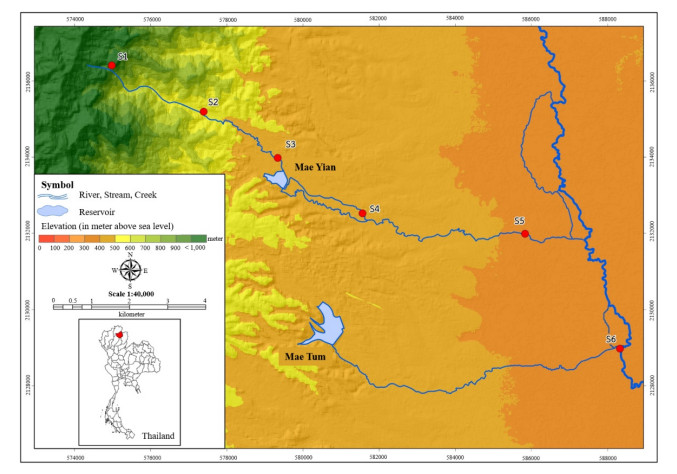
Citation: Jun Gao, Qian Jiang, Bo Zhou, Daozheng Chen. Convolutional neural networks for computer-aided detection or diagnosis in medical image analysis: An overview[J]. Mathematical Biosciences and Engineering, 2019, 16(6): 6536-6561. doi: 10.3934/mbe.2019326
[1] | Muhammad Imran Asjad, Naeem Ullah, Asma Taskeen, Fahd Jarad . Study of power law non-linearity in solitonic solutions using extended hyperbolic function method. AIMS Mathematics, 2022, 7(10): 18603-18615. doi: 10.3934/math.20221023 |
[2] | Mahmoud Soliman, Hamdy M. Ahmed, Niveen Badra, M. Elsaid Ramadan, Islam Samir, Soliman Alkhatib . Influence of the β-fractional derivative on optical soliton solutions of the pure-quartic nonlinear Schrödinger equation with weak nonlocality. AIMS Mathematics, 2025, 10(3): 7489-7508. doi: 10.3934/math.2025344 |
[3] | Chun Huang, Zhao Li . New soliton solutions of the conformal time derivative generalized q-deformed sinh-Gordon equation. AIMS Mathematics, 2024, 9(2): 4194-4204. doi: 10.3934/math.2024206 |
[4] | Tianyong Han, Ying Liang, Wenjie Fan . Dynamics and soliton solutions of the perturbed Schrödinger-Hirota equation with cubic-quintic-septic nonlinearity in dispersive media. AIMS Mathematics, 2025, 10(1): 754-776. doi: 10.3934/math.2025035 |
[5] | Emad H. M. Zahran, Omar Abu Arqub, Ahmet Bekir, Marwan Abukhaled . New diverse types of soliton solutions to the Radhakrishnan-Kundu-Lakshmanan equation. AIMS Mathematics, 2023, 8(4): 8985-9008. doi: 10.3934/math.2023450 |
[6] | Kun Zhang, Tianyong Han, Zhao Li . New single traveling wave solution of the Fokas system via complete discrimination system for polynomial method. AIMS Mathematics, 2023, 8(1): 1925-1936. doi: 10.3934/math.2023099 |
[7] | Da Shi, Zhao Li, Dan Chen . New traveling wave solutions, phase portrait and chaotic patterns for the dispersive concatenation model with spatio-temporal dispersion having multiplicative white noise. AIMS Mathematics, 2024, 9(9): 25732-25751. doi: 10.3934/math.20241257 |
[8] | Mahmoud Soliman, Hamdy M. Ahmed, Niveen Badra, Taher A. Nofal, Islam Samir . Highly dispersive gap solitons for conformable fractional model in optical fibers with dispersive reflectivity solutions using the modified extended direct algebraic method. AIMS Mathematics, 2024, 9(9): 25205-25222. doi: 10.3934/math.20241229 |
[9] | Hamood Ur Rehman, Aziz Ullah Awan, Sayed M. Eldin, Ifrah Iqbal . Study of optical stochastic solitons of Biswas-Arshed equation with multiplicative noise. AIMS Mathematics, 2023, 8(9): 21606-21621. doi: 10.3934/math.20231101 |
[10] | Dumitru Baleanu, Kamyar Hosseini, Soheil Salahshour, Khadijeh Sadri, Mohammad Mirzazadeh, Choonkil Park, Ali Ahmadian . The (2+1)-dimensional hyperbolic nonlinear Schrödinger equation and its optical solitons. AIMS Mathematics, 2021, 6(9): 9568-9581. doi: 10.3934/math.2021556 |
Pesticides are chemical substances that are used to prevent, reduce or eliminate the harmful effects of insects, weeds, fungi and plant pathogens. Overuse of pesticides for agricultural and nonagricultural purposes has led to the presence of chemical residues in surface and groundwater sources, which is a serious public health risk that can negatively affect human health and the environment [1,2]. Pesticides contaminate aquatic ecosystems through a variety of mechanisms, including runoff, spray dispersion, leaching and tile drainage [3,4]. Only 0.1% of pesticides affect their intended target; the remaining 99.9% damage the environment [5]. Although pesticides are designed to eliminate fungi, insects, and other pests, their mode of action also impacts nontarget organisms, such as algae, which are the primary producers in aquatic ecosystems [6].
The Mae Yian Stream is located in Phayao Province, Thailand, where the water flows through an area with a variety of activities; along the stream, there are agricultural activities, farms, cattle ranches and fish farming, and it flows through a densely populated urban area. Lychee Orchard, which has a total planting area of 1,949.6 hectares, is the most extensive agricultural operation in this part of the province [7]. The infestation of fruit borers, a pest that causes product damage, is the most common problem. It causes 65.41% of the damage to lychee fruit [8]. The level of cypermethrin residue in lychee samples ranged from 0.077 mg/kg in China to 2.86 mg/kg in Thailand, indicating that it is widely used in several regions of Asia [9,10]. Furthermore, cypermethrin is not only found in lychee fruit samples but also frequently detected in agricultural watersheds in northern Thailand [11].
Pesticide-contaminated water has a negative impact on phytoplankton communities, which are important primary producers in aquatic food chains [12]. Munaron et al. [13] and Wijewardene et al. [14] studied the effects of pesticides on phytoplankton communities and found that they are significantly affected by the toxicity of pesticides. Pesticides inhibit algae development by decreasing chlorophyll concentration and cell activity, decreasing carbohydrates, proteins and flavonoids and increasing superoxide dismutase enzymatic activity [15,16]. Phytoplankton communities are threatened by individual pesticides and pesticide mixtures. Furthermore, the correlation between nutrients and pesticide content results in stressors to the structure of the phytoplankton community [13,14]. However, these effects depend on the concentration of the pesticide and the frequency of exposure [17]. Furthermore, pesticides may have indirect impacts on phytoplankton communities through their toxic effects on zooplankton respiration, consequently influencing phytoplankton populations [18,19].
Numerous previous studies have investigated pesticide residues in water in agricultural areas [20,21,22]. Several studies have examined the detrimental effects of pesticides on phytoplankton [4,23], while some have focused on the relationship between phytoplankton and water quality in one or more geographic regions [24]. Nevertheless, few studies have examined the connection among pesticide residue, water quality, and phytoplankton distribution. Consequently, the objective of this study was to examine the relationship between these factors in a lychee plantation catchment area that has a wide range of altitudes and a variety of land uses. It is important to understand the impact of diverse land use patterns and geographical differences on changes in water quality and phytoplankton diversity and provide important data for the future environmental management of this region.
The study was carried out in Muang district, Phayao Province, Thailand. The geographical and land use characteristics of the study area ranged from the upstream and agricultural zones to the community area, with altitudes between 800 and 400 meters above sea level. From January to December 2022, phytoplankton, water quality and cypermethrin residue samples were collected at six sampling stations, including the Mae Yian waterfall station (S1), lychee orchard station (S2), fish farming station (S3), agricultural farming station (S4), urban community station (S5) and Mae Yian and Mae Tum stream confluence station (S6). (Figure 1)
The residue of cypermethrin insecticide in water was analyzed using the modified method of Mihaylova et al. [25]. Briefly, water samples were collected in an amber glass bottle. Then 100 mL was filtered using a 45 µm nylon membrane filter and extracted three times with 60 mL of dichloromethane for each extraction. The extracted organic materials were then concentrated by a rotary evaporator. The completed sample was mixed with 10 mL of methanol and added to the vial for high-performance liquid chromatography (HPLC) analysis.
The cypermethrin residue was examined. Using a mobile phase consisting of methanol, acetonitrile and water (38:38:24, v/v/v) with a flow rate of 1.0 mL/min and an injection volume of 10 µL, the eluate was detected at 235 nm at a temperature of 20 ℃. The percent recovery of the pesticides studied was estimated by spiking deionized water with three concentrations (0.5, 1.0 and 10.0 mg/L) of each pesticide. The spiked samples were extracted according to the extraction procedure described in the above section and analyzed by HPLC. The precision of the method was evaluated by the relative standard deviation (RSD, %) of the areas of six replicate injections of each pesticide at three concentrations (0.5, 1.0 and 10.0 mg/L) [25]. Sensitivity was determined by establishing the limit of detection (LOD) and the limit of quantification (LOQ). The LOD and LOQ of cypermethrin were calculated by preparing spiked solutions of cypermethrin at low concentrations in signal-to-noise ratios of 3:1 and 10:1, respectively [26].
Water temperature, conductivity and pH were measured in the field using a portable Multi-Parameter CyberScan PCD650 (Thermo Scientific, Singapore). The turbidity of the water was determined using a turbidimeter (Thermo Scientific, Germany). Ammonium nitrogen (NH4-N), nitrate nitrogen (NO3-N) and orthophosphate (PO43-) were measured using a HACH Spectrophotometer Model 890 (HACH, USA). The dissolved oxygen (DO) and the biochemical oxygen demand (BOD) were analyzed using the azide modification method [27]. Total coliform bacteria (TCB) and fecal coliform bacteria (FCB) were determined as the most probable number (MPN) counts using the multiple-tube fermentation technique [27]. The chlorophyll a content was analyzed according to Saijo [28] and Wintermans and De Mots [29]. The water quality index (WQI) was calculated using DO, BOD, NH4-N, TCB and FCB [30].
Phytoplankton samples were collected by filtering 20 liters of a water sample with a plankton net and squeezing aquatic plants or the surface of a rock with a brush to collect epiphytic algae. The samples were fixed with Lugol's iodine solution to preserve the collected phytoplankton. The Sedgewick Rafter Counting Chamber (T. Science, Thailand) was used for the counting of phytoplankton under a light microscope. The number of species and the number of individuals were used to calculate the diversity index (H') and evenness (J') [31]. Taxonomic identification was carried out using monographs based on the specialized taxonomic literature [32,33,34].
All experiments were carried out in triplicate. The results are expressed as the average ± standard deviation (SD). All data were statistically analyzed using analysis of variance and Duncan's multiple range test (DMRT) to verify significant differences between treatments at p ≤ 0.05. The concentration of the cypermethrin residues, the quality of the water and the phytoplankton species were calculated for their correlation using canonical correspondence analysis (CCA) by Past 4.3. Pearson's correlation was also used to determine the relationship between indicators of water quality and cypermethrin residues.
The cypermethrin residues in the surface water were monitored using HPLC. Initially, the standard curve between 0 and 50 mg/L was established using seven calibration levels (0, 0.5, 1, 5, 10, 25 and 50 mg/L cypermethrin) to assess sensitivity. The LOD and the LOQ were determined with values of 0.08 and 0.26 mg/L, respectively. Using three concentrations (0.5, 1.0 and 10.0 mg/L), the percent recovery of cypermethrin was determined at values of 98.39,101.12 and 101.07, respectively. In addition, the RSD was calculated to evaluate the precision of the method, i.e., 0.32, 0.35 and 0.18%, respectively (Table 1).
Cypermethrin (mg/L) | % Recovery | RSD |
0.5 | 98.39 | 0.32 |
1.0 | 101.12 | 0.35 |
10.0 | 101.07 | 0.18 |
LOD | 0.08 | |
LOQ | 0.26 |
The monitoring period was January to May 2022 and covered the period from agricultural land preparation through lychee harvest. In January, the amount of residue increased from Station S1 to Station S5 but decreased at Station S6, where the Mae Yian and Mae Tum streams converge. Farmers began spraying cypermethrin for fruit border control in February, and cypermethrin residue increased significantly from January to March. The highest residue level was observed in March, notably at Station S4, where a level of 29.43 mg/L was detected. In April and May, the cypermethrin residue decreased significantly. In April, the highest concentrations of cypermethrin residues were found at Station S4, while no cypermethrin residues were detected at Station S1. In May, no significant differences in residues were found between the sampling stations. (Figure 2). In summary, cypermethrin contamination of surface water in the investigated area was consistent with the agricultural practices of the lychee producers, whose insecticide spraying period began in the middle of February and peaked in March. This finding indicates that the time of application of cypermethrin was the primary factor in the contamination of surface water in the lychee catchment area. The presence of some agrochemicals may have been attributable to geological composition, but contamination of the water samples with high concentrations of pesticides demonstrated the excessive application of pesticides at a landscape scale. In general, there was a stronger tendency toward temporal variation than toward spatial variation [35]. In addition, according to Vryzas et al. [36], pesticide residues can be detected at higher concentrations after rainfall. This finding is consistent with our results, as tropical storms occurred during the month of March, resulting in significant rainfall in several regions [37].
Another observation was that the altitude of the area was a crucial factor in determining the level of residue, as seen at Stations S1–S3 (steep slopes) (Figure 1), where the residue levels were lower than those at Stations S4–S6. The use of pesticides on mountains has increased the transfer of pesticides to surface water. High application of pesticides on steep slopes, high and intense rainfall and well-developed preferential flow pathways have transported a large percentage of pesticide residues from their application site to nearby environmental regions [11]. Lastly, the confluence of two streams is an additional factor that influenced the cypermethrin residue, as evident at Station 6, where the level of residue reversed direction compared to that at Station S5. Pesticide concentrations decreased with increasing distance from the application area [38]. However, the merging of two rivers can either increase or decrease the concentration of pesticides, depending on the composition of the combined waters [39].
The analysis of water quality using 12 parameters from January to May is shown in Figure 3. The average water temperature varied from 22–26.9 ℃ and increased with season, with the cold dry season occurring from January to February and the summer season occurring from March to May. Weather changes have a tremendous influence on the environment. Higher water temperatures result from an increase in air temperature, and both variables are also impacted by seasons in different geographic areas [40]. The pH and conductivity decreased significantly from Station S1 to Station S4, with Stations S1 and S2 being upstream of a flow, where the dissolution of various minerals caused the pH and conductivity to be relatively high. The terrains of Stations S1, S2 and S3 are sloped, which can influence the flow rate of water and the leaching of minerals into the water source. The presence of inorganic dissolved compounds such as chloride, nitrate, sulfate and phosphate anions is a significant factor that affects the conductivity of electricity [41].
When investigating the turbidity of the water, Station S6 had the highest turbidity level because it is the confluence of the Mae Yian Stream with other streams with different water qualities and its water flows constantly. The presence of turbidity prevents the transmission of light and other environmental factors, resulting in increased sedimentation and siltation, which can be detrimental to aquatic habitats, especially for algae [42]. Therefore, high water turbidity levels can have a direct effect on the abundance of phytoplankton, as seen at Station 6, where the lowest abundance of phytoplankton was observed (Figure 5B).
DO and BOD are crucial environmental indices that are essential indicators for the evaluation of river ecosystems [43,44]. Across all sampling stations, the levels of DO and BOD tended to be inversely related. DO levels decreased markedly at Stations S4, S5 and S6, particularly at Station S4 (agricultural farming station), where the DO level decreased to 3 mg/L (Figure 3), which was below the standard for surface water quality [45]. The level of DO could be a factor that has a direct effect on phytoplankton abundance, which was markedly lower at station S4 than at stations S3 and S5 (Figure 5B). Low levels of dissolved oxygen can limit the availability of oxygen for cellular respiration, thereby reducing the growth and abundance of phytoplankton [46]. Furthermore, in low-oxygen environments, phytoplankton can face increased competition from bacteria, which can thrive in low-oxygen conditions. This competition for limited resources may further restrict the growth of phytoplankton [47]. Simultaneously, BOD level exhibited a significant upward trend, with its concentrations ranging from 1.31–2.82 mg/L. According to Verma and Singh [48], moderately polluted water sources have BOD values between 2 and 8 mg/L. Therefore, Stations S3 to S6 had BOD values within the specified range and were categorized as moderately polluted water. On the basis of these results, it was found that the levels of DO and BOD changed in response to variations in geographical land use and human activities. Organic waste, specifically domestic household waste from population activity and animal sewage, as well as agricultural land wastewater, are sources of organic pollutants that have the greatest influence on DO and BOD concentrations [49,50].
Nutrients, especially nitrogen and phosphorus, are important factors that influence the growth of algae [51,52]. Station S3 had the highest average ammonia content at 0.33 mg/L, followed by that at Station S5 at 0.27 mg/L, as Station S3 is in a fisheries area and Station S5 is in a highly populated community. Similarly, orthophosphate and nitrate concentrations were high at Stations S3, S5 and S6, with Station S5 showing the highest average orthophosphate content of 0.18 mg/L. This station is in a local community, which is an important factor in the amount of orthophosphate in water sources [53]. Plants in agricultural areas obtain their nutrients from the N, P and K groups. These nutrients may enter a water body when provided in excess compared to the needs of the plants [54]. Station S6 had the highest nitrate nitrogen values at 1.47 mg/L. This finding could have been due to the impact of the water quality of another stream connected to our studied stream that flows through a paddy area notable for its high rate of nitrogen fertilizer application. Consequently, this scenario led to a high concentration of nitrate at Station S6. According to Gu and Yang [55], more than 60% of the nitrogen fertilizer applied to rice fields is lost in the form of ammonia, nitrate and nitrous oxide instead of being absorbed by rice plants. However, reports indicate that most of the nitrogen used in paddy fields is in the form of ammonia rather than nitrate [56,57,58].
TCB and FCB are indicators of deterioration of a water source. In this study, Station S5 had the highest average levels of total coliform and fecal coliform bacteria at 21,800 and 2,600 MPN per 100 mL, respectively. The amount of TCB exceeded the standards set by the Thailand Pollution Control Department, indicating contamination of water sources with sewage, mammalian waste products from various activities and discharge into drainage pipes or natural water bodies without prior treatment [59]. Although Station S5 is in a densely populated area, there is no established sewage treatment system. This must be one of the reasons why this station had the highest average total coliform and fecal coliform bacteria levels. Apirukmontri et al. [60] reported that compared to in untreated wastewater, in wastewater treatment systems, TCB and FCB levels were significantly reduced up to 15 and 2 times, respectively.
According to OECD criteria, chlorophyll concentrations indicate algal biomass in water bodies and determine the trophic status [61]. Chlorophyll a concentration was used to assess the trophic status of water, and the stations were classified as follows. Station S3 was in a eutrophic state, and Stations S4, S5 and S6 were in a mesotrophic state. Stations S1 and S2 were in an oligotrophic state. Specifically, the eutrophic status of Station S3 indicated a situation in which the water body was loaded with nutrients, causing algal blooms [62]. Furthermore, the amount of ammonia nitrogen and phytoplankton seen at this location also corresponded to this finding (Figure 3; Figure 5B).
In describing the water quality of the research area, five parameters, i.e., DO, BOD, TCB, FCB and ammonia content, were used to calculate the water quality index (WQI), which indicates the state of each water source based on station scores. The WQI scores for each station ranged from 93–32, indicating significantly excellent to deteriorated water quality. It was determined that the water quality score decreased substantially from Station S1 to Station S5 and increased at Station S6 due to the variation in land use and human activities, such as fisheries, agriculture and residential density, between stations. The station with the lowest water quality was S5, with a score between 36 and 53 (Figure 4), indicating a deterioration in water quality.
The study of phytoplankton diversity in the Mae Yian Stream over a five-month period (January to May) classified 174 phytoplankton species into five divisions, including Cyanophyta, Chlorophyta, Euglenophyta, Bacillariophyta and Pyrrophyta. Chlorophyta comprised 61.49% of the species, followed by Bacillariophyta (28.16%) and Cyanophyta (6.32%). The three most dominant algal species were Navicula sp., Phacus sp. and Coelastrum astroideum (Supplementary Table A1). The abundance of phytoplankton tended to increase from January to March, when it peaked at 1,608,500 cells/L (Figure 5A) and then decreased considerably from April to May. On a monthly basis, Figure 5B summarizes the presence of different species observed at each sampling site, and it was determined that Station S3 had the highest number of phytoplankton, with 1,984,500 cells/L.
Shannon's diversity (H') and evenness (J') indices were applied to the sample sites to evaluate phytoplankton diversity. Of the stations, Station S3 had the highest Shannon's diversity index for most of the study period (Figure 6), which corresponded to the maximum cell abundance at this station (Figure 5B). The evenness of species (J') in a habitat refers to how close each species is in number. Of the stations, Station S3 had the highest species evenness in January and April, whereas Station S4 had the highest evenness in February and March. In contrast, Station S6 in May had the lowest Shannon's diversity index, with the highest species evenness due to only two species of phytoplankton being observed. When considering the seasonal variation in the phytoplankton communities, the diversity index was found to be higher during the cold dry season (January-February) than during the summer season (March-May). This result is consistent with the findings of Khuantrairong and Traichaiyaporn [63], who discovered that phytoplankton communities in Doi Tao Lake in northern Thailand had the highest and lowest Shannon-Weiner diversity indices in winter and summer, respectively. The flow of nutrients into water bodies and a decrease in water availability were the most critical determinants of algal blooms and spread throughout the dry season [64,65,66].
Figure 7 highlights the presence of the specific species found at each sampling location. In January, February, March and April, a common phytoplankton taxon was observed at all sampling sites. However, in May, no common species were recorded. The presence of Navicula sp. at every sample location in January, which was a cold dry season with the lowest water level during our investigation, implied moderate to poor water quality. This finding is consistent with those of previous research that found the prevalence of Navicula species is an indicator of pollution-related strain on the river. High levels of nutrients during the dry season, which is characterized by low flow conditions, higher evaporation rates and lower water levels, probably contributed to the development of these species [67]. However, in February, Nitzschia palea was found at all sampling locations. This species has been identified as an indicator of intermediate water quality in Thailand's Ping River [68]. Pediastrum duplex var. duplex was found at all stations during the March sampling. This species is generally found in water bodies with a water quality level ranging from oligo-mesotrophic to eutrophic and is known to be resistant to water contaminants and adaptable to various water quality levels [69].
Another notable aspect was the presence of different algal species at Stations S2 and S3, where the highest number of common species was identified during the study period. Despite the significant differences in water quality between the two sites, Station S2 had a higher concentration of DO, while Station S3 had higher concentrations of all three nutrients and chlorophyll a. Both sampling locations had similar species of phytoplankton, namely, Pediastrum spp. and Scenedesmus spp. It is important to note that although these species belong to the same genus, they represent different species. Pediastrum simplex var. sturmii and P. simplex var. clathratum were found in water with good to moderate (oligo-eutrophic) water quality, while P. alternans was found in water with an average mesotrophic status [67]. The presence of the genus Pediastrum spp. indicated a wide range of tolerance to water quality, as it occurred under both excellent and poor water quality conditions. In their study on the ecological habitat of Scenedesmus in northern Thailand, Phinyo et al. [70] reported the presence of Scenedesmus species and their association with water quality. S. parisiensis was discovered in oligotrophic water. S. acuminatus was found in oligo-mesotrophic water, while S. denticulatus was found in mesotrophic water. According to Peerapornpisal et al. [71], Pediastrum spp. and Scenedesmus spp. are commonly found in mesotrophic to eutrophic environments.
Furthermore, the highest concentration of cypermethrin in the water was observed at Station S4 in March. This station was found to have a unique group of phytoplankton, including Cosmarium sexangulare f. minama, Pleurotaenium trabecula and Closterium dianae var. minus, that was not present in other months. This finding revealed a possible link between the presence of cypermethrin and the identified phytoplankton species and that they may be used as aquatic bioindicators of cypermethrin residues, demonstrating their sensitivity to the presence of this chemical in water. Phacus sp. was discovered at all sample locations in April. Organic pollution is often associated with a large population of euglenoids, particularly Phacus sp. These taxa persist in high-nutrient water habitats such as ponds, marshes and ditches [72].
Figure 8 shows that the CCA results for Axes 1 and 2 accounted for 56.93% and 32.75% of the total variance in the dataset, respectively. The correlations between the cypermethrin residues, water quality parameters and phytoplankton species were classified into four groups. In Group 1, fish farming Station S3 was related to chlorophyll a, ammonia nitrogen and three algal species: Pediastrum simplex var. echinulatum, Pediastrum duplex var. duplex and Scenedesmus acutus. Furthermore, cypermethrin residues were shown to be strongly correlated with phytoplankton abundance and water quality parameters. The excretion of fish in fishponds leads to the accumulation of ammonia [73]. Nitrogen is generally considered the primary limiting nutrient for the growth and biomass of phytoplankton, which affects chlorophyll concentration. A study by Ding et al. [74] demonstrated that the nitrogen-to-phosphorus ratio decreased significantly at more than sixfold after an algal bloom. It is widely known that the persistence of pesticides in water bodies can be toxic to algae. In contrast, there have been reports that pesticides can also facilitate algal growth and be used as an energy source [75,76].
In Group 2, Mae Yian waterfall Station S1 was substantially related to conductivity, DO, pH and two species of benthic diatoms, Nitzschia palea and Synedra ulna (Figure 8). Benthic diatoms exhibit higher abundance in waterfalls characterized by higher water pH and conductivity due to the favorable conditions that promote the growth of diatoms resistant to such environmental parameters [68,77]. In Group 3, TCB and FCB had a significant correlation with Station S5, which was in a densely populated area close to a cattle farming area. Group 4 consisted of three locations, including the Lychee plantation station (S2), the agricultural cultivation station (S4), and the confluence of the Mae Yian and Mae Tum stream stations (S6). These stations were associated with BOD, nitrate nitrogen, water temperature, orthophosphate, water turbidity and five species of algae, including Coelastrum astroideum, Nitzschia sp., Navicula sp. and Phacus longicaudatus. In addition, a subcorrelation was observed at Station S6, where there was a close correlation with the turbidity of the water (Figure 8). Several studies have found that Coelastrum astroideum and Navicula sp. are commonly found in rivers and agricultural water bodies [78,79]. Additionally, research by Huang et al. [80] indicates that Nitzschia sp. and Navicula sp. are species frequently found in shallow and turbid water enriched with inorganic matter. At the same time, Phacus sp. is a species that tolerates high BOD conditions.
In addition, Pearson's correlation was applied to provide a more comprehensive analysis of the relationships between water quality indicators and cypermethrin residues. It was determined that the pH of the water was the only parameter with a significant negative correlation with the cypermethrin residue (Supplemental Table A2). The degradation of cypermethrin in water is influenced by variables such as pH, temperature and radiation exposure. In general, cypermethrin is more stable under acidic (lower pH) conditions and degrades faster under alkaline (higher pH) conditions. Lower pH levels may delay the degradation of cypermethrin, potentially resulting in higher residue levels in water [81,82]. Consistent with our findings, the highest levels of cypermethrin residue were detected at Station S4 in March (Figure 2), where the pH of the water was the lowest (Figure 3).
Pesticide residue in agricultural water is one of the most serious environmental challenges that harms biodiversity, particularly phytoplankton communities, which are the primary producers in aquatic food chains. In the present study, the residual cypermethrin, water quality and phytoplankton distribution were studied in a highly distinct location of a lychee plantation with variable elevations and land uses. The cypermethrin contamination in the surface water was highest in March, which corresponds to the period when farmers sprayed the highest amounts of cypermethrin. Based on the analysis of the water quality index (WQI), the water quality decreased significantly from upstream Station S1 to the flat area of Station S5. A total of 174 phytoplankton species were identified, the majority belonging to the Chlorophyta division, followed by Bacillariophyta and Cyanophyta. There were distinct correlations between cypermethrin residues, water quality and phytoplankton species at the different sites and in different months. The cypermethrin residues were highly associated with chlorophyll a and ammonium nitrogen, as well as with the abundance of three species of phytoplankton, Pediastrum simplex var. echinulatum, Pediastrum duplex var. duplex and Scenedesmus acutus at Station S3. These findings suggested that cypermethrin residues and water quality have a direct impact on the phytoplankton ecosystem. However, the distribution of phytoplankton is influenced by a multitude of environmental factors that vary by geographical location. For a comprehensive understanding, additional research on the effect of cypermethrin toxicity on the growth of these phytoplankton species is needed.
The authors declare they have not used Artificial Intelligence (AI) tools in the creation of this article.
This research project was supported by the Thailand science research and innovation fund and the University of Phayao (Grant No. FF65-RIM 1568850).
The authors have no conflicts of interest to declare.
[1] | G. J. Tearney, M. E. Brezinski and B. E. Bouma, In vivo endoscopic optical biopsy with optical coherence tomography, Science, 276 (1997), 2037–2039. |
[2] | R. William and Hendee, The impact of future technology on oncologic diagnosis: Oncologic imaging and diagnosis, Int. J. Radiat. Oncol. Biol. Phys., 9 (1983), 1851–1865. |
[3] | A. Heidenreich, F. Desgrandschamps and F. Terrier, Modern approach of diagnosis and management of acute flank pain: Review of all imaging modalities, Eur. Urol., 41 (2002), 351–362. |
[4] | R. E. Bunge and C. L. Herman, Usage of diagnostic imaging procedures: A nationwide hospital study, Radiology, 163 (1987), 569–573. |
[5] | G. B. A. Quekel, G. H. Kessels and R. Goei, Miss rate of lung cancer on the chest radiograph in clinical practice, Chest, 115 (1999), 720–724. |
[6] | F. Li, S. Shusuke and A. Hiroyuki, Lung cancers missed at low-dose helical CT screening in a general population: Comparison of clinical, histopathologic, and imaging findings, Radiology, 225 (2002), 673–683. |
[7] | Q. Li, F. Li and S. Kenji, Computer-aided diagnosis in thoracic CT, Semin. Ultrasound. Ct Mr, 26 (2005), 357–363. |
[8] | K. Suzuki, I. Sheu, M. Epstein, et al., An MTANN CAD for detection of polyps in false-negative CT colonography cases in a large multicenter clinical trial: Preliminary results, in Medical Imaging 2008: Computer-Aided Diagnosis, Med. Imaging. Int. Soc. Opt. Photonics., (2008). |
[9] | L. Ralph, Attempts to use computers as diagnostic aids in medical decision making: A thirty-year experience, Perspect. Biol. Med., 35(1992), 207–219. |
[10] | K. Doi, Current status and future potential of computer-aided diagnosis in medical imaging, Br. J. Radiol., 78(2005), S3–S19. |
[11] | K. Doi, Computer-aided diagnosis in medical imaging: Historical review, current status and future potential, Comput. Med. Imaging Graph., 31 (2007), 198–211. |
[12] | L. G. Maryellen, P. C. Heang and B. John, Anniversary paper: History and status of CAD and quantitative image analysis: The role of Medical Physics and AAPM, Med. Phys., 35 (2008), 5799–5820. |
[13] | K. Doi, H. Macmahon, S. Katsuragawa, et al., Computer-aided diagnosis in radiology: Potential and pitfalls, Eur. J. Radiol., 31 (1999), 97–109. |
[14] | K. Kerlikowske, P. A. Carney, B. Geller, et al., Performance of screening mammography among women with and without a first-degree relative with breast cancer, Ann. Intern. Med., 133 (2000), 855–863. |
[15] | H. Sittek, K. Herrmann, C. Perlet, et al., Computer-aided diagnosis in mammography, Der Radiologe, 37 (1997), 610–616. |
[16] | R. Takahashi and Y. Kajikawa, Computer-aided diagnosis: A survey with bibliometric analysis, Int. J. Med. Inf., 101 (2017), 58–67. |
[17] | A. Mansoor, U. Bagci, B. Foster, et al., Segmentation and image analysis of abnormal lungs at CT: Current approaches, challenges, and future trends, Radiographics, 35 (2015), 1056–1076. |
[18] | S. Kenji, Computer-aided detection of lung cancer, in image-based computer-assisted radiation therapy, Springer, (2017), 9–40. |
[19] | A. El-Baz, G. M. Beache, G. Gimel'Farb, et al., Computer-aided diagnosis systems for lung cancer: Challenges and methodologies, Int. J. Biomed. Imaging, 2013 (2013), 1–46. |
[20] | M. Nishio and C. Nagashima, Computer-aided diagnosis for lung cancer: Usefulness of nodule heterogeneity, Acad. Radiol., 24 (2017), 328–336. |
[21] | M. Kawagishi, B. Chen, D. Furukawa, et al., A study of computer-aided diagnosis for pulmonary nodule: Comparison between classification accuracies using calculated image features and imaging findings annotated by radiologists, Int. J. Comput. Assist. Radiol. Surg., 12 (2017), 1–10. |
[22] | A. O. D. C. Filho, A. C. Silva, A. C. D. Paiva, et al., Computer-aided diagnosis of lung nodules in computed tomography by using phylogenetic diversity, genetic algorithm, and SVM, J. Digit. Imaging, 30 (2017), 812–822. |
[23] | Y. Nomura, T. Higaki, M. Fujita, et al., Effects of iterative reconstruction algorithms on computer-assisted detection (CAD) software for lung nodules in ultra-low-dose CT for lung cancer screening, Acad. Radiol., 24 (2017), 124–130. |
[24] | M. Liang, W. Tang, D. M. Xu, et al., Low-dose CT screening for lung cancer: Computer-aided detection of missed lung cancers, Radiology, 281 (2016), 279–288. |
[25] | D. Shen, G. Wu and H. I. Suk, Deep learning in medical image analysis, Annu. Rev. Biomed. Eng., 19 (2017), 221–248. |
[26] | Q. Song, L. Zhao, X. Luo, et al., Using deep learning for classification of lung nodules on computed tomography images, J. Healthcare Eng., 2017 (2017), 1–7. |
[27] | Y. Lecun, L. Bottou, Y. Bengio, et al., Gradient-based learning applied to document recognition, Proc. IEEE, 86 (1998), 2278–2324. |
[28] | K. Alex, S. Ilya and E. H. Geoffrey, ImageNet classification with deep convolution neural networks, 25th International Conference on Neural Information Processing Sys tems, Curran Associates Inc., (2012), 1097–1105. Available from: https://dl.acm.org/ci tation.cfm?id=2999257. |
[29] | T. Xiao, J. X. Zhang, K. Y. Yang, et al., Error-driven Incremental learning in deep convolutional neural network for large-scale image classification, ACM Multimedia, (2014), 177–186. |
[30] | C. Szegedy, W. Liu, Y. Q. Jia, et al., Going Deeper with Convolutions, 2015 IEEE Conference on Computer Vision and Pattern Recognition (CVPR), IEEE, (2015), 1–9. Available from: https://ieeexplore.ieee.org/document/7298594. |
[31] | S. T. Wu, S. H. Zhong and Y. Liu, Deep residual learning for image steganalysis, Multimedia. Tools. Appl., 77 (2017), 10437–10453. |
[32] | K. M. He, X. Y. Zhang, S. Q. Ren, et al., Spatial Pyramid Pooling in Deep Convolutional Networks for Visual Recognition, in European Conference on Computer Vision (ECCV) 2014, Springer, (2014), 346–361. |
[33] | H. Greenspan, B. V. Ginneken and R. M. Summers, Guest Editorial Deep Learning in Medical Imaging: Overview and Future Promise of an Exciting New Technique, IEEE Trans. Med. Imaging, 35 (2016), 1153–1159. |
[34] | D. Wang, A. Khosla, R. Gargeya, et al., Deep Learning for Identifying Metastatic Breast Cancer, ArXiv 2016, (2016). |
[35] | D. Kumar, A. Wong and D. A. Clausi, Lung Nodule Classification Using Deep Features in CT Images, 12th Conference on Computer and Robot Vision, IEEE, (2015), 133–138. Available from: https://ieeexplore.ieee.org/document/7158331. |
[36] | F. Liu, C. Y. Wee, H. Chen, et al., Inter-modality relationship constrained multi-modality multi-task feature selection for Alzheimer's Disease and mild cognitive impairment identification, Neuroimage, 84 (2014), 466–475. |
[37] | H. I. Suk, S. W. Lee and D. Shen, Hierarchical feature representation and multimodal fusion with deep learning for AD/MCI diagnosis, Neuroimage, 101 (2014), 569–582. |
[38] | S. Liu, S. D. Liu, W. D. Cai, et al., Early diagnosis of Alzheimer's disease with deep learning, 11th International Symposium on Biomedical Imaging (ISBI), IEEE, (2014), 1015–1018. Available from: https://ieeexplore.ieee.org/abstract/document/6868045. |
[39] | H. Fujita and D. Cimr, Computer Aided detection for fibrillations and flutters using deep convolutional neural network, Inf. Sci., 486 (2019), 231–239. |
[40] | L. C. S. Afonso, G. H. Rosa, C. R. Pereira, et al., A recurrence plot-based approach for Parkinson's disease identification, Future Gener. Comput. Syst., 94 (2019), 282–292. |
[41] | W. Li, Y. Zhao, X. Chen, et al., Detecting Alzheimer's Disease on Small Dataset: A Knowledge Transfer Perspective, IEEE J. Biomed. Health. Inf., 23 (2019), 1234–1242. |
[42] | L. Martin, J. Jendeberg, P. Thunberg, et al., Computer aided detection of ureteral stones in thin slice computed tomography volumes using Convolutional Neural Networks, Comput. Biol. Med., 97 (2018), 153–160. |
[43] | I. Sajid, M. U. Ghani, T. Saba, et al., Brain tumor segmentation in multi-spectral MRI using convolutional neural networks (CNN), Microsc. Res. Tech., 81 (2018), 419–427. |
[44] | F. Z. Liao, L. Ming, L. Zhe, et al., Evaluate the Malignancy of Pulmonary Nodules Using the 3D Deep Leaky Noisy-or Network, IEEE Trans. Neural Netw. Learn. Syst., 14 (2017), 1–12. |
[45] | A. Rajkomar, S. Lingam, A. G. Taylor, et al., High-throughput classification of radiographs using deep convolutional neural networks, J. Digit. Imaging, 30 (2017), 95–101. |
[46] | Z. N. Yan, Y. Q. Zhan, Z. G. Peng, et al., Multi-Instance Deep Learning: Discover Discriminative Local Anatomies for Bodypart Recognition, IEEE Trans. Med. Imaging, 35 (2016), 1332–1343. |
[47] | H. Pratt, F. Coenen, D. M. Broadbent, et al., Convolutional Neural Networks for Diabetic Retinopathy, Procedia. Comput. Sci., 90 (2016), 200–205. |
[48] | M. Gao, U. Bagci, L. Lu, et al., Holistic classification of CT attenuation patterns for interstitial lung diseases via deep convolutional neural networks, Comput. Methods Biomech. Biomed. Eng. Imaging Vis., 6 (2016), 1–6. |
[49] | H. C. Shin, H. R. Roth, M. Gao, et al., Deep Convolutional Neural Networks for Computer-Aided Detection: CNN Architectures, Dataset Characteristics and Transfer Learning, IEEE Trans. Med. Imaging, 35 (2016), 1285–1298. |
[50] | O. Ronneberger, P. Fischer and T. Brox, U-Net: Convolutional Networks for Biomedical Image Segmentation, in MICCAI 2015, Springer, (2015), 234–241. |
[51] | O. F. Ahmad, A. Soares, E. B. Mazomenos, et al., Artificial intelligence and computer-aided diagnosis in colonoscopy: Current evidence and future directions, Lancet. Gastroenterol. Hepatol., 41 (2019), 71–80. |
[52] | I. González-Díaz, DermaKNet: Incorporating the Knowledge of Dermatologists to Convolutional Neural Networks for Skin Lesion Diagnosis, IEEE J. Biomed. Health. Inf., 23 (2019), 547–559. |
[53] | P. R. Jeyaraj, E. R. J. J. o. C. R. Samuel Nadar and C. Oncology, Computer-assisted medical image classification for early diagnosis of oral cancer employing deep learning algorithm, J. Cancer Res. Clin. Oncol., 145 (2019), 829–837. |
[54] | U. Raghavendra, H. Fujita, S. V. Bhandary, et al., Deep convolution neural network for accurate diagnosis of glaucoma using digital fundus images, Inf. Sci., 441 (2018), 41–49. |
[55] | U. Raghavendra, H. Fujita, A. Gudigar, et al., Automated technique for coronary artery disease characterization and classification using DD-DTDWT in ultrasound images, Biomed. Signal Process Control, 40 (2018), 324–334. |
[56] | E. Hosseini-Asl, M. Ghazal, A. Mahmoud, et al., Alzheimer's disease diagnostics by a 3D deeply supervised adaptable convolutional network, Front Biosci (Landmark Ed), 23 (2018), 584–596. |
[57] | A. Farooq, S. M. Anwar, M. Awais, et al., A deep CNN based multi-class classification of Alzheimer's disease using MRI, 2017 IEEE International Conference on Imaging System and Techniques (IST), IEEE, (2018), 182–187. Available from: https://ieeexplore.ieee.org/document/8261460. |
[58] | G. V. Tulder and M. D. Bruijne, Combining Generative and Discriminative Representation Learning for Lung CT Analysis With Convolutional Restricted Boltzmann Machines, IEEE Trans. Med. Imaging, 35 (2016), 1262–1272. |
[59] | M. Anthimopoulos, S. Christodoulidis, L. Ebner, et al., Lung Pattern Classification for Interstitial Lung Diseases Using a Deep Convolutional Neural Network, IEEE Trans. Med. Imaging, 35 (2016), 1207–1216. |
[60] | A. Jalalian, S. Mashohor, R. Mahmud, et al., Foundation and methodologies in computer-aided diagnosis systems for breast cancer detection, EXCLI J, 16 (2017), 113–137. |
[61] | M. L. Giger, N. Karssemeijer and J. A. Schnabel, Breast image analysis for risk assessment, detection, diagnosis, and treatment of cancer, Annu. Rev. Biomed. Eng., 15 (2013), 327–357. |
[62] | Q. H. Huang, F. B. Yang, L. Z. Liu, et al., Automatic segmentation of breast lesions for interaction in ultrasonic computer-aided diagnosis, Inf. Sci., 314 (2015), 293–310. |
[63] | T. C. Chiang, Y. S. Huang, R. T. Chen, et al., Tumor Detection in Automated Breast Ultrasound Using 3-D CNN and Prioritized Candidate Aggregation, IEEE Trans. Med. Imaging, 38 (2018), 240–249. |
[64] | R. K. Samala, H. Chan, L. Hadjiiski, et al., Breast Cancer Diagnosis in Digital Breast Tomosynthesis: Effects of Training Sample Size on Multi-Stage Transfer Learning Using Deep Neural Nets, IEEE Trans. Med. Imaging, 38 (2019), 686–696. |
[65] | A. S. Becker, M. Marcon, S. Ghafoor, et al., Deep Learning in Mammography: Diagnostic Accuracy of a Multipurpose Image Analysis Software in the Detection of Breast Cancer, Invest. Radiol., 52 (2017), 434–440. |
[66] | X. R. Zhou, T. Kano, H. Koyasu, et al., Automated assessment of breast tissue density in non-contrast 3D CT images without image segmentation based on a deep CNN, in Medical Imaging 2017: Computer-Aided Diagnosis, Proc. SPIE, (2017). |
[67] | T. Kooi, G. B. Van, N. Karssemeijer, et al., Discriminating Solitary Cysts from Soft Tissue Lesions in Mammography using a Pretrained Deep Convolutional Neural Network, Med. Phys., 44 (2017), 1017–1027. |
[68] | A. B. Ayelet, L. Karlinsky, S. Alpert, et al., A Region Based Convolutional Network for Tumor Detection and Classification in Breast Mammography, in Deep Learning and Data Labeling for Medical Applications, Springer, (2016), 197–205. |
[69] | J. G. Posada, D. M. Zapata and O. L. Q. Montoya, Detection and Diagnosis of Breast Tumors using Deep Convolutional Neural Networks, Conference Proceedings of the XVI I Latin American Conference on Automatic Control (2016), 11-17. Available from: http s://pdfs.semanticscholar.org/9566/d1f27a0e5f926827d3eaf8546dab51e40e21.pdf. |
[70] | R. K. Samala, H. P. Chan, L. Hadjiiski, et al., Mass detection in digital breast tomosynthesis: Deep convolutional neural network with transfer learning from mammography, Med. Phys., 43 (2016), 6654. |
[71] | N. Dhungel, G. Carneiro and A. P. Bradley, The Automated Learning of Deep Features for Breast Mass Classification from Mammograms, in MICCAI 2016,Springer, (2016), 106–114. |
[72] | Y. J. Zhou, J. X. Xu, Q. G. Liu, et al., A Radiomics Approach with CNN for Shear-wave Elastography Breast Tumor Classification, IEEE Trans. Biomed. Eng., 65 (2018), 1935–1942. |
[73] | F. Gao, T. Wu, J. Li, et al., SD-CNN: A Shallow-Deep CNN for Improved Breast Cancer Diagnosis, Comput Med Imaging Graph, 70 (2018), 53–62. |
[74] | J. Li, M. Fan, J. Zhang, et al., Discriminating between benign and malignant breast tumors using 3D convolutional neural network in dynamic contrast enhanced MR images, in Medical Imaging 2017: Imaging Informatics for Healthcare, Research, and Applications, Proc. SPIE, (2017). |
[75] | T. Kooi, G. Litjens, B. V. Ginneken, et al., Large scale deep learning for computer aided detection of mammographic lesions, Med. Image. Anal., 35 (2017), 303–312. |
[76] | R. Samala, H. P. Chan, L. Hadjiiski, et al., Deep-learning convolution neural network for computer-aided detection of microcalcifications in digital breast tomosynthesis, in Medical Imaging 2016: Computer-Aided Diagnosis, Proc. SPIE, (2016). |
[77] | D. M. Parkin, Global cancer statistics in the year 2000, Lancet Oncol., 2 (2001), 533–543. |
[78] | A. Motohiro, H. Ueda, H. Komatsu, et al., Prognosis of non-surgically treated, clinical stage I lung cancer patients in Japan, Lung Cancer, 36 (2002), 65–69. |
[79] | K. L. Hua, C. H. Hsu, S. C. Hidayati, et al., Computer-aided classification of lung nodules on computed tomography images via deep learning technique, Onco. Targets Ther., 8 (2015), 2015–2022. |
[80] | Z. H. Shi, H. Hao, M. H. Zhao, et al., A deep CNN based transfer learning method for false positive reduction, Multimed. Tools Appl., 78 (2018), 1017–1033. |
[81] | F. Ciompi, B. D. Hoop, S. J. V. Riel, et al., Automatic classification of pulmonary peri-fissural nodules in computed tomography using an ensemble of 2D views and a convolutional neural network out-of-the-box, Med. ImageAnal., 26 (2015), 195–202. |
[82] | M. Nishio, O. Sugiyama, M. Yakami, et al., Computer-aided diagnosis of lung nodule classification between benign nodule, primary lung cancer, and metastatic lung cancer at different image size using deep convolutional neural network with transfer learning, PLoS One, 13 (2018), e0200721. |
[83] | G. Savitha and P. Jidesh, Lung Nodule Identification and Classification from Distorted CT Images for Diagnosis and Detection of Lung Cancer, in Machine Intelligence and Signal Analysis, Springer, (2019), 11–23. |
[84] | X. Z. Zhao, L. Y. Liu, S. Qi, et al., Agile convolutional neural network for pulmonary nodule classification using CT images, Int. J. Comput. Assist Radiol. Surg., 13 (2018), 585–595. |
[85] | J. Ding, A. Li, Z. Q. Hu, et al., Accurate Pulmonary Nodule Detection in Computed Tomography Images Using Deep Convolutional Neural Networks, in MICCAI 2017,Springer, (2017), 559–567. |
[86] | Q. Dou, H. Chen, Y. M. Jin, et al., Automated Pulmonary Nodule Detection via 3D ConvNets with Online Sample Filtering and Hybrid-Loss Residual Learning, in MICCAI 2017,Springer, (2017), 630–638. |
[87] | J. Z. Cheng, D. Ni, Y. H. Chou, et al., Computer-Aided Diagnosis with Deep Learning Architecture: Applications to Breast Lesions in US Images and Pulmonary Nodules in CT Scans, Sci. Rep., 6 (2016), 24454. |
[88] | K. Liu and G. Kang, 3D multi-view convolutional neural networks for lung nodule classification, PLoS One, 12 (2017), e0188290. |
[89] | R. Dey, Z. J. Lu and H. Yi, Diagnostic Classification Of Lung Nodules Using 3D Neural Networks, 2018 IEEE 15th International Symposium on Biomedical Imaging (ISBI), IEEE, (2018), 774–778. Available from: https://ieeexplore.ieee.org/document/8363687. |
[90] | D. Anton, K. Ramil, K. Adil, et al., Large Residual Multiple View 3D CNN for False Positive Reduction in Pulmonary Nodule Detection, 2017 IEEE Conference on Computational Intelligence in Bioinformatics and Computational Biology (CIBCB), IEEE, (2017). Available from: https://ieeexplore.ieee.org/document/8058549. |
[91] | W. Li, P. Cao, D. Z. Zhao, et al., Pulmonary Nodule Classification with Deep Convolutional Neural Networks on Computed Tomography Images, Comput. Math. Method. Med., 2016 (2016), 1–7. |
[92] | R. L. Siegel, K. D. Miller and A. Jemal, Cancer Statistics, 2017, CA Cancer J. Clin., 67 (2017), 7–30. |
[93] | R. Chou, J. M. Croswell, D. Tracy, et al., Screening for prostate cancer: A review of the evidence for the U.S. Preventive Services Task Force, Ann. Intern. Med., 137 (2011), 55–73. |
[94] | A. Rampun, L. Zheng, P. Malcolm, et al., Computer-aided detection of prostate cancer in T2-weighted MRI within the peripheral zone, Phys. Med. Biol., 61 (2016), 4796–4825. |
[95] | W. Li, J. Li, K. V. Sarma, et al., Path R-CNN for Prostate Cancer Diagnosis and Gleason Grading of Histological Images, IEEE Trans. Med. Imaging, 38 (2018), 945–954. |
[96] | E. Leng, J. C. Henriksen, A. E. Rizzardi, et al., Signature maps for automatic identification of prostate cancer from colorimetric analysis of H&E and IHC-stained histopathological specimens, Sci. Rep., 9 (2019), 6992. |
[97] | Q. Chen, X. Xu, S. L. Hu, et al., A transfer learning approach for classification of clinical significant prostate cancers from mpMRI scans, in Medical Imaging 2017: Computer-Aided Diagnosis, Proc. SPIE, (2017). |
[98] | Y. Song, Y. D. Zhang, X. Yan, et al., Computer-aided diagnosis of prostate cancer using a deep convolutional neural network from multiparametric MRI, J. Magn. Reson. Imaging, 48 (2018), 1570–1577. |
[99] | Z. Wang, C. Liu, D. Cheng, et al., Automated Detection of Clinically Significant Prostate Cancer in mp-MRI Images Based on an End-to-End Deep Neural Network, IEEE Trans. Med. Imaging, 37 (2018), 1127–1139. |
[100] | J. Ishioka, Y. Matsuoka, S. Uehara, et al., Computer-aided diagnosis of prostate cancer on magnetic resonance imaging using a convolutional neural network algorithm, BJU Int., 122 (2018), 411–417. |
[101] | S. Kohl, D. Bonekamp, H.P. Schlemmer, et al., Adversarial Networks for the Detection of Aggressive Prostate Cancer, in ArXiv, (2017). Available from: https://arxiv.org/abs/17 02.08014. |
[102] | X. Yang, C. Y. Liu, Z. W. Wang, et al., Co-trained convolutional neural networks for automated detection of prostate cancer in multi-parametric MRI, Med. Image Anal., 42 (2017), 212–227. |
[103] | T. K. Jin and S. M. Hewitt, Nuclear Architecture Analysis of Prostate Cancer via Convolutional Neural Networks, IEEE Access, 5 (2017), 18526–18533. |
[104] | X. Wang, W. Yang, J. Weinreb, et al., Searching for prostate cancer by fully automated magnetic resonance imaging classification: Deep learning versus non-deep learning, Sci. Rep., 7 (2017), 15415. |
[105] | S. F. Liu, H. X. Zheng, Y. Feng, et al., Prostate Cancer Diagnosis using Deep Learning with 3D Multiparametric MRI, in Medical Imaging 2017: Computer-Aided Diagnosis, Proc. SPIE, (2017). |
[106] | M. H. Le, J. Y. Chen, L. Wang, et al., Automated diagnosis of prostate cancer in multi-parametric MRI based on multimodal convolutional neural networks, Phys. Med. Biol., 62 (2017), 6497–6514. |
[107] | X. Yang, Z. W. Wang, C. Y. Liu, et al., Joint Detection and Diagnosis of Prostate Cancer in Multi-parametric MRI Based on Multimodal Convolutional Neural Networks, in MICCAI 2017,Springer, (2017), 426–434. |
[108] | M. F. McNitt-Gray, S. G. Armato, C. R. Meyer, et al., The Lung Image Database Consortium (LIDC) data collection process for nodule detection and annotation, Acad. Radiol., 14 (2007), 1464–1474. |
[109] | A. P. Reeves, A. M. Biancardi, D. Yankelevitz, et al., A public image database to support research in computer aided diagnosis, Conf. Proc. IEEE Eng. Med. Biol. Soc., 2009(2009), 3715–3718. |
[110] | K. S. Man, C. Ramachandran, A. Yianni, et al., Automatic pectoral muscle segmentation on mediolateral oblique view mammograms, IEEE Trans. Med. Imaging, 23 (2004), 1129–1140. |
[111] | D. Saraswathi and E. Srinivasan, An ensemble approach to diagnose breast cancer using fully complex-valued relaxation neural network classifier, Int. J. Biomed. Eng. Technol., 15 (2014), 243–260. |
[112] | M. Lamard, G. Cazuguel, G. Quellec, et al., Content Based Image Retrieval based on Wavelet Transform coefficients distribution, 2007 29th Annual International Conference of the IEEE Engineering in Medicine and Biology Society, IEEE, (2007). Available from: https://ieeexplore.ieee.org/document/4353347. |
[113] | G. D. Tourassi, in Intelligent Paradigms for Healthcare Enterprises, Current Status of Computerized Decision Support Systems in Mammography, Springer, (2005), 173–208. |
[114] | R. M. Rangayyan, F. J. Ayres and J. E. L. Desautels, A review of computer-aided diagnosis of breast cancer: Toward the detection of subtle signs, J. Franklin Inst., 344 (2007), 312–348. |
[115] | M. Sundaram, K. Ramar, N. Arumugam, et al., Histogram Modified Local Contrast Enhancement for mammogram images, Appl. Soft. Comput., 11 (2011), 5809–5816. |
[116] | P. M. Bolton, S. L. James, J. M. Davidson, et al., Proceedings: Diagnostic and prognostic significance of immune competence testing in patients with breast cancer, Br. J. Surg., 61 (1974), 325–326. |
[117] | N. Pérez, M. A. Guevara and A. Silva, Improving Breast Cancer Classification with Mammography, supported on an appropriate Variable Selection Analysis, in Medical Imaging 2013: Computer-Aided Diagnosis, Proc. SPIE, (2013). |
[118] | T. Messay, R. C. Hardie and T. R. Tuinstra, Segmentation of pulmonary nodules in computed tomography using a regression neural network approach and its application to the Lung Image Database Consortium and Image Database Resource Initiative dataset, Med. Image Anal., 22 (2015), 48–62. |
[119] | W. S. Wang, J. W. Luo, X. D. Yang, et al., Data analysis of the Lung Imaging Database Consortium and Image Database Resource Initiative, Acad. Radiol., 22 (2015), 488–495. |
[120] | Y. Rowena, C. I. Henschke, D. F. Yankelevitz, et al., CT screening for lung cancer: Alternative definitions of positive test result based on the national lung screening trial and international early lung cancer action program databases, Radiology, 273 (2014), 591–596. |
[121] | M. Oudkerk and M. A. Heuvelmans, Screening for lung cancer by imaging: The Nelson study, JBR-BTR, 96 (2013), 163–166. |
[122] | Z. Y. Ru, X. Xie, H. J. d. Koning, et al., NELSON lung cancer screening study, Cancer Imaging, 11 (2011), S79–S84. |
[123] | G. B. Van, A. V. D. Van, T. Duindam, et al., Comparing and combining algorithms for computer-aided detection of pulmonary nodules in computed tomography scans: The ANODE09 study, Med. Image Anal., 14 (2010), 707–722. |
[124] | L. Geert, D. Oscar, B. Jelle, et al., Computer-aided detection of prostate cancer in MRI, IEEE Trans. Med. Imaging, 33 (2014), 1083–1092. |
[125] | K. W. Clark, B. A. Vendt, K. E. Smith, et al., The Cancer Imaging Archive (TCIA): Maintaining and Operating a Public Information Repository, J. Digit. Imaging, 26 (2013), 1045–1057. |
[126] | V. M. Gonçalves, M. E. Delamaro and F. L. S. Nunes, A systematic review on the evaluation and characteristics of computer-aided diagnosis systems, Rev. Bras. Eng. Bioméd., 30 (2014), 355–383. |
[127] | J. Ma, F. Wu, J. Zhu, et al., A pre-trained convolutional neural network based method for thyroid nodule diagnosis, Ultrasonics, 73 (2017), 221–230. |
[128] | W. Sun, T. B. Tseng, J. Zhang, et al., Enhancing deep convolutional neural network scheme for breast cancer diagnosis with unlabeled data, Comput. Med. Imaging Graph., 57 (2017), 4–9. |
[129] | W. L. Chen, Y. Zhang, J. J. He, et al., Prostate Segmentation using 2D Bridged U-net, ArXiv 2018, (2018). |
[130] | F. Milletari, N. Navab and S. A. Ahmadi, V-Net: Fully Convolutional Neural Networks for Volumetric Medical Image Segmentation, 2016 Fourth International Conference on 3D Vision (3DV), IEEE, (2016). Available from: https://ieeexplore.ieee.org/document/7785132. |
[131] | S. Hussain, S. M. Anwar and M. Majid, Segmentation of glioma tumors in brain using deep convolutional neural network, Neurocomputing, 282 (2017), 248–261. |
[132] | R. Caruana, in Machine Learning, Multitask Learning, Springer, (1997), 41–75. |
[133] | I. Guyon, G. Dror, V. Lemaire, et al., Unsupervised and transfer learning challenge, The 2011 International Joint Conference on Neural Networks, IEEE, (2011).Available from: https://ieeexplore.ieee.org/document/6033302. |
[134] | R. J. Gillies, P. E. Kinahan and H. Hricak, Radiomics: Images Are More than Pictures, They Are Data, Radiology, 278 (2016), 563–577. |
[135] | T. Tran and R. Kavuluru, Predicting Mental Conditions Based on "History of Present Illness" in Psychiatric Notes with Deep Neural Networks, J. Biomed. Inf., 75 (2017), S138–S148. |
1. | Prithu Baruah, Akanksha Srivastava, Yogesh Mishra, Neha Chaurasia, Modulation in growth, oxidative stress, photosynthesis, and morphology reveals higher toxicity of alpha-cypermethrin than chlorpyrifos towards a non-target green alga at high doses, 2024, 106, 13826689, 104376, 10.1016/j.etap.2024.104376 | |
2. | Sana Ullah, Amina Zuberi, Imdad Ullah, Mahmoud M. Azzam, Ameliorative Role of Vitamin C against Cypermethrin Induced Oxidative Stress and DNA Damage in Labeo rohita (Hamilton, 1822) Using Single Cell Gel Electrophoresis, 2024, 12, 2305-6304, 664, 10.3390/toxics12090664 | |
3. | Arledys Albino-Bohórquez, Yeisson Gutiérrez, German Bohórquez, Tito Bacca, Integrated analysis of lethal and sublethal effects of rice pesticides on the natural predator Microvelia pulchella (Insecta: Hemiptera: Veliidae): insights into direct and indirect effects, 2025, 0730-7268, 10.1093/etojnl/vgaf076 |
Cypermethrin (mg/L) | % Recovery | RSD |
0.5 | 98.39 | 0.32 |
1.0 | 101.12 | 0.35 |
10.0 | 101.07 | 0.18 |
LOD | 0.08 | |
LOQ | 0.26 |