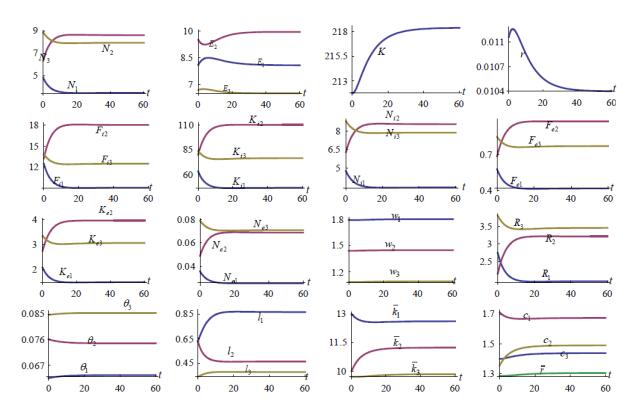
E-bikes have become one of China's most popular travel modes. The authorities have issued helmet-wearing regulations to increase wearing rates to protect e-bike riders' safety, but the effect is unsatisfactory. To reveal the factors influencing the helmet-wearing behavior of e-bike riders, this study constructed a theoretical Push-Pull-Mooring (PPM) model to analyze the factor's relationship from the perspective of travel behavior switching. A two-step SEM-ANFIS method is proposed to test relationships, rank importance and analyze the combined effect of psychological variables. The Partial Least Squares Structural Equation Model (PLS-SEM) was used to obtain the significant influencing factors. The Adaptive Network-based Fuzzy Inference System (ANFIS), a nonlinear approach, was applied to analyze the importance of the significant influencing factors and draw refined conclusions and suggestions from the analysis of the combined effects. The PPM model we constructed has a good model fit and high model predictive validity (GOF = 0.381, R2 = 0.442). We found that three significant factors tested by PLS-SEM, perceived legal norms (β = 0.234, p < 0.001), perceived inconvenience (β = -0.117, p < 0.001) and conformity tendency (β = 0.241, p < 0.05), are the most important factors in the effects of push, mooring and pull. The results also demonstrated that legal norm is the most important factor but has less effect on people with low perceived vulnerability, and low subjective norms will make people with high conformity tendency to follow the crowd blindly. This study could contribute to developing refined interventions to improve the helmet-wearing rate effectively.
Citation: Peng Jing, Weichao Wang, Chengxi Jiang, Ye Zha, Baixu Ming. Determinants of switching behavior to wear helmets when riding e-bikes, a two-step SEM-ANFIS approach[J]. Mathematical Biosciences and Engineering, 2023, 20(5): 9135-9158. doi: 10.3934/mbe.2023401
[1] | Massimiliano Calvia . Fossil energy use and carbon emissions: An easy-to-implement technical policy experiment. Green Finance, 2024, 6(3): 407-429. doi: 10.3934/GF.2024016 |
[2] | Yuhang Zheng, Shuanglian Chen, Nan Wang . Does financial agglomeration enhance regional green economy development? Evidence from China. Green Finance, 2020, 2(2): 173-196. doi: 10.3934/GF.2020010 |
[3] | Hatice Şimşek, Gökhan Öztürk . Evaluation of the relationship between environmental accounting and business performance: the case of Istanbul province. Green Finance, 2021, 3(1): 46-58. doi: 10.3934/GF.2021004 |
[4] | Vladimir Kurdyukov, Alla Ovcharenko . Assessing the economic efficiency of green investments in the context of sustainable development of the territory. Green Finance, 2023, 5(3): 343-372. doi: 10.3934/GF.2023014 |
[5] | Sabuj Saha, Ahmed Rizvan Hasan, Kazi Rezwanul Islam, Md Asraful Islam Priom . Sustainable Development Goals (SDGs) practices and firms' financial performance: Moderating role of country governance. Green Finance, 2024, 6(1): 162-198. doi: 10.3934/GF.2024007 |
[6] | Mengze Wu, Dejun Xie . The impact of ESG performance on the credit risk of listed companies in Shanghai and Shenzhen stock exchanges. Green Finance, 2024, 6(2): 199-218. doi: 10.3934/GF.2024008 |
[7] | Inna Semenenko, Yana Bilous, Ruslan Halhash . The compliance of the regional development strategies and funding with the sustainable development concept: The case of Ukraine. Green Finance, 2022, 4(2): 159-178. doi: 10.3934/GF.2022008 |
[8] | Mukul Bhatnagar, Sanjay Taneja, Ercan Özen . A wave of green start-ups in India—The study of green finance as a support system for sustainable entrepreneurship. Green Finance, 2022, 4(2): 253-273. doi: 10.3934/GF.2022012 |
[9] | Gonzalo Maldonado-Guzmán . Green innovation mediates between financial innovation and business sustainability? Proof in the mexican manufacturing industry. Green Finance, 2024, 6(3): 563-584. doi: 10.3934/GF.2024021 |
[10] | Yicong Huang, Kaidong Yu, Chao Huang . Green finance engagement: An empirical study of listed companies on Chinese main board. Green Finance, 2023, 5(1): 1-17. doi: 10.3934/GF.2023001 |
E-bikes have become one of China's most popular travel modes. The authorities have issued helmet-wearing regulations to increase wearing rates to protect e-bike riders' safety, but the effect is unsatisfactory. To reveal the factors influencing the helmet-wearing behavior of e-bike riders, this study constructed a theoretical Push-Pull-Mooring (PPM) model to analyze the factor's relationship from the perspective of travel behavior switching. A two-step SEM-ANFIS method is proposed to test relationships, rank importance and analyze the combined effect of psychological variables. The Partial Least Squares Structural Equation Model (PLS-SEM) was used to obtain the significant influencing factors. The Adaptive Network-based Fuzzy Inference System (ANFIS), a nonlinear approach, was applied to analyze the importance of the significant influencing factors and draw refined conclusions and suggestions from the analysis of the combined effects. The PPM model we constructed has a good model fit and high model predictive validity (GOF = 0.381, R2 = 0.442). We found that three significant factors tested by PLS-SEM, perceived legal norms (β = 0.234, p < 0.001), perceived inconvenience (β = -0.117, p < 0.001) and conformity tendency (β = 0.241, p < 0.05), are the most important factors in the effects of push, mooring and pull. The results also demonstrated that legal norm is the most important factor but has less effect on people with low perceived vulnerability, and low subjective norms will make people with high conformity tendency to follow the crowd blindly. This study could contribute to developing refined interventions to improve the helmet-wearing rate effectively.
Regional agglomeration and environmental changes are main features of contemporary economies. Moreover, economies experience business cycles during development processes. It is a greatly challenging task for economists to develop genuine dynamic models which exhibit business cycles, economic growth, and spatial agglomeration on microeconomic foundation. This paper is to develop a nonlinear multi-regional dynamic model with wealth and pollutant accumulation over time. We generalize the dynamic model by Zhang (2016). The original model examines multi-regional dynamics with wealth and pollutant accumulation over time. This study generalizes the model by making all exogenous constant parameters as exogenous time-dependent parameters. We demonstrate business cycles due to different exogenous periodic perturbations.
This study is concerned with regional agglomeration commonly observed in developed economies as well as in many underdeveloped economies. Kuznets (1966) discussed spatial unequal economic development over space long time ago. He examined interdependence between spatial agglomeration and industrialization. Myrdal (1957) and Hirschman (1958) earlier argued for dynamic interactions between industrial growth and the geographical concentration of industry. There are numerous studies on various aspects of spatial economic developments (e.g., Krugman, 1991; Fujita et al., 1999; Forslid and Ottaviano, 2003). Different from earlier literature on spatial agglomeration, Zhang (2016) extends neoclassical growth theory to multi-regional economies. This approach is different from the so-called new economic geography (e.g., Krugman, 1993; and Tabuchi, 2014). In the new economic geography, the role of physical capital is omitted in spatial dynamics. Moreover, In the literature of the new economic geography, Tabuchi (2014: 50) reviews: "The scopes of most of the theoretical studies published thus far have been limited to two regions in order for researchers to reach meaningful analytical results. The two-region NEG models tend to demonstrate that spatial distribution is dispersed in the early period (high trade costs or low manufacturing share) and agglomerated in one of the two regions in the late period (low trade costs or high manufacturing share). However, it is no doubt that the two-region NEG models are too simple to describe the spatial distribution of economic activities in real-world economies. Since there are only two regions, their geographical locations are necessarily symmetric, and thus diverse spatial distributions cannot occur." This study examines the model with any number of regions in order to properly address issues related to interregional growth and agglomeration.
Dynamic interdependence between economic growth and environmental changes is extensively studied (e.g., Ploude, 1972; John and Pecchenino, 1994; Gassebner et al., 2011; Lin and Liscow, 2012; Apergis and Payne, 2014; Yang and Zhao, 2014; Antonakakis et al., 2017; and Walenta, 2018). It is pointed out that theoretical economics fails to address many issues related economic growth and environmental change with a comprehensive framework with microeconomic foundation. As pointed out by Fullerton and Kim (2008), existing research has proposed a number of different models for analyzing different questions not in an integrated way. This study deals with growth, interregional trade, and environmental change within an integrated framework. It should be noted that following Zhang (1996), we also consider amenity endogenous. Amenity is assumed to be related to environmental quality (and other factors such as historical building and regional population, residential densities) and household location (e.g., Glaeser et al., 2001; Partridge et al., 2008; Chen et al., 2013; Lai, 2019; and Tivadar and Jayet, 2019). Zhang (1993) first introduced spatial amenity into utility in a general equilibrium framework. This study incorporates amenity into the consumer location decision. According to Chen et al. (2013), "Empirical evidence demonstrates a growing link between the presence of high-valued natural amenities—including pleasant climate and proximity to lakes, oceans, forests, and mountains—and higher rates of population and income growth in the U.S." Chen et al. (2013) developed a two-region model in which labor distribution, production externalities and natural resources are endogenous. According to their research, preference for natural amenities tends to lead population dispersion. This study shows how amenity is related to regional agglomeration.
Business cycles occur due to exogenous or endogenous. For instance, real business-cycle theory treats business cycles responses to various exogenous changes in the real economic variables. In the literature of economic theory, only a few formal models can properly deal with exogenous cycles on basis of microeconomics. This study introduces exogenous real shocks to the neoclassical growth model to demonstrate the existence of business cycles due to real shocks. It should be noted that in modern nonlinear dynamic economic theory business cycles occur with or without any exogenous changes (e.g., Zhang, 1991, 2005, 2006; Lorenz, 1993; Flaschel et al. 1997; Chiarella and Flaschel, 2000; Shone, 2002; Gandolfo, 2005; and Puu, 2011). Although there are many models of business cycles for national economies, there are a few nonlinear multi-regional dynamic models with microeconomic foundation for identifying business cycles. Different theories emphasize various origins of economic periodic changes. The rest of the study is organized as follows. Section 2 extends Zhang's model to allow for existence of different time-dependent changeable parameters. In Section 3 we demonstrate that the movement of the economy is described by differential equations. The section also simulates the model for a three-regional economy. Section 4 studies comparative dynamic analysis in some exogenous periodic oscillations. Section 5 concludes the study.
This section is to generalize Zhang's model by making all exogenous constant parameters to be exogenous time-dependent (Zhang, 2016). The economy is composed of J regions, indexed by j=1,…,J Each region has one production sector and one environment sector. The production sector is the same as in the the Solow growth model. The system produces one (durable) good. Assets are owned by households. Capital and labor as inputs of the production sector. Productivities of the production sector fully utilized. Saving is undertaken only by households. Commodities are traded without any barriers. We neglect transport costs. We measure prices in terms of the commodity and the price of the commodity be unity. We represent wage and interest rates by wj(t) and rj(t) respectively, in the jth region. The interest rate is equal across regions, i.e., r(t)=rj(t) We consider that the national population N(t) is homogenous. Workers are free to choose their work place and residential location. Workers work and reside in the same region. Each region has land Lj(t) which is used only for residential purposes. Regions have different productivities and living conditions. We use subscripts, i, e, to denote the production and environment sectors, respectively. Let Fqj(t) stand for the output levels of q's sector in region j at time t,q=i,e. Each region has one environment sector. The role of the regional government is to collect different taxes on producers and consumers to financially support the sector. The tax income is used up for employing labor and capital to purify environment.
The production sectors use labor and physical capital inputs. Environment affects productivities. The production functions are specified as follows:
Fij(t)=Aij(t)Γij(Ej(t))Kαij(t)ij(t)Nβij(t)ij(t),Aij(t),αij(t),βij(t)>0,αij(t)+βij=1 | (1) |
where Aij(t), αij(t), and βij(t) are positive parameters. Here, Γij(Ej(t)) is a function of the environmental quality measured by the level of pollution, Ej(t), in region j. It is assumed that the productivity of the capital goods sector is non-positively related to the pollution level. Let δkj stand for the depreciation rate of physical capital in region j. We use τij(t) to stand for the tax rate on the capital goods sector. The marginal conditions of the production sector are:
r(t)+δkj(t)=αij(t)ˉτij(t)Fij(t)Kij(t),wj(t)=βij(t)ˉτij(t)Fij(t)Nij(t) | (2) |
where ¯τij(t)≡1−τij(t),0<τij(t)<1.
Following Zhang (2016) we specify the dynamics of the stock of pollutants as follows:
˙Ej(t)=θij(t)Fij(t)+θcj(t)Cj(t)−Fej(t)−ˉθj(t)Ej(t)+Ωj((Eq(t))) | (3) |
in which θijt, θcj(t) and θj(t) are positive parameters and
Fej(t)=Aej(t)Γej(Ej(t))K˜αej(t)ej(t)N˜βejej(t),Aej(t),˜αej(t),˜βej(t)>0 | (4) |
where Aej(t), αej(t), and βej(t) are positive parameters, and Γej(Ej)(≥0) is a function of Ej(t). The term θij(t) Fij(t) means that pollutants that are emitted during production processes are linearly positively proportional to the output level. The parameter, θij(t), means that in consuming one unit of the good the quantity θij(t) is left as waste. The creation of pollutants by consumers is given θcj(t) Cj(t). The parameter θj(t) is called the rate of natural purification.
We take account of effects of transboundary pollution by Ωj((Eq(t))) where is a function of pollutants of all the regions.
All the income from land is evenly redistributed among the population. Region j's land is Lj(t) and the land rent is Rj(t). The land revenue r(t) per worker is as follows:
¯r(t)=1N∑Jj=1Lj(t)Rj(t) | (5) |
Let kj(t) stand for per capita wealth of region j. We have kj(t) = Kj(t)/Nj(t), where K(t) is the total wealth held by region j. We use τkj(t) and τwj(t) to stand for the tax rates on the wealth income and wage income in region j, respectively. Per capita current income from the land ownership, the interest payment r(t)kj(t), and the wage payment wj(t), is
yj(t)=¯r(t)+¯τkj(t)r(t)¯kj(t)+¯τwj(t)wj(t) | (6) |
where τkj(t)≡1-τkj(t) and τwj(t) ≡1-τwj(t). The per sable income an disposable income is the sum of the current disposable income and the value of wealth:
ˆyj(t)=¯kj(t)+yj(t) | (7) |
The disposable income is used for saving and consumption. At each point of time, a consumer would distribute the total available budget between housing, lj(t), saving sj(t) and consumption cj(t). The budget constraint is given by
(1+τRj(t))Rj(t)lj(t)+(1+τcj(t))pj(t)cj(t)+sj(t)=ˆyj(t) | (8) |
where τRj(t) is the tax rate on housing and τcj(t) is the tax rate on consumption.
Utility level Uj(t) is dependent on the consumption levels of lot size and commodity, and saving. The utility level of the typical consumer in region j is represented by
Uj(t)=θj(t)lη0(t)j(t)cξ0(t)jsλ0(t)j(t),η0(t),ξ0(t),λ0(t)>0 | (9) |
in which η0(t), ξ0(t), and λ0(t) are the elasticities of utility with regard to lot size, commodity and saving. We call η0(t), ξ0(t), and λ0(t) propensities to consume lot size, and goods, and to hold wealth, respectively. In (9), θj(t) is region j's amenity level (e.g., Diamond and Tolley, 1981; Landry and Chakraborty, 2009; Lanz and Provins, 2013; Debarsy and Ertur, 2019; and Saracoglu and Roe, 2019). We specify θj(t) as follows:
θj(t)=¯θj(t)Eω(t)j(t)Nd(t)j(t),j=1,…,J | (10) |
where θj(t) (>0), ω(≤0), and d(t) are parameters, and Nj(t) is region j's population. We don't specify signs of d(t) as population may have either positive or negative effects on regional attractiveness.
Maximizing Uj(t) subject to (8) yields
lj(t)Rj(t)=ηj(t)ˆyj(t),cj(t)=ξj(t)ˆyj(t),sj(t)=λ(t)ˆyj(t) | (11) |
where
ηj(t)≡η0(t)ρ(t)1+τRj(t),ξj(t)≡ξ0(t)ρ(t)1+τcj,λ(t)≡λ0(t)ρ(t) | (12) |
ρ(t)≡1η0(t)+ξ0(t)+λ0(t) | (13) |
From (11) we see
sj(t)=λ(t)[¯r(t)+¯kj(t)+¯τkj(t)r(t)¯kj(t)+¯τwj(t)wj(t)] | (14) |
According to the definitions of sj(t), the wealth accumulation of the representative household in region j is:
˙¯kj(t)=sj(t)−¯kj(t) | (15) |
We assume that households are freely mobile between the regions. The utility levels of homogenous population between regions are assumed to be equal at any point in time. That is
Uj(t)=Uq(t),j,q=1,…,J | (16) |
Each region's governmental tax income consists of the tax incomes on the production sector, consumption, wage income and wealth income as follows:
Yej(t)=τij(t)Fij(t)+τRj(t)lj(t)Rj(t)Nj(t)+τcj(t)cj(t)Nj(t)+τwj(t)wj(t)Nj(t)+τkj(t)r(t)ˉkj(t)Nj(t) | (17) |
The government's income is used up only for the environmental purpose. The government budget is as follows:
(r(t)+δk(t))Kej(t)+wj(t)Nej(t)=Yej(t) | (18) |
The government employs labor and capital stocks for purifying environment in such a way that the purification rate is maximized under the given budget constraint. The government's optimal problem is thus formed by:
Max{Kej(t),Nej(t)}Fej(t)s.t.: | (19) |
The optimal solution is:
(r(t)+δk(t))Kej(t)=αej(t)Yej(t),wj(t)Nej(t)=βej(t)Yej(t) | (20) |
where
αej(t)≡˜αej(t)˜αej(t)+˜βej(t),βej(t)≡˜βej(t)˜αej(t)+˜βej(t) | (21) |
Each region's capital stocks Kj(t) is fully
Kij(t)+Kej(t)=Kj(t) | (22) |
The total capital stock K(t) employed by the production sectors is equal to the total wealth owned by the households of all the regions:
K(t)=∑Jj=1Kj(t)=∑Jj=1¯kj(t)Nj(t) | (23) |
The assumption that labor force and land are fully employed is represented by:
Nij(t)+Nej(t)=Nj(t),ΣJj=1Nj(t)=N(t) | (24) |
lj(t)Nj(t)=Lj(t),j=1,…,J | (25) |
We have thus built the model.
The economic system contains many variables which are nonlinearly interrelated. No general analytical method is available to solve such nonlinear differential equations. For illustration, we simulate the model. In the appendix, we show that the dynamics of the national economy can be expressed as differential equations. First, we introduce a variable z1(t)
z1(t)≡r(t)+δk1(t)w1(t) | (26) |
The following lemma provides the procedure to simulate the model.
The motion of the national economy is given by the following 2J+2 differential equations with z1(t),¯r(t),(¯kj(t)) and (Ej(t)) as variables
˙ˉkj(t)=Φj(z1(t),ˉr(t),(ˉkq(t)),(Eq(t)),t),˙Ej(t)=Λj(z1(t),ˉr(t),(ˉkq(t)),(Eq(t)),t),j=1,…,J,˙z1(t)=ˉΦ(z1(t),ˉr(t),(ˉkq(t)),(Eq(t)),t),˙ˉr(t)=ˉΛ(z1(t),ˉr(t),(ˉkq(t)),(Eq(t)),t) | (27) |
where Φj(t),ˉΦ(t),Λj(t) and ˉΛ(t) are functions of t,z1(t),¯r(t),(¯kj(t)) and (Ej(t)) defined in the appendix. For any given positive values of z1(t),¯r(t),(¯kj(t)) and (Ej(t)) at any point in time, the other variables are uniquely determined by the following procedure: r(t) by (A2) → wj(t) by (A4) →ˆyj(t) by (A5) → Rj(t) by (A6) → N1(t) by (A11) → Nj(t) by (A15) → Nej(t) and Nij(t) by (A14) → Kej(t) and Kij(t) by (A1) → Fij(t) by (4) → Fej(t) by (4) → cj(t), lj(t), and sj(t) by (11) → θj(t) by (10) →Uj(t) by (9) → Yej(t) by (A12) → Kj(t) = Kij(t)+Kej(t) → K(t) by (23).
We simulate the case that the economy is composed of three regions. We specify the functions dependent on environmental quality as follows
Γmj(Ej(t),t)=E−bmj(t)j(t),Γj(Ej(t),t)=E−bj(t)j(t),j=1,2,3,m=i,e | (28) |
We require bji, bjs, bj ≥ 0 and bje ≤ 0. We specify transboundary pollution functions as:
Ωq((Eq(t)),t)=∑j,j≠qJθjq(t)Ej(t) | (29) |
The transboundary pollution functions imply that any region is also polluted by other two regions and the speed is linearly related to the pollutant levels of other regions. The rest of this section is concerned with the case that all the parameters are constant. This case is investigated by Zhang (2016) and we simply state the results which are the reference point for following the motion of the system when some parameters are time-dependent. We specify the parameters as follows:
N=20,λ0=0.75,ξ0=0.1,η0=0.1,d=−0.05,ω=−0.08,τij=τcj,θjq=0.01,j≠q,j,q=1,2,3,(L1L2L3)=(343),(Ai1Ai2Ai3)=(1.210.8),(Ae1Ae2Ae3)=(1.110.9),(αi1αi2αi3)=(0.310.310.31),(bi1bi2bi3)=(be1be2be3)=(0.010.020.01),(ˉθ1ˉθ2ˉθ3)=(0.080.10.11),(τk1τk2τk3)=(τw1τw2τw3)=(0.0050.0060.007),(˜αej˜βejτij)=(0.40.20.005),(bjθijθcj)=(0.010.080.03),(δk1δk2δk3)=(0.050.040.04) | (30) |
We plot the motion of the system under (30) with the following initial conditions:
z1(0)=0.038,ˉr(0)=1.42,ˉk1(0)=12.7,ˉk2(0)=11,ˉk3(0)=9.7,E1(0)=8.3,E2(0)=9.8,E3(0)=6.4. |
The motion of the variables is plotted in Figure 1.
From the plot we see that the system becomes stationary over time. This implies the existence of an equilibrium point. We list the equilibrium values as follows:
r=0.001,ˉr=1.46,K=219.7,(N1N2N3)=(3.458.607.95),(K1K2K3)=(49.98115.7280.71),(E1E2E3)=(8.059.926.52),(Fi1Fi2Fi3)=(9.0318.0912.57),(Ki1Ki2Ki3)=(42.46111.7177.59),(Ni1Ni2Ni3)=(3.428.547.88),(Fe1Fe2Fe3)=(0.420.990.78),(Ke1Ke2Ke3)=(1.524.013.12),(Ne1Ne2Ne3)=(0.0250.0690.071),(R1R2R3)=(1.943.233.49),(w1w2w3)=(1.811.461.1),(θ1θ2θ3)=(0.0640.0750.085),(ˉk1ˉk2ˉk3)=(12.711.39.92),(l1l2l3)=(0.870.470.38),(c1c2c3)=(1.681.501.32) | (31) |
It is straightforward to calculate the eigenvalues as follows
{−0.18±0.02I,−0.2,−0.15,−0.1,−0.07,−0.005,0}. |
The real parts of the seven eigenvalues are negative and one is zero. The unique equilibrium is locally stable. This implies the validity of conducting comparative dynamic analysis.
We simulated the motion of the national economy under (30). This section identifies business cycles by allowing various exogenous periodic shocks. We use ¯Δxj(t) to stand for the change rate of the variable, xj(t) in percentage due to changes in a parameter value.
We now study the effects of the following periodic oscillations in the national population:
N(t)=20+0.2sin(t). |
The simulation results are plotted in Figure 2. The change rates in the regional populations are regionally oscillatory. The per household consumption and wealth levels cross the regions are slightly affected, even though the other variables changes greatly in amplitude. The national economy experiences business cycles over time.
The population may affect amenity in different ways. In this study we consider that the population has negative impact on the amenity. We assume parameter d equal cross regions. We now examine the effects when the negative impact of the population is periodically perturbed as follows:
d(t)=−0.05+0.02sin(t). |
The simulation results are plotted in Figure 3. Although the regional variables change periodically, region 1's macro variables vary largely in amplitude.
We now examine the effects when the negative impact of pollutants on amenities is oscillating as follows: ω(t)=−0.08+0.04sin(t).
The simulation results are plotted in Figure 4. It is worthwhile to mention that the regional macroeconomic variables change, but the amplitudes are much smaller than the other two regions'. No region's microeconomic variables are strongly affected.
We now examine the effects of a rise in the MPR's tax rate on consumption as follows:
τc1(t)=0.005+0.004sin(t). |
The simulation results are plotted in Figure 5. The MPR spends more resource on purifying environment. The two input factors and output level of the MPR's sector are increased. The level of pollutants is reduced and the region's amenity is enhanced. As pollutants are reduced in the MPR, the productivity of the region is improved. The better environment attracts more people to live in the MPR. The lot size falls in the MPR and the land rent rises. The PR's and LPR's populations are enhanced. The lot sizes in the PR and the LPR fall. The two input factors and output levels of the PR's and LPR's two sectors are reduced. The levels of pollutants are enhanced and the regions' amenities are improved. The consumption and wealth levels in all the regions are improved. We see that a rise in the tax rate brings positive effects on all aspects of regional and national economies.
We now analyze the effects of the following oscillations in region 3's consumption on environment: θc3(t)=0.03+0.02sin(t).
The simulation results are plotted in Figure 6.
In growth theory effects of saving propensity changes are different in different theories. In Keynesian economic theory savings tend to reduce national income, while neoclassical growth theory claims the opposite effect. We study what happen to the economic system when the propensity to save is oscillated as follows: λ0(t)=0.75+0.03sin(t).
The simulation results are plotted in Figure 7. There are business cycles in both macro and economic variables when the propensity to save is oscillating.
This paper made a unique contribution to the literature of business cycles and multi-regional economic growth. As far as I am aware, there is almost mathematical economic model of business cycles with multiple regions and capital accumulation, and endogenous amenities which are built on microeconomic foundation. This paper showed how economic cycles appear across regions in association with spatial agglomeration and economic growth. It generalized the dynamic model of interdependence between regional economic growth and endogenous environment change proposed by Zhang (2016). The original model examined multi-regional dynamics with wealth and pollutant accumulation over time. The dynamics of J-region economy is described by differential equations. This paper generalized the model by making all exogenous constant parameters as exogenous time-dependent parameters. We demonstrate business cycles due to different exogenous periodic perturbations. The unique contribution to the literature of business cycles theory is that it demonstrates existence of business cycles in a multi-regional neoclassical growth model with endogenous environment built on microeconomic foundation. As the model takes account of various sources of tax, it is possible to study the impact of changes in the tax policy on growth and oscillations in the economic system. Although this study does not take account of dynamics of political parties in a democratic society, our results on oscillations in the tax policies which might be due to powershifts tell us that business cycles can also result from political competition. As the model is built on some well-established economic theories and each theory has a vast amount of literature, the model may be extended and generalized in multiple directions.
The author is grateful to the constructive comments of the two anonymous referees.
I, the author, declare that there is no conflict of interests regarding the publication of this paper.
[1] | J. A. Zhu, S. Dai, X. Y. Zhu, Characteristics of Electric Bike Accidents and Safety Enhancement Strategies, Urban Transp. China, 2018 (2018), 15–20. |
[2] | CNBN, Electric Bicycles Are Nearly 300 Million in China, Available from: http://news.cnr.cn/rebang/20211011/t20211011_525629773.shtml. |
[3] |
D. Zhang, T. F. Ren, M. M. Zhang, Y. C. Zheng, H. Y Zhou, Analysis and prevention of the causes of electric bicycle accidents based on safety checklists (in Chinese), Sci. Technol. Innovation, 07 (2021), 37–39. https://doi.org/10.15913/j.cnki.kjycx.2021.07.011 doi: 10.15913/j.cnki.kjycx.2021.07.011
![]() |
[4] |
H. Leijdesdorff, J. van Dijck, P. Krijnen, C. Vleggeert-Lankamp, I. Schipper, Injury pattern, hospital triage, and mortality of 1250 patients with severe traumatic brain injury caused by road traffic accidents, J. Neurotrauma, 31 (2014), 459–465. https://doi.org/10.1089/neu.2013.3111 doi: 10.1089/neu.2013.3111
![]() |
[5] |
M. F. Zavareh, A. M. Hezaveh, T. Nordfjærn, Intention to use bicycle helmet as explained by the Health Belief Model, comparative optimism and risk perception in an Iranian sample, Transp. Res. Part F Psychol. Behav., 54 (2018), 248–263. https://doi.org/10.1016/j.trf.2018.02.003 doi: 10.1016/j.trf.2018.02.003
![]() |
[6] |
J. Olivier, P. Creighton, Bicycle injuries and helmet use: a systematic review and meta-analysis, Int. J. Epidemiol., 46 (2017), 278–292. https://doi.org/10.1093/ije/dyw153 doi: 10.1093/ije/dyw153
![]() |
[7] |
Y. N. Song, W. W. Ma, J. Shen, J. G. Shen, Analysis of the relationship between helmet wearing and casualty among electric vehicle drivers (in Chinese), Urban Rural Enterp. Health China, 35 (2020), 7–9. https://doi.org/10.16286/j.1003-5052.2020.12.003 doi: 10.16286/j.1003-5052.2020.12.003
![]() |
[8] |
J. Kumphong, T. Satiennam, W. Satiennam, The determinants of motorcyclists helmet use: urban arterial road in Khon Kaen City, Thailand, J. Saf. Res., 67 (2018), 93–97. https://doi.org/10.1016/j.jsr.2018.09.011 doi: 10.1016/j.jsr.2018.09.011
![]() |
[9] |
N. Xu, N. Gao, J. H. Su, Y. Yan, D. D. Zhou, J. J. Peng, Investigation on knowledge, attitude and behavior of electric bicycle drivers and riders wearing safety helmets based on wechat public account (in Chinese), Shanghai J. Preventative Med., 30 (2018), 744–749. https://doi.org/10.19428/j.cnki.sjpm.2018.18803 doi: 10.19428/j.cnki.sjpm.2018.18803
![]() |
[10] |
C. X. Ma, D. Yang, J. B. Zhou, Z. X. Feng, Q. Yuan, Risk riding behaviors of urban e-bikes: a literature review, Int. J. Environ. Res. Public Health, 16 (2019), 2308. https://doi.org/10.3390/ijerph16132308 doi: 10.3390/ijerph16132308
![]() |
[11] | J. B. Zhou, Y. Y. Guo, Y. Wu, S. Dong, Assessing factors related to e-bike crash and e-bike license Plate Use, J. Transp. Syst. Eng. Inf. Technol., 17 (2017), 229–234. |
[12] |
L. T. Truong, H. T. T. Nguyen, C. De Gruyter, Mobile phone use among motorcyclists and electric bike riders: a case study of Hanoi, Vietnam, Accid. Anal. Prev., 91 (2016), 208–215. https://doi.org/10.1016/j.aap.2016.03.007 doi: 10.1016/j.aap.2016.03.007
![]() |
[13] |
N. Haworth, A. K. Debnath, How similar are two-unit bicycle and motorcycle crashes, Accid. Anal. Prev., 58 (2013), 15–25. https://doi.org/10.1016/j.aap.2013.04.014 doi: 10.1016/j.aap.2013.04.014
![]() |
[14] |
J. Zhou, T. Zheng, S. Dong, X. Mao, C. Ma, Impact of helmet-wearing policy on e-bike safety riding behavior: a bivariate ordered probit analysis in Ningbo, China, Int. J. Environ. Res. Public Health, 19 (2022), 2830. https://doi.org/10.3390/ijerph19052830 doi: 10.3390/ijerph19052830
![]() |
[15] |
X. S. Wang, J. Chen, M. Quddus, W. Zhou, M. Shen, Influence of familiarity with traffic regulations on delivery riders' e-bike crashes and helmet use: two mediator ordered logit models, Accid. Anal. Prev., 159 (2021), 106277. https://doi.org/10.1016/j.aap.2021.106277 doi: 10.1016/j.aap.2021.106277
![]() |
[16] | The Ministry of Public Security of the People's Republic of China, The Traffic Management Bureau of the Ministry of Public Security has deployed a "One Helmet, One Belt" security operation, 2020, Available from: http://www.gov.cn/xinwen/2020-04/21/content_5504613.htm. |
[17] |
M. Karkhaneh, Effectiveness of bicycle helmet legislation to increase helmet use: a systematic review, Inj. Prev., 12 (2006), 76–82. https://doi.org/10.1136/ip.2005.010942 doi: 10.1136/ip.2005.010942
![]() |
[18] | Guangzhou Public Security Bureau, In January, these cities in Guangdong had the lowest helmet wearing rate, 2021, Available from: https://baijiahao.baidu.com/s?id = 1691405130964298455 & wfr = spider & for = pc |
[19] |
T. Tang, H. Wang, B. Guo, Study on helmet wearing intention of electric bicycle riders (in Chinese), J. Transp. Eng. Inf., 20 (2022), 1–17. https://doi.org/10.19961/j.cnki.1672-4747.2021.11.024 doi: 10.19961/j.cnki.1672-4747.2021.11.024
![]() |
[20] |
B. Foroughi, P. V. Nhan, M. Iranmanesh, M. Ghobakhloo, M. Nilashi, E. Yadegaridehkordi, Determinants of intention to use autonomous vehicles: findings from PLS-SEM and ANFIS, J. Retailing Consum. Serv., 70 (2023), 103158. https://doi.org/10.1016/j.jretconser.2022.103158 doi: 10.1016/j.jretconser.2022.103158
![]() |
[21] |
Q. F. Li, O. Adetunji, C. V. Pham, N. T. Tran, E. Chan, A. M. Bachani, Helmet use among motorcycle riders in ho chi minh city, vietnam: results of a five-year repeated cross-sectional study, Accid. Anal. Prev., 144 (2020), 105642. https://doi.org/10.1016/j.aap.2020.105642 doi: 10.1016/j.aap.2020.105642
![]() |
[22] |
K. Brijs, T. Brijs, S. Sann, T. A. Trinh, G. Wets, R. A. C. Ruiter, Psychological determinants of motorcycle helmet use among young adults in Cambodia, Transp. Res. Part F Traffic Psychol. Behav., 26 (2014), 273–290. https://doi.org/10.1016/j.trf.2014.08.002 doi: 10.1016/j.trf.2014.08.002
![]() |
[23] |
Y. C. Ho, C. T. Tsai, Comparing ANFIS and SEM in linear and nonlinear forecasting of new product development performance, Expert Syst. Appl., 38 (2011), 6498–6507. https://doi.org/10.1016/j.eswa.2010.11.095 doi: 10.1016/j.eswa.2010.11.095
![]() |
[24] |
E. Yadegaridehkordi, M. Nilashi, M. H. N. B. M. Nasir, O. Ibrahim, Predicting determinants of hotel success and development using structural equation modelling (SEM)-ANFIS method, Tourism Manage., 66 (2018), 364–386. https://doi.org/10.1016/j.tourman.2017.11.012 doi: 10.1016/j.tourman.2017.11.012
![]() |
[25] |
B. Moon, Paradigms in migration research: exploring 'moorings' as a schema, Prog. Hum. Geogr., 19 (1995), 504–524. https://doi.org/10.1177/030913259501900404 doi: 10.1177/030913259501900404
![]() |
[26] |
J. K. Hsieh, Y. C. Hsieh, H. C. Chiu, Y. C. Feng, Post-adoption switching behavior for online service substitutes: a perspective of the push–pull–mooring framework, Comput. Hum. Behav., 28 (2012), 1912–1920. https://doi.org/10.1016/j.chb.2012.05.010 doi: 10.1016/j.chb.2012.05.010
![]() |
[27] |
Y. Sun, D. Liu, S. J. Chen, X. R. Wu, X. L. Shen, X. Zhang, Understanding users' switching behavior of mobile instant messaging applications: an empirical study from the perspective of push-pull-mooring framework, Comput. Hum. Behav., 75 (2017), 727–738. https://doi.org/10.1016/j.chb.2017.06.014 doi: 10.1016/j.chb.2017.06.014
![]() |
[28] |
J. Y. Lai, J. Wang, Switching attitudes of taiwanese middle-aged and elderly patients toward cloud healthcare services: an exploratory study, Technol. Forecasting Social Change, 92 (2015), 155–167. https://doi.org/10.1016/j.techfore.2014.06.004 doi: 10.1016/j.techfore.2014.06.004
![]() |
[29] |
H. S. Bansal, S. Taylor, Y. St. James, "Migrating" to new service providers: toward a unifying framework of consumers' switching behaviors, J. Acad. Mark. Sci., 33 (2005), 96–115. https://doi.org/10.1177/0092070304267928 doi: 10.1177/0092070304267928
![]() |
[30] |
S. Wang, J. Wang, F. Yang, From willingness to action: do push-pull-mooring factors matter for shifting to green transportation, Transp. Res. Part D Transp. Environ., 79 (2020), 102242. https://doi.org/10.1016/j.trd.2020.102242 doi: 10.1016/j.trd.2020.102242
![]() |
[31] |
H. Kim, The role of legal and moral norms to regulate the behavior of texting while driving, Transp. Res. Part F Traffic Psychol. Behav., 52 (2018), 21–31. https://doi.org/10.1016/j.trf.2017.11.004 doi: 10.1016/j.trf.2017.11.004
![]() |
[32] |
M. J. Paschall, J. W. Grube, S. Thomas, C. Cannon, R. Treffers, Relationships between local enforcement, alcohol availability, drinking norms, and adolescent alcohol use in 50 california cities, J. Stud. Alcohol Drugs, 73 (2012), 657–665. https://doi.org/10.15288/jsad.2012.73.657 doi: 10.15288/jsad.2012.73.657
![]() |
[33] | M. Limayem, S. G. Hirt, W. W. Chin, Intention does not always matter: the contingent role of habit on it usage behavior, in the 9th European Conference on Information Systems, 13 (2001). |
[34] |
C. Barbarossa, P. De Pelsmacker, Positive and negative antecedents of purchasing eco-friendly products: a comparison between green and non-green consumers, J. Bus. Ethics, 134 (2016), 229–247. https://doi.org/10.1007/s10551-014-2425-z doi: 10.1007/s10551-014-2425-z
![]() |
[35] |
A. Mehrabian, C. A. Stefl, Basic temperament components of loneliness, shyness, and conformity, Social Behav. Pers. Int. J., 23 (1995), 253–263. https://doi.org/10.2224/sbp.1995.23.3.253 doi: 10.2224/sbp.1995.23.3.253
![]() |
[36] |
R. Zhou, W. J. Horrey, Predicting adolescent pedestrians' behavioral intentions to follow the masses in risky crossing situations, Transp. Res. Part F Traffic Psychol. Behav., 13 (2010), 153–163. https://doi.org/10.1016/j.trf.2009.12.001 doi: 10.1016/j.trf.2009.12.001
![]() |
[37] |
T. P. Tang, Y. T. Guo, X. Z. Zhou, S. Labi, S. L. Zhu, Understanding electric bike riders' intention to violate traffic rules and accident proneness in China, Travel Behav. Soc., 23 (2021), 25–38. https://doi.org/10.1016/j.tbs.2020.10.010 doi: 10.1016/j.tbs.2020.10.010
![]() |
[38] |
P. Janmaimool, Application of protection motivation theory to investigate sustainable waste management behaviors, Sustainability, 9 (2017), 1079. https://doi.org/10.3390/su9071079 doi: 10.3390/su9071079
![]() |
[39] |
K. Chamroonsawasdi, S. Chottanapund, R. A. Pamungkas, P. Tunyasitthisundhorn, B. Sornpaisarn, O. Numpaisan, Protection motivation theory to predict intention of healthy eating and sufficient physical activity to prevent Diabetes Mellitus in Thai population: A path analysis, Diabetes Metab. Syndr. Clin. Res. Rev., 15 (2021), 121–127. https://doi.org/10.1016/j.dsx.2020.12.017 doi: 10.1016/j.dsx.2020.12.017
![]() |
[40] | L. Ajzen, From intentions to actions: a theory of planned behavior, in Action Control, Springer, (1985), 11–39. https://doi.org/10.1007/978-3-642-69746-3_2 |
[41] |
A. Shafiei, H. Maleksaeidi, Pro-environmental behavior of university students: application of protection motivation theory, Glob. Ecol. Conserv., 22 (2020), e00908. https://doi.org/10.1016/j.gecco.2020.e00908 doi: 10.1016/j.gecco.2020.e00908
![]() |
[42] |
R. Meade, W. Barnard, Conformity and anticonformity among Americans and Chinese, J. Social Psychol., 89 (1973), 15–24. https://doi.org/10.1080/00224545.1973.9922563 doi: 10.1080/00224545.1973.9922563
![]() |
[43] |
M. N. Borhan, A. N. H. Ibrahim, M. A. A. Miskeen, Extending the theory of planned behaviour to predict the intention to take the new high-speed rail for intercity travel in Libya: Assessment of the influence of novelty seeking, trust and external influence, Transp. Res. Part A Policy Pract., 130 (2019), 373–384. https://doi.org/10.1016/j.tra.2019.09.058 doi: 10.1016/j.tra.2019.09.058
![]() |
[44] |
L. Ross, T. Ross, S. Farber, C. Davidson, M. Trevino, A. Hawkins, The theory of planned behavior and helmet use among college students, Am. J. Health Behav., 35 (2011), 581–590. https://doi.org/10.5993/AJHB.35.5.7 doi: 10.5993/AJHB.35.5.7
![]() |
[45] |
S. O. Olsen, J. Scholderer, K. Brunsø, W. Verbeke, Exploring the relationship between convenience and fish consumption: A cross-cultural study, Appetite, 49 (2007), 84–91. https://doi.org/10.1016/j.appet.2006.12.002 doi: 10.1016/j.appet.2006.12.002
![]() |
[46] |
T. N. Nguyen, A. Lobo, S. Greenland, Pro-environmental purchase behaviour: the role of consumers' biospheric values, J. Retailing Consum. Serv., 33 (2016), 98–108. https://doi.org/10.1016/j.jretconser.2016.08.010 doi: 10.1016/j.jretconser.2016.08.010
![]() |
[47] | National Bureau of Statistics, China Statistical Yearbook (2022). Available from: http://www.stats.gov.cn/tjsj./ndsj/. |
[48] | SUHO.COM, Characteristics of electric bicycle traffic accidents and safety improvement measures, (2019). Available from: https://www.sohu.com/a/289681314_782444. |
[49] |
J. Mandhani, J. K. Nayak, M. Parida, Interrelationships among service quality factors of Metro Rail Transit System: An integrated Bayesian networks and PLS-SEM approach, Transp. Res. Part A Policy Pract., 140 (2020), 320–336. https://doi.org/10.1016/j.tra.2020.08.014 doi: 10.1016/j.tra.2020.08.014
![]() |
[50] |
T. F. Golob, Structural equation modeling for travel behavior research, Transp. Res. Part B Methodol., 37 (2003), 1–25. https://doi.org/10.1016/S0191-2615(01)00046-7 doi: 10.1016/S0191-2615(01)00046-7
![]() |
[51] |
J. Henseler, G. Hubona, P. A. Ray, Using PLS path modeling in new technology research: updated guidelines, Ind. Manage. Data Syst., 116 (2016), 2–20. https://doi.org/10.1108/IMDS-09-2015-0382 doi: 10.1108/IMDS-09-2015-0382
![]() |
[52] |
A. Leguina, A primer on partial least squares structural equation modeling (PLS-SEM), Int. J. Res. Method Educ., 38 (2015), 220–221. https://doi.org/10.1080/1743727X.2015.1005806 doi: 10.1080/1743727X.2015.1005806
![]() |
[53] |
J. F. Hair Jr, M. Sarstedt, L. Hopkins, V. Kuppelwieser, Partial least squares structural equation modeling (PLS-SEM): An emerging tool in business research, Eur. Bus. Rev., 26 (2014), 106–121. https://doi.org/10.1108/EBR-10-2013-0128 doi: 10.1108/EBR-10-2013-0128
![]() |
[54] |
M. Tenenhaus, V. E. Vinzi, Y. M. Chatelin, C. Lauro, PLS path modeling, Comput. Stat. Data Anal., 48 (2005), 159–205. https://doi.org/10.1016/j.csda.2004.03.005 doi: 10.1016/j.csda.2004.03.005
![]() |
[55] | J. R. Jang, ANFIS: adaptive-network-based fuzzy inference system, IEEE Trans. Syst. Man Cybern., 23 (1993), 665–685. |
[56] | D. Nauck, F. Klawonn, R. Kruse, Foundation of Neuro-fuzzy Systems, Wiley, 1997. |
[57] |
S. Zhang, P. Jing, D. Yuan, C. Yang, On parents' choice of the school travel mode during the covid-19 pandemic, Math. Biosci. Eng., 19 (2022), 9412–9436. https://doi.org/10.3934/mbe.2022438 doi: 10.3934/mbe.2022438
![]() |
[58] |
W. Chin, A. Gopal, W. D. Salisbury, Advancing the theory of adaptive structuration: the development of a scale to measure faithfulness of appropriation, Inf. Syst. Res., 8 (1997), 342–367. https://doi.org/10.1287/isre.8.4.342 doi: 10.1287/isre.8.4.342
![]() |
[59] |
C. Fornell, D. F. Larcker, Evaluating structural equation models with unobservable variables and measurement error. J. Mark. Res., 18 (1981), 39–50. https://doi.org/10.2307/3151312 doi: 10.2307/3151312
![]() |
[60] |
A. García-Ferrer, A. de Juan, P. Poncela, Forecasting traffic accidents using disaggregated data. Int. J. Forecasting, 22 (2006), 203–222. https://doi.org/10.1016/j.ijforecast.2005.11.001 doi: 10.1016/j.ijforecast.2005.11.001
![]() |
[61] |
H. Zhou, S. B. Romero, X. Qin, An extension of the theory of planned behavior to predict pedestrians' violating crossing behavior using structural equation modeling, Accid. Anal. Prev., 95 (2016), 417–424. https://doi.org/10.1016/j.aap.2015.09.009 doi: 10.1016/j.aap.2015.09.009
![]() |
1. | Bodo Herzog, Optimal sequencing to reform the European economic and Monetary Union: a roadmap, 2021, 5, 2573-0134, 311, 10.3934/QFE.2021014 |