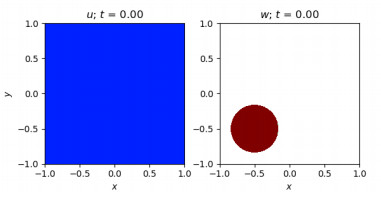
There is an absence of valid and specific psychometric tools to assess TikTok addiction. Considering that the use of TikTok is increasing rapidly and the fact that TikTok addiction may be a different form of social media addiction, there is an urge for a valid tool to measure TikTok addiction.
To develop and validate a tool to measure TikTok addiction.
First, we performed an extensive literature review to create a pool of items to measure TikTok addiction. Then, we employed a panel of experts from different backgrounds to examine the content validity of the initial set of items. We examined face validity by performing cognitive interviews with TikTok users and calculating the item-level face validity index. Our study population included 429 adults who have been TikTok users for at least the last 12 months. We employed exploratory and confirmatory factor analysis to examine the construct validity of the TikTok Addiction Scale (TTAS). We examined the concurrent validity by using the Bergen Social Media Addiction Scale (BSMAS), the Patient Health Questionnaire-4 (PHQ-4), and the Big Five Inventory-10 (BFI-10). We used Cronbach's alpha, McDonald's Omega, Cohen's kappa, and intraclass correlation coefficient to examine reliability.
We found that the TTAS is a six-factor 15-item scale with robust psychometric properties. Factor analysis revealed a six-factor structure, (1) salience, (2) mood modification, (3) tolerance, (4) withdrawal symptoms, (5) conflict, and (6) relapse, which accounted for 80.70% of the total variance. The concurrent validity of the TTAS was excellent since we found significant correlations between TTAS and BSMAS, PHQ-4, and BFI-10. Cronbach's alpha and McDonald's Omega for the TTAS were 0.911 and 0.914, respectively.
The TTAS appears to be a short, easy-to-use, and valid scale to measure TikTok addiction. Considering the limitations of our study, we recommend the translation and validation of the TTAS in other languages and populations to further examine the validity of the scale.
Citation: Petros Galanis, Aglaia Katsiroumpa, Ioannis Moisoglou, Olympia Konstantakopoulou. The TikTok Addiction Scale: Development and validation[J]. AIMS Public Health, 2024, 11(4): 1172-1197. doi: 10.3934/publichealth.2024061
[1] | Gianni Gilioli, Sara Pasquali, Fabrizio Ruggeri . Nonlinear functional response parameter estimation in a stochastic predator-prey model. Mathematical Biosciences and Engineering, 2012, 9(1): 75-96. doi: 10.3934/mbe.2012.9.75 |
[2] | Wenjie Qin, Yue Xia, Yi Yang . An eco-epidemic model for assessing the application of integrated pest management strategies. Mathematical Biosciences and Engineering, 2023, 20(9): 16506-16527. doi: 10.3934/mbe.2023736 |
[3] | Yuan Tian, Sanyi Tang . Dynamics of a density-dependent predator-prey biological system with nonlinear impulsive control. Mathematical Biosciences and Engineering, 2021, 18(6): 7318-7343. doi: 10.3934/mbe.2021362 |
[4] | Xinli Hu, Wenjie Qin, Marco Tosato . Complexity dynamics and simulations in a discrete switching ecosystem induced by an intermittent threshold control strategy. Mathematical Biosciences and Engineering, 2020, 17(3): 2164-2178. doi: 10.3934/mbe.2020115 |
[5] | Yi Yang, Lirong Liu, Changcheng Xiang, Wenjie Qin . Switching dynamics analysis of forest-pest model describing effects of external periodic disturbance. Mathematical Biosciences and Engineering, 2020, 17(4): 4328-4347. doi: 10.3934/mbe.2020239 |
[6] | Yanyun Li, Juhua Liang . Models for determining the optimal switching time in chemical control of pest with pesticide resistance. Mathematical Biosciences and Engineering, 2021, 18(1): 471-494. doi: 10.3934/mbe.2021026 |
[7] | Teklebirhan Abraha, Fahad Al Basir, Legesse Lemecha Obsu, Delfim F. M. Torres . Farming awareness based optimum interventions for crop pest control. Mathematical Biosciences and Engineering, 2021, 18(5): 5364-5391. doi: 10.3934/mbe.2021272 |
[8] | Hao Zhou, Xia Wang, Sanyi Tang . Global dynamics of non-smooth Filippov pest-natural enemy system with constant releasing rate. Mathematical Biosciences and Engineering, 2019, 16(6): 7327-7361. doi: 10.3934/mbe.2019366 |
[9] | Rinaldo M. Colombo, Mauro Garavello . Stability and optimization in structured population models on graphs. Mathematical Biosciences and Engineering, 2015, 12(2): 311-335. doi: 10.3934/mbe.2015.12.311 |
[10] | Liping Wu, Zhongyi Xiang . A study of integrated pest management models with instantaneous and non-instantaneous impulse effects. Mathematical Biosciences and Engineering, 2024, 21(2): 3063-3094. doi: 10.3934/mbe.2024136 |
There is an absence of valid and specific psychometric tools to assess TikTok addiction. Considering that the use of TikTok is increasing rapidly and the fact that TikTok addiction may be a different form of social media addiction, there is an urge for a valid tool to measure TikTok addiction.
To develop and validate a tool to measure TikTok addiction.
First, we performed an extensive literature review to create a pool of items to measure TikTok addiction. Then, we employed a panel of experts from different backgrounds to examine the content validity of the initial set of items. We examined face validity by performing cognitive interviews with TikTok users and calculating the item-level face validity index. Our study population included 429 adults who have been TikTok users for at least the last 12 months. We employed exploratory and confirmatory factor analysis to examine the construct validity of the TikTok Addiction Scale (TTAS). We examined the concurrent validity by using the Bergen Social Media Addiction Scale (BSMAS), the Patient Health Questionnaire-4 (PHQ-4), and the Big Five Inventory-10 (BFI-10). We used Cronbach's alpha, McDonald's Omega, Cohen's kappa, and intraclass correlation coefficient to examine reliability.
We found that the TTAS is a six-factor 15-item scale with robust psychometric properties. Factor analysis revealed a six-factor structure, (1) salience, (2) mood modification, (3) tolerance, (4) withdrawal symptoms, (5) conflict, and (6) relapse, which accounted for 80.70% of the total variance. The concurrent validity of the TTAS was excellent since we found significant correlations between TTAS and BSMAS, PHQ-4, and BFI-10. Cronbach's alpha and McDonald's Omega for the TTAS were 0.911 and 0.914, respectively.
The TTAS appears to be a short, easy-to-use, and valid scale to measure TikTok addiction. Considering the limitations of our study, we recommend the translation and validation of the TTAS in other languages and populations to further examine the validity of the scale.
We develop a model describing the evolution, in time and space, of a predator prey system, where predators can be "used" to eliminate prey. Whenever prey are any sort of harmful pest or parasite and predators constitute a biological counter measure to prey diffusion, the present model provides a prototype for some sort of biological pest control strategy. Within this framework, various possible strategies can be tested and compared. While the analytic framework provides the necessary existence and stability results, it is a biological or economical question to choose which criterion has to be used to prefer a strategy to another.
More precisely, prey are assumed to diffuse all over the available space, while predators move following the prey density gradient towards regions with a higher prey density. In this setting, we introduce a term in the predators' equation able to describe the artificial insertion of predators in the environment.
The mathematical modeling of pest distribution, evolution and control has a long tradition, see for instance the review [1]. Biological pest control, in particular, is receiving a growing attention in the recent literature, see e.g., [2,3,4]. We recall in particular [5], where the same subject is considered, but in the entirely different setting provided by ordinary differential equations. The present approach relies on a thorough use of the techniques of non–local partial differential equations and is amenable to rigorously state and tackle a variety of optimal control problems.
From the analytic point of view, we stress that in the present model the propagation speed of predators is finite. While prey's movement is described through the usual diffusion operator (i.e., the Laplacian), here predators move according to a Hyperbolic Balance Law, which notoriously display finite speed of propagation [6]. Moreover, as it is reasonable from the biological point of view, each predator moves according to the distribution of prey in a region all around it, not only at its exact location, leading to a so called non–local interaction and, hence, non–local equation. Thus, the dynamics of predators essentially depends also on their horizon, i.e., on the distance at which they can "feel" the presence of prey.
As it is natural to expect, and as it is confirmed by the model's equations (2.1), various functions and parameters contribute to the description of the present predator-prey dynamics. Here, we target the role of the control parameter, namely q in (2.1), which represents an external time and space dependent intervention aimed at increasing the predators' population to limit the prey harmful presence.
The variety of strategies that can be adopted is vast and many questions naturally arise: are a limited number of intense global spraying more effective than less intense but periodic treatments? Is it relevant to introduce predators uniformly all over the space domain? Are there effective and, presumably, cheaper strategies where predators are introduced only in suitable carefully selected regions? Present day analytic techniques are, to our knowledge, currently not able to single out the best strategies: the use of numerical integrations is essential and unavoidable.
The next section is devoted to the description of the model and to the rigorous statement of its well posedness and stability properties. Then, in the subsequent section, various numerical integrations allow to compare the results of various pest control strategies.
The pest population is described through its density w=w(t,x) while its antagonist's density is u=u(t,x), where as usual t∈R+ is the time variable and x∈R2 is the space coordinate. The pest population w is assumed
(w.1) to diffuse isotropically, with a movement governed by the usual Laplace operator Δw;
(w.2) to naturally increase through reproduction with a net birth rate γ;
(w.3) to be predated by the u population, so that the w birth rate is reduced by the quantity δu.
On the other hand, the predators in the u population
(u.1) move where the pest density is higher, their velocity v being a non–local function of the prey gradient ∇w;
(u.2) have a birth rate αw proportional to the prey density;
(u.3) suffer a fixed mortality rate β.
In both cases, the latter terms (w.2)–(w.3) and (u.2)–(u.3) reflect the usual Lotka–Volterra interaction, see for instance [7,§ 3.1].
We thus get the following mixed hyperbolic-parabolic system,
{∂tu+div(uv(w))=(αw−β)u+q∂tw−μΔw=(γ−δu)w(t,x)∈R+×R2 | (2.1) |
which extends [8] thanks to the control term q and fits into the more general setting of [9], where the various coefficients are time and space dependent.
We allow the velocity vector field of the predators v=v(w) to depend on ∇w through a space average. Indeed, according to (u.1), it is proportional to the average density gradient ∇w seen by the predators within their horizon, so that, in general,
v(w)=κ∇(w∗η)√1+‖∇(w∗η)‖2 where η∈C3(R2;R+),∫R2η=1sptη⊆B(0,ℓ). | (2.2) |
The role of adimensional function η, chosen as specified above, is to provide an average of the gradient ∇w. Indeed, as soon as w is sufficiently regular, ∇(w∗η)=(∇w)∗η. Hence, v(w) in (2.2) is κ times the average gradient of w, smoothly normalized. Thus, κ is the maximal predators' speed. The constant ℓ is the predators' horizon, i.e., the maximal distance at which predators feel prey.
A key role is played by the quantity q in the first equation: it is this term that is meant to describe an external action aiming at increasing the u population, thus allowing predators to naturally reduce the pest population w. In general, q may depend on space and time, when describing an open–loop control, or also on the w population, resulting in a feedback strategy.
The case of the various coefficients α,β,γ,δ,κ or of v to be explicitly dependent also on time t and on the space coordinate x can also be included in the well posedness and stability results related to (2.1)–(2.2), as well as the case of speed laws more general than (2.2), see [9] for more details.
Remark that the above choice (2.2) of the speed law is grounded on our specific modeling setting. For instance, with the different choice v(w)=∇w, which is quite usual in the different context of chemotaxis, the notion of predators horizon would be lost, as also the fact that the propagation of predators is a priori bounded. The requirement that η is of class C3 is of a merely technical nature.
The next result ensures the well posedness of the model (2.1)–(2.2) and provides the key stability estimates that describe the dependence of the solution on the initial datum (uo,wo) and on the open-loop control q.
We refer to [8,9] for the rigorous description of the analytic framework. Here, we only recall that by solution to (2.1)–(2.2) we mean a pair (u∗,w∗), where u∗ is a weak entropy solution to the balance law ∂tu+div(uv(w∗))=(αw∗−β)u+q, while w∗ is a solution to the parabolic equation ∂tw−μΔw=(γ−δu∗)w.
Theorem 3.1. Fix positive α,β,γ,δ,κ,μ and ℓ. Let v and η be as in (2.2). Then, for any initial datum (uo,wo) and for any control q, with
uo∈(L1∩L∞∩BV)(R2;R+)wo∈(L1∩L∞)(R2;R+)q∈L∞(R+×Rn;R+)∩L∞(R+;L1(Rn;R+))andq(t)∈BV(Rn;R+)for all t∈R+ |
problem (2.1)–(2.2) admits a unique global solution (u∗,w∗) with
u∗∈C0,1(R+;L1(R2;R+))andu∗(t)∈(L∞∩BV)(R2;R+)for all t∈R+,w∗∈C0,1(R+;L1(R2;R+))andw∗(t)∈L∞(R2;R+)for all t∈R+. |
Moreover, with obvious notation, we have the following estimates on the dependence of the solutions (u∗,w∗) and (u′∗,w′∗) on the initial data (uo,wo) and (u′o,w′o):
‖u∗(t)−u′∗(t)‖L1(R2;R)+‖w∗(t)−w′∗(t)‖L1(R2;R)≤ O(t)(‖uo(t)−u′o(t)‖L1(R2;R)+‖wo(t)−w′o(t)‖L1(R2;R)). |
On the other hand, if (u∗,w∗) and (u′∗,w′∗) are the solutions corresponding to the control q and q′,
‖u∗(t)−u′∗(t)‖L1(R2;R)+‖w∗(t)−w′∗(t)‖L1(R2;R)≤O(t)‖q−q′‖L1([0,t]×R2;R). |
The quantity O(t) depends on the parameters in (2.1)–(2.2), on q and on upper bounds on norms of the initial data in L1, L∞ and on the predators' initial total variation; typically, t→O(t) grows exponentially.
We refer for the proof of Theorem 3.1 to [9], where the more general case of time and space dependent coefficients is considered and further stability estimates are provided. In particular, [8,Lemma 4.1] shows that the assumptions above on η yield a non–local operator v in (2.2) meeting the requirements necessary for Theorem 3.1 to hold.
Here, we stress the qualitative information provided by Theorem 3.1. First, it ensures the existence, uniqueness and continuous dependence on the initial data of the evolution prescribed by (2.1)–(2.2). Then, it opens the way to the search for optimal strategies, ensuring the continuous dependence of the solution on the control. Mostly, Theorem 3.1 tells that the relevant norm, that is, the one that allows to consider data or controls as near or not, is the L1 norm. In particular, we do not claim that the solution, in particular w, can not be more regular than merely L1.
For completeness, we also note that the dependence of the L1 difference between solutions corresponding to different controls or initial data resembles that typically appearing in ordinary differential equations. As time increases, this difference may well grow unboundedly. Concerning the dependence of the solution on η, L1 estimates can be found in [8,9], while the case of η approaching a Dirac delta is treated in [10].
The next paragraphs show various evolutions as described by the model (2.1)–(2.2). Our aim is to investigate the different evolutions resulting from different control choices. Therefore, we let only q in (2.1)–(2.2) vary, keeping all other parameters (both physical and numerical) fixed.
We use throughout the Lax–Friedrichs scheme [11,§ 12.5] with dimensional splitting [11,§ 19.5] and a further splitting to take care of the source term [11,§ 17.1]. Further details on the numerical algorithm exploited below can be found in [12], while related numerical studies are in [13,14].
Solutions are displayed on the domain Ω=[−1,1]×[−1,1] and along its boundary ∂Ω we fix u and w to 0, which roughly corresponds to set all parameters in (2.1) and the initial data to 0 outside Ω. The presence of the non–local speed (2.2) imposes to use a numerical domain larger than Ω, that is [−1−ℓ,1+ℓ]×[−1−ℓ,1+ℓ], where ℓ quantifies the distance at which predators "feel" prey, see (2.2).
The integration time is T=12, the space mesh consists of 29×29 squares. Moreover, with reference to the notation in (2.1)–(2.2), we set:
α=0.25β=2γ=10δ=0.25κ=2μ=0.5ℓ=0.1η(x)={4πℓ2(1−‖x‖2ℓ2)3‖x‖≤ℓ,0‖x‖>ℓ. | (4.1) |
The initial datum used in this section is detailed in (4.2), see also Figure 1.
uo(x)=20χΩ(x),∫Ωuo(x)dx=80,wo(x)={50if (x1+12)2+(x2+12)2≤19,0otherwise, ∫Ωwo(x)dx=17.5. | (4.2) |
Remark that the diffusion term in the second equation causes the initial position of the prey to be essentially irrelevant at large times. To evaluate the efficiency of each strategy, we choose the total number of prey, averaged over time between time to=4 and the final time T=12, that is we compute the quantity
¯W=1T−to∫Tto∫Ωw(t,x)dxdt. | (4.3) |
Our dropping the initial interval t∈[0,4] is aimed at reducing the initial oscillations due to the particular initial datum chosen. As it is clear in the examples below, it may well happen that different strategies lead to similar values of the average ¯W, but with very different ranges in the values attained by the total number ∫Ωw of prey at different times. Therefore, we also measure the variation of ∫Ωw in time through the L1–oscillation defined as*
*If A is a measurable subset of Rm, by |A| we denotes its Lebesgue measure.
Ow=1T−to∫Tto∫Ω|w(t,x)−1|Ω|¯W|dxdt. | (4.4) |
Our preferring the L1 based quantity above is justified by system (2.1)–(2.2) being well posed in L1, see [9]. Note however that in a realistic environment several other aspects of the pest distribution can be relevant, such as the size of their peaks or their location, or also their reaching very low values, essentially suggesting extinction.
First, as a reference case, we integrate (2.1)–(2.2) with no external input of predators, i.e., with q≡0, which corresponds to the case considered in [8,12]. The total number of predators and prey display a typical Lotka–Volterra like behavior, with synchronized oscillations in the total number of individuals in the two populations, see Figure 2, left. The geometric distribution of prey is strongly affected by the very fast diffusion driven by the Laplacian, see Figure 2, right. In turn, the predators' geometric distribution follows, since predators move towards high prey density regions, due to (2.2).
The resulting key final total values of predators and prey, as well as the values of ¯W and of Ow are the following:
∫Ωu(T,x)dx=98.865,∫Ωw(T,x)dx=27.321,¯W=28.809,Ow=8.126. | (4.5) |
Below, while comparing different strategies, an obvious key role is played by the quantity ∫T0∫R2q(t,x)dxdt, which represents the total amount of predators introduced in the environment during the time interval [0,T]. Clearly, in general, higher values of this integral lead to higher effects on the prey population. Therefore, whenever q is present in the integrations below, we keep
∫T0∫Ωq(t,x)dxdt=1200. | (4.6) |
The first strategy we investigate consists in releasing predators periodically into the environment all along two strips symmetric with respect to the origin:
q(t,x)={100(1+sin(2πνt))|x2|∈[0.5,0.75],0otherwise, | (4.7) |
We now let the frequency ν in (4.7) vary. The resulting average presence ¯W, as defined in (4.3), of the w population is affected up to about 10% by changes of ν in (4.7), while the oscillation Ow, defined in (4.4), spans a wider range:
ν in (4.7)01/41/31/22/312¯W in (4.3)11.83013.40212.97412.02011.64111.86711.844Ow in (4.4)2.16717.80216.3918.3714.7482.6452.182 | (4.8) |
The best performance, measured through the value of ¯W, is obtained with ν=2/3. The total populations resulting from the integrations with the smallest and largest choices of ν are shown in Figure 3. These results open the way to a variety of further investigations. Indeed, while the mean presence of pest ¯W is similar in the two situations (with variations of about 10%), the two evolutions are entirely different. A single criterion can be too little to evaluate the success of a strategy. When ν=1/4 pests almost vanish and then rise to rather high values. On the contrary, when ν=2, the total pest population displays small oscillations around the mean value. Clearly, on the basis of considerations specific to the real situation at hand, a measure of the dispersion of t→∫Ωw(t,x)dx should better be taken into account. A possible choice can be for instance Ow, as defined in (4.4), so that the optimal strategy q might be sought as the one minimizing a function of the type q→¯W(q)+λOw(q), for a carefully chosen positive weight λ.
It is now natural to investigate the effects of strategies similar to that in (4.7), but alternating the insertion of predators between the two strips, that is:
q(t,x)={100(1+sin(2πνt))x2∈[0.5,0.75],100(1−sin(2πνt))x2∈[−0.75,−0.5],0otherwise, | (4.9) |
Clearly, the case ν=0 is equivalent to the reference case (4.5). The values of ¯W resulting from different frequencies, defined in (4.3), are now:†
†In some of the integrations detailed here, the chosen values of ν lead to a small error in the constraint (4.6).
ν in (4.7)0.01/41/31/22/312¯W in (4.3)11.83011.85711.85911.85911.86111.87111.922Ow in (4.4)2.1672.2202.2222.2252.2312.2522.347 | (4.10) |
The values of the cost ¯W are similar in the two cases (4.10) and (4.8), now the minimal cost being obtained with ν=2/3 in (4.7). However, the behavior of the total amount of prey is significantly different, as it clearly results comparing Figure 4, related to the present case (4.9), to Figure 3. Indeed, alternating the introduction of prey in the two strips as in (4.9) appears significantly more effective in reducing the peaks in the pest density than the strategy (4.7). Coherently, the range of values attained by the oscillation Ow, as defined in (4.4), is narrower than that in (4.8), as shown in the table in (4.10).
An example of the dynamics resulting from (2.1)–(2.2)–(4.1) with initial datum (4.2) and control (4.9), where ν=1/4, is in Figure 5: the deployment of predators reaches its maximum for x2∈[0.5,0.75] at t=1, leftmost diagrams, and for x2∈[−0.75,−0.5] at t=3, rightmost diagrams. At time t=2, middle diagrams, the same amount of predators is released in the two regions x2∈[0.5,0.75] and x2∈[−0.75,−0.5]. The effects of the increased presence of predators is a temporary reduction in the pest density in the same region.
For completeness, we remark that the actual deploying of alternated treatments might reasonably have a higher cost.
A strategy entirely different from the one considered above consists in deploying predators uniformly over all of Ω. Below we investigate in detail the effects of treatments applied for relatively short, but carefully chosen, time intervals.
First, as a reference situation, we present the effects of a uniform insertion of predators all during the interval [0,T] and all over the region Ω, always fulfilling the constraint (4.6). The plots of the resulting total amount of predators and of prey are in Figure 6. Remarkably, this strategy leads to the rather low value ¯W=11.932, but pests remain significantly present throughout all the time interval [0,T] with relatively small oscillations, as testified by the value Ow=2.185.
We now consider the (probably to some extent rather realistic) strategy consisting in a single treatment deployed to the whole domain for a relatively short period, so that
q(t,x)=ˉqχI(t) with ˉq>0 and I interval in [0,T]. | (4.11) |
Different choices of ˉq and I lead to quite different evolutions of the model (2.1)–(2.2). When effective, such a treatment causes a sharp increase in the u population which, in turn, may bring the pests w near to extinction. Note however that, as it is usual in Lotka–Volterra type systems, as soon as w is not identically and exactly 0, the term γw in the source of the second equation in (2.1) eventually ensures an exponential growth to the w population. Therefore, the value of ˉq and the position of the interval I have to be carefully tuned to the interval of interest [to,T].
We choose I of length 2, so that ˉq=150 according to (4.6). The integration of (2.1)–(2.2)–(4.1)–(4.11) thus yields the following results:
ˉq=150.I in (4.11)[3,5][4,6][5,7][6,8][7,9][8,10][9,11]¯W in (4.3)16.93816.14120.92112.32711.68115.32018.442Ow in (4.4)29.24727.58531.39215.93013.83814.48813.660 | (4.12) |
Table (4.12) clearly shows that the cost ¯W is not a monotone function of the position of the interval I (or, more precisely, say of its minimum). Indeed, the fact that the cost ¯W depends on the solution computed on the interval [4,12] imposes that applying a treatment too early may cause the pest first to essentially disappear, but then to be reborn before time T=12. On the contrary, a late treatment allows the growth of the pests for a too long time and is then not able any more to significantly reduce them. Indeed, the first treatment (see Figure 7, left), is carried on the time interval [3,5] and brings prey almost to extinction. But it is too early and pests have the chance to re-develop. Similarly to a typical Lotka–Volterra dynamics far from the stable equilibrium, the total masses of predators and prey display very wide oscillations.
The opposite situation is that of a too late treatment, see Figure 7, right. Here, predators are able to wipe out pests, but it happens too late, so that the contribution to ¯W from the times before predators were introduced is too high to make this strategy convenient.
The middle diagrams in Figure 7 display the most convenient choice among the ones considered. Pests essentially disappear thanks to the insertion of predators and are not able to recover before the final time T=12.
The present global intense treatments introduce a peak in the predators' density and, as a consequence, pests almost disappear. Thus, the L1–oscillation (4.4) only gives a rough measure of the dispersion of the prey density around the value ¯W.
A possible refinement of the strategy (4.11) might consist in repeating the insertion of predators twice, so that
q(t,x)=ˉq1χI1(t)+ˉq2χI2(t) with ˉq1,ˉq2>0 and I1,I2 intervals in [0,T]. | (4.13) |
In the present setting, the only constraint on (4.13) is (4.6), which now reads
ˉq1|I1|+ˉq2|I2|=1200. | (4.14) |
A thorough optimization of ¯W as a function of the 6 variables ˉq1,ˉq2,infI1,supI1,infI2,supI2 subject to the constraints (4.13) and (4.14) is now a question of a conceptually simple but numerically quite heavy procedure, the Lipschitz continuous dependence of the solution to (2.1)–(2.2)–(4.1)–(4.13) from these variables being ensured in [9], see also Theorem 3.1. Here, we only consider the two sample cases detailed below:
Case 1: {ˉq1=150,I1=[4,5],ˉq2=150,I2=[8,9],¯W=10.274,Ow=17.034, | (4.15) |
Case 2: {ˉq1=200,I1=[4,5],ˉq2=100,I2=[8,9],¯W=10.441,Ow=15.298. | (4.16) |
Again, it stems also from the integrations detailed in Figure 8 that the choice of the strategy q may lead to a variety of evolutions. Depending on the relevance for the controller of the possible appearance of peaks in the pest population and of their geometric distribution, the fine tuning of the various parameters can lead to quite different results. For instance, a key role is played by the positions of these peaks resulting from the Lotka–Volterra type dynamics relative to the time interval chosen for the optimization.
Note that, in spite of the high peak appearing in the pest distribution after time t=10, the latter integration results in the lowest values of ¯W obtained so far: ¯W=10.44.
The present modeling framework allows to investigate the role of the regions where predators are deployed. We consider below two somewhat extreme situations, with q supported either in the corners of Ω, so that
q(t,x)={400|x1|∈[0.75,1] and |x2|∈[0.75,1]0 otherwise, | (4.17) |
or supported in the center of Ω, that is
q(t,x)={1600|x1|∈[0,0.125] and |x2|∈[0,0.125]0 otherwise. | (4.18) |
Once again, the fact that pests' movements are driven by the Laplacian, with predators hunting for pests, characterizes the overall behavior of both populations. Prey accumulate at the center of Ω, attracting predators. In the former case, the "hunting" attitude of the predators described by the speed law (2.2) makes them move towards the center of Ω, where prey accumulate, see Figure 9, left. In the latter case, predators are released directly where the pests density is higher. Hence, treatments that privilege deployments of predators at the center of Ω can be more effective, as confirmed by the comparison of the values of ¯W in the two cases:
q as in (4.17):¯W=13.452,Ow=3.205,q as in (4.18):¯W=11.950,Ow=2.011. | (4.19) |
In both cases (4.17) and (4.18), in the long run the pest population is stabilized and oscillations are relatively weak, see Figure 10.
We note, as it has to be expected, that the dynamic equilibrium in the case (4.17) of q supported near to corners also leads to a higher average presence of predators, coherently with the higher average presence of prey.
The above paragraphs show that by means of model (2.1)–(2.2) we are able to test a variety of pest control strategies. Above, we describe some of the possible scenarios that fall within the proposed approach. Many different criteria can be used in testing, comparing and choosing among the different strategies. Here, particular relevance is given to the cost ¯W and to the quantity Ow as a measure of the variability of the solution, but a variety of alternatives are conceivable and can be addressed through the present techniques.
A key aspect in the dynamics described by (2.1)–(2.2) is the presence of the Laplace operator in the second equation to describe pest diffusion. While it is a very usual choice, it allows an extremely fast propagation of the pest population, which is in contrast with the predator speed, bounded by κ, see (2.2). Whenever modeling reasons justify it, a possible improvement of the present model might consist in modifying the second equation in (2.1) so that also prey move with bounded speed.
The first author was partly supported by the PRIN 2015 project Hyperbolic Systems of Conservation Laws and Fluid Dynamics: Analysis and Applications and by the GNAMPA 2018 project Conservation Laws: Hyperbolic Games, Vehicular Traffic and Fluid dynamics. The second author acknowledges the support of the Lorentz Center. The IBM Power Systems Academic Initiative substantially contributed to the numerical integrations.
The authors declare there is no conflict of interest.
[1] | StatistaStatista, Social Media & User-Generated Content, 2024 (2024). Available from: https://www.statista.com/statistics/278414/number-of-worldwide-social-network-users/ |
[2] |
Meng SQ, Cheng JL, Li YY, et al. (2022) Global prevalence of digital addiction in general population: A systematic review and meta-analysis. Clin Psychol Rev 92: 102128. https://doi.org/10.1016/j.cpr.2022.102128 ![]() |
[3] |
Cheng C, Lau Y, Chan L, et al. (2021) Prevalence of social media addiction across 32 nations: Meta-analysis with subgroup analysis of classification schemes and cultural values. Addict Behav 117: 106845. https://doi.org/10.1016/j.addbeh.2021.106845 ![]() |
[4] |
Arrivillaga C, Rey L, Extremera N (2022) A mediated path from emotional intelligence to problematic social media use in adolescents: The serial mediation of perceived stress and depressive symptoms. Addict Behav 124: 107095. https://doi.org/10.1016/j.addbeh.2021.107095 ![]() |
[5] |
Bányai F, Zsila Á, Király O, et al. (2017) Problematic Social Media Use: Results from a Large-Scale Nationally Representative Adolescent Sample. PLoS ONE 12: e0169839. https://doi.org/10.1371/journal.pone.0169839 ![]() |
[6] |
Sindermann C, Elhai JD, Montag C (2020) Predicting tendencies towards the disordered use of Facebook's social media platforms: On the role of personality, impulsivity, and social anxiety. Psychiatry Res 285: 112793. https://doi.org/10.1016/j.psychres.2020.112793 ![]() |
[7] |
Keles B, McCrae N, Grealish A (2020) A systematic review: The influence of social media on depression, anxiety and psychological distress in adolescents. Int J Adolesc Youth 25: 79-93. https://doi.org/10.1080/02673843.2019.1590851 ![]() |
[8] |
Kuss D, Griffiths M, Karila L, et al. (2014) Internet Addiction: A Systematic Review of Epidemiological Research for the Last Decade. CPD 20: 4026-4052. https://doi.org/10.2174/13816128113199990617 ![]() |
[9] |
Xanidis N, Brignell CM (2016) The association between the use of social network sites, sleep quality and cognitive function during the day. Comput Human Behav 55: 121-126. https://doi.org/10.1016/j.chb.2015.09.004 ![]() |
[10] |
Huang C (2022) A meta-analysis of the problematic social media use and mental health. Int J Soc Psychiatry 68: 12-33. https://doi.org/10.1177/0020764020978434 ![]() |
[11] |
Shannon H, Bush K, Villeneuve PJ, et al. (2022) Problematic social media use in adolescents and young adults: Systematic review and meta-analysis. JMIR Ment Health 9: e33450. https://doi.org/10.2196/33450 ![]() |
[12] |
Hong Y, Rong X, Liu W (2024) Construction of influencing factor segmentation and intelligent prediction model of college students' cell phone addiction model based on machine learning algorithm. Heliyon 10: e29245. https://doi.org/10.1016/j.heliyon.2024.e29245 ![]() |
[13] | DatareportalDatareportal, Global social media statistics, 2024 (2024). Available from: https://datareportal.com/social-media-users |
[14] | HumbleGreeks & social media research: An up-to-date Whitepaper for the Greek market, 2024 (2024). Available from: https://humble.gr/blog/insights/humble-research/ |
[15] |
Montag C, Yang H, Elhai JD (2021) On the psychology of TikTok use: A first glimpse from empirical findings. Front Public Health 9: 641673. https://doi.org/10.3389/fpubh.2021.641673 ![]() |
[16] |
Lodice R, Papapicco C (2021) To be a TikToker in COVID-19 era: An experience of social influence. Online J Commun Medi 11: e202103. https://doi.org/10.30935/ojcmt/9615 ![]() |
[17] |
Smith T, Short A (2022) Needs affordance as a key factor in likelihood of problematic social media use: Validation, latent Profile analysis and comparison of TikTok and Facebook problematic use measures. Addict Behav 129: 107259. https://doi.org/10.1016/j.addbeh.2022.107259 ![]() |
[18] |
Casale S, Rugai L, Fioravanti G (2018) Exploring the role of positive metacognitions in explaining the association between the fear of missing out and social media addiction. Addict Behav 85: 83-87. https://doi.org/10.1016/j.addbeh.2018.05.020 ![]() |
[19] |
Tarafdar M, Maier C, Laumer S, et al. (2020) Explaining the link between technostress and technology addiction for social networking sites: A study of distraction as a coping behavior. Inf Syst J 30: 96-124. https://doi.org/10.1111/isj.12253 ![]() |
[20] |
Iram, Aggarwal H (2020) Time series analysis of pubg and tiktok applications using sentiments obtained from social media-twitter. Adv Math, Sci J 9: 4047-4057. https://doi.org/10.37418/amsj.9.6.86 ![]() |
[21] |
Zhang X, Wu Y, Liu S (2019) Exploring short-form video application addiction: Socio-technical and attachment perspectives. Telemat Inform 42: 101243. https://doi.org/10.1016/j.tele.2019.101243 ![]() |
[22] |
Lu L, Liu M, Ge B, et al. (2022) Adolescent addiction to short video applications in the mobile internet era. Front Psychol 13: 893599. https://doi.org/10.3389/fpsyg.2022.893599 ![]() |
[23] |
Qin Y, Musetti A, Omar B (2023) Flow experience is a key factor in the likelihood of adolescents' problematic TikTok use: The moderating role of active parental mediation. IJERPH 20: 2089. https://doi.org/10.3390/ijerph20032089 ![]() |
[24] |
Montag C, Lachmann B, Herrlich M, et al. (2019) Addictive features of social media/messenger platforms and freemium games against the background of psychological and economic theories. IJERPH 16: 2612. https://doi.org/10.3390/ijerph16142612 ![]() |
[25] | Burhan R, Moradzadeh J (2020) Neurotransmitter Dopamine (DA) and its role in the development of social media addiction. J Neurol Neurophysiol 11: 1-2. |
[26] |
Su C, Zhou H, Gong L, et al. (2021) Viewing personalized video clips recommended by TikTok activates default mode network and ventral tegmental area. NeuroImage 237: 118136. https://doi.org/10.1016/j.neuroimage.2021.118136 ![]() |
[27] |
Varona MN, Muela A, Machimbarrena JM (2022) Problematic use or addiction? A scoping review on conceptual and operational definitions of negative social networking sites use in adolescents. Addict Behav 134: 107400. https://doi.org/10.1016/j.addbeh.2022.107400 ![]() |
[28] |
Andreassen CS, Torsheim T, Brunborg GS, et al. (2012) Development of a facebook addiction scale. Psychol Rep 110: 501-517. https://doi.org/10.2466/02.09.18.PR0.110.2.501-517 ![]() |
[29] |
Andreassen CS, Billieux J, Griffiths MD, et al. (2016) The relationship between addictive use of social media and video games and symptoms of psychiatric disorders: A large-scale cross-sectional study. Psychol Addict Behav 30: 252-262. https://doi.org/10.1037/adb0000160 ![]() |
[30] |
Van Den Eijnden RJJM, Lemmens JS, Valkenburg PM (2016) The social media disorder scale. Comput Human Behav 61: 478-487. https://doi.org/10.1016/j.chb.2016.03.038 ![]() |
[31] |
Elphinston RA, Noller P (2011) Time to face it! Facebook intrusion and the implications for romantic jealousy and relationship satisfaction. Cyberpsychol Behav Soc Netw 14: 631-635. https://doi.org/10.1089/cyber.2010.0318 ![]() |
[32] |
Caplan SE (2010) Theory and measurement of generalized problematic Internet use: A two-step approach. Comput Human Behav 26: 1089-1097. https://doi.org/10.1016/j.chb.2010.03.012 ![]() |
[33] |
Young KS (1998) Internet addiction: The emergence of a new clinical disorder. Cyberpsychol Behav 1: 237-244. https://doi.org/10.1089/cpb.1998.1.237 ![]() |
[34] |
Dadiotis A, Bacopoulou F, Kokka I, et al. (2021) Validation of the Greek version of the Bergen social media addiction scale in undergraduate students. EMBnet J 26: e975. https://doi.org/10.14806/ej.26.1.975 ![]() |
[35] |
Floros G, Siomos K (2012) Patterns of choices on video game genres and internet addiction. Cyberpsychol Behav Soc Netw 15: 417-424. https://doi.org/10.1089/cyber.2012.0064 ![]() |
[36] |
Kokka I, Mourikis I, Michou M, et al. (2021) Validation of the Greek version of social media disorder scale. GeNeDis 2020. Cham: Springer International Publishing 107-116. https://doi.org/10.1007/978-3-030-78775-2_13 ![]() |
[37] |
Zhu J, Ma Y, Xia G, et al. (2024) Self-perception evolution among university student TikTok users: evidence from China. Front Psychol 14: 1217014. https://doi.org/10.3389/fpsyg.2023.1217014 ![]() |
[38] |
Alhabash S, Smischney TM, Suneja A, et al. (2024) So similar, yet so different: How motivations to use Facebook, Instagram, Twitter, and TikTok predict problematic use and use continuance intentions. Sage Open 14: 21582440241255426. https://doi.org/10.1177/21582440241255426 ![]() |
[39] |
Hendrikse C, Limniou M (2024) The use of Instagram and TikTok in relation to problematic use and well-being. J Technol Behav Sci 9: 1-12. https://doi.org/10.1007/s41347-024-00399-6 ![]() |
[40] | Yang Y, Adnan H, Sarmiti N (2023) The relationship between anxiety and TikTok addiction among university students in China: Mediated by escapism and use intensity. Int J Media Inf Lit 8. https://doi.org/10.13187/ijmil.2023.2.458 |
[41] |
Rogowska AM, Cincio A (2024) Procrastination mediates the relationship between problematic TikTok Use and depression among young adults. JCM 13: 1247. https://doi.org/10.3390/jcm13051247 ![]() |
[42] |
Pontes HM, Schivinski B, Sindermann C, et al. (2021) Measurement and conceptualization of gaming disorder according to the World Health Organization framework: The development of the gaming disorder test. Int J Ment Health Addiction 19: 508-528. https://doi.org/10.1007/s11469-019-00088-z ![]() |
[43] |
Montag C, Markett S (2024) Depressive inclinations mediate the association between personality (neuroticism/conscientiousness) and TikTok Use Disorder tendencies. BMC Psychol 12: 81. https://doi.org/10.1186/s40359-024-01541-y ![]() |
[44] |
McCoach D, Gable R, Madura J (2013) Review of the steps for designing an instrument. Instrument development in the affective domain. New York: Springer 277-284. https://doi.org/10.1007/978-1-4614-7135-6_8 ![]() |
[45] |
Bekalu MA, Sato T, Viswanath K (2023) Conceptualizing and measuring social media use in health and well-being studies: Systematic review. J Med Internet Res 25: e43191. https://doi.org/10.2196/43191 ![]() |
[46] |
Darvesh N, Radhakrishnan A, Lachance CC, et al. (2020) Exploring the prevalence of gaming disorder and internet gaming disorder: A rapid scoping review. Syst Rev 9: 68. https://doi.org/10.1186/s13643-020-01329-2 ![]() |
[47] |
Pan YC, Chiu YC, Lin YH (2020) Systematic review and meta-analysis of epidemiology of internet addiction. Neurosci Biobehav Rev 118: 612-622. https://doi.org/10.1016/j.neubiorev.2020.08.013 ![]() |
[48] | Brown R (1993) Some contributions of the study of gambling to the study of other addictions. Gambling Behaviour and Problem Gambling. Reno: University of Nevada Press 241-272. |
[49] |
Griffiths M (1996) Nicotine, tobacco and addiction. Nature 384: 18. https://doi.org/10.1038/384018a0 ![]() |
[50] | Griffiths M (2005) A ‘components’ model of addiction within a biopsychosocial framework. J Subst Abuse 10: 191-197. https://doi.org/10.1080/14659890500114359 |
[51] | WHOInternational classification of diseases 11th revision, 2024 (2024). Available from: https://icd.who.int/en |
[52] |
Ayre C, Scally AJ (2014) Critical values for Lawshe's content validity ratio: Revisiting the original methods of calculation. Meas Eval Counsel Dev 47: 79-86. https://doi.org/10.1177/0748175613513808 ![]() |
[53] |
Meadows K (2021) Cognitive interviewing methodologies. Clin Nurs Res 30: 375-379. https://doi.org/10.1177/10547738211014099 ![]() |
[54] |
Yusoff MSB (2019) ABC of response process validation and face validity index calculation. EIMJ 11: 55-61. https://doi.org/10.21315/eimj2019.11.3.6 ![]() |
[55] | Costello AB, Osborne J (2005) Best practices in exploratory factor analysis: Four recommendations for getting the most from your analysis. Pract Assess Res Eval 10: 1-9. |
[56] |
DeVon HA, Block ME, Moyle-Wright P, et al. (2007) A psychometric toolbox for testing validity and reliability. J Nurs Scholarsh 39: 155-164. https://doi.org/10.1111/j.1547-5069.2007.00161.x ![]() |
[57] | De Vaus D (2004) Surveys in social research. London: Routledge 180-200. |
[58] |
Yusoff MSB, Arifin WN, Hadie SNH (2021) ABC of questionnaire development and validation for survey research. EIMJ 13: 97-108. https://doi.org/10.21315/eimj2021.13.1.10 ![]() |
[59] | Hair J, Black W, Babin B, et al. (2017) Multivariate data analysis. New Jersey: Prentice Hall 45-55. |
[60] |
De Winter JCF, Dodou D, Wieringa PA (2009) Exploratory factor analysis with small sample sizes. Multivariate Behav Res 44: 147-181. https://doi.org/10.1080/00273170902794206 ![]() |
[61] | Klein R (2016) Principles and practice of structural equation modelling. New York: Guilford Press 188-210. |
[62] |
Bland JM, Altman DG (1997) Statistics notes: Cronbach's alpha. BMJ 314: 572-572. https://doi.org/10.1136/bmj.314.7080.572 ![]() |
[63] | Brown T (2015) Confirmatory factor analysis for applied research. New York: The Guilford Press 72-87. |
[64] |
Hu L, Bentler PM (1998) Fit indices in covariance structure modeling: Sensitivity to underparameterized model misspecification. Psychol Methods 3: 424-453. https://doi.org/10.1037/1082-989X.3.4.424 ![]() |
[65] |
Baumgartner H, Homburg C (1996) Applications of structural equation modeling in marketing and consumer research: A review. Int J Res Mark 13: 139-161. https://doi.org/10.1016/0167-8116(95)00038-0 ![]() |
[66] | Kroenke K, Spitzer RL, Williams JBW, et al. (2009) An ultra-brief screening scale for anxiety and depression: The PHQ-4. Psychosomatics 50: 613-621. https://doi.org/10.1176/appi.psy.50.6.613 |
[67] |
Rammstedt B, John OP (2007) Measuring personality in one minute or less: A 10-item short version of the Big Five Inventory in English and German. J Res Pers 41: 203-212. https://doi.org/10.1016/j.jrp.2006.02.001 ![]() |
[68] |
Copez-Lonzoy A, Vallejos-Flores M, Capa-Luque W, et al. (2023) Adaptation of the Bergen Social Media Addiction Scale (BSMAS) in Spanish. Acta Psychol (Amst) 241: 104072. https://doi.org/10.1016/j.actpsy.2023.104072 ![]() |
[69] | Brailovskaia J, Margraf J (2022) Addictive social media use during Covid-19 outbreak: Validation of the Bergen Social Media Addiction Scale (BSMAS) and investigation of protective factors in nine countries. Curr Psychol 1–19. https://doi.org/10.1007/s12144-022-03182-z |
[70] |
Shin NY (2022) Psychometric properties of the bergen social media addiction scale in Korean young adults. Psychiatry Investig 19: 356-361. https://doi.org/10.30773/pi.2021.0294 ![]() |
[71] |
Žmavc M, Šorgo A, Gabrovec B, et al. (2022) The protective role of resilience in the development of social media addiction in tertiary students and psychometric properties of the Slovenian Bergen Social Media Addiction Scale (BSMAS). Int J Environ Res Public Health 19: 13178. https://doi.org/10.3390/ijerph192013178 ![]() |
[72] |
Rouleau RD, Beauregard C, Beaudry V (2023) A rise in social media use in adolescents during the COVID-19 pandemic: the French validation of the Bergen Social Media Addiction Scale in a Canadian cohort. BMC Psychol 11: 92. https://doi.org/10.1186/s40359-023-01141-2 ![]() |
[73] |
Cunningham S, Hudson CC, Harkness K (2021) Social media and depression symptoms: A meta-analysis. Res Child Adolesc Psychopathol 49: 241-253. https://doi.org/10.1007/s10802-020-00715-7 ![]() |
[74] |
Hussain Z, Wegmann E, Yang H, et al. (2020) Social networks use disorder and associations with depression and anxiety symptoms: A systematic review of recent research in China. Front Psychol 11: 211. https://doi.org/10.3389/fpsyg.2020.00211 ![]() |
[75] |
Caro-Fuentes S, Sanabria-Mazo JP (2024) A systematic review of the psychometric properties of the patient Health Questionnaire-4 in clinical and nonclinical populations. J Acad Consult Liaison Psychiatry 65: 178-194. https://doi.org/10.1016/j.jaclp.2023.11.685 ![]() |
[76] |
Meidl V, Dallmann P, Leonhart R, et al. (2024) Validation of the patient health Questionnaire-4 for longitudinal mental health evaluation in elite Para athletes. PM R 16: 141-149. https://doi.org/10.1002/pmrj.13011 ![]() |
[77] |
Rodríguez-Muñoz M de la F, Ruiz-Segovia N, Soto-Balbuena C, et al. (2020) The psychometric properties of the patient health Questionnaire-4 for pregnant women. Int J Environ Res Public Health 17: 7583. https://doi.org/10.3390/ijerph17207583 ![]() |
[78] |
Tan YK, Siau CS, Ibrahim N, et al. (2024) Validation of the Malay version of the Patient Health Questionnaire-4 (PHQ-4) among Malaysian undergraduates. Asian J Psychiatr 99: 104134. https://doi.org/10.1016/j.ajp.2024.104134 ![]() |
[79] |
Karekla M, Pilipenko N, Feldman J (2012) Patient health questionnaire: Greek language validation and subscale factor structure. Compr Psychiatry 53: 1217-1226. https://doi.org/10.1016/j.comppsych.2012.05.008 ![]() |
[80] |
Correa T, Hinsley AW, De Zúñiga HG (2010) Who interacts on the Web?: The intersection of users' personality and social media use. Comput Human Behav 26: 247-253. https://doi.org/10.1016/j.chb.2009.09.003 ![]() |
[81] |
Kuss DJ, Griffiths MD (2011) Online social networking and addiction—A review of the psychological literature. IJERPH 8: 3528-3552. https://doi.org/10.3390/ijerph8093528 ![]() |
[82] |
Wilson K, Fornasier S, White KM (2010) Psychological predictors of young adults' use of social networking sites. Cyberpsychol Behav Soc Netw 13: 173-177. https://doi.org/10.1089/cyber.2009.0094 ![]() |
[83] |
Kunnel John R, Xavier B, Waldmeier A, et al. (2019) Psychometric evaluation of the BFI-10 and the NEO-FFI-3 in Indian adolescents. Front Psychol 10: 1057. https://doi.org/10.3389/fpsyg.2019.01057 ![]() |
[84] |
Costa Mastrascusa R, De Oliveira Fenili Antunes ML, De Albuquerque NS, et al. (2023) Evaluating the complete (44-item), short (20-item) and ultra-short (10-item) versions of the Big Five Inventory (BFI) in the Brazilian population. Sci Rep 13: 7372. https://doi.org/10.1038/s41598-023-34504-1 ![]() |
[85] |
Balgiu BA (2018) The psychometric properties of the Big Five inventory-10 (BFI-10) including correlations with subjective and psychological well-being. GJPR 8: 61-69. https://doi.org/10.18844/gjpr.v8i2.3434 ![]() |
[86] |
Soto CJ, John OP (2017) The next Big Five Inventory (BFI-2): Developing and assessing a hierarchical model with 15 facets to enhance bandwidth, fidelity, and predictive power. J Pers Soc Psychol 113: 117-143. https://doi.org/10.1037/pspp0000096 ![]() |
[87] | World Medical Association.World medical association declaration of Helsinki: Ethical principles for medical research involving human subjects. JAMA (2013) 310: 2191. https://doi.org/10.1001/jama.2013.281053 |
![]() |
![]() |
1. | Rinaldo M. Colombo, Magali Lecureux-Mercier, Mauro Garavello, 2020, Chapter 5, 978-3-030-50449-6, 83, 10.1007/978-3-030-50450-2_5 | |
2. | Dietmar Hömberg, Robert Lasarzik, Luisa Plato, On the existence of generalized solutions to a spatio-temporal predator–prey system with prey-taxis, 2023, 23, 1424-3199, 10.1007/s00028-023-00871-5 | |
3. | Alberto Bressan, Maria Teresa Chiri, Najmeh Salehi, On the optimal control of propagation fronts, 2022, 32, 0218-2025, 1109, 10.1142/S0218202522500257 | |
4. | Toni Bakhtiar, Ihza Rizkia Fitri, Farida Hanum, Ali Kusnanto, Mathematical Model of Pest Control Using Different Release Rates of Sterile Insects and Natural Enemies, 2022, 10, 2227-7390, 883, 10.3390/math10060883 | |
5. | Rinaldo M. Colombo, Elena Rossi, Well‐posedness and control in a hyperbolic–parabolic parasitoid–parasite system, 2021, 147, 0022-2526, 839, 10.1111/sapm.12402 | |
6. | Estefanía Rodríguez, Mario Porcel, Lidia Lara, Tomás Cabello, Manuel Gámez, Leticia Navarro, Alberto Domingo, Francisco Javier Burguillo, María del Mar Téllez, Role of eggplant trichome in whitefly oviposition and its relevance to biological control under greenhouse conditions, 2024, 14, 2045-2322, 10.1038/s41598-024-73327-6 | |
7. | Alberto Bressan, Minyan Zhang, Controlled traveling profiles for models of invasive biological species, 2024, 30, 1292-8119, 28, 10.1051/cocv/2024018 | |
8. | Robert Mamada, Edge formation along population gradients: Modeling the interplay of population growth and mobility in ecological and social–ecological systems, 2025, 11, 25902911, 101516, 10.1016/j.ssaho.2025.101516 |