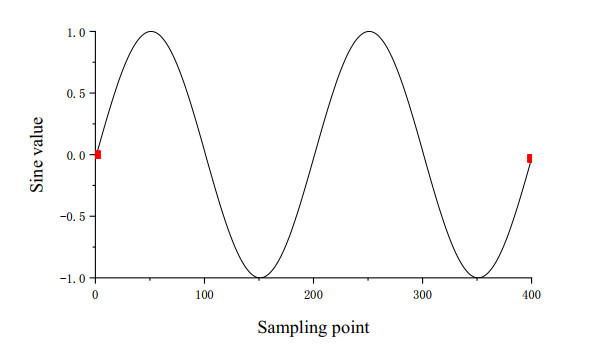
Citation: R. M. Yulmetyev, E. V. Khusaenova, D. G. Yulmetyeva, P. Hänggi, S. Shimojo, K. Watanabe, J. Bhattacharya. Dynamic effects and information quantifiers of statistical memoryof MEG's signals at photosensitive epilepsy[J]. Mathematical Biosciences and Engineering, 2009, 6(1): 189-206. doi: 10.3934/mbe.2009.6.189
[1] | Luis Almeida, Federica Bubba, Benoît Perthame, Camille Pouchol . Energy and implicit discretization of the Fokker-Planck and Keller-Segel type equations. Networks and Heterogeneous Media, 2019, 14(1): 23-41. doi: 10.3934/nhm.2019002 |
[2] | Karoline Disser, Matthias Liero . On gradient structures for Markov chains and the passage to Wasserstein gradient flows. Networks and Heterogeneous Media, 2015, 10(2): 233-253. doi: 10.3934/nhm.2015.10.233 |
[3] | José Antonio Carrillo, Yingping Peng, Aneta Wróblewska-Kamińska . Relative entropy method for the relaxation limit of hydrodynamic models. Networks and Heterogeneous Media, 2020, 15(3): 369-387. doi: 10.3934/nhm.2020023 |
[4] | Panpan Xu, Yongbin Ge, Lin Zhang . High-order finite difference approximation of the Keller-Segel model with additional self- and cross-diffusion terms and a logistic source. Networks and Heterogeneous Media, 2023, 18(4): 1471-1492. doi: 10.3934/nhm.2023065 |
[5] | Beatrice Crippa, Anna Scotti, Andrea Villa . A monotone finite volume scheme for linear drift-diffusion and pure drift equations on one-dimensional graphs. Networks and Heterogeneous Media, 2025, 20(2): 670-700. doi: 10.3934/nhm.2025029 |
[6] | Raul Borsche, Axel Klar, T. N. Ha Pham . Nonlinear flux-limited models for chemotaxis on networks. Networks and Heterogeneous Media, 2017, 12(3): 381-401. doi: 10.3934/nhm.2017017 |
[7] | Kenneth H. Karlsen, Süleyman Ulusoy . On a hyperbolic Keller-Segel system with degenerate nonlinear fractional diffusion. Networks and Heterogeneous Media, 2016, 11(1): 181-201. doi: 10.3934/nhm.2016.11.181 |
[8] | Jin Cui, Yayun Fu . A high-order linearly implicit energy-preserving Partitioned Runge-Kutta scheme for a class of nonlinear dispersive equations. Networks and Heterogeneous Media, 2023, 18(1): 399-411. doi: 10.3934/nhm.2023016 |
[9] | Michael T. Redle, Michael Herty . An asymptotic-preserving scheme for isentropic flow in pipe networks. Networks and Heterogeneous Media, 2025, 20(1): 254-285. doi: 10.3934/nhm.2025013 |
[10] | Tingting Ma, Yayun Fu, Yuehua He, Wenjie Yang . A linearly implicit energy-preserving exponential time differencing scheme for the fractional nonlinear Schrödinger equation. Networks and Heterogeneous Media, 2023, 18(3): 1105-1117. doi: 10.3934/nhm.2023048 |
With the development of automation, electromechanical systems play an important role in modern industry [1,2]. Steering gear and rolling bearing are the core components of the mechanical and electrical systems. Once there is a fault, it might cause economic costs and even serious accidents [3,4,5]. Hence, a practical fault diagnosis scheme is a key to improve the reliability and safety. During the past several decades, Mechanical Failure Prevention Group (MFPG) and Mechanical Health Monitor Center (MHMC) have been established for fault diagnosis and prediction. Simultaneously, the mechanism of fault diagnosis is also investigated [6,7]. However, the operation of electromechanical system is a dynamic process, and the existing fault detection methods are mostly static. It is difficult to use the vibrational data directly for the rolling bearing fault diagnosis.
To solve this problem, it is recommended that the features should be extracted from the vibrational data before the fault diagnosis. Ramachandran et al. [8] proposed a proximal support vector machine (PSVM) for fault diagnosis, which uses decision tree to select the best features. In [9], the SVM, naive Bayes and K-nearest neighbor were combined to extract the envelope spectrum of current as the feature. Nishchal et al. [10] employed unsupervised learning sparse auto-encoder (SAE) to extract fault features, and then the SVM is used to diagnose the shafts and valves. In addition, the effectiveness was verified in comparison with the Mahalanobis distance fast classifier. However, modern electromechanical equipment usually has multi-monitoring points, long monitoring time and high sensor sampling frequency, which can collect massive data [11,12]. These factors intensify the difficulty of fault diagnosis. Specifically, the previous feature extraction methods based on expert knowledge are no longer suitable. Regarding this new scenario, a practical method, which can extract features automatically from massive data, is urgently needed.
In the past decade, the deep learning (DL), which has strong feature extraction ability, is developing rapidly [13]. The DL can extract the features from the original signal, which will greatly enhance the accuracy of fault diagnosis and prediction [14]. Ahmed et al. [15] introduced the artificial neural network into expert system, and the fault diagnosis accuracy can be improved. In order to optimize the fault diagnosis performance of sparse automatic encoder, Jayaswal et al. [16] utilized sparse auto-encoder depth network and backpropagation algorithm in the fine-tuning stage (the second stage), which can achieve high classification accuracy even from highly compressed measurements. In [17], a health monitoring method based on convolution network is used for fault diagnosis of bearings, wherein the data is transformed from the time domain to the frequency domain and then the data are fed into a convolution network to carry out training. By doing so, the accuracy can be increased by 6%. Kumbhar et al. [18] combined the adaptive neural fuzzy inference system (ANFIS) and dimensional analysis to conduct the fault diagnosis under different working conditions. Ajagekar et al. [19] applied quantum computing based DL to fault diagnosis, which is suitable for big data problem. In [20], a three-channel data set was established by integrating time/frequency/time-frequency domain information and a new transfer learning (TL) model was provided. Zhao et al. [21] combined dynamic wavelet weighting coefficients (DWWC) with planetary gearbox diagnosis based on the deep residual network, which can dynamically tune the weights and greatly improve the diagnosis performance. Wu et al. [22] integrated empirical wavelet transform, fuzzy entropy and SVM to diagnose the motor bearing fault. Here, the vibrational signal is decomposed into amplitude and frequency modulation components to calculate the fuzzy entropy, then the faults can be identified by using the SVM. Yu et al. [23] utilized denoising autoencoder and elastic network to denoise the signal, and then the sparse exponential discriminant analysis was used to identify the fault neurons. By doing so, the relevant fault variables on each fault neuron can be separated. A deep enhanced fusion network (DEFN) was proposed for the fault diagnosis in [24], wherein three sparse auto-encoders are applied to extract deep features from three-axial vibrational signals, respectively. By using a feature enhancement mapping, the fused three-axis features are then fed into an echo state network for fault classification. Yang et al. [25] proposed a fault diagnosis method that combines hierarchical symbolic analysis with convolutional neural network (CNN). The initial feature extraction and automatic feature learning are implemented by using a simplified network structure. In summary, the above mentioned methods have good performance in fault diagnosis under "Big Data". In practice, the useful measurements are insufficient. In addition, the CNN has remarkable capability in image recognition, classification, target detection and other areas, however, it will encounter difficulties in dealing with 1-dimensional time series.
Motivated by these previous investigations, the bearing fault diagnosis by using the Gramian angular field (GAF) and the DenseNet is proposed in this paper. The GAF can transform the 1-dimensional time series into a 2-dimensional image, which can maintain the time-dependence. Then, the converted 2-dimensional images can train a DenseNet, which can extract the image features to be used in the fault detection. In order to deal with insufficient samples, the TL is combined with the DenseNet to enhance the accuracy and effectiveness of the training model.
The rest of this paper is organized as follows. Section 2 presents the visualization of time series by using the GAF. The DenseNet based fault diagnosis is provided in Section 3. The data sets are preprocessed in Section 4. Section 5 offers the simulation results. The concluding remarks are given in Section 6.
The GAF, which maintains the time dependency, can transform a 1-dimensional time series into a 2-dimensional image [26]. The polar coordinate is used to represent the time series, and then the sum/difference trigonometric function are calculated. The Gramian angular summation field (GASF) and Gramian angular difference field (GADF) represent the sum and difference of two corners, respectively.
Consider a time series X={x1,x2,⋯,xn}. The normalization can map each element onto [-1, 1] and [0, 1] as
xi=xi−xminxminmax | (1) |
˜xi=(xi−xmin)+(xi−xmax)xmax−xmin | (2) |
where xmin and xmax are the minimum and maximum values of the data sequence, respectively. Time series can be expressed in the polar coordinates by using the angle ϕi and the radius ri, (i=1,2,⋯,n). ϕi preserves the absoluteness of time relations, ri is represented by a timestamp ti, which guarantees the time dependence. They have the forms of
{ϕi=arccos( xi),−1≤ xi≤1ri=tin | (3) |
when the time series is mapped onto [-1, 1], ϕi is mapped onto [0, π]. n is the constant factor of the regularized polar coordinate system, [0, 1] can be divided into n equivalent subintervals. Except the initial point 0, we can obtain n segmentation points, which are associated with X={ x1, x2,⋯, xn}.
Due to the monotonicity of the function, each sequence has a unique polar mapping. The GAF matrixes are constructed by using the sum and difference formulae of
GASF=[cos(φ1+φ1)⋯cos(φ1+φn)cos(φ2+φ1)⋯cos(φ2+φn)⋮⋮⋮cos(φn+φ1)⋯cos(φn+φn)]=˜XT⋅˜X−(√I−˜X2)T⋅√I−˜X2 | (4) |
GADF=[cos(φ1−φ1)⋯cos(φ1−φn)cos(φ2−φ1)⋯cos(φ2−φn)⋮⋮⋮cos(φn−φ1)⋯cos(φn−φn)]=(√I−˜X2)T⋅˜X−˜XT⋅√I−˜X2 | (5) |
where, I=[1,1,⋯,1]. From the above equations, we can see that the matrix elements move from the upper left to the lower right over time, the time dimension is encoded into the geometry of the matrix, and the matrix diagonal elements are single angle values, therefore, the approximate reconstruction of time series can be achieved. However, the expansion of matrix size will increase the computational complexity. To solve this problem, the piecewise aggregation approximation is introduced, which can not only maintain the sequence tendency but also can greatly reduce the sequence size.
Take y=sint as an example, which is a clean signal without noise, wherein t∈[0,4π] and the number of sampling points n=400 (in Figure 1). The time series are converted into 2-dimensional images by using the GAF (in Figure 2).
In order to realize the reconstruction of the original time series and avoid the loss of characteristic information, the GAF is used to preprocess the original signal. It can clearly show the difference of data features, which is helpful for the subsequent image recognition and classification. In fact, other approaches might also be suitable for the conversion from 1-dimensional signals into a 2-dimensional image, we concentrate on the GAF to perform the mission in this paper.
DenseNet is a dense CNN, wherein the layers are pairwise connected. The outputs of each layer are the inputs of subsequent layers to maximize the characteristic information between these two layers. In addition, the gradient disappearance in the training process can also be alleviated. The DenseNet can reuse the features and the CNN architecture is easy to train. Each layer is designed to be very narrow when specifying the network structure, and only a few new features are needed to learn at a time. The 5-layer network of Figure 3 is shown as a classical model that has won the world award [27]. According to Figure 3, each layer maps the features as the characteristic inputs and pass them to other layers. For the bearing vibrational data x0, the output characteristic matrix xl of the l layer module can be expressed as
xl=Hl([x0,x1,⋯,xl−1]) | (6) |
where, x1,⋯,xl−1 are the outputs of each dense layer. Hl is a composite function, which includes batch normalization (BN), ReLU and 3×3 convolution function.
When the feature size is changed, Eq (6) is no longer applicable. The pooling layer, which is included in the transition layer, is needed to reduce the dimensional size of the feature. Assuming that Hl generates additional k features, and k is also the DenseNet growth rate, then the l layer has k0+k(l−1) features, where k0 is the number of input features channels. Therefore, k=4 in Figure 3.
The DenseNet has fewer parameters with small storage requirement and easier network training. Therefore, it is more suitable for feature extraction and fault diagnosis. However, CNN is a data-driven model, which requires a large number of labeled data to obtain satisfactory results, which leads to limitations in some applications.
In this section, the TL is introduced to address the sample dependency of the DenseNet in training models. In combination of CNN and TL, the network framework trained on specific data sets can be applied to new problems and new fields that need to be solved. The TL can generalize the source field knowledge to solve the target field task [28,29,30]. The definition is as follows.
Definition 1. Given the source definition domain Ds, the learning task Ts, the object definition domain Dt and the learning task Tt. The knowledge of Ds and Ts are used to improve the results of object prediction function ft(⋅), which is on Dt, wherein Ds≠Dt and Ts≠Tt.
Remark 1. The domain D={X,P(X)}, the task T={Y,P(Y|X)}, f(⋅)=P(Y|X), therefore Ds≠Dt represents Xs≠Xt or Ps(X)≠Pt(X), the same as T.
In this paper, the source dataset is the CIFAR (Canadian Institute for Advanced Research), and the target dataset is the CWRU (Case Western Reserve University). The combination of TL and CNN implementation process is as follows:
a) the CIFAR dataset is used to train the random initialization parameters;
b) the trained network framework is applied to the specific problem to be solved, and the data features are extracted automatically;
c) the extracted features are input into the CNN to diagnose and classify bearing faults.
The combination of TL and CNN can greatly improve the accuracy of network fault diagnosis, reduce the complexity of network training, avoid the dependence of artificial parameters on expert experience. It can also handle the insufficient samples problem, reducing training time and memory consumption. At the same time, the inherent pattern of the network model is changed, which enhances the network model applicability. Therefore, we can train a general model with insufficient samples in the absence of supervision.
The method is verified on the CWRU bearing data center, which has become the evaluation criterion for rolling bearing fault diagnosis [31,32].
The CWRU bearing experimental platform, which consists of a 2 horsepower (1.5 kw) motor, a torque sensor/decoder and a power tester, is shown in Figure 4 [31]. One motor end is connected to the fan end bearing, and the other end is connected to the drive end bearing. The acceleration sensor, which receives the bearing vibrational signal, is installed on each bearing. An electrical discharge machining (EDM) is used to cause inner/outer ring and rolling element faults and the faults diameters are 0.007, 0.014 and 0.021 inches, respectively. The single point fault of rolling bearing is reloaded to the test motor. The vibrational acceleration signal data, which have 0, 1, 2 and 3 horsepower loads, respectively, are collected by using a 16-channel data recorder. In addition, the sampling frequency is 12 kHz.
The samples include 8 normal samples, 23 inner ring fault samples, 53 outer ring fault samples and 11 rolling element fault samples. In this paper, we use 9 samples, which include three different fault diameters and locations, to train the CNN.
In this paper, the samples of fan end without load, which composes 240, 000 normal data and 120, 000 fault data of each type, are used. The non-overlapping sliding window is selected, and the image size is 300×300. Figure 5 shows the GASF and GADF images by using the same samples.
According to Figure 5, the GADF image is easy to distinguish. Therefore, GADF image can be selected for subsequent fault diagnosis. The fault-free GADF image is numbered 0 (in Figure 6), the GADF images corresponding to the different faults are numbered from 1 to 9 (in Figure 7), respectively.
The fault detection of the bearing is carried out by using the Tensorflow. The simulation platform is configured as follows: 64-bit Windows10 operating system, i7-10700k (CPU), NVIDIA Tesla P100 (GPU), the code is written in python3.7. The compilation software is Spyder and Jupyter Notebook. The Pytorch and Scikit-learn are used to train the CNN.
The analysis steps are as follows:
a) the 1-dimensional time series samples can be transformed into 2-dimensional images by using GAF;
b) the samples are divided, and then the CNN is trained. By changing different batch sizes, the results of different optimization methods can be obtained;
c) the sample datasets are extended by different coincidence rate, and CNN is trained with different batch sizes of samples;
d) the 2/3 coincidence rate of the samples are selected to train the DenseNet121 and ResNet18 networks in combination with the TL.
The GADF images of bearings (Figures 6 and 7), which are divided into 70% training set and 30% test set, are put into the CNN with the structure parameters as shown in Table 1. The initial learning rate is 10−3, the epochs is 5, and the batch sizes are 20, 10 and 2, respectively. Two optimizers, the stochastic gradient descent (SGD) and the Adams, are selected. Table 2 shows the training accuracy results, and the training loss values are shown in Table 3.
Convolution layer | Input (N, C, W, H) | Convolution kernel (size, input, output) | Activation function | Output (N, C, W, H) |
1 | [128,4,300,300] | [5 × 5, 4, 16] | ReLU | [128,16,150,150] |
2 | [128,16,150,150] | [5 × 5, 16, 32] | ReLU | [128,32,75,75] |
3 | [128,32,75,75] | [5 × 5, 32, 64] | ReLU | [128,64,36,36] |
4 | [128,64,36,36] | [5 × 5, 64, 64] | ReLU | [128,64,4,4] |
Batch size | SGD | Adam |
20 | 83.03% | 73.26% |
10 | 83.33% | 72.58% |
2 | 86.44% | 71.46% |
Batch size | SGD | Adam |
20 | 0.5040 | 1.0498 |
10 | 0.5522 | 1.6858 |
2 | 0.5817 | 1.8479 |
According to Tables 2 and 3, the accuracy is not high enough and the training loss value cannot be reduced to a reasonable range. Although all the samples have been used for model training, the results are still not satisfactory. Therefore, the samples need to be expanded to obtain a useful training model.
The repetition rate of the sliding window is set to 2/3, then the normal samples (Figure 8) and different fault samples (Figure 9) can come to 2398 and 9 × 1198, respectively.
In addition, 4795 normal samples and 9 × 2395 different fault samples are obtained by setting 5/6 repetition rate. The CNN with the same structure is used for model training. The training accuracy and loss values for different batch sizes and repetition rates are shown in Tables 4 and 5, respectively.
Batch size | Repetition rate: 2/3 | Repetition rate: 5/6 |
20 | 92.10% | 96.28% |
10 | 91.69% | 94.70% |
2 | 92.65% | 90.34% |
Batch size | Repetition rate: 2/3 | Repetition rate: 5/6 |
20 | 0.3478 | 0.1201 |
10 | 0.4082 | 0.1886 |
2 | 0.3655 | 0.3635 |
According to Tables 4 and 5, the model accuracy can be greatly enhanced and the loss function can also reach within an acceptable range when the number of samples increases. The best accuracy can reach 96.28% through the basic CNN. Also each index has reached at a reasonable range. However, further improvement is needed.
To achieve better results, the ResNet and the DenseNet are trained by using the extended GADF images, wherein the TL is employed. The structure parameters of the two networks are shown in Tables 6 and 7, respectively.
Convolution layer | Parameters | Output |
Conv 1 | 7 × 7, 64, step length = 2 | 112 × 112 |
Conv 2 | [3×3,643×3,64]×2 | 56 × 56 |
Conv 3 | [3×3,1283×3,128]×2 | 28 × 28 |
Conv 4 | [3×3,2563×3,256]×2 | 14 × 14 |
Conv 5 | [3×3,5123×3,512]×2 | 7 × 7 |
Pooling layer | Average pooling | 1 × 1 |
Layer | Parameter | Output Size |
Convolution layer | 7 × 7, conv, stride = 2 | 112 × 112 |
Pooling | 3 × 3 max pooling, stride = 2 | 56 × 56 |
Block1 | [1×13×3]×6 | 56 × 56 |
Transition layer 1 | 1 × 1 conv | 56 × 56 |
2 × 2 average pooling, stride = 2 | 28 × 28 | |
Block 2 | [1×13×3]×12 | 28 × 28 |
Transition layer 2 | 1 × 1 conv | 28 × 28 |
2 × 2 average pooling, stride = 2 | 14 × 14 | |
Block 3 | [1×13×3]×24 | 14 × 14 |
Transition layer 3 | 1 × 1 conv | 14 × 14 |
2 × 2 average pooling, stride = 2 | 7 × 7 | |
Block 4 | [1×13×3]×16 | 7 × 7 |
Taxonomic hierarchies | 7 × 7 global average pooling | 1 × 1 |
A fully connected layer of 1000 dimensions, Softmax |
The results show that the training model accuracy can be as high as 99.69% (ResNet18) and 99.83% (DenseNet121), respectively; and the loss functions are also reduced to 0.013 (ResNet18) and 0.005 (DenseNet121). Compared with the existing basic CNN method, the augmented CNN approach based on expanded samples and the ResNet, the proposed scheme can achieve the best performance with regard to the accuracy and loss values.
In this paper, a bearing fault diagnosis method based on the GAF and the CNN, which could realize the classification diagnosis, was proposed. The GAF could convert time series information into a GADF image, which makes the fault diagnosis to be more intuitive. In addition, the GAF could also ensure the integrity of feature information and handle the time-dependent issue, which is suitable for the processing of non-stationary signals such as bearing vibration signals. The DenseNet was trained by using the GADF images, which could enhance the low classification accuracy caused by measurement noise in the original bearing signal. The TL, which could avoid the inaccurate training model caused by insufficient samples, was applied in the DenseNet. The simulation results demonstrate the effectiveness of the proposed method.
This work was supported by the National Natural Science Foundation of China (grant number 62073177, 61973175, 52175038).
The authors declare there is no conflict of interest.
1. | Luis Almeida, Gissell Estrada-Rodriguez, Lisa Oliver, Diane Peurichard, Alexandre Poulain, Francois Vallette, Treatment-induced shrinking of tumour aggregates: a nonlinear volume-filling chemotactic approach, 2021, 83, 0303-6812, 10.1007/s00285-021-01642-x | |
2. | Giorgia Ciavolella, Effect of a Membrane on Diffusion-Driven Turing Instability, 2022, 178, 0167-8019, 10.1007/s10440-022-00475-0 | |
3. | André Schlichting, Christian Seis, The Scharfetter–Gummel scheme for aggregation–diffusion equations, 2022, 42, 0272-4979, 2361, 10.1093/imanum/drab039 | |
4. | Yangyang Qiao, Steinar Evje, A general cell–fluid Navier–Stokes model with inclusion of chemotaxis, 2020, 30, 0218-2025, 1167, 10.1142/S0218202520400096 | |
5. | Xueling Huang, Jie Shen, Bound/positivity preserving SAV schemes for the Patlak-Keller-Segel-Navier-Stokes system, 2023, 480, 00219991, 112034, 10.1016/j.jcp.2023.112034 | |
6. | Yong Zhang, Yu Zhao, Zhennan Zhou, A Unified Structure Preserving Scheme for a Multispecies Model with a Gradient Flow Structure and Nonlocal Interactions via Singular Kernels, 2021, 43, 1064-8275, B539, 10.1137/20M1348911 | |
7. | Federica Bubba, Camille Pouchol, Nathalie Ferrand, Guillaume Vidal, Luis Almeida, Benoît Perthame, Michèle Sabbah, A chemotaxis-based explanation of spheroid formation in 3D cultures of breast cancer cells, 2019, 479, 00225193, 73, 10.1016/j.jtbi.2019.07.002 | |
8. | Federica Bubba, Benoit Perthame, Daniele Cerroni, Pasquale Ciarletta, Paolo Zunino, A coupled 3D-1D multiscale Keller-Segel model of chemotaxis and its application to cancer invasion, 2022, 15, 1937-1632, 2053, 10.3934/dcdss.2022044 | |
9. | Jingwei Hu, Xiangxiong Zhang, Positivity-preserving and energy-dissipative finite difference schemes for the Fokker–Planck and Keller–Segel equations, 2022, 0272-4979, 10.1093/imanum/drac014 | |
10. | Clément Cancès, Thomas O. Gallouët, Gabriele Todeschi, A variational finite volume scheme for Wasserstein gradient flows, 2020, 146, 0029-599X, 437, 10.1007/s00211-020-01153-9 | |
11. | Federica Bubba, Alexandre Poulain, A nonnegativity preserving scheme for the relaxed Cahn–Hilliard equation with single-well potential and degenerate mobility, 2022, 56, 2822-7840, 1741, 10.1051/m2an/2022050 | |
12. | Jose A. Carrillo, Daniel Matthes, Marie-Therese Wolfram, 2021, 22, 9780444643056, 271, 10.1016/bs.hna.2020.10.002 | |
13. | Clément Cancès, Benoît Gaudeul, A Convergent Entropy Diminishing Finite Volume Scheme for a Cross-Diffusion System, 2020, 58, 0036-1429, 2684, 10.1137/20M1316093 | |
14. | Noemi David, Xinran Ruan, An asymptotic preserving scheme for a tumor growth model of porous medium type, 2022, 56, 2822-7840, 121, 10.1051/m2an/2021080 | |
15. | Clément Cancès, Virginie Ehrlacher, Laurent Monasse, Finite volumes for the Stefan–Maxwell cross-diffusion system, 2024, 44, 0272-4979, 1029, 10.1093/imanum/drad032 | |
16. | José A. Carrillo, Katy Craig, Yao Yao, 2019, Chapter 3, 978-3-030-20296-5, 65, 10.1007/978-3-030-20297-2_3 | |
17. | Rafael Bailo, José A. Carrillo, Jingwei Hu, Bound-Preserving Finite-Volume Schemes for Systems of Continuity Equations with Saturation, 2023, 83, 0036-1399, 1315, 10.1137/22M1488703 | |
18. | Ilka Budde, André Schlichting, David Ing, Sandra Schimmelpfennig, Anna Kuntze, Benedikt Fels, Joelle M.-J. Romac, Sandip M. Swain, Rodger A. Liddle, Angela Stevens, Albrecht Schwab, Zoltán Pethő, Piezo1-induced durotaxis of pancreatic stellate cells depends on TRPC1 and TRPV4 channels, 2025, 138, 0021-9533, 10.1242/jcs.263846 | |
19. | Gissell Estrada-Rodriguez, Diane Peurichard, Xinran Ruan, From a nonlinear kinetic equation to a volume-exclusion chemotaxis model via asymptotic preserving methods, 2025, 0, 1937-5093, 0, 10.3934/krm.2025012 |
Convolution layer | Input (N, C, W, H) | Convolution kernel (size, input, output) | Activation function | Output (N, C, W, H) |
1 | [128,4,300,300] | [5 × 5, 4, 16] | ReLU | [128,16,150,150] |
2 | [128,16,150,150] | [5 × 5, 16, 32] | ReLU | [128,32,75,75] |
3 | [128,32,75,75] | [5 × 5, 32, 64] | ReLU | [128,64,36,36] |
4 | [128,64,36,36] | [5 × 5, 64, 64] | ReLU | [128,64,4,4] |
Batch size | SGD | Adam |
20 | 83.03% | 73.26% |
10 | 83.33% | 72.58% |
2 | 86.44% | 71.46% |
Batch size | SGD | Adam |
20 | 0.5040 | 1.0498 |
10 | 0.5522 | 1.6858 |
2 | 0.5817 | 1.8479 |
Batch size | Repetition rate: 2/3 | Repetition rate: 5/6 |
20 | 92.10% | 96.28% |
10 | 91.69% | 94.70% |
2 | 92.65% | 90.34% |
Batch size | Repetition rate: 2/3 | Repetition rate: 5/6 |
20 | 0.3478 | 0.1201 |
10 | 0.4082 | 0.1886 |
2 | 0.3655 | 0.3635 |
Convolution layer | Parameters | Output |
Conv 1 | 7 × 7, 64, step length = 2 | 112 × 112 |
Conv 2 | [3×3,643×3,64]×2 | 56 × 56 |
Conv 3 | [3×3,1283×3,128]×2 | 28 × 28 |
Conv 4 | [3×3,2563×3,256]×2 | 14 × 14 |
Conv 5 | [3×3,5123×3,512]×2 | 7 × 7 |
Pooling layer | Average pooling | 1 × 1 |
Layer | Parameter | Output Size |
Convolution layer | 7 × 7, conv, stride = 2 | 112 × 112 |
Pooling | 3 × 3 max pooling, stride = 2 | 56 × 56 |
Block1 | [1×13×3]×6 | 56 × 56 |
Transition layer 1 | 1 × 1 conv | 56 × 56 |
2 × 2 average pooling, stride = 2 | 28 × 28 | |
Block 2 | [1×13×3]×12 | 28 × 28 |
Transition layer 2 | 1 × 1 conv | 28 × 28 |
2 × 2 average pooling, stride = 2 | 14 × 14 | |
Block 3 | [1×13×3]×24 | 14 × 14 |
Transition layer 3 | 1 × 1 conv | 14 × 14 |
2 × 2 average pooling, stride = 2 | 7 × 7 | |
Block 4 | [1×13×3]×16 | 7 × 7 |
Taxonomic hierarchies | 7 × 7 global average pooling | 1 × 1 |
A fully connected layer of 1000 dimensions, Softmax |
Convolution layer | Input (N, C, W, H) | Convolution kernel (size, input, output) | Activation function | Output (N, C, W, H) |
1 | [128,4,300,300] | [5 × 5, 4, 16] | ReLU | [128,16,150,150] |
2 | [128,16,150,150] | [5 × 5, 16, 32] | ReLU | [128,32,75,75] |
3 | [128,32,75,75] | [5 × 5, 32, 64] | ReLU | [128,64,36,36] |
4 | [128,64,36,36] | [5 × 5, 64, 64] | ReLU | [128,64,4,4] |
Batch size | SGD | Adam |
20 | 83.03% | 73.26% |
10 | 83.33% | 72.58% |
2 | 86.44% | 71.46% |
Batch size | SGD | Adam |
20 | 0.5040 | 1.0498 |
10 | 0.5522 | 1.6858 |
2 | 0.5817 | 1.8479 |
Batch size | Repetition rate: 2/3 | Repetition rate: 5/6 |
20 | 92.10% | 96.28% |
10 | 91.69% | 94.70% |
2 | 92.65% | 90.34% |
Batch size | Repetition rate: 2/3 | Repetition rate: 5/6 |
20 | 0.3478 | 0.1201 |
10 | 0.4082 | 0.1886 |
2 | 0.3655 | 0.3635 |
Convolution layer | Parameters | Output |
Conv 1 | 7 × 7, 64, step length = 2 | 112 × 112 |
Conv 2 | [3×3,643×3,64]×2 | 56 × 56 |
Conv 3 | [3×3,1283×3,128]×2 | 28 × 28 |
Conv 4 | [3×3,2563×3,256]×2 | 14 × 14 |
Conv 5 | [3×3,5123×3,512]×2 | 7 × 7 |
Pooling layer | Average pooling | 1 × 1 |
Layer | Parameter | Output Size |
Convolution layer | 7 × 7, conv, stride = 2 | 112 × 112 |
Pooling | 3 × 3 max pooling, stride = 2 | 56 × 56 |
Block1 | [1×13×3]×6 | 56 × 56 |
Transition layer 1 | 1 × 1 conv | 56 × 56 |
2 × 2 average pooling, stride = 2 | 28 × 28 | |
Block 2 | [1×13×3]×12 | 28 × 28 |
Transition layer 2 | 1 × 1 conv | 28 × 28 |
2 × 2 average pooling, stride = 2 | 14 × 14 | |
Block 3 | [1×13×3]×24 | 14 × 14 |
Transition layer 3 | 1 × 1 conv | 14 × 14 |
2 × 2 average pooling, stride = 2 | 7 × 7 | |
Block 4 | [1×13×3]×16 | 7 × 7 |
Taxonomic hierarchies | 7 × 7 global average pooling | 1 × 1 |
A fully connected layer of 1000 dimensions, Softmax |