2,cls±12,p:=cls,p+[Hy,±1(s,p)cls,p+I(s±1,p)cls±1,p]2, | (B.7) |
and
[cx]ls,p+12:=[Hx,+1(s,p)cls,p+I(s,p+1)cls,p+1]−cls,pΔx,[cx]ls,p−12:=cls,p−[Hx,−1(s,p)cls,p+I(s,p−1)cls,p−1]Δx,[cy]ls+12,p:=[Hy,+1(s,p)cls,p+I(s+1,p)cls+1,p]−cls,pΔy,[cy]ls−12,p:=cls,p−[Hy,−1(s,p)cls,p+I(s−1,p)cls−1,p]Δy, | (B.8) |
and for e:
[ex]ls,p+12:=els,p+1−els,ph,and[ex]ls,p−12:=els,p−els,p−1h,[ey]ls+12,p:=els+1,p−els,ph,and[ey]ls−12,p:=els,p−els−1,ph. | (B.9) |
Finally, for the haptotactic-like virus spatial operator ηv∇⋅(v∇e) is discretised as
(−ηv∇⋅(v∇e))ls,p≈−ηvvls,p+12⋅[ex]ls,p+12+ηvvls,p−12⋅[ex]ls,p−12Δx+−ηvvls+12,p⋅[ey]ls+12,p+ηvvls−12,p⋅[ey]ls−12,pΔy, | (B.10) |
We denote by Hc(⋅,⋅,⋅) the right-hand side spatial operator for each of the tumour subpopulations Eq 2.25(a)-(d), with the non-transport part of this operator further denoted by fc(u,v). Then, maintaining the same notation style, at any discretised spatio-temporal node ((xs,xp),tl), we have that
Hc(Flc,s,p,cls,p,uls,p):=(∇⋅Fc)ls,p+fc(uls,p,vlp). | (C.1) |
On the time interval [tl,tl+1], we first predict c at tl+12 using an explicit method as follows
˜cl+12s,p=cls,p+Δt2Hc(Flc,s,p,cli,j,uls,p). | (C.2) |
Further, using ˜cl+12s,p we calculate the corresponding predicted flux ˜Fl+12c,s,p at tl+12. Then, we construct a non-local corrector that involves the average of the flux at the active neighbouring spatial locations
{(xs,xp±1)},{(xs±1,xp)},{(xs±1,xp−1)},{(xs±1,xp+1)}∩Ω(t0). | (C.3) |
Denoting the set of indices corresponding to these active locations by N, we we establish a corrector for the spatial flux, given by
F∗l+12c,s,p=1card(N)∑(σ,ζ)∈N˜Fl+12c,σ,ζ, | (C.4) |
which ultimately enables us to use a trapezoidal-type approximation and obtain a corrected value for c at tl+12 as
cl+12s,p=cls,p+Δt4[Hc(Fls,p,cli,j,uls,p)+Hc(F∗l+12s,p,˜cl+12s,p,˜ul+12s,p)], | (C.5) |
Here, ˜ul+12s,p is the "half-time predicted" tumour vector obtained for the current subpopulation c given by the half-time predicted value ˜cl+12s,p, while all the other tumour subpopulations and the ECM (that enter as components of u) being given by their accepted values at ((xs,xp),tl).
Then, using
ˉcl+12s,p:=cl+12s,p+cls,p2, | (C.6) |
to calculate the flux Fl+12c at tl+12, we initiate the predictor-corrector steps described above on the second half of this time interval, which we now repeat on [tl+12,tl+1], and we finally obtain cl+1s,p given through the analogue of Eq (C.5) that results (through the same procedure, described in Eqs (C.2)-(C.5)) on this second half-interval (i.e., on [tl+12,tl+1]. Finally, the same time-marching steps are adopted also for virus equations Eq (2.25f).
[1] | FAOSTAT (2023) Available from: https://www.fao.org/faostat/en/#data/QCL/visualizeFAOSTAT. Access date 23rd February 2023. |
[2] |
El Sheikha AF, Hu D-M (2018) How to trace the geographic origin of mushrooms? Trends Food Sci Technol 78: 292–303. https://doi.org/10.1016/j.tifs.2018.06.008 doi: 10.1016/j.tifs.2018.06.008
![]() |
[3] |
Yadav D, Negi PS (2021) Bioactive components of mushrooms: Processing effects and health benefits. Food Res Int 148: 23. https://doi.org/10.1016/j.foodres.2021.110599 doi: 10.1016/j.foodres.2021.110599
![]() |
[4] |
Ritota M, Manzi P (2019) Pleurotus spp. cultivation on different agri-food by-products: Example of biotechnological application. Sustainability 11: 5049. https://doi.org/10.3390/su11185049 doi: 10.3390/su11185049
![]() |
[5] |
Bao HN, Osako K, Ohshima T (2010) Value-added use of mushroom ergothioneine as a colour stabilizer in processed fish meats. J Sci Food Agric 90: 1634–1641. https://doi.org/10.1002/jsfa.3992 doi: 10.1002/jsfa.3992
![]() |
[6] |
Wan-Mohtar WAAQI, Halim-Lim SA, Kamarudin NZ, et al. (2020) Fruiting-body-base flour from an Oyster mushroom waste in the development of antioxidative chicken patty. J Food Sci 85: 3124–3133. https://doi.org/10.1111/1750-3841.15402 doi: 10.1111/1750-3841.15402
![]() |
[7] |
Zhang Y, Venkitasamy C, Pan Z, et al. (2013) Recent developments on umami ingredients of edible mushrooms—A review. Trends Food Sci Technol 33: 78–92. https://doi.org/10.1016/j.tifs.2013.08.002 doi: 10.1016/j.tifs.2013.08.002
![]() |
[8] |
Chowdhury MMH, Kubra K, Ahmed SR (2015) Screening of antimicrobial, antioxidant properties and bioactive compounds of some edible mushrooms cultivated in Bangladesh. Ann Clin Microbiol Antimicrob 14: 6. https://doi.org/10.1186/s12941-015-0067-3 doi: 10.1186/s12941-015-0067-3
![]() |
[9] |
Kozarski M, Klaus A, Jakovljevic D, et al. (2015) Antioxidants of edible mushrooms. Molecules 20: 19489–19525. https://doi.org/10.3390/molecules201019489 doi: 10.3390/molecules201019489
![]() |
[10] |
Chung SI, Kim SY, Nam YJ, et al. (2010) Development of surimi gel from king oyster mushroom and cuttlefish meat paste. Food Sci Biotechnol 19: 51–56. https://doi.org/10.1007/s10068-010-0007-0 doi: 10.1007/s10068-010-0007-0
![]() |
[11] |
Chuang WY, Hsieh YC, Lee TT (2020) The effects of fungal feed additives in animals: A review. Animals 10: 805. https://doi.org/10.3390/ani10050805 doi: 10.3390/ani10050805
![]() |
[12] |
Moon B, Lo YM (2014) Conventional and novel applications of edible mushrooms in today's food industry. J Food Process. Preserv 38: 2146–2153. https://doi.org/10.1111/jfpp.12185 doi: 10.1111/jfpp.12185
![]() |
[13] |
Ulziijargal E, Yang JH, Lin LY, et al. (2013) Quality of bread supplemented with mushroom mycelia. Food Chem 138: 70–76. https://doi.org/10.1016/j.foodchem.2012.10.051 doi: 10.1016/j.foodchem.2012.10.051
![]() |
[14] |
Süfer Ö, Bozok F (2021) Gluten-free tarhana fortified with different ratios of edible mushroom Lactarius deliciosus. Int Food Res J 28: 1131–1140. https://doi.org/10.47836/ifrj.28.6.04 doi: 10.47836/ifrj.28.6.04
![]() |
[15] | Park M, Lee J, Lee B (2001) Development of natural seasoning based on mushroom. J East Asian Soc Diet Life 11: 196–203. |
[16] |
Okamura-Matsui T, Tomoda T, Fukuda S, et al. (2003) Discovery of alcohol dehydrogenase from mushrooms and application to alcoholic beverages. J Mol Catal B: Enzym 23: 133–144. https://doi.org/10.1016/S1381-1177(03)00079-1 doi: 10.1016/S1381-1177(03)00079-1
![]() |
[17] |
Okamura-Matsui T, Takemura K, Sera M, et al. (2001) Characteristics of a cheese-like food produced by fermentation of the mushroom Schizophyllum commune. J Biosci Bioeng 92: 30–32. https://doi.org/10.1016/S1389-1723(01)80194-8 doi: 10.1016/S1389-1723(01)80194-8
![]() |
[18] |
Correa RCG, Brugnari T, Bracht A, et al. (2016) Biotechnological, nutritional and therapeutic uses of Pleurotus spp. (Oyster mushroom) related with its chemical composition: A review on the past decade findings. Trends Food Sci Technol 50: 103–117. https://doi.org/10.1016/j.tifs.2016.01.012 doi: 10.1016/j.tifs.2016.01.012
![]() |
[19] | Patel Y, Naraian R, Singh V (2012) Medicinal properties of Pleurotus species (oyster mushroom): A review. World J Fungal Plant Biol 3: 1–12. |
[20] |
Fernandes A, Barros L, Martins A, et al. (2015) Nutritional characterisation of Pleurotus ostreatus (Jacq. ex Fr.) P. Kumm. produced using paper scraps as substrate. Food Chem 169: 396–400. https://doi.org/10.1016/j.foodchem.2014.08.027 doi: 10.1016/j.foodchem.2014.08.027
![]() |
[21] |
Ng SH, Robert SD, Ahmad WANW, et al. (2017) Incorporation of dietary fibre-rich oyster mushroom (Pleurotus sajor-caju) powder improves postprandial glycaemic response by interfering with starch granule structure and starch digestibility of biscuit. Food Chem 227: 358–368. https://doi.org/10.1016/j.foodchem.2017.01.108 doi: 10.1016/j.foodchem.2017.01.108
![]() |
[22] |
Kumar K (2020) Nutraceutical potential and processing aspects of oyster mushrooms (Pleurotus Species). Curr Nutr Food Sci 16: 3–14. https://doi.org/10.2174/1573401314666181015111724 doi: 10.2174/1573401314666181015111724
![]() |
[23] |
Proserpio C, Pagliarini E, Laureati M, et al. (2019) Acceptance of a new food enriched in beta-glucans among adolescents: Effects of food technology neophobia and healthy food habits. Foods 8: 433. https://doi.org/10.3390/foods8100433 doi: 10.3390/foods8100433
![]() |
[24] |
Cerón-Guevara MI, Rangel-Vargas E, Lorenzo JM, et al. (2019) Effect of the addition of edible mushroom flours (Agaricus bisporus and Pleurotus ostreatus) on physicochemical and sensory properties of cold-stored beef patties. J Food Process Preserv 44: e14351. https://doi.org/10.1111/jfpp.14351 doi: 10.1111/jfpp.14351
![]() |
[25] |
Diplock AT, Action EC, et al. (1999) Scientific concepts of functional foods in Europe: Consensus document. Br J Nutr 81: S1–S27. https://doi.org/10.1017/S0007114599000471 doi: 10.1017/S0007114599000471
![]() |
[26] |
Manzi P, Gambelli L, Marconi S, et al. (1999) Nutrients in edible mushrooms: an inter-species comparative study. Food Chem 65: 477–482. https://doi.org/10.1016/S0308-8146(98)00212-X doi: 10.1016/S0308-8146(98)00212-X
![]() |
[27] |
Valencia del Toro G, Vega RC, Garín-Aguilar ME, et al. (2006) Biological quality of proteins from three strains of Pleurotus spp. Food Chem 94: 494–497. https://doi.org/10.1016/j.foodchem.2004.11.053 doi: 10.1016/j.foodchem.2004.11.053
![]() |
[28] |
Chirinang P, Intarapichet KO (2009) Amino acids and antioxidant properties of the oyster mushrooms, Pleurotus ostreatus and Pleurotus sajor-caju. Scienceasia 35: 326–331. https://doi.org/10.2306/scienceasia1513-1874.2009.35.326 doi: 10.2306/scienceasia1513-1874.2009.35.326
![]() |
[29] |
Akyüz M, İnci Ş, Kırbağ S (2021) Nutrient Content of Pleurotus pulmonarius (Fr.) Quel. Grown on Some Local Lignocellulosic Wastes. KSU J Agric Nat 25: 25–30. https://doi.org/10.18016/ksutarimdoga.vi.890663 doi: 10.18016/ksutarimdoga.vi.890663
![]() |
[30] |
Ivarsson E, Gruden M, Sodergren J, et al. (2021) Use of faba bean (Vicia faba L.) hulls as substrate for Pleurotus ostreatus—Potential for combined mushroom and feed production. J Cleaner Prod 313: 127969. https://doi.org/10.1016/j.jclepro.2021.127969 doi: 10.1016/j.jclepro.2021.127969
![]() |
[31] | Maheswari S, Rajarajan P, Pandian PM, et al. (2021) Yield performance of mushroom (Pleurotus Ostreatus) on different treatment of sugarcane bagasse and saw dust and its nutrient analysis. Plant Cell Biotechnol Mol Biol 22: 7–13. |
[32] |
Naraian R, Sahu RK, Kumar S, et al. (2008) Influence of different nitrogen rich supplements during cultivation of Pleurotus florida on corn cob substrate. Environmentalist 29: 1–7. https://doi.org/10.1007/s10669-008-9174-4 doi: 10.1007/s10669-008-9174-4
![]() |
[33] |
Lin P, Yan ZF, Kook M, et al. (2022) Genetic and chemical diversity of edible mushroom pleurotus species. BioMed Res Int 2022: 6068185. https://doi.org/10.1155/2022/6068185 doi: 10.1155/2022/6068185
![]() |
[34] |
Kayode RMO, Olakulehin TF, Adedeji BS, et al. (2015) Evaluation of amino acid and fatty acid profiles of commercially cultivated oyster mushroom (Pleurotus sajor-caju) grown on gmelina wood waste. Niger Food J 33: 18–21. https://doi.org/10.1016/j.nifoj.2015.04.001 doi: 10.1016/j.nifoj.2015.04.001
![]() |
[35] |
Bach F, Helm CV, Bellettini MB, Maciel GM, Haminiuk CWI. (2017) Edible mushrooms: A potential source of essential amino acids, glucans and minerals. Int J Food Sci Technol 52: 2382–2392. https://doi.org/10.1111/ijfs.13522 doi: 10.1111/ijfs.13522
![]() |
[36] |
Dabbour IR, Takruri HR (2002) Protein Digestibility using Corrected Amino Acid Score method (PDCAAS) of four types of mushrooms grown in Jordan. Plant Foods Hum Nutr 57: 13–24. https://doi.org/10.1023/A:1013110707567 doi: 10.1023/A:1013110707567
![]() |
[37] |
Pellegrino RM, Blasi F, Angelini P, et al. (2022) LC/MS Q-TOF metabolomic investigation of amino acids and dipeptides in Pleurotus ostreatus grown on different substrates. J Agric Food Chem 70: 10371–10382. https://doi.org/10.1021/acs.jafc.2c04197 doi: 10.1021/acs.jafc.2c04197
![]() |
[38] |
Vetter J (2019) Biological values of cultivated mushrooms—A review. Acta Aliment 48: 229–240. https://doi.org/10.1556/066.2019.48.2.11 doi: 10.1556/066.2019.48.2.11
![]() |
[39] |
Raman J, Jang KY, Oh YL, et al. (2021) Cultivation and nutritional value of prominent Pleurotus Spp.: An overview. Mycobiology 49: 1–14. https://doi.org/10.1080/12298093.2020.1835142 doi: 10.1080/12298093.2020.1835142
![]() |
[40] | Gupta S, Summuna B, Gupta M, et al. (2018) Edible mushrooms: Cultivation, bioactive molecules, and health benefits. In: Mérillon JM, Ramawat K (Eds), Bioactive Molecules in Food. Reference Series in Phytochemistry. Springer, Cham 1: 1–33. https://doi.org/10.1007/978-3-319-54528-8_86-1 |
[41] |
Lavelli V, Proserpio C, Gallotti F, et al. (2018) Circular reuse of bio-resources: the role of Pleurotus spp. in the development of functional foods. Food Funct 9: 1353–1372. https://doi.org/10.1039/C7FO01747B doi: 10.1039/C7FO01747B
![]() |
[42] |
Sande D, de Oliveira GP, Moura MAFE, et al. (2019) Edible mushrooms as a ubiquitous source of essential fatty acids. Food Res Int 125: 108524. https://doi.org/10.1016/j.foodres.2019.108524 doi: 10.1016/j.foodres.2019.108524
![]() |
[43] |
Sales-Campos H, Reis de Souza P, Crema Peghini B, et al. (2013) An overview of the modulatory effects of oleic acid in health and disease. Mini-Rev Med Chem 13: 201–210. https://doi.org/10.2174/138955713804805193 doi: 10.2174/138955713804805193
![]() |
[44] |
Gunc Ergonul P, Akata I, Kalyoncu F, et al. (2013) Fatty acid compositions of six wild edible mushroom species. Sci World J 2013: 163964. https://doi.org/10.1155/2013/163964 doi: 10.1155/2013/163964
![]() |
[45] |
Saini RK, Rauf A, Khalil AA, et al. (2021) Edible mushrooms show significant differences in sterols and fatty acid compositions. S Afr J Bot 141: 344–356. https://doi.org/10.1016/j.sajb.2021.05.022 doi: 10.1016/j.sajb.2021.05.022
![]() |
[46] |
Nieto IJ, CHEGWIN A C (2008) Triterpenoids and fatty acids identified in the edible mushroom Pleurotus sajor-caju. J Chil Chem Soc 53: 1515–1517. https://doi.org/10.4067/S0717-97072008000200015 doi: 10.4067/S0717-97072008000200015
![]() |
[47] |
Pedneault K, Angers P, Avis TJ, et al. (2007) Fatty acid profiles of polar and non-polar lipids of Pleurotus ostreatus and P. cornucopiae var. 'citrino-pileatus' grown at different temperatures. Mycol Res 111: 1228–1234. https://doi.org/10.1016/j.mycres.2007.06.014 doi: 10.1016/j.mycres.2007.06.014
![]() |
[48] |
Taofiq O, Fernandes A, Barros L, et al. (2017) UV-irradiated mushrooms as a source of vitamin D-2: A review. Trends Food Sci Technol 70: 82–94. https://doi.org/10.1016/j.tifs.2017.10.008 doi: 10.1016/j.tifs.2017.10.008
![]() |
[49] |
Jiang QY, Zhang M, Mujumdar AS (2020) UV induced conversion during drying of ergosterol to vitamin D in various mushrooms: Effect of different drying conditions. Trends Food Sci Technol 105: 200–210. https://doi.org/10.1016/j.tifs.2020.09.011 doi: 10.1016/j.tifs.2020.09.011
![]() |
[50] |
Jasinghe VJ, Perera CO, Sablani SS (2007) Kinetics of the conversion of ergosterol in edible mushrooms. J Food Eng 79: 864–869. https://doi.org/10.1016/j.jfoodeng.2006.01.085 doi: 10.1016/j.jfoodeng.2006.01.085
![]() |
[51] |
Jasinghe VJ, Perera CO (2005) Distribution of ergosterol in different tissues of mushrooms and its effect on the conversion of ergosterol to vitamin D2 by UV irradiation. Food Chem 92: 541–546. https://doi.org/10.1016/j.foodchem.2004.08.022 doi: 10.1016/j.foodchem.2004.08.022
![]() |
[52] |
Jasinghe VJ, Perera CO (2006) Ultraviolet irradiation: The generator of Vitamin D2 in edible mushrooms. Food Chem 95: 638–643. https://doi.org/10.1016/j.foodchem.2005.01.046 doi: 10.1016/j.foodchem.2005.01.046
![]() |
[53] |
Kortei NK, Odamtten GT, Obodai M, et al. (2017) Influence of low dose of gamma radiation and storage on some vitamins and mineral elements of dried oyster mushrooms (Pleurotus ostreatus). Food Sci Nutr 5: 570–578. https://doi.org/10.1002/fsn3.432 doi: 10.1002/fsn3.432
![]() |
[54] | Agarwal S, Kushwaha A, Verma V, et al. (2017) Nutritional attributes of Pleurotus mushroom. In: Singh MP, Verma V (Eds.), Incredible World of Biotechnology, Nova Science Publishers, 13–24. |
[55] |
Lesa KN, Khandaker MU, Iqbal FMR, et al. (2022) Nutritional value, medicinal importance, and health-promoting effects of dietary mushroom (Pleurotus ostreatus). J Food Qual 2022: 2454180. https://doi.org/10.1155/2022/2454180 doi: 10.1155/2022/2454180
![]() |
[56] |
Zhou S, Ma F, Zhang X, et al. (2016) Carbohydrate changes during growth and fruiting in Pleurotus ostreatus. Fungal Biol 120: 852–861. https://doi.org/10.1016/j.funbio.2016.03.007 doi: 10.1016/j.funbio.2016.03.007
![]() |
[57] | Deepalakshmi K, Mirunalini S (2014) Pleurotus ostreatus: an oyster mushroom with nutritional and medicinal properties. J Biochem Technol 5: 718–726. |
[58] |
Liu X, WU X, GAO W, et al. (2019) Protective roles of trehalose in Pleurotus pulmonarius during heat stress response. J Integr Agric 18: 428–437. https://doi.org/10.1016/S2095-3119(18)62010-6 doi: 10.1016/S2095-3119(18)62010-6
![]() |
[59] |
Yan ZY, Zhao MR, Huang CY, et al. (2021) Trehalose alleviates high-temperature stress in Pleurotus ostreatus by affecting central carbon metabolism. Microbial Cell Factories 20: 1–11. https://doi.org/10.1186/s12934-021-01572-9 doi: 10.1186/s12934-021-01572-9
![]() |
[60] |
Cheung PCK (2013) Mini-review on edible mushrooms as source of dietary fiber: Preparation and health benefits. Food Sci Hum Wellness 2: 162–166. https://doi.org/10.1016/j.fshw.2013.08.001 doi: 10.1016/j.fshw.2013.08.001
![]() |
[61] |
Manzi P, Aguzzi A, Pizzoferrato L (2001) Nutritional value of mushrooms widely consumed in Italy. Food Chem 73: 321–325. https://doi.org/10.1016/S0308-8146(00)00304-6 doi: 10.1016/S0308-8146(00)00304-6
![]() |
[62] |
Manzi P, Marconi S, Aguzzi A, et al. (2004) Commercial mushrooms: nutritional quality and effect of cooking. Food Chem 84: 201–206. https://doi.org/10.1016/S0308-8146(03)00202-4 doi: 10.1016/S0308-8146(03)00202-4
![]() |
[63] |
Nitschke J, Altenbach H, Malolepszy T, et al. (2011) A new method for the quantification of chitin and chitosan in edible mushrooms. Carbohydr Res 346: 1307–1310. https://doi.org/10.1016/j.carres.2011.03.040 doi: 10.1016/j.carres.2011.03.040
![]() |
[64] |
Vetter J (2007) Chitin content of cultivated mushrooms Agaricus bisporus, Pleurotus ostreatus and Lentinula edodes. Food Chem 102: 6–9. https://doi.org/10.1016/j.foodchem.2006.01.037 doi: 10.1016/j.foodchem.2006.01.037
![]() |
[65] |
Morin-Crini N, Lichtfouse E, Torri G, et al. (2019) Applications of chitosan in food, pharmaceuticals, medicine, cosmetics, agriculture, textiles, pulp and paper, biotechnology, and environmental chemistry. Environ Chem Lett 17: 1667–1692. https://doi.org/10.1007/s10311-019-00904-x doi: 10.1007/s10311-019-00904-x
![]() |
[66] |
Khan AA, Gani A, Khanday F, et al. (2018) Biological and pharmaceutical activities of mushroom β-glucan discussed as a potential functional food ingredient. Bioact Carbohydr Diet Fibre 16: 1–13. https://doi.org/10.1016/j.bcdf.2017.12.002 doi: 10.1016/j.bcdf.2017.12.002
![]() |
[67] |
Rop O, Mlcek J, Jurikova T (2009) Beta-glucans in higher fungi and their health effects. Nutrition Rev 67: 624–631. https://doi.org/10.1111/j.1753-4887.2009.00230.x doi: 10.1111/j.1753-4887.2009.00230.x
![]() |
[68] | Sobieralski K, Siwulski M, Lisiecka J, et al. (2012) Fungi-derived β-Glucans as a component of functional food. Acta Sci Pol, Hortorum Cultus 11: 111–128. |
[69] |
Manzi P, Pizzoferrato L (2000) Beta-glucans in edible mushrooms. Food Chem 68: 315–318. https://doi.org/10.1016/S0308-8146(99)00197-1 doi: 10.1016/S0308-8146(99)00197-1
![]() |
[70] |
Ruthes AC, Cantu-Jungles TM, Cordeiro LMC, et al. (2021) Prebiotic potential of mushroom D-glucans: implications of physicochemical properties and structural features. Carbohydrate Polymers 262: 117940. https://doi.org/10.1016/j.carbpol.2021.117940 doi: 10.1016/j.carbpol.2021.117940
![]() |
[71] |
Karácsonyi Š, Kuniak Ľ (1994) Polysaccharides of Pleurotus ostreatus: Isolation and structure of pleuran, an alkali-insoluble β-D-glucan. Carbohydr Polym 24: 107–111. https://doi.org/10.1016/0144-8617(94)90019-1 doi: 10.1016/0144-8617(94)90019-1
![]() |
[72] |
Murphy EJ, Rezoagli E, Major I, et al. (2020) β-glucan metabolic and immunomodulatory properties and potential for clinical application. J Fungi 6: 356. https://doi.org/10.3390/jof6040356 doi: 10.3390/jof6040356
![]() |
[73] |
Urbancikova I, Hudackova D, Majtan J, et al. (2020) Efficacy of pleuran (beta-glucan from Pleurotus ostreatus) in the management of herpes simplex virus type 1 infection. Evidence-Based Complementary Altern Med 2020: 8562309. https://doi.org/10.1155/2020/8562309 doi: 10.1155/2020/8562309
![]() |
[74] |
Rennerova Z, Sirvent LP, Roca EC, et al. (2022) Beta-(1, 3/1, 6)-D-glucan from Pleurotus ostreatus in the prevention of recurrent respiratory tract infections: An international, multicentre, open-label, prospective study. Front Pediatr 2022: 1833. https://doi.org/10.3389/fped.2022.999701 doi: 10.3389/fped.2022.999701
![]() |
[75] |
Vlassopoulou M, Yannakoulia M, Pletsa V, et al. (2021) Effects of fungal beta-glucans on health—a systematic review of randomized controlled trials. Food Funct 12: 3366–3380. https://doi.org/10.1039/D1FO00122A doi: 10.1039/D1FO00122A
![]() |
[76] |
Mkhize SS, Cedric Simelane MB, Mongalo IN, et al. (2022) The Effect of supplementing mushroom growing substrates on the bioactive compounds, antimicrobial activity, and antioxidant activity of Pleurotus ostreatus. Biochem Res Int 2022: 9436614. https://doi.org/10.1155/2022/9436614 doi: 10.1155/2022/9436614
![]() |
[77] |
Valverde ME, Hernandez-Perez T, Paredes-Lopez O (2015) Edible mushrooms: Improving human health and promoting quality life. Int J Microbiol 2015: 376387–376387. https://doi.org/10.1155/2015/376387 doi: 10.1155/2015/376387
![]() |
[78] |
Izham I, Avin F, Raseetha S (2022) Systematic review: Heat treatments on phenolic content, antioxidant activity, and sensory quality of Malaysian mushroom: Oyster (Pleurotus spp.) and black jelly (Auricularia spp.). Front Sustain Food Syst 6: 16. https://doi.org/10.3389/fsufs.2022.882939 doi: 10.3389/fsufs.2022.882939
![]() |
[79] |
Gąsecka M, Mleczek M, Siwulski M, et al. (2015) Phenolic composition and antioxidant properties of Pleurotus ostreatus and Pleurotus eryngii enriched with selenium and zinc. Eur Food Res Technol 242: 723–732. https://doi.org/10.1007/s00217-015-2580-1 doi: 10.1007/s00217-015-2580-1
![]() |
[80] | Bruno GL, Lafortezza MA, Tommasi F (2020) Il Cardoncello, Pleurotus eryngii (DC.) Quél., una risorsa del territorio: caratterizzazione di ceppi pugliesi tra fisiologia e nutraceutica. Notiziario della Società Botanica Italiana 4: 1–4. |
[81] |
Mutukwa IB, Hall III CA, Cihacek L, et al. (2019) Evaluation of drying method and pretreatment effects on the nutritional and antioxidant properties of oyster mushroom (Pleurotus ostreatus). J Food Process Preserv 43: e13910. https://doi.org/10.1111/jfpp.13910 doi: 10.1111/jfpp.13910
![]() |
[82] |
Tepsongkroh B, Jangchud K, Trakoontivakorn G (2019) Antioxidant properties and selected phenolic acids of five different tray-dried and freeze-dried mushrooms using methanol and hot water extraction. J Food Meas Charact 13: 3097–3105. https://doi.org/10.1007/s11694-019-00232-2 doi: 10.1007/s11694-019-00232-2
![]() |
[83] | OECD (2006) Section 11—Oyster Mushroom (PLEUROTUS SPP.) in Safety Assessment of Transgenic Organisms. In. OECD Publishing, Paris. |
[84] |
Bellettini MB, Fiorda FA, Maieves HA, et al. (2019) Factors affecting mushroom Pleurotus spp. Saudi J Biol Sci 26: 633–646. https://doi.org/10.1016/j.sjbs.2016.12.005 doi: 10.1016/j.sjbs.2016.12.005
![]() |
[85] |
Santos MV, Lespinard AR (2011) Numerical simulation of mushrooms during freezing using the FEM and an enthalpy: Kirchhoff formulation. Heat Mass Transfer 47: 1671–1683. https://doi.org/10.1007/s00231-011-0831-7 doi: 10.1007/s00231-011-0831-7
![]() |
[86] |
Demiray E (2019) Effect of drying temperature on color and desorption characteristics of oyster mushroom. Food Sci Technol 40: 187–193. https://doi.org/10.1590/fst.37118 doi: 10.1590/fst.37118
![]() |
[87] |
El-Ramady H, Abdalla N, Badgar K, et al. (2022) Edible mushrooms for sustainable and healthy human food: Nutritional and medicinal attributes. Sustainability 14: 30. https://doi.org/10.3390/su14094941 doi: 10.3390/su14094941
![]() |
[88] |
Michael HW, Bultosa G, Pant LM (2011) Nutritional contents of three edible oyster mushrooms grown on two substrates at Haramaya, Ethiopia, and sensory properties of boiled mushroom and mushroom sauce. Int J Food Sci Technol 46: 732–738. https://doi.org/10.1111/j.1365-2621.2010.02543.x doi: 10.1111/j.1365-2621.2010.02543.x
![]() |
[89] |
Jacinto-Azevedo B, Valderrama N, Henriquez K, et al. (2021) Nutritional value and biological properties of Chilean wild and commercial edible mushrooms. Food Chem 356: 8. https://doi.org/10.1016/j.foodchem.2021.129651 doi: 10.1016/j.foodchem.2021.129651
![]() |
[90] |
Czapski J, Szudyga K (2000) Frozen mushrooms quality as affected by strain, flush, treatment before freezing, and time of storage. J Food Sci 65: 722–725. https://doi.org/10.1111/j.1365-2621.2000.tb16079.x doi: 10.1111/j.1365-2621.2000.tb16079.x
![]() |
[91] |
Marcal S, Sousa AS, Taofiq O, et al. (2021) Impact of postharvest preservation methods on nutritional value and bioactive properties of mushrooms. Trends Food Sci Technol 110: 418–431. https://doi.org/10.1016/j.tifs.2021.02.007 doi: 10.1016/j.tifs.2021.02.007
![]() |
[92] | Wan Rosli WI, Nurhanan AR, Aishah MS (2012) Effect of partial replacement of wheat flour with oyster mushroom (Pleurotus sajor-caju) powder on nutritional composition and sensory properties of butter biscuit. Sains Malays 41: 1565–1570. |
[93] |
Demiray E, Çalışkan Koç G (2021) Effect of vacuum drying temperature and pressure on the drying and desorption characteristics, and lovastatin content of the oyster mushroom (Pleurotus ostreatus) slices. J Food Process Preserv 45: e15301. https://doi.org/10.1111/jfpp.15301 doi: 10.1111/jfpp.15301
![]() |
[94] |
Jirasatid S, Nopharatana M, Kitsubun P, et al. (2013) Degradation kinetics of monacolin K in red yeast rice powder using multiresponse modeling approach. J Food Eng 116: 436–443. https://doi.org/10.1016/j.jfoodeng.2012.12.018 doi: 10.1016/j.jfoodeng.2012.12.018
![]() |
[95] | Aishah MS, Wan Rosli WI (2013) Effect of different drying techniques on the nutritional values of oyster mushroom (Pleurotus sajor-caju). Sains Malays 42: 937–941. |
[96] |
Çelebi Sezer Y, Süfer Ö, Sezer G (2017) Extraction of phenolic compounds from oven and microwave dried mushrooms (Agaricus bisporus and Pleurotus ostreatus) by using methanol, ethanol and aceton as solvents. Indian J Pharm Educ Res 51: 393–397. https://doi.org/10.5530/ijper.51.3s.55 doi: 10.5530/ijper.51.3s.55
![]() |
[97] |
Villaescusa R, Gil MI (2003) Quality improvement of Pleurotus mushrooms by modified atmosphere packaging and moisture absorbers. Postharv Biol Technol 28: 169–179. https://doi.org/10.1016/S0925-5214(02)00140-0 doi: 10.1016/S0925-5214(02)00140-0
![]() |
[98] |
Lyn HF, Maryam Adilah ZA, Nor-Khaizura MAR, et al. (2020) Application of modified atmosphere and active packaging for oyster mushroom (Pleurotus ostreatus). Food Packag Shelf Life 23: 100451. https://doi.org/10.1016/j.fpsl.2019.100451 doi: 10.1016/j.fpsl.2019.100451
![]() |
[99] | Ng SH, Ahmad WANW, Ishak WRW (2016) Quality characteristics of Pleurotus sajor-caju powder: Study on nutritional compositions, functional properties and storage stability. Sains Malays 45: 1617–1623. |
[100] |
Grigelmo-Miguel N, Martı́n-Belloso O (1999) Comparison of dietary fibre from by-products of processing fruits and greens and from cereals. LWT-Food Sci Technol 32: 503–508. https://doi.org/10.1006/fstl.1999.0587 doi: 10.1006/fstl.1999.0587
![]() |
[101] |
Lan G, Chen H, Chen S, et al. (2012) Chemical composition and physicochemical properties of dietary fiber from Polygonatum odoratum as affected by different processing methods. Food Res Int 49: 406–410. https://doi.org/10.1016/j.foodres.2012.07.047 doi: 10.1016/j.foodres.2012.07.047
![]() |
[102] |
Raghavendra S, Ramachandra Swamy SR, Rastogi nk, et al. (2006) Grinding characteristics and hydration properties of coconut residue: A source of dietary fiber. J Food Eng 72: 281–286. https://doi.org/10.1016/j.jfoodeng.2004.12.008 doi: 10.1016/j.jfoodeng.2004.12.008
![]() |
[103] |
Viuda-Martos M, Ruiz-Navajas Y, Martin-Sánchez A, et al. (2012) Chemical, physico-chemical and functional properties of pomegranate (Punica granatum L.) bagasses powder co-product. J Food Eng 110: 220–224. https://doi.org/10.1016/j.jfoodeng.2011.05.029 doi: 10.1016/j.jfoodeng.2011.05.029
![]() |
[104] |
Fasolin LH, Pereira RN, Pinheiro AC, et al. (2019) Emergent food proteins—Towards sustainability, health and innovation. Food Res Int 125: 108586. https://doi.org/10.1016/j.foodres.2019.108586 doi: 10.1016/j.foodres.2019.108586
![]() |
[105] |
Cocking C, Walton J, Kehoe L, et al. (2020) The role of meat in the European diet: current state of knowledge on dietary recommendations, intakes and contribution to energy and nutrient intakes and status. Nutr Res Rev 33: 181–189. https://doi.org/10.1017/S0954422419000295 doi: 10.1017/S0954422419000295
![]() |
[106] |
Pintado T, Delgado-Pando G (2020) Towards more sustainable meat products: Extenders as a way of reducing meat content. Foods 9: 1044. https://doi.org/10.1017/S0954422419000295 doi: 10.1017/S0954422419000295
![]() |
[107] |
Moon B, Lo YM (2014) Conventional and novel applications of edible mushrooms in today's food industry. J Food Process Preserv 38: 2146–2153. https://doi.org/10.1111/jfpp.12185 doi: 10.1111/jfpp.12185
![]() |
[108] |
Phat C, Moon B, Lee C (2016) Evaluation of umami taste in mushroom extracts by chemical analysis, sensory evaluation, and an electronic tongue system. Food Chem 192: 1068–1077. https://doi.org/10.1016/j.foodchem.2015.07.113 doi: 10.1016/j.foodchem.2015.07.113
![]() |
[109] |
Pateiro M, Munekata PES, Cittadini A, et al. (2021) Metallic-based salt substitutes to reduce sodium. Curr Opin Food Sci 38: 21–31. https://doi.org/10.1016/j.cofs.2020.10.029 doi: 10.1016/j.cofs.2020.10.029
![]() |
[110] |
Ceron-Guevara MI, Rangel-Vargas E, Lorenzo JM, et al. (2020) Reduction of salt and fat in Frankfurter sausages by addition of Agaricus bisporus and Pleurotus ostreatus flour. Foods 9: 760. https://doi.org/10.3390/foods9060760 doi: 10.3390/foods9060760
![]() |
[111] |
Cerón-Guevara MI, Santos EM, Lorenzo JM, et al. (2021) Partial replacement of fat and salt in liver pâ té by addition of Agaricus bisporus and Pleurotus ostreatus flour. Int J Food Sci Technol 56: 6171–6181. https://doi.org/10.1111/ijfs.15076 doi: 10.1111/ijfs.15076
![]() |
[112] |
Mau JL, Lin YP, Chen PT, et al. (1998) Flavor compounds in king oyster mushrooms Pleurotus eryngii. J Agric Food Chem 46: 4587–4591. https://doi.org/10.1021/jf980508+ doi: 10.1021/jf980508+
![]() |
[113] |
Cho IH, Choi HK, Kim YS (2010) Comparison of umami-taste active components in the pileus and stipe of pine-mushrooms (Tricholoma matsutake Sing.) of different grades. Food Chem 118: 804–807. https://doi.org/10.1016/j.foodchem.2009.05.084 doi: 10.1016/j.foodchem.2009.05.084
![]() |
[114] |
Tsai SY, Wu TP, Huang SJ, et al. (2007) Nonvolatile taste components of Agaricus bisporus harvested at different stages of maturity. Food Chem 103: 1457–1464. https://doi.org/10.1016/j.foodchem.2006.10.073 doi: 10.1016/j.foodchem.2006.10.073
![]() |
[115] |
Mau JL, Chyau CC, Li JY, et al. (1997) Flavor compounds in straw mushrooms Volvariella volvacea harvested at different stages of maturity. J Agric Food Chem 45: 4726–4729. https://doi.org/10.1021/jf9703314 doi: 10.1021/jf9703314
![]() |
[116] |
Tseng YH, Mau JL (1999) Contents of sugars, free amino acids and free 5'-nucleotides in mushrooms, Agaricus bisporus, during post-harvest storage. J Sci Food Agric79: 1519–1523. https://doi.org/10.1002/(SICI)1097-0010(199908)79:11%3C1519::AID-JSFA399%3E3.0.CO;2-M doi: 10.1002/(SICI)1097-0010(199908)79:11%3C1519::AID-JSFA399%3E3.0.CO;2-M
![]() |
[117] |
Sun L, Zhang Z, Xin G, et al. (2020) Advances in umami taste and aroma of edible mushrooms. Trends Food Sci Technol 96: 176–187. https://doi.org/10.1016/j.tifs.2019.12.018 doi: 10.1016/j.tifs.2019.12.018
![]() |
[118] | Wan Rosli WI, Solihah MA, Aishah M, et al. (2011) Colour, textural properties, cooking characteristics and fibre content of chicken patty added with oyster mushroom (Pleurotus sajor-caju). Int Food Res J 18: 621–627. |
[119] |
Gaglio R, Guarcello R, Venturella G, et al. (2019) Microbiological, chemical and sensory aspects of bread supplemented with different percentages of the culinary mushroom Pleurotus eryngii in powder form. Int J Food Sci Technol 54: 1197–1205. https://doi.org/10.1111/ijfs.13997 doi: 10.1111/ijfs.13997
![]() |
[120] |
Lang M (2020) Consumer acceptance of blending plant-based ingredients into traditional meat-based foods: Evidence from the meat-mushroom blend. Food Qual Preference 79: 103758. https://doi.org/10.1016/j.foodqual.2019.103758 doi: 10.1016/j.foodqual.2019.103758
![]() |
[121] |
Qing Z, Cheng J, Wang X, et al. (2021) The effects of four edible mushrooms (Volvariella volvacea, Hypsizygus marmoreus, Pleurotus ostreatus and Agaricus bisporus) on physicochemical properties of beef paste. LWT-Food Sci Technol 135: 110063. https://doi.org/10.1016/j.lwt.2020.110063 doi: 10.1016/j.lwt.2020.110063
![]() |
[122] |
Wu X, Wang P, Xu Q, et al. (2022) Effects of Pleurotus ostreatus on physicochemical properties and residual nitrite of the pork sausage. Coatings 12: 484. https://doi.org/10.3390/coatings12040484 doi: 10.3390/coatings12040484
![]() |
[123] | Wan Rosli WI, Solihah MA, Mohsin SSJ (2011) On the ability of oyster mushroom (Pleurotus sajor-caju) confering changes in proximate composition and sensory evaluation of chicken patty. Int Food Res J 18: 1463–1469. |
[124] |
Süfer Ö, Bozok F, Demir H (2016) Usage of edible mushrooms in various food products. Turk J Agric-Food Sci Technol 4: 144–149. https://doi.org/10.24925/turjaf.v4i3.144-149.599 doi: 10.24925/turjaf.v4i3.144-149.599
![]() |
[125] | Wan Rosli WI, Solihah MA (2012) Effect on the addition of Pleurotus sajor-caju (PSC) on physical and sensorial properties of beef patty. Int Food Res J 19: 993–999. |
[126] | Wan Rosli WI, Solihah MA (2014) Nutritive qualities of patties prepared with mixture of meat and oyster mushroom. Int Food Res J 21: 2001–2006. |
[127] | Wan Rosli WI, Nor Maihiza MS, Raushan M (2015) The ability of oyster mushroom in improving nutritional composition, β-glucan and textural properties of chicken frankfurter. Int Food Res J 22: 311–317. |
[128] |
Ozunlu O, Ergezer H (2021) Possibilities of using dried oyster mushroom (Pleurotus ostreatus) in the production of beef salami. J Food Process Preserv 45: e15117. https://doi.org/10.1111/jfpp.15117 doi: 10.1111/jfpp.15117
![]() |
[129] |
Zhang N, Chen H, Zhang Y, et al. (2015) Chemical composition and antioxidant properties of five edible Hymenomycetes mushrooms. Int J Food Sci Technol 50: 465–471. https://doi.org/10.1111/ijfs.12642 doi: 10.1111/ijfs.12642
![]() |
[130] |
Pachekrepapol U, Thangrattana M, Kitikangsadan A (2022) Impact of oyster mushroom (Pleurotus ostreatus) on chemical, physical, microbiological and sensory characteristics of fish burger prepared from salmon and striped catfish filleting by-product. Int J Gastronomy Food Sci 30: 100598. https://doi.org/10.1016/j.ijgfs.2022.100598 doi: 10.1016/j.ijgfs.2022.100598
![]() |
[131] |
Wang LY, Guo H, Liu X, et al. (2019) Roles of Lentinula edodes as the pork lean meat replacer in production of the sausage. Meat Sci 156: 44–51. https://doi.org/10.1016/j.meatsci.2019.05.016 doi: 10.1016/j.meatsci.2019.05.016
![]() |
[132] |
Lu F, Luo L, Li X, et al. (2014) Effect of edible gums on the qualities of sausage of Pleurotus eryngii. Adv J Food Sci Technol 6: 973–980. https://doi.org/10.19026/ajfst.6.142 doi: 10.19026/ajfst.6.142
![]() |
[133] |
Arora B, Kamal S, Sharma VP (2017) Effect of binding agents on quality characteristics of mushroom based sausage analogue. J Food Process Preserv 41: e13134. https://doi.org/10.1111/jfpp.13134 doi: 10.1111/jfpp.13134
![]() |
[134] |
Lu F, Chen Y, He C, et al. (2014) Sensory evaluation and textural properties of mushroom sausages. Adv J Food Sci Technol 6: 792–796. https://doi.org/10.19026/ajfst.6.112 doi: 10.19026/ajfst.6.112
![]() |
[135] |
FU HY, SHIEH DE, HO CT (2002) Antioxidant and free radical scavenging activities of edible mushrooms. J Food Lipids 9: 35–43. https://doi.org/10.1111/j.1745-4522.2002.tb00206.x doi: 10.1111/j.1745-4522.2002.tb00206.x
![]() |
[136] |
Franzoni F, Colognato R, Galetta F, et al. (2006) An in vitro study on the free radical scavenging capacity of ergothioneine: comparison with reduced glutathione, uric acid and trolox. Biomed Pharmacother 60: 453–457. https://doi.org/10.1016/j.biopha.2006.07.015 doi: 10.1016/j.biopha.2006.07.015
![]() |
[137] |
Akinwande BA, Abegunde AO (2013) Preliminary investigation on use of mushroom to replace beef and turkey for pepper soup. Food Environ 1: 233–239. https://doi.org/10.2495/FENV130211 doi: 10.2495/FENV130211
![]() |
[138] |
Dominguez R, Pateiro M, Munekata PES, et al. (2017) Influence of partial pork backfat replacement by fish oil on nutritional and technological properties of liver pate. Eur J Lipid Sci Technol 119: 1600178. https://doi.org/10.1002/ejlt.201600178 doi: 10.1002/ejlt.201600178
![]() |
[139] |
Martin-Sanchez AM, Ciro-Gomez G, Vilella-Espla J, et al. (2017) Physicochemical and sensory characteristics of spreadable liver pates with annatto extract (Bixa orellana L.) and date palm co-products (Phoenix dactylifera L.). Foods 6: 94. https://doi.org/10.3390/foods6110094 doi: 10.3390/foods6110094
![]() |
[140] |
Rangel-Vargas E, Rodriguez JA, Domínguez R, et al. (2021) Edible mushrooms as a natural source of food ingredient/additive replacer. Foods 10: 2687. https://doi.org/10.3390/foods10112687 doi: 10.3390/foods10112687
![]() |
[141] |
Carrasco-Gonzalez JA, Serna-Saldivar SO, Gutierrez-Uribe JA (2017) Nutritional composition and nutraceutical properties of the Pleurotus fruiting bodies: Potencial use as food ingredient. J Food Compos Anal 58: 69–81. https://doi.org/10.1016/j.jfca.2017.01.016 doi: 10.1016/j.jfca.2017.01.016
![]() |
[142] |
Aida F, Shuhaimi M, Yazid M, et al. (2009) Mushroom as a potential source of prebiotics: A review. Trends Food Sci Technol 20: 567–575. https://doi.org/10.1016/j.tifs.2009.07.007 doi: 10.1016/j.tifs.2009.07.007
![]() |
[143] |
Synytsya A, Míčková K, Synytsya A, et al. (2009) Glucans from fruit bodies of cultivated mushrooms Pleurotus ostreatus and Pleurotus eryngii: Structure and potential prebiotic activity. Carbohydr Polym 76: 548–556. https://doi.org/10.1016/j.carbpol.2008.11.021 doi: 10.1016/j.carbpol.2008.11.021
![]() |
[144] |
Pelaes Vital AC, Goto PA, Hanai LN, et al. (2015) Microbiological, functional and rheological properties of low fat yogurt supplemented with Pleurotus ostreatus aqueous extract. LWT-Food Sci Technol 64: 1028–1035. https://doi.org/10.1016/j.lwt.2015.07.003 doi: 10.1016/j.lwt.2015.07.003
![]() |
[145] |
Kondyli E, Pappa EC, Kremmyda A, et al. (2020) Manufacture of reduced fat white-brined cheese with the addition of β-glucans biobased polysaccharides as textural properties improvements. Polymers 12: 2647. https://doi.org/10.3390/polym12112647 doi: 10.3390/polym12112647
![]() |
[146] |
Kondyli E, Pappa EC, Arapoglou D, et al. (2022) Effect of fortification with mushroom polysaccharide β-glucan on the quality of ovine soft spreadable cheese. Foods 11: 417. https://doi.org/10.3390/foods11030417 doi: 10.3390/foods11030417
![]() |
[147] |
Khorshidian N, Yousefi M, Shadnoush M, et al. (2018) An overview of β-glucan functionality in dairy products. Curr Nutr Food Sci 14: 280–292. https://doi.org/10.2174/1573401313666170609092748 doi: 10.2174/1573401313666170609092748
![]() |
[148] |
Bouzgarrou C, Amara K, Reis FS, et al. (2018) Incorporation of tocopherol-rich extracts from mushroom mycelia into yogurt. Food Funct 9: 3166–3172. https://doi.org/10.1039/C8FO00482J doi: 10.1039/C8FO00482J
![]() |
[149] |
Salehi F (2019) Characterization of different mushrooms powder and its application in bakery products: A review. Int J Food Prop 22: 1375–1385. https://doi.org/10.1080/10942912.2019.1650765 doi: 10.1080/10942912.2019.1650765
![]() |
[150] |
Okafor JNC, Okafor GI, Ozumba AU, et al. (2011) Quality characteristics of bread made from wheat and Nigerian Oyster mushroom (Pleurotus plumonarius) powder. Pak J Nutr 11: 5–10. https://doi.org/10.3923/pjn.2012.5.10 doi: 10.3923/pjn.2012.5.10
![]() |
[151] |
Prasad AS (2009) Zinc: Role in immunity, oxidative stress and chronic inflammation. Curr Opin Clin Nutr Metab Care 12: 646–652. https://doi.org/10.1097/MCO.0b013e3283312956 doi: 10.1097/MCO.0b013e3283312956
![]() |
[152] |
Siyame P, Kassim N, Makule E (2021) Effectiveness and suitability of oyster mushroom in improving the nutritional value of maize flour used in complementary foods. Int J Food Sci 2021: 8863776. https://doi.org/10.1155/2021/8863776 doi: 10.1155/2021/8863776
![]() |
[153] |
Bamidele OP, Fasogbon BM (2020) Nutritional and functional properties of maize-oyster mushroom (Zea mays-Pleurotus ostreatus) based composite flour and its storage stability. Open Agric 5: 40–49. https://doi.org/10.1515/opag-2020-0007 doi: 10.1515/opag-2020-0007
![]() |
[154] |
Süfer Ö (2022) Gluten-free traditional Turkish noodle with Macrolepiota procera mushroom: Functional, textural, thermal and sensory characteristics. Cereal Chem 99: 1074–1085. https://doi.org/10.1002/cche.10581 doi: 10.1002/cche.10581
![]() |
[155] | Report A (2001) The Definition of Dietary Fiber. In: Directors RotDFDCttBo, Chemists otAAOC (Eds.). |
[156] | Ng SH, Nizam WAWA, Rosli WWI (2017) Incorporation of Pleurotus sajor-caju powder in cinnamon biscuit: study on nutritional, physical, colour and sensorial properties. Int Food Res J 24: 2442–2450. |
[157] |
Pai S, Ghugre P, Udipi S (2005) Satiety from rice-based, wheat-based and rice–pulse combination preparations. Appetite 44: 263–271. https://doi.org/10.1016/j.appet.2005.01.004 doi: 10.1016/j.appet.2005.01.004
![]() |
[158] |
McKEE LH, Latner T (2000) Underutilized sources of dietary fiber: A review. Plant Foods Hum Nutr 55: 285–304. https://doi.org/10.1023/A:1008144310986 doi: 10.1023/A:1008144310986
![]() |
[159] | Wan Rosli WI, Aishah MS (2012) Pleurotus sajor-caju (PSC) improves nutrient contents and maintains sensory properties of carbohydrate-based products. Int J Nutr Food Eng 6: 87–89. |
[160] |
Kweon M, Slade L, Levine H (2011) Solvent retention capacity (SRC) testing of wheat flour: Principles and value in predicting flour functionality in different wheat-based food processes and in wheat breeding—A review. Cereal Chem 88: 537–552. https://doi.org/10.1094/CCHEM-07-11-0092 doi: 10.1094/CCHEM-07-11-0092
![]() |
[161] |
Ndungu SW, Otieno CA, Onyango C, et al. (2015) Nutritional composition, physical qualities and sensory evaluation of wheat bread supplemented with oyster mushroom. Am J Food Technol 10: 279–288. https://doi.org/10.3923/ajft.2015.279.288 doi: 10.3923/ajft.2015.279.288
![]() |
[162] |
Ndungu SW, Otieno CA, Onyango C, et al. (2015) Composition of polyphenols in wheat bread supplemented with Pleurotus ostreatus mushroom. Am J Food Technol 10: 273–278. https://doi.org/10.3923/ajft.2015.273.278 doi: 10.3923/ajft.2015.273.278
![]() |
[163] |
Cirlincione F, Venturella G, Gargano ML, et al. (2022) Functional bread supplemented with Pleurotus eryngii powder: A potential new food for human health. Int J Gastronomy Food Sci 27: 100449. https://doi.org/10.1016/j.ijgfs.2021.100449 doi: 10.1016/j.ijgfs.2021.100449
![]() |
[164] | Kim YJ, Jung IK, Kwak EJ (2010) Quality characteristics and antioxidant activities of cookies added with Pleurotus eryngii powder. Korean J Food Sci Technol 42: 183–189. |
[165] |
Prodhan UK, Linkon KMMR, Al-Amin MF, et al. (2015) Development and quality evaluation of mushroom (pleurotussajor-caju) enriched biscuits. Emirates J Food Agric 27: 542–547. https://doi.org/10.9755/ejfa.2015.04.082 doi: 10.9755/ejfa.2015.04.082
![]() |
[166] |
Bello M, Oluwamukomi M, Enujiugha V (2017) Nutrient composition and sensory properties of biscuit from mushroom-wheat composite flours. Arch Curr Res Int 9: 1–11. https://doi.org/10.9734/ACRI/2017/35686 doi: 10.9734/ACRI/2017/35686
![]() |
[167] |
Biao Y, Chen X, Wang S, et al. (2020) Impact of mushroom (Pleurotus eryngii) flour upon quality attributes of wheat dough and functional cookies-baked products. Food Sci Nutr 8: 361–370. https://doi.org/10.1002/fsn3.1315 doi: 10.1002/fsn3.1315
![]() |
[168] | Nelson AL (2001) High fiber ingredients. Minnesota, USA: Eagan Press. https://doi.org/10.1094/1891127233 |
[169] |
Proserpio C, Lavelli V, Gallotti F, et al. (2019) Effect of vitamin D-2 fortification using Pleurotus ostreatus in a whole-grain cereal product on child acceptability. Nutrients 11: 2441. https://doi.org/10.3390/nu11102441 doi: 10.3390/nu11102441
![]() |
[170] |
EFSA Panel on Dietetic Products, Nutrition and Allergies (NaA) (2016) Dietary reference values for vitamin D. EFSA J 14: e04547. https://doi.org/10.2903/j.efsa.2016.4547 doi: 10.2903/j.efsa.2016.4547
![]() |
[171] |
Kim S, Lee JW, Heo Y, et al. (2016) Effect of Pleurotus eryngii mushroom beta-glucan on quality characteristics of common wheat pasta. J Food Sci 81: C835–C840. https://doi.org/10.1111/1750-3841.13249 doi: 10.1111/1750-3841.13249
![]() |
[172] |
Song X, Zhu W, Pei Y, et al. (2013) Effects of wheat bran with different colors on the qualities of dry noodles. J Cereal Sci 58: 400–407. https://doi.org/10.1016/j.jcs.2013.08.005 doi: 10.1016/j.jcs.2013.08.005
![]() |
[173] |
Islas-Rubio AR, de la Barca AMC, Cabrera-Chávez F, et al. (2014) Effect of semolina replacement with a raw: Popped amaranth flour blend on cooking quality and texture of pasta. LWT-Food Sci Technol 57: 217–222. https://doi.org/10.1016/j.lwt.2014.01.014 doi: 10.1016/j.lwt.2014.01.014
![]() |
[174] | Bhattacharya M, Corke H (1996) Selection of desirable starch pasting properties in wheat for use in white salted or yellow alkaline noodles. Cereal chemistry (USA). |
[175] |
Arora B, Kamal S, Sharma VP (2017) Nutritional and quality characteristics of instant noodles supplemented with oyster mushroom (P. ostreatus). J Food Proc Preserv 42: e13521. https://doi.org/10.1111/jfpp.13521 doi: 10.1111/jfpp.13521
![]() |
[176] |
Parvin R, Farzana T, Mohajan S, et al. (2020) Quality improvement of noodles with mushroom fortified and its comparison with local branded noodles. NFS J 20: 37–42. https://doi.org/10.1016/j.nfs.2020.07.002 doi: 10.1016/j.nfs.2020.07.002
![]() |
[177] | Wahyono A, Novianti, Bakri A, et al. (2017) Physicochemical and sensorial characteristics of noodle enriched with oyster mushroom (Pleorotus ostreatus) powder. In: Journal of Physics: Conference Series, The 2nd International Joint Conference on Science and Technology (IJCST), Bali State Polytechn, Bali, Indonesia, 953: 012120. |
[178] |
Symons L, Brennan C (2004) The effect of barley β-glucan fiber fractions on starch gelatinization and pasting characteristics. J Food Sci 69: FCT257–FCT261. https://doi.org/10.1111/j.1365-2621.2004.tb06325.x doi: 10.1111/j.1365-2621.2004.tb06325.x
![]() |
[179] |
Beta T, Corke H (2001) Noodle quality as related to sorghum starch properties. Cereal Chem 78: 417–420. https://doi.org/10.1094/CCHEM.2001.78.4.417 doi: 10.1094/CCHEM.2001.78.4.417
![]() |
[180] | Bahri SS, Wan Rosli WI (2016) Effect of oyster mushroom (Pleurotus sajor-caju) addition on the nutritional composition and sensory evaluation of herbal seasoning. Int Food Res J 23: 262–268. |
[181] | Bahri SS, Wan Rosli WI (2017) Physical, rheological and textural characterization of herbal seasoning enriched with oyster mushroom (pleurotus sajor-caju) powder. Int Food Res J 24: 1445–1452. |
[182] |
Parab DN, Dhalagade JR, Sahoo AK, et al. (2012) Effect of incorporation of mushroom (Pleurotus sajor-caju) powder on quality characteristics of Papad (Indian snack food). Int J Food Sci Nutr 63: 866–870. https://doi.org/10.3109/09637486.2012.681629 doi: 10.3109/09637486.2012.681629
![]() |
[183] |
Gothandapani L, Parvathi K, John Kennedy Z (1997) Evaluation of different methods of drying on the quality of oyster mushroom (Pleurotus sp). Drying Technol 15: 1995–2004. https://doi.org/10.1080/07373939708917344 doi: 10.1080/07373939708917344
![]() |
[184] |
Balan V, Novak D, Knudson W, et al. (2021) Nutritious mushroom protein crisp—healthy alternative to starchy snack. Food Prod Proc Nutr 3: 33. https://doi.org/10.1186/s43014-021-00077-7 doi: 10.1186/s43014-021-00077-7
![]() |
[185] |
Verma A, Singh V (2017) Formulation and quality evaluation of mushroom (Oyster mushroom) powder fortified potato pudding. Asian J Dairy Food Res 36: 72–75. https://doi.org/10.18805/ajdfr.v36i01.7463 doi: 10.18805/ajdfr.v36i01.7463
![]() |
[186] |
Proserpio C, Lavelli V, Laureati M, et al. (2019) Effect of Pleurotus ostreatus powder addition in vegetable soup on beta-glucan content, sensory perception, and acceptability. Food Sci Nutr 7: 730–737. https://doi.org/10.1002/fsn3.917 doi: 10.1002/fsn3.917
![]() |
[187] |
Oh YS, Hwang JH, Lim SB (2012) Physiological activity of tofu fermented with mushroom mycelia. Food Chem 133: 728–734. https://doi.org/10.1016/j.foodchem.2012.01.083 doi: 10.1016/j.foodchem.2012.01.083
![]() |
[188] |
Asensio-Grau A, Calvo-Lerma J, Heredia A, et al. (2020) Enhancing the nutritional profile and digestibility of lentil flour by solid state fermentation with Pleurotus ostreatus. Food Funct 11: 7905–7912. https://doi.org/10.1039/D0FO01527J doi: 10.1039/D0FO01527J
![]() |
[189] |
Pandey A (2003) Solid–state fermentation. Biochem Eng J 13: 81–84. https://doi.org/10.1016/S1369-703X(02)00121-3 doi: 10.1016/S1369-703X(02)00121-3
![]() |
[190] |
Rodriguez S, Sanromán M (2006) Application of solid-state fermentation to food industry—a review. J Food Eng 76: 291–302. https://doi.org/10.1016/j.jfoodeng.2005.05.022 doi: 10.1016/j.jfoodeng.2005.05.022
![]() |
[191] |
Zhao Y, Sun-Waterhouse D, Zhao M, et al. (2018) Effects of solid-state fermentation and proteolytic hydrolysis on defatted soybean meal. LWT-Food Sci Technol 97: 496–502. https://doi.org/10.1016/j.lwt.2018.06.008 doi: 10.1016/j.lwt.2018.06.008
![]() |
[192] |
Adamović M, Grubić G, Milenković I, et al. (1998) The biodegradation of wheat straw by Pleurotus ostreatus mushrooms and its use in cattle feeding. Anim Feed Sci Technol 71: 357–362. https://doi.org/10.1016/S0377-8401(97)00150-8 doi: 10.1016/S0377-8401(97)00150-8
![]() |
[193] |
Cohen R, Persky L, Hadar Y (2002) Biotechnological applications and potential of wood-degrading mushrooms of the genus Pleurotus. Appl Microbiol Biotechnol 58: 582–594. https://doi.org/10.1007/s00253-002-0930-y doi: 10.1007/s00253-002-0930-y
![]() |
[194] | Kadiri M (1999) Changes in intracellular and extracellular enzyme activities of Lentinus subnudus during sporophore development. Biosci Res Commun 11: 127–130. |
[195] |
Espinosa-Paez E, Alanis-Guzmán MG, Hernández-Luna CE, et al. (2017) Increasing antioxidant activity and protein digestibility in Phaseolus vulgaris and Avena sativa by fermentation with the Pleurotus ostreatus fungus. Molecules 22: 11. https://doi.org/10.3390/molecules22122275 doi: 10.3390/molecules22122275
![]() |
[196] |
Granito M, Paolini M, Perez S (2008) Polyphenols and antioxidant capacity of Phaseolus vulgaris stored under extreme conditions and processed. LWT-Food Sci Technol 41: 994–999. https://doi.org/10.1016/j.lwt.2007.07.014 doi: 10.1016/j.lwt.2007.07.014
![]() |
[197] |
Hur SJ, Lee SY, Kim YC, et al. (2014) Effect of fermentation on the antioxidant activity in plant-based foods. Food Chem 160: 346–356. https://doi.org/10.1016/j.foodchem.2014.03.112 doi: 10.1016/j.foodchem.2014.03.112
![]() |
[198] | Bautista Justo M, Alanís Guzmán MG, González de Mejía E, et al. (1998) Composición química de tres cepas mexicanas de setas (Pleurotus ostreatus). Arch Latinoam Nutr: 359–363. |
[199] |
Rodrigues da Luz JM, Paes SA, Torres DP, et al. (2013) Production of edible mushroom and degradation of antinutritional factors in jatropha biodiesel residues. LWT-Food Sci Technol 50: 575–580. https://doi.org/10.1016/j.lwt.2012.08.006 doi: 10.1016/j.lwt.2012.08.006
![]() |
[200] |
Sánchez-García J, Asensio-Grau A, García-Hernández J, et al. (2022) Nutritional and antioxidant changes in lentils and quinoa through fungal solid-state fermentation with Pleurotus ostreatus. Bioresour Bioprocess 9: 51. https://doi.org/10.1186/s40643-022-00542-2 doi: 10.1186/s40643-022-00542-2
![]() |
[201] |
Xu LN, Guo S, Zhang S (2018) Effects of solid-state fermentation with three higher fungi on the total phenol contents and antioxidant properties of diverse cereal grains. FEMS Microbiol Letters 365: fny163. https://doi.org/10.1093/femsle/fny163 doi: 10.1093/femsle/fny163
![]() |
[202] |
Miura T, Yuan L, Sun B, et al. (2002) Isoflavone aglycon produced by culture of soybean extracts with basidiomycetes and its anti-angiogenic activity. Biosci, Biotechnol, Biochem 66: 2626–2631. https://doi.org/10.1271/bbb.66.2626 doi: 10.1271/bbb.66.2626
![]() |
[203] |
Setchell KD, Brown NM, Desai P, et al. (2001) Bioavailability of pure isoflavones in healthy humans and analysis of commercial soy isoflavone supplements. J Nutr 131: 1362S–1375S. https://doi.org/10.1093/jn/131.4.1362S doi: 10.1093/jn/131.4.1362S
![]() |
[204] |
Lopez-Moreno M, Garces-Rimon M, Miguel M (2022) Antinutrients: Lectins, goitrogens, phytates and oxalates, friends or foe? J Funct Foods 89: 9. https://doi.org/10.1016/j.jff.2022.104938 doi: 10.1016/j.jff.2022.104938
![]() |
[205] |
Akindahunsi A, Oyetayo F (2006) Nutrient and antinutrient distribution of edible mushroom, Pleurotus tuber-regium (fries) singer. LWT-Food Sci Technol 39: 548–553. https://doi.org/10.1016/j.lwt.2005.04.005 doi: 10.1016/j.lwt.2005.04.005
![]() |
[206] | Duru M, Eboagwu I, Kalu W, et al. (2019) Nutritional, anti-nutritional and biochemical studies on the oyster mushroom, Pleurotus ostreatus. EC Nutr 14: 36–59. |
[207] |
Petroski W, Minich DM (2020) Is there such a thing as "anti-nutrients"? A narrative review of perceived problematic plant compounds. Nutrients 12: 2929. https://doi.org/10.3390/nu12102929 doi: 10.3390/nu12102929
![]() |
[208] |
Das AK, Nanda PK, Dandapat P, et al. (2021) Edible mushrooms as functional ingredients for development of healthier and more sustainable muscle foods: A flexitarian approach. Molecules 26: 2463. https://doi.org/10.3390/molecules26092463 doi: 10.3390/molecules26092463
![]() |
[209] |
Pierpoint W (1969) o-Quinones formed in plant extracts. Their reactions with amino acids and peptides. Biochem J 112: 609–616. https://doi.org/10.1042/bj1120609 doi: 10.1042/bj1120609
![]() |
[210] |
Lu FSH, Bruheim I, Jacobsen C (2015) Maillard reaction and lipid peroxidation contribute to non-enzymatic browning in krill-based products: A model study on proposed mechanisms. Eur J Lipid Sci Technol 117: 421–430. https://doi.org/10.1002/ejlt.201400281 doi: 10.1002/ejlt.201400281
![]() |
[211] |
Elleuch M, Bedigian D, Roiseux O, et al. (2011) Dietary fibre and fibre-rich by-products of food processing: Characterisation, technological functionality and commercial applications: A review. Food Chem 124: 411–421. https://doi.org/10.1016/j.foodchem.2010.06.077 doi: 10.1016/j.foodchem.2010.06.077
![]() |
[212] | Pomeranz Y, Shogren M, Finney K, et al. (1976) Fiber in breadmaking—effects on functional properties. Bakers Digest. |
[213] | Dubois D (1978) The practical application of fiber materials in bread production. Proc Annu Meet Am Soc Bakery Eng 54: 48–54. |
[214] | Regulation EC (2006) No. 1924/2006 of the European Parliament and of the Council of 20 December 2006 on nutrition and health claims made on foods. Official Journal of the European Union, 9–25. |
[215] |
Fu Q, Shi H, Hu D, et al. (2022) Pork longissimus dorsi marinated with edible mushroom powders: Evaluation of quality traits, microstructure, and protein degradation. Food Res Int 158: 111503. https://doi.org/10.1016/j.foodres.2022.111503 doi: 10.1016/j.foodres.2022.111503
![]() |
[216] |
Wang X, Zhou P, Cheng J, et al. (2018) Use of straw mushrooms (Volvariella volvacea) for the enhancement of physicochemical, nutritional and sensory profiles of Cantonese sausages. Meat Sci 146: 18–25. https://doi.org/10.1016/j.meatsci.2018.07.033 doi: 10.1016/j.meatsci.2018.07.033
![]() |
[217] | Xiong YL (2000) Protein oxidation and implications for muscle food quality. Antioxid Muscle Foods: 85–111. |
[218] |
Poore J, Nemecek T (2018) Reducing food's environmental impacts through producers and consumers. Science 360: 987–992. https://doi.org/10.1126/science.aaq0216 doi: 10.1126/science.aaq0216
![]() |
[219] |
Sá AGA, Moreno YMF, Carciofi BAM (2020) Plant proteins as high-quality nutritional source for human diet. Trends Food Sci Technol 97: 170–184. https://doi.org/10.1016/j.tifs.2020.01.011 doi: 10.1016/j.tifs.2020.01.011
![]() |
[220] |
Erjavec J, Kos J, Ravnikar M, et al. (2012) Proteins of higher fungi–from forest to application. Trends Biotechnol 30: 259–273. https://doi.org/10.1016/j.tibtech.2012.01.004 doi: 10.1016/j.tibtech.2012.01.004
![]() |
[221] |
Perez-Montes A, Rangel-Vargas E, Manuel Lorenzo J, et al. (2021) Edible mushrooms as a novel trend in the development of healthier meat products. Curr Opin Food Sci 37: 118–124. https://doi.org/10.1016/j.cofs.2020.10.004 doi: 10.1016/j.cofs.2020.10.004
![]() |
1. | Abdulhamed Alsisi, Raluca Eftimie, Dumitru Trucu, Nonlocal multiscale modelling of tumour-oncolytic viruses interactions within a heterogeneous fibrous/non-fibrous extracellular matrix, 2022, 19, 1551-0018, 6157, 10.3934/mbe.2022288 | |
2. | Léon Masurel, Carlo Bianca, Annie Lemarchand, Space-velocity thermostatted kinetic theory model of tumor growth, 2021, 18, 1551-0018, 5525, 10.3934/mbe.2021279 | |
3. | Sabrina Glaschke, Hana M. Dobrovolny, Spatiotemporal spread of oncolytic virus in a heterogeneous cell population, 2024, 183, 00104825, 109235, 10.1016/j.compbiomed.2024.109235 | |
4. | O. E. Adebayo, S. Urcun, G. Rolin, S. P. A. Bordas, D. Trucu, R. Eftimie, Mathematical investigation of normal and abnormal wound healing dynamics: local and non-local models, 2023, 20, 1551-0018, 17446, 10.3934/mbe.2023776 | |
5. | Carlo Bianca, A decade of thermostatted kinetic theory models for complex active matter living systems, 2024, 50, 15710645, 72, 10.1016/j.plrev.2024.06.015 | |
6. | Hana M. Dobrovolny, How do viruses get around? A review of mathematical modeling of in-host viral transmission, 2025, 604, 00426822, 110444, 10.1016/j.virol.2025.110444 |
Param. | Value | Description | Reference |
Dcm | 0.00035 | Uninfected migrating cancer cell diffusion coefficient | [43] |
Dim | 0.0054 | Infected migrating cancer cell diffusion coefficient | [54] |
Dip | 0.00054 | Infected proliferative cancer cell diffusion coefficient | Estimated |
Dcp | 10−5 | Uninfected proliferative cancer cell diffusion coefficient | Estimated |
Dv | 0.0036 | Constant diffusion coefficient for OV | [54] |
ηcp | 0.00285 | Infected proliferative cancer cell haptotaxis coefficient | Estimated |
ηim | 0.0285 | Infected migrating cancer cell haptotaxis coefficient | [29] |
ηip | 0.00285 | Infected proliferative cancer cell haptotaxis coefficient | Estimated |
ηv | 0.0285 | OV haptotaxis coefficient | [29] |
μm | 0.5 | Proliferation rate for uninfected migrating cancer cells | [41] |
μp | 0.75 | Proliferation rate for uninfected proliferative cancer cells | [41] |
Scmcm | 0.1 | Maximum rate of cell-cell adhesion strength | [44] |
Scpcp | 0.05 | Maximum rate of cell-cell adhesion strength | Estimated |
Simim | 0.1 | Maximum rate of cell-cell adhesion strength | Estimated |
Sipip | 0.05 | Maximum rate of cell-cell adhesion strength | Estimated |
Scme | 0.5 | Rate of Cell-ECM adhesion strength | [55] |
Scpe | 0.001 | Rate of Cell-ECM adhesion strength | Estimated |
Sime | 0.5 | Rate of Cell-ECM adhesion strength | Estimated |
Sipe | 0.001 | Rate of Cell-ECM adhesion strength | Estimated |
ω1 | 0.1 | Rate of switching from proliferative state (cp) to migration state (cm) | Estimated |
ω2 | 0.4 | Rate of switching from migration (cm) to proliferative state (cp). | Estimated |
αcm | 0.075 | ECM degradation rate by uninfected cancer cells | Estimated |
αim | αcm2 | ECM degradation rate by infected cancer cells | [29] |
αcp | 0.075 | ECM degradation rate by uninfected cancer cells | Estimated |
αip | αcm2 | ECM degradation rate by infected cancer cells | Estimated |
μ2 | 0.02 | Remodelling term coefficient | [34] |
ϱm | 0.079 | Infection rate of cm cells by OV | [29] |
ϱp | 0.079 | Infection rate of cells by OV | Estimated |
δim | 0.05 | Death rate of infected cancer cells | [54] |
δip | 0.05 | Death rate of infected cancer cells | Estimated |
bm | 40 | Replicating rate of OVs in infected cancer cells cm | Estimated |
bp | 40 | Replicating rate of OVs in infected cancer cells cp | Estimated |
δv | 0.05 | Death rate of OV | [54] |
νe | 1 | The fraction of physical space occupied by the ECM | [47] |
νc | 1 | The fraction of physical space occupied by cancer cells | [47] |
γcm | 1.5 | MDEs secretion rate by uninfected cancer cell | [56] |
γim | 1 | MDEs secretion rate by infected cancer cell | [56] |
γcp | 1 | MDEs secretion rate by uninfected cancer cell | Estimated |
γip | 1.5 | MDEs secretion rate by infected cancer cell | Estimated |
Dm | 0.004 | MDE diffusion coefficient | [57] |
Param. | Value | Description | Reference |
Dcm | 0.00035 | Uninfected migrating cancer cell diffusion coefficient | [43] |
Dim | 0.0054 | Infected migrating cancer cell diffusion coefficient | [54] |
Dip | 0.00054 | Infected proliferative cancer cell diffusion coefficient | Estimated |
Dcp | 10−5 | Uninfected proliferative cancer cell diffusion coefficient | Estimated |
Dv | 0.0036 | Constant diffusion coefficient for OV | [54] |
ηcp | 0.00285 | Infected proliferative cancer cell haptotaxis coefficient | Estimated |
ηim | 0.0285 | Infected migrating cancer cell haptotaxis coefficient | [29] |
ηip | 0.00285 | Infected proliferative cancer cell haptotaxis coefficient | Estimated |
ηv | 0.0285 | OV haptotaxis coefficient | [29] |
μm | 0.5 | Proliferation rate for uninfected migrating cancer cells | [41] |
μp | 0.75 | Proliferation rate for uninfected proliferative cancer cells | [41] |
Scmcm | 0.1 | Maximum rate of cell-cell adhesion strength | [44] |
Scpcp | 0.05 | Maximum rate of cell-cell adhesion strength | Estimated |
Simim | 0.1 | Maximum rate of cell-cell adhesion strength | Estimated |
Sipip | 0.05 | Maximum rate of cell-cell adhesion strength | Estimated |
Scme | 0.5 | Rate of Cell-ECM adhesion strength | [55] |
Scpe | 0.001 | Rate of Cell-ECM adhesion strength | Estimated |
Sime | 0.5 | Rate of Cell-ECM adhesion strength | Estimated |
Sipe | 0.001 | Rate of Cell-ECM adhesion strength | Estimated |
ω1 | 0.1 | Rate of switching from proliferative state (cp) to migration state (cm) | Estimated |
ω2 | 0.4 | Rate of switching from migration (cm) to proliferative state (cp). | Estimated |
αcm | 0.075 | ECM degradation rate by uninfected cancer cells | Estimated |
αim | αcm2 | ECM degradation rate by infected cancer cells | [29] |
αcp | 0.075 | ECM degradation rate by uninfected cancer cells | Estimated |
αip | αcm2 | ECM degradation rate by infected cancer cells | Estimated |
μ2 | 0.02 | Remodelling term coefficient | [34] |
ϱm | 0.079 | Infection rate of cm cells by OV | [29] |
ϱp | 0.079 | Infection rate of cells by OV | Estimated |
δim | 0.05 | Death rate of infected cancer cells | [54] |
δip | 0.05 | Death rate of infected cancer cells | Estimated |
bm | 40 | Replicating rate of OVs in infected cancer cells cm | Estimated |
bp | 40 | Replicating rate of OVs in infected cancer cells cp | Estimated |
δv | 0.05 | Death rate of OV | [54] |
νe | 1 | The fraction of physical space occupied by the ECM | [47] |
νc | 1 | The fraction of physical space occupied by cancer cells | [47] |
γcm | 1.5 | MDEs secretion rate by uninfected cancer cell | [56] |
γim | 1 | MDEs secretion rate by infected cancer cell | [56] |
γcp | 1 | MDEs secretion rate by uninfected cancer cell | Estimated |
γip | 1.5 | MDEs secretion rate by infected cancer cell | Estimated |
Dm | 0.004 | MDE diffusion coefficient | [57] |