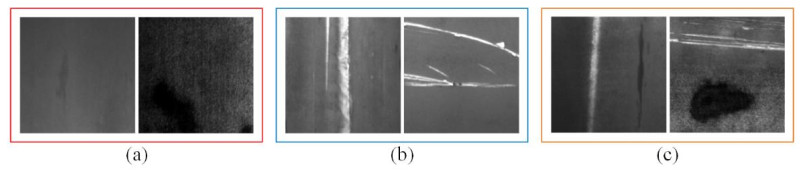
The automatic surface defect detection system supports the real-time surface defect detection by reducing the information and high-lighting the critical defect regions for high level image under-standing. However, the defects exhibit low contrast, different textures and geometric structures, and several defects making the surface defect detection more difficult. In this paper, a pixel-wise detection framework based on convolutional neural network (CNN) for strip steel surface defect detection is proposed. First we extract the salient features by a pre-trained backbone network. Secondly, contextual weighting module, with different convolutional kernels, is used to extract multi-scale context features to achieve overall defect perception. Finally, the cross integrate is employed to make the full use of these context information and decoded the information to realize feature information complementation. The experimental results of this study demonstrate that the proposed method outperforms against the previous state-of-the-art methods on strip steel surface defect dataset (MAE: 0.0396; Fβ: 0.8485).
Citation: Guozhen Dong. A pixel-wise framework based on convolutional neural network for surface defect detection[J]. Mathematical Biosciences and Engineering, 2022, 19(9): 8786-8803. doi: 10.3934/mbe.2022408
[1] | Yong Tian, Tian Zhang, Qingchao Zhang, Yong Li, Zhaodong Wang . Feature fusion–based preprocessing for steel plate surface defect recognition. Mathematical Biosciences and Engineering, 2020, 17(5): 5672-5685. doi: 10.3934/mbe.2020305 |
[2] | Fang Luo, Yuan Cui, Xu Wang, Zhiliang Zhang, Yong Liao . Adaptive rotation attention network for accurate defect detection on magnetic tile surface. Mathematical Biosciences and Engineering, 2023, 20(9): 17554-17568. doi: 10.3934/mbe.2023779 |
[3] | Xian Fu, Xiao Yang, Ningning Zhang, RuoGu Zhang, Zhuzhu Zhang, Aoqun Jin, Ruiwen Ye, Huiling Zhang . Bearing surface defect detection based on improved convolutional neural network. Mathematical Biosciences and Engineering, 2023, 20(7): 12341-12359. doi: 10.3934/mbe.2023549 |
[4] | Lili Wang, Chunhe Song, Guangxi Wan, Shijie Cui . A surface defect detection method for steel pipe based on improved YOLO. Mathematical Biosciences and Engineering, 2024, 21(2): 3016-3036. doi: 10.3934/mbe.2024134 |
[5] | Yinghong Xie, Biao Yin, Xiaowei Han, Yan Hao . Improved YOLOv7-based steel surface defect detection algorithm. Mathematical Biosciences and Engineering, 2024, 21(1): 346-368. doi: 10.3934/mbe.2024016 |
[6] | Liyong Ma, Wei Xie, Haibin Huang . Convolutional neural network based obstacle detection for unmanned surface vehicle. Mathematical Biosciences and Engineering, 2020, 17(1): 845-861. doi: 10.3934/mbe.2020045 |
[7] | Jiaming Ding, Peigang Jiao, Kangning Li, Weibo Du . Road surface crack detection based on improved YOLOv5s. Mathematical Biosciences and Engineering, 2024, 21(3): 4269-4285. doi: 10.3934/mbe.2024188 |
[8] | Zhangjie Wu, Minming Gu . A novel attention-guided ECA-CNN architecture for sEMG-based gait classification. Mathematical Biosciences and Engineering, 2023, 20(4): 7140-7153. doi: 10.3934/mbe.2023308 |
[9] | Zhongliang Zhang, Yao Fu, Huiling Huang, Feng Rao, Jun Han . Lightweight network study of leather defect segmentation with Kronecker product multipath decoding. Mathematical Biosciences and Engineering, 2022, 19(12): 13782-13798. doi: 10.3934/mbe.2022642 |
[10] | Yalong Yang, Zhen Niu, Liangliang Su, Wenjing Xu, Yuanhang Wang . Multi-scale feature fusion for pavement crack detection based on Transformer. Mathematical Biosciences and Engineering, 2023, 20(8): 14920-14937. doi: 10.3934/mbe.2023668 |
The automatic surface defect detection system supports the real-time surface defect detection by reducing the information and high-lighting the critical defect regions for high level image under-standing. However, the defects exhibit low contrast, different textures and geometric structures, and several defects making the surface defect detection more difficult. In this paper, a pixel-wise detection framework based on convolutional neural network (CNN) for strip steel surface defect detection is proposed. First we extract the salient features by a pre-trained backbone network. Secondly, contextual weighting module, with different convolutional kernels, is used to extract multi-scale context features to achieve overall defect perception. Finally, the cross integrate is employed to make the full use of these context information and decoded the information to realize feature information complementation. The experimental results of this study demonstrate that the proposed method outperforms against the previous state-of-the-art methods on strip steel surface defect dataset (MAE: 0.0396; Fβ: 0.8485).
Strip steel is widely used in industrial production, including automobile, electromechanical, aerospace, ship and so on. Fundamentally speaking, there are inherent problems in the quality of strip steel, which will not only affect the beauty and comfort of products, but also these areas are usually the starting point of physical damage or chemical corrosion, which also has an adverse impact on the quality and service performance. The main defects of strip steel surface products are poor appearance, quality and use safety. Therefore, effective, rapid and accurate detection of surface defects is the primary problem in the iron and steel industry. At present, the whole manufacturing industry pays more and more attention to the surface defect detection technology, so as to find and effectively control the product quality in time, infer the causes of defects according to the detection results, and improve the production process, so as to reduce or eliminate the occurrence of defects.
The early detection methods of surface defects are mainly based on the manual inspection techniques, which have low efficiency and high cost. Recently, automatic defect inspection (ADI) technology methods based on machine learning have developed rapidly. ADI method not only has higher detection efficiency and accuracy, but also significantly reduces the human and financial resources. Despite this, it is still a very challenging task for ADI to identify the intrinsic and diverse defects in steel. The varieties of strip steel surface defects are shown in Figure 1. The surface defects of strip steel mainly have the following three characteristics, which make it difficult for surface detect detection.
1) Low contrast quality. Surface defect images are usually captured by CDD cameras. However, the environment for the image acquisition of surface defects are affected by light and dust, which resulting in low contrast between background and defects, as shown in Figure 1(a). This case increases the difficulty in defect detection.
2) Different textural and geometric structures. Generally, the defect images collected from different materials exhibit diverse textures. The Figure 1(b) depicts differences in texture features in same type of defects in different materials, where the boundary of defects is fuzzy and irregular. These factors also increase the difficulty in surface defect detection.
3) Diversity of defects. Surface defects always include many categories like inclusion, patches and scratches in which, some features are obvious while others are ambiguous. Further, the defects of the same category invariably show significant differences in appearance, while some defects of different categories have great similarities in appearance, as shown in Figure 1(c). These factors further improve the difficulty in detection process.
To address the above challenges, local binary pattern was applied for surface defects detection [1,2]. Djukic et al. [3] distinguished real defects from random noise pixels by dynamic threshold processing. An entity sparsity pursuit approach was also proposed for surface defects inspection [4]. Neogi et al. [5] suggested a global adaptive percentile thresholding of gradient images, which segment the defect regions and retain the characteristics of the defect without considering the size of the defect. In [6], a Gabor filter combination is proposed to detect the tiny holes on steel slabs. Li et al. [7] proposed an unsupervised approach based on a small number of flawless samples to detect and locate defects in random colour texture. On the other hand, Cohen et al. [8] connected the Markov with Gaussian distribution, and proposed Gaussian Markov Random Field to model the texture image of a non-defective fabric texture. However, all these methods are designed to identify defect detection by designing some artificial features, which lack generality.
Recently, especially CNN based methods are outstanding in the field of machine vision. These methods can automatically extract target features, find the internal feature relationship and law in the sample through iterative optimization, adaptively learn image features and complete object detection tasks, and solve the shortcomings of low efficiency and low detection accuracy of manual design features. A semi-supervised approach based on CNN was used to classify the strip steel surface defect [9]. Since the industrial defect images are difficult to collect, Natarajan et al. [10] adopted transfer learning to extract multi-level features and then input these features into SVM classifiers to avoid the over fitting caused by the small samples. However, the accuracy of these methods needs further improvement.
In this work, a pixel-wise detection framework based on CNN for strip steel surface defect detection is proposed to obtain multi-scale context information from high-level features by different sizes of convolution kernels. A cross integration is adopted to realize the effective utilization of these context information and to decode the information, which realizes the feature information complementation. The output of the framework is accurate pixel-wise classification and location. The main contributions of this study are:
● A pixel-wise detection framework based on CNN for strip steel surface defect detection is introduced. The output of the detection framework is the pixel-wise binary saliency maps of defect regions, which can effectively evaluate the quality of strip steel products.
● A contextual weighting module is proposed, which uses convolutional kernels with different size to obtain multi-scale context feature information from the convolution layers to achieve overall perception of the defect.
● In the decoder module, the cross integration is used to integrate the context information and previous decoded information into the current decoding block to realize feature information complementation.
● The proposed method is tested on the NEU-strip steel surface defect dataset, and the experimental results prove the effectiveness of the proposed method.
In this section, two kinds of detection methods for surface defect will be introduced, including: i) traditional approaches; ii) deep learning-based approaches.
The traditional methods for surface defect detection mainly include three categories: the statistical-based approaches, the filter-based approaches and the model-based approaches.
These methods use random phenomenon to analysis the distribution of random variables from the perspective of statistics, so as to realize the description of the image texture. Neogi et al. [5] proposed a global adaptive percentile thresholding of gradient images, which segment the defect regions and retain the characteristics of the defect without considering the size of the defect. Win et al. [11] proposed two thresholding methods namely, contrast-adjusted Otsu's method and contrast-adjusted median-based Otsu's method for automated defect detection system. Ricci et al. [12] used canny operator to detect the defect edges. Hu et al. [13] used Fourier shape descriptors for description of outline features in steel surface defects. Zhao et al. [14] proposed a two-level labelling technique based on super pixels. This method clustered pixels into super pixels and then the super pixels into sub-regions. Wang et al. [15] extracted and fused features of co-occurrence matrix and the histogram of oriented gradient to describe the local and the global texture characteristics, respectively. Chu et al. [16] proposed a smoothed local binary patterns by applying weight on the local neighbourhood. Fekri-Ershad et al. [17] applied a new noise-resistant and multi-resolution version of the LBP to extract jointly the colour and texture features jointly. Song et al. [1] proposed an adjacent evaluation completed local binary patterns against noise for defect inspection. Zhang et al. [18] used gray level co-occurrence matrix (GLCM) and HU invariant moments for feature extraction, and then applied adaptive genetic algorithm for feature selection.
The principle of this method was to transform the original image in frequency domain, and then use the corresponding filter to consider the image and to remove the features with low noise and correlation, so that the algorithm can extract more valuable information. Ai et al. [19] adopted kernel locality preserving projections and curvelet transform extract feature for the surface longitudinal cracks detection of the slabs. In [6,20], a Gabor filter combination is proposed to detect the tiny holed on steel slabs. Other method [On the other hand, Choi et al. [21] adopted two Gabor filters to detect the seam cracks on the steel plates, which have high detection performance and can effectively reduce noise. Wu et al. [22] used modular maximum of inter scale correlation of wavelet coefficient to determine the positions of the defects, and then used the prior knowledge about the characteristics of the surface defect defects for their classification. Öztürk et al. [23] proposed novel BiasFeed cellular neural network model for glass defect inspection. Li et al. [24] proposed a second-order derivative and morphology operations, the row-by-row adaptive thresholding, and 2-D wavelet transform to process the images showing different defects of the castings. Liu et al. [25] applied a non-subsampled shearlet transform and the kernel locality preserving projection to the surface defect detection. Akdemir et al. [26] adopted wavelet transforms to glass surface defects detection.
These methods are based on the construction model of the image, and uses the statistics of model parameters as texture features. Different textures are expressed as different values of model parameters under some assumptions. In [7], an unsupervised approach based on a small number of flawless samples was used to detect and locate the defects in random color texture. Cohen et al. [27] connected Markov with Gaussian distribution, and proposed Gaussian Markov Random Field to model the texture image of a non-defective fabric texture. Song et al. [28] proposed a saliency propagation algorithm based on multiple constraints and improved texture features (MCITF) for surface defect detection.
Recently, deep learning based on CNN approaches have achieved outperformed in the field of machine vision tasks. Many scholars have solved the problem of industrial defect detection by deep learning. In [9], a semi-supervised approach based on CNN was used to classify the strip steel surface defect. Since the industrial defect images are difficult to collect, Natarajan et al. [10] adopted transfer learning to extract the multi-level features and then input these features into SVM classifiers to avoid the over fitting caused by small samples. Masci et al. [29] proposed a Multi-scale pyramidal pooling network for generic steel defect classification. He et al. [30] proposed a multi-group convolutional neural network (MG-CNN) to inspect the defects of the steel surface. In [31], an end-to-end detection framework was proposed, which integrated multi-level features to complete the detection of the strip steel surface defect. The output of the network located the defect areas through some dense bounding boxes and gave the category name to these defects. Kou et al. [32] developed an end-to-end defect detection model based on YOLO-V3 for the surface defect detection on strip steel. In [33], a pre-trained deep learning network is used to extract multi-scale features from raw image patches to achieve image classification and defect segmentation. In [34], a multi-scale feature-clustering-based fully convolutional was proposed for the texture surface defect detection. Neven et al. [35] proposed a multi-branch U-Net for steel surface defect type and severity segmentation. Zhou et al. [36] proposed edge-aware multi-level interactive network for salient object detection of strip steel surface defects. Song et al. [37] adopted encoder-decoder residual network for salient object detection of strip steel surface defects. Dong et al. [38] proposed a pyramid feature fusion and global context attention network for automated surface defect segmentation. Although these methods achieved outstanding performance in the defects detection, they still need to be improved especially, in the feature extraction and utilization. Unlike previous studies, this paper proposes a pixel-wise detection framework based on CNN for strip steel surface defect detection.
The surface defect inception is formulated in this work as a pixel-wise segmentation task. Given a defect image, the proposed framework outputs a binary map, the defect area is represented by "1", while the non-defect area is represented by "0". The architecture of the framework mainly includes three parts: an encoder, the contextual weighting module and a decoder as shown in Figure 2.
Given a defect image, the framework first extracts the multi-level features from fine, shallow layers (enc1) to coarse, deep layers (enc5) by a pre-trained VGG-16 [39] network which is called an encoder module. The encoder module is composed of convolution layers and max pooling layers. In order to retain the spatial information of each pixel, the fully connection layers of VGG-16 network is removed. Subsequently, a contextual weighting module is adopted to obtain multi-scale contextual information from the high-level features to keep the shape and size in variance of the final features. In the encoder, the features extracted from enc3, enc4 and enc5 are considered as high-level features. In the decoder, the output of each con-textual weighting network is fused to the input of the same decoder in a feedback fashion. The final output of the decoder is a defect binary saliency map.
The encoder is used to extract multi-level features of the defect images, which is built on the pre-trained VGG-16 network. The encoder module mainly consists of 5 convolution layers and 4 max pooling layers. The details of the encoder module, i.e. blocks encx where, x = 1, …, 5 are listed in Table 1. In the encoder, the convolutional layer performs sliding on the input local areas through a series of convolutional kernels to obtain the features of the input image, followed by ReLU and BN. Let T={(Xn,Zn),n=1,...,N} represents training data, where Xn={xni,i=1,⋅⋅⋅,|Xn|} denotes the input image and Zn={zni,i=1,⋅⋅⋅,|Zn|} is the corresponding ground truth for Xn. The convolution of Xn is as follows:
C=σ(Wxni+b) | (1) |
Stage | Template | ||
enc1 | conv 3 × 3, stride = 1, D = 64, BN + ReLU | max_pooling 2 × 2, stride = 2 | ×2 |
enc2 | conv 3 × 3, stride = 1, D = 128, BN + ReLU | max_pooling 2 × 2, stride = 2 | ×2 |
enc3 | conv 3 × 3, stride = 1, D = 256, BN + ReLU | max_pooling 2 × 2, stride = 2 | ×2 |
enc4 | conv 3 × 3, stride = 1, D = 512, BN + ReLU | max_pooling 2 × 2, stride = 2 | ×2 |
enc5 | conv 3 × 3, stride = 1, D = 512, BN + ReLU | max_pooling 2 × 2, stride = 2 | ×2 |
where, W denotes weights, b refers bias, and σ represents the ReLU activation. By sliding the convolution kernels to obtain the feature sets. The pooling layers adopt 2 × 2 pool filter to down-scale the input feature maps, which is to change the spatial dimension and reduce the amount of calculation. The output of pooling layer is given below:
ˆC=pool(C) | (2) |
where pool denotes the max pooling with 2×2 pool filter and stride 2. The encoder finally generates five resolution feature maps F = {f1, f2, …, f5}, and f1 denotes the enc1 features and so on.
The fusion of convolutional features obtained from different stages is a common mechanism in most detection methods, because these features not only contain low-level visual information, but also include high-level abstract information. The earlier methods [40,41] combine these features directly from bottom to top. However, this simple combination may induce some bad features in the images to be integrated into the final prediction. To address this issue, a contextual weighting module, inspired by [42], is proposed in Figure 3. The CWM applies different convolution kernels to extract multi-scale contextual information from high-level features, which provides entire description for interpretation of the whole scene especially, multi-scale and multi shape objects. In the CWM, the features f3, f4 and f5 are used as high-level features. CWM used four stacked convolutional kernels (1×1, 3×3, 5×5, 7×7) to obtain multi-scale contextual information from the high-level features, and each kernel generates a feature map with the size of high-level features. For high-level feature f3, the output multi-scale contextual information can be denoted by F3:
M13=BN(σ(W1×1f3+b)) | (3) |
M33=BN(σ(W3×3f3+b)) | (4) |
M53=BN(σ(W5×5f3+b)) | (5) |
M73=BN(σ(W7×7f3+b)) | (6) |
Where BN denotes Batch Normalization, σ is nonlinear activation function ReLU. Wi×i denote the i × i convolutional kernel. The size of each generated features Mi3 (i = 1, 3, 5, 7) is the same as that of f3, and the number of channels is 32.
Then these feature maps are fused by concatenation. After that, 1×1 convolutional kernels are used to resize the channel of concatenated features to reduce the computation of the contextual weighting. The output saliency map G3 is formulated as:
G3=BN(σ(W1×1CAT(M13+M33+M53+M73)+b)) | (7) |
Where BN denotes Batch Normalization, σ is nonlinear activation function ReLU, CAT denotes concatenation. W1×1 is 1×1 convolutional kernel with 128 channels. The number of channels of G3 is 128.
For high-level feature f4 and f5, the model generates G4 and G5 in the same way as G3.
In this section, a novel decoder module is proposed, which includes 4 blocks (dec2, dec3, dec4, dec5), as shown in Figure 2. The dec3 and dec4 are fusion decoders, which are composed of the former one or two decoders and the output from contextual weighting module connected with enc3 and enc4, respectively. To enable effective fusion of these features, which must ensure that they have the same dimensionality. Firstly, a series of 3×3×D convolution kernels are applied to reduce channel dimension of these fused feature maps, where D is 32. Then a bilinear interpolation is applied to upsample low-resolution features to the target spatial resolution of the features that will be fused. Subsequently, these feature maps are fused by element-wise concatenation, as shown in Figure 4. The output decx is defined as follows:
decx={up×2(decx+1)x=2CAT(up×4(ϕ(ch(decx+2;θ))),up×2(ϕ(ch(decx+1;θ))),ϕ(ch(Gx;θ)))x=3CAT(up×2(ϕ(ch(decx+1;θ))),ϕ(ch(Gx;θ)))x=4 | (8) |
The final prediction Yp is formulated as:
Yp=up×2(W1×1∗dec2+b) | (9) |
where, CAT refers concatenation, up denotes upsample, ϕ represents the ReLU activation and ch is 3×3 convolution.
Loss function is the most basic and key factor in machine learning, which is used to measure the quality of model prediction. In this paper, three losses are applied to optimize the model. The final loss is defined as:
L=lBCE+lIoU+lSSIM | (10) |
where lBCE, lIoU and lSSIM represent the BCE loss, IoU loss and SSIM loss, respectively.
The BCE [43] loss is applied to compute the similarity between the prediction and ground truth, which is defined as:
lBCE=−∑TlogP−∑(1−T)log(1−P) | (11) |
where T ∈[0, 1] denotes the ground truth, and P∈[0, 1] is the predicted probability.
The IOU [44] loss is used to measure the repeatability between the prediction and the ground truth, which is defined as:
lIoU=1−∑TP∑[P+T−PT] | (12) |
where T ∈[0, 1] denotes the ground truth, and P∈[0, 1] is the predicted probability.
The SSIM [45] are originally applied to measure the structural similarity of two images. Let p={pi=1,⋅⋅⋅,N2} and t={ti=1,⋅⋅⋅,N2} represent the pixel values of two corresponding patches (size: N × N) cropped from the prediction P and ground truth T, respectively. The SSIM is computed as:
lIoU=1−(2vpvt+C1)(2σpt+C2)(v2p+v2t+C1)(σ2p+σ2t+C2) | (13) |
where vp and vt are the mean of p and t, respectively. σ2p and σ2t are the variance of p and t, respectively. σpt is their covariance. C1 and C2 are small constants that are applied to avoid dividing by zero.
This section mainly consists of six experimental parts: the details of implementation, the dataset and the evaluation metrics, the performance of the proposed method and other previous methods, followed by the ablation study and analysis of failure cases.
The proposed method is implemented based on TensorFlow [46] framework. The weights of new convolution layers in the framework are initialized with standard deviation 0.01 and biases are initialized to 0. The weights of backbone network are initialized using pre-trained ImageNet [47] network. The momentum and weight decay are set to 0.9 and 0.0005, respectively. The initial learning rate is set to 5e-5, which decreased by 10 after 10 epochs. The framework is trained for 300 epochs in total.
In the experiment of this study, three kinds of surface defect of strip steel [1] are selected, including Scratches, Patches, and Inclusion, as shown in Figure 5. All categories of defects are considered as detection targets. In the dataset, the training set includes 3630 defect samples, and the test set includes 792 defect samples. All the samples are resized to 256×256 during in the process of training network.
To evaluate the proposed framework, four metrics are used along with other previous state-of-the-art approaches, namely precision-recall (PR) curves, F-measure score and mean absolute error (MAE). The PR curve demonstrates the average recall and precision and of saliency maps at different thresholds, formulated as follows:
Recall=TPTP+FN | (14) |
Precision=TPTP+FP | (15) |
where FN, FP and TP indicate correctly the number judge of false negative pixels, false positive and true positive, respectively. F-measure, refers Fβ and is computed by weighted harmonic mean of recall and precision under nonnegative weight β, which defined as:
Fβ=(1+β2)Precision×Recallβ2×Precision+Recall | (16) |
the β2 = 0.3 is used in other methods.
MAE [48] is used to calculate the mean absolute error between the ground truth and the prediction. First, the prediction and the ground truth are binarized. Then, the MAE score is computed by:
MAE=1W×HW∑x=1H∑y=1|P(x,y)−S(x,y)| | (17) |
where P and S refer the prediction and the ground truth, respectively, while H and W are the height and width of images, respectively.
In this subsection, the proposed method is compared with 10 previous state-of-the-art methods, including BSCA [49], FT [50], MIL [51], RC [52], SMD [53], FCN [40], UNet [41], DN [54], DHSNet [55] and DSS [56], all the compared are pixel-wised method. For the sake of comparison, the same evaluation metrics and code are used to evaluate the output prediction maps.
Qualitative comparison results are shown in Figure 5, the proposed method can accurately detect defects and highlight them evenly in various challenging cases, i) low contrast quality between the defect region and background (e.g., row 1 and 3); ii) different textural and geometric structures (e.g., row 4 and 5); iii) diversity of defects (e.g., row 6, 7 and 8). For low contrast quality: some methods are missing or detecting a rough defect area which cannot express the defects vividly. For different textural and geometric structures, the defect region detected by methods are with little noise and not obvious. For diversity of defects, some methods cannot detect all categories of defects. The detection effect of deep learning-based methods is better than that of the traditional methods. However, for some minor defects, FCN, UNet, DN, DSS and DHSNet are either missing or incomplete detection areas. Instead, the proposed approach not only can distinguish the defect area and background effectively under low contrast, but also locate and detect the defects in different positions, scales and shapes accurately.
The advantages of the proposed method are shown in Figure 6. The method achieves outstanding performance among all the compared methods on strip steel surface defect dataset in terms of all evaluation metrics. It further improves the P-R curve and F-measure, and reduces MAE significantly. As listed in Table 2, the proposed method outperforms the competitive methods in Fβ and MAE. Compared with the traditional methods, Fβ is improved 36.12%, and decreased by 12.08% in MAE. Compared with deep learning method, the Fβ is improved by 0.38%, and decreased by 0.02% in MAE. The comparison of the above qualitative and quantitative analysis further proves the effectiveness of this method.
Method | BSCA[49] | FT[50] | MIL[51] | RC[52] | SMD[53] | FCN[40] | UNet[41] | DN[54] | DHSNet[55] | DSS[56] | My |
MAE↓ | 0.2462 | 0157. 7 | 0.1764 | 0.1228 | 0.1913 | 0.0604 | 0.0643 | 0.0481 | 0.0399 | 0.0371 | 0.0369 |
Fβ | 0.3404 | 0.4462 | 0.4873 | 0.3719 | 0.4665 | 0.6788 | 0.6783 | 0.7272 | 0.8447 | 0.8051 | 0.8485 |
In this work, the ablation study on the proposed CWN to verify the effectiveness of CWN. First, the CWN is removed, and directly combined the feature maps output from the encoder with the decoded feature maps through dense short connections, and output the optimal model after training the overall network. The ablation study further add the CWN module into the proposed method, and output the trained model after the same training. Finally, the two trained models are tested separately and output saliency prediction maps. As listed in Table 3, the contextual weighting module get declines by 0.21% in MAE and improve the performance by 0.48% in Fβ. These results prove the effectiveness of contextual weighting module to in the framework. In addition, the ablation study on the loss function to verify the effectiveness of the loss function. As listed in Table 3, the loss function get declines by 1.21% in MAE and improve the performance by 14.60% in Fβ.
Method | MAE | Fβ |
CMN−+ lBCE | 0.0511 | 0.6977 |
CMN++lBCE | 0.0490 | 0.7025 |
CMN++L | 0.0369 | 0.8485 |
The results of this study show that the proposed method is outstanding over the previous state-of-the-art methods on the strip steel surface defect dataset. However, some defect images still pose challenges to these methods. The images (c) and (d) of Figure 7 show that the detection of some defect images are lack of integrity. The images (a), (b), (e) and (f) show that some defects are missed. Figure 7 shows the reasons leading to failure detection are attributed to some defects are too small to be detected; some defects show low contrast, so it is difficult to judge whether they really are defects, and in some cases, the characteristics of some defect areas apparently change. In the future, I plan to focus on solving these problems.
In this paper, a pixel-wise inspection framework based on CNN for the surface defect inspection of strip steel is proposed. Firstly, the encoder of the framework is built on the pre-trained VGG-16 network, which is used to extract multi-level features. Next, the contextual weighting module uses convolutional kernels with different size to obtain multi-scale context feature information from the convolution layers, which achieve overall perception of defect. Finally, in the decoder module, the cross integration is used to integrate the context information and previously decoded information into the current decoding block, which realizes the feature information complementation. The experiments of this study demonstrate that the proposed method is outstanding over the previous state-of-the-art methods in detection of strip steel defect dataset. To sum up, the proposed method can detect defects accurately, which makes the network strong robust and effective in defect detection. In the future, I will further optimize the algorithm model.
This work is supported by the National Key R & D Program of China with No.2018YFB1800402.
The authors declared that they have no conflicts of interest in this work.
[1] |
K. Song, Y. Yan, A noise robust method based on completed local binary patterns for hot-rolled steel strip surface defects, Appl. Surf. Sci., 285 (2013), 858-864. https://doi.org/10.1016/j.apsusc.2013.09.002 doi: 10.1016/j.apsusc.2013.09.002
![]() |
[2] |
Y. Liu, K. Xu, D. Wang, Online surface defect identification of cold rolled strips based on local binary pattern and extreme learning machine, Metals, 8 (2018), 197. https://doi.org/10.3390/met8030197 doi: 10.3390/met8030197
![]() |
[3] | D. Djukic, S. Spuzic, Statistical discriminator of surface defects on hot rolled steel, Image Vis. Comput., (2007), 158-163. |
[4] |
J. Wang, Q. Li, J. Gan, H. Yu, X. Yang, Surface defect detection via entity sparsity pursuit with intrinsic priors, IEEE Trans. Ind. Inform., 16 (2019), 141-150. https://doi.org/10.1109/TII.2019.2917522 doi: 10.1109/TII.2019.2917522
![]() |
[5] |
N. Neogi, D. K. Mohanta, P. K. Dutta, Defect detection of steel surfaces with global adaptive percentile thresholding of gradient image, J. Institut. Eng. (India) Series B, 98 (2017), 557-565. https://doi.org/10.1007/s40031-017-0296-2 doi: 10.1007/s40031-017-0296-2
![]() |
[6] |
D. C. Choi, Y. J. Jeon, S. H. Kim, S. Moon, J. P. Yun, S. W. Kim, Detection of pinholes in steel slabs using Gabor filter combination and morphological features, ISIJ Int., 57 (2017), 1045-1053. https://doi.org/10.2355/isijinternational.ISIJINT-2016-160 doi: 10.2355/isijinternational.ISIJINT-2016-160
![]() |
[7] |
X. Xie, M. Mirmehdi, TEXEMS: Texture exemplars for defect detection on random textured surfaces, IEEE. Trans. Pattern Anal. Mach. Intell., 29 (2007), 1454-1464. https://doi.org/10.1109/TPAMI.2007.1038 doi: 10.1109/TPAMI.2007.1038
![]() |
[8] |
F. S. Cohen, Z. Fan, S. Attali, Automated inspection of textile fabrics using textural models, IEEE. Trans. Pattern Anal. Mach. Intell., 13 (1991), 803-808. https://doi.org/10.1109/34.85670 doi: 10.1109/34.85670
![]() |
[9] | Y. He, K. Song, H. Dong, Y. Yan, Semi-supervised defect classification of steel surface based on multi-training and generative adversarial network, Opt. Lasers Eng., 122,294-302. https://doi.org/10.1016/j.optlaseng.2019.06.020 |
[10] |
V. Natarajan, T. Y. Hung, S. Vaikundam, L. T. Chia, Convolutional networks for voting-based anomaly classification in metal surface inspection, IEEE International Conference on Industrial Technology (ICIT), (2017), 986-991. https://10.1109/ICIT.2017.7915495 doi: 10.1109/ICIT.2017.7915495
![]() |
[11] |
M. Win, A. R. Bushroa, M. A. Hassan, N. M. Hilman, A. Ide-Ektessabi, A contrast adjustment thresholding method for surface defect detection based on mesoscopy, IEEE Trans. Ind. Inform., 11 (2015), 642-649. https://doi.org/10.1109/TⅡ.2015.2417676 doi: 10.1109/TII.2015.2417676
![]() |
[12] |
M. Ricci, A. Ficola, M. Fravolini, L. Battaglini, A. Palazzi, P. Burrascano, et al., Magnetic imaging and machine vision NDT for the on-line inspection of stainless steel strips, Meas. Sci. Technol., 24 (2012), 025401. https://doi.org/10.1007/s11276-012-0479-3 doi: 10.1007/s11276-012-0479-3
![]() |
[13] |
H. Hu, Y. Liu, M. Liu, L. Nie, Surface defect classification in large-scale strip steel image collection via hybrid chromosome genetic algorithm, Neurocomputing, 181 (2016), 86-95. https://doi.org/10.1016/j.neucom.2015.05.134 doi: 10.1016/j.neucom.2015.05.134
![]() |
[14] |
Y. J. Zhao, Y. H. Yan, K. C. Song, Vision-based automatic detection of steel surface defects in the cold rolling process: considering the influence of industrial liquids and surface textures, Int. J. Adv. Manuf. Technol., 90 (2017), 1665-1678. https://doi.org/10.1007/s00170-016-9489-0 doi: 10.1007/s00170-016-9489-0
![]() |
[15] |
Y. Wang, H. Xia, X. Yuan, L. Li, B. Sun, Distributed defect recognition on steel surfaces using an improved random forest algorithm with optimal multi-feature-set fusion, Multimed. Tools Appl., 77 (2018), 16741-16770. https://doi.org/10.1007/s11042-017-5238-0 doi: 10.1007/s11042-017-5238-0
![]() |
[16] | M. Chu, R. Gong, S. Gao, J. Zhao, Steel surface defects recognition based on multi-type statistical features and enhanced twin support vector machine, Chemometrics Intell. Lab. Syst., 171,140-150. https://doi.org/10.1016/j.chemolab.2017.10.020 |
[17] |
S. Fekri-Ershad, F. Tajeripour, Multi-resolution and noise-resistant surface defect detection approach using new version of local binary patterns, Appl. Artif. Intell., 31 (2017), 395-410. https://doi.org/10.1080/08839514.2017.1378012 doi: 10.1080/08839514.2017.1378012
![]() |
[18] |
X. Zhang, W. Li, J. Xi, Z. Zhang, X. Fan, Surface defect target identification on copper strip based on adaptive genetic algorithm and feature saliency, Math. Probl. Eng., 2013. https://doi.org/10.1155/2013/504895 doi: 10.1155/2013/504895
![]() |
[19] |
Y. H. Ai, K. Xu, Surface detection of continuous casting slabs based on curvelet transform and kernel locality preserving projections, J. Iron Steel Res. Int., 20 (2013), 80-86. https://doi.org/10.1016/S1006-706X(13)60102-8 doi: 10.1016/S1006-706X(13)60102-8
![]() |
[20] |
Ş. Öztürk, B. Akdemir, Real-time product quality control system using optimized Gabor filter bank, Int. J. Adv. Manuf. Technol., 96 (2018), 11-19. https://doi.org/10.1007/s00170-018-1585-x doi: 10.1007/s00170-018-1585-x
![]() |
[21] |
D. C. Choi, Y. J. Jeon, S. J. Lee, J. P. Yun, S. W. Kim, Algorithm for detecting seam cracks in steel plates using a Gabor filter combination method, Appl. optics, 53 (2014), 4865-4872. https://doi.org/10.1364/AO.53.004865 doi: 10.1364/AO.53.004865
![]() |
[22] |
X. Y. Wu, K. Xu, J. W. Xu, Application of undecimated wavelet transform to surface defect detection of hot rolled steel plates, In 2008 Congress on Image and Signal Processing, (2008), 528-532. https://10.1109/CISP.2008.278 doi: 10.1109/CISP.2008.278
![]() |
[23] |
Ş. Öztürk, B. Akdemır, Novel BiasFeed cellular neural network model for glass defect inspection, In 2016 International Conference on Control, Decision and Information Technologies (CoDIT), (2016), 366-371. https://doi.org/10.1109/CoDIT.2016.7593590 doi: 10.1109/CoDIT.2016.7593590
![]() |
[24] |
X. Li, S. K. Tso, X. P. Guan, Q. Huang, Improving automatic detection of defects in castings by applying wavelet technique, IEEE Trans. Ind. Electron., 53 (2006), 1927-1934. https://doi.org/10.1109/TIE.2006.885448 doi: 10.1109/TIE.2006.885448
![]() |
[25] |
X. Liu, K. Xu, P. Zhou, D. Zhou, Y. Zhou, Surface defect identification of aluminium strips with non-subsampled shearlet transform, Opt. Lasers Eng., (2020). https://doi.org/10.1016/j.optlaseng.2019.105986 doi: 10.1016/j.optlaseng.2019.105986
![]() |
[26] |
B. Akdemir, S. Öztürk, Glass surface defects detection with wavelet transforms, Int. J. Mater., Mechan. Manuf., 3 (2015), 170-173. https://doi.org/10.7763/IJMMM.2015.V3.189 doi: 10.7763/IJMMM.2015.V3.189
![]() |
[27] |
F. S. Cohen, Z. Fan, S. Attali, Automated inspection of textile fabrics using textural models, IEEE. Trans. Pattern Anal. Mach. Intell., 13 (1991), 803-808. https://doi.org/10.1109/34.85670 doi: 10.1109/34.85670
![]() |
[28] |
G. Song, K. Song, Y. Yan, Saliency detection for strip steel surface defects using multiple constraints and improved texture features, Opt. Lasers Eng., 2019. https://doi.org/10.1016/j.optlaseng.2019.106000 doi: 10.1016/j.optlaseng.2019.106000
![]() |
[29] | J. Masci, U. Meier, G. Fricout, J. Schmidhuber, Multi-scale pyramidal pooling network for generic steel defect classification, In The 2013 International Joint Conference on Neural Networks (IJCNN), 2013. https://doi.org/10.1109/IJCNN.2013.6706920 |
[30] |
D. He, K. Xu, P. Zhou, Defect detection of hot rolled steels with a new object detection framework called classification priority network, Comput. Ind. Eng., 128 (2018), 290-297. https://doi.org/10.1016/j.cie.2018.12.043 doi: 10.1016/j.cie.2018.12.043
![]() |
[31] |
Y. He, K. Song, Q. Meng, Y. Yan, An end-to-end steel surface defect detection approach via fusing multiple hierarchical features, IEEE Trans. Instrum. Meas., 69 (2019), 1493-1504. https://doi.org/10.1109/TIM.2019.2915404 doi: 10.1109/TIM.2019.2915404
![]() |
[32] |
X. Kou, S. Liu, K. Cheng, Y. Qian, Development of a YOLO-V3-based model for detecting defects on steel strip surface, Measurement, 182 (2021). https://doi.org/10.1016/j.measurement.2021.109454 doi: 10.1016/j.measurement.2021.109454
![]() |
[33] |
R. Ren, T. Hung, K. C. Tan, A generic deep-learning-based approach for automated surface inspection, IEEE T. Cybern., 48 (2017), 929-940. https://doi.org/10.1109/TCYB.2017.2668395 doi: 10.1109/TCYB.2017.2668395
![]() |
[34] |
H. Yang, Y. Chen, K. Song, Z. Yin, Multiscale feature-clustering-based fully convolutional autoencoder for fast accurate visual inspection of texture surface defects, IEEE Trans. Autom. Sci. Eng., 16 (2019), 1450-1467. https://doi.org/10.1109/TASE.2018.2886031 doi: 10.1109/TASE.2018.2886031
![]() |
[35] |
R. Neven, T. Goedemé, A multi-branch U-Net for steel surface defect type and severity segmentation, Metals, 11 (2021), 870. https://doi.org/10.3390/met11060870 doi: 10.3390/met11060870
![]() |
[36] |
X. Zhou, H. Fang, X. Fei, R. Shi, J. Zhang, Edge-aware multi-level interactive network for salient object detection of strip steel surface defects, IEEE Access, (2021). https://doi.org/10.1109/ACCESS.2021.3124814 doi: 10.1109/ACCESS.2021.3124814
![]() |
[37] |
G. Song, K. Song, Y. Yan, EDRNet: Encoder-decoder residual network for salient object detection of strip steel surface defects, IEEE Trans. Instrum. Meas., 69 (2020), 9709-9719. https://doi.org/10.1109/TIM.2020.3002277 doi: 10.1109/TIM.2020.3002277
![]() |
[38] |
H. Dong, K. Song, Y. He, J. Xu, Y. Yan, Q. Meng, PGA-Net: Pyramid feature fusion and global context attention network for automated surface defect detection, IEEE Trans. Ind. Inform., 16 (2019), 7448-7458. https://doi.org/10.1109/TII.2019.2958826 doi: 10.1109/TII.2019.2958826
![]() |
[39] | K. Simonyan, A. Zisserman, Very deep convolutional networks for large-scale image recognition, arXiv preprint, (2014), arXiv: 1409.1556. https://doi.org/10.48550/arXiv.1409.1556 |
[40] |
J. Long, E. Shelhamer, T. Darrell, Fully convolutional networks for semantic segmentation, In Proceedings of the IEEE conference on computer vision and pattern recognition (CVPR), (2015), 3431-3440. https://doi.org/10.1109/CVPR.2015.7298965 doi: 10.1109/CVPR.2015.7298965
![]() |
[41] |
O. Ronneberger, P. Fischer, T. Brox, U-net: Convolutional networks for biomedical image segmentation, In International Conference on Medical image computing and computer-assisted intervention, (2015), 234-241. https://doi.org/10.1007/978-3-319-24574-4_28 doi: 10.1007/978-3-319-24574-4_28
![]() |
[42] |
H. J. Kim, E. Dunn, J. M. Frahm, Learned contextual feature reweighting for image geo-localization, In 2017 IEEE Conference on Computer Vision and Pattern Recognition (CVPR), (2017), 3251-3260. https://doi.org/10.1109/CVPR.2017.346 doi: 10.1109/CVPR.2017.346
![]() |
[43] |
P. T. De Boer, D. P. Kroese, S. Mannor, R. Y. Rubinstein, A tutorial on the cross-entropy method, Ann. Oper. Res., 134 (2005), 19-67. https://doi.org/10.1007/s10479-005-5724-z doi: 10.1007/s10479-005-5724-z
![]() |
[44] |
M. A. Rahman, Y. Wang, Optimizing intersection-over-union in deep neural networks for image segmentation, In International symposium on visual computing, (2016), 234-244. https://doi.org/10.1007/978-3-319-50835-1_22 doi: 10.1007/978-3-319-50835-1_22
![]() |
[45] |
Z. Wang, E. P. Simoncelli, A. C. Bovik, Multiscale structural similarity for image quality assessment, In The Thrity-Seventh Asilomar Conference on Signals, Systems & Computers, 2 (2003), 1398-1402. https://doi.org/10.1109/ACSSC.2003.1292216 doi: 10.1109/ACSSC.2003.1292216
![]() |
[46] |
M. Abadi, P. Barham, J. Chen, Z. Chen, A. Davis, J. Dean, et al., TensorFlow: A system for large-scale machine learning, In 12th USENIX symposium on operating systems design and implementation (OSDI 16), (2016), 265-283. https://dl.acm.org/doi/10.5555/3026877.3026899 doi: 10.5555/3026877.3026899
![]() |
[47] |
A. Krizhevsky, I. Sutskever, G. E. Hinton, Imagenet classification with deep convolutional neural networks, Adv. Neural Inform. Process. Syst., 2017. https://doi.org/10.1145/3065386 doi: 10.1145/3065386
![]() |
[48] |
F. Perazzi, P. Krähenbühl, Y. Pritch, A. Hornung, Saliency filters: Contrast based filtering for salient region detection, In 2012 IEEE conference on computer vision and pattern recognition (CVPR), (2012), 733-740. https://doi.org/10.1109/CVPR.2012.6247743 doi: 10.1109/CVPR.2012.6247743
![]() |
[49] |
Y. Qin, H. Lu, Y. Xu, H. Wang, Saliency detection via cellular automata, In Proceedings of the IEEE conference on computer vision and pattern recognition (CVPR), (2012), 110-119. https://doi.org/10.1109/CVPR.2012.6247743 doi: 10.1109/CVPR.2012.6247743
![]() |
[50] |
R. Achanta, S. Hemami, F. Estrada, S. Susstrunk, Frequency-tuned salient region detection, In 2009 IEEE conference on computer vision and pattern recognition (CVPR), (2012), 1597-1604. https://doi.org/10.1109/CVPR.2009.5206596 doi: 10.1109/CVPR.2009.5206596
![]() |
[51] |
F. Huang, J. Qi, H. Lu, L. Zhang, X. Ruan, Salient object detection via multiple instance learning, IEEE Trans. Image Process., 26 (2017), 1911-1922. https://doi.org/10.1109/TIP.2017.2669878 doi: 10.1109/TIP.2017.2669878
![]() |
[52] |
M. M. Cheng, N. J. Mitra, X. Huang, P. H. Torr, S. M. Hu, Global contrast based salient region detection, IEEE. Trans. Pattern Anal. Mach. Intell., 37 (2014), 569-582. https://doi.org/10.1109/TPAMI.2014.2345401 doi: 10.1109/TPAMI.2014.2345401
![]() |
[53] |
H. Peng, B. Li, H. Ling, W. Hu, W. Xiong, S. J. Maybank, Salient object detection via structured matrix decomposition, IEEE. Trans. Pattern Anal. Mach. Intell., 39 (2016), 818-832. https://doi.org/10.1109/TPAMI.2016.2562626 doi: 10.1109/TPAMI.2016.2562626
![]() |
[54] | H. Noh, S. Hong, B. Han, Learning deconvolution network for semantic segmentation, In Proceedings of the IEEE international conference on computer vision, (2015), 1520-1528. https://doi.org/10.1109/ICCV.2015.178 |
[55] |
N. Liu, J. Han, Dhsnet: Deep hierarchical saliency network for salient object detection, In Proceedings of the IEEE conference on computer vision and pattern recognition (CVPR), (2016), 678-686. https://doi.org/10.1109/CVPR.2016.80 doi: 10.1109/CVPR.2016.80
![]() |
[56] |
Q. Hou, M. M. Cheng, X. Hu, A. Borji, Z. Tu, P. H. Torr, Deeply supervised salient object detection with short connections, In Proceedings of the IEEE conference on computer vision and pattern recognition (CVPR), (2016), 3203-3212. https://doi.org/10.1109/TPAMI.2018.2815688 doi: 10.1109/TPAMI.2018.2815688
![]() |
1. | Wei Chen, Bin Zou, GongXian Yang, QinBing Zheng, Ting Lei, Chuanzhen Huang, JiKai Liu, Lei Li, A real-time detection system for multiscale surface defects of 3D printed ceramic parts based on deep learning, 2024, 50, 02728842, 13101, 10.1016/j.ceramint.2024.01.220 | |
2. | Wenyue Sun, Jindong Zhang, Yitong Liu, Adversarial-based refinement dual-branch network for semi-supervised salient object detection of strip steel surface defects, 2024, 0178-2789, 10.1007/s00371-024-03442-y | |
3. | Zefeng Li, Zeyu Zhang, Jixin Ma, Lele Tian, 2023, Improved YOLOV5 based industrial surface defect detection method, 979-8-3503-4271-0, 367, 10.1109/CIPAE60493.2023.00077 | |
4. | Emine Aşar, Atilla Özgür, 2024, Chapter 3, 978-3-031-57467-2, 37, 10.1007/978-3-031-57468-9_3 | |
5. | Fang Luo, Yuan Cui, Xu Wang, Zhiliang Zhang, Yong Liao, Adaptive rotation attention network for accurate defect detection on magnetic tile surface, 2023, 20, 1551-0018, 17554, 10.3934/mbe.2023779 | |
6. | Rong Wang, Zhenzhong Zhang, Guozhao Wei, Haijun Zhang, Xiaoliang Liang, Intelligent recognition method for material removal mode during high-quality ground surface of RB-SiC ceramics based on YOLOv8-Slim-Neck-Ca model, 2025, 1058-9759, 1, 10.1080/10589759.2025.2453945 | |
7. | Chenjie Li, Mian Zhou, Weiwei Pan, 2024, Visual inspection of coatings in hydrogen fuel cells, 9798400707308, 298, 10.1145/3707292.3707380 |
Stage | Template | ||
enc1 | conv 3 × 3, stride = 1, D = 64, BN + ReLU | max_pooling 2 × 2, stride = 2 | ×2 |
enc2 | conv 3 × 3, stride = 1, D = 128, BN + ReLU | max_pooling 2 × 2, stride = 2 | ×2 |
enc3 | conv 3 × 3, stride = 1, D = 256, BN + ReLU | max_pooling 2 × 2, stride = 2 | ×2 |
enc4 | conv 3 × 3, stride = 1, D = 512, BN + ReLU | max_pooling 2 × 2, stride = 2 | ×2 |
enc5 | conv 3 × 3, stride = 1, D = 512, BN + ReLU | max_pooling 2 × 2, stride = 2 | ×2 |
Method | BSCA[49] | FT[50] | MIL[51] | RC[52] | SMD[53] | FCN[40] | UNet[41] | DN[54] | DHSNet[55] | DSS[56] | My |
MAE↓ | 0.2462 | 0157. 7 | 0.1764 | 0.1228 | 0.1913 | 0.0604 | 0.0643 | 0.0481 | 0.0399 | 0.0371 | 0.0369 |
Fβ | 0.3404 | 0.4462 | 0.4873 | 0.3719 | 0.4665 | 0.6788 | 0.6783 | 0.7272 | 0.8447 | 0.8051 | 0.8485 |
Method | MAE | Fβ |
CMN−+ lBCE | 0.0511 | 0.6977 |
CMN++lBCE | 0.0490 | 0.7025 |
CMN++L | 0.0369 | 0.8485 |
Stage | Template | ||
enc1 | conv 3 × 3, stride = 1, D = 64, BN + ReLU | max_pooling 2 × 2, stride = 2 | ×2 |
enc2 | conv 3 × 3, stride = 1, D = 128, BN + ReLU | max_pooling 2 × 2, stride = 2 | ×2 |
enc3 | conv 3 × 3, stride = 1, D = 256, BN + ReLU | max_pooling 2 × 2, stride = 2 | ×2 |
enc4 | conv 3 × 3, stride = 1, D = 512, BN + ReLU | max_pooling 2 × 2, stride = 2 | ×2 |
enc5 | conv 3 × 3, stride = 1, D = 512, BN + ReLU | max_pooling 2 × 2, stride = 2 | ×2 |
Method | BSCA[49] | FT[50] | MIL[51] | RC[52] | SMD[53] | FCN[40] | UNet[41] | DN[54] | DHSNet[55] | DSS[56] | My |
MAE↓ | 0.2462 | 0157. 7 | 0.1764 | 0.1228 | 0.1913 | 0.0604 | 0.0643 | 0.0481 | 0.0399 | 0.0371 | 0.0369 |
Fβ | 0.3404 | 0.4462 | 0.4873 | 0.3719 | 0.4665 | 0.6788 | 0.6783 | 0.7272 | 0.8447 | 0.8051 | 0.8485 |
Method | MAE | Fβ |
CMN−+ lBCE | 0.0511 | 0.6977 |
CMN++lBCE | 0.0490 | 0.7025 |
CMN++L | 0.0369 | 0.8485 |