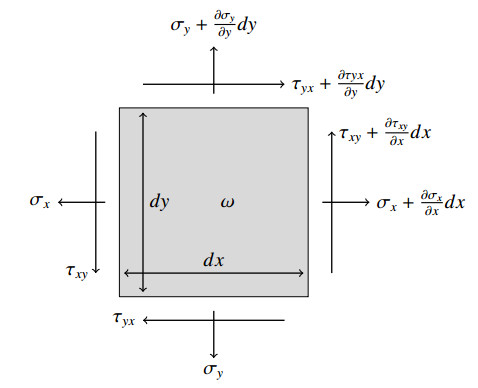
Preventive identification of mechanical parts failures has always played a crucial role in machine maintenance. Over time, as the processing cycles are repeated, the machinery in the production system is subject to wear with a consequent loss of technical efficiency compared to optimal conditions. These conditions can, in some cases, lead to the breakage of the elements with consequent stoppage of the production process pending the replacement of the element. This situation entails a large loss of turnover on the part of the company. For this reason, it is crucial to be able to predict failures in advance to try to replace the element before its wear can cause a reduction in machine performance. Several systems have recently been developed for the preventive faults detection that use a combination of low-cost sensors and algorithms based on machine learning. In this work the different methodologies for the identification of the most common mechanical failures are examined and the most widely applied algorithms based on machine learning are analyzed: Support Vector Machine (SVM) solutions, Artificial Neural Network (ANN) algorithms, Convolutional Neural Network (CNN) model, Recurrent Neural Network (RNN) applications, and Deep Generative Systems. These topics have been described in detail and the works most appreciated by the scientific community have been reviewed to highlight the strengths in identifying faults and to outline the directions for future challenges.
Citation: Giuseppe Ciaburro. Machine fault detection methods based on machine learning algorithms: A review[J]. Mathematical Biosciences and Engineering, 2022, 19(11): 11453-11490. doi: 10.3934/mbe.2022534
[1] | Ricardo Almeida . Variational problems of variable fractional order involving arbitrary kernels. AIMS Mathematics, 2022, 7(10): 18690-18707. doi: 10.3934/math.20221028 |
[2] | Yongjian Hu, Huifeng Hao, Xuzhou Zhan . On the solvability of the indefinite Hamburger moment problem. AIMS Mathematics, 2023, 8(12): 30023-30037. doi: 10.3934/math.20231535 |
[3] | Xiaojing Du, Xiaotong Liang, Yonghong Xie . Integral expressions of solutions to higher order λ-weighted Dirac equations valued in the parameter dependent Clifford algebra. AIMS Mathematics, 2025, 10(1): 1043-1060. doi: 10.3934/math.2025050 |
[4] | Hong Li, Keyu Zhang, Hongyan Xu . Solutions for systems of complex Fermat type partial differential-difference equations with two complex variables. AIMS Mathematics, 2021, 6(11): 11796-11814. doi: 10.3934/math.2021685 |
[5] | Kun Li, Peng Wang . Properties for fourth order discontinuous differential operators with eigenparameter dependent boundary conditions. AIMS Mathematics, 2022, 7(6): 11487-11508. doi: 10.3934/math.2022640 |
[6] | Valérie Gauthier-Umaña, Henryk Gzyl, Enrique ter Horst . Decoding as a linear ill-posed problem: The entropy minimization approach. AIMS Mathematics, 2025, 10(2): 4139-4152. doi: 10.3934/math.2025192 |
[7] | Tuba Gulsen, Emrah Yilmaz, Ayse Çiğdem Yar . Proportional fractional Dirac dynamic system. AIMS Mathematics, 2024, 9(4): 9951-9968. doi: 10.3934/math.2024487 |
[8] | Yong Liu, Chaofeng Gao, Shuai Jiang . On meromorphic solutions of certain differential-difference equations. AIMS Mathematics, 2021, 6(9): 10343-10354. doi: 10.3934/math.2021599 |
[9] | Clara Burgos, Juan Carlos Cortés, Elena López-Navarro, Rafael Jacinto Villanueva . Probabilistic analysis of linear-quadratic logistic-type models with hybrid uncertainties via probability density functions. AIMS Mathematics, 2021, 6(5): 4938-4957. doi: 10.3934/math.2021290 |
[10] | Noureddine Bahri, Abderrahmane Beniani, Abdelkader Braik, Svetlin G. Georgiev, Zayd Hajjej, Khaled Zennir . Global existence and energy decay for a transmission problem under a boundary fractional derivative type. AIMS Mathematics, 2023, 8(11): 27605-27625. doi: 10.3934/math.20231412 |
Preventive identification of mechanical parts failures has always played a crucial role in machine maintenance. Over time, as the processing cycles are repeated, the machinery in the production system is subject to wear with a consequent loss of technical efficiency compared to optimal conditions. These conditions can, in some cases, lead to the breakage of the elements with consequent stoppage of the production process pending the replacement of the element. This situation entails a large loss of turnover on the part of the company. For this reason, it is crucial to be able to predict failures in advance to try to replace the element before its wear can cause a reduction in machine performance. Several systems have recently been developed for the preventive faults detection that use a combination of low-cost sensors and algorithms based on machine learning. In this work the different methodologies for the identification of the most common mechanical failures are examined and the most widely applied algorithms based on machine learning are analyzed: Support Vector Machine (SVM) solutions, Artificial Neural Network (ANN) algorithms, Convolutional Neural Network (CNN) model, Recurrent Neural Network (RNN) applications, and Deep Generative Systems. These topics have been described in detail and the works most appreciated by the scientific community have been reviewed to highlight the strengths in identifying faults and to outline the directions for future challenges.
The step-length-based techniques and the trust region methods are two types of optimization methods for the least-squares problem (LSP): 12‖F(x)‖2, where F={f1,f2,…,fN}T is the vector of the non-linear functions, M is the number of unknowns x, (M≤N).
The Newton method and the Gauss-Newton (GN) method are frequently utilized for solving LSPs, belong to the step-length-based category [1,2]. The Newton approach calculates the least-squares function's optimum descent direction, resulting in a faster rate of convergence. However, for determining the descent direction, one needs to obtain the precise derivatives of the least-squares function and the time consuming. On the other hand, the GN method approximates the derivatives using a simple approach that is relatively straightforward to evaluate, however the solution may not converge owing to the over-simplification of the derivatives, which may result in an incorrect descent direction. The Levenberg-Marquardt method (LMM) was introduced by Levenberg [3] and Marquardt [4] for solving the (LSP) using a trust region approach [5,6]. In the GN method, the simplified Hessian matrix HGN is not always invertible, to avoid this problem a modified positive definite Hessian HLM=HGN+Sk, is usually used. The LMM takes Sk=βkIN×M, where βk represents the Levenberg-Marquardt parameter which is a scalar quantity that changes during optimization and IN×M is the identity matrix. The gradient descent method and the GN method are combined in the LM algorithm. When the parameters are far from their ideal value (βk increases) the LM process conducts as a gradient-descent method, while it works as the GN method when the parameters are close to their optimal value (βk decreases) [4,7,8,9,10]. Many researchers have expressed interest in Newton-type methods for solving inverse problems because of their rapid convergence for the well-posed problems. The LMM is known to have a quadratic rate of convergence under non singularity conditions [6,11,12,13,14,15]. Yamashita et al. [6], investigated the solution of a nonlinear system of equations F(x)=0 under the assumption ‖F(x)‖, which provides a local error bound, where the convergence rate is still quadratic when βk=‖F(xk)‖2. Khayat [12] has established the quadratic convergence of the LMM for the inverse problem of identifying a Robin coefficient R for the Stokes system in the case where R is a piecewise constant on some non-accessible part of the boundaryand the velocity of a given reference solution does not vanish on the boundary. In this case, the regularizing parameter is defined by βk=‖U(Rk)−Zη‖2, where Zη is the noisy observed data.
Elasticity imaging is a relatively new medical technique used to detect several pathologies and especially cancerous tumors. These pathologies are known to affect the elastic properties (elastic modulus, Poisson's ratio and stiffness, …, etc.) of soft tissues.
Many authors have dealt with the elasticity imaging inverse problem (EIIP). Mei et al. [16] have estimated the elastic modulus from surface displacements during multiple observations. Raghavan et al. [17] have solved the equilibrium equations using finite difference schemes to recover elastic properties. Doyley et al. [18] applied a modified Newton-Raphson method. A variational method based on error functional decomposition was applied by Constantinescu [19] for anisotropic elastic body. Oberai et al. [20] reconstructed the identification parameter using a first-order adjoint method. Jadamba et al. [21] have reconstructed the Lamé parameters using a conjugate gradient trust-region technique. Arnold et al. [22] have utilized a numerical clustering procedure to identify the Young's modulus. Abdelhamid et al. [23] have investigated the identification problem of reconstructing the modulus of elasticity using the nonlinear conjugate gradient method. Mohammadi et al. [24] have used a statistical technique combined with a fixed-point algorithm. In this study we introduce an interesting and rapid converging method (the LMM) for solving the inverse problem of the elasticity imaging inverse problems. This method has the particularity to transform a nonlinear and a non-convex optimization into a convex one. Recently, the LMM is used in a closely related problems in the field. Indeed, Raja et al. [25] have combined the LMM with artificial neural networks to study the thermal radiation and Hall effect on boundary layer flow. Shoaib et al. [26] have used the same method to study the influence of activation energy on the Third-Grade Nanofluid flow. In the following, a mathematical treatment of the LMM method and an exhaustive numerical study are done. A realistic examples are treated using the proposed procedure.
Worldwide, about 19.3 million new cancer cases, where 18.1 million of them excluding nonmelanoma skin cancerand almost 10.0 million cancer deaths occurred in 2020 [27]. It has estimated that about 18.1 million new cancer cases recorded and 9.6 million cancer deaths in 2018, across 20 world regions [28]. In the literature the soft tissue can be modeled as an incompressible, isotropicand continuous elastic object. The linear elasticity system is introduced to simulate the external and tractional forces on the soft tissue. In this study, the Young's modulus E is defined as a function of the position (x,y) and Poisson's ratio is a given constant ν (ν≈0.48). Reconstructing E of the elasticity imaging inverse problem is important for characterizing the complex mechanical behaviour of the soft tissue. We derive the basic equations for the theory of elasticity. A free body diagram (FBD) is a diagrammatic depiction of a single body or a subsystem of bodies that is separated from its surroundings and depicts all the forces operating on it as shown in Figure 1.
Let ω be an infinitesimal element [29,30], the FBD of ω is represented in Figure 1 and the stresses components are shown as positive. Summation of the forces in the x and x+dx axes at equilibrium are introduced as follows:
∑Fx=(σx+∂σx∂x)dxdy−σxdxdy+(τxy+∂τxy∂y)dxdy−τxydxdy+fxdxdy=0, | (2.1) |
we do the same to the y and y+dy axes:
∑Fy=(τxy+∂τxy∂x)dxdy−τxydxdy+(σy+∂σy∂y)dxdy−σydxdy+fydxdy=0. | (2.2) |
We denote by fx and fy the body forces in x- and y-axes which are generally assumed to be positive when acted along the positive axes, simplifying Eqs (2.1) and (2.2) we derive the basic equations of the theory of elasticity bellow
∂σx∂x+∂τxy∂y+fx=0, | (2.3) |
∂τxy∂x+∂σy∂y+fy=0. | (2.4) |
The stresses-strains relationship (for an isotropic material) is defined by
(σ)=[D](ε), | (2.5) |
where (σ)=(σx,σy,τxy)T denotes the stress and (ε)=(εx,εy,γxy)T is called small strain tensor:
εij(u)=12(∂ui∂xj+∂uj∂xi). |
εx=ε11(u)=∂u∂x,εy=ε22(u)=∂v∂y,γxy=2ε12(u)=2ε21(u)=∂u∂y+∂v∂x. |
Where, u=(u,v) represents the displacement field. Here, Eq (2.5) equivalent to
(σxσyτxy)=E[D](εxεyγxy). | (2.6) |
An approximation of the plane stress situation can be used to approximate the body's deformation, for this reason we take the material property matrix D as follow
Dσ=ρ1[1v0v1000ρ2],such thatρ1=11−ν2, ρ2=1−ν2. | (2.7) |
Where, E and ν are the Young's modulus and the Poisson's ratio, ρ1 and ρ2, are given constants depend on ν.
A tumor can be identified by investigating the elastic properties of the soft tissue such as Young's modulus and Poisson ratio from a given measurements. The following forward system of partial differential equations describes the response of an elastic object, when the external body forces and traction are applied to the boundary. Let Ω⊂Rd, for d=2,3, be an open bounded and connected domain such that ∂Ω=Γi∪Γc. Consider the following elasticity problem with essential (Dirichlet) and natural (Neumann) boundary conditions, governing the displacement u=(u,v) in x and y directions when the body forces f=(fx,fy) are applied to Ω:
(DP):{∂σx∂x+∂τxy∂y+fx=0inΩ, ∂τxy∂x+∂σy∂y+fy=0inΩ, σxnx+τxyny=qxonΓi, τxynx+σyny=qyonΓi, (u,v)=(u0,v0)onΓc. | (2.8) |
On the section of the boundary designated by Γi (left, right and top), the traction vectors q=(qx,qy) are specified as Neumann boundary conditions, n=(nx,ny) its unit outward normal, while Γc (bottom) is taken as constant Dirichlet boundary condition. Young's modulus E is considered as a function of location E(x,y) and Poisson's ratio is given as a constant (i.e., ν=0.48). The test function space, represented by W, is defined by
W={w:(w1,w2)∈H1(Ω):w=0 onΓc}. | (2.9) |
To determine the weak formulation of the linear elasticity Eq (2.8), we apply the weighted residual method by multiplying Eq (2.8) by a test function and integrate over Ω, such that:
∫Ω(w1(∂σx∂x+∂τxy∂y)w2(∂τxy∂x+∂σy∂y))dΩ+∫Ω(w1fxw2fy)dΩ=0. | (2.10) |
By utilizing the Green's identity, using the integration by parts for the first term of the Eq (2.10) and using the boundary conditions we get:
−∫Ω(∂w1∂xσx+∂w1∂yτxy∂w2∂xτxy+∂w2∂yσy)dΩ+∫Ω(w1fxw2fy)dΩ+∫Γi(w1qxw2qy)dΓi=0. | (2.11) |
The variational formulation of Eq (2.8) can be rewritten as follows:
∫Ω(∂w1∂x0∂w1∂y0∂w2∂y∂w2∂x)(σxσyτxy)dΩ=∫Ω(w1fxw2fy)dΩ+∫Γi(w1qxw2qy)dΓi. | (2.12) |
Finally, using Eqs (2.6) and (2.7), the forward problem can be solved to find the displacement field (u,v):
∫Ω(∂w1∂x0∂w1∂y0∂w2∂y∂w2∂x)EDσ(∂u∂x∂v∂y∂u∂y+∂v∂x)dΩ=∫Ω(w1fxw2fy)dΩ+∫Γi(w1qxw2qy)dΓi. | (2.13) |
The body deformation represents the solution of the forward problem to find the displacement field on the problem domain. This deformation helps in solving the elasticity imaging inverse problem of identifying the elastic properties from the available measurements on subdomain of the problem.
Let X and Y two Hilbert spaces, J:D(J)⊂X⟶Y. Consider the system of nonlinear equations as a continuously differentiable function:
J(x)=0. | (3.1) |
Assume that Eq (3.1) has a solution set K∗ that is not empty. The system (3.1) may not have a solution, i.e., J(x) is not necessarily invertible. To remedy this, we replace Eq (3.1) by the following minimization problem:
minx∈KJ(x)=minx∈K{12‖J(x)‖2}. | (3.2) |
The LMM is a Newton type technique for solving nonlinear least squares problems [4,8,9,10]. Particularly, LMM is a kind of GN technique for reducing a least squares cost 12‖J(x)‖2. In its purest form, the GN technique effectively takes in each iteration:
xk+1=xk+dk, |
where the direction of descent dk is
[∇J(xk)∇J(xk)′]dk=∇J(xk)J(xk). | (3.3) |
As in the GN method, the operator ∇J(xk)∇J(xk)′ is not always non-singular. So, the matrix is substituted by the following positive definite matrix:
J(xk)∇J(xk)′+Sk. |
Here Sk is the identity matrix I multiplied by βk (Sk=βkI) which is selected as a positive multiple and it is chosen so that the modified matrix is always non-singular. This method has been widely used because of its simplicity. It is used also to have a quadratic convergence rate under some suitable hypothesis.
Remark 1. This is equivalent to the problem of unconstrained minimization:
mind∈XOk(d), | (3.4) |
where Ok:X⟶R is a strictly convex function defined by:
Ok(d)=‖J′(xk)d+J(xk)‖2+βk‖d‖2. |
First we must define the set of admissible coefficients set for E:
K={E∈H1(Ω):E0≤E(x,y)≤E1<∞,a.e.inΩ}, | (3.5) |
where E0 and E1 are two positive constants.
We are concerned in this section with the inverse problem of identifying E on Ω from measurements available on Γi :
(PI){Find E(x,y)∈K s.t. u=(u,v)∈H1(Ω)×H1(Ω) is the solution of Eq(2.8). | (3.6) |
For analyzing inverse problems, the usual output least-squares approach is:
J(E)=12‖u(E)−zη‖2L2(Ω). | (3.7) |
We assume the noise level in the observation data zη=(zu,zv) of the true solution u of the system (2.8) is of order η. Hence
‖u(˜E)−zη‖L2(Ω)≤η, | (3.8) |
where ˜E is the exact solution of Eq (3.6). We transform the linear elasticity imaging inverse problem of identifying Young's modulus E to the following stable minimization with Tikhonov regularization,
minE∈KJ(E); andJ(E)=12‖u(E)−zη‖2L2(Ω)+β‖E−˜E‖2L2(Ω). | (3.9) |
where ‖E−˜E‖2L2(Ω) is the Tikhonov regularization term and β is the regularization parameter. The LMM iteration is obtained by following the formality as explained above.
Ek+1=argminE∈KJ(E),=argminE∈K{‖u(Ek)−zη‖2L2(Γ0)+μk‖E−Ek‖2L2(Γout)}. | (3.10) |
We assume that the identification parameter E(x,y) is perturbed by a small amount E+δE, where δE can be any direction in L∞(Ω).
u(E+δE)≈u(E)+u′(E)δE+O(‖δE‖2L∞(Ω)), |
and
v(E+δE)≈v(E)+v′(E)δE+O(‖δE‖2L∞(Ω)). |
Let (u1,v1)≡(u′(E)δE,v′(E)δE)∈L2(Ω)×L2(Ω) the Fréchet derivative at direction δE of the forward solution u(E)=(u(E),v(E)) in Eq (2.8). Abdelhamid et al. [23] obtained the sensitivity problem of elasticity as follows:
(SP):{∂σ1x∂x+∂τ1xy∂y=−(∂˜σx∂x+∂˜τxy∂y),inΩ ∂τ1xy∂x+∂σ1y∂y=−(∂˜τxy∂x+∂˜σy∂y),inΩ σ1xnx+τ1xyny=−(˜σxnx+˜τxyny),onΓi τ1xynx+σ1yny=−(˜τxynx+˜σyny),onΓi u1=v1=u0.onΓc | (3.11) |
For the sensitivity Eq (3.11), we may describe the relationship between stresses and strains as follows:
(σ1x σ1y τ1xy)=EDσ(∂u1∂x ∂v1∂x ∂u1∂y+∂v1∂x). | (3.12) |
The right-hand side of Eq (3.11) can be rewritten similarly:
(˜σx ˜σy ˜τxy)=δEDσ(∂u∂x ∂v∂x ∂u∂y+∂v∂x). | (3.13) |
Now, we'll complete the sensitivity problem's variational formulation:
−∫Ω(σ1x∂w1∂x+τ1xy∂w1∂yτ1xy∂w2∂x+σ1y∂w2∂y)dΩ+∫Ω(w1(∂˜σx∂x+∂˜τxy∂y)w2(∂˜τxy∂x+∂˜σy∂y))dΩ−∫Γi(w1(˜σxnx+˜τxyny)w2(˜τxynx+˜σyny))dΓi=0. | (3.14) |
We get the following result as we use the integration by parts for the second term of (3.14):
−∫Ω(σ1x∂w1∂x+τ1xy∂w1∂yτ1xy∂w2∂x+σ1y∂w2∂y)dΩ−∫Ω(˜σx∂w1∂x+˜τxy∂w1∂y˜τxy∂w2∂x+˜σy∂w2∂y)dΩ=0. | (3.15) |
After that,
∫Ω(σ1x∂w1∂x+∣τ1xy∂w1∂yτ1xy∂w2∂x+σ1y∂w2∂y)dΩ=−∫Ω(˜σx∂w1∂x+˜τxy∂w1∂y˜τxy∂w2∂x+˜σy∂w2∂y)dΩ. | (3.16) |
Using Eqs (3.12) and (3.13), the Eq (3.16) can be rewritten in the following manner:
∫Ω(∂w1∂x0∂w1∂y0∂w2∂y∂w2∂x)EDσ(∂u1∂x∂v1∂y∂u1∂y+∂v1∂x)dΩ=−∫Ω(∂w1∂x0∂w1∂y0∂w2∂y∂w2∂x)δEDσ(∂u∂x∂v∂y∂u∂y+∂v∂x)dΩ. | (3.17) |
where Dσ refers to the material property of the applied plane stress condition.
The Lagrangian multipliers L are introduced first in this section. Consider the following problem:
{minE∈KJ(u,v,E), subject tocx(u,v,E)=0inΩ,cy(u,v,E)=0inΩ,B⋅Ccx=0onΓi,B⋅Ccy=0onΓi. | (3.18) |
The state equations in the x and y directions are represented by cx and cy, respectively. Consequently, the Neumann boundary conditions on Γi corresponding to cx and cy are defined by B.Ccx and B.Ccy, respectively. The Lagrangian may be shown as:
L(u,v,E,λu,λv)=J(u,v,E)+∫Ωλucx(u,v,E)dΩ+∫Ωλvcy(u,v,E)dΩ−∫Γi(λuB⋅Ccx+λvB⋅Ccy)dΓi. | (3.19) |
The Lagrange multiplier is represented with (λu,λv). To find the adjoint equation, the differential of L must be computed:
∂L∂uδu=∂J∂uδu+∫Ωλu∂∂u(∂σx∂x+∂τxy∂y+fx−(σxnx+τxyny))δudΩ+∫Ωλv∂∂u(∂τxy∂x+∂σy∂y+fy−(τxynx+σyny))δudΩ=0, | (3.20) |
and
∂L∂vδv=∂J∂vδv+∫Ωλu∂∂v(∂σx∂x+∂τxy∂y+fx−(σxnx+τxyny))δvdΩ+∫Ωλv∂∂v(∂τxy∂x+∂σy∂y+fy−(τxynx+σyny))δvdΩ=0. | (3.21) |
By subtracting Eq (3.21) from Eq (3.20) and two times utilizing integration par part we obtain the adjoint problem:
(AP):{∂σ∗x∂x+∂τ∗xy∂y=−ρ1[∂∂x(E(∂(u−zu)∂x+v∂(v−zv)∂y))+ρ2∂∂y(E(∂(u−zu)∂y+∂(v−zv)∂x))]inΩ, ∂τ∗xy∂x+∂σ∗y∂y=−ρ1[ρ2∂∂x(E(∂(u−zu)∂y+∂(v−zv)∂x))+∂∂y(E(v∂(u−zu)∂x+∂(v−zv)∂y))]inΩ, σ∗xnx+τ∗xyny=0inΓi, τ∗xynx+σ∗yny=0onΓi, λu=λv=λ0onΓc. | (3.22) |
where (u,v) solves the original forward problem of elasticity (2.8) and λ0 is the initial condition. The relationship between stresses σ∗ and the adjoint solution can be shown as follows:
(σ∗x σ∗y τ∗xy)=EDσ(∂λu∂x∂λv∂y∂λu∂y+∂λv∂x). | (3.23) |
Using the conditions to the limits in Eq (3.22) and the relation Eq (3.23), we obtain the variational formulation of Eq (3.22):
Ω(∂w1∂x0∂w1∂y0∂w2∂y∂w2∂x)EDσ(∂λu∂x∂λv∂y∂λu∂y+∂λv∂x)dΩ=−∫Ω(ρ1E(∂(u−zu)∂x+v∂(v−zv)∂y)∂w1∂x+ρ1ρ2E(∂(u−zu)∂y+∂(v−zv)∂x)∂w1∂yρ1ρ2E(∂(u−zu)∂y+∂(v−zv)∂x)∂w2∂x+ρ1E(v∂(u−zu)∂x+∂(v−zv)∂y)∂w2∂y)dΩ. | (3.24) |
This section explains how to use the LMM to solve the proposed optimization inverse problem. Table 1 introduces the specifications of different algorithms compared with the LMM in terms of the convergence and computation complexity.
Algorithms | Convergence | Computation Complexity |
Gradient descent | Stable, slow | Gradient |
Newton | Unstable, fast | Gradient and Hessian |
Gauss-Newton | Unstable, fast | Jacobian |
Levenberg-Marquardt | Stable, fast | Jacobian |
According to the study done above, the different steps for computing the Young's modulus E(x,y) are given by the following algorithm:
Algorithm 1: |
1. Initialization (1) Choose the initial value E0. (2) Choose the observation data zη=(zu,zv) and its noise order η>0. (3) Choose a tolerance parameter ϵ>0. (4) Set k:=0. 2. Solve (1) Forward problem (2.8) with E=Ek. (2) Sensitivity problem (3.11) with E=Ek. (3) Adjoint problem (3.22) with E=Ek. 3. Calculate: Using the current value of Ek βk=‖u(Ek)−zη‖2L2(Ω) 4. Compute the descent direction dk: [u′(Ek)⋆u′(Ek)+βkI]dk=−u′(Ek)⋆[u(Ek)−zη] 5. Update the identification parameter Ek+1: Ek+1:=Ek+dk. 6. if: ∥Ek+1−Ek∥2L2(Ω)∥Ek∥2L2(Ω)≤ϵ then ![]() |
The Young's modulus E(x,y) can be reconstructed using this process. For each iteration with E(x,y)=E(x,y)k the algorithm necessitates the resolution of the forward problem, sensitivity problem and the adjoint problem then calculate the regularization parameter βk and the descent direction dk, next updating the identification parameter E, finally we verify the stopping criteria, the estimated parameter must be accurate enough or satisfies the discrepancy principle.
Let us consider a two dimensional square domain [0,1]×[0,1] with Γc=[0,1]×{y=0} and Γi is the remaining part of the boundary ∂Ω. In order to identify a smooth E(x,y), we give numerical examples to prove our study's effectiveness. All calculations are made using Freefem++4.6 [31]. CPU: Intel(R) Core(TM) i7-4510U CPU @ 2.00GHz, 2601 MHz, 2 cores, 4 processors, Memory: 8 GB. We generate data zη=(zu,zv)∈L2(Ω) experimentally by:
zη=u(x,y)(1+ηξ)onΩ, | (5.1) |
where η is the amount of noise and ξ is a uniformly distributed random variable in [−1,1], then using the FreeFem function Rand1(⋅) we generate this random variable. The exact solution is synthetically generated using the fundamental of elasticity problem:
u(x,y)=(u,v)=(xy,xy). | (5.2) |
The body forces f=(fx,fy) and the traction q=(qx,qy) applied on its boundaries can be derived directly on borders Γi.
Due to changes in sample composition and test technique, Young's modulus might vary somewhat. The constant values presented here [32,33,34,35] are approximated and solely intended for relative comparison.
Example 1. We consider a constant Young's modulus for various materials such as Aluminum, Titanium, …, etc.
Table 2 presents the reconstruction of E at a number of elements NE=1800, η=1% for different materials at constant initial guesses. The obtained results show the rapid convergence and the precision of the introduced procedure.
Material | EExact | E0 | ECalculated | k | eE |
Aluminium (13Al) | 68 | 70.25 | 67.9904 | 25 | 0.0096133 |
Titanium (22Ti) | 116 | 115 | 116.011 | 13 | 0.0109185 |
Bronze | 112 | 113 | 112.250 | 13 | 0.0066388 |
Zinc (30Zn) | 108 | 110.25 | 108.007 | 25 | 0.0074127 |
Nylon (66) | 2.93 | 2.5 | 2.92746 | 6 | 0.0025417 |
Figure 2 shows the convergence speed of the proposed LMM at different levels of noise η in the measurement data for the Titanium material with initial guess E0=115. Figure 3 introduces the convergence speed at η=1% and η=2% for the Nylon material with initial guess E0=2.5. It is observed that the best convergence speed is obtained for low level of noise in the data. At large level of noise, the proposed algorithm takes more than 50 iterations to get the optimal solution of the reconstructed parameter. Table 3 presents the decline of the relative error eE, until the convergence occurs at the 13th iteration.
k | eE | k | eE | k | eE |
0 | 1 | 5 | 0.729589 | 10 | 0.311645 |
1 | 0.925356 | 6 | 0.662241 | 11 | 0.116378 |
2 | 0.857706 | 7 | 0.595521 | 12 | 0.042788 |
3 | 0.809445 | 8 | 0.553536 | 13 | 0.010918 |
4 | 0.768314 | 9 | 0.493262 |
Example 2. The exact identification of the Young's modulus is defined by:
E(x,y)=3+0.05e(x+y)2inΩ. | (5.3) |
In this Example the initial guess is given by E0=3.3.
Table 4 presents the variation of the relative error eE with respect to the noise level in the data. We notice that as the amounts of noise η rises, eE rises as well at chosen NE=1800. However, this relative error remains interesting upto η=1.5%. We also remark on this table that the solution of the inverse problem fails for η>2.75%. Figure 4 shows the variation of the residual error (left) and the relative error (right) with respect to the number of iterations k. We remark that these errors suddenly decrease upto k=10 and then they remain almost constant and independent of k.
NE | η(%) | k | eE | NE | η(%) | k | eE |
1800 | 0.25 | 19 | 0.0012 | 1800 | 1.75 | 09 | 0.011 |
1800 | 0.50 | 10 | 0.0031 | 1800 | 2.00 | 05 | 0.019 |
1800 | 0.75 | 09 | 0.0044 | 1800 | 2.25 | 03 | 0.041 |
1800 | 1.00 | 07 | 0.0058 | 1800 | 2.50 | 06 | 0.053 |
1800 | 1.25 | 09 | 0.0070 | 1800 | 2.75 | 03 | 0.068 |
1800 | 1.50 | 09 | 0.0074 | 1800 | >2.75 | fail! | fail! |
Figures 5(a), (b) and 6 show the exact (left) and reconstructed (middle) Ek for Example 2 in 2D and 3D at η=0.5% and NE=20,000. The obtained results are satisfactory at k=9, where the relative error eE=0.0032. Figures 5(c) and 6 (right) show the residual error of the coefficient Ek. It is observed that the relative error grows at a part of the boundary of the problem domain. We have also examined the procedure for different values of NE. Figures 7 and 8 show the exact (left) and reconstructed (middle) Ekand residual error (right) at η=0.5% for NE=9800 and 1800 respectively. Figure 9 represents the variations and sensitivity of the measured data zη with respect to the noise level.
Example 3. The exact coefficient is given by:
E(x,y)=1+0.51+e50((0.6−x)2+(0.3−y)2)−3+0.31+e100((0.4−x)2+(0.75−y)2)−3inΩ, | (5.4) |
with initial guess E0=1.1.
Table 5 shows the numerical convergence for Ek with respect to the number of iterations k. We observe that the relative and the residual errors drop rapidly until the 8th iteration. Then they decline slowly as shown in Figure 10. Figure 11 depicts the exact (left), reconstructed (middle) Ek and residual error (right) for η=0.5% at iterations k=1,2,4,6and8.
k | eE | k | eE | k | eE |
1 | 0.103244 | 7 | 0.019356 | 13 | 0.003495 |
2 | 0.078826 | 8 | 0.010968 | 14 | 0.003495 |
3 | 0.050282 | 9 | 0.006122 | 15 | 0.003693 |
4 | 0.040450 | 10 | 0.004676 | 16 | 0.002940 |
5 | 0.031286 | 11 | 0.003857 | 17 | 0.002623 |
6 | 0.025083 | 12 | 0.002983 | 18 | 0.002521 |
Figures 12(a), (b) and 13 show the exact (left) and reconstructed (middle) Ek for Example 3 in 2D and 3D at η=0.5% and NE=20,000. It is observed that the reconstructed modulus of elasticity Ek has a very satisfying relative error (eE=0.0025) at k=18. Figures 12(c) and 13 (right) show the residual error of Ek for Example 3 in 2D and 3D. Jadamba et al. [36] have implemented both first and second-order adjoint methods and the Newton method with a simple backtracking line search method for solving Example 3. They found that it is possible to achieve a good precision at the 139th iteration (for the first-order adjoint method) as well as at the 49th iteration (for the second-order adjoint method), then decreased very slowly with increasing k. These results are in a good accord with the literature. Furthermore, Abdelhamid et al. [23] implemented the nonlinear conjugate gradient method for solving this example observing that at the first 25th iteration. The relative and residual errors are decreasing rapidly with increasing k, after which they decrease very slow to reach the minimizer at k=34 with relative error eE=0.0128. It is found that the LMM gives better results in less number of iterations k.
Example 4. The modulus of elasticity coefficient E(x,y), body force function f(x,y) and boundary conditions are as follows:
E(x,y)=1−12sinc[6π(x+110)(y+110)],f(x,y)=[−15xcos(πx)]inΩ.g(x,y)=110[sin(πy)sin(πx)]onΓ1andh(x,y)=110[1+10x1+10y]onΓ2, | (5.5) |
where ∂Ω=Γ1∪Γ2, Γ1 represents the bottom and left sides of the border and Γ2 represents the top and the right edges.
Remark 2. The sinc function also called the "sampling function" defined as following:
sinc(x)={1forx=0,sinxxOtherwise. |
For this example, the suggested method is tested with η=0.5% and NE=20,000. Table 6 shows that the relative error eE slowly decreases with increasing k. We reach to the minimizer and the stopping criteria is satisfied at k=19. Figure 14 shows that the relative error eE reduces rapidly as the number of iterations k increases for the first 10 iterations and then slowly approach to the minimizer.
k | eE | k | eE | k | eE | k | eE |
1 | 0.14524 | 6 | 0.06519 | 11 | 0.02621 | 16 | 0.00397 |
2 | 0.13081 | 7 | 0.05603 | 12 | 0.01979 | 17 | 0.00591 |
3 | 0.11370 | 8 | 0.04337 | 13 | 0.17039 | 18 | 0.00287 |
4 | 0.09535 | 9 | 0.03191 | 14 | 0.00910 | 19 | 0.00278 |
5 | 0.07731 | 10 | 0.02882 | 15 | 0.00701 |
Figures 15(a), (b) and 16 represent the exact (left) and the reconstructed (middle) Ek for Example 4 in 2D and 3D respectively for η=0.5% and NE=20,000. Figure 17 shows the same representation for NE=9800. The proposed method represents a reasonable results at k=19 for which the relative error is eE=0.0028. Figures 15(c) and 16 (right) introduces the residual error of eE for Example 4 in 2D and 3D. It is observed that the eE grows at the corners of the boundary due to the influence of the gradient computations.
Figure 18 gives a graphical representation of the exact, the reconstructed Ek and the residual error at k=5,12,15and17, the method converges rapidly to the solution in the first 10th iterations, then gives a good reconstruction Ek in the 19th iteration as shown in Figures 15–17 and Table 6. Table 7 introduces a comparison of the relative eE, residual error E and the number of iterations k using the present work compared to that of Abdelhamid et al. [23].
k | Relative error eE | Residual error E | |
Abdelhamid et al. [23], NE=12,800 | 67 | 0.038095 | 0.036702 |
Present work, NE=9800 | 23 | 0.003532 | 0.005026 |
In this paper we develop a theoretical framework for the inverse problem of identifying the modulus of elasticity E at some measurement data on the boundary. The inverse problem is discretized using the finite element approach. The optimization problem of reconstructing the elastic modulus (Young's modulus) of elasticity imaging inverse problem is formulated. The identification parameter is defined on the domain and can be identified from given measurement data at some parts of the boundaries. The LMM method is used to treat this ill-posed inverse problem and the non-convex minimization is changed into a convex one. The mathematical formulation for the forward problem of elasticity is introduced in the 2D plane, where Ω⊂Rd,d=2,3. The descent direction dk is introduced from the solution of the sensitivity and adjoint equations. The obtained results from the identification problem of the constant and variable identification parameter for various real materials are satisfactory. The obtained results show the accuracy and efficiency of the proposed algorithm. The obtained results of the 2D and 3D view for the reconstruction of the Young's modulus are compared with those obtained from the exact one. At different levels of noise, the proposed algorithm is implemented and show efficient and accurate results upto η=2.75%.
The work of Talaat Abdelhamid is supported by Science, Technology & Innovation Funding Authority (STDF) under grant number 39385.
The authors declare that there is no conflict of interests regarding the publication of this paper.
[1] |
A. Muller, A. C. Marquez, B. Iung, On the concept of e-maintenance: Review and current research, Reliab. Eng. Syst. Saf., 93 (2008), 1165–1187. https://doi.org/10.1016/j.ress.2007.08.006 doi: 10.1016/j.ress.2007.08.006
![]() |
[2] | K. Gandhi, A. H. Ng, Machine maintenance decision support system: a systematic literature review, in Advances in Manufacturing Technology XXXⅡ: Proceedings of the 16th International Conference on Manufacturing Research, incorporating the 33rd National Conference on Manufacturing Research, September 11–13, University of Skö vde, IOS Press, Sweden, 8 (2018), 349. |
[3] |
A. Garg, S. G. Deshmukh, Maintenance management: literature review and directions, J. Qual. Maint. Eng., 12 (2006), 205–238. https://doi.org/10.1108/13552510610685075 doi: 10.1108/13552510610685075
![]() |
[4] |
D. Sherwin, A review of overall models for maintenance management, J. Qual. Maint. Eng., 6 (2000), 138–164. https://doi.org/10.1108/13552510010341171 doi: 10.1108/13552510010341171
![]() |
[5] | K. C. Ng, G. G. G. Goh, U. C. Eze, Critical success factors of total productive maintenance implementation: a review, in 2011 IEEE international conference on industrial engineering and engineering management, IEEE, Singapore, 269–273. https://doi.org/10.1109/IEEM.2011.6117920 |
[6] |
E. Sisinni, A. Saifullah, S. Han, U. Jennehag, M. Gidlund, Industrial internet of things: Challenges, opportunities, and directions, IEEE Trans. Ind. Inf., 14 (2018), 4724–4734. https://doi.org/10.1109/TⅡ.2018.2852491 doi: 10.1109/TⅡ.2018.2852491
![]() |
[7] |
H. Boyes, B. Hallaq, J. Cunningham, T. Watson, The industrial internet of things (ⅡoT): An analysis framework, Comput. Ind., 101 (2018), 1–12. https://doi.org/10.1016/j.compind.2018.04.015 doi: 10.1016/j.compind.2018.04.015
![]() |
[8] |
J. Wan, S. Tang, Z. Shu, D. Li, S. Wang, M. Imran, et al., Software-defined industrial internet of things in the context of industry 4.0, IEEE Sens. J., 16 (2016), 7373–7380. https://doi.org/10.1109/JSEN.2016.2565621 doi: 10.1109/JSEN.2016.2565621
![]() |
[9] |
Y. Liao, E. D. F. R. Loures, F. Deschamps, Industrial Internet of Things: A systematic literature review and insights, IEEE Internet Things J., 5 (2018), 4515–4525. https://doi.org/10.1109/JIOT.2018.2834151 doi: 10.1109/JIOT.2018.2834151
![]() |
[10] | M. Hartmann, B. Halecker, Management of innovation in the industrial internet of things, in The International Society for Professional Innovation Management ISPIM Conference Proceedings, 2015. |
[11] | M. Mohri, A. Rostamizadeh, A. Talwalkar, Foundations of Machine Learning, MIT press, 2018. |
[12] | C. Sammut, G. I. Webb, Encyclopedia of Machine Learning, Springer Science & Business Media, 2011. |
[13] |
G. Carleo, I. Cirac, K. Cranmer, L. Daudet, M. Schuld, N. Tishby, et al., Machine learning and the physical sciences, Rev. Mod. Phys., 91 (2019), 045002. https://doi.org/10.1103/RevModPhys.91.045002 doi: 10.1103/RevModPhys.91.045002
![]() |
[14] |
M. Du, N. Liu, X. Hu, Techniques for interpretable machine learning, Commun. ACM, 63 (2019), 68–77. https://doi.org/10.1145/3359786 doi: 10.1145/3359786
![]() |
[15] | H. Sahli, An introduction to machine learning, in TORUS 1-Toward an Open Resource Using Services: Cloud Computing for Environmental Data, (2020), 61–74. https://doi.org/10.1002/9781119720492.ch7 |
[16] |
R. H. P. M. Arts, G. M. Knapp, L. Mann, Some aspects of measuring maintenance performance in the process industry, J. Qual. Maint. Eng., 4 (1998) 6–11. https://doi.org/10.1108/13552519810201520 doi: 10.1108/13552519810201520
![]() |
[17] |
C. Stenströ m, P. Norrbin, A. Parida, U. Kumar, Preventive and corrective maintenance-cost comparison and cost-benefit analysis, Struct. Infrastruct. Eng., 12 (2016), 603–617. https://doi.org/10.1080/15732479.2015.1032983 doi: 10.1080/15732479.2015.1032983
![]() |
[18] |
H. P. Bahrick, L. K. Hall, Preventive and corrective maintenance of access to knowledge, Appl. Cognit. Psychol., 5 (1991), 1–18. https://doi.org/10.1002/acp.2350050102 doi: 10.1002/acp.2350050102
![]() |
[19] |
J. Shin, H. Jun, On condition based maintenance policy, J. Comput. Des. Eng., 2 (2015), 119–127. https://doi.org/10.1016/j.jcde.2014.12.006 doi: 10.1016/j.jcde.2014.12.006
![]() |
[20] |
R. Ahmad, S. Kamaruddin, An overview of time-based and condition-based maintenance in industrial application, Comput. Ind. Eng., 63 (2012), 135–149. https://doi.org/10.1016/j.cie.2012.02.002 doi: 10.1016/j.cie.2012.02.002
![]() |
[21] | J. H. Williams, A. Davies, P. R. Drake, Condition-Based Maintenance and Machine Diagnostics, Springer Science & Business Media, 1994. |
[22] | R. K. Mobley, An Introduction to Predictive Maintenance, 2nd edition, Elsevier, 2002. https://doi.org/10.1016/B978-0-7506-7531-4.X5000-3 |
[23] | C. Scheffer, P. Girdhar, Practical Machinery Vibration Analysis and Predictive Maintenance, Elsevier, 2004. |
[24] |
K. Efthymiou, N. Papakostas, D. Mourtzis, G. Chryssolouris, On a predictive maintenance platform for production systems, Procedia CIRP, 3 (2012), 221–226. https://doi.org/10.1016/j.procir.2012.07.039 doi: 10.1016/j.procir.2012.07.039
![]() |
[25] |
G. A. Susto, A. Schirru, S. Pampuri, S. McLoone, A. Beghi, Machine learning for predictive maintenance: A multiple classifier approach, IEEE Trans. Ind. Inf., 11 (2014), 812–820. https://doi.org/10.1109/TⅡ.2014.2349359 doi: 10.1109/TⅡ.2014.2349359
![]() |
[26] | R. Isermann, Fault-Diagnosis Systems: An Introduction from Fault Detection to Fault Tolerance, Springer Science & Business Media, 2005. |
[27] |
Z. Gao, C. Cecati, S. X. Ding, A survey of fault diagnosis and fault-tolerant techniques—Part I: Fault diagnosis with model-based and signal-based approaches, IEEE Trans. Ind. Electron., 62 (2015), 3757–3767. https://doi.org/10.1109/TIE.2015.2417501 doi: 10.1109/TIE.2015.2417501
![]() |
[28] |
S. Leonhardt, M. Ayoubi, Methods of fault diagnosis, Control Eng. Pract., 5 (1997), 683–692. https://doi.org/10.1016/S0967-0661(97)00050-6 doi: 10.1016/S0967-0661(97)00050-6
![]() |
[29] | R. J. Patton, P. M. Frank, R. N Clark, Issues of Fault Diagnosis for Dynamic Systems, Springer Science & Business Media, 2013. |
[30] |
M. I. Jordan, T. M. Mitchell, Machine learning: Trends, perspectives, and prospects, Science, 349 (2015), 255–260. https://doi.org/10.1126/science.aaa8415 doi: 10.1126/science.aaa8415
![]() |
[31] | U. S. Shanthamallu, A. Spanias, C. Tepedelenlioglu, M. Stanley, A brief survey of machine learning methods and their sensor and IoT applications, in 2017 8th International Conference on Information, Intelligence, Systems & Applications (ⅡSA), IEEE, (2017), 1–8. https://doi.org/10.1109/ⅡSA.2017.8316459 |
[32] | D. A. Pisner, D. M. Schnyer, Support vector machine, in Machine Learning, Academic Press, (2020), 101–121. https://doi.org/10.1016/B978-0-12-815739-8.00006-7 |
[33] |
W. S. Noble, What is a support vector machine, Nat. Biotechnol., 24 (2006), 1565–1567. https://doi.org/10.1038/nbt1206-1565 doi: 10.1038/nbt1206-1565
![]() |
[34] | L. Wang, Support Vector Machines: Theory and Applications, Springer Science & Business Media, 2005. https://doi.org/10.1007/b95439 |
[35] |
S. I. Amari, S. Wu, Improving support vector machine classifiers by modifying kernel functions, Neural Networks, 12 (1999), 783–789. https://doi.org/10.1016/S0893-6080(99)00032-5 doi: 10.1016/S0893-6080(99)00032-5
![]() |
[36] | O. L. Mangasarian, D. R. Musicant, Lagrangian support vector machines, J. Mach. Learn. Res., 1 (2001), 161–177. |
[37] |
A. Widodo, B. S. Yang, Support vector machine in machine condition monitoring and fault diagnosis, Mech. Syst. Sig. Process., 21 (2007), 2560–2574. https://doi.org/10.1016/j.ymssp.2006.12.007 doi: 10.1016/j.ymssp.2006.12.007
![]() |
[38] |
S. W. Fei, X. B. Zhang, Fault diagnosis of power transformer based on support vector machine with genetic algorithm, Expert Syst. Appl., 36 (2009), 11352–11357. https://doi.org/10.1016/j.eswa.2009.03.022 doi: 10.1016/j.eswa.2009.03.022
![]() |
[39] |
S. D. Wu, P. H. Wu, C. W. Wu, J. J. Ding, C. C. Wang, Bearing fault diagnosis based on multiscale permutation entropy and support vector machine, Entropy, 14 (2012), 1343–1356. https://doi.org/10.3390/e14081343 doi: 10.3390/e14081343
![]() |
[40] | W. Aziz, M. Arif, Multiscale permutation entropy of physiological time series, in 2005 Pakistan Section Multitopic Conference, IEEE, (2005), 1–6. https://doi.org/10.1109/INMIC.2005.334494 |
[41] |
B. Tang, T. Song, F. Li, L. Deng, Fault diagnosis for a wind turbine transmission system based on manifold learning and Shannon wavelet support vector machine, Renewable Energy, 62 (2014), 1–9. https://doi.org/10.1016/j.renene.2013.06.025 doi: 10.1016/j.renene.2013.06.025
![]() |
[42] |
Z. Wang, L. Yao, Y. Cai, J. Zhang, Mahalanobis semi-supervised mapping and beetle antennae search based support vector machine for wind turbine rolling bearings fault diagnosis, Renewable Energy, 155 (2020), 1312–1327. https://doi.org/10.1016/j.renene.2020.04.041 doi: 10.1016/j.renene.2020.04.041
![]() |
[43] |
L. Yao, Z. Fang, Y. Xiao, J. Hou, Z. Fu, An intelligent fault diagnosis method for lithium battery systems based on grid search support vector machine, Energy, 214 (2021), 118866. https://doi.org/10.1016/j.energy.2020.118866 doi: 10.1016/j.energy.2020.118866
![]() |
[44] |
Y. P. Zhao, J. J. Wang, X. Y. Li, G. J. Peng, Z. Yang, Extended least squares support vector machine with applications to fault diagnosis of aircraft engine, ISA Trans., 97 (2020), 189–201. https://doi.org/10.1016/j.isatra.2019.08.036 doi: 10.1016/j.isatra.2019.08.036
![]() |
[45] |
F. Marini, B. Walczak, Particle swarm optimization (PSO). A tutorial, Chemom. Intell. Lab. Syst., 149 (2015), 153–165. https://doi.org/10.1016/j.chemolab.2015.08.020 doi: 10.1016/j.chemolab.2015.08.020
![]() |
[46] |
M. Van, D. T. Hoang, H. J. Kang, Bearing fault diagnosis using a particle swarm optimization-least squares wavelet support vector machine classifier, Sensors, 20 (2020), 3422. https://doi.org/10.3390/s20123422 doi: 10.3390/s20123422
![]() |
[47] |
X. Li, S. Wu, X. Li, H. Yuan, D. Zhao, Particle swarm optimization-support vector machine model for machinery fault diagnoses in high-voltage circuit breakers, Chin. J. Mech. Eng., 33 (2020), 1–10. https://doi.org/10.1186/s10033-019-0428-5 doi: 10.1186/s10033-019-0428-5
![]() |
[48] |
Y. Fan, C. Zhang, Y. Xue, J. Wang, F. Gu, A bearing fault diagnosis using a support vector machine optimised by the self-regulating particle swarm, Shock Vib., 2020 (2020). https://doi.org/10.1155/2020/9096852 doi: 10.1155/2020/9096852
![]() |
[49] | E. Mirakhorli, Fault diagnosis in a distillation column using a support vector machine based classifier, Int. J. Smart Electr. Eng., 8 (2020), 105–113. |
[50] |
S. Gao, C. Zhou, Z. Zhang, J. Geng, R. He, Q. Yin, C. Xing, Mechanical fault diagnosis of an on-load tap changer by applying cuckoo search algorithm-based fuzzy weighted least squares support vector machine, Math. Probl. Eng., 2020 (2020). https://doi.org/10.1155/2020/3432409 doi: 10.1155/2020/3432409
![]() |
[51] |
X. Huang, X. Huang, B. Wang, Z. Xie, Fault diagnosis of transformer based on modified grey wolf optimization algorithm and support vector machine, IEEJ Trans. Electr. Electron. Eng., 15 (2020), 409–417. https://doi.org/10.1002/tee.23069 doi: 10.1002/tee.23069
![]() |
[52] |
Y. Zhang, J. Li, X. Fan, J. Liu, H. Zhang, Moisture prediction of transformer oil-immersed polymer insulation by applying a support vector machine combined with a genetic algorithm, Polymers, 12 (2020), 1579. https://doi.org/10.3390/polym12071579 doi: 10.3390/polym12071579
![]() |
[53] |
Y. Liu, H. Chen, L. Zhang, X. Wu, X. J. Wang, Energy consumption prediction and diagnosis of public buildings based on support vector machine learning: A case study in China, J. Cleaner Prod., 272 (2020), 122542. https://doi.org/10.1016/j.jclepro.2020.122542 doi: 10.1016/j.jclepro.2020.122542
![]() |
[54] |
S. K. Ibrahim, A. Ahmed, M. A. E. Zeidan, I. E. Ziedan, Machine learning techniques for satellite fault diagnosis, Ain Shams Eng. J., 11 (2020), 45–56. https://doi.org/10.1016/j.asej.2019.08.006 doi: 10.1016/j.asej.2019.08.006
![]() |
[55] |
Y. P. Zhao, G. Huang, Q. K. Hu, B. Li, An improved weighted one class support vector machine for turboshaft engine fault detection, Eng. Appl. Artif. Intell., 94 (2020), 103796. https://doi.org/10.1016/j.engappai.2020.103796 doi: 10.1016/j.engappai.2020.103796
![]() |
[56] | M. Guo, L. Xie, S. Q. Wang, J. M. Zhang, Research on an integrated ICA-SVM based framework for fault diagnosis, in SMC'03 Conference Proceedings. 2003 IEEE International Conference on Systems, Man and Cybernetics. Conference Theme-System Security and Assurance (Cat. No. 03CH37483), IEEE, 3 (2003), 2710–2715. https://doi.org/10.1109/ICSMC.2003.1244294 |
[57] | S. Poyhonen, P. Jover, H. Hyotyniemi, Signal processing of vibrations for condition monitoring of an induction motor, in First International Symposium on Control, Communications and Signal Processing, IEEE, Tunisia, (2004), 499–502. https://doi.org/10.1109/ISCCSP.2004.1296338 |
[58] |
M. C. Moura, E. Zio, I. D. Lins, E. Droguett, Failure and reliability prediction by support vector machines regression of time series data, Reliab. Eng. Syst. Saf., 96 (2011), 1527–1534. https://doi.org/10.1016/j.ress.2011.06.006 doi: 10.1016/j.ress.2011.06.006
![]() |
[59] |
K. Y. Chen, L. S. Chen, M. C. Chen, C. L. Lee, Using SVM based method for equipment fault detection in a thermal power plant, Comput. Ind., 62 (2011), 42–50. https://doi.org/10.1016/j.compind.2010.05.013 doi: 10.1016/j.compind.2010.05.013
![]() |
[60] |
K. He, X. Li, A quantitative estimation technique for welding quality using local mean decomposition and support vector machine, J. Intell. Manuf., 27 (2016), 525–533. https://doi.org/10.1007/s10845-014-0885-8 doi: 10.1007/s10845-014-0885-8
![]() |
[61] |
K. Yan, C. Zhong, Z. Ji, J. Huang, Semi-supervised learning for early detection and diagnosis of various air handling unit faults, Energy Build., 181 (2018), 75–83. https://doi.org/10.1016/j.enbuild.2018.10.016 doi: 10.1016/j.enbuild.2018.10.016
![]() |
[62] |
Z. Yin, J. Hou, Recent advances on SVM based fault diagnosis and process monitoring in complicated industrial processes, Neurocomputing, 174 (2016), 643–650. https://doi.org/10.1016/j.neucom.2015.09.081 doi: 10.1016/j.neucom.2015.09.081
![]() |
[63] |
M. M. Islam, J. M. Kim, Reliable multiple combined fault diagnosis of bearings using heterogeneous feature models and multiclass support vector Machines, Reliab. Eng. Syst. Saf., 184 (2019), 55–66. https://doi.org/10.1016/j.ress.2018.02.012 doi: 10.1016/j.ress.2018.02.012
![]() |
[64] |
R. P. Monteiro, M. Cerrada, D. R. Cabrera, R. V. Sánchez, C. J. Bastos-Filho, Using a support vector machine based decision stage to improve the fault diagnosis on gearboxes, Comput. Intell. Neurosci., 2019 (2019). https://doi.org/10.1155/2019/1383752 doi: 10.1155/2019/1383752
![]() |
[65] |
D. Yang, J. Miao, F. Zhang, J. Tao, G. Wang, Y. Shen, Bearing fault diagnosis using a support vector machine optimized by an improved ant lion optimizer, Shock Vib., 2019 (2019). https://doi.org/10.1155/2019/9303676 doi: 10.1155/2019/9303676
![]() |
[66] |
S. Mirjalili, The ant lion optimizer, Adv. Eng. Software, 83 (2015), 80–98. https://doi.org/10.1016/j.advengsoft.2015.01.010 doi: 10.1016/j.advengsoft.2015.01.010
![]() |
[67] |
L. You, W. Fan, Z. Li, Y. Liang, M. Fang, J. Wang, A fault diagnosis model for rotating machinery using VWC and MSFLA-SVM based on vibration signal analysis, Shock Vib., 2019 (2019). https://doi.org/10.1155/2019/1908485 doi: 10.1155/2019/1908485
![]() |
[68] |
A. Kumar, R. Kumar, Time-frequency analysis and support vector machine in automatic detection of defect from vibration signal of centrifugal pump, Measurement, 108 (2017), 119–133. https://doi.org/10.1016/j.measurement.2017.04.041 doi: 10.1016/j.measurement.2017.04.041
![]() |
[69] |
Z. Chen, F. Zhao, J. Zhou, P. Huang, X. Zhang, Fault diagnosis of loader gearbox based on an Ica and SVM algorithm, Int. J. Environ. Res. Public Health, 16 (2019), 4868. https://doi.org/10.3390/ijerph16234868 doi: 10.3390/ijerph16234868
![]() |
[70] | T. W. Lee, Independent component analysis, in Independent Component Analysis, Springer, Boston, (1998), 27–66. https://doi.org/10.1007/978-1-4757-2851-4_2 |
[71] |
W. Liu, Z. Wang, J. Han, G. Wang, Wind turbine fault diagnosis method based on diagonal spectrum and clustering binary tree SVM, Renewable Energy, 50 (2013), 1–6. https://doi.org/10.1016/j.renene.2012.06.013 doi: 10.1016/j.renene.2012.06.013
![]() |
[72] |
M. A. Djeziri, O. Djedidi, N. Morati, J. L. Seguin, M. Bendahan, T. Contaret, A temporal-based SVM approach for the detection and identification of pollutant gases in a gas mixture, Appl. Intell., 52 (2022), 6065–6078. https://doi.org/10.1007/s10489-021-02761-0 doi: 10.1007/s10489-021-02761-0
![]() |
[73] |
G. Ciaburro, G. Iannace, J. Passaro, A. Bifulco, D. Marano, M. Guida, et al., Artificial neural network-based models for predicting the sound absorption coefficient of electrospun poly (vinyl pyrrolidone)/silica composite, Appl. Acoust., 169 (2020), 107472. https://doi.org/10.1016/j.apacoust.2020.107472 doi: 10.1016/j.apacoust.2020.107472
![]() |
[74] |
S. Agatonovic-Kustrin, R. Beresford, Basic concepts of artificial neural network (ANN) modeling and its application in pharmaceutical research, J. Pharm. Biomed. Anal., 22 (2000), 717–727. https://doi.org/10.1016/S0731-7085(99)00272-1 doi: 10.1016/S0731-7085(99)00272-1
![]() |
[75] |
G. Ciaburro, G. Iannace, M. Ali, A. Alabdulkarem, A. Nuhait, An artificial neural network approach to modelling absorbent asphalts acoustic properties, J. King Saud Univ. Eng. Sci., 33 (2021), 213–220. https://doi.org/10.1016/j.jksues.2020.07.002 doi: 10.1016/j.jksues.2020.07.002
![]() |
[76] |
J. Misra, I. Saha, Artificial neural networks in hardware: A survey of two decades of progress, Neurocomputing, 74 (2010), 239–255. https://doi.org/10.1016/j.neucom.2010.03.021 doi: 10.1016/j.neucom.2010.03.021
![]() |
[77] |
Z. Zhang, K. Friedrich, Artificial neural networks applied to polymer composites: a review, Compos. Sci. Technol., 63 (2003), 2029–2044. https://doi.org/10.1016/S0266-3538(03)00106-4 doi: 10.1016/S0266-3538(03)00106-4
![]() |
[78] |
G. Iannace, G. Ciaburro, A. Trematerra, Modelling sound absorption properties of broom fibers using artificial neural networks, Appl. Acoust., 163 (2020), 107239. https://doi.org/10.1016/j.apacoust.2020.107239 doi: 10.1016/j.apacoust.2020.107239
![]() |
[79] |
K. P. Singh, A. Basant, A. Malik, G. Jain, Artificial neural network modeling of the river water quality—a case study, Ecol. Modell., 220 (2009), 888–895. https://doi.org/10.1016/j.ecolmodel.2009.01.004 doi: 10.1016/j.ecolmodel.2009.01.004
![]() |
[80] |
H. Zhu, X. Li, Q. Sun, L. Nie, J. Yao, G. Zhao, A power prediction method for photovoltaic power plant based on wavelet decomposition and artificial neural networks, Energies, 9 (2015), 1–15. https://doi.org/10.3390/en9010011 doi: 10.3390/en9010011
![]() |
[81] |
V. P. Romero, L. Maffei, G. Brambilla, G. Ciaburro, Modelling the soundscape quality of urban waterfronts by artificial neural networks, Appl. Acoust., 111 (2016), 121–128. https://doi.org/10.1016/j.apacoust.2016.04.019 doi: 10.1016/j.apacoust.2016.04.019
![]() |
[82] |
S. Fabio, D. N. Giovanni, P. Mariano, Airborne sound insulation prediction of masonry walls using artificial neural networks, Build. Acoust., 28 (2021), 391–409. https://doi.org/10.1177/1351010X21994462 doi: 10.1177/1351010X21994462
![]() |
[83] |
Y. Zhang, X. Ding, Y. Liu, P. J. Griffin, An artificial neural network approach to transformer fault diagnosis, IEEE Trans. Power Delivery, 11 (1996), 1836–1841. https://doi.org/10.1109/61.544265 doi: 10.1109/61.544265
![]() |
[84] |
J. C. Hoskins, K. M. Kaliyur, D. M. Himmelblau, Fault diagnosis in complex chemical plants using artificial neural networks, AIChE J., 37 (1991), 137–141. https://doi.org/10.1002/aic.690370112 doi: 10.1002/aic.690370112
![]() |
[85] |
J. B. Ali, N. Fnaiech, L. Saidi, B. Chebel-Morello, F. Fnaiech, Application of empirical mode decomposition and artificial neural network for automatic bearing fault diagnosis based on vibration signals, Appl. Acoust., 89 (2015), 16–27. https://doi.org/10.1016/j.apacoust.2014.08.016 doi: 10.1016/j.apacoust.2014.08.016
![]() |
[86] |
T. Sorsa, H. N. Koivo, Application of artificial neural networks in process fault diagnosis, Automatica, 29 (1993), 843–849. https://doi.org/10.1016/0005-1098(93)90090-G doi: 10.1016/0005-1098(93)90090-G
![]() |
[87] |
N. Saravanan, K. I. Ramachandran, Incipient gear box fault diagnosis using discrete wavelet transform (DWT) for feature extraction and classification using artificial neural network (ANN), Expert Syst. Appl., 37 (2010), 4168–4181. https://doi.org/10.1016/j.eswa.2009.11.006 doi: 10.1016/j.eswa.2009.11.006
![]() |
[88] |
W. Chine, A. Mellit, V. Lughi, A. Malek, G. Sulligoi, A. M. Pavan, A novel fault diagnosis technique for photovoltaic systems based on artificial neural networks, Renewable Energy, 90 (2016), 501–512. https://doi.org/10.1016/j.renene.2016.01.036 doi: 10.1016/j.renene.2016.01.036
![]() |
[89] |
B. Li, M. Y. Chow, Y. Tipsuwan, J. C. Hung, Neural-network-based motor rolling bearing fault diagnosis, IEEE Trans. Ind. Electron., 47 (2000), 1060–1069. https://doi.org/10.1109/41.873214 doi: 10.1109/41.873214
![]() |
[90] |
B. Samanta, K. R. Al-Balushi, S. A. Al-Araimi, Artificial neural networks and genetic algorithm for bearing fault detection, Soft Comput., 10 (2006), 264–271. https://doi.org/10.1007/s00500-005-0481-0 doi: 10.1007/s00500-005-0481-0
![]() |
[91] |
T. Han, B. S. Yang, W. H. Choi, J. S. Kim, Fault diagnosis system of induction motors based on neural network and genetic algorithm using stator current signals, Int. J. Rotating Mach., 2006 (2006). https://doi.org/10.1155/IJRM/2006/61690 doi: 10.1155/IJRM/2006/61690
![]() |
[92] |
H. Wang, P. Chen, Intelligent diagnosis method for rolling element bearing faults using possibility theory and neural network, Comput. Ind. Eng., 60 (2011), 511–518. https://doi.org/10.1016/j.cie.2010.12.004 doi: 10.1016/j.cie.2010.12.004
![]() |
[93] |
M. A. Hashim, M. H. Nasef, A. E. Kabeel, N. M. Ghazaly, Combustion fault detection technique of spark ignition engine based on wavelet packet transform and artificial neural network, Alexandria Eng. J., 59 (2020), 3687–3697. https://doi.org/10.1016/j.aej.2020.06.023 doi: 10.1016/j.aej.2020.06.023
![]() |
[94] |
G. Iannace, G. Ciaburro, A. Trematerra, Fault diagnosis for UAV blades using artificial neural network, Robotics, 8 (2019), 59. https://doi.org/10.3390/robotics8030059 doi: 10.3390/robotics8030059
![]() |
[95] |
M. Kordestani, M. F. Samadi, M. Saif, K. Khorasani, A new fault diagnosis of multifunctional spoiler system using integrated artificial neural network and discrete wavelet transform methods, IEEE Sens. J., 18 (2018), 4990–5001. https://doi.org/10.1109/JSEN.2018.2829345 doi: 10.1109/JSEN.2018.2829345
![]() |
[96] |
S. Shi, G. Li, H. Chen, J. Liu, Y. Hu, L. Xing, et al., Refrigerant charge fault diagnosis in the VRF system using Bayesian artificial neural network combined with ReliefF filter, Appl. Therm. Eng., 112 (2017), 698–706. https://doi.org/10.1016/j.applthermaleng.2016.10.043 doi: 10.1016/j.applthermaleng.2016.10.043
![]() |
[97] |
X. Xu, D. Cao, Y. Zhou, J. Gao, Application of neural network algorithm in fault diagnosis of mechanical intelligence, Mech. Syst. Sig. Process., 141 (2020), 106625. https://doi.org/10.1016/j.ymssp.2020.106625 doi: 10.1016/j.ymssp.2020.106625
![]() |
[98] | A. Viveros-Wacher, J. E. Rayas-Sánchez, Analog fault identification in RF circuits using artificial neural networks and constrained parameter extraction, in 2018 IEEE MTT-S International Conference on Numerical Electromagnetic and Multiphysics Modeling and Optimization (NEMO), IEEE, (2018), 1–3. https://doi.org/10.1109/NEMO.2018.8503117 |
[99] |
S. Heo, J. H. Lee, Fault detection and classification using artificial neural networks, IFAC-PapersOnLine, 51 (2018), 470–475. https://doi.org/10.1016/j.ifacol.2018.09.380 doi: 10.1016/j.ifacol.2018.09.380
![]() |
[100] |
P. Agrawal, P. Jayaswal, Diagnosis and classifications of bearing faults using artificial neural network and support vector machine, J. Inst. Eng. (India): Ser. C, 101 (2020), 61–72. https://doi.org/10.1007/s40032-019-00519-9 doi: 10.1007/s40032-019-00519-9
![]() |
[101] | Y. LeCun, B. E. Boser, J. S. Denker, D. Henderson, R. E. Howard, W. E. Hubbard, et al., Handwritten digit recognition with a back-propagation network, in Advances in Neural Information Processing Systems, (1990), 396–404. |
[102] |
T. Chen, Y. Sun, T. H. Li, A semi-parametric estimation method for the quantile spectrum with an application to earthquake classification using convolutional neural network, Comput. Stat. Data Anal., 154 (2021), 107069. https://doi.org/10.1016/j.csda.2020.107069 doi: 10.1016/j.csda.2020.107069
![]() |
[103] |
F. Perla, R. Richman, S. Scognamiglio, M. V. Wüthrich, Time-series forecasting of mortality rates using deep learning, Scand. Actuarial J., 2021 (2021), 1–27. https://doi.org/10.1080/03461238.2020.1867232 doi: 10.1080/03461238.2020.1867232
![]() |
[104] |
G. Ciaburro, G. Iannace, V. Puyana-Romero, A. Trematerra, A comparison between numerical simulation models for the prediction of acoustic behavior of giant reeds shredded, Appl. Sci., 10 (2020), 6881. https://doi.org/10.3390/app10196881 doi: 10.3390/app10196881
![]() |
[105] |
C. Yildiz, H. Acikgoz, D. Korkmaz, U. Budak, An improved residual-based convolutional neural network for very short-term wind power forecasting, Energy Convers. Manage., 228 (2021), 113731. https://doi.org/10.1016/j.enconman.2020.113731 doi: 10.1016/j.enconman.2020.113731
![]() |
[106] |
G. Ciaburro, Sound event detection in underground parking garage using convolutional neural network, Big Data Cognit. Comput., 4 (2020), 20. https://doi.org/10.3390/bdcc4030020 doi: 10.3390/bdcc4030020
![]() |
[107] |
R. Ye, Q. Dai, Implementing transfer learning across different datasets for time series forecasting, Pattern Recognit., 109 (2021), 107617. https://doi.org/10.1016/j.patcog.2020.107617 doi: 10.1016/j.patcog.2020.107617
![]() |
[108] |
J. Han, L. Shi, Q. Yang, K. Huang, Y. Zha, J. Yu, Real-time detection of rice phenology through convolutional neural network using handheld camera images, Precis. Agric., 22 (2021), 154–178. https://doi.org/10.1016/j.patcog.2020.107617 doi: 10.1016/j.patcog.2020.107617
![]() |
[109] |
G. Ciaburro, G. Iannace, Improving smart cities safety using sound events detection based on deep neural network algorithms, Informatics, 7 (2020), 23. https://doi.org/10.3390/informatics7030023 doi: 10.3390/informatics7030023
![]() |
[110] |
L. Wen, X. Li, L. Gao, Y. Zhang, A new convolutional neural network-based data-driven fault diagnosis method, IEEE Trans. Ind. Electron., 65 (2017), 5990–5998. https://doi.org/10.1109/TIE.2017.2774777 doi: 10.1109/TIE.2017.2774777
![]() |
[111] | Y. LeCun, LeNet-5, Convolutional Neural Networks, 2015, Available from: http://yann.lecun.com/exdb/lenet/, Accessed date: 28 April 2022. |
[112] |
H. Wu, J. Zhao, Deep convolutional neural network model based chemical process fault diagnosis, Comput. Chem. Eng., 115 (2018), 185–197. https://doi.org/10.1016/j.compchemeng.2018.04.009 doi: 10.1016/j.compchemeng.2018.04.009
![]() |
[113] |
W. Zhang, C. Li, G. Peng, Y. Chen, Z. Zhang, A deep convolutional neural network with new training methods for bearing fault diagnosis under noisy environment and different working load, Mech. Syst. Sig. Process., 100 (2018), 439–453. https://doi.org/10.1016/j.ymssp.2017.06.022 doi: 10.1016/j.ymssp.2017.06.022
![]() |
[114] |
L. Jing, M. Zhao, P. Li, X. Xu, A convolutional neural network based feature learning and fault diagnosis method for the condition monitoring of gearbox, Measurement, 111 (2017), 1–10. https://doi.org/10.1016/j.measurement.2017.07.017 doi: 10.1016/j.measurement.2017.07.017
![]() |
[115] |
Z. Chen, C. Li, R. V. Sanchez, Gearbox fault identification and classification with convolutional neural networks, Shock Vib., 2015 (2015). https://doi.org/10.1155/2015/390134 doi: 10.1155/2015/390134
![]() |
[116] |
X. Guo, L. Chen, C. Shen, Hierarchical adaptive deep convolution neural network and its application to bearing fault diagnosis, Measurement, 93 (2016), 490–502. https://doi.org/10.1016/j.measurement.2016.07.054 doi: 10.1016/j.measurement.2016.07.054
![]() |
[117] |
O. Janssens, V. Slavkovikj, B. Vervisch, K. Stockman, M. Loccufier, S. Verstockt, et al., Convolutional neural network based fault detection for rotating machinery, J. Sound Vib., 377 (2016), 331–345. https://doi.org/10.1016/j.jsv.2016.05.027 doi: 10.1016/j.jsv.2016.05.027
![]() |
[118] |
W. Zhang, G. Peng, C. Li, Y. Chen, Z. Zhang, A new deep learning model for fault diagnosis with good anti-noise and domain adaptation ability on raw vibration signals, Sensors, 17 (2017), 425. https://doi.org/10.3390/s17020425 doi: 10.3390/s17020425
![]() |
[119] |
Y. Li, N. Wang, J. Shi, X. Hou, J. Liu, Adaptive batch normalization for practical domain adaptation, Pattern Recognit., 80 (2018), 109–117. https://doi.org/10.1016/j.patcog.2018.03.005 doi: 10.1016/j.patcog.2018.03.005
![]() |
[120] |
T. Ince, S. Kiranyaz, L. Eren, M. Askar, M. Gabbouj, Real-time motor fault detection by 1-D convolutional neural networks, IEEE Trans. Ind. Electron., 63 (2016), 7067–7075. https://doi.org/10.1109/TIE.2016.2582729 doi: 10.1109/TIE.2016.2582729
![]() |
[121] |
Y. Zhang, K. Xing, R. Bai, D. Sun, Z. Meng, An enhanced convolutional neural network for bearing fault diagnosis based on time-frequency image, Measurement, 157 (2020), 107667. https://doi.org/10.1016/j.measurement.2020.107667 doi: 10.1016/j.measurement.2020.107667
![]() |
[122] |
M. Azamfar, J. Singh, I. Bravo-Imaz, J. Lee, . Multisensor data fusion for gearbox fault diagnosis using 2-D convolutional neural network and motor current signature analysis, Mech. Syst. Sig. Process., 144 (2020), 106861. https://doi.org/10.1016/j.ymssp.2020.106861 doi: 10.1016/j.ymssp.2020.106861
![]() |
[123] |
Q. Zhou, Y. Li, Y. Tian, L. Jiang, A novel method based on nonlinear auto-regression neural network and convolutional neural network for imbalanced fault diagnosis of rotating machinery, Measurement, 161 (2020), 107880. https://doi.org/10.1016/j.measurement.2020.107880 doi: 10.1016/j.measurement.2020.107880
![]() |
[124] |
K. Zhang, J. Chen, T. Zhang, Z. Zhou, A compact convolutional neural network augmented with multiscale feature extraction of acquired monitoring data for mechanical intelligent fault diagnosis, J. Manuf. Syst., 55 (2020), 273–284. https://doi.org/10.1016/j.jmsy.2020.04.016 doi: 10.1016/j.jmsy.2020.04.016
![]() |
[125] |
Y. Li, X. Du, F. Wan, X. Wang, H. Yu, Rotating machinery fault diagnosis based on convolutional neural network and infrared thermal imaging, Chin. J. Aeronaut., 33 (2020), 427–438. https://doi.org/10.1016/j.cja.2019.08.014 doi: 10.1016/j.cja.2019.08.014
![]() |
[126] |
Z. Chen, A. Mauricio, W. Li, K. Gryllias, A deep learning method for bearing fault diagnosis based on cyclic spectral coherence and convolutional neural networks, Mech. Syst. Sig. Process., 140 (2020), 106683. https://doi.org/10.1016/j.ymssp.2020.106683 doi: 10.1016/j.ymssp.2020.106683
![]() |
[127] |
J. Antoni, Cyclic spectral analysis in practice, Mech. Syst. Sig. Process., 21 (2007), 597–630. https://doi.org/10.1016/j.ymssp.2006.08.007 doi: 10.1016/j.ymssp.2006.08.007
![]() |
[128] |
D. Zhou, Q. Yao, H. Wu, S. Ma, H. Zhang, Fault diagnosis of gas turbine based on partly interpretable convolutional neural networks, Energy, 200 (2020), 117467. https://doi.org/10.1016/j.energy.2020.117467 doi: 10.1016/j.energy.2020.117467
![]() |
[129] | T. Chen, T. He, M. Benesty, V. Khotilovich, Y. Tang, H. Cho, Xgboost: extreme gradient boosting, R package version 0.4-2, 1 (2015), 1–4. |
[130] |
X. Li, J. Zheng, M. Li, W. Ma, Y. Hu, Frequency-domain fusing convolutional neural network: A unified architecture improving effect of domain adaptation for fault diagnosis, Sensors, 21 (2021), 450. https://doi.org/10.3390/s21020450 doi: 10.3390/s21020450
![]() |
[131] | C. C. Chen, Z. Liu, G. Yang, C. C. Wu, Q. Ye, An improved fault diagnosis using 1D-convolutional neural network model, electronics, 10 (2021), 59. https://doi.org/10.3390/electronics10010059 |
[132] |
Y. Liu, Y. Yang, T. Feng, Y. Sun, X. Zhang, Research on rotating machinery fault diagnosis method based on energy spectrum matrix and adaptive convolutional neural network, Processes, 9 (2021), 69. https://doi.org/10.3390/pr9010069 doi: 10.3390/pr9010069
![]() |
[133] |
D. T. Hoang, X. T. Tran, M. Van, H. J. Kang, A deep neural network-based feature fusion for bearing fault diagnosis, Sensors, 21 (2021), 244. https://doi.org/10.3390/s21010244 doi: 10.3390/s21010244
![]() |
[134] | T. Mikolov, M. Karafiát, L. Burget, J. Černocký, S. Khudanpur, Recurrent neural network based language model, in Eleventh Annual Conference of the International Speech Communication Association, 2010. |
[135] | K. Gregor, I. Danihelka, A. Graves, D. Rezende, D. Wierstra, Draw: A recurrent neural network for image generation, in International Conference on Machine Learning (PMLR), 37 (2015), 1462–1471. |
[136] | T. Mikolov, G. Zweig, Context dependent recurrent neural network language model, in 2012 IEEE Spoken Language Technology Workshop (SLT), IEEE, (2012), 234–239. https://doi.org/10.1109/SLT.2012.6424228 |
[137] | G. Ciaburro, Time series data analysis using deep learning methods for smart cities monitoring, in Big Data Intelligence for Smart Applications, Springer, Cham, (2022), 93–116. https://doi.org/10.1007/978-3-030-87954-9_4 |
[138] |
H. Sak, A. W. Senior, F. Beaufays, Long short-term memory recurrent neural network architectures for large scale acoustic modeling, Interspeech, (2014), 338–342. https://doi.org/10.21437/Interspeech.2014-80 doi: 10.21437/Interspeech.2014-80
![]() |
[139] | J. Kim, J. Kim, H. L. T. Thu, H. Kim, Long short term memory recurrent neural network classifier for intrusion detection, in 2016 International Conference on Platform Technology and Service (PlatCon), IEEE, (2016), 1–5. https://doi.org/10.1109/PlatCon.2016.7456805 |
[140] | Y. Tian, L. Pan, Predicting short-term traffic flow by long short-term memory recurrent neural network, in 2015 IEEE International Conference on Smart City/SocialCom/SustainCom (SmartCity), IEEE, (2015), 153–158. https://doi.org/10.1109/SmartCity.2015.63 |
[141] |
H. Jiang, X. Li, H. Shao, K. Zhao, Intelligent fault diagnosis of rolling bearings using an improved deep recurrent neural network, Meas. Sci. Technol., 29 (2018), 065107. https://doi.org/10.1088/1361-6501/aab945 doi: 10.1088/1361-6501/aab945
![]() |
[142] |
T. De Bruin, K. Verbert, R. Babuška, Railway track circuit fault diagnosis using recurrent neural networks, IEEE Trans. Neural Networks Learn. Syst., 28 (2016), 523–533. https://doi.org/10.1109/TNNLS.2016.2551940 doi: 10.1109/TNNLS.2016.2551940
![]() |
[143] |
R. Yang, M. Huang, Q. Lu, M. Zhong, Rotating machinery fault diagnosis using long-short-term memory recurrent neural network, IFAC-PapersOnLine, 51 (2018), 228–232. https://doi.org/10.1016/j.ifacol.2018.09.582 doi: 10.1016/j.ifacol.2018.09.582
![]() |
[144] |
H. A. Talebi, K. Khorasani, S. Tafazoli, A recurrent neural-network-based sensor and actuator fault detection and isolation for nonlinear systems with application to the satellite's attitude control subsystem, IEEE Trans. Neural Networks, 20 (2008), 45–60. https://doi.org/10.1109/TNN.2008.2004373 doi: 10.1109/TNN.2008.2004373
![]() |
[145] |
S. Zhang, K. Bi, T. Qiu, Bidirectional recurrent neural network-based chemical process fault diagnosis, Ind. Eng. Chem. Res., 59 (2019), 824–834. https://doi.org/10.1021/acs.iecr.9b05885 doi: 10.1021/acs.iecr.9b05885
![]() |
[146] |
Z. An, S. Li, J. Wang, X. Jiang, A novel bearing intelligent fault diagnosis framework under time-varying working conditions using recurrent neural network, ISA Trans., 100 (2020), 155–170. https://doi.org/10.1016/j.isatra.2019.11.010 doi: 10.1016/j.isatra.2019.11.010
![]() |
[147] |
W. Liu, P. Guo, L. Ye, A low-delay lightweight recurrent neural network (LLRNN) for rotating machinery fault diagnosis, Sensors, 19 (2019), 3109. https://doi.org/10.3390/s19143109 doi: 10.3390/s19143109
![]() |
[148] |
K. Liang, N. Qin, D. Huang, Y. Fu, Convolutional recurrent neural network for fault diagnosis of high-speed train bogie, Complexity, 2018 (2018). https://doi.org/10.1155/2018/4501952 doi: 10.1155/2018/4501952
![]() |
[149] |
D. Huang, Y. Fu, N. Qin, S. Gao, Fault diagnosis of high-speed train bogie based on LSTM neural network, Sci. Chin. Inf. Sci., 64 (2021), 1–3. https://doi.org/10.1007/s11432-018-9543-8 doi: 10.1007/s11432-018-9543-8
![]() |
[150] |
H. Shahnazari, P. Mhaskar, J. M. House, T. I. Salsbury, Modeling and fault diagnosis design for HVAC systems using recurrent neural networks, Comput. Chem. Eng., 126 (2019), 189–203. https://doi.org/10.1016/j.compchemeng.2019.04.011 doi: 10.1016/j.compchemeng.2019.04.011
![]() |
[151] |
H. Shahnazari, Fault diagnosis of nonlinear systems using recurrent neural networks, Chem. Eng. Res. Des., 153 (2020), 233–245. https://doi.org/10.1016/j.cherd.2019.09.026 doi: 10.1016/j.cherd.2019.09.026
![]() |
[152] |
L. Guo, N. Li, F. Jia, Y. Lei, J. Lin, A recurrent neural network based health indicator for remaining useful life prediction of bearings, Neurocomputing, 240 (2017), 98–109. https://doi.org/10.1016/j.neucom.2017.02.045 doi: 10.1016/j.neucom.2017.02.045
![]() |
[153] | M. Yuan, Y. Wu, L. Lin, Fault diagnosis and remaining useful life estimation of aero engine using LSTM neural network, in 2016 IEEE international conference on aircraft utility systems (AUS), IEEE, (2016), 135–140. https://doi.org/10.1109/AUS.2016.7748035 |
[154] |
Z. Wu, H. Jiang, K. Zhao, X. Li, An adaptive deep transfer learning method for bearing fault diagnosis, Measurement, 151 (2020), 107227. https://doi.org/10.1016/j.measurement.2019.107227 doi: 10.1016/j.measurement.2019.107227
![]() |
[155] |
A. Yin, Y. Yan, Z. Zhang, C. Li, R. V. Sánchez, Fault diagnosis of wind turbine gearbox based on the optimized LSTM neural network with cosine loss, Sensors, 20 (2020), 2339. https://doi.org/10.3390/s20082339 doi: 10.3390/s20082339
![]() |
[156] |
M. Xia, X. Zheng, M. Imran, M. Shoaib, Data-driven prognosis method using hybrid deep recurrent neural network, Appl. Soft Comput., 93 (2020), 106351. https://doi.org/10.1016/j.asoc.2020.106351 doi: 10.1016/j.asoc.2020.106351
![]() |
[157] |
Z. Wang, Y. Dong, W. Liu, Z. Ma, A novel fault diagnosis approach for chillers based on 1-D convolutional neural network and gated recurrent unit, Sensors, 20 (2020), 2458. https://doi.org/10.3390/s20092458 doi: 10.3390/s20092458
![]() |
[158] |
R. Salakhutdinov, Learning deep generative models, Annu. Rev. Stat. Appl., 2 (2015), 361–385. https://doi.org/10.1146/annurev-statistics-010814-020120 doi: 10.1146/annurev-statistics-010814-020120
![]() |
[159] | A. Gupta, A. Agarwal, P. Singh, P. Rai, A deep generative framework for paraphrase generation, in Proceedings of the AAAI Conference on Artificial Intelligence, 32 (2018). https://doi.org/10.1609/aaai.v32i1.11956 |
[160] | I. J. Goodfellow, J. Pouget-Abadie, M. Mirza, B. Xu, D. Warde-Farley, S. Ozair, et al., Generative adversarial networks, 2014, preprint, arXiv: 1406.2661. |
[161] | L. Metz, B. Poole, D. Pfau, J. Sohl-Dickstein, Unrolled generative adversarial networks, 2016, preprint, arXiv: 1611.02163. |
[162] | G. Ciaburro, Security systems for smart cities based on acoustic sensors and machine learning applications, in Machine Intelligence and Data Analytics for Sustainable Future Smart Cities, Springer, Cham, (2021), 369–393. https://doi.org/10.1007/978-3-030-72065-0_20 |
[163] | X. Hou, L. Shen, K. Sun, G. Qiu, Deep feature consistent variational autoencoder, in 2017 IEEE Winter Conference on Applications of Computer Vision (WACV), IEEE, (2017), 1133–1141. https://doi.org/10.1109/WACV.2017.131 |
[164] | M. J. Kusner, B. Paige, J. M. Hernández-Lobato, Grammar variational autoencoder, in International Conference on Machine Learning (PMLR), 70 (2017), 1945–1954. |
[165] | Y. Pu, Z. Gan, R. Henao, X. Yuan, C. Li, A. Stevens, et al., Variational autoencoder for deep learning of images, labels and captions, 2016, preprint, arXiv: 1609.08976. |
[166] | A. Makhzani, J. Shlens, N. Jaitly, I. Goodfellow, B. Frey, Adversarial autoencoders, 2015, preprint, arXiv: 1511.05644. |
[167] | Z. Zhang, Y. Song, H. Qi, Age progression/regression by conditional adversarial autoencoder, in Proceedings of the IEEE Conference on Computer Vision and Pattern Recognition, (2017), 5810–5818. https://doi.org/10.1109/CVPR.2017.463 |
[168] |
H. Liu, J. Zhou, Y. Xu, Y. Zheng, X. Peng, W. Jiang, Unsupervised fault diagnosis of rolling bearings using a deep neural network based on generative adversarial networks, Neurocomputing, 315 (2018), 412–424. https://doi.org/10.1016/j.neucom.2018.07.034 doi: 10.1016/j.neucom.2018.07.034
![]() |
[169] |
S. Shao, P. Wang, R. Yan, Generative adversarial networks for data augmentation in machine fault diagnosis, Comput. Ind., 106 (2019), 85–93. https://doi.org/10.1016/j.compind.2019.01.001 doi: 10.1016/j.compind.2019.01.001
![]() |
[170] |
W. Zhang, X. Li, X. D. Jia, H. Ma, Z. Luo, X. Li, Machinery fault diagnosis with imbalanced data using deep generative adversarial networks, Measurement, 152 (2020), 107377. https://doi.org/10.1016/j.measurement.2019.107377 doi: 10.1016/j.measurement.2019.107377
![]() |
[171] |
Z. Wang, J. Wang, Y. Wang, An intelligent diagnosis scheme based on generative adversarial learning deep neural networks and its application to planetary gearbox fault pattern recognition, Neurocomputing, 310 (2018), 213–222. https://doi.org/10.1016/j.neucom.2018.05.024 doi: 10.1016/j.neucom.2018.05.024
![]() |
[172] | P. Vincent, H. Larochelle, I. Lajoie, Y. Bengio, P. A. Manzagol, L. Bottou, Stacked denoising autoencoders: Learning useful representations in a deep network with a local denoising criterion, J. Mach. Learn. Res., 11 (2010), 3371–3408. |
[173] |
Q. Li, L. Chen, C. Shen, B. Yang, Z. Zhu, Enhanced generative adversarial networks for fault diagnosis of rotating machinery with imbalanced data, Meas. Sci. Technol., 30 (2019), 115005. https://doi.org/10.1088/1361-6501/ab3072 doi: 10.1088/1361-6501/ab3072
![]() |
[174] |
J. Wang, S. Li, B. Han, Z. An, H. Bao, S. Ji, Generalization of deep neural networks for imbalanced fault classification of machinery using generative adversarial networks, IEEE Access, 7 (2019), 111168–111180. https://doi.org/10.1109/ACCESS.2019.2924003 doi: 10.1109/ACCESS.2019.2924003
![]() |
[175] | Y. Xie, T. Zhang, Imbalanced learning for fault diagnosis problem of rotating machinery based on generative adversarial networks, in 2018 37th Chinese Control Conference (CCC), IEEE, (2018), 6017–6022. https://doi.org/10.23919/ChiCC.2018.8483334 |
[176] |
C. Zhong, K. Yan, Y. Dai, N. Jin, B. Lou, Energy efficiency solutions for buildings: Automated fault diagnosis of air handling units using generative adversarial networks, Energies, 12 (2019), 527. https://doi.org/10.3390/en12030527 doi: 10.3390/en12030527
![]() |
[177] |
D. Zhao, S. Liu, D. Gu, X. Sun, L. Wang, Y. Wei, et al., Enhanced data-driven fault diagnosis for machines with small and unbalanced data based on variational auto-encoder, Meas. Sci. Technol., 31 (2019), 035004. https://doi.org/10.1088/1361-6501/ab55f8 doi: 10.1088/1361-6501/ab55f8
![]() |
[178] | J. An, S. Cho, Variational autoencoder based anomaly detection using reconstruction probability, Spec. Lect. IE, 2 (2015), 1–18. |
[179] |
G. San Martin, E. López Droguett, V. Meruane, M. das Chagas Moura, Deep variational auto-encoders: A promising tool for dimensionality reduction and ball bearing elements fault diagnosis, Struct. Health Monit., 18 (2019), 1092–1128. https://doi.org/10.1177/1475921718788299 doi: 10.1177/1475921718788299
![]() |
[180] | Y. Kawachi, Y. Koizumi, N. Harada, Complementary set variational autoencoder for supervised anomaly detection, in 2018 IEEE International Conference on Acoustics, Speech and Signal Processing (ICASSP), IEEE, (2018), 2366–2370. https://doi.org/10.1109/ICASSP.2018.8462181 |
[181] |
D. Park, Y. Hoshi, C. C. Kemp, A multimodal anomaly detector for robot-assisted feeding using an LSTM-based variational autoencoder, IEEE Rob. Autom. Lett., 3 (2018), 1544–1551. https://doi.org/10.1109/LRA.2018.2801475 doi: 10.1109/LRA.2018.2801475
![]() |
[182] |
S. Lee, M. Kwak, K. L. Tsui, S. B. Kim, Process monitoring using variational autoencoder for high-dimensional nonlinear processes, Eng. Appl. Artif. Intell., 83 (2019), 13–27. https://doi.org/10.1016/j.engappai.2019.04.013 doi: 10.1016/j.engappai.2019.04.013
![]() |
[183] |
K. Wang, M. G. Forbes, B. Gopaluni, J. Chen, Z. Song, Systematic development of a new variational autoencoder model based on uncertain data for monitoring nonlinear processes, IEEE Access, 7 (2019), 22554–22565. https://doi.org/10.1109/ACCESS.2019.2894764 doi: 10.1109/ACCESS.2019.2894764
![]() |
[184] |
G. Ping, J. Chen, T. Pan, J. Pan, Degradation feature extraction using multi-source monitoring data via logarithmic normal distribution based variational auto-encoder, Comput. Ind., 109 (2019), 72–82. https://doi.org/10.1016/j.compind.2019.04.013 doi: 10.1016/j.compind.2019.04.013
![]() |
[185] |
J. Wu, Z. Zhao, C. Sun, R. Yan, X. Chen, Fault-attention generative probabilistic adversarial autoencoder for machine anomaly detection, IEEE Trans. Ind. Inf., 16 (2020), 7479–7488. https://doi.org/10.1109/TⅡ.2020.2976752 doi: 10.1109/TⅡ.2020.2976752
![]() |
[186] | G. Ciaburro, An ensemble classifier approach for thyroid disease diagnosis using the AdaBoostM algorithm, in Machine Learning, Big Data, and IoT for Medical Informatics, Academic Press, (2021), 365–387. https://doi.org/10.1016/B978-0-12-821777-1.00002-1 |
[187] |
Z. Gao, C. Cecati, S. X. Ding, A survey of fault diagnosis and fault-tolerant techniques—Part I: fault diagnosis with model-based and signal-based approaches, IEEE Trans. Ind. Electron., 62 (2015), 3757–3767. https://doi.org/10.1109/TIE.2015.2417501 doi: 10.1109/TIE.2015.2417501
![]() |
[188] |
M. Djeziri, O. Djedidi, S. Benmoussa, M. Bendahan, J. L. Seguin, Failure prognosis based on relevant measurements identification and data-driven trend-modeling: Application to a fuel cell system, Processes, 9 (2021), 328. https://doi.org/10.3390/pr9020328 doi: 10.3390/pr9020328
![]() |
[189] |
M. Aliramezani, C. R. Koch, M. Shahbakhti, Modeling, diagnostics, optimization, and control of internal combustion engines via modern machine learning techniques: A review and future directions, Prog. Energy Combust. Sci., 88 (2022), 100967. https://doi.org/10.1016/j.pecs.2021.100967 doi: 10.1016/j.pecs.2021.100967
![]() |
[190] |
D. Passos, P. Mishra, A tutorial on automatic hyperparameter tuning of deep spectral modelling for regression and classification tasks, Chemom. Intell. Lab. Syst., 233 (2022), 104520. https://doi.org/10.1016/j.chemolab.2022.104520 doi: 10.1016/j.chemolab.2022.104520
![]() |
[191] |
A. Zakaria, F. B. Ismail, M. H. Lipu, M. A. Hannan, Uncertainty models for stochastic optimization in renewable energy applications, Renewable Energy, 145 (2020), 1543–1571. https://doi.org/10.1016/j.renene.2019.07.081 doi: 10.1016/j.renene.2019.07.081
![]() |
[192] |
M. H. Lin, J. F. Tsai, C. S. Yu, A review of deterministic optimization methods in engineering and management, Math. Probl. Eng., 2012 (2012). https://doi.org/10.1155/2012/756023 doi: 10.1155/2012/756023
![]() |
1. | Mehmet Kayalar, A uniqueness theorem for singular Sturm-liouville operator, 2023, 34, 1012-9405, 10.1007/s13370-023-01097-x |
Algorithms | Convergence | Computation Complexity |
Gradient descent | Stable, slow | Gradient |
Newton | Unstable, fast | Gradient and Hessian |
Gauss-Newton | Unstable, fast | Jacobian |
Levenberg-Marquardt | Stable, fast | Jacobian |
Material | EExact | E0 | ECalculated | k | eE |
Aluminium (13Al) | 68 | 70.25 | 67.9904 | 25 | 0.0096133 |
Titanium (22Ti) | 116 | 115 | 116.011 | 13 | 0.0109185 |
Bronze | 112 | 113 | 112.250 | 13 | 0.0066388 |
Zinc (30Zn) | 108 | 110.25 | 108.007 | 25 | 0.0074127 |
Nylon (66) | 2.93 | 2.5 | 2.92746 | 6 | 0.0025417 |
k | eE | k | eE | k | eE |
0 | 1 | 5 | 0.729589 | 10 | 0.311645 |
1 | 0.925356 | 6 | 0.662241 | 11 | 0.116378 |
2 | 0.857706 | 7 | 0.595521 | 12 | 0.042788 |
3 | 0.809445 | 8 | 0.553536 | 13 | 0.010918 |
4 | 0.768314 | 9 | 0.493262 |
NE | η(%) | k | eE | NE | η(%) | k | eE |
1800 | 0.25 | 19 | 0.0012 | 1800 | 1.75 | 09 | 0.011 |
1800 | 0.50 | 10 | 0.0031 | 1800 | 2.00 | 05 | 0.019 |
1800 | 0.75 | 09 | 0.0044 | 1800 | 2.25 | 03 | 0.041 |
1800 | 1.00 | 07 | 0.0058 | 1800 | 2.50 | 06 | 0.053 |
1800 | 1.25 | 09 | 0.0070 | 1800 | 2.75 | 03 | 0.068 |
1800 | 1.50 | 09 | 0.0074 | 1800 | >2.75 | fail! | fail! |
k | eE | k | eE | k | eE |
1 | 0.103244 | 7 | 0.019356 | 13 | 0.003495 |
2 | 0.078826 | 8 | 0.010968 | 14 | 0.003495 |
3 | 0.050282 | 9 | 0.006122 | 15 | 0.003693 |
4 | 0.040450 | 10 | 0.004676 | 16 | 0.002940 |
5 | 0.031286 | 11 | 0.003857 | 17 | 0.002623 |
6 | 0.025083 | 12 | 0.002983 | 18 | 0.002521 |
k | eE | k | eE | k | eE | k | eE |
1 | 0.14524 | 6 | 0.06519 | 11 | 0.02621 | 16 | 0.00397 |
2 | 0.13081 | 7 | 0.05603 | 12 | 0.01979 | 17 | 0.00591 |
3 | 0.11370 | 8 | 0.04337 | 13 | 0.17039 | 18 | 0.00287 |
4 | 0.09535 | 9 | 0.03191 | 14 | 0.00910 | 19 | 0.00278 |
5 | 0.07731 | 10 | 0.02882 | 15 | 0.00701 |
k | Relative error eE | Residual error E | |
Abdelhamid et al. [23], NE=12,800 | 67 | 0.038095 | 0.036702 |
Present work, NE=9800 | 23 | 0.003532 | 0.005026 |
Algorithms | Convergence | Computation Complexity |
Gradient descent | Stable, slow | Gradient |
Newton | Unstable, fast | Gradient and Hessian |
Gauss-Newton | Unstable, fast | Jacobian |
Levenberg-Marquardt | Stable, fast | Jacobian |
Material | EExact | E0 | ECalculated | k | eE |
Aluminium (13Al) | 68 | 70.25 | 67.9904 | 25 | 0.0096133 |
Titanium (22Ti) | 116 | 115 | 116.011 | 13 | 0.0109185 |
Bronze | 112 | 113 | 112.250 | 13 | 0.0066388 |
Zinc (30Zn) | 108 | 110.25 | 108.007 | 25 | 0.0074127 |
Nylon (66) | 2.93 | 2.5 | 2.92746 | 6 | 0.0025417 |
k | eE | k | eE | k | eE |
0 | 1 | 5 | 0.729589 | 10 | 0.311645 |
1 | 0.925356 | 6 | 0.662241 | 11 | 0.116378 |
2 | 0.857706 | 7 | 0.595521 | 12 | 0.042788 |
3 | 0.809445 | 8 | 0.553536 | 13 | 0.010918 |
4 | 0.768314 | 9 | 0.493262 |
NE | η(%) | k | eE | NE | η(%) | k | eE |
1800 | 0.25 | 19 | 0.0012 | 1800 | 1.75 | 09 | 0.011 |
1800 | 0.50 | 10 | 0.0031 | 1800 | 2.00 | 05 | 0.019 |
1800 | 0.75 | 09 | 0.0044 | 1800 | 2.25 | 03 | 0.041 |
1800 | 1.00 | 07 | 0.0058 | 1800 | 2.50 | 06 | 0.053 |
1800 | 1.25 | 09 | 0.0070 | 1800 | 2.75 | 03 | 0.068 |
1800 | 1.50 | 09 | 0.0074 | 1800 | >2.75 | fail! | fail! |
k | eE | k | eE | k | eE |
1 | 0.103244 | 7 | 0.019356 | 13 | 0.003495 |
2 | 0.078826 | 8 | 0.010968 | 14 | 0.003495 |
3 | 0.050282 | 9 | 0.006122 | 15 | 0.003693 |
4 | 0.040450 | 10 | 0.004676 | 16 | 0.002940 |
5 | 0.031286 | 11 | 0.003857 | 17 | 0.002623 |
6 | 0.025083 | 12 | 0.002983 | 18 | 0.002521 |
k | eE | k | eE | k | eE | k | eE |
1 | 0.14524 | 6 | 0.06519 | 11 | 0.02621 | 16 | 0.00397 |
2 | 0.13081 | 7 | 0.05603 | 12 | 0.01979 | 17 | 0.00591 |
3 | 0.11370 | 8 | 0.04337 | 13 | 0.17039 | 18 | 0.00287 |
4 | 0.09535 | 9 | 0.03191 | 14 | 0.00910 | 19 | 0.00278 |
5 | 0.07731 | 10 | 0.02882 | 15 | 0.00701 |
k | Relative error eE | Residual error E | |
Abdelhamid et al. [23], NE=12,800 | 67 | 0.038095 | 0.036702 |
Present work, NE=9800 | 23 | 0.003532 | 0.005026 |