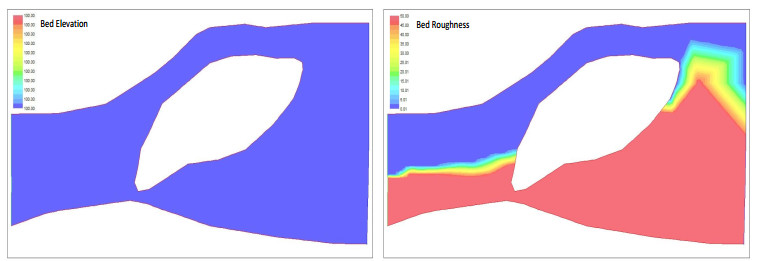
Rural residents' income is one of the core issues of rural economic development, and digital financial inclusion is one of the important influencing factors of rural residents' income. Especially under the background of the implementation of digital financial technology, the relationship between the two has become more complex. Based on the panel data set of 1624 counties in Chinese mainland in the past 2014–2019 years, the paper uses panel regression models to study the impact of digital financial inclusion on rural residents' income. Further, by analyzing the industrial structure, education level and financial development level, the following conclusions are drawn. First, digital financial inclusion significantly promotes the increase of rural residents' income, but there are differences in regional level and different quantiles of rural residents' income. At the regional level, the promotion of control effect at the provincial level is stronger than that at the county level; in different quantiles of residents' income, with the increase of residents' income quantile, the promoting effect is gradually enhanced. Second, the heterogeneous impact of digital financial inclusion on rural residents' income is reflected in three aspects: regional development, education level and financial development level. Third, industrial structure, education level and financial development level will enhance the promotion effect of digital financial inclusion on rural residents' income, but there are significant differences in the intensity of the regulatory effect of the three variables.
Citation: Tinghui Li, Jiehua Ma. Does digital finance benefit the income of rural residents? A case study on China[J]. Quantitative Finance and Economics, 2021, 5(4): 664-688. doi: 10.3934/QFE.2021030
[1] | Renjie Chu, Peiyuan Jin, Hanli Qiao, Quanxi Feng . Intrusion detection in the IoT data streams using concept drift localization. AIMS Mathematics, 2024, 9(1): 1535-1561. doi: 10.3934/math.2024076 |
[2] | Xiangjun Dai, Suli Wang, Baoping Yan, Zhi Mao, Weizhi Xiong . Survival analysis of single-species population diffusion models with chemotaxis in polluted environment. AIMS Mathematics, 2020, 5(6): 6749-6765. doi: 10.3934/math.2020434 |
[3] | Xiangyun Shi, Xiwen Gao, Xueyong Zhou, Yongfeng Li . Analysis of an SQEIAR epidemic model with media coverage and asymptomatic infection. AIMS Mathematics, 2021, 6(11): 12298-12320. doi: 10.3934/math.2021712 |
[4] | Paul Bosch, Héctor J. Carmenate, José M. Rodríguez, José M. Sigarreta . Generalized inequalities involving fractional operators of the Riemann-Liouville type. AIMS Mathematics, 2022, 7(1): 1470-1485. doi: 10.3934/math.2022087 |
[5] | Asma Hanif, Azhar Iqbal Kashif Butt . Atangana-Baleanu fractional dynamics of dengue fever with optimal control strategies. AIMS Mathematics, 2023, 8(7): 15499-15535. doi: 10.3934/math.2023791 |
[6] | Lijuan Chen, Tingting Liu, Fengde Chen . Stability and bifurcation in a two-patch model with additive Allee effect. AIMS Mathematics, 2022, 7(1): 536-551. doi: 10.3934/math.2022034 |
[7] | Miled El Hajji, Mohammed Faraj S. Aloufi, Mohammed H. Alharbi . Influence of seasonality on Zika virus transmission. AIMS Mathematics, 2024, 9(7): 19361-19384. doi: 10.3934/math.2024943 |
[8] | Yousef Alnafisah, Moustafa El-Shahed . Deterministic and stochastic model for the hepatitis C with different types of virus genome. AIMS Mathematics, 2022, 7(7): 11905-11918. doi: 10.3934/math.2022664 |
[9] | Fahad Al Basir, Konstantin B. Blyuss, Ezio Venturino . Stability and bifurcation analysis of a multi-delay model for mosaic disease transmission. AIMS Mathematics, 2023, 8(10): 24545-24567. doi: 10.3934/math.20231252 |
[10] | Jinsheng Guo, Shuang-Ming Wang . Threshold dynamics of a time-periodic two-strain SIRS epidemic model with distributed delay. AIMS Mathematics, 2022, 7(4): 6331-6355. doi: 10.3934/math.2022352 |
Rural residents' income is one of the core issues of rural economic development, and digital financial inclusion is one of the important influencing factors of rural residents' income. Especially under the background of the implementation of digital financial technology, the relationship between the two has become more complex. Based on the panel data set of 1624 counties in Chinese mainland in the past 2014–2019 years, the paper uses panel regression models to study the impact of digital financial inclusion on rural residents' income. Further, by analyzing the industrial structure, education level and financial development level, the following conclusions are drawn. First, digital financial inclusion significantly promotes the increase of rural residents' income, but there are differences in regional level and different quantiles of rural residents' income. At the regional level, the promotion of control effect at the provincial level is stronger than that at the county level; in different quantiles of residents' income, with the increase of residents' income quantile, the promoting effect is gradually enhanced. Second, the heterogeneous impact of digital financial inclusion on rural residents' income is reflected in three aspects: regional development, education level and financial development level. Third, industrial structure, education level and financial development level will enhance the promotion effect of digital financial inclusion on rural residents' income, but there are significant differences in the intensity of the regulatory effect of the three variables.
In water resource planning, to quantify the flow requirements in space and time that are necessary to sustain desired ecosystem services [8,40] is referred to as instream flow need (IFN) assessment [3]. Ecologists, mathematicians and environment managers have paid increasing attention to the investigation of spatial population dynamics and invasions of stream or river species, which suggests solutions to IFNs and provides evidence on the influence of flows on ecosystems.
River ecologists are interested in the "drift paradox" problem, which asks how river organisms can persist in a river with unidirectional water flow [34,35]. Population persistence over large spatial and temporal scales is an important component of instream flow need assessment studies [3]. Krkosěk and Lewis [25] proposed three measures for population persistence that relate to lifetime reproductive output of a species in a spatially variable environment and they previously have been applied to river population models [20,31]. These measures are connected through the next-generation operator, which maps the population forward in time from one generation to the next. The first measure, Rloc(x), describes the fundamental niche of the species. It represents the local persistence at any location x in the absence of dispersal, depending only on reproduction and survival at the location x. The second measure, Rδ(x), describes the realized niche. It is the lifetime reproductive output of an individual, initially introduced at x, undergoing survival, reproduction, and dispersal, and hence, its value, larger than one or less than one, also determines the source and sink regions in the habitat. The third measure is the net reproductive rate R0. It represents the average number of offspring produced by a single individual over its lifetime where the spatial distribution of the individual is given by the dominant eigenfunction of the linearized next-generation operator; see theories for R0 in e.g., [9,47]. The net reproductive rate has a long history as measures for population persistence/extinction in ecological modeling. Intuitively, if R0>1 the population will grow, but if R0<1 the population will likely become extinct. While this has the potential to make R0 a powerful tool for studying population persistence, further mathematical work is required if R0 is to be linked rigorously to behavior of the associated nonlinear model. Existing studies of the three measures for river species have revealed the influence of hydrodynamics on long term behaviors of river populations, especially of the net reproductive rate R0, but it is only for idealized spatially homogeneous or one-dimensional (longitudinal) varying rivers (see e.g., [20,31]). Therefore, it is necessary and important to establish persistence theories in more realistic two or three-dimensional rivers.
Most population models in river ecology have been investigated under simple assumptions for hydrodynamics, such as a constant water depth or a constant flow velocity throughout the river (see e.g., [19,29,38,39,41]). A few have taken into account spatial or temporal variations in riverbed structure or the flow regime, but only for simplified and essentially one-dimensional structures (see e.g., [20,23,24,28]). The results from these models are interesting but only provide limited information about the influence of realistic hydrodynamics on spatial population dynamics. There also have been methodologies relying on habitat suitability models and physical habitat availability as a proxy for population status (see e.g., [3,14,21,26,40]). They link flow regimes with ecology qualitatively, but lack a mechanistic foundation, and hence they can well predict habitat quality rather than species long term behavior in the habitat. Thus, direct integration of ecological systems into water flow dynamics and quantitative analysis of the impact of flows on ecology are still rare but in urgent need. A hybrid modeling approach was proposed to simulate the spatial dynamics of macroinvertebrates in a section of the Merced River in central California in [2]. The hydrodynamic model in MIKE 21 Flow Model FM [11] was used to characterize the two dimensional flow field in the longitudinal and lateral directions through the Robinson Reach of this river. The hydraulic prediction from the two dimensional model was then coupled with a particle tracking algorithm [12] to compute the drift dispersal. While the work focused on the effect of hydraulic flow on population dispersal in a two dimensional river, the main work of integration of hydraulic dynamics and ecology was simplified to the study of the steady state of a one dimensional representation model. In [22], a hybrid physical-biological modeling approach was presented to directly link river hydrology with river population models. A governing equation for the gradually varied flow was first coupled to a single population equation in one-dimensional rivers and then in two-dimensional rivers. Moreover, population spread and invasion ratchet phenomenon were studied for these models.
By coupling hydrodynamics and ecological dynamics, one can explicitly investigate the influence of physical factors and hydraulic conditions on spatiotemporal dynamics of a population in a river, which strengthens the ecological component of environmental flow assessments that is currently still lacking. By using the hybrid physical-biological modeling approach coupled to River2D [22], one can observe the evolution of the density of a single stage drifting population in a two-dimensional river. While such calculation can certainly predict the long-term dynamics of persistence or extinction of a population in a specific river, the calculation itself is expensive and the transient running time may be long. Therefore it is advantageous to develop qualitative and quantitative methods that can directly determine population persistence in a two dimensional river.
In this paper, we are interested in species living both in the flowing water and on the benthos of a river (see e.g., [1,13,20,28,38]). We extend the results of persistence measures (Rloc, Rδ and R0) for a benthic-drift population [20] in a one-dimensional river to a benthic-drift model in a two-dimensional depth-averaged river environment, in order to see how different factors, especially hydraulic factors, influence population persistence, and to distinguish how the sedentary stage helps populations persist in a two dimensional river. Our hybrid biological-physical modeling approach, coupled to the River2D program, provides a method to analyze the impact of river morphology on population persistence in a realistic river. It can be used to calculate not only the benthic and drift population densities but also the net reproductive rate of a specific species and its source/sink regions in any depth-averaged river model, given the river morphologic information and population demographic information. To our knowledge, this is the first study that quantitatively describes population persistence in a two-dimensional river environment based on the explicit bio-physical coupling of hydrology and benthic and drift compartments. It is expected that this work could provide an important methodology for ecologists or environment managers to determine population dynamics of a specific species in a specific river.
This paper is organized as follows. In Section 2, we introduce a benthic-drift model for a population in a two-dimensional depth-averaged model of a river. In Section 3, we define the next generating operator for the linearized system and derive three measures of persistence, Rloc(x,y), Rδ(x,y), and R0. We show that R0 can be used as a threshold to determine global population persistence or extinction for the nonlinear model. In Section 4, we present a numerical method to calculate R0 and Rδ when coupling the population model and hydrodynamic model in River2D. In Section 5, we choose two typical rivers and investigate how the water flow, birth rate, transfer rates, diffusion rate, river bottom slope, and river bottom roughness influence population persistence via numerical simulations for R0 and Rδ under different conditions. Finally, a short section of discussion completes the paper.
Denote the two dimensional river region by a bounded and smooth domain Ω in R2 with boundary ∂Ω. Consider the following two dimensional benthic-drift population model, a derivation of which is provided in Appendix A:
{∂Nd∂t=μ(x,y)h(x,y)Nb⏟transfer from Nb−σ(x,y)Nd⏟transfer to Nb−md(x,y)Nd⏟death−1h(x,y)▽⋅(v(x,y)h(x,y)Nd)⏟advection+1h(x,y)▽⋅(D(x,y)h(x,y)▽Nd)⏟diffusion,(x,y)∈Ω,t>0,∂Nb∂t=f(x,y,Nb)Nb⏟reproduction−mb(x,y)Nb⏟death−μ(x,y)Nb⏟transfer to Nd+σ(x,y)h(x,y)Nd⏟transfer from Nd,(x,y)∈Ω,t>0,Nd(x,y,0)=N0d(x,y),Nb(x,y,0)=N0b(x,y),(x,y)∈Ω,a(x,y)Nd+b(x,y)∂Nd∂→n=0,(x,y)∈∂Ω,t>0, | (2.1) |
where Nd(x,y,t) is the population density in the drifting water (unit: 1/volume) at location (x,y) and time t, Nb(x,y,t) is the population density on the benthos (unit: 1/area) at location (x,y) and time t, N0d and N0b are initial population densities, f is the reproduction rate of the population (unit: 1/time), md(x,y) is the mortality rate of the drift population at location (x,y) (unit: 1/time), mb(x,y) is the mortality rate of the benthic population at location (x,y) (unit: 1/time), D(x,y) is the diffusion rate (unit: area/time), μ(x,y) is the per capita rate at which individuals on the benthos enter the drift (unit: 1/time), σ(x,y) is the per capita rate at which individuals return to the benthos from the drift (unit: 1/time), h(x,y) is the river depth at location (x,y) (unit: length), v(x,y)=(v1(x,y),v2(x,y)) is the depth-averaged flow velocity at location (x,y) with v1(x,y) the flow velocity (unit: length/time) in the x direction and v2(x,y) the flow velocity (unit: length/time) in the y direction, ▽=(∂/∂x,∂/∂y), →n is the unit outward normal vector on the boundary, and a(x,y) and b(x,y) are nonnegative bounded functions on ∂Ω satisfying a2+b2≠0. For each t≥0, the solutions Nb(⋅,⋅,t) and Nd(⋅,⋅,t) are in the function space X, which is the continuous function space C(ˉΩ,R) or the subspace of C(ˉΩ,R) consisting of continuously differentiable functions vanishing on the boundary if Dirichlet conditions are applied to some part of the boundary.
The boundary conditions of (2.1) can be Dirichlet, Neumann or Robin conditions. The following typical conditions could be assumed in different portions of the boundary of a river.
(BI) At the river inflow (upstream boundary condition): zero-flux or zero density.
(ⅰ) Zero-flux (individuals cannot enter or leave at the source). The condition is
a(x,y)Nd+b(x,y)∂Nd∂→n=0 with a(x,y)=−v(x,y)⋅→n>0 since v and →n have opposite directions and b(x,y)=D(x,y).
(ⅱ) Zero density (hostile condition) (e.g., the living condition at the upstream end is extremely bad so that individuals die whenever they reach there): Nd=0.
(BB) Along the bank: zero-flux a(x,y)Nd+b(x,y)∂Nd∂→n=0 with a(x,y)=−v(x,y)⋅→n and b(x,y)=D(x,y).
(ⅰ) If no discharge flows into the river through the bank, then v and →n are perpendicular along the bank, hence a=0. Thus, the boundary condition becomes ∂Nd∂→n=0.
(ⅱ) If water flows into the river from the bank of the river (e.g., small streams or tributaries or groundwater flow entering the larger main river), then we have a(x,y)Nd+b(x,y)∂Nd∂→n=0 with a≥0 since v and →n have opposite directions.
(BO) At the river outflow (downstream boundary condition): free-flux or zero density.
(ⅰ) Free-flux (Danckwert's condition): ∂Nd∂→n=0. Individuals can freely leave the river with water flow.
(ⅱ) Zero density (hostile condition) (e.g., all individuals die at the downstream boundary): Nd=0.
We make the following assumptions throughout this paper.
(H1) D,h,v1,v2,∈C2(ˉΩ,(0,∞)).
(H2) μ,σ,md,mb are positive continuous functions in Ω.
(H3) The function f(x,y,Nb) is Lipschitz continuous with respect to Nb with Lipschitz constant c, f(x,y,0)−mb(x,y)<∞, f(x,y,Nb) is monotonically decreasing in Nb and for each (x,y) there exists a unique value K(x,y)>0 such that f(x,y,K(x,y))−mb(x,y)=0, f(x,y,0)−mb(x,y)−μ(x,y)≤0 on Ω.
(H4) μ,σ,md,mb, D, f, a and b do not depend on the vertical height of the location in the river.
In this section, we extend the theories of persistence measures for a one-dimensional benthic-drift model in [20] to obtain three river metrics for population persistence based on the linearized system of (2.1) at the trivial solution (0,0). To this end, we first introduce the next generation operator.
Linearizing system (2.1) at the trivial steady state (N∗d,N∗b)≡(0,0) yields
{∂Nd∂t=μ(x,y)h(x,y)Nb−σ(x,y)Nd−md(x,y)Nd+LNd,(x,y)∈Ω,t>0,∂Nb∂t=f(x,y,0)Nb−mb(x,y)Nb−μ(x,y)Nb+σ(x,y)h(x,y)Nd,(x,y)∈Ω,t>0,Nd(x,y,0)=N0d(x,y),Nb(x,y,0)=N0b(x,y),(x,y)∈ˉΩ,a(x,y)Nd+b(x,y)∂Nd∂→n=0,(x,y)∈∂Ω, | (3.1) |
where L is the linear partial differential operator defined as
LNd:=−1h(x,y)▽⋅(v(x,y)h(x,y)Nd)+1h(x,y)▽⋅(D(x,y)h(x,y)▽Nd), |
for any Nd∈C2(Ω,R).
Remark 1. If f(x,y,0)−mb(x,y)−μ(x,y)>0 on Ω, then
∂Nb∂t=(f(x,y,0)−mb(x,y)−μ(x,y))Nb+σ(x,y)h(x,y)Nd≥(f(x,y,0)−mb(x,y)−μ(x,y))Nb, |
which implies that Nb grows exponentially as t→∞ and (0,0) is unstable for (3.1). Hence, population persists for (2.1) regardless of other conditions. For this reason, we impose the last condition in (H3) in the rest of the paper for interesting results.
Suppose that a population is introduced into the river environment ˉΩ with initial distribution (ψ0d,ψ0b)∈X×X. The individuals in this population then experience dispersal, transfer between mobile and stationary classes and reproduction until they die, so the distribution of these initially introduced individuals at time t, denoted by (ψd(x,y,t),ψb(x,y,t)), is governed by the following system
{∂ψd∂t=μ(x,y)h(x,y)ψb−σ(x,y)ψd−md(x,y,0)ψd+Lψd,(x,y)∈Ω,t>0,∂ψb∂t=−mb(x,y,0)ψb−μ(x,y)ψb+σ(x,y)h(x,y)ψd,(x,y)∈Ω,t>0ψd(x,y,0)=ψ0d,ψb(x,y,0)=ψ0b(x,y),(x,y)∈Ω,a(x,y)ψd+b(x,y)∂ψd∂→n=0,(x,y)∈∂Ω. | (3.2) |
Then f(x,y,0)ψb(x,y,t) is the rate of reproduction by the initially introduced individuals at location (x,y) at time t, and therefore the total reproduction by the initially introduced individuals during their lifetime at (x,y) is given by ∫∞0f(x,y,0)ψb(x,y,t)dt. Noting that offsprings are reproduced only on the benthos, we have the following definition.
Definition 2. The next generation operator Γ:X×X→{0}×X associated with (3.1) is defined by
Γ(ψ0dψ0b)(x,y)=∫∞0(000f(x,y,0))(ψd(x,y,t)ψb(x,y,t))dt=(0f(x,y,0)∫∞0ψb(x,y,t)dt), | (3.3) |
where (ψd(x,y,t),ψb(x,y,t)), the distribution of the initially introduced individuals in the river at time t, is the solution of (3.2).
This next generation operator Γ maps an initial population distribution to its "next generation" (offspring) distribution.
Now we define three river metrics for population persistence as those in [20,31].
River metric 1: Fundamental niche Rloc(x,y) – a local persistence metric that describes the full range of environmental conditions and resources (biological and physical) that the organism can possibly occupy and use, especially when limiting factors are absent.
Assume that an individual only experiences birth and death after being introduced into the river but does not disperse during its lifetime. Define Rloc(x,y) as the number of offspring produced over its lifetime by an individual introduced at location (x,y) in the benthic zone. For (x,y)∈Ω,
Rloc(x,y)=f(x,y,0)∫∞0nb(x,y,t)dt=f(x,y,0)mb(x,y), | (3.4) |
where nb(x,y,t)=e−mb(x,y)tdt is the solution of
{dnbdt=−mb(x,y)nb(x,y,t),x∈Ω,t>0,nb(x,y,0)=1,x∈Ω. | (3.5) |
Then Rloc(x,y)>1 implies that an individual introduced at (x,y) will produce more than one offspring at (x,y) in the next generation, hence the population size at (x,y) will increase over generations. Therefore, locations with Rloc(x,y)>1 correspond to the fundamental niche of the species.
River metric 2: Source-sink distribution Rδ(x,y) – a local persistence metric that determines source and sink regions in the river.
Assume that an individual undergoes birth, death and dispersal after being introduced into the river and we use Rδ(x,y) to describe the number of offspring produced over its lifetime by an individual introduced at location (x,y) in the benthic zone.
Note that the next generation distribution from a single individual introduced at location (x0,y0) is defined by
f(x,y,0)∫∞0ψb(x,y,t)dt, |
where (ψd(x,y,t),ψb(x,y,t)) is the solution of (3.2) with initial conditions ψd(x,y,0)=0, ψb(x,y,0)=δ(x−x0)δ(y−y0) and δ(⋅) is the Dirac delta distribution. Then Rδ(x0,y0) is defined as
Rδ(x0,y0)=∫Ωf(x,y,0)∫∞0ψb(x,y,t)dtdxdy. | (3.6) |
If Rδ(x0,y0)>1, then an individual introduced at location (x0,y0) will produce more than one offspring in the whole river, we call location (x0,y0) a source. If Rδ(x0,y0)<1, then an individual introduced at location (x0,y0) will produce less than one offspring in the whole river, we call location (x0,y0) a sink.
River metric 3: Net reproductive rate R0 – a global persistence metric that determines population persistence or extinction in the whole river.
For any nonnegative initial distribution (N0d(x,y),N0b(x,y)) of the model (3.1), the associated next generation distribution is
Γ(N0dN0b)(x,y)=(0f(x,y,0)∫∞0Nb(x,y,t)dt), | (3.7) |
where (Nd(x,y,t),Nb(x,y,t)) solves (3.2) with initial condition (N0d,N0b).
Define
R0:=r(Γ), | (3.8) |
where r(Γ) is the spectral radius of the linear operator Γ on X×X. Then R0 represents the average number of offspring an individual may produce during its lifetime. We call R0 the net reproductive rate. Similarly as we did in [20], we can show the following result. A sketch of the proof is given in Appendix B.
Theorem 3. Assume (H1)–(H4) for (2.1). The following statements are valid:
(i) If R0<1, then the extinction equilibrium (0,0) is asymptotically stable for (2.1) for all nonnegative initial value conditions.
(ii) If R0>1, then there exists ϵ0>0 such that any nonnegative solution (Nd(x,y,t),Nb(x,y,t)) of (2.1) with (N0d,N0b)≥,≢0 satisfies
lim supt→∞‖(Nd(⋅,⋅,t),Nb(⋅,⋅,t)‖∞=lim supt→∞max(x,y)∈ˉΩ{Nd(x,y,t),Nb(x,y,t)}≥ϵ0. | (3.9) |
Remark 4. Theorem 3 implies that R0 is a threshold for global dynamics of the population in the whole river. Population persists in the river in the sense that the inequality (3.9) is valid if R0>1; population will be extinct in the river if R0<1.
River2D is a hydrodynamic and fish habitat model developed specifically for use in natural streams and rivers. We implemented the population model into River2D to calculate river metrics R0 and Rδ for a species in a river with two dimensional depth-averaged velocities. Starting with creating a preliminary bed topography file from the raw river field data, we edited and refined the data, developed a computational discretization of the river, and then solved for the water depths and velocities throughout the discretization under given inflow and outflow conditions. To obtain steady river flow conditions the River2D model is run from an initial set of conditions with constant inflow and outflow conditions until a steady state of the river is obtained. We then implemented the two dimensional population model equations into the steady river flow in River2D to calculate R0 (see (3.8)) as well the next generation distribution and Rδ (see (3.6)). River2D is a finite element model, based on a conservative Petrov-Galerkin upwinding formulation (see e.g., [7]). The hydrodynamic component of the River2D model is based on the two-dimensional, depth averaged Saint Venant Equations expressed in conservative form. See [15,16,43] for more details about River2D.
In what follows, we present the methods to calculate Rδ and R0 for a benthic-drift model in a spatially two-dimensional (longitudinal-lateral) river in River2D.
For numerical calculation convenience, we introduce a new variable Nv for the offspring that the initially introduced individuals reproduce and consider the following system based on the linearization of model (2.1):
∂Nd(x,y,t)∂t=−σ(x,y)⋅Nd(x,y,t)+μ(x,y)h(x,y)⋅Nb(x,y,t)−md(x,y)⋅Nd(x,y,t)−1h(x,y)∂∂x[v1(x,y)h(x,y)Nd(x,y,t)]+1h(x,y)∂∂x[D(x,y)h(x,y)∂Nd(x,y,t)∂x]−1h(x,y)∂∂y[v2(x,y)h(x,y)Nd(x,y,t)]+1h(x,y)∂∂y[D(x,y)h(x,y)∂Nd(x,y,t)∂y]∂Nb(x,y,t)∂t=σ(x,y)⋅Nd(x,y,t)⋅h(x,y)−μ(x,y)⋅Nb(x,y,t)−mb(x,y)⋅Nb(x,y,t)∂Nv(x,y,t)∂t=f(x,y,0)Nb(x,y,t)Nd(x,y,0)=0,Nb(x,y,0)=N0b,Nv(x,y,0)=0,a(x,y)Nd+b(x,y)∂Nd∂→n=0,(x,y)∈∂Ω,t>0. | (4.1) |
Note that individuals can stay on the benthos or drift in water but the recruitment only occurs when they stay on the benthos. Here Nb and Nd are densities of benthic population and drift population of the first generation (i.e., initially introduced population), respectively; Nv is the population density of the second generation (i.e., offspring of the first generation population). Individuals are only introduced on the benthos. The boundary condition along the river bank is chosen as the no-flux condition and the conditions at the upstream and downstream ends can be chosen as any from boundary assumptions (BI) and (BO) in Section 2. In numerical simulations in the next section, we use the following hostile upstream and Dankwert's downstream conditions,
Nd|upstream=0,∂Nd∂→n|downstream=0, | (4.2) |
or zero flux upstream and Dankwert's downstream conditions,
−(v(x,y)⋅→n)Nd+D(x,y)∂Nd∂→n|upstream=0,∂Nd∂→n|downstream=0. | (4.3) |
For a well-defined river, we first run the river in River2D to the steady flow to obtain v(x,y) and h(x,y). Then we implement the above population model into the steady flow to calculate river metrics. The algorithms for computing Rδ and R0 are given as below.
Note that Nv(x,y,∞) is the total number of new offspring produced at location (x,y) and ∫ΩNv(x,y,∞)dxdy is the total number of offspring produced by initially introduced individuals in their lifetime in the whole river. If a single individual is introduced at location (x0,y0)∈Ω, then
Rδ(x0,y0)=∫ΩNv(x,y,∞)dxdy, | (4.4) |
where Nv(x,y,∞) is the third compartment of the solution to system (4.1) as t→∞, with initial distribution Nb(x0,y0,0)=N0b(x,y)=δ(x−x0,y−y0)=δ(x−x0)⋅δ(y−y0), where δ is the Dirac delta function. Numerically, in River2D, (4.4) is approximated by
Rδ(x0,y0)=∑(xi,yj)∈ˉΩNv(xi,yj,∞), | (4.5) |
where Nv(xi,yj,∞) is approximated by Nv(xi,yj,t1) for sufficiently large t1 and {(xi,yj)i∈I,j∈J} is a division of the river region ˉΩ in the simulation.
Consider system (4.1). For any initially introduced population distribution (0,N0b,0) with ∫ΩN0b(ξ,η)dξdη=1, define
Nl+1v(x,y)=N(l)v(x,y,∞),l=0,1,2,⋯,Nlb(x,y)=Nlv(x,y)∫ΩNlv(ξ,η)dξdη,l=1,2,3,⋯, | (4.6) |
where N(l)v(x,y,∞) is the third compartment of the solution to system (4.1) with the normalized initial condition (0,Nlb,0) as t→∞. Note that the total population size of Nlb is 1 since ∫ΩNlb(ξ,η)dξdη=1. Equations in (4.6) indicate that N(l)v(⋅,⋅,∞) is the final distribution of offspring of the initial population Nlb of total size 1.
In the numerical program in River2D, we approximate R0 by
R0≈∑(xi,yj)∈ˉΩNlv(xi,yj)=∑(xi,yj)∈ˉΩN(l−1)v(xi,yj,∞), | (4.7) |
and the next generation function by
ϕ∗(xi,yj)≈Nlv(xi,yj),(xi,yj)∈ˉΩ, | (4.8) |
for sufficiently large positive integer l, where {(xi,yj)i∈I,j∈J} is a division of the river region ˉΩ in simulation. Intuitively, the above approximations in (4.7) and (4.8) illustrate that R0 represents the average number of offspring a single individual produces in the whole river in its lifetime and that the distribution of all offspring of a single individual is ϕ∗n(x,y). Mathematically, the reason why the net reproductive rate R0 defined in (3.8) can be approximated by (4.7) is given in Appendix C.
Remark 5. The approximations (4.5), (4.7) and (4.8) for Rδ, R0 and the eigenfunction are derived for the general model (4.1); nevertheless, our current numerical program in River2D only assumes constant parameters for (4.1).
We consider a river with an island (see Figure 1) and find persistence metrics of a population in the river. The river is in a region with dimensions 458 m by 247 m (unit: m). The bed elevation and effective bed roughness height are defined as in Figure 1. We consider two flow cases: the instream flow of 200 m3/s and the outflow water depth of 0.5 m; the instream flow of 400 m3/s and the outflow water depth of 0.5 m. Under such flow boundary conditions, the steady flow conditions can be calculated in River2D and the plan view distributions of the water depth and flow velocity are shown in Figure 2.
We then introduce a population into the river and calculate Rδ and R0 as well as the corresponding next generation function under these two different flow conditions. The zero-flux condition is chosen along the bank and hostile conditions (4.2) are chosen at the upstream and downstream ends. We choose the following model parameters: D(x,y)≡1 m2/s, σ(x,y)≡0.75/s, μ(x,y)≡1.8/s, mb(x,y)≡md(x,y)≡0.1/s, and f(x,y,0)≡r=0.5 or 0.322/s.
Two different cases are considered. Case 1: Q=200 m3/s and r=0.5/s; case 2: Q=400 m3/s and r=0.322/s. We obtain that R0=1.54978 in case 1 and R0=0.986818 in case 2. The Rδ distribution and the next generation function are shown in Figure 3, respectively. It follows from Theorem 3 that the population persists in the river in case 1 (when Q=200 m3/s) and will be extinct in case 2 (when Q=400 m3/s). When the flow discharge is low (i.e., in case 1), the Rδ distribution shows that most parts of the river are source regions (Rδ(x,y)>1) that are suitable to introduce the population if it is expected to grow in the river. The next generation function distribution shows that the next generation offspring will not be distributed near the upstream end. They occupy other parts of the river, especially in the middle parts, and this ensures the persistence of the population in the whole river. However, when the flow discharge is large (i.e., in case 2), the population will go extinct even though there are some source regions in the river. Note that we choose different birth rate r(x,y) in the examples here to easily illustrate the phenomenon of persistence and extinction. In fact, even with the same birth rate, the population may persist under low flow but will be extinct under high flow.
We consider a two dimensional periodic meandering river and study the influence of ecological factors and flow characteristics on population persistence metrics in such a river.
The channel of a meandering river is represented by a sine generated curve θ=θmsin(2πs/Ls) (see Figure 4), where θ represents the angle between the channel and the longitudinal line with the maximum θm, s is the distance from the upstream end of the river, Ls is the length of one period of the channel, and the lateral bed slope is tanα where α=αmsin(2πs/Ls) with maximum |αm|. See also, e.g., [27]. We selected the following parameter values to build the meandering river profile for input to the River2D environment: the river length L=800m, the channel period Ls=200 m, the width 20 m, the longitudinal slope of the river bottom S0=0.0002, θm=π/4, αm=−π/1000, the bottom roughness n=0.1m. See Figure 5. In particular, most nodal points inside the numerical domain are uniformly distributed with a 5 m spacing, but nodes near the boundary are slightly shifted and the space discretization on the boundary is smaller than 5m, for better numerical results.
With a specific constant flow discharge at the upstream boundary, River2D can simulate the steady state flow for this river, where the water depths and flow velocities vary periodically. See Figure 6 for the steady flow when Q=0.1m3/s and Q=10m3/s.
1. The co-influence of the flow discharge and the population growth rate. Consider model (2.1) in the meandering river with boundary conditions (4.2). We calculate the net reproductive rate R0 and obtain the next generation function as well as the source/sink distribution Rδ under conditions of different birth rates, death rates, and flow discharges. Figure 7 shows the relationship between R0 and the flow discharge Q under different growth rate conditions. R0 decreases as the flow increases, but increases as the birth rate increases. When the flow only carries organisms to the downstream but does not help them grow, it has only a negative effect on population persistence in the river; a higher growth rate clearly helps population persistence. This coincides with the analysis and simulations for persistence conditions for single population models and benthic-drift population models; see [20,31,38]. Figures 8 and 9 show the next generation function distribution and the distribution of Rδ under different flow conditions. While the next generation function values are almost zero in the first three periods of the river, Figures 8 only shows the next generation function distribution in the last period of the river. Note that the next generation function corresponds to the steady distribution of offspring. This indicates that if the upstream boundary condition is hostile, then in a long run, the next generation concentrates near the downstream end, especially in the region with low flow velocity at the downstream end. Figure 9 shows that the source regions are located in the shallow areas with relatively low flow velocities and are more likely in the upstream. When the flow discharge becomes larger, the downstream contains more sink regions and hence becomes a worse environment for the population to live. Note that here we assume that the flow only has a negative effect on the population. In some rivers, the flow may help persistence by bringing nutrients to organisms; see [19] for drift feeders. In our simulations, the birth rate is assumed to be constant. If the birth rate increases in the downstream direction, then the increased flow plays a trade-off role (see [31] and [20] for models in one-dimensional rivers). Moreover, higher flow rate may be associated with changes in dissolved oxygen [50], hence it would be interesting to investigate how the flow rate affects the concentration of dissolved oxygen in a river, and thus affects the population survival.
2. The effect of the transmission rates. We consider the influence of individuals' transfer rates between the benthos and the water column on population persistence. Figure 10 shows the net reproductive rate and corresponding next generation function under boundary conditions (4.3) for different transfer rates. We choose the ratio between σ (the transfer rate from benthos to water column) and μ (the transfer rate from water column to benthos) to be 1/4. It shows that the smaller the transfer rate is, the larger the net reproductive rate is, which coincides with the result from the benthic-drift model in a one-dimensional river (see Figure 5 in [20]). Therefore, when the ratio between transfer rates is fixed, more frequent transfer between the benthos and the water column does not help population persistence in the whole river. Note that Remark 1 implies that if f(x,y,0)≡r>μ(x,y)+mb(x,y), then population persistence is guaranteed. In our situation, this means that if σ(x,y)<(f(x,y,0)−mb(x,y))/4, then there is unconditional persistence, regardless of the flow rate or other parameter conditions. This is consistent with the result for the benthic-drift model in a one-dimensional river in [38] and again indicates that the lower the transfer is, the better it would be for population persistence.
3. The effect of the diffusion rate. The net reproductive rate and corresponding next generation function with boundary conditions (4.3) for different diffusion rates are shown in Figure 11. The net reproductive rate slightly increases with the diffusion rate here. Hence, under the flow condition given in the example, diffusion in the water column helps population persistence in the river. This is because in a river with low flow more random movement of the population increases the chance of individuals' upstream dispersal and hence helps persistence since the upstream end is not lethal. This is consistent with the results from the one-dimensional models; see e.g., Figure 3.2 in [23].
4. The effect of the river bottom roughness height. The net reproductive rate and next generation function with boundary conditions (4.2) corresponding to different river bottom roughness heights are shown in Figure 12. The net reproductive rate increases with the bottom roughness height n of the river. As the bottom roughness indicates the living condition on the benthos, this implies that a river with a rough bottom (e.g., a river with rocks or stones on the bottom) is better for a population to live and persist than a river with smooth bottom (e.g. sand). It has been shown in [22] (see Figure 3(d) in [22]) that the river bottom roughness increases the upstream speed of a population in a one-dimensional river. Since the conditions for positive upstream speed in an infinitely long river and that for population persistence in a bounded river have been proved to be the same in many literatures (see e.g., [23,24,29]), our result that the river bottom roughness helps population persistence in a two-dimensional river is essentially consistent with that in [22].
5. The effect of the river bottom slope. The net reproductive rate and next generation function with boundary conditions (4.2) corresponding to different river bottom slopes are shown in Figure 13. The net reproductive rate decreases as the river bottom slope increases. Therefore, it is easier for the population to persist in a river with flatter bottom than in a river with steeper bottom. This is also consistent with the result in [22] that the river bottom slope decreases the upstream speed of a population in a one-dimensional river. In a previous work [20] for a one-dimensional benthic-drift model, we observed that when deep pools are close to the upstream, more offspring are produced and live upstream to avoid being washed out and hence deep pools close to upstream help population persistence. In a meandering river constructed as in our examples, deep pools and shallow regions may appear on the same cross section, so the effect of pools on persistence may not be as clear as in the one-dimensional case.
6. The effect of boundary conditions. We use boundary conditions (4.2) (with hostile upstream boundary condition) and (4.3) (with zero-flux upstream boundary conditions) in above simulations. It can been seen from Figures 8 to 15 that boundary conditions greatly influence the value of the net reproductive rate and the next generation function distribution as well as the source/sink regions. When a hostile boundary condition is applied at the upstream end, the upstream part of the river becomes a poor region for the distribution of the next generation function; the offspring occupy only the region near the downstream end and the density near the downstream end becomes high. See Figures 8, 12, and 13. By way of contrast, when the zero-flux boundary condition is applied at the upstream end, the entire river becomes a more suitable region for the next generation distribution, and hence, the next generation offspring are more evenly distributed in the river, although the population density is higher in deep water than in shallow water. See Figures 10, 11, and 14. Moreover, the zero-flux boundary condition at the upstream end yields a much larger net reproductive rate than a hostile boundary condition does. The source/sink regions in the river are also very different under the two different boundary conditions. Under the hostile boundary condition at the upstream, the upstream end is the sink region and the source regions are concentrated in the shallow areas in the river (see Figure 9). However, under the zero-flux condition at the upstream, Rδ attains its maximum at the upstream end and the upstream end becomes the most important source region for the population; see Figure 15. The different scales in the lower part of Figure 15 also illustrate why R0 is higher when Q=10 than when Q=0.1; Rδ is an order of magnitude larger when Q=10 compared to when it is Q=0.1. We also see a strange phenomenon, unlike in Figure 7, about the next generation function distribution for system (4.1) under the zero-flux upstream boundary condition (4.3), when varying the flow discharge; see Figure 14. When the flow discharge increases, the net reproductive rate first increases and then decreases. This seems to be hard to understand. When the water discharge increases, the water depth and flow velocity increase throughout the river. It is hard to see which parameter causes the drop of the net reproductive rate when the discharge increases from 5 to 10.
Understanding hydrodynamics and ecological dynamics is crucial in stream and river management. While there is a need to integrate hydraulic and biological features to discover how river morphology and water flows affect the ecological status of rivers [8,40], a reasonable and efficient method for such integration becomes critical. Based on the fruitful development of habitat models in river habitat assessment, most existing methods (e.g., the physical habitat simulation model (PHABSIM), ecological limits of hydrologic alteration (ELOHA) framework, Software for Assisted Habitat Modeling (SAHM), etc.) link habitat suitability index of river species (e.g., fish) to physical conditions, focusing primarily on habitat suitability and availability; see e.g., [4,32,33,36,40,43,44,46,48].
A few works have attempted to incorporate ecological factors explicitly into hydrodynamic modeling analysis; see e.g., [2,6,18,22,30]. The modelling framework in this paper extends the approach in [22]. The results therein have made it possible to directly analyze how river morphology influences short and long term behaviors of a population in a river. In the current work, by analyses and calculations for the coupled hydrodynamics and population models in partial differential equations, we are able to determine the source/sink regions and global dynamics of a specific population in a two-dimensional depth-averaged river model. Hydraulic, physical and demographic features are explicitly incorporated into the model and their effects on suitable habitat or population persistence/extinction can be numerically analyzed.
Our results showed that the increase of the growth rate and the diffusion rate yields larger net reproductive rate and helps population persistence while larger transfer rate decreases the net reproductive rate and drives population to be extinct. Moreover, higher river bottom roughness height helps population persist but higher bottom slope leads to population extinction more quickly, which might be due to the fact that changes to these parameters change the flow velocity, to a lower level in the former case and to a higher level in the latter case. When the upstream end is imposed with hostile boundary condition, the offspring concentrate in the downstream region and the source regions are in the shallow areas throughout the river. When the upstream end is imposed with the zero-flux boundary condition, the offspring concentrate in deep water regions and the source regions are mainly in the shallow water regions with the most significant source at the upstream end. We also saw a challenging phenomenon which is very hard to understand: When the upstream end is imposed with the zero-flux conditions, when the flow discharge increases, the net reproductive rate may increase first and then decrease.
In order to use the River2D program to calculate Rδ and R0 for a population in a river, the following data need to be collected by ecologists and river managers: Biological data including the birth rate, the death rate, the diffusion rate, transfer rates between the benthos and the drifting water, and population boundary conditions; river bed topography data including bed location (x-coordinate, y-coordinate), bed elevation, bed roughness height, and boundary type; and flow data such as the upstream inflow and the downstream water surface level. It is our hope that this work would be a useful tool for ecologists to predict or control long term behaviors of river and stream species and for water resources managers in identifying more accurately the targets for flow regulation.
In the current River2D program, the biological parameters (birth, death, diffusion, and transfer rates) are assumed to be constants. We will further incorporate spatial heterogeneities of these parameters in the program so that it can be widely used to investigate population persistence and source-sink dynamics in more realistic scenarios. Moreover, since the living conditions for aquatic species in rivers can vary seasonally, the theory developed in this work could be extended to more general situations by incorporating temporal variations in population demography features and flow regime. As a future work, we may consider dynamics of interacting species models coupled with hydrodynamic equations and hence provide better river ecology management strategies.
The authors thank Peter M. Steffler for valuable discussions and comments. The authors also wish to thank Lewis Lab for fruitful discussions. Y. J. gratefully acknowledges an AMS-Simons Travel grant and NSF Grant (DMS 1411703). Q. H. gratefully acknowledges support from NSF of China (11871060), the Fundamental Research Funds for the Central Universities (XDJK2018B031), and the faculty startup fund from Southwest University (20710948). M. A. L. also gratefully acknowledges a Canada Research Chair, NSERC Discovery and Acceleration grants, and a Killam Research Fellowship.
The authors declare no conflict of interest.
We first develop a single-compartment model, and then extend it to a benthic-drift model by dividing the river channel into two zones and the population into two corresponding groups.
Let N(x,y,t) be the population density (unit: quantity/volume) at location (x,y) and time t. Let h(x,y) be the water depth (unit: length) at location (x,y) and v(x,y)=(v1(x,y),v2(x,y)) be the depth averaged flow velocity (unit: length/time) at (x,y) with v1 and v2 being the flow velocities in the x and y directions, respectively.
Consider a water flow box containing the cross-section through (x,y) with the volume estimated by ΔV=ΔxΔyh(x,y) (see Figure 16). We want to understand how the population density in the box changes over time due to flows of population into and out of the box. In the x-direction, the population enters the box with a flux Jx and leaves the box with a flux Jx+Δx. In the y-direction, the population enters the box with a flux Jy and leaves the box with a flux Jy+Δy. The flux expresses the density of population that passes a unit area per unit of time. It has dimension density/(area⋅time). The population density that flows into or out of the box in the x direction per unit time can be estimated by the product of the flux in the x direction and the area of the surface over which the flux occurs, Jx⋅Ax, or Jx+Δx⋅Ax+Δx. Similar formulas apply for the population inflow and outflow in the y direction. We then have the balance equation:
∂N∂t=Jx⋅Ax−Jx+Δx⋅Ax+Δx+Jy⋅Ay−Jy+Δy⋅Ay+ΔyΔV=JxΔyh(x,y)−Jx+ΔxΔyh(x+Δx,y)+JyΔxh(x,y)−Jy+ΔyΔxh(x,y+Δy)ΔxΔyh(x,y)+o(Δx)+o(Δy). | (A.1) |
Letting Δx,Δy→0, we have
∂N∂t=−1h[∂(hJx)∂x+∂(hJy)∂y]. | (A.2) |
Microscopically, the flux due to advection can be written as
Jx|advection=v1(x,y)N,Jy|advection=v2(x,y)N. | (A.3) |
The flux due to dispersion can be written as
Jx|dispersion=−D(x,y)∂N∂x,Jy|dispersion=−D(x,y)∂N∂y, | (A.4) |
where D>0 is the diffusion coefficient. Moreover, Jx=Jx|advection+Jx|dispersion and Jy=Jy|advection+Jy|dispersion.
By combining the flux divergence formula (A.2) with the microscopic formula for advection (A.3) and dispersive flux (A.4) and also considering a reproduction or decay process of the population, we obtain the following general 2-dimensional model for a population that only lives in the drifting water.
∂N∂t=g(x,y,N)N−1h(x,y)[∂∂x(v1(x,y)h(x,y)N(x,y,t))+∂∂y(v2(x,y)h(x,y)N(x,y,t))]+1h(x,y)[∂∂x(D(x,y)h(x,y)∂N(x,y,t)∂x)+∂∂y(D(x,y)h(x,y)∂N(x,y,t)∂y)], | (A.5) |
where g(x,y,N) is the population growth rate.
Hydrologically, the presence of free-flowing water zones on the top and transient storage zones along the bottom in rivers is an important hydraulic characteristics in the ecology of streams. In the storage zones, water movement can be approximated as zero flow [5,10]. Ecologically, many aquatic organisms reside mainly in the storage zone but occasionally jump into the free-flowing zone and drifting downstream until they settle down on the benthos again [1]. Motivated by these facts, we extend the single-compartment model (A.5) to the following benthic-drift model by partitioning the river into two zones, drift zone and benthic zone, and dividing the population into two interacting compartments, individuals residing on the benthos and individuals dispersing in the drift zone:
{∂Nd∂t=μ(x,y)h(x,y)Nb⏟transfer from Nb−σ(x,y)Nd⏟transfer to Nb−md(x,y)Nd⏟death−1h(x,y)▽⋅(v(x,y)h(x,y)Nd)⏟advection+1h(x,y)▽⋅(D(x,y)h(x,y)▽Nd)⏟diffusion,∂Nb∂t=f(x,y,Nb)Nb⏟reproduction−mb(x,y)Nb⏟death−μ(x,y)Nb⏟transfer to Nd+σ(x,y)h(x,y)Nd⏟transfer from Nd, | (A.6) |
where Nd and Nb represent the population density in the drifting water and the population density on the benthos, respectively, f is the reproduction rate of the population md and mb are the mortality rates of individuals in the drift and individuals on the benthos, respectively, and ▽=(∂/∂x,∂/∂y).
Theorem 3 can be proved by following the same process as in Section 3.3 in [20]. Substituting Nd(x,y,t)=eλtϕ1(x,y) and Nb(x,y,t)=eλtϕ2(x,y) into (3.1), we obtain the associated eigenvalue problem
{Lϕ1−(σ(x,y)+md(x,y))ϕ1+μ(x,y)h(x,y)ϕ2=λϕ1,(x,y)∈Ω,(f(x,y,0)−mb(x,y)−μ(x,y))ϕ2+σ(x,y)h(x,y)ϕ1=λϕ2,(x,y)∈Ω,a(x,y)ϕ1+b(x,y)∂ϕ1∂→n=0,(x,y)∈∂Ω. | (B.1) |
By applying similar arguments as in Theorem 3 and Lemma 4 in [20], we can prove the following results.
Lemma 6. (a) The eigenvalue problem (B.1) has a simple principal eigenvalue λ∗ with a positive eigenfunction if f(x,y,0)−mb(x,y)−μ(x,y)<0.
(b) R0−1 and λ∗ have the same sign, where λ∗ is the principal eigenvalue of (B.1).
Then by using similar arguments as in the proof of Theorem 5 in [20], we can obtain the following results.
(ⅰ) If λ∗<0, then the extinction equilibrium (0,0) is asymptotically stable for (2.1) for all nonnegative initial value conditions.
(ⅱ) If λ∗>0, then there exists ϵ0>0 such that any positive solution of (2.1) satisfies
lim supt→∞‖(Nd(⋅,⋅,t),Nb(⋅,⋅,t)−(0,0)‖∞=lim supt→∞max(x,y)∈ˉΩ{Nd(x,y,t),Nb(x,y,t)}≥ϵ0. |
These results together with Lemma B.1 complete the proof of Theorem 3.
Let (Nd(x,y,t),Nb(x,y,t),Nv(x,y,t)) be the solution of system (4.1) with initially introduced population distribution (0,N0b,0). Denote the final distribution of offsprings by N1v, i.e.,
N1v(x,y)=Nv(x,y,∞)=limt→∞Nv(x,y,t). |
Let ˉΓ:X→X be defined as
ˉΓ(N0b)(x,y):=N1v(x,y)=Nv(x,y,∞). | (C.1) |
By using similar derivation as in Appendix A.5 in [20], we can obtain the formula of the operator ˉΓ:
ˉΓ(φ)(x,y)=f(x,y,0)φ(x,y)mb(x,y)+μ(x,y)+σ(x,y)Ad(x,y)f(x,y,0)(mb(x,y)+μ(x,y))Ab(x,y)∫L0∫L0k(x,y,ξ,η)μ(ξ,η)Ab(ξ,η)φ(ξ,η)(mb(ξ,η)+μ(ξ,η))Ad(ξ,η)dξdη | (C.2) |
for any φ∈X, where k(x,y,ξ,η) is the solution of the ordinary boundary value problem
{(L−σ(x,y)−md(x,y)+σ(x,y)μ(x,y)mb(x,y)+μ(x,y))k(x,y,ξ,η)=−δ(x−ξ)δ(y−η),(x,y)∈Ωa(x,y)Nd+b(x,y)∂Nd∂→n=0,(x,y)∈∂Ω,t>0, | (C.3) |
for a fixed point (ξ,η)∈Ω. Note that the solution to (C.3) is a Green's function (see (2–11) in Chapter 7 in [17], (2.9) in Chapter 3 in [42], or Appendix B in [31]). Furthermore, we obtain the following result.
Proposition 7. The spectral radius of Γ is equal to the spectral radius of ˉΓ, i.e.,
R0=r(Γ)=r(ˉΓ). | (C.4) |
Proof. Define an operator ˆΓ:X→X as
ˆΓ(φ)(x,y)=f(x,y,0)φ(x,y)mb(x,y)+μ(x,y)+σ(x,y)Ad(x,y)(mb(x,y)+μ(x,y))Ab(x,y)∫L0∫L0k(x,y,ξ,η)f(ξ,η,0)μ(ξ,η)Ab(ξ,η)φ(ξ,η)(mb(ξ,η)+μ(ξ,η))Ad(ξ,η)dξdη, | (C.5) |
for all φ∈X, where k(x,y,ξ,η) is the solution of the ordinary boundary value problem (C.3). By similar proof as for Theorem 6 in [20], we obtain that the spectral radius of Γ is equal to the spectral radius of ˆΓ, i.e.,
r(Γ)=r(ˆΓ). |
Define two operators T1,T2:X→X:
T1(φ)(x,y)=f(x,y,0)φ(x,y),T2(φ)(x,y)=φ(x,y)mb(x,y)+μ(x,y)+σ(x,y)Ad(x,y)(mb(x,y)+μ(x,y))Ab(x,y)∫L0∫L0k(x,y,ξ,η)μ(ξ,η)Ab(ξ,η)φ(ξ,η)(mb(ξ,η)+μ(ξ,η))Ad(ξ,η)dξdη, | (C.6) |
for all φ∈X, where k is defined in (C.3). Then both T1 and T2 are bounded and linear, and we further have ˆΓ=T2∘T1, ˉΓ=T1∘T2. This yields the result that the spectral radii of these two operators are the same, i.e.,
r(ˆΓ)=limn→∞||(T2∘T1)n||1/n=limn→∞||(T1∘T2)n||1/n=r(ˉΓ). |
Hence, we obtain
R0=r(Γ)=r(ˆΓ)=r(ˉΓ). |
To numerically approximate ˉΓ, we can discretize (C.2) in the river region ˉΩ. Divide ˉΩ into a grid (uniform except in the area near the boundary). Let {(xi,yj)i∈I,j∈J} represent all the nodes of the grid. Let n be the total number of the nodes and rearrange all nodes such that they are represented by a sequence of points {Ph,1≤h≤n}. By applying a numerical quadrature (e.g., Newton-Cotes, Gauss, Simpson, etc.) to (C.2), we can approximate (C.2) by
ˉΓ(φ)(Ph)≈n∑s=1ηh,sφ(Ps),1≤h≤n, |
where ηh,s>0 depends on parameter functions in (4.1) and the numerical quadrature (see e.g., [37,45]). Let
ˉΓn=(ηh,s). |
Then we can approximate the operator ˉΓ by the positive matrix operator ˉΓn and hence approximate r(ˉΓ) by r(ˉΓn), i.e.,
r(ˉΓ)≈r(ˉΓn), | (C.7) |
for large positive integer n.
The Perron-Frobenius Theorem implies that ˉΓn admits a positive principal eigenvalue λ∗n which is equal to r(ˉΓn) and is associated with a positive eigenvector ϕ∗n. Since ˉΓn is a positive matrix, we can use the power method to numerically calculate λ∗n. For any nonnegative function N0b with ∑nh=1N0b(Ph)=1, let
Nlb(Ph)=ˉΓnNl−1b(Ph)∑nh=1ˉΓnNl−1b(Ph),1≤h≤n,l=1,2,3,⋯. | (C.8) |
We then have
(Nlb)T(ˉΓnNlb)(Nlb)TNlb→λ∗n and Nlb→ϕ∗n, as l→∞. | (C.9) |
Note that we use ˉΓn to approximate ˉΓ. The definition of ˉΓ in (C.1) implies that ˉΓnNl−1b approximates the offspring distribution of system (4.1) with the initial population Nl−1b. Denote
Nlv(Ph)=ˉΓnNl−1b(Ph),1≤h≤n,l=1,2,⋯. |
(C.9) implies that
n∑h=1Nlv(Ph)=n∑h=1ˉΓnNl−1b(Ph)→λ∗n,asl→∞ | (C.10) |
and
Nlv=ˉΓnNl−1b→λ∗nϕ∗n, as l→∞. | (C.11) |
Following this idea, we do not directly find ˉΓn to estimate λ∗n (and hence R0) in our River2D simulations. Instead, we solve (4.1) numerically for any nonnegative function N0b satisfying ∑(xi,yj)∈ˉΩN0b(xi,yj)=1, and define the following sequence by following the idea as in (C.1) and (C.8):
Nl+1v(xi,yj)=N(l)v(xi,yj,∞),l=0,1,2,⋯,Nlb(xi,yj)=Nlv(xi,yj)∑(xi,yj)∈ˉΩNlv(xi,yj),l=1,2,3,⋯, | (C.12) |
where N(l)v(xi,yj,∞) is the offspring compartment of the numerical solution to system (4.1) with the normalized initial condition (0,Nlb,0) as t→∞. Then by (C.4), (C.7), (C.10) and (C.11), we approximate R0 by
R0=r(Γ)=r(ˉΓ)≈r(ˉΓn)=λ∗n≈∑(xi,yj)∈ˉΩNlv(xi,yj), | (C.13) |
and the next generation function by
ϕ∗(xi,yj)≈ϕ∗n(xi,yj)≈Nlv(xi,yj), | (C.14) |
for sufficiently large positive integer l.
[1] |
Aisaiti G, Liu L, Xie J, et al. (2019) An empirical analysis of rural farmers' financing intention of inclusive finance in China The moderating role of digital finance and social enterprise embeddedness. Ind Manage Data Syst 119: 1535–1563. doi: 10.1108/IMDS-08-2018-0374
![]() |
[2] |
Ao G, Liu Q, Qin L, et al. (2021) Organization model, vertical integration, and farmers' income growth: Empirical evidence from large-scale farmers in Lin'an, China. PLoS One 16: e0252482. doi: 10.1371/journal.pone.0252482
![]() |
[3] | Chen B, Ni Y (2018) Study on the Influence of Inclusive Finance Development Level on Urban-Rural Income Gap in Guizhou Province. Proceedings of the 3rd International Seminar on Education Innovation and Economic Management (SEIEM 2018). Available from: https://doi.org/10.2991/seiem-18.2019.144. |
[4] | Fan Q, Wang X (2013) An Empirical Analysis of the Relationship between the Residents's Education Years and Income, In: Q. Luo & T. Zhang (Eds. ), 2013 International Conference on Education and Educational Research, 2: 524–528. |
[5] |
Geng Z, He G (2021) Digital financial inclusion and sustainable employment: Evidence from countries along the belt and road. Borsa Istan Rev 21: 307–316. doi: 10.1016/j.bir.2021.04.004
![]() |
[6] | Guo F, Wang J, Wang F, et al. (2020) Measuring China's digital financial inclusion: Index Compilation and Spatial Characteristics. China Econ Q 19: 1401–1418. |
[7] |
Gorelick J, Walmsley N (2020) The greening of municipal infrastructure investments: technical assistance, instruments, and city champions. Green Financ 2: 114–134. doi: 10.3934/GF.2020007
![]() |
[8] |
Han W, Wei Y, Cai J, et al. (2021) Rural nonfarm sector and rural residents' income research in China. An empirical study on the township and village enterprises after ownership reform (2000–2013). J Rural Stud 82: 161–175. doi: 10.1016/j.jrurstud.2021.01.001
![]() |
[9] |
Kawabata T (2020) Private governance schemes for green bond standard: influence on public authorities' policy making. Green Financ 2: 35–54. doi: 10.3934/GF.2020003
![]() |
[10] |
Kemfert C, Schmalz S (2019) Sustainable finance: political challenges of development and implementation of framework conditions. Green Financ 1: 237–248. doi: 10.3934/GF.2019.3.237
![]() |
[11] |
Li J, Wu Y, Xiao JJ (2020) The impact of digital finance on household consumption: Evidence from China. Econ Model 86: 317–326. doi: 10.1016/j.econmod.2019.09.027
![]() |
[12] | Li K, Zhao H, Liu X (2017) The Impact of Rural Financial Development on the Income Gap of Rural Residents in China: An Empirical Analysis Based on Semi-parametric Additive Model, In: Y. Hou & W. Zheng (Eds. ), Proceedings of the 2017 3rd International Conference on Economics, Social Science, Arts, Education and Management Engineering, 119: 1650–1655. |
[13] |
Li T, Liao G (2020a) The Heterogeneous Impact of Financial Development on Green Total Factor Productivity. Front Energy Res 8: 29. doi: 10.3389/fenrg.2020.00029
![]() |
[14] |
Li T, Li X (2020b) Does structural deceleration happen in China? Evidence from the effect of industrial structure on economic growth quality. Natl Account Rev 2: 155–173. doi: 10.3934/NAR.2020009
![]() |
[15] | Li W, Wu P, Destech Publicat I (2018) Rural Financial Development, Agricultural Fiscal Investment and Rural Residents' Income, In 2018 International Conference on Economic Management Science and Financial Innovation, 198–204. |
[16] | Li Y (2020) Empirical analysis of the impact of financial development on the income gap between urban and rural residents in the context of large data using fuzzy Kmeans clustering algorithm. Int J Electr Eng Educ, 0020720920936837. |
[17] |
Li Y, Zhong Q, Xie L (2020) Has Inclusive Finance Narrowed the Income Gap between Urban and Rural Areas? An Empirical Analysis Based on Coastal and Noncoastal Regions' Panel Data. J Coastal Res, 305–308. doi: 10.2112/SI106-071.1
![]() |
[18] |
Li Z, Chen L, Dong H (2020a) What are bitcoin market reactions to its-related events? Int Rev Econ Financ 73: 1–10. doi: 10.1016/j.iref.2020.12.020
![]() |
[19] | Li Z, Dong H, Floros C, et al. (2021) Re-examining Bitcoin Volatility: A CAViaR-based Approach. Emerg Mark Financ Trade, 1–19. |
[20] |
Li Z, Liao G, Albitar K (2019) Does corporate environmental responsibility engagement affect firm value? The mediating role of corporate innovation. Bus Strat Environ 29: 1045–1055. doi: 10.1002/bse.2416
![]() |
[21] |
Li Z, Wang Y, Huang Z (2020b) Risk connectedness heterogeneity in the cryptocurrency markets. Front Phys 8: 243. doi: 10.3389/fphy.2020.00243
![]() |
[22] | Li Z, Zou F, Tan Y, et al. (2021) Does Financial Excess Support Land Urbanization-An Empirical Study of Cities in China. Land 10. |
[23] | Liu G (2009) Analysis of Economic Factors on Restricting Consumption of Rural Residents in China, Proceedings of 2009 International Conference on Management Science and Engineering, 1016–1020. |
[24] |
Liu T, He G, Turvey CG (2021) Inclusive Finance, Farm Households Entrepreneurship, and Inclusive Rural Transformation in Rural Poverty-stricken Areas in China. Emerg Mark Financ Trade 57: 1929–1958. doi: 10.1080/1540496X.2019.1694506
![]() |
[25] | Liu X, Mu Q, ChenY (2021) Reform and Improvement in China's Rural Financial Sector Based on Internet Technology. Paper presented at the 2021 2nd International Conference on E-Commerce and Internet Technology (ECIT). |
[26] | Liu Y, Li Z, Xu M (2019) The Influential Factors of Financial Cycle Spillover: Evidence from China. Emerg Mark Financ Tr, 1–15. |
[27] |
Liu Y, Liu C, Zhou M (2021) Does digital financial inclusion promote agricultural production for rural households in China? Research based on the Chinese family database (CFD). China Agric Econ Rev 13: 475–494. doi: 10.1108/CAER-06-2020-0141
![]() |
[28] | Liu Z, Wei Y, Li Q, et al. (2021) The Mediating Role of Social Capital in Digital Information Technology Poverty Reduction an Empirical Study in Urban and Rural China. Land 10. |
[29] |
Luo Y, Peng Y, Zeng L (2020) Digital financial capability and entrepreneurial performance. Int Rev Econ Financ 76: 55–74. doi: 10.1016/j.iref.2021.05.010
![]() |
[30] |
Matei I (2020) Is financial development good for economic growth? Empirical insights from emerging European countries. Quant Financ Econ 4: 653–678. doi: 10.3934/QFE.2020030
![]() |
[31] |
Pan H, Liu Y, Gao H (2012) Impact of agricultural industrial structure adjustment on energy conservation and income growth in Western China: a statistical study. Ann Oper Res 228: 23–33. doi: 10.1007/s10479-012-1291-2
![]() |
[32] | Rahman MM, Guan F, Ara LA (2019) Nonlinear Dynamics in the Finance-Inequality Nexus in China-CHNS Data. Sustainability 11. |
[33] |
Ren B, Li L, Zhao H, et al. (2018) The Financial Exclusion in The Development of Digital Finance— A Study Based on Survey Data in The Jingjinji Rural Area. Singap Econ Rev 63: 65–82. doi: 10.1142/S0217590818500017
![]() |
[34] | Song Q, Li J, Wu Y, et al. (2020) Accessibility of financial services and household consumption in China: Evidence from micro data. North Am J Econ Financ, 53. |
[35] |
Sukharev OS (2020) Structural analysis of income and risk dynamics in models of economic growth. Quant Financ Econ 4: 1–18. doi: 10.3934/QFE.2020001
![]() |
[36] | Tan Y, Yuan Y (2013) Agricultural Insurance and the Urban-Rural Income Gap—Based on a Dynamic Panel Model of the GMM Estimation, Proceedings of 2013 China International Conference on Insurance and Risk Management, 215–224. |
[37] | Tong G, Li C (2011) An Empirical Analysis on Effecting Factor of Urban-Rural Income Disparity in Heilongjiang Province, In: M. L. Dai (Ed. ), Innovative Computing and Information, 2011, Pt Ii 232: 499–509. |
[38] |
Wang J, Hu Y (2018) The impact of trade liberalization on poverty reduction in rural China. China Agric Econ Rev 10: 683–694. doi: 10.1108/CAER-01-2018-0019
![]() |
[39] | Wang X, Chen M, He X, et al. (2018) Credit Constraint, Credit Adjustment, and Sustainable Growth of Farmers' Income. Sustainability 10. |
[40] | Wang X, He G (2020) digital financial inclusion and Farmers' Vulnerability to Poverty: Evidence from Rural China. Sustainability 12. |
[41] | Wang X, Liu L (2016) How County-Level Agricultural Loans and Fiscal Expenditure Impact Rural Residents' Income in China-An Empirical Study of the Hierarchical Effect by Quantile Regression. Front Econ China 11: 302–320. |
[42] |
Xie W, Wang T, Zhao X (2020) Does Digital financial inclusion Promote Coastal Rural Entrepreneurship? J Coastal Res, 240–245. doi: 10.2112/SI103-052.1
![]() |
[43] | Yang M, Wang S (2012) Empirical Study on Financial Development and the Income of Urban Residents in China—Example as Our Countries Data. Contemp Innovation Dev Stat Sci 2012: 684–689. |
[44] | Yang Y, Fu C (2019) Inclusive Financial Development and Multidimensional Poverty Reduction: An Empirical Assessment from Rural China. Sustainability 11. |
[45] | Yu LL, Zhao DY, Xue ZH, et al. (2020) Research on the use of digital finance and the adoption of green control techniques by family farms in China. Technol Society 62. |
[46] | Yu N, Wang Y (2021) Can Digital financial inclusion Narrow the Chinese Urban–Rural Income Gap? The Perspective of the Regional Urban–Rural Income Structure. Sustainability 13. |
[47] |
Zhang H, Xiong X (2019) Is financial education an effective means to improve financial literacy? Evidence from rural China. Agric Financ Rev 80: 305–320. doi: 10.1108/AFR-03-2019-0027
![]() |
[48] | Zhao P, Yu Z (2021) Rural poverty and mobility in China: A national-level survey. J Transp Geogr 93. |
[49] | Zhong J, Li T (2020) Impact of Financial Development and Its Spatial Spillover Effect on Green Total Factor Productivity: Evidence from 30 Provinces in China. Math Probl Eng, 1–11. |
[50] | Zhou Y, She D, Cai Y (2015) An Empirical Study of the Effect of Financial Development on the Urban-Rural Residents Income Gap in China, Proceedings of 2015 International Symposium—Open Economy & Financial Engineering, 67–71. |