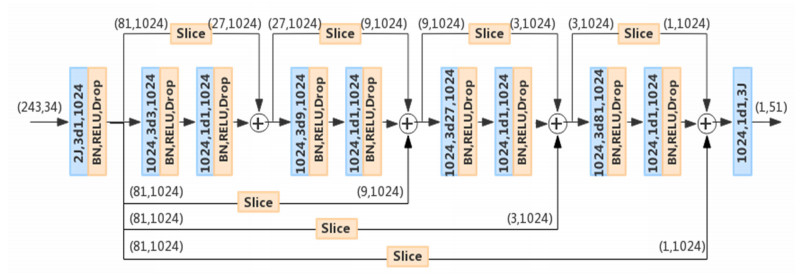
Neurosurgeons receive extensive and lengthy training to equip themselves with various technical skills, and neurosurgery require a great deal of pre-, intra- and postoperative clinical data collection, decision making, care and recovery. The last decade has seen a significant increase in the importance of artificial intelligence (AI) in neurosurgery. AI can provide a great promise in neurosurgery by complementing neurosurgeons' skills to provide the best possible interventional and noninterventional care for patients by enhancing diagnostic and prognostic outcomes in clinical treatment and help neurosurgeons with decision making during surgical interventions to improve patient outcomes. Furthermore, AI is playing a pivotal role in the production, processing and storage of clinical and experimental data. AI usage in neurosurgery can also reduce the costs associated with surgical care and provide high-quality healthcare to a broader population. Additionally, AI and neurosurgery can build a symbiotic relationship where AI helps to push the boundaries of neurosurgery, and neurosurgery can help AI to develop better and more robust algorithms. This review explores the role of AI in interventional and noninterventional aspects of neurosurgery during pre-, intra- and postoperative care, such as diagnosis, clinical decision making, surgical operation, prognosis, data acquisition, and research within the neurosurgical arena.
Citation: Mohammad Mofatteh. Neurosurgery and artificial intelligence[J]. AIMS Neuroscience, 2021, 8(4): 477-495. doi: 10.3934/Neuroscience.2021025
[1] | Jaouhra Cherif, Anis Raddaoui, Ghofrane Ben Fraj, Asma Laabidi, Nada Souissi . Escherichia coli's response to low-dose ionizing radiation stress. AIMS Biophysics, 2024, 11(2): 130-141. doi: 10.3934/biophy.2024009 |
[2] | Tika Ram Lamichhane, Hari Prasad Lamichhane . Structural changes in thyroid hormone receptor-beta by T3 binding and L330S mutational interactions. AIMS Biophysics, 2020, 7(1): 27-40. doi: 10.3934/biophy.2020003 |
[3] | Irina A. Zamulaeva, Kristina A. Churyukina, Olga N. Matchuk, Alexander A. Ivanov, Vyacheslav O. Saburov, Alexei L. Zhuze . Dimeric bisbenzimidazoles DB(n) in combination with ionizing radiation decrease number and clonogenic activity of MCF-7 breast cancer stem cells. AIMS Biophysics, 2020, 7(4): 339-361. doi: 10.3934/biophy.2020024 |
[4] | Reham Ebrahim, Aya Abdelrazek, Hamed El-Shora, Abu Bakr El-Bediwi . Effect of ultraviolet radiation on molecular structure and photochemical compounds of Salvia hispanica medical seeds. AIMS Biophysics, 2022, 9(2): 172-181. doi: 10.3934/biophy.2022015 |
[5] | Soad Hasanin, A. G. ELshahawy, Hamed M El-Shora, Abu Bakr El-Bediwi . Gamma radiation effects on vitamins, antioxidant, internal and molecular structure of Purslane seeds. AIMS Biophysics, 2022, 9(3): 246-256. doi: 10.3934/biophy.2022021 |
[6] | Francesca Ballarini, Mario P. Carante, Alessia Embriaco, Ricardo L. Ramos . Effects of ionizing radiation in biomolecules, cells and tissue/organs: basic mechanisms and applications for cancer therapy, medical imaging and radiation protection. AIMS Biophysics, 2022, 9(2): 108-112. doi: 10.3934/biophy.2022010 |
[7] | Mohamed A. Elblbesy . The refractive index of human blood measured at the visible spectral region by single-fiber reflectance spectroscopy. AIMS Biophysics, 2021, 8(1): 57-65. doi: 10.3934/biophy.2021004 |
[8] | Erma Prihastanti, Sumariyah Sumariyah, Febiasasti Trias Nugraheni . Increasing growth of monobulb garlic through the application of corona glow discharge plasma radiation and organic fertilizers. AIMS Biophysics, 2024, 11(1): 85-96. doi: 10.3934/biophy.2024006 |
[9] | Tika Ram Lamichhane, Hari Prasad Lamichhane . Heat conduction by thyroid hormone receptors. AIMS Biophysics, 2018, 5(4): 245-256. doi: 10.3934/biophy.2018.4.245 |
[10] | C. Dal Lin, M. Falanga, E. De Lauro, S. De Martino, G. Vitiello . Biochemical and biophysical mechanisms underlying the heart and the brain dialog. AIMS Biophysics, 2021, 8(1): 1-33. doi: 10.3934/biophy.2021001 |
Neurosurgeons receive extensive and lengthy training to equip themselves with various technical skills, and neurosurgery require a great deal of pre-, intra- and postoperative clinical data collection, decision making, care and recovery. The last decade has seen a significant increase in the importance of artificial intelligence (AI) in neurosurgery. AI can provide a great promise in neurosurgery by complementing neurosurgeons' skills to provide the best possible interventional and noninterventional care for patients by enhancing diagnostic and prognostic outcomes in clinical treatment and help neurosurgeons with decision making during surgical interventions to improve patient outcomes. Furthermore, AI is playing a pivotal role in the production, processing and storage of clinical and experimental data. AI usage in neurosurgery can also reduce the costs associated with surgical care and provide high-quality healthcare to a broader population. Additionally, AI and neurosurgery can build a symbiotic relationship where AI helps to push the boundaries of neurosurgery, and neurosurgery can help AI to develop better and more robust algorithms. This review explores the role of AI in interventional and noninterventional aspects of neurosurgery during pre-, intra- and postoperative care, such as diagnosis, clinical decision making, surgical operation, prognosis, data acquisition, and research within the neurosurgical arena.
artificial intelligence;
brain-computer interface;
computer-assisted diagnosis;
computerised tomography;
deep learning;
machine learning;
magnetic resonance imaging;
traumatic brain injury;
temporal lobe epilepsy
Human pose estimation is a key technology in the field of computer vision. Its output is the basis of down-stream tasks such as action recognition, visual tracking and action analysis. The early work in human pose estimation was mainly limited to a 2D plane, and the goal is to get the body joints' 2D coordinates from 2D images or videos. In recent years, 3D body pose estimation has become popular because it provides more accurate data with depth information. 3D pose estimation can be categorized into three types, according to the input: from a monocular image [1,2,3,4], from multi-camera images [5,6] and from a depth image [7,8,9]. Monocular 3D human pose estimation is the most popular, and it is widely used in applications such as virtual reality, intelligent video analysis and human-computer interaction.
At present, there are two main branches for monocular 3D human pose estimation. One is the so-called two-stage method, which first estimates the 2D human pose and then lifts it to a 3D human pose. An example is the weakly supervised model [10]. The other is the end-to-end 3D human pose estimation method, which predicts the 3D human pose directly from images or videos. Examples are the adversarial learning method [11], the self-supervised approach [12] and the famous Transformer [13]. Because the human pose shows spatial correlation, some work tried to extract skeleton features in the spatial domain. Liu et al. [14] employed graph networks with weight sharing to do 3D pose estimation. The stacked graph hourglass model [15] tried to capture multi-scale spatial correlation. With the goal of capturing both the spatial and temporal correlation of a human pose, Zhang et al. [16] proposed a spatial-temporal encoder to learn spatial-temporal correlations. In comparison with the two-stage model, the end-to-end model regresses the 3D pose directly from the 2D image, which provides the model with rich information. However, it usually requires the support of large-scale human pose datasets. The 2D pose datasets includes Leeds Sports Pose Dataset (LSP) [17], Frames Labeled In Cinema (FLIC) [18], Max Planck Institut Informatik (MPII) [19] and Microsoft COCO: Common Objects in Context (MSCOCO) [20]. The 3D pose datasets include HumanEva [21], MPI-INF-3DHP and human 3.6M [22]. For 3D human poses, it is a very challenging task to obtain large-scale labels. Therefore, most of the existing data are collected in the laboratory using motion capture systems (such as human 3.6M), and the backgrounds are relatively simple and very limited in number. Due to these limitations, the end-to-end methods usually perform better in some specific scenarios but cannot generalize well to applications in natural scenes. In order to improve the model's generalizability, Gholami et. al. [23] proposed adapting the training data to the test dataset, such as camera viewpoint, position, human actions and body size.
The two-stage model estimates the 3D pose by two steps. It first gets a 2D pose by a 2D detector, and then regresses the 3D pose from the 2D. Obviously, the two-stage model will heavily rely on the 2D detector; however, the 2D pose datasets are more sufficient than 3D and contain much in-the-wild data. Thus, the 2D detector will be trained by more diverse data, and the two-stage model can be expected to show better generalizability. In addition, the two-stage model also has an advantage of low complexity. Therefore, in this paper, we adopt it to predict the 3D human pose with the 2D human skeleton as input.
The main contributions of this work include the following:
1) We design a multi-stage supervision temporal convolution network to capture human dynamics by temporal continuity constraints. In addition, the network is trained in a multi-stage supervision manner to improve the model.
2) We impose that the model is to be consistent with general human pose dynamic knowledge and introduce human body pose geometry to the network training step, so as to improve the model generality.
The task of 3D pose estimation is more challenging than 2D pose estimation, because it needs to regress relative depth between body joints, which suffers from severe ambiguity.
For the two-stage 3D pose estimation models, Martinez et. al. [24] designed a simple multi-layer network and regressed the 3D pose from the 2D pose skeleton. At first, this work analyzed the reasons for low accuracy in 3D pose estimation, and determined two aspects: the low accuracy of the 2D estimation, and the mapping from 2D to 3D. In fact, the 2D detector has achieved very high performance, so this paper focused on estimating the 3D pose from the 2D. Therefore, at second, they designed a very simple and lightweight network, and achieved good performance. Their work demonstrated the effectiveness of the two-stage model.
Based on Martinez's work, Fang et al. [25] extended it by a pose semantic network to code joints' dependency and correlations. Because recurrent neural network is good at learning temporal correlations, it was also introduced in Martinez's model. Hossian and Little [26] used Long short-term memory (LSTM) to capture the temporal continuity and achieved accuracy improvement. However, this model cannot deal with long-term sequential data, because it will lead to the gradient vanishing and gradient explosion problems.
Temporal convolution networks provide a new way to capture temporal continuity, so they are also used in the field of pose estimation. WaveNet [27] proved the convolution model's advantages in capturing temporal information. WaveNet is constructed by 1D convolution, and it can prevent the problems of gradient vanishing and gradient explosion. In addition, it is of high efficiency, because it can process temporal data in parallel. Based on this model, Pavllo et. al. [28] designed a temporal convolution model to estimate 3D body pose. This model generates the 2D pose sequence first by the 2D detector and then estimates the 3D pose. In comparison with WaveNet, the temporal convolution model is advantaged in learning the implicit kinematics knowledge. Instead of estimating 3D human pose from monocular images, videos can provide temporal information to improve accuracy and robustness. Several works [29,30,31] utilized spatial-temporal relationships and constraints such as bone-length and left-right symmetry to improve performance. In this paper, we employed the temporal convolution model and improved it in two ways: First, we design a multi-stage supervision model to further explore the periodic motion pattern; second, we introduce the prior geometry knowledge to generalize the model.
The performance of data-driven model is limited by the dataset, so prior knowledge is imposed to the deep models in many computer vision fields [32,33,34]. With the goal of decreasing depth ambiguity, some work tried to introduce human geometric knowledge into the 3D pose estimation model. Belagiannis et. al. [35] imposed kinematic constraints on the translation and rotation between body parts in the 3D pictorial model, and the symmetric body parts are constrained not to collide with each other. Ronchi et. al. [36] imposed limb length loss and measured the difference in length between the predicted limb and the predefined reference length. In fact, we can develop many kinematic constraints, such as limb lengths, limb length proportions, joint angles, occlusion constraints, appearance constraints and temporal smoothness constraints. Kinematics is crucial prior knowledge for the deep models, and it constrains the model predictions to be reasonable when measured by body geometry.
In the temporal domain, human movement always shows continuity according to human kinematics. If we can capture the temporal continuity, it will provide important information for the 3D pose estimation model. In this paper, we employed the temporal convolution network to model the periodical human kinematics.
The model structure is shown in Figure 1. The network takes a 2D human pose sequence of size (17 joints' 2D pose coordinates, and 243 is the sequence length) as input. The input sequence is passed through four same consecutive modules, which are composed of a 1D convolution with 3 convolution kernels and 1024 output channels, batch normalization layer, Rectified Linear Unit (ReLu) and dropout. Each module is added by a residual connection, as shown in the upper part of Figure 1. The dimension of the input data is directly reduced through a specific slice function (Slice), and the results are added to the output data of the module. The channel number is 1024 in the module. Each module contains two different convolution layers. The first convolution layer applies extended convolution, which is mainly used to extract data features. The kernel size is 3, and the expansion rate is 3 (3d3 in the figure). With the increase of modules, the kernel size is fixed, but the expansion rate increases exponentially. As shown in this figure, the expansion rate of the second module is 9, while it is 27 for the third and 81 for the last. Different from the first convolution layer, the kernel size for the second one is 1, which is used to increase the depth of the network and improve the nonlinearity. At the end of the network, a convolution layer is applied to output 3D human posture.
In order to avoid the gradient vanishing problem, we add residual connection to enhance the gradient propagation. As shown in Figure 1, we add multi-level residual connection to supervise the network in multiple stages. In the process of forward propagation, multi-level residual connection enables shallow features to be directly propagated to the upper layer. The features of the shallow layer are combined with high-level features as input to the next layer. Combining features at different levels helps to reduce network degradation and improve network generalization performance. In the process of back propagation, the gradient can be transmitted to the lower layer faster without too much intermediate weight matrix transformation, so it can effectively alleviate gradient vanishing. The time-series convolution network based on multi-stage supervision makes the feature information more smoothly spread in the forward and backward directions, so the network has better optimization performance, which will further improve the model accuracy.
The input is the joints' 2D coordinates of the consecutive frames , and the model will output the joints' 3D coordinate estimations (). The loss function is defined as the Euclidean distance between the estimated 3D pose and the ground-truth ():
(1) |
in which N is the batch size and is set to be 1024 in our case. We use gradient descent to optimize the model and exponential decay to update the learning rate. The learning rate is set to be 0.001, and the decay rate is 0.95.
Relying on joints' coordinates only tends to cause ambiguity when restoring 3D coordinates from 2D. Therefore, the geometric prior knowledge of human kinematics is introduced in this part. We employed the distance between the ground-truth coordinates and the estimated joints' coordinates after translation, rotation and scale transformation.
(2) |
where is the transformation operation. In addition, we also introduced the geometry consistency as constraints, including bone length symmetry and proportions. The skeleton of a normal human body is symmetrical: for example, the bone length of the left shoulder is the same as that of the right shoulder.
The bone length symmetry constraint:
(3) |
The bones connecting human joints are divided into four groups . includes left and right upper arms and left and right lower arms. includes the left and right thighs and the left and right calves. contains the left shoulder bone and the right shoulder bone, and contains the left hip bone and the right hip bone. is the length of the selected bone. The length of the bone at the symmetrical position is obtained through the function. For example, if is the right thigh, will be the left thigh. controls the bone length by calculating the error of each group of bones and constrains the bone length at symmetrical positions.
The bone length proportions constraint:
(4) |
(5) |
The bones are also grouped into four groups . is the bone length, is its corresponding mean length in its datasets. The proportion should be consistent in the same group. is the mean proportion in group .
The constraints and the accuracy loss are integrated as the final loss:
(6) |
in which are the weights of each constraint.
We tested our method on the Human3.6M and MPI-INF-3DHP datasets. The Human3.6M dataset contains 15 actions of 11 testers, with a total of 3.6 million video frames. For the task of 3D human pose estimation, there are mainly three standard evaluation protocols based on this dataset: Protocol 1 (MPJPE) is the average joint position error in millimeters, which is the Euclidean distance between the predicted joint position and the real position. Protocol 2 (P-MPJPE) is the error after the predicted joint position is aligned with the real position after translation, rotation and retraction. Protocol 3 (N-MPJPE) is the error after aligning the predicted joint position with the real position only after scaling. Among the three protocols, Protocol 1 (MPJPE) is the most widely used. However, for the method of predicting 3D human pose based on sequence, absolute position error cannot measure the smoothness of prediction over time. In order to evaluate this, Pavllo et al. [28] measured the joint velocity error (MPJVE), which is a time-based velocity motion measurement and the first-order derivative of MPJPE's 3D pose error. For the Human 3.6M dataset, we employed the 17 joint skeletons, used 5 testers (S1, S5, S6, S7, S8) for training and 2 testers (S9, S11) for testing and trained a general model for 15 actions. The MPI-INF-3DHP test set [37] provides images in three different scenarios: studio with a green screen (GS), studio without green screen (noGS) and outdoor scene (Out- door). We use this dataset to test the generalization ability of our model and use 3D-PCK and AUC as evaluation metrics.
In this section, we employ the 2D ground-truth data (2D skeletons of 243 frames) as the input to the second stage. The channel number is set to be 1024. As shown in Tables 1–3, the proposed model with multi-stage intermediate supervision achieved lower error when evaluated by all three protocols.
Dir. | Disc. | Eat | Greet | Phone | Photo | Pose | Purch. | |
Pavllo et al. [28] | 26.0 | 29.8 | 24.6 | 27.0 | 25.8 | 29.4 | 29.2 | 26.7 |
Ours | 25.7 | 29.2 | 25.1 | 27.0 | 25.8 | 30.4 | 28.7 | 25.9 |
Sit. | SitD. | Smoke | Wait | WalkD | Walk | WalkT | Avg | |
Pavllo et al. [28] | 31.7 | 34.6 | 27.4 | 27.3 | 27.9 | 21.5 | 21.8 | 27.4 |
Ours | 30.7 | 35.0 | 27.2 | 26.8 | 27.7 | 21.4 | 22.6 | 27.3 |
Dir. | Disc. | Eat | Greet | Phone | Photo | Pose | Purch. | |
Pavllo et al. [28] | 26.0 | 29.8 | 24.6 | 27.0 | 25.8 | 29.4 | 29.2 | 26.7 |
Ours | 25.7 | 29.2 | 25.1 | 27.0 | 25.8 | 30.4 | 28.7 | 25.9 |
Sit. | SitD. | Smoke | Wait | WalkD | Walk | WalkT | Avg | |
Pavllo et al. [28] | 31.7 | 34.6 | 27.4 | 27.3 | 27.9 | 21.5 | 21.8 | 27.4 |
Ours | 30.7 | 35.0 | 27.2 | 26.8 | 27.7 | 21.4 | 22.6 | 27.3 |
Dir. | Disc. | Eat | Greet | Phone | Photo | Pose | Purch. | |
Pavllo et al. [28] | 36.0 | 39.1 | 31.4 | 35.6 | 33.5 | 38.0 | 40.5 | 34.7 |
Ours | 34.5 | 37.4 | 31.7 | 34.8 | 33.5 | 39.1 | 39.2 | 33.2 |
Sit. | SitD. | Smoke | Wait | WalkD | Walk | WalkT | Avg | |
Pavllo et al. [28] | 41.8 | 41.4 | 34.9 | 36.8 | 34.5 | 26.4 | 27.1 | 35.5 |
Ours | 40.1 | 40.9 | 33.9 | 35.4 | 34.3 | 26.6 | 27.7 | 34.8 |
Table 1 shows the evaluation by Protocol 1 (MPJPE). The proposed method shows performance improvement on most actions, even for some relatively difficult actions, such as "sitting", "sittingD", and "Discussion". Averagely, the error is reduced by about 1 mm, and the prediction accuracy is increased by 2.7%. Table 2 shows the evaluation by Protocol 2 (P-MPJPE). The proposed model achieved 0.1 mm error reduction and about 1 mm for the actions of "Sitting". Table 3 is based on Protocol 3 (N-MPJPE), where the proposed model achieved about 0.7 mm error reduction and about 1.7 mm for the actions of "Sitting" and "Discussion". The proposed model also achieved lower error in joint velocity, as shown in Table 4, which means better temporal smoothness.
Dir. | Disc. | Eat | Greet | Phone | Photo | Pose | Purch. | |
Pavllo et al. [28] | 1.92 | 1.97 | 1.48 | 2.27 | 1.42 | 1.79 | 1.85 | 2.16 |
Ours | 1.92 | 1.94 | 1.45 | 2.24 | 1.39 | 1.75 | 1.80 | 2.11 |
Sit. | SitD. | Smoke | Wait | WalkD | Walk | WalkT | Avg | |
Pavllo et al. [28] | 1.11 | 1.53 | 1.40 | 1.59 | 2.68 | 2.29 | 1.91 | 1.83 |
Ours | 1.07 | 1.49 | 1.37 | 1.56 | 2.64 | 2.27 | 1.90 | 1.79 |
In order to test performance of regressing the 3D pose directly from the 2D image, we employed a Cascade Pyramid Network (CPN) [38] as the 2D detector in the two-stage model, and the predicted 2D skeletons' sequence is input to the 3D estimator. Table 5 compares the proposed model with the state-of-the-art models, where "U" is the model with multi-stage supervision, and "U+L" is the model with multi-stage supervision and geometry constraints. Our method achieved the best result on almost all the actions. For the actions "Phone" and "Photo", our model performs worse than the baseline model. For these two actions, the kinematics feature is not as obvious as other actions in both spatial and temporal domain, especially for the action "Phone, " so our model did not show advantages. For the action "Photo, " the "U+L" model performs better than "U" model, which means the geometry constraints are effective for this action.
Dir. | Disc. | Eat | Greet | Phone | Photo | Pose | Purch. | |
Martinez et al. [24] | 51.8 | 56.2 | 58.1 | 59.0 | 69.5 | 78.4 | 55.2 | 58.1 |
Sun et al. [39] | 52.8 | 54.8 | 54.2 | 54.3 | 61.8 | 67.2 | 53.1 | 53.6 |
Fang et al. [25] | 50.1 | 54.3 | 57.0 | 57.1 | 66.6 | 73.3 | 53.4 | 55.7 |
Pavlakos et al. [40] | 48.5 | 54.4 | 54.4 | 52.0 | 59.4 | 65.3 | 49.9 | 52.9 |
Yang et al. [41] | 51.5 | 58.9 | 50.4 | 57.0 | 62.1 | 65.4 | 49.8 | 52.7 |
Luvizon et al. [42] | 49.2 | 51.6 | 47.6 | 50.5 | 51.8 | 60.3 | 48.5 | 51.7 |
Hossain and Little [26] | 48.4 | 50.7 | 57.2 | 55.2 | 63.1 | 72.6 | 53.0 | 51.7 |
Lee et al. [43] | 40.2 | 49.2 | 47.8 | 52.6 | 50.1 | 75.0 | 50.2 | 43.0 |
Pavllo et al. [28] | 45.2 | 46.7 | 43.3 | 45.6 | 48.1 | 55.1 | 44.6 | 44.3 |
Liu et al. [14] | 46.3 | 52.2 | 47.3 | 50.7 | 55.5 | 67.1 | 49.2 | 46.0 |
Xu and Takano [15] | 45.2 | 49.9 | 47.5 | 50.9 | 54.9 | 66.1 | 48.5 | 46.3 |
Ours (U) | 44.6 | 46.5 | 43.0 | 45.4 | 48.4 | 57.3 | 43.9 | 43.7 |
Ours (U+L) | 44.3 | 46.1 | 42.5 | 45.2 | 48.4 | 56.0 | 43.9 | 43.5 |
Sit. | SitD. | Smoke | Wait | WalkD. | Walk | WalkT | Avg. | |
Martinez et al. [24] | 74.0 | 94.6 | 62.3 | 59.1 | 65.1 | 49.5 | 52.4 | 62.9 |
Sun et al. [39] | 71.7 | 86.7 | 61.5 | 53.4 | 61.6 | 47.1 | 53.4 | 59.1 |
Fang et al. [25] | 72.8 | 88.6 | 60.3 | 57.7 | 62.7 | 47.5 | 50.6 | 60.4 |
Pavlakos et al. [40] | 65.8 | 71.1 | 56.6 | 52.9 | 60.9 | 44.7 | 47.8 | 56.2 |
Yang et al. [41] | 69.2 | 85.2 | 57.4 | 58.4 | 43.6 | 60.1 | 47.7 | 58.6 |
Luvizon et al. [42] | 61.5 | 70.9 | 53.7 | 48.9 | 57.9 | 44.4 | 48.9 | 53.2 |
Hossain and Little [26] | 66.1 | 80.9 | 59.0 | 57.3 | 62.4 | 46.6 | 49.6 | 58.3 |
Lee et al. [43] | 55.8 | 73.9 | 54.1 | 55.6 | 58.2 | 43.3 | 43.3 | 52.8 |
Pavllo et al. [28] | 57.3 | 65.8 | 47.1 | 44.0 | 49.0 | 32.8 | 33.9 | 46.8 |
Liu et al. [14] | 60.4 | 71.1 | 51.5 | 50.1 | 54.5 | 40.3 | 43.7 | 52.7 |
Xu and Takano [15] | 59.7 | 71.5 | 51.4 | 48.6 | 53.9 | 39.9 | 44.1 | 51.9 |
Ours (U) | 56.6 | 64.3 | 47.0 | 43.9 | 49.2 | 32.7 | 33.7 | 46.7 |
Ours (U+L) | 56.7 | 64.6 | 45.6 | 43.6 | 48.9 | 32.6 | 33.7 | 46.4 |
With predicted 2D pose as input, the model shows less accuracy than the model with ground-truth 2D pose as input. As shown in Table 5, the average prediction error is reduced by about 2.3%. In comparison, the "U+L" model shows better performance than the "U" model, and averagely achieved 0.8% improvement. The model with "U+L" achieved the lowest error on almost all the actions. We can see that the "U+L" model has the same number of parameters as the "U" model but better generalization ability.
We applied the model trained on Human 3.6m to the MPI-INF-3DHP dataset, to test the model's generalizability. Table 6 shows the results and comparison with the state-of-the-art models. Trained only on the Human 3.6M dataset, our model shows good generalizability, due to the general knowledge being data independent.
Training Data | GS | noGS | Outdoor | All (PCK) | All (AUC) | |
Martinez et al. [24] | Human3.6M | 49.8 | 42.5 | 31.2 | 42.5 | 17.0 |
Zhou et al. [44] | Human3.6M+MPII | 75.6 | 71.3 | 80.3 | 75.3 | 38.0 |
Xu and Takano [15] | Human3.6M | 81.5 | 81.7 | 75.2 | 80.1 | 45.8 |
Ours (U) | Human 3.6M | 81.7 | 81.6 | 75.4 | 80.2 | 46.0 |
Ours (U+L) | Human3.6M | 81.6 | 81.7 | 75.0 | 80.1 | 45.8 |
In addition to the above quantitative experimental results, we also visualize the 3D pose results for the Human3.6M dataset. Figure 2 shows the prediction effects of some actions, including eating, talking on the phone and smoking. It can be seen from the figure that the proposed model effectively restores the human pose in 3D space with a high prediction accuracy.
For 3D pose estimation, this paper proposed developing human kinematics in two ways. First, we employed the temporal convolution network to extract the temporal continuity and supervised by constructing multi-stage intermediate connections to alleviate gradient vanishing. Second, we introduced geometry constraints to improve the model generalizability. When tested on two public datasets, the proposed model showed comparable performance with the state-of-the-art models. Developing human kinematics is important information for a data-driven model. This paper presents a preliminary study, and developing more kinematics will provide the data-driven model with more effective prior knowledge, which is also our future work.
We would like to thank for the support by the Science and Technology Development Program of Jilin Province (20220101102JC) and Jilin Province Professional Degree Postgraduate Teaching Case Construction Project.
The authors declare there is no conflict of interest.
[1] |
Wise J (2020) Life as a neurosurgeon. BMJ 368: m395. doi: 10.1136/bmj.m395
![]() |
[2] |
Kaptigau WM, Rosenfeld JV, Kevau I, et al. (2016) The establishment and development of neurosurgery services in Papua New Guinea. World J Surg 40: 251-257. doi: 10.1007/s00268-015-3268-1
![]() |
[3] |
Rolston JD, Zygourakis CC, Han SJ, et al. (2014) Medical errors in neurosurgery. Surg Neurol Int 5: S435-S440. doi: 10.4103/2152-7806.142777
![]() |
[4] |
Kwoh YS, Hou J, Jonckheere EA, et al. (1988) A robot with improved absolute positioning accuracy for CT guided stereotactic brain surgery. IEEE Trans Biomed Eng 35: 153-160. doi: 10.1109/10.1354
![]() |
[5] | Aziz T, Roy H (2021) Deep Brain Stimulation. Oxford Research Encyclopedia of Psychology Oxford University Press. |
[6] |
Panesar SS, Kliot M, Parrish R, et al. (2020) Promises and perils of artificial intelligence in neurosurgery. Neurosurgery 87: 33-44. doi: 10.1093/neuros/nyz471
![]() |
[7] | Bohl MA, Oppenlander ME, Spetzler R (2016) A prospective cohort evaluation of a robotic, auto-navigating operating microscope. Cureus 8: e662-e662. |
[8] |
Lanfranco AR, Castellanos AE, Desai JP, et al. (2004) Robotic surgery: a current perspective. Ann Surg 239: 14-21. doi: 10.1097/01.sla.0000103020.19595.7d
![]() |
[9] |
Shimizu S, Kuroda H, Mochizuki T, et al. (2020) Ergonomics-based positioning of the operating handle of surgical microscopes. Neurol Med-Chir 60: 313-316. doi: 10.2176/nmc.rc.2020-0018
![]() |
[10] | Van Bavel J (2013) The world population explosion: causes, backgrounds and pro-jections for the future. Facts Views Vision Obgyn 5: 281-291. |
[11] |
Vaupel JW (2010) Biodemography of human ageing. Nature 464: 536-542. doi: 10.1038/nature08984
![]() |
[12] |
You D, Hug L, Ejdemyr S, et al. (2015) Global, regional, and national levels and trends in under-5 mortality between 1990 and 2015, with scenario-based projections to 2030: a systematic analysis by the UN Inter-agency Group for Child Mortality Estimation. Lancet 386: 2275-2286. doi: 10.1016/S0140-6736(15)00120-8
![]() |
[13] |
Aluttis C, Bishaw T, Frank MW (2014) The workforce for health in a globalized context-global shortages and international migration. Global Health Action 7: 23611-23611. doi: 10.3402/gha.v7.23611
![]() |
[14] |
Senders JT, Arnaout O, Karhade AV, et al. (2018) Natural and artificial intelligence in neurosurgery: a systematic review. Neurosurgery 83: 181-192. doi: 10.1093/neuros/nyx384
![]() |
[15] |
Sullivan R, Alatise OI, Anderson BO, et al. (2015) Global cancer surgery: delivering safe, affordable, and timely cancer surgery. Lancet Oncol 16: 1193-1224. doi: 10.1016/S1470-2045(15)00223-5
![]() |
[16] |
Michael CD, Abbas R, Graham F, et al. (2019) Global neurosurgery: the current capacity and deficit in the provision of essential neurosurgical care. Executive summary of the global neurosurgery initiative at the program in global surgery and social change. J Neurosurg 130: 1055-1064. doi: 10.3171/2017.11.JNS171500
![]() |
[17] |
Swagoto M, Maria P, Abbas R, et al. (2019) The global neurosurgical workforce: a mixed-methods assessment of density and growth. J Neurosurg 130: 1142-1148. doi: 10.3171/2018.10.JNS171723
![]() |
[18] |
Kato Y, Liew BS, Sufianov AA, et al. (2020) Review of global neurosurgery education: horizon of neurosurgery in the developing countries. Chin Neurosurg J 6: 19. doi: 10.1186/s41016-020-00194-1
![]() |
[19] |
Solomou G, Murphy S, Bandyopadhyay S, et al. (2020) Neurosurgery specialty training in the UK: What you need to know to be shortlisted for an interview. Ann Med Surg 57: 287-290. doi: 10.1016/j.amsu.2020.07.047
![]() |
[20] |
Mooney MA, Yoon S, Cole T, et al. (2019) Cost transparency in neurosurgery: a single-institution analysis of patient out-of-pocket spending in 13673 consecutive neurosurgery cases. Neurosurgery 84: 1280-1289. doi: 10.1093/neuros/nyy185
![]() |
[21] |
Yoon JS, Tang OY, Lawton MT (2019) Volume–cost relationship in neurosurgery: analysis of 12,129,029 admissions from the national inpatient sample. World Neurosurg 129: e791-e802. doi: 10.1016/j.wneu.2019.06.034
![]() |
[22] |
Obermeyer Z, Emanuel EJ (2016) Predicting the future–Big data, Machine learning, and Clinical medicine. N Engl J Med 375: 1216-1219. doi: 10.1056/NEJMp1606181
![]() |
[23] | Cruz JA, Wishart DS (2007) Applications of machine learning in cancer prediction and prognosis. Cancer Inform 2: 59-77. |
[24] |
Marcus HJ, Williams S, Hughes-Hallett A, et al. (2017) Predicting surgical outcome in patients with glioblastoma multiforme using pre-operative magnetic resonance imaging: development and preliminary validation of a grading system. Neurosurg Rev 40: 621-631. doi: 10.1007/s10143-017-0817-0
![]() |
[25] |
Rudie JD, Rauschecker AM, Bryan RN, et al. (2019) Emerging applications of artificial intelligence in neuro-oncology. Radiology 290: 607-618. doi: 10.1148/radiol.2018181928
![]() |
[26] |
Deo RC (2015) Machine learning in medicine. Circulation 132: 1920-1930. doi: 10.1161/CIRCULATIONAHA.115.001593
![]() |
[27] |
Lane T (2018) A short history of robotic surgery. Ann R Coll Surge Engl 100: 5-7. doi: 10.1308/rcsann.supp1.5
![]() |
[28] |
Sheetz KH, Claflin J, Dimick JB (2020) Trends in the adoption of robotic surgery for common surgical procedures. JAMA Network Open 3: e1918911-e1918911. doi: 10.1001/jamanetworkopen.2019.18911
![]() |
[29] |
Topol EJ (2019) High-performance medicine: the convergence of human and artificial intelligence. Nat Med 25: 44-56. doi: 10.1038/s41591-018-0300-7
![]() |
[30] |
Jordan MI, Mitchell TM (2015) Machine learning: Trends, perspectives, and prospects. Science 349: 255. doi: 10.1126/science.aaa8415
![]() |
[31] |
Senders JT, Staples PC, Karhade AV, et al. (2018) Machine learning and neurosurgical outcome prediction: a systematic review. World Neurosurg 109: 476-486. doi: 10.1016/j.wneu.2017.09.149
![]() |
[32] |
LeCun Y, Bengio Y, Hinton G (2015) Deep learning. Nature 521: 436-444. doi: 10.1038/nature14539
![]() |
[33] |
Alvin MD, Lubelski D, Alam R, et al. (2018) Spine surgeon treatment variability: the impact on costs. Global Spine J 8: 498-506. doi: 10.1177/2192568217739610
![]() |
[34] |
Daniels AH, Ames CP, Smith JS, et al. (2014) Variability in spine surgery procedures performed during orthopaedic and neurological surgery residency training: an analysis of ACGME case log data. J Bone Joint Surg Am 96: e196. doi: 10.2106/JBJS.M.01562
![]() |
[35] |
Deyo RA, Mirza SK (2006) Trends and variations in the use of spine surgery. Clin Orthop Relat Res 443: 139-146. doi: 10.1097/01.blo.0000198726.62514.75
![]() |
[36] |
Mroz TE, Lubelski D, Williams SK, et al. (2014) Differences in the surgical treatment of recurrent lumbar disc herniation among spine surgeons in the United States. Spine J 14: 2334-2343. doi: 10.1016/j.spinee.2014.01.037
![]() |
[37] |
Rasouli JJ, Shao J, Neifert S, et al. (2020) Artificial intelligence and robotics in spine surgery. Global Spine J 11: 556-564. doi: 10.1177/2192568220915718
![]() |
[38] |
Arvind V, Kim JS, Oermann EK, et al. (2018) Predicting surgical complications in adult patients undergoing anterior cervical discectomy and fusion using nachine learning. Neurospine 15: 329-337. doi: 10.14245/ns.1836248.124
![]() |
[39] |
Galbusera F, Casaroli G, Bassani T (2019) Artificial intelligence and machine learning in spine research. JOR Spine 2: e1044. doi: 10.1002/jsp2.1044
![]() |
[40] |
Kim JS, Merrill RK, Arvind V, et al. (2018) Examining the ability of artificial neural networks machine learning models to accurately predict complications following posterior lumbar spine fusion. Spine 43: 853-860. doi: 10.1097/BRS.0000000000002442
![]() |
[41] |
Emblem KE, Nedregaard B, Hald JK, et al. (2009) Automatic glioma characterization from dynamic susceptibility contrast imaging: brain tumor segmentation using knowledge-based fuzzy clustering. J Magne Reson Imaging 30: 1-10. doi: 10.1002/jmri.21815
![]() |
[42] |
Emblem KE, Pinho MC, Zöllner FG, et al. (2014) A generic support vector machine model for preoperative glioma survival associations. Radiology 275: 228-234. doi: 10.1148/radiol.14140770
![]() |
[43] |
Brady AP (2017) Error and discrepancy in radiology: inevitable or avoidable? Insights Imaging 8: 171-182. doi: 10.1007/s13244-016-0534-1
![]() |
[44] |
Siarkowski M, Lin K, Li SS, et al. (2020) Meta-analysis of interventions to reduce door to needle times in acute ischaemic stroke patients. BMJ Open Qual 9: e000915. doi: 10.1136/bmjoq-2020-000915
![]() |
[45] |
Mun SK, Wong KH, Lo S-CB, et al. (2021) Artificial Intelligence for the Future Radiology Diagnostic Service. Front Mol Biosci 7: 614258. doi: 10.3389/fmolb.2020.614258
![]() |
[46] |
Furlan Anthony J (2006) Time is brain. Stroke 37: 2863-2864. doi: 10.1161/01.STR.0000251852.07152.63
![]() |
[47] |
Fonarow Gregg C, Smith Eric E, Saver Jeffrey L, et al. (2011) Improving door-to-needle times in acute ischemic stroke. Stroke 42: 2983-2989. doi: 10.1161/STROKEAHA.111.621342
![]() |
[48] |
Man S, Xian Y, Holmes DN, et al. (2020) Association between thrombolytic door-to-needle time and 1-year mortality and readmission in patients with acute ischemic stroke. JAMA 323: 2170-2184. doi: 10.1001/jama.2020.5697
![]() |
[49] |
Nagaratnam K, Harston G, Flossmann E, et al. (2020) Innovative use of artificial intelligence and digital communication in acute stroke pathway in response to COVID-19. Future Healthcare J 7: 169-173. doi: 10.7861/fhj.2020-0034
![]() |
[50] |
Yamashita K, Yoshiura T, Arimura H, et al. (2008) Performance evaluation of radiologists with artificial neural network for differential diagnosis of intra-axial cerebral tumors on MR images. Am J Neuroradiol 29: 1153-1158. doi: 10.3174/ajnr.A1037
![]() |
[51] |
Kassahun Y, Perrone R, De Momi E, et al. (2014) Automatic classification of epilepsy types using ontology-based and genetics-based machine learning. Artif Intell Med 61: 79-88. doi: 10.1016/j.artmed.2014.03.001
![]() |
[52] |
Bidiwala S, Pittman T (2004) Neural network classification of pediatric posterior fossa tumors using clinical and imaging data. Pediatr Neurosurg 40: 8-15. doi: 10.1159/000076571
![]() |
[53] |
Zhang B, Chang K, Ramkissoon S, et al. (2017) Multimodal MRI features predict isocitrate dehydrogenase genotype in high-grade gliomas. Neuro Oncol 19: 109-117. doi: 10.1093/neuonc/now121
![]() |
[54] |
Titano JJ, Badgeley M, Schefflein J, et al. (2018) Automated deep-neural-network surveillance of cranial images for acute neurologic events. Nat Med 24: 1337-1341. doi: 10.1038/s41591-018-0147-y
![]() |
[55] |
Ueda D, Yamamoto A, Nishimori M, et al. (2018) Deep Learning for MR Angiography: Automated Detection of Cerebral Aneurysms. Radiology 290: 187-194. doi: 10.1148/radiol.2018180901
![]() |
[56] |
Dolz J, Betrouni N, Quidet M, et al. (2016) Stacking denoising auto-encoders in a deep network to segment the brainstem on MRI in brain cancer patients: A clinical study. Comput Med Imag Grap 52: 8-18. doi: 10.1016/j.compmedimag.2016.03.003
![]() |
[57] |
Cohen KB, Glass B, Greiner HM, et al. (2016) Methodological issues in predicting pediatric epilepsy surgery candidates through natural language processing and machine learning. Biomed Inform Insights 8: 11-18. doi: 10.4137/BII.S38308
![]() |
[58] |
Dumont TM, Rughani AI, Tranmer BI (2011) Prediction of symptomatic cerebral vasospasm after aneurysmal subarachnoid hemorrhage with an artificial neural network: feasibility and comparison with logistic regression models. World Neurosurgery 75: 57-63. doi: 10.1016/j.wneu.2010.07.007
![]() |
[59] |
Nielsen A, Hansen Mikkel B, Tietze A, et al. (2018) Prediction of tissue outcome and assessment of treatment effect in acute ischemic stroke using deep learning. Stroke 49: 1394-1401. doi: 10.1161/STROKEAHA.117.019740
![]() |
[60] |
Lüders H, Acharya J, Baumgartner C, et al. (1998) Semiological seizure classification. Epilepsia 39: 1006-1013. doi: 10.1111/j.1528-1157.1998.tb01452.x
![]() |
[61] |
Emblem KE, Nedregaard B, Nome T, et al. (2008) Glioma grading by using histogram analysis of blood volume heterogeneity from MR-derived cerebral blood volume maps. Radiology 247: 808-817. doi: 10.1148/radiol.2473070571
![]() |
[62] | Lev MH, Ozsunar Y, Henson JW, et al. (2004) Glial tumor grading and outcome prediction using dynamic spin-echo MR susceptibility mapping compared with conventional contrast-enhanced MR: confounding effect of elevated rCBV of oligodendroglimoas. Am J Neuroradiol 25: 214-221. |
[63] |
Marcus AP, Marcus HJ, Camp SJ, et al. (2020) Improved prediction of surgical resectability in patients with glioblastoma using an artificial neural network. Sci Rep 10: 5143. doi: 10.1038/s41598-020-62160-2
![]() |
[64] |
Young R, Babb J, Law M, et al. (2007) Comparison of region-of-interest analysis with three different histogram analysis methods in the determination of perfusion metrics in patients with brain gliomas. J Magn Reson Imaging 26: 1053-1063. doi: 10.1002/jmri.21064
![]() |
[65] |
Duffau H, Capelle L (2004) Preferential brain locations of low-grade gliomas. Cancer 100: 2622-2626. doi: 10.1002/cncr.20297
![]() |
[66] |
Clarke LP, Velthuizen RP, Clark M, et al. (1998) MRI measurement of brain tumor response: comparison of visual metric and automatic segmentation. Magn Reson Imaging 16: 271-279. doi: 10.1016/S0730-725X(97)00302-0
![]() |
[67] |
Pfirrmann CWA, Metzdorf A, Zanetti M, et al. (2001) Magnetic resonance classification of lumbar intervertebral disc degeneration. Spine 26: 1873-1878. doi: 10.1097/00007632-200109010-00011
![]() |
[68] |
Toyoda H, Takahashi S, Hoshino M, et al. (2017) Characterizing the course of back pain after osteoporotic vertebral fracture: a hierarchical cluster analysis of a prospective cohort study. Arch Osteoporos 12: 82. doi: 10.1007/s11657-017-0377-5
![]() |
[69] |
Jeffrey EA, Craig M, Zhiyue JW, et al. (1997) Prediction of posterior fossa tumor type in children by means of magnetic resonance image properties, spectroscopy, and neural networks. J Neurosurg 86: 755-761. doi: 10.3171/jns.1997.86.5.0755
![]() |
[70] |
Kitajima M, Hirai T, Katsuragawa S, et al. (2009) Differentiation of common large sellar-suprasellar masses: effect of artificial neural network on radiologists' diagnosis performance. Acad Radiol 16: 313-320. doi: 10.1016/j.acra.2008.09.015
![]() |
[71] |
Christy PS, Tervonen O, Scheithauer BW, et al. (1995) Use of a neural network and a multiple regression model to predict histologic grade of astrocytoma from MRI appearances. Neuroradiology 37: 89-93. doi: 10.1007/BF00588619
![]() |
[72] |
Juntu J, Sijbers J, De Backer S, et al. (2010) Machine learning study of several classifiers trained with texture analysis features to differentiate benign from malignant soft-tissue tumors in T1-MRI images. J Magn Reson Imaging 31: 680-689. doi: 10.1002/jmri.22095
![]() |
[73] |
Zhao Z-X, Lan K, Xiao JH, et al. (2010) A new method to classify pathologic grades of astrocytomas based on magnetic resonance imaging appearances. Neurology India 58: 685-690. doi: 10.4103/0028-3886.72161
![]() |
[74] |
Sinha M, Kennedy CS, Ramundo, et al. (2001) Artificial neural network predicts CT scan abnormalities in pediatric patients with closed head injury. J Trauma 50: 308-312. doi: 10.1097/00005373-200102000-00018
![]() |
[75] |
Chiang S, Levin HS, Haneef Z (2015) Computer-automated focus lateralization of temporal lobe epilepsy using fMRI. J Magn Reson Imaging 41: 1689-1694. doi: 10.1002/jmri.24696
![]() |
[76] |
Berg AT, Vickrey BG, Langfitt JT, et al. (2003) The multicenter study of epilepsy surgery: recruitment and selection for surgery. Epilepsia 44: 1425-1433. doi: 10.1046/j.1528-1157.2003.24203.x
![]() |
[77] |
Tankus A, Yeshurun Y, Fried I (2009) An automatic measure for classifying clusters of suspected spikes into single cells versus multiunits. J Neural Eng 6: 056001. doi: 10.1088/1741-2560/6/5/056001
![]() |
[78] |
Anand IR, Travis MD, Zhenyu L, et al. (2010) Use of an artificial neural network to predict head injury outcome. J Neurosurg 113: 585-590. doi: 10.3171/2009.11.JNS09857
![]() |
[79] |
Chang K, Bai HX, Zhou H, et al. (2018) Residual convolutional neural network for the determination of IDH status in low- and high-grade gliomas from MR Imaging. Clin Cancer Res 24: 1073-1081. doi: 10.1158/1078-0432.CCR-17-2236
![]() |
[80] |
Yu J, Shi Z, Lian Y, et al. (2017) Noninvasive IDH1 mutation estimation based on a quantitative radiomics approach for grade II glioma. Eur Radiol 27: 3509-3522. doi: 10.1007/s00330-016-4653-3
![]() |
[81] |
Hollon TC, Pandian B, Adapa AR, et al. (2020) Near real-time intraoperative brain tumor diagnosis using stimulated raman histology and deep neural networks. Nat Med 26: 52-58. doi: 10.1038/s41591-019-0715-9
![]() |
[82] |
Gal AA, Cagle PT (2005) The 100-year anniversary of the description of the frozen section procedure. JAMA 294: 3135-3137. doi: 10.1001/jama.294.24.3135
![]() |
[83] | Novis D, Zarbo R (1997) Interinstitutional comparison of frozen section turnaround time. A college of American Pathologists Q-Probes study of 32868 frozen sections in 700 hospitals. Arch Pathol Lab Med 121: 559-567. |
[84] |
Mosa ASM, Yoo I, Sheets L (2012) A systematic review of healthcare applications for smartphones. BMC Med Inform Decis Mak 12: 67. doi: 10.1186/1472-6947-12-67
![]() |
[85] | Bardram JE, Bossen C (2005) Mobility Work: The spatial dimension of collaboration at a hospital. CSCW 14: 131-160. |
[86] |
Hector EJ (2016) Pediatric neurosurgery telemedicine clinics: a model to provide care to geographically underserved areas of the United States and its territories. J Neurosurg Pediatr 18: 753-757. doi: 10.3171/2016.6.PEDS16202
![]() |
[87] |
Reider-Demer M, Raja P, Martin N, et al. (2018) Prospective and retrospective study of videoconference telemedicine follow-up after elective neurosurgery: results of a pilot program. Neurosurg Rev 41: 497-501. doi: 10.1007/s10143-017-0878-0
![]() |
[88] | Susan RS (2018) Editorial. Telemedicine for elective neurosurgical routine follow-up care: a promising patient-centered and cost-effective alternative to in-person clinic visits. Neurosurg Focus 44: E18. |
[89] |
Semple JL, Armstrong KA (2017) Mobile applications for postoperative monitoring after discharge. CMAJ 189: E22-E24. doi: 10.1503/cmaj.160195
![]() |
[90] |
Layard Horsfall H, Palmisciano P, Khan DZ, et al. (2021) Attitudes of the surgical team toward artificial intelligence in neurosurgery: international 2-stage cross-sectional survey. World Neurosurg 146: e724-e730. doi: 10.1016/j.wneu.2020.10.171
![]() |
[91] |
Tsermoulas G, Zisakis A, Flint G, et al. (2020) Challenges to neurosurgery during the coronavirus disease 2019 (COVID-19) pandemic. World Neurosurg 139: 519-525. doi: 10.1016/j.wneu.2020.05.108
![]() |
[92] |
Zemmar A, Lozano AM, Nelson BJ (2020) The rise of robots in surgical environments during COVID-19. Nat Mach Intell 2: 566-572. doi: 10.1038/s42256-020-00238-2
![]() |
[93] | Michael CD, Ronnie EB, Abbas R, et al. (2018) Pediatric neurosurgical workforce, access to care, equipment and training needs worldwide. Neurosurg Focus 45: E13. |
[94] |
Mofatteh M (2021) Risk factors associated with stress, anxiety, and depression among university undergraduate students. AIMS Public Health 8: 36-65. doi: 10.3934/publichealth.2021004
![]() |
[95] |
Stein SC (2018) Cost-effectiveness research in neurosurgery: we can and we must. Neurosurgery 83: 871-878. doi: 10.1093/neuros/nyx583
![]() |
[96] |
Sejnowski TJ (2020) The unreasonable effectiveness of deep learning in artificial intelligence. Proc Natl Acad Sci 117: 30033-30038. doi: 10.1073/pnas.1907373117
![]() |
[97] |
Bell C, Shenoy P, Chalodhorn R, et al. (2008) Control of a humanoid robot by a noninvasive brain-computer interface in humans. J Neural Eng 5: 214-220. doi: 10.1088/1741-2560/5/2/012
![]() |
[98] |
Zhang X, Ma Z, Zheng H, et al. (2020) The combination of brain-computer interfaces and artificial intelligence: applications and challenges. Ann Transl Med 8: 712. doi: 10.21037/atm.2019.11.109
![]() |
[99] | National Spinal Cord Injury Statistical Center (2021) Facts and Figures at a Glance Birmingham, AL: University of Alabama at Birmingham. |
[100] |
Li M, Cui Y, Hao D, et al. (2015) An adaptive feature extraction method in BCI-based rehabilitation. J Intell Fuzzy Syst 28: 525-535. doi: 10.3233/IFS-141329
![]() |
[101] |
Bouton CE, Shaikhouni A, Annetta NV, et al. (2016) Restoring cortical control of functional movement in a human with quadriplegia. Nature 533: 247-250. doi: 10.1038/nature17435
![]() |
[102] |
Flesher SN, Downey JE, Weiss JM, et al. (2021) A brain-computer interface that evokes tactile sensations improves robotic arm control. Science 372: 831. doi: 10.1126/science.abd0380
![]() |
[103] |
Bauer R, Gharabaghi A (2015) Reinforcement learning for adaptive threshold control of restorative brain-computer interfaces: a Bayesian simulation. Front Neurosci 9: 36. doi: 10.3389/fnins.2015.00036
![]() |
[104] |
Palmisciano P, Jamjoom AAB, Taylor D, et al. (2020) Attitudes of patients and their relatives toward artificial intelligence in neurosurgery. World Neurosurg 138: e627-e633. doi: 10.1016/j.wneu.2020.03.029
![]() |
[105] |
Leite M, Leal A, Figueiredo P (2013) Transfer function between EEG and BOLD signals of epileptic activity. Front Neurology 4: 1. doi: 10.3389/fneur.2013.00001
![]() |
[106] |
Abdolmaleki P, Mihara F, Masuda K, et al. (1997) Neural networks analysis of astrocytic gliomas from MRI appearances. Cancer Lett 118: 69-78. doi: 10.1016/S0304-3835(97)00233-4
![]() |
[107] |
Chari A, Budhdeo S, Sparks R, et al. (2021) Brain-machine interfaces: The role of the neurosurgeon. World Neurosurg 146: 140-147. doi: 10.1016/j.wneu.2020.11.028
![]() |
[108] |
Bonaci T, Calo R, Chizeck HJ (2015) App stores for the brain: privacy and security in brain-computer interfaces. IEEE Technol Soc Mag 34: 32-39. doi: 10.1109/MTS.2015.2425551
![]() |
[109] | Collins JW, Marcus HJ, Ghazi A, et al. (2021) Ethical implications of AI in robotic surgical training: A Delphi consensus statement. Eur Urol Focus . |
[110] |
Groiss SJ, Wojtecki L, Südmeyer M, et al. (2009) Deep brain stimulation in Parkinson's disease. Ther Adv Neurol Disord 2: 20-28. doi: 10.1177/1756285609339382
![]() |
[111] |
Mofatteh M (2020) mRNA localization and local translation in neurons. AIMS Neurosci 7: 299-310. doi: 10.3934/Neuroscience.2020016
![]() |
[112] | Mofatteh M (2021) Neurodegeneration and axonal mRNA transportation. Am J Neurodegener Dis 10: 1-12. |
[113] |
Pinto dos Santos D, Giese D, Brodehl S, et al. (2019) Medical students' attitude towards artificial intelligence: a multicentre survey. Eur Radiol 29: 1640-1646. doi: 10.1007/s00330-018-5601-1
![]() |
1. | Akankshya Priyadarshini, Mihir Kumar Sutar, Sarojrani Pattnaik, Investigation of mechanical properties on functionally graded material reinforced with graphene nanoplatelets for biomedical applications, 2024, 0927-6440, 1, 10.1080/09276440.2024.2406164 | |
2. | T. S. Mohan Kumar, Sharnappa Joladarashi, S. M. Kulkarni, Saleemsab Doddamani, Influence of sea sand reinforcement on the static and dynamic properties of functionally graded epoxy composites, 2024, 33, 1026-1265, 1609, 10.1007/s13726-024-01340-7 |
Dir. | Disc. | Eat | Greet | Phone | Photo | Pose | Purch. | |
Pavllo et al. [28] | 26.0 | 29.8 | 24.6 | 27.0 | 25.8 | 29.4 | 29.2 | 26.7 |
Ours | 25.7 | 29.2 | 25.1 | 27.0 | 25.8 | 30.4 | 28.7 | 25.9 |
Sit. | SitD. | Smoke | Wait | WalkD | Walk | WalkT | Avg | |
Pavllo et al. [28] | 31.7 | 34.6 | 27.4 | 27.3 | 27.9 | 21.5 | 21.8 | 27.4 |
Ours | 30.7 | 35.0 | 27.2 | 26.8 | 27.7 | 21.4 | 22.6 | 27.3 |
Dir. | Disc. | Eat | Greet | Phone | Photo | Pose | Purch. | |
Pavllo et al. [28] | 26.0 | 29.8 | 24.6 | 27.0 | 25.8 | 29.4 | 29.2 | 26.7 |
Ours | 25.7 | 29.2 | 25.1 | 27.0 | 25.8 | 30.4 | 28.7 | 25.9 |
Sit. | SitD. | Smoke | Wait | WalkD | Walk | WalkT | Avg | |
Pavllo et al. [28] | 31.7 | 34.6 | 27.4 | 27.3 | 27.9 | 21.5 | 21.8 | 27.4 |
Ours | 30.7 | 35.0 | 27.2 | 26.8 | 27.7 | 21.4 | 22.6 | 27.3 |
Dir. | Disc. | Eat | Greet | Phone | Photo | Pose | Purch. | |
Pavllo et al. [28] | 36.0 | 39.1 | 31.4 | 35.6 | 33.5 | 38.0 | 40.5 | 34.7 |
Ours | 34.5 | 37.4 | 31.7 | 34.8 | 33.5 | 39.1 | 39.2 | 33.2 |
Sit. | SitD. | Smoke | Wait | WalkD | Walk | WalkT | Avg | |
Pavllo et al. [28] | 41.8 | 41.4 | 34.9 | 36.8 | 34.5 | 26.4 | 27.1 | 35.5 |
Ours | 40.1 | 40.9 | 33.9 | 35.4 | 34.3 | 26.6 | 27.7 | 34.8 |
Dir. | Disc. | Eat | Greet | Phone | Photo | Pose | Purch. | |
Pavllo et al. [28] | 1.92 | 1.97 | 1.48 | 2.27 | 1.42 | 1.79 | 1.85 | 2.16 |
Ours | 1.92 | 1.94 | 1.45 | 2.24 | 1.39 | 1.75 | 1.80 | 2.11 |
Sit. | SitD. | Smoke | Wait | WalkD | Walk | WalkT | Avg | |
Pavllo et al. [28] | 1.11 | 1.53 | 1.40 | 1.59 | 2.68 | 2.29 | 1.91 | 1.83 |
Ours | 1.07 | 1.49 | 1.37 | 1.56 | 2.64 | 2.27 | 1.90 | 1.79 |
Dir. | Disc. | Eat | Greet | Phone | Photo | Pose | Purch. | |
Martinez et al. [24] | 51.8 | 56.2 | 58.1 | 59.0 | 69.5 | 78.4 | 55.2 | 58.1 |
Sun et al. [39] | 52.8 | 54.8 | 54.2 | 54.3 | 61.8 | 67.2 | 53.1 | 53.6 |
Fang et al. [25] | 50.1 | 54.3 | 57.0 | 57.1 | 66.6 | 73.3 | 53.4 | 55.7 |
Pavlakos et al. [40] | 48.5 | 54.4 | 54.4 | 52.0 | 59.4 | 65.3 | 49.9 | 52.9 |
Yang et al. [41] | 51.5 | 58.9 | 50.4 | 57.0 | 62.1 | 65.4 | 49.8 | 52.7 |
Luvizon et al. [42] | 49.2 | 51.6 | 47.6 | 50.5 | 51.8 | 60.3 | 48.5 | 51.7 |
Hossain and Little [26] | 48.4 | 50.7 | 57.2 | 55.2 | 63.1 | 72.6 | 53.0 | 51.7 |
Lee et al. [43] | 40.2 | 49.2 | 47.8 | 52.6 | 50.1 | 75.0 | 50.2 | 43.0 |
Pavllo et al. [28] | 45.2 | 46.7 | 43.3 | 45.6 | 48.1 | 55.1 | 44.6 | 44.3 |
Liu et al. [14] | 46.3 | 52.2 | 47.3 | 50.7 | 55.5 | 67.1 | 49.2 | 46.0 |
Xu and Takano [15] | 45.2 | 49.9 | 47.5 | 50.9 | 54.9 | 66.1 | 48.5 | 46.3 |
Ours (U) | 44.6 | 46.5 | 43.0 | 45.4 | 48.4 | 57.3 | 43.9 | 43.7 |
Ours (U+L) | 44.3 | 46.1 | 42.5 | 45.2 | 48.4 | 56.0 | 43.9 | 43.5 |
Sit. | SitD. | Smoke | Wait | WalkD. | Walk | WalkT | Avg. | |
Martinez et al. [24] | 74.0 | 94.6 | 62.3 | 59.1 | 65.1 | 49.5 | 52.4 | 62.9 |
Sun et al. [39] | 71.7 | 86.7 | 61.5 | 53.4 | 61.6 | 47.1 | 53.4 | 59.1 |
Fang et al. [25] | 72.8 | 88.6 | 60.3 | 57.7 | 62.7 | 47.5 | 50.6 | 60.4 |
Pavlakos et al. [40] | 65.8 | 71.1 | 56.6 | 52.9 | 60.9 | 44.7 | 47.8 | 56.2 |
Yang et al. [41] | 69.2 | 85.2 | 57.4 | 58.4 | 43.6 | 60.1 | 47.7 | 58.6 |
Luvizon et al. [42] | 61.5 | 70.9 | 53.7 | 48.9 | 57.9 | 44.4 | 48.9 | 53.2 |
Hossain and Little [26] | 66.1 | 80.9 | 59.0 | 57.3 | 62.4 | 46.6 | 49.6 | 58.3 |
Lee et al. [43] | 55.8 | 73.9 | 54.1 | 55.6 | 58.2 | 43.3 | 43.3 | 52.8 |
Pavllo et al. [28] | 57.3 | 65.8 | 47.1 | 44.0 | 49.0 | 32.8 | 33.9 | 46.8 |
Liu et al. [14] | 60.4 | 71.1 | 51.5 | 50.1 | 54.5 | 40.3 | 43.7 | 52.7 |
Xu and Takano [15] | 59.7 | 71.5 | 51.4 | 48.6 | 53.9 | 39.9 | 44.1 | 51.9 |
Ours (U) | 56.6 | 64.3 | 47.0 | 43.9 | 49.2 | 32.7 | 33.7 | 46.7 |
Ours (U+L) | 56.7 | 64.6 | 45.6 | 43.6 | 48.9 | 32.6 | 33.7 | 46.4 |
Training Data | GS | noGS | Outdoor | All (PCK) | All (AUC) | |
Martinez et al. [24] | Human3.6M | 49.8 | 42.5 | 31.2 | 42.5 | 17.0 |
Zhou et al. [44] | Human3.6M+MPII | 75.6 | 71.3 | 80.3 | 75.3 | 38.0 |
Xu and Takano [15] | Human3.6M | 81.5 | 81.7 | 75.2 | 80.1 | 45.8 |
Ours (U) | Human 3.6M | 81.7 | 81.6 | 75.4 | 80.2 | 46.0 |
Ours (U+L) | Human3.6M | 81.6 | 81.7 | 75.0 | 80.1 | 45.8 |
Dir. | Disc. | Eat | Greet | Phone | Photo | Pose | Purch. | |
Pavllo et al. [28] | 26.0 | 29.8 | 24.6 | 27.0 | 25.8 | 29.4 | 29.2 | 26.7 |
Ours | 25.7 | 29.2 | 25.1 | 27.0 | 25.8 | 30.4 | 28.7 | 25.9 |
Sit. | SitD. | Smoke | Wait | WalkD | Walk | WalkT | Avg | |
Pavllo et al. [28] | 31.7 | 34.6 | 27.4 | 27.3 | 27.9 | 21.5 | 21.8 | 27.4 |
Ours | 30.7 | 35.0 | 27.2 | 26.8 | 27.7 | 21.4 | 22.6 | 27.3 |
Dir. | Disc. | Eat | Greet | Phone | Photo | Pose | Purch. | |
Pavllo et al. [28] | 26.0 | 29.8 | 24.6 | 27.0 | 25.8 | 29.4 | 29.2 | 26.7 |
Ours | 25.7 | 29.2 | 25.1 | 27.0 | 25.8 | 30.4 | 28.7 | 25.9 |
Sit. | SitD. | Smoke | Wait | WalkD | Walk | WalkT | Avg | |
Pavllo et al. [28] | 31.7 | 34.6 | 27.4 | 27.3 | 27.9 | 21.5 | 21.8 | 27.4 |
Ours | 30.7 | 35.0 | 27.2 | 26.8 | 27.7 | 21.4 | 22.6 | 27.3 |
Dir. | Disc. | Eat | Greet | Phone | Photo | Pose | Purch. | |
Pavllo et al. [28] | 36.0 | 39.1 | 31.4 | 35.6 | 33.5 | 38.0 | 40.5 | 34.7 |
Ours | 34.5 | 37.4 | 31.7 | 34.8 | 33.5 | 39.1 | 39.2 | 33.2 |
Sit. | SitD. | Smoke | Wait | WalkD | Walk | WalkT | Avg | |
Pavllo et al. [28] | 41.8 | 41.4 | 34.9 | 36.8 | 34.5 | 26.4 | 27.1 | 35.5 |
Ours | 40.1 | 40.9 | 33.9 | 35.4 | 34.3 | 26.6 | 27.7 | 34.8 |
Dir. | Disc. | Eat | Greet | Phone | Photo | Pose | Purch. | |
Pavllo et al. [28] | 1.92 | 1.97 | 1.48 | 2.27 | 1.42 | 1.79 | 1.85 | 2.16 |
Ours | 1.92 | 1.94 | 1.45 | 2.24 | 1.39 | 1.75 | 1.80 | 2.11 |
Sit. | SitD. | Smoke | Wait | WalkD | Walk | WalkT | Avg | |
Pavllo et al. [28] | 1.11 | 1.53 | 1.40 | 1.59 | 2.68 | 2.29 | 1.91 | 1.83 |
Ours | 1.07 | 1.49 | 1.37 | 1.56 | 2.64 | 2.27 | 1.90 | 1.79 |
Dir. | Disc. | Eat | Greet | Phone | Photo | Pose | Purch. | |
Martinez et al. [24] | 51.8 | 56.2 | 58.1 | 59.0 | 69.5 | 78.4 | 55.2 | 58.1 |
Sun et al. [39] | 52.8 | 54.8 | 54.2 | 54.3 | 61.8 | 67.2 | 53.1 | 53.6 |
Fang et al. [25] | 50.1 | 54.3 | 57.0 | 57.1 | 66.6 | 73.3 | 53.4 | 55.7 |
Pavlakos et al. [40] | 48.5 | 54.4 | 54.4 | 52.0 | 59.4 | 65.3 | 49.9 | 52.9 |
Yang et al. [41] | 51.5 | 58.9 | 50.4 | 57.0 | 62.1 | 65.4 | 49.8 | 52.7 |
Luvizon et al. [42] | 49.2 | 51.6 | 47.6 | 50.5 | 51.8 | 60.3 | 48.5 | 51.7 |
Hossain and Little [26] | 48.4 | 50.7 | 57.2 | 55.2 | 63.1 | 72.6 | 53.0 | 51.7 |
Lee et al. [43] | 40.2 | 49.2 | 47.8 | 52.6 | 50.1 | 75.0 | 50.2 | 43.0 |
Pavllo et al. [28] | 45.2 | 46.7 | 43.3 | 45.6 | 48.1 | 55.1 | 44.6 | 44.3 |
Liu et al. [14] | 46.3 | 52.2 | 47.3 | 50.7 | 55.5 | 67.1 | 49.2 | 46.0 |
Xu and Takano [15] | 45.2 | 49.9 | 47.5 | 50.9 | 54.9 | 66.1 | 48.5 | 46.3 |
Ours (U) | 44.6 | 46.5 | 43.0 | 45.4 | 48.4 | 57.3 | 43.9 | 43.7 |
Ours (U+L) | 44.3 | 46.1 | 42.5 | 45.2 | 48.4 | 56.0 | 43.9 | 43.5 |
Sit. | SitD. | Smoke | Wait | WalkD. | Walk | WalkT | Avg. | |
Martinez et al. [24] | 74.0 | 94.6 | 62.3 | 59.1 | 65.1 | 49.5 | 52.4 | 62.9 |
Sun et al. [39] | 71.7 | 86.7 | 61.5 | 53.4 | 61.6 | 47.1 | 53.4 | 59.1 |
Fang et al. [25] | 72.8 | 88.6 | 60.3 | 57.7 | 62.7 | 47.5 | 50.6 | 60.4 |
Pavlakos et al. [40] | 65.8 | 71.1 | 56.6 | 52.9 | 60.9 | 44.7 | 47.8 | 56.2 |
Yang et al. [41] | 69.2 | 85.2 | 57.4 | 58.4 | 43.6 | 60.1 | 47.7 | 58.6 |
Luvizon et al. [42] | 61.5 | 70.9 | 53.7 | 48.9 | 57.9 | 44.4 | 48.9 | 53.2 |
Hossain and Little [26] | 66.1 | 80.9 | 59.0 | 57.3 | 62.4 | 46.6 | 49.6 | 58.3 |
Lee et al. [43] | 55.8 | 73.9 | 54.1 | 55.6 | 58.2 | 43.3 | 43.3 | 52.8 |
Pavllo et al. [28] | 57.3 | 65.8 | 47.1 | 44.0 | 49.0 | 32.8 | 33.9 | 46.8 |
Liu et al. [14] | 60.4 | 71.1 | 51.5 | 50.1 | 54.5 | 40.3 | 43.7 | 52.7 |
Xu and Takano [15] | 59.7 | 71.5 | 51.4 | 48.6 | 53.9 | 39.9 | 44.1 | 51.9 |
Ours (U) | 56.6 | 64.3 | 47.0 | 43.9 | 49.2 | 32.7 | 33.7 | 46.7 |
Ours (U+L) | 56.7 | 64.6 | 45.6 | 43.6 | 48.9 | 32.6 | 33.7 | 46.4 |
Training Data | GS | noGS | Outdoor | All (PCK) | All (AUC) | |
Martinez et al. [24] | Human3.6M | 49.8 | 42.5 | 31.2 | 42.5 | 17.0 |
Zhou et al. [44] | Human3.6M+MPII | 75.6 | 71.3 | 80.3 | 75.3 | 38.0 |
Xu and Takano [15] | Human3.6M | 81.5 | 81.7 | 75.2 | 80.1 | 45.8 |
Ours (U) | Human 3.6M | 81.7 | 81.6 | 75.4 | 80.2 | 46.0 |
Ours (U+L) | Human3.6M | 81.6 | 81.7 | 75.0 | 80.1 | 45.8 |