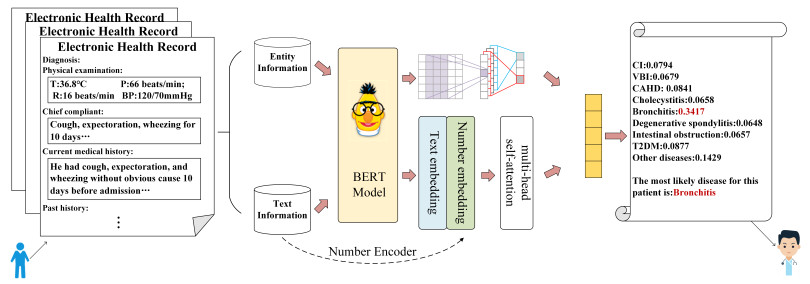
Disease prediction by using a variety of healthcare data to assist doctors in disease diagnosis is becoming a more and more important research topic recently. This paper proposes a disease prediction model that fuses multiple types of encoded representations of Chinese electronic health records (EHRs). The model framework utilizes a multi-head self-attention mechanism, which combines textual and numerical features to enhance text representations. The BiLSTM-CRF and TextCNN models are used, respectively, to extract entities and then obtain the embedding representations of them. The representations of text and entities in it are combined together for formulating representations of EHRs. The experimental results on EHRs data collected from a Three Grade Class B Hospital General in Gansu Province, China, show that our model achieved an F1 score of 91.92%, which outperforms the previous baseline methods.
Citation: Zhaoyu Liang, Zhichang Zhang, Haoyuan Chen, Ziqin Zhang. Disease prediction based on multi-type data fusion from Chinese electronic health record[J]. Mathematical Biosciences and Engineering, 2022, 19(12): 13732-13746. doi: 10.3934/mbe.2022640
[1] | Ruirui Han, Zhichang Zhang, Hao Wei, Deyue Yin . Chinese medical event detection based on event frequency distribution ratio and document consistency. Mathematical Biosciences and Engineering, 2023, 20(6): 11063-11080. doi: 10.3934/mbe.2023489 |
[2] | Feng Li, Mingfeng Jiang, Hongzeng Xu, Yi Chen, Feng Chen, Wei Nie, Li Wang . Data governance and Gensini score automatic calculation for coronary angiography with deep-learning-based natural language extraction. Mathematical Biosciences and Engineering, 2024, 21(3): 4085-4103. doi: 10.3934/mbe.2024180 |
[3] | Xiaoqing Lu, Jijun Tong, Shudong Xia . Entity relationship extraction from Chinese electronic medical records based on feature augmentation and cascade binary tagging framework. Mathematical Biosciences and Engineering, 2024, 21(1): 1342-1355. doi: 10.3934/mbe.2024058 |
[4] | Chaofan Li, Kai Ma . Entity recognition of Chinese medical text based on multi-head self-attention combined with BILSTM-CRF. Mathematical Biosciences and Engineering, 2022, 19(3): 2206-2218. doi: 10.3934/mbe.2022103 |
[5] | Hongyang Chang, Hongying Zan, Shuai Zhang, Bingfei Zhao, Kunli Zhang . Construction of cardiovascular information extraction corpus based on electronic medical records. Mathematical Biosciences and Engineering, 2023, 20(7): 13379-13397. doi: 10.3934/mbe.2023596 |
[6] | Kunli Zhang, Shuai Zhang, Yu Song, Linkun Cai, Bin Hu . Double decoupled network for imbalanced obstetric intelligent diagnosis. Mathematical Biosciences and Engineering, 2022, 19(10): 10006-10021. doi: 10.3934/mbe.2022467 |
[7] | Lu Jiang, Shasha Xie, Yuqi Wang, Xin Xu, Xiaosa Zhao, Ye Zhang, Jianan Wang, Lihong Hu . SeekDoc: Seeking eligible doctors from electronic health record. Mathematical Biosciences and Engineering, 2021, 18(5): 5347-5363. doi: 10.3934/mbe.2021271 |
[8] | Hangle Hu, Chunlei Cheng, Qing Ye, Lin Peng, Youzhi Shen . Enhancing traditional Chinese medicine diagnostics: Integrating ontological knowledge for multi-label symptom entity classification. Mathematical Biosciences and Engineering, 2024, 21(1): 369-391. doi: 10.3934/mbe.2024017 |
[9] | Qinhua Tang, Xingxing Cen, Changqing Pan . Explainable and efficient deep early warning system for cardiac arrest prediction from electronic health records. Mathematical Biosciences and Engineering, 2022, 19(10): 9825-9841. doi: 10.3934/mbe.2022457 |
[10] | Kunli Zhang, Bin Hu, Feijie Zhou, Yu Song, Xu Zhao, Xiyang Huang . Graph-based structural knowledge-aware network for diagnosis assistant. Mathematical Biosciences and Engineering, 2022, 19(10): 10533-10549. doi: 10.3934/mbe.2022492 |
Disease prediction by using a variety of healthcare data to assist doctors in disease diagnosis is becoming a more and more important research topic recently. This paper proposes a disease prediction model that fuses multiple types of encoded representations of Chinese electronic health records (EHRs). The model framework utilizes a multi-head self-attention mechanism, which combines textual and numerical features to enhance text representations. The BiLSTM-CRF and TextCNN models are used, respectively, to extract entities and then obtain the embedding representations of them. The representations of text and entities in it are combined together for formulating representations of EHRs. The experimental results on EHRs data collected from a Three Grade Class B Hospital General in Gansu Province, China, show that our model achieved an F1 score of 91.92%, which outperforms the previous baseline methods.
Medical and health care has a bearing on the health of hundreds of millions of people and is a basic livelihood issue for people around the world. Specifically in China, the most populous country in the world, the total amount of medical resources is not abundant enough, resulting in an unbalanced supply and demand of medical services. According to information released by the National Health Commission of China, by the end of 2020, China had only 2.9 doctors per 1000 people, which means that there is only one doctor for every 300 people. To solve these problems, disease prediction has received increasing attention from academia and industry. While image-based [1] disease prediction has been well studied, research on text-based disease prediction [2] is still difficult due to the difficulty of understanding the Chinese language itself and obtaining a real and reliable clinical corpus.
Since the National Health and Family Planning Commission of China issued the "Basic Specifications for Electronic Health Records (Trial), " many hospitals have accumulated a lot of electronic health records (EHRs). EHRs are detailed records of medical activities by medical personnel, mostly written by doctors, including structured data (lab tests, vital signs, etc.) and unstructured data (chief complaints, current illness history, etc.). With the development and popularity of EHRs, more and more scholars are interested in disease prediction. Existing work has focused on graph-based [3] methods and classification-based [4] methods for disease prediction from EHRs. Graph-based methods focus on the relationships between symptoms and diseases for disease prediction. Classification-based methods mainly extract features from EHRs and predict disease for patients. Early research is mainly based on manually designed rules and traditional machine learning methods. The rule-based method has a high accuracy rate, but the construction of the rules requires the participation of personnel in the medical field, which is time-consuming and labor-intensive. Traditional machine learning methods, such as Support Vector Machine (SVM) [5] and Random Forest [6], can avoid this problem, but it is difficult to express deeper semantic information of EHRs. With the development of deep learning, its application in disease prediction [7, 8] has significantly improved the performance. However, the existing methods mainly focus on a single type of structured medical data [9] but ignore the differences and connections between varied types of medical data [10]. Such as gender information in EHRs, may be insufficient for these texts to use the same encoder for representation. Furthermore, the information of entities in disease prediction is often ignored. In order to solve the above problems, we propose a novel disease prediction model with multi-type data, and the overall structure is shown in Figure 1.
The contributions of this paper are as follows:
1) Entity information is integrated with text information to better obtain the representation of EHRs.
2) A multi-type data fusion model is proposed, which focuses on different ways to represent information respectively and improves obviously the accuracy of prediction and the interpretability of feature representations.
3) Evaluation of real EHRs from a Three Grade Class B General Hospital in Gansu Province, China, shows that the multi-type data fusion model outperforms previous disease prediction methods with EHRs.
Disease prediction is to use computer-related technology to extract features from EHRs and predict disease. Early research was mainly based on rules and knowledge reasoning expert systems [11]. Such methods are simple and easy to understand, but they require a lot of experts in the medical field to construct rules and are not flexible enough. With the continuous development of machine learning technology, more and more researchers apply these technologies for disease prediction. Palaniappand et al. [12] proposed a method for predicting heart disease using Naive Bayes and Decision Tree, which developed into a heart disease prediction system. Ananthakrishnan et al. [13] used logistic regression to diagnose Crohn's disease and ulcerative colitis. Drriseitl et al. [14] compared the algorithmic performances of K-nearest Neighbor, SVM and logistic regression in the diagnosis of skin diseases, and they found that SVM showed better performance.
With the development of deep learning in NLP tasks, there are many ways to use deep learning methods for disease prediction. Yang et al. [15] proposed a Convolutional Neural Network (CNN) model to obtain textual information in EHRs and perform disease prediction. An et al. [16] obtained different features of EHRs based on the BiLSTM model and fused different types of features to predict cardiovascular disease. Wang et al. [17] proposed a prediction method based on BiLSTM and CNN to model characters and words in EHRs, respectively. Du et al. [18] utilized a multigraph structural LSTM model and considered the Spatio-temporal characteristics to predict foodborne diseases. Rasmy et al. [19] utilized the CovRNN model to learn the representations of patients with COVID-19 and make relevant predictions, such as mortality and hospital stay. Sha and Wang [20] proposed a hierarchical GRU-based model to predict clinical outcomes based on the medical code of the patient's previous visits. With the proposed pre-training models of ELMO [21], OpenAI GPT [22] and BERT [23], significant improvements have been achieved in various NLP tasks, which have also been applied in the medical field. Zhang et al. [24] proposed a BERT based model with an enhanced layer to encode EHRs for auxiliary diagnosis in obstetrics. BioBERT [25] is a pre-trained model that was trained on general and biomedical domain corpora. Mugisha et al. [26] utilized BioBERT to obtain representations in EHRs and make predictions of pneumonia diseases. These research methods have improved the accuracy of disease prediction to a certain extent, but there are still some shortcomings. On the one hand, the disease prediction models based on traditional machine learning are often limited by the shortcomings of feature engineering and the algorithm itself, and they are heavily dependent on manual rules, leading to the failure of generalization of the models. On the other hand, these methods are mainly modeled by a single type of data information, and few of them pay enough attention to different data types.
In this paper, we propose a multi-type data fusion model based on EHRs, and the model structure is shown in Figure 2. The model can be divided into two parts: text representation and entity representation. The text representation module introduces BERT to get the encoded representation of the textual information; the encoding of the numerical information is achieved by one-hot and max-minimum normalization methods. Then, the textual and numerical encodings are sent to a multi-head self-attention layer, using the numerical information to enhance the text information and get a better text representation. The entity information is extracted by using the developed and relatively mature entity recognition technology. The pre-trained model BERT is used for encoding the characters of entities. Then, TextCNN is used for extracting features and obtaining entity representations. Finally, the two types of information are fused to get the final representation of the patient and make predictions about the disease.
The information contained in the EHRs text can be divided into structured and unstructured data. Unstructured data refers to textual information, while structured data refers to the information of demographics and physical examinations in this study, which are significant for disease prediction. For example, an older patient is more likely to have a cerebral infarction. It is considerable to convert structured data into numerical information for better representations. The specific situation is shown in Table 1.
Initial description | Gender:Female; Age:67; Marital Status:Married; Family History:None; |
T:36.8 ℃; P:62 beats/min; R:18 beats/min; Bp:140/90 mmHg | |
Numerical information | {(0, 67, 1, 0, 36.8, 62, 18, 140, 90)} |
Patient demographics include age, gender, marital status, family history and more. Only adult patients are concerned in this study, and their ages are split into 5 groups: (18, 25), (25, 45), (45, 65), (65, 89), (89, ). The patient's physical examination includes blood pressure (BP), heartbeat (R), pulse (P), body temperature (T), etc. The physical examination is encoded by max-min normalization. The demographic information is spliced to obtain the numerical representation of patients as Znum.
Text information in the EHRs includes the chief complaint, history of present illness, etc. Using appropriate algorithms to extract features from EHRs texts can better help patients with disease prediction. Due to the sparseness of Chinese EHRs, traditional methods, such as Doc2vec, can not accurately obtain the text representation of Chinese EHRs. However, a pre-training model based on transfer learning can achieve better results after fine-tuning in small scale samples after pre-training in large data sets. Therefore, we utilize the pre-trained language model BERT to obtain textual representations of EHRs. The input text sequence is as follows:
[CLS] Chinese Electronic Health Record [SEP] |
where, [CLS] indicates the start tag of the text, and [SEP] indicates the separator tag of the text. After the EHR is fed into the BERT model, the last layer of [CLS] is used to represent the entire EHR C. To better integrate numerical and textual information to obtain better text representations, we introduces the multi-head self-attention to enhance the textual representations of EHRs:
Q=K=V=Wcconcat(Znum,C), | (3.1) |
Attention(Q,K,V)=softmax(QKT√dk)V, | (3.2) |
Ztext=concat(head1,head2,…,headh)Wowhere headi=Attention(QWQi,KWKi,VWVi), | (3.3) |
where WiQ, WiK, WiV, Wc, Wo are trainable parameters, and Ztext is the final representation after enhancing of numerical information in the EHRs text.
Through the analysis of EHRs, we found that entity type information (symptoms, medicine, etc.) is important for disease prediction. For example, the symptoms of "coughing" may increase the risk of bronchitis. Therefore, it is a great necessity of introducing relatively mature named entity recognition technology to extract entity information from EHRs. The BiLSTM-CRF model we use can easily extract entity information, which can obtain contextual information more comprehensively and learn the relationship between contexts easily, and convert the extracted context information into corresponding labels for each Chinese character. The architecture of the BiLSTM-CRF model is illustrated in Figure 3. In the model, the BIO (Begin, Inside, Outside) tagging scheme is used. First, the Skip-Gram [27] algorithm is introduced to train character embedding in EHRs. The sentence is represented as a sequence of characters vector Q=(q1,q2,...,qn), where n is the length of the EHRs. Secondly, the embeddings (q1,q2,...,qn) are given as input to the BiLSTM layer. In the BiLSTM layer and at step t, the output state of the forward LSTM is the hidden vector →ht, and the output state of the other backward LSTM is hidden vectors ←ht. These two distinct networks use different parameters, and then the representation of a character ht=[→ht;←ht] is obtained by concatenating its forward and backward the hidden vector. Next, a full connection layer is used to map the hidden state vector (h1,h2,...,hn)∈Rn×m to k dimensions, where k is the number of labels in the label set. As a result, the sentence features are extracted that are represented as a matrix P=(p1,p2,...,pn)∈Rn×k. Finally, the parameters of the CRF layer are represented by a matrix A, and Aij denotes the score of the transition from the i-th label to the j-th label. Considering a sequence of labels y=(y1,y2,...,yn), the formula for calculating the score of the tag sequence is as follows.
score(x,y)=n∑i=1Pi,yi+n+1∑j=1Ayj−1,yj | (3.4) |
The score of the whole sequence is equal to the sum of the scores of all words within the sentence, which is determined by the output matrix P of BiLSTM layer and the transition matrix A of the CRF layer. Then, a softmax function is used to yield the conditional probability of the path by normalizing the above score over all possible tag paths y′.
P(y∣x)=escore(x,y)k∑y′=1escore(x,y′) | (3.5) |
During the training phase, the goal of this model is to maximize the log-probability of the correct tag sequence. In the prediction process, the score corresponding to each candidate sequence is calculated according to the trained parameters, and the optimal path is calculated using the Viterbi algorithm with dynamic programming as the core.
argmaxy′score(x,y′) | (3.6) |
For input sequence S={x1,x2,...,xn} of the EHR, xi represents i-th character in the EHR. Inputting the sequence into the BERT model to obtain the representation of each character,
H=[h1,h2,...,hn]=BERT([x1,x2,...,xn]). | (3.7) |
Suppose that vectors hi to hj are the final hidden state vectors from BERT for symptom entity esyi; we apply the average operation to obtain a vector representation for each of the entities. This process can be mathematically formalized as:
esyi=1j−i+1j∑t=iht. | (3.8) |
We get the symptom entity embeddings Esy=[esy1,esy2,...,esyn], and in the same way, we get the medicine and abnormal inspection result entity embeddings: Emed=[emed1,emed2,...,emedn], Eabn=[eabn1,eabn2,...,eabnn]. We separately performed convolution operations on the entity information of symptom, medicine and abnormal inspection results to extract various types of entity features. The convolution operation is carried out between convolution kernel w and symptom entity embedding in the ith window esyi:i+h−1 in the symptom entity Esy and obtained feature csyi :
csyi=f(esyi:i+h−1⋅w+b), | (3.9) |
where the size of the convolution kernel is w∈Rh×d, h is the height of the convolution kernel, and d is the dimension of the character embedding in BERT. b∈R is a bias term, and f is a non-linear function.
This filter is applied to each possible window of features in the event matrix {esy1:h,esy2:h+1,...,esyn−h+1:n} to produce a feature map csy=[csy1,csy2,...,csyn−h+1]. Then, max pooling is applied over the feature map, and the average c′sy=max{csy} is taken. In the same way, we convolved medicine and abnormal inspection results information to obtain the medicine and abnormal inspection results representation c′med, c′abn. The symptom, medicine and abnormal inspection results representation are spliced to obtain the final representation of the patient entity:
Zentity=concat(c′sy;c′med;c′abn). | (3.10) |
By splicing the representation of text and entity information, the final representation of EHR is denoted as Zpatient=concat(Ztext;Zentity), where the size of this vector is the sum of the components dtext+dentity. The EHR representation Zpatient is sent to the fully connected layer, and the probability of each type of disease is calculated by the softmax activation function. The formula is
y=softmax(w⋅Zpatient+b) | (3.11) |
where y denotes the prediction probability distribution of K disease classes (K=9). yi indicates the probability that the input EHR is related to the i-th disease.
In this paper, the cross-entropy loss function is used to train the model with the goal of minimizing the Loss:
Loss=−∑T∈Corpus∑Ki=1yi(T)log(yi(T)) | (3.12) |
where T is the input EHR, Corpus denotes training sample set and K is the number of classes.
Large-scale Chinese EHRs datasets with entity information are not always readily accessible. To facilitate research on Chinese EHRs, we collected a large raw dataset in a Three Grade Class B Hospital General in Gansu Province, China, which contained 61, 233 EHRs. We select 8 kinds of diseases, including cerebral infarction (CI), vertebrobasilar insufficiency (VBI), coronary atherosclerotic heart disease (CAHD), cholecystitis, bronchitis, degenerative spondylitis, intestinal obstruction, type 2 diabetic peripheral neuropathy (T2DM), and select some other diseases as the Chinese Electronic Health Record dataset (CEHR). Before the experiments of our study, the following preprocessing was carried out on the CEHR text:
1) De-privacy: Delete the patient's personal private information from CEHRs, such as: 'name', 'place of birth', 'occupation' and other private information.
2) Selecting the required CEHRs: Chinese EHRs contain a large number of missing values. Therefore, those with unfilled personal information and less than 200 words will be removed.
3) Label entity information: We refer to a large number of annotation specifications [28, 29] to label entity information. The CEHR corpus contains 3 types of entities: symptom (Sym), medicine (Med), and abnormal inspection result (Abn). Sym: Symptom refers to the subjective feelings described by the patient or the objective facts observed by the outside world, such as dizziness. Med: Medicine refers to the name of the medicine used in the process of treatment, excluding dosage, method of administration, etc. such as aspirin. Abn: Abnormal inspection result refers to abnormal changes and abnormal examination results that occur in patients through examination procedures or as observed by doctors, such as lung marking increase.
After the above processing, we selected 8290 CEHRs as experimental data, and further splitted the CEHRs by 70, 10 and 20% as training, validation, and test sets, respectively. Table 2 shows the distribution of CEHRs, in descending order of data volume. The statistics of the entity information for our experiments are shown in Table 3.
Disease | Training set | Test set | Validation set |
CI | 700 | 200 | 100 |
VBI | 700 | 200 | 100 |
CAHD | 700 | 200 | 100 |
bronchitis | 700 | 200 | 100 |
degenerative spondylitis | 700 | 200 | 100 |
T2DM | 700 | 200 | 100 |
other diseases | 700 | 200 | 100 |
cholecystitis | 511 | 146 | 73 |
intestinal obstruction | 392 | 112 | 56 |
Disease | Avg number | Max number | Min number |
CI | 16.83 | 23 | 9 |
VBI | 13.71 | 20 | 8 |
CAHD | 15.16 | 27 | 7 |
bronchitis | 17.47 | 29 | 6 |
degenerative spondylitis | 12.18 | 14 | 5 |
T2DM | 16.38 | 32 | 8 |
other diseases | 16.08 | 33 | 6 |
cholecystitis | 18.94 | 30 | 9 |
intestinal obstruction | 16.16 | 27 | 8 |
The goal of this paper is to get the EHR features and use them for disease prediction. Using evaluation metrics for classification tasks to assess the quality of disease prediction, such as Accuracy, Precision, Recall and F1-score, these are defined as follows:
Accuracy=TP+TNTP+TN+FP+FN | (4.1) |
Recall=TPTP+FN | (4.2) |
Precision=TPTP+FP | (4.3) |
F1−score=2×Precision×RecallPrecision+Recall | (4.4) |
where TP indicates the number of positive samples that were predicted as positive, FP indicates the number of negative samples that were predicted as positive and FN indicates the number of positive samples that were predicted to be negative. TN indicates the number of negative samples that were predicted as negative.
In order to protect the patient privacy and data denoising, EHRs in this paper are preprocessed in various ways, such as privacy removing, data cleaning, entity labeling and disease standardizing. The version of the BERT model is BERT-base-Chinese, the main super-parameter is the size of hidden layer, which we set at 768, the Transformer blocks are 12, the number of attention heads is 12, and maximum input length is 512. In the convolutional module, the heights of the filters are 2, 3 and 4. During the training, we applied the learning rate of 5e-5 and the dropout rate of 0.5, and the batch size is 32.
We conducted experiments to compare the performance of our model with other disease prediction models.
SVM [5]: PKUSEG is a tool for word segmentation of Chinese EHRs. Then, the TF-IDF algorithm is used for extracting key information to obtain the representation of Chinese EHRs and then use SVM for disease prediction.
CNN [15]: CNN is used for obtaining features from Chinese EHRs, and then the probability of the patient's disease can be computed by sending features to fully connected layers.
BiLSTM: The model utilizes BiLSTM to extract features and feed them into fully connected activation layers for disease prediction.
RCNN [30]: The model utilizes RCNN to obtain the textual features of EHRs, and then sends them into fully connected layers and activation layers for disease prediction.
BERT [23]: The model uses the pre-trained model BERT to extract the features of Chinese EHRs for disease prediction tasks.
We compared overall performance of our proposed model with baseline models on a test set of CEHR datasets. Table 4 shows the experimental results of baseline models and our proposed model.
Method | Accuracy (%) | Precision (%) | Recall (%) | F1-score (%) |
SVM | 89.06 | 87.39 | 86.91 | 87.15 |
CNN | 89.55 | 88.44 | 87.55 | 87.99 |
BiLSTM | 89.39 | 88.53 | 86.97 | 87.74 |
RCNN | 89.76 | 89.04 | 87.51 | 88.27 |
BERT | 91.68 | 90.83 | 89.26 | 90.04 |
Our-model | 94.66 | 93.62 | 90.28 | 91.92 |
As shown in Table 4, we can see that our method is more effective than other methods, and the F1-score reaches 91.92%. The methods in Table 4 can be divided into traditional machine learning and deep learning methods. SVM, as a traditional machine learning model, cannot deeply learn the complex feature representation of EHRs. The BERT model only obtains the text information of the EHRs, while ignoring the entity and numerical information, which leads our model to improve the F1-score of the mainstream BERT model by 1.88%. It means that entity information is very important for both patient representation and disease prediction. The experimental results show that the multi-type data fusion model can fully obtain the features of Chinese EHRs, and it is effective and feasible to conduct disease prediction based on this model.
As shown in Table 5, 8 kinds of disease and other diseases are in descending order of data volume (the number of each disease is shown in Table 2). We list the F1-score corresponding to each disease. Our model has the highest F1-score in all 8 kinds of diseases and other diseases, indicating that we can effectively represent patients in these 8 kinds of diseases and other diseases. In terms of the diseases with fewer quantities of data, our model shows a significant performance improvement compared to other baseline models, such as cholecystitis, and intestinal obstruction, which improved by 2.61% to 2.5%. For VBI and degenerative spondylitis, our model has less improvement, 1.25 and 1.31%, respectively. The main reason is that due to the small number of entities in these two diseases, the model cannot learn the features of entity information well.
Disease | SVM | CNN | BiLSTM | RCNN | BERT | Our model |
CI | 82.26 | 82.91 | 82.94 | 83.38 | 86.41 | 88.78 |
VBI | 82.45 | 83.19 | 83.83 | 86.27 | 87.18 | 88.43 |
CAHD | 89.72 | 90.74 | 90.43 | 90.88 | 92.03 | 93.83 |
bronchitis | 89.79 | 89.87 | 90.17 | 90.59 | 93.48 | 95.15 |
degenerative spondylitis | 89.91 | 90.14 | 89.81 | 90.41 | 90.22 | 91.53 |
T2DM | 90.37 | 90.91 | 89.85 | 89.49 | 91.48 | 93.43 |
other diseases | 84.27 | 86.23 | 85.17 | 85.94 | 88.54 | 89.96 |
cholecystitis | 88.69 | 89.72 | 88.75 | 88.35 | 90.91 | 93.52 |
intestinal obstruction | 86.89 | 88.23 | 88.72 | 89.14 | 90.18 | 92.68 |
To verify the importance and role of different types of information on CEHR representation and to better understand the behavior of the proposed fusion model, we employ an ablation study and conduct extensive experiments on different models. T, E and N represent textual information, entity information and numerical information, respectively. As shown in Table 6, using T + E + N, the model achieved 91.92% F1-score in the test set, which is 1.09, 1.56 and 0.75% higher than of the models without textual, entity and numerical information, indicating that different types of information have an impact on disease prediction. Among them, entity information has the greatest impact on the model, which shows that entity information plays a key role in our model. By fusing multiple types of data, the performance of the model is improved, and at the same time, the model is more explanatory.
Method | Precision (%) | Recall (%) | F1-score (%) |
T + E + N | 93.62 | 90.28 | 91.92 |
T + E | 92.18 | 90.18 | 91.17 |
T + N | 91.69 | 89.06 | 90.36 |
E + N | 91.19 | 90.47 | 90.83 |
In order to choose a better entity acquisition method, we compared the CRF and BiLSTM-CRF models, and the results are shown in Figure 4.
We performed a comparison of the CRF and BiLSTM-CRF models for the identification of entity information in CEHRs. The Precisions for the two models are 88.57 and 89.24%. The Recalls for the two models are 87.54 and 88.76%. The F1-scores for the two models are 88.05 and 89.01%. We find that the BiLSTM-CRF model outperforms the CRF model. So, the BiLSTM-CRF model is selected as the entity extraction model in CEHRs.
Multi-head attention was adopted to fuse textual information and numerical information. As shown in Figure 5, when the number of heads of the multi-head attention is 8, the model achieves the best performance, with an F1-score of 91.92%. As the number of heads increases, the performance of the model gets better, but the number of heads should not be set too large, as otherwise F1-score will decrease. When the number of heads reached 12, the F1-score decreased by 0.31%, because excessive attention would introduce noise and reduce the performance of the model.
The purpose of this experiment is to study whether the EHRs representation adopted in the BERT model is better than the traditional Word2vec and Doc2vec in effect. As shown in Table 7, the effect of using BERT as text and entity embedding is better than Word2vec and Doc2vec embeddings, and the F1-score of the BERT model is 3.17% better than that of the Word2vec+Doc2vec combined model. The reason is that the training method of the BERT model based on character vectors can alleviate the problem of polysemy to a certain extent.
Model | Precision (%) | Recall (%) | F1-score (%) |
Word2vec+Doc2vec | 89.15 | 88.36 | 88.75 |
Our-model | 93.62 | 90.28 | 91.92 |
This paper proposes a disease prediction method based on a multi-type data fusion mechanism for EHRs. The model uses multi-head self-attention to fuse numerical features into textual information and enhance text representation. Using the TextCNN model to formulate entity representation, the representations of text and entities in it are mixed together to obtain the final representation of the EHR. This method solves the problems of unreasonable representation and difficulty in feature extraction when various data of EHRs exist. The experimental results show that the multi-type data fusion model can effectively learn the feature representation of EHRs and achieve disease prediction. In future work, we will try to incorporate more information, such as time series data, external knowledge bases, etc., to further improve the quality and efficiency of disease prediction.
We would like to thank the anonymous reviewers for their valuable comments. The Publication of the article is supported by the National Natural Science Foundation of China (No. 62163033), the Natural Science Foundation of Gansu Province (No. 21JR7RA781, No. 21JR7RA116), Lanzhou Talent Innovation and Entrepreneurship Project (No. 2021-RC-49) and Northwest Normal University Major Research Project Incubation Program (No. NWNU-LKZD2021-06).
The authors declare there is no conflict of interest.
[1] |
G. Litjens, T. Kooi, B. E. Bejnordi, A. A. A. Setio, F. Ciompi, M. Ghafoorian, et al., A survey on deep learning in medical image analysis, Med. Image Anal., 42 (2017), 60–88. https://doi.org/10.1016/j.media.2017.07.005 doi: 10.1016/j.media.2017.07.005
![]() |
[2] | J. Mullenbach, S. Wiegreffe, J. Duke, J. Sun, J. Eisenstein, Explainable prediction of medical codes from clinical text, preprint, arXiv: 1802.05695. |
[3] | L. Chen, X. Li, J. Han, MedRank: discovering influential medical treatments from literature by information network analysis, in Proceedings of the Twenty-Fourth Australasian Database Conference, 137 (2013), 3–12. |
[4] | W. Farhan, Z. Wang, Y. Huang, S. Wang, F. Wang, X. Jiang, A predictive model for medical events based on contextual embedding of temporal sequences, JMIR Med. Inf., 4 (2016), e5977. https://medinform.jmir.org/2016/4/e39 |
[5] |
W. Yu, T. Liu, R. Valdez, M. Gwinn, M. J. Khoury, Application of support vector machine modeling for prediction of common diseases: the case of diabetes and pre-diabetes, BMC Med. Inf. Decis. Making, 10 (2010), 1–7. https://doi.org/10.1186/1472-6947-10-16 doi: 10.1186/1472-6947-10-16
![]() |
[6] |
M. Khalilia, S. Chakraborty, M. Popescu, Predicting disease risks from highly imbalanced data using random forest, BMC Med. Inf. Decis. Making, 11 (2011), 1–13. https://doi.org/10.1186/1472-6947-11-51 doi: 10.1186/1472-6947-11-51
![]() |
[7] |
Z. Liang, J. Liu, A. Ou, H. Zhang, Z. Li, J. X. Huang, Deep generative learning for automated EHR diagnosis of traditional Chinese medicine, Comput. Methods Programs Biomed., 174 (2019), 17–23. https://doi.org/10.1016/j.cmpb.2018.05.008 doi: 10.1016/j.cmpb.2018.05.008
![]() |
[8] | B. Jin, C. Che, Z. Liu, S. Zhang, X. Yin, X. Wei, Predicting the risk of heart failure with EHR sequential data modeling, IEEE Access, 6 (2018), 9256–9261. https://ieeexplore.ieee.org/abstract/document/8245772 |
[9] | Z. Zhu, C. Yin, B. Qian, Y. Cheng, J. Wei, F. Wang, Measuring patient similarities via a deep architecture with medical concept embedding, in 2016 IEEE 16th International Conference on Data Mining (ICDM), (2016), 749–758. https://ieeexplore.ieee.org/abstract/document/7837899 |
[10] | J. W. Ha, A. Kim, D. Kim, J. Kim, J. W. Kim, J. J. Park, et al., Predicting high-risk prognosis from diagnostic histories of adult disease patients via deep recurrent neural networks, in 2017 IEEE International Conference on Big Data and Smart Computing (BigComp), (2017), 394–399. https://ieeexplore.ieee.org/abstract/document/7881742 |
[11] | J. Pestian, C. Brew, P. Matykiewicz, D. J. Hovermale, N. Johnson, K. B. Cohen, et al., A shared task involving multi-label classification of clinical free text, Biol., Transl., Clin. Lang. Process., 2007 (2007), 97–104. |
[12] | S. Palaniappan, R. Awang, Intelligent heart disease prediction system using data mining techniques, in IEEE/ACS International Conference on Computer Systems and Applications, (2008), 108–115. https://ieeexplore.ieee.org/abstract/document/4493524 |
[13] | N. Ananthakrishnan, T. Cai, G. Savova, S. C. Cheng, P. Chen, R. G. Perez, et al., Improving case definition of Crohn's disease and ulcerative colitis in electronic medical records using natural language processing: a novel informatics approach, Inflammatory Bowel Dis., 19 (2013), 1441–1420. https://ieeexplore.ieee.org/abstract/document/4493524 |
[14] |
S. Dreiseitl, L. Ohno-Machado, Logistic regression and artificial neural network classification models: a methodology review, J. Biomed. Inf., 35 (2002), 352–359. https://doi.org/10.1016/S1532-0464(03)00034-0 doi: 10.1016/S1532-0464(03)00034-0
![]() |
[15] |
Z. Yang, Y. Huang, Y. Jiang, Y. Sun, Y. J. Zhang, P. Luo, Clinical assistant diagnosis for electronic medical record based on convolutional neural network, Sci. Rep., 8 (2018), 1–9. https://doi.org/10.1038/s41598-018-24389-w doi: 10.1038/s41598-018-24389-w
![]() |
[16] | Y. An, K. Tang, J. Wang, Time-aware multi-type data fusion representation learning framework for risk prediction of cardiovascular diseases, in IEEE/ACM Transactions on Computational Biology and Bioinformatics 2021, 2021. https://ieeexplore.ieee.org/abstract/document/9563246 |
[17] |
T. Wang, P. Xuan, Z. Liu, T. Zhang, Assistant diagnosis with Chinese electronic medical records based on CNN and BiLSTM with phrase-level and word-level attentions, BMC Bioinf., 21 (2020), 1–16. https://doi.org/10.1186/s12859-020-03554-x doi: 10.1186/s12859-020-03554-x
![]() |
[18] |
Y. Du, H. Wang, W. Cui, H. Zhu, Y. Guo, F. A. Dharejo, et al., Foodborne disease risk prediction using multigraph structural long short-term memory networks: Algorithm design and validation study, JMIR Med. Inf., 9 (2021), e29433. https://doi.org/10.2196/29433 doi: 10.2196/29433
![]() |
[19] |
L. Rasmy, M. Nigo, B. S. Kannadath, Z. Xie, B. Mao, K. Patel, et al., Recurrent neural network models (CovRNN) for predicting outcomes of patients with COVID-19 on admission to hospital: model development and validation using electronic health record data, Lancet Digital Health, 4 (2022), E415–E425. https://doi.org/10.1016/S2589-7500(22)00049-8 doi: 10.1016/S2589-7500(22)00049-8
![]() |
[20] | Y. Sha, M. D. Wang, Interpretable predictions of clinical outcomes with an attention-based recurrent neural network, in Proceedings of the 8th ACM International Conference on Bioinformatics, Computational Biology, and Health Informatics 2017, (2017), 233–240. https://doi.org/10.1145/3107411.3107445 |
[21] | M. E. Peters, M. Neumann, M. lyyer, M. Gardner, C. Clark, K. Lee, et al., Deep contextualized word representations, in Proceedings of the 2018 Conference of the North American Chapter of the Association for Computational Linguistics: Human Language Technologies, 1 (2018), 2227–2237. https://doi.org/10.18653/v1/N18-1202 |
[22] | A. Radford, K. Narasimhan, T. Salimans, I. Sutskever, Improving language understanding by generative pre-training, OpenAI, 2018. |
[23] | J. Devlin, M. W. Chang, K. Lee, K. Toutanova, Bert: Pre-training of deep bidirectional transformers for language understanding, preprint, 2018, arXiv: 1810.04805. |
[24] | K. Zhang, C. Liu, X. Duan, L. Zhou, Y. Zhao, H. Zan, Bert with enhanced layer for assistant diagnosis based on Chinese obstetric EMRs, in 2019 International Conference on Asian Language Processing (IALP), (2019), 384–389. https://ieeexplore.ieee.org/abstract/document/9037721 |
[25] |
J. Lee, W. Yoon, S. Kim, D. Kim, S. Kim, C. H. So, et al., BioBERT: a pre-trained biomedical language representation model for biomedical text mining, Bioinformatics, 36 (2020), 1234–1240. https://doi.org/10.1093/bioinformatics/btz682 doi: 10.1093/bioinformatics/btz682
![]() |
[26] | C. Mugisha, I. Paik, Pneumonia outcome prediction using structured and unstructured data from EHR, in 2020 IEEE International Conference on Bioinformatics and Biomedicine (BIBM), (2020), 2640–2646. https://ieeexplore.ieee.org/abstract/document/9312987 |
[27] | T. Mikolov, K. Chen, G. Corrado, J. Dean, Efficient estimation of word representations in vector space, 2020, preprint, arXiv: 1301.3781 2013. |
[28] |
A. Stubbs, Ö. Uzuner, Annotating risk factors for heart disease in clinical narratives for diabetic patients, J. Biomed. Inf., 58 (2015), S78–S91. https://doi.org/10.1016/j.jbi.2015.05.009 doi: 10.1016/j.jbi.2015.05.009
![]() |
[29] |
Z. Zhang, L. Zhu, P. Yu, Multi-level representation learning for Chinese medical entity recognition: Model development and validation, JMIR Med. Inf., 8 (2020), e17637. https://doi.org/10.2196/17637 doi: 10.2196/17637
![]() |
[30] | M. Usama, B. Ahmad, J. Wan, M. S. Hossain, M. F. Alhamid, M. A. Hossain, Deep feature learning for disease risk assessment based on convolutional neural network with intra-layer recurrent connection by using hospital big data, IEEE Access, 6 (2018), 67927–67939. https://ieeexplore.ieee.org/abstract/document/8519726 |
1. | Yong Li, Li Feng, Patient multi-relational graph structure learning for diabetes clinical assistant diagnosis, 2023, 20, 1551-0018, 8428, 10.3934/mbe.2023369 | |
2. | Yuhang Chen, Di Yang, Peng Wang, Cheng Gao, 2023, E-bidNER: A Two-stage Enhanced Named Entity Recognition for Chinese Bid Announcements, 979-8-3503-5773-8, 749, 10.1109/IAECST60924.2023.10502612 | |
3. | Richard J. Woodman, Arduino A. Mangoni, A comprehensive review of machine learning algorithms and their application in geriatric medicine: present and future, 2023, 35, 1720-8319, 2363, 10.1007/s40520-023-02552-2 | |
4. | Yuanyuan Cai, Hao Liang, Qingchuan Zhang, Haitao Xiong, Fei Tong, Food safety in health: a model of extraction for food contaminants, 2023, 20, 1551-0018, 11155, 10.3934/mbe.2023494 | |
5. | Ariel Soares Teles, Ivan Rodrigues de Moura, Francisco Silva, Angus Roberts, Daniel Stahl, EHR-based prediction modelling meets multimodal deep learning: A systematic review of structured and textual data fusion methods, 2025, 118, 15662535, 102981, 10.1016/j.inffus.2025.102981 |
Initial description | Gender:Female; Age:67; Marital Status:Married; Family History:None; |
T:36.8 ℃; P:62 beats/min; R:18 beats/min; Bp:140/90 mmHg | |
Numerical information | {(0, 67, 1, 0, 36.8, 62, 18, 140, 90)} |
Disease | Training set | Test set | Validation set |
CI | 700 | 200 | 100 |
VBI | 700 | 200 | 100 |
CAHD | 700 | 200 | 100 |
bronchitis | 700 | 200 | 100 |
degenerative spondylitis | 700 | 200 | 100 |
T2DM | 700 | 200 | 100 |
other diseases | 700 | 200 | 100 |
cholecystitis | 511 | 146 | 73 |
intestinal obstruction | 392 | 112 | 56 |
Disease | Avg number | Max number | Min number |
CI | 16.83 | 23 | 9 |
VBI | 13.71 | 20 | 8 |
CAHD | 15.16 | 27 | 7 |
bronchitis | 17.47 | 29 | 6 |
degenerative spondylitis | 12.18 | 14 | 5 |
T2DM | 16.38 | 32 | 8 |
other diseases | 16.08 | 33 | 6 |
cholecystitis | 18.94 | 30 | 9 |
intestinal obstruction | 16.16 | 27 | 8 |
Method | Accuracy (%) | Precision (%) | Recall (%) | F1-score (%) |
SVM | 89.06 | 87.39 | 86.91 | 87.15 |
CNN | 89.55 | 88.44 | 87.55 | 87.99 |
BiLSTM | 89.39 | 88.53 | 86.97 | 87.74 |
RCNN | 89.76 | 89.04 | 87.51 | 88.27 |
BERT | 91.68 | 90.83 | 89.26 | 90.04 |
Our-model | 94.66 | 93.62 | 90.28 | 91.92 |
Disease | SVM | CNN | BiLSTM | RCNN | BERT | Our model |
CI | 82.26 | 82.91 | 82.94 | 83.38 | 86.41 | 88.78 |
VBI | 82.45 | 83.19 | 83.83 | 86.27 | 87.18 | 88.43 |
CAHD | 89.72 | 90.74 | 90.43 | 90.88 | 92.03 | 93.83 |
bronchitis | 89.79 | 89.87 | 90.17 | 90.59 | 93.48 | 95.15 |
degenerative spondylitis | 89.91 | 90.14 | 89.81 | 90.41 | 90.22 | 91.53 |
T2DM | 90.37 | 90.91 | 89.85 | 89.49 | 91.48 | 93.43 |
other diseases | 84.27 | 86.23 | 85.17 | 85.94 | 88.54 | 89.96 |
cholecystitis | 88.69 | 89.72 | 88.75 | 88.35 | 90.91 | 93.52 |
intestinal obstruction | 86.89 | 88.23 | 88.72 | 89.14 | 90.18 | 92.68 |
Method | Precision (%) | Recall (%) | F1-score (%) |
T + E + N | 93.62 | 90.28 | 91.92 |
T + E | 92.18 | 90.18 | 91.17 |
T + N | 91.69 | 89.06 | 90.36 |
E + N | 91.19 | 90.47 | 90.83 |
Model | Precision (%) | Recall (%) | F1-score (%) |
Word2vec+Doc2vec | 89.15 | 88.36 | 88.75 |
Our-model | 93.62 | 90.28 | 91.92 |
Initial description | Gender:Female; Age:67; Marital Status:Married; Family History:None; |
T:36.8 ℃; P:62 beats/min; R:18 beats/min; Bp:140/90 mmHg | |
Numerical information | {(0, 67, 1, 0, 36.8, 62, 18, 140, 90)} |
Disease | Training set | Test set | Validation set |
CI | 700 | 200 | 100 |
VBI | 700 | 200 | 100 |
CAHD | 700 | 200 | 100 |
bronchitis | 700 | 200 | 100 |
degenerative spondylitis | 700 | 200 | 100 |
T2DM | 700 | 200 | 100 |
other diseases | 700 | 200 | 100 |
cholecystitis | 511 | 146 | 73 |
intestinal obstruction | 392 | 112 | 56 |
Disease | Avg number | Max number | Min number |
CI | 16.83 | 23 | 9 |
VBI | 13.71 | 20 | 8 |
CAHD | 15.16 | 27 | 7 |
bronchitis | 17.47 | 29 | 6 |
degenerative spondylitis | 12.18 | 14 | 5 |
T2DM | 16.38 | 32 | 8 |
other diseases | 16.08 | 33 | 6 |
cholecystitis | 18.94 | 30 | 9 |
intestinal obstruction | 16.16 | 27 | 8 |
Method | Accuracy (%) | Precision (%) | Recall (%) | F1-score (%) |
SVM | 89.06 | 87.39 | 86.91 | 87.15 |
CNN | 89.55 | 88.44 | 87.55 | 87.99 |
BiLSTM | 89.39 | 88.53 | 86.97 | 87.74 |
RCNN | 89.76 | 89.04 | 87.51 | 88.27 |
BERT | 91.68 | 90.83 | 89.26 | 90.04 |
Our-model | 94.66 | 93.62 | 90.28 | 91.92 |
Disease | SVM | CNN | BiLSTM | RCNN | BERT | Our model |
CI | 82.26 | 82.91 | 82.94 | 83.38 | 86.41 | 88.78 |
VBI | 82.45 | 83.19 | 83.83 | 86.27 | 87.18 | 88.43 |
CAHD | 89.72 | 90.74 | 90.43 | 90.88 | 92.03 | 93.83 |
bronchitis | 89.79 | 89.87 | 90.17 | 90.59 | 93.48 | 95.15 |
degenerative spondylitis | 89.91 | 90.14 | 89.81 | 90.41 | 90.22 | 91.53 |
T2DM | 90.37 | 90.91 | 89.85 | 89.49 | 91.48 | 93.43 |
other diseases | 84.27 | 86.23 | 85.17 | 85.94 | 88.54 | 89.96 |
cholecystitis | 88.69 | 89.72 | 88.75 | 88.35 | 90.91 | 93.52 |
intestinal obstruction | 86.89 | 88.23 | 88.72 | 89.14 | 90.18 | 92.68 |
Method | Precision (%) | Recall (%) | F1-score (%) |
T + E + N | 93.62 | 90.28 | 91.92 |
T + E | 92.18 | 90.18 | 91.17 |
T + N | 91.69 | 89.06 | 90.36 |
E + N | 91.19 | 90.47 | 90.83 |
Model | Precision (%) | Recall (%) | F1-score (%) |
Word2vec+Doc2vec | 89.15 | 88.36 | 88.75 |
Our-model | 93.62 | 90.28 | 91.92 |