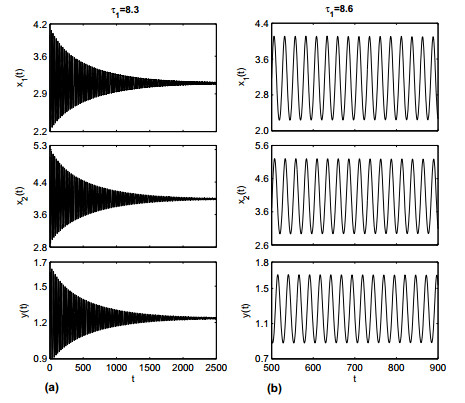
Citation: Qian Zhang, Haigang Li. An improved least squares SVM with adaptive PSO for the prediction of coal spontaneous combustion[J]. Mathematical Biosciences and Engineering, 2019, 16(4): 3169-3182. doi: 10.3934/mbe.2019157
[1] | Xiaoqiang Dai, Kuicheng Sheng, Fangzhou Shu . Ship power load forecasting based on PSO-SVM. Mathematical Biosciences and Engineering, 2022, 19(5): 4547-4567. doi: 10.3934/mbe.2022210 |
[2] | Xiao Liang, Taiyue Qi, Zhiyi Jin, Wangping Qian . Hybrid support vector machine optimization model for inversion of tunnel transient electromagnetic method. Mathematical Biosciences and Engineering, 2020, 17(4): 3998-4017. doi: 10.3934/mbe.2020221 |
[3] | Bei Liu, Hongzi Bai, Wei Chen, Huaquan Chen, Zhen Zhang . Automatic detection method of epileptic seizures based on IRCMDE and PSO-SVM. Mathematical Biosciences and Engineering, 2023, 20(5): 9349-9363. doi: 10.3934/mbe.2023410 |
[4] | Xin Zhou, Shangbo Zhou, Yuxiao Han, Shufang Zhu . Lévy flight-based inverse adaptive comprehensive learning particle swarm optimization. Mathematical Biosciences and Engineering, 2022, 19(5): 5241-5268. doi: 10.3934/mbe.2022246 |
[5] | Ming Zhu, Kai Wu, Yuanzhen Zhou, Zeyu Wang, Junfeng Qiao, Yong Wang, Xing Fan, Yonghong Nong, Wenhua Zi . Prediction of cooling moisture content after cut tobacco drying process based on a particle swarm optimization-extreme learning machine algorithm. Mathematical Biosciences and Engineering, 2021, 18(3): 2496-2507. doi: 10.3934/mbe.2021127 |
[6] | Dashe Li, Xueying Wang, Jiajun Sun, Huanhai Yang . AI-HydSu: An advanced hybrid approach using support vector regression and particle swarm optimization for dissolved oxygen forecasting. Mathematical Biosciences and Engineering, 2021, 18(4): 3646-3666. doi: 10.3934/mbe.2021182 |
[7] | Cong Wang, Chang Liu, Mengliang Liao, Qi Yang . An enhanced diagnosis method for weak fault features of bearing acoustic emission signal based on compressed sensing. Mathematical Biosciences and Engineering, 2021, 18(2): 1670-1688. doi: 10.3934/mbe.2021086 |
[8] | Zhanying Tong, Yingying Zhou, Ke Xu . An intelligent scheduling control method for smart grid based on deep learning. Mathematical Biosciences and Engineering, 2023, 20(5): 7679-7695. doi: 10.3934/mbe.2023331 |
[9] | Yunpeng Ma, Shilin Liu, Shan Gao, Chenheng Xu, Wenbo Guo . Optimizing boiler combustion parameters based on evolution teaching-learning-based optimization algorithm for reducing NOx emission concentration. Mathematical Biosciences and Engineering, 2023, 20(11): 20317-20344. doi: 10.3934/mbe.2023899 |
[10] | Rongmei Geng, Renxin Ji, Shuanjin Zi . Research on task allocation of UAV cluster based on particle swarm quantization algorithm. Mathematical Biosciences and Engineering, 2023, 20(1): 18-33. doi: 10.3934/mbe.2023002 |
In the real world, many species have two distinctive stages---immature and mature, of life in their lives. A delayed single-specie model with two stages is introduced by Aiello and Freedman [1,2] in 1990. A single-specie model with stage-structured is considered by Wang and Chen [3] in 1997, and found that there exists a stable periodic solution in that model. The single-specie model with two stage-structured have been received much attentions and summarized by Liu et al. [4]. In these papers, the authors assume that the species have two different stages---immature and mature, and only the mature member can reproduce themselves. But, some species go through three different life stages---immature, mature and old. A single-specie model with delay and three different life history stages and cannibalism has investigated by Gao [5], and shown that there would be a stability switches for the positive equilibrium when time delays are increased from zero. A nonautonomous predator-prey system (1.1)
{x′(t)=x(t)[a(t)−b(t)x(t)−c(t)y2(t)−d(t)y3(t)],y′1(t)=α(t)x(t)y3(t−τ)−β1(t)y1(t)−γ1(t)y1(t),y′2(t)=γ1(t)y1(t)−β2(t)y2(t)−γ2(t)y2(t)−η1(t)y22(t),y′3(t)=γ2(t)y2(t)−η2(t)y3(t), | (1.1) |
with three-stage-structured and time delay has considered by Yang and Shi [6], and the conditions for the existence of the positive periodic solution are obtained.
Time delays play an important role in population dynamics, which can cause the loss of stability of the equilibrium, bifurcate various types of periodic solutions, unbounded solutions and even chaotic solutions. Time delay is common in biodynamic systems[7], and harmful delays can cause fluctuation(period solution) in population density, and which would make the system subject to chaotic oscillation, unstable oscillation and extinct [8,9,10,11,12,13,14,15], even the time delay is very small.
Recently, a prey-predator model (1.2)
{x′1(t)=αx2(t)−(γ1+Ω)x1(t)−ηx21(t)−Ex1(t)y(t−τ2),x′2(t)=Ωx1(t)−(θ1+a)x2(t),x′3(t)=ax2(t)−bx3(t),y′(t)=kEx1(t−τ1)y(t)−dy(t)−fy2(t), | (1.2) |
with three stage structure and time delay is studied in [16]. The conditions for the positive equilibrium occurring local and global Hopf bifurcation are obtained. And the properties (direction, stability, etc) of the local Hopf bifurcation are analyzed. Furthermore, a prey-predator system (1.3)
{x′1(t)=αx2(t)−(γ1+Ω)x1(t)−ηx21(t)−Ex1(t)y(t),x′2(t)=Ωx1(t)−(θ1+a)x2(t),x′3(t)=ax2(t)−bx3(t),y′(t)=y(t)[kEx1(t)−d−fy(t−τ)], | (1.3) |
with three stage structure and predator density dependent delay has been considered in [17,18], by choosing time delay as a bifurcation parameter, the local and global Hopf bifurcation are investigated. The authors focus on the existence of global Hopf bifurcation in systems (1.2) and (1.3), by using the global Hopf bifurcating theorem for general functional differential equations which introduced by Wu [19]. Meanwhile, the harsh conditions for the positive equilibrium occurring local Hopf bifurcation are obtained, i.e. there are only a pair of pure imaginary roots for the characteristic equation about the positive equilibrium.
Note that, the sufficient conditions for the existence of local Hopf bifurcation of systems (1.2) and (1.3) are C13:fη<KE2, C23:fη>KE2, respectively, where K,E,η,f are positive. K is the rate of conversing prey into predator and E is the predation coefficient for predator population. η is the density dependent coefficient for prey populations, reflecting the competition effect between prey populations; and f is the density dependent coefficient for predator population, reflecting the competition effect between predator populations; respectively. But, the conditions C13 and C23 cannot hold at the same time. Then, one of them holds for any parameter values of the system exclude the special case fη=KE2, if both digestion delay and density dependent delay considered in a new model. Therefore, there would be a natural Hopf bifurcation for the system with two different time delays τ1 and τ2 without any conditions for the values of the parameters. And, how does the dynamic behavior go when τ1=τ2=τ? Does there exist a bifurcating periodic solution, stability switches or other complex dynamic behaviors, if there exist at least a pair of pure imaginary roots for the characteristic equation about the positive equilibrium?
Motivation by aforementioned observations, we consider the following prey-predator model with three stage structure and two time delays:
{x′1(t)=αx2(t)−x1(t)(γ1+Ω+ηx1(t)+Ey(t)),x′2(t)=Ωx1(t)−θ1x2(t)−ax2(t),x′3(t)=ax2(t)−bx3(t),y′(t)=y(t)(KEx1(t−τ1)−d−fy(t−τ2)), | (1.4) |
where x′1(t),x′2(t),x′3(t) are the change of density of the prey population in the three stages of immature, mature and old, and y′(t) is the change of density of the predator population at time t, respectively. All of the parameters are positive. For prey population, α is the birth rate; γ1,θ1,b are the death rate of the immature, mature and old stages; Ω and a are the maturity rate and ageing rate, respectively. For predator population, d is the death rate; τ1 and τ2 are digestion delay [16] and density dependent delay [17,18], respectively. The delays τ1 and τ2 in system (1.4) can be regarded as a digestion time(or conversion time) and density dependent time of the predators. For τ1, when the predator catches the prey at time t, it needs τ1 time to convert the energy of the prey into its own energy. For τ2, the competition between predator populations has a time delay τ2, as in classical delayed Logistic equation x′(t)=rx(t)[1−x(t−τ)/K]. That is to say, the change rate of the predators y′(t) depends on the number of immature preys and of predators present at some previous time x1(t−τ1) and y(t−τ2), respectively.
From the third equation of system (1.4), which is a linear nonhomogeneous equation about x3(t), then the asymptotic behavior of x3(t) is dependent on x2(t). Therefore, we only need to consider the following subsystem
{x′1(t)=αx2(t)−x1(t)(γ+ηx1(t)+Ey(t)),x′2(t)=Ωx1(t)−θx2(t),y′(t)=y(t)(KEx1(t−τ1)−d−fy(t−τ2)), | (1.5) |
where γ=γ1+Ω,θ=θ1+a. And the initial conditions for system (1.5) are
xi(t)=φi(t)≥0(i=1,2),y(t)=φ3(t)≥0,t∈[−τmax,0],τmax=max{τ1,τ2}. |
The organization of this paper is as follows. We consider the stability of the equilibrium point and the existence of Hopf bifurcation, by choosing time delays as a bifurcation parameter in five different cases, firstly. And, in section 2, the conditions for the positive equilibrium occurring local Hopf bifurcation are obtained in each case. Secondly, in section 3, some numerical examples are given to support the theoretical results, which show that the delayed system considered has not only periodic oscillation, stability switches but also chaotic oscillation, even unbounded oscillation under some parameter sets of values. Finally, in section 4, delays induced Hopf bifurcation, stability switches, complicated dynamic behaviors of the system are analyzed in detail.
For system (1.5), if condition C1:αΩ−γθ>0 holds, there're two boundary equilibrium E0=(0,0,0),E1(ˆx1,ˆx2,0); and if condition C2:KEx∗1−d>0 holds, a unique positive equilibrium E2(x∗1,x∗2,y∗) exists, where
ˆx1=αΩ−γθηθ,ˆx2=Ωθx1,x∗1=f(αΩ−γθ)+dEθ(KE2+ηf)θ,x∗2=Ωθx∗1,y∗=KEx∗1−df. |
Let X(t)=(x1(t),x2(t),y(t)), and ˉE=(ˉx1,ˉx2,ˉy) be any arbitrary equilibrium. The linearized equation about ˉE is
X′(t)=AX(t)+B1X(t−τ1)+B2X(t−τ2), | (2.1) |
where
A=(−γ−2ηˉx1−ˉyEα−ˉx1EΩ−θ000ˉx1KE−d−ˉyf), |
B1=(000000KˉyE00),B2=(00000000−ˉyf), |
and the characteristic equation about it is given by
H(λ,τ1,τ2)=det(A+B1e−λτ1+B2e−λτ2−λI)=0. | (2.2) |
Note that, ˉy=0 for the boundary equilibrium E0 and E1, then the characteristic equation about E0 and E1 are same as in [16,17]. Therefore, we obtain following lemma.
Lemma 2.1. (i) If γθ>αΩ then E0 is local stable. And, if γθ<αΩ then E0 is unstable and E1 exists.
(ii) If KEˆx1<d then E1 is local stable. And if KEˆx1>d then E1 is unstable and E2 exists.
From (2.2), one obtain the characteristic equation about the positive equilibrium E2:
H(λ,τ1,τ2)=M(λ)+N(λ)e−λτ1+P(λ)e−λτ2=0, | (2.3) |
where
M(λ)=λ3+m2λ2+m1λ+m0,N(λ)=n2λ2+n1λ+n0,P(λ)=p2λ2+p1λ+p0,m2=γ+Ey∗+θ+2ηx∗1,m1=θηx∗1,m0=0,n2=0,n1=KE2x∗1y∗,n0=KE2x∗1y∗θ,p2=fy∗,p1=fy∗(γ+Ey∗+θ+2ηx∗1),p0=fy∗θηx∗1. |
When τ1=τ2=0, (2.3) becomes to
H(λ,0,0)=λ3+h2λ2+h1λ+h0=0, | (2.4) |
where
h2=γ+2ηx∗1+Ey∗+θ+fy∗>0,h1=θ(ηx∗1+fy∗)+fy∗(γ+2ηx∗1+Ey∗)+KE2x∗1y∗>0,h0=θ(fη+KE2)x∗1y∗>0. |
By Routh-Hurwits criterion, all roots of (2.4) have negative real parts, since
h2h1−h0>θ{[2ηfx∗1y∗+Ey∗(d+2fy∗)]−(fη+KE2)x∗1y∗}>0. |
Meanwhile, E2 is local stable. We investigate the Hopf bifurcation about E2 in following five cases.
The equation (2.3) is
H(λ,τ1,0)=Mτ1(λ)+Nτ1(λ)e−λτ1=0, | (2.5) |
where
Mτ1(λ)=M(λ)+P(λ),Nτ1(λ)=N(λ). |
Suppose λ=iω(ω>0) is a pure imaginary root of (2.5) and separating the real and imaginary parts, one obtain
{(m2+p2)ω2−(m0+p0)=(n0−n2ω2)cosωτ1+n1ωsinωτ1,ω3−(m1+p1)ω=n1ωcosωτ1−(n0−n2ω2)sinωτ1. |
and
(n0−n2ω2)2+n21ω2=[(m2+p2)ω2−(m0+p0)]2+[ω3−(m1+p1)ω]2. |
That is
Fτ1(ϖ)=ϖ3+f12ϖ2+f11ϖ+f10=0, | (2.6) |
where
ϖ=ω2,f12=(m2+p2)2−2(m1+p1)−n22>0,f11=(m1+p1)2+2n2n0−n21−2(m2+p2)(m0+p0),f10=(m0+p0)2−n20=θx∗1y∗(m0+p0+n0)(fη−KE2). | (2.7) |
If condition C13:fη<KE2 holds, from (2.7) we know that (2.6) has at least one positive root. Without loss of generality, we assume that (2.6) has three different positive roots, denoted by ωk=√ϖk(k=1,2,3). And, one have
cosωkτ1=[(m2+p2)ω2k−(m0+p0)](n0−n2ω2k)+n1ωk[ω3k−(m1+p1)ωk](n0−n2ω2k)2+(n1ωk)2Δ=Fωk. |
Thus
τ(n)1k=1ωkcos−1[Fωk]+2nπωk,k=1,2,3;n=0,1,2,⋯, | (2.8) |
and the direction of τ(n)1k passing through the imaginary axis [20] when ω=ωk is determined by
sign[dRe(λ(τ))dτ|τ=τ(n)1k]=sign[F′τ1(ϖk)|ϖk=ω2k]=sign(Δkτ1). |
Then sign(Δkτ1)≠0, since ϖk(k=1,2,3) are three distinct positive roots of (2.6). Therefore, system (1.5) undergoes a local Hopf bifurcation at E2 when τ1=τ(n)1k, by the Hopf bifurcation theorem for functional differential equations [21]. Furthermore, system (1.5) undergoes a local Hopf bifurcation at E2 and sign(Δ1τ1)=1, if f11>0 and condition C13:fη<KE2 hold. Then, (2.6) has a unique positive root ω1, and τ1=τ(n)1(n=0,1,2,⋯) corresponding to ω1.
Define
Sτ1={τ1|H(λ,τ1,0)=0,Re(λ)<0},τ10=min{τ(n)1k|1≤k≤3,n=0,1,2,⋯}, |
when τ1∈Sτ1, E2 is local stable. Note that, if (2.6) have more than one positive roots, there would be finite stability switches when time delay τ1 passing through the critical points τ1=τ(n)1k(k=1,2,3;n=0,1,2,⋯) and [0,τ10)⊆Sτ1. If (2.6) has only one positive root, there is no stability switches when time delay τ1 passing through the critical points τ1=τ(n)1(n=1,2,⋯) and Sτ1=[0,τ(0)1).
Theorem 2.1 (i) Suppose (2.6) has at least one positive roots denoted by ϖk(1≤k≤3). There exists a nonempty set Sτ1 and [0,τ10)⊆Sτ1, when τ1∈Sτ1 the positive equilibrium E2 of system (1.5) is local stable. There is a Hopf bifurcation for system (1.5) at E2 when τ1=τ(n)1k(k=1,2,3;n=0,1,2,⋯).
(ii) Suppose (2.6) has only one positive root denoted by ϖ1. There exists a nonempty set Sτ1 and Sτ1=[0,τ(0)1), when τ1∈Sτ1 the positive equilibrium E2 of system (1.5) is local stable and unstable when τ1>τ(0)1. There is a Hopf bifurcation for system (1.5) at E2 when τ1=τ(n)1(n=0,1,2,⋯).
Note 2.1 If f11>0 and condition C13:fη<KE2 hold, then (2.6) have only one positive root, and this is a special case of Theorem 2.1 (ii). The local and global Hopf bifurcation in this special situation have been considered in [16]. Meanwhile, theorem 2.1 generalizes the result about local Hopf bifurcation in [16].
The equation (2.3) becomes to
H(λ,0,τ2)=Mτ2(λ)+Nτ2(λ)e−λτ2=0, | (2.9) |
where
Mτ2(λ)=M(λ)+N(λ),Nτ2(λ)=P(λ). |
Suppose λ=iω(ω>0) is a pure imaginary root of (2.9), similar to the case 2.2.1, one have
Fτ2(ϖ)=ϖ3+f22ϖ2+f21ϖ+f20=0, | (2.10) |
where
ϖ=ω2,f22=(m2+n2)2−2(m1+n1)−p22, |
f21=(m1+n1)2+2p2p0−p21−2(m2+n2)(m0+n0), |
f20=(m0+n0)2−p20=θx∗1y∗(m0+p0+n0)(KE2−fη). | (2.11) |
From (2.11) we know that (2.10) has at least one positive root, if condition C23:fη>KE2 hold. Without loss of generality, we assume that (2.10) has three distinct positive roots, denoted by ωk=√ϖk(k=1,2,3) and we obtain
cosωkτ2=[(m2+n2)ω2k−(m0+n0)](p0−p2ω2k)+p1ωk[ω3k−(m1+n1)ωk](p0−p2ω2k)2+(p1ωk)2Δ=Fωk. |
Thus
τ(n)2k=1ωkcos−1[Fωk]+2nπωk,k=1,2,3;n=0,1,2,⋯, | (2.12) |
and the direction of τ(n)2k passing through the imaginary axis [20] when ω=ωk is determined by
sign[dRe(λ(τ))dτ|τ=τ(n)2k]=sign[F′τ2(ϖk)|ϖk=ω2k]=sign(Δkτ2). |
System (1.5) undergoes a Hopf bifurcation at E2 when τ2=τ(n)2k since sign(Δkτ2)≠0. Furthermore, if f21>0,f22>0 and condition C23:fη>KE2 hold, then (2.10) has a unique positive root ω1, and τ2=τ(n)2(n=0,1,2,⋯) corresponding to ω1. There is a Hopf bifurcation at E2 since sign(Δ1τ2)=1.
Define
Sτ2={τ2|H(λ,0,τ2)=0,Re(λ)<0},τ20=min{τ(n)2k|1≤k≤3,n=0,1,2,⋯}. |
Theorem 2.2 (i) Suppose (2.10) has at least one positive roots denoted by ϖk(1≤k≤3). There exists a nonempty set Sτ2 and [0,τ20)⊆Sτ2, when τ2∈Sτ2 the positive equilibrium E2 of system (1.5) is local stable. There is a Hopf bifurcation for system (1.5) at E2 when τ2=τ(n)2k(k=1,2,3;n=0,1,2,⋯).
(ii) Suppose (2.10) has only one positive root denoted by ϖ1. There exists a nonempty set Sτ2 and Sτ2=[0,τ(0)2), when τ2∈Sτ2 the positive equilibrium E2 of (1.5) is local stable and unstable when τ2>τ(0)2. There is a Hopf bifurcation for system (1.5) at E2 when τ2=τ(n)2(n=0,1,2,⋯).
Note 2.2 If f21>0,f22>0 and condition C23:fη>KE2 hold, then (2.10) has only one positive root, and this is a special case of Theorem 2.2 (ii). The local and global Hopf bifurcation in this special situation have been considered in [17,18]. Meanwhile, theorem 2.2 generalizes the result about local Hopf bifurcation in [17].
The equation (2.3) is
H(λ,τ,τ)=Mτ(λ)+Nτ(λ)e−λτ=0, | (2.13) |
where
Mτ(λ)=M(λ),Nτ(λ)=P(λ)+N(λ). |
Suppose λ=iω(ω>0) is a pure imaginary root of (2.13), similar to the case 2.2.1, we have
Fτ(ϖ)=ϖ3+f32ϖ2+f31ϖ+f30=0, | (2.14) |
where
ϖ=ω2,f32=m22−2m1−(n2+p2)2, |
f31=m21+2(p2+n2)(p0+n0)−(p1+n1)2−2m2m0, |
f30=m20−(p0+n0)2=−(p0+n0)2<0. |
(2.14) has at least one positive root since f30<0. Without loss of generality, we assume that (2.14) has three different positive roots, denoted by ωk=√ϖk(k=1,2,3) and we get
cosωkτ=(m2ω2k−m0)[p0+n0−(p2+n2)ω2k]+(p1+n1)ωk(ω3k−m1ωk)[(p0+n0)−(p2+n2)ω2k]2+[(p1+n1)ωk]2Δ=Fωk. |
Thus
τ(n)k=1ωkcos−1[Fωk]+2nπωk,k=1,2,3;n=0,1,2,⋯, | (2.15) |
and the direction of τ(n)k passing through the imaginary axis [20] when ω=ωk is determined by
sign[dRe(λ(τ))dτ|τ=τ(n)k]=sign[F′τ(ϖk)|ϖk=ω2k]=sign(Δkτ). |
System (1.5) undergoes a Hopf bifurcation at E2 when τ=τ(n)k. Furthermore, if f31>0,f32>0 hold, then (2.14) has a unique positive root ω1, and τ=τ(n)(n=0,1,2,⋯) corresponding to ω1. There is a Hopf bifurcation at the positive equilibrium E2 since sign(Δ1τ)=1.
Define
Sτ={τ|H(λ,τ,τ)=0,Re(λ)<0},τ0=min{τ(n)k|1≤k≤3,n=0,1,2,⋯}. |
Theorem 2.3 (i) Suppose (2.14) has at least one positive roots denoted by ϖk(1≤k≤3). There exists a nonempty set Sτ and [0,τ0)⊆Sτ, when τ∈Sτ the positive equilibrium E2 of system (1.5) is local stable. There is a Hopf bifurcation for system (1.5) at E2 when τ=τ(n)k(k=1,2,3;n=0,1,2,⋯).
(ii) Suppose (2.14) has only one positive root denoted by ϖ1. There exists a nonempty set Sτ and Sτ=[0,τ(0)), when τ∈Sτ the positive equilibrium E2 of system (1.5) is local stable and unstable when τ>τ(0). There is a Hopf bifurcation for system (1.5) at E2 when τ=τ(n)(n=0,1,2,⋯).
Note 2.3 If f32>0,f31>0 hold, then (2.14) has only one positive root, and this is a special case of Theorem 2.3 (ii).
The characteristic equation about E2 becomes to
H(λ,τ1,τ2)=(M(λ)+P(λ)e−λτ2)+N(λ)e−λτ1=0, | (2.16) |
Suppose λ=iω(ω>0) is a pure imaginary root of (2.16), similar to the case 2.2.1, one have
{A1+B1cosωτ2−C1sinωτ2=−E1cosωτ1+F1sinωτ1,D1−B1sinωτ2−C1cosωτ2=E1sinωτ1+F1cosωτ1, |
where
A1=m2ω2−m0,B1=p2ω2−p0,C1=p1,D1=ω3−m1ω,E1=n2ω2−n0,F1=n1ω. |
And
Fτ1(τ2)(ω)=ω6+f45ω5+f44ω4+f43ω3+f42ω2+f41ω+f40=0, | (2.17) |
where
f45=−2p2sinωτ2,f44=m22−2m1−n22+p22+2(m2p2−p1)cosωτ2,f43=2(p0+m1p2−m2p1)sinωτ2,f42=m21−2m2m0+2n2n0−n21+p21−2p2p0+2(p1m1−p0m2−m0p2)cosωτ2,f41=2(m0p1−p0m1)sinωτ2,f40=p20+m20+2p0m0cosωτ2−n20. |
Assumed that condition C13:fη<KE2 holds, then
Fτ1(τ2)(0)=f0=(m0+p0)2−n20=θx∗1y∗(m0+p0+n0)(fη−KE2)<0, | (2.18) |
and Fτ1(τ2)(+∞)=+∞. Therefore, (2.17) has at least one positive root. Without loss of generality, we assume that (2.17) has N1(N1∈N+) different positive roots, denoted by ωk=√ϖk(k=1,2,⋯,N1) and we have
cosωkτ1=F1D1−E1A1−(F1C1+E1B1)cosωkτ2+(E1C1−F1B1)sinωkτ2E21+F21Δ=Fωk. |
Thus
τ(n)1k(τ2)=1ωkcos−1[Fωk]+2nπωk,k=1,2,⋯,N1;n=0,1,2,⋯, | (2.19) |
and the direction of τ(n)1k(τ2) passing through the imaginary axis [20] when ω=ωk is determined by
sign[dRe(λ(τ))dτ|τ=τ(n)1k]=sign[F′τ1(τ2)(ϖk)|ϖk=ω2k]=sign(Δkτ1(τ2)). |
Then sign(Δkτ1(τ2))≠0, since ωk(k=1,2,⋯,N1) are N1 distinct positive roots of (2.17). And, system (1.5) undergos a Hopf bifurcation at E2 when τ1=τ(n)1k(τ2).
Define
Sτ1(τ2)={τ1|H(λ,τ1,τ2)=0,Re(λ)<0,τ2∈Sτ2}, |
τ10(τ2)=min{τ(n)1k(τ2)|1≤k≤N1,n=0,1,2,⋯}, |
when τ1∈Sτ1(τ2) the positive equilibrium E2 is local stable. Note that, if (2.17) has more than one positive root, there would be finite stability switches when time delay τ1 passing through the critical points
τ1=τ(n)1k(τ2)(k=1,2,⋯,N1;n=0,1,2,⋯) |
and [0,τ10(τ2))⊆Sτ1(τ2). If f4i>0(i=1,2,⋯,5) and condition C13:fη<KE2 hold, (2.17) has only one positive root, there is no stability switches when time delay τ1 passing through the critical points τ1=τ(n)1(τ2)(n=1,2,⋯) and Sτ1(τ2)=[0,τ(0)1(τ2)).
Theorem 2.4 (i) Suppose (2.17) has at least one positive roots denoted by ωk(1≤k≤N1). There exists a nonempty set Sτ1(τ2) and [0,τ10(τ2))⊆Sτ1(τ2), when τ1∈Sτ1(τ2) the positive equilibrium E2 of (1.5) is local stable, system (1.5) can undergoes a Hopf bifurcation at the positive equilibrium E2 when
τ1=τ(n)1k(τ2)(k=1,2,⋯,N1;n=0,1,2,⋯). |
(ii) Suppose (2.17) has only one positive root denoted by ω1. There exists a nonempty set Sτ1(τ2) and Sτ1(τ2)=[0,τ(0)1(τ2)), when τ1(τ2)∈Sτ1(τ2) the positive equilibrium E2 of (1.5) is local stable and unstable when τ1>τ(0)1(τ2), system (1.5) can undergoes a Hopf bifurcation at the positive equilibrium E2 when τ1=τ(n)1(τ2)(n=0,1,2,⋯).
Note 2.4 If f4i>0(i=1,2,⋯,5) and condition C13:fη<KE2 hold, then (2.17) has only one positive root, and this is a special case of Theorem 2.4 (ii).
The characteristic equation about E2 is given by
H(λ,τ1,τ2)=(M(λ)+N(λ)e−λτ1)+P(λ)e−λτ2=0, | (2.20) |
Suppose λ=iω(ω>0) is a pure imaginary root of (2.20), similar to the case 2.2.1, we have
{A2+B2cosω0τ1−C2sinω0τ1=−E2cosω0τ2+F2sinω0τ2,D2−B2sinω0τ1−C2cosω0τ1=E2sinω0τ2+F2cosω0τ2, |
where
A2=m2ω20−m0,B2=n2ω20−n0,C2=n1,D2=ω30−m1ω0,E2=p2ω20−p0,F2=p1ω0. |
And
Fτ2(τ1)(ω)=ω6+f55ω5+f54ω4+f53ω3+f52ω2+f51ω+f50 = 0, | (2.21) |
where
f55=−2n2sinωτ1,f54=m22−2m1−p22+n22+2(m2n2−p1)cosωτ1,f53=2(n0+m1n2−m2n1)sinωτ1,f52=m21−2m2m0+2p2p0−p21+n21−2n2n0+2(n1m1−n0m2−m0n2)cosωτ1,f51=2(m0n1−n0m1)sinωτ1,f50=n20+m20+2n0m0cosωτ1−p20, |
Assumed that condition C23:fη>KE2 hold, then
Fτ2(τ1)(0)=f0=(m0+n0)2−p20=θx∗1y∗(m0+p0+n0)(KE2−fη)<0, | (2.22) |
and Fτ2(τ1)(+∞)=+∞, therefore, (2.21) has at least one positive root. Without loss of generality, we assume that (2.21) has N2(N2∈N+) distinct positive roots, denoted by ωk=√ϖk(k=1,2,⋯,N2) and we have
cosωkτ2=F2D2−E2A2−(F2C2+E2B2)cosωkτ1+(E2C2−F2B2)sinωkτ1E22+F22≜Fωk. |
Thus
τ(n)2k(τ1)=1ωkcos−1[Fωk]+2nπωk,k=1,2,⋯,N2;n=0,1,2,⋯, | (2.23) |
and the direction of τ(n)2k(τ1) passing through the imaginary axis [20] when ω=ωk is determined by
sign[dRe(λ(τ))dτ|τ=τ(n)2k]=sign[F′τ2(τ1)(ϖk)|ϖk=ω2k]=sign(Δkτ2(τ1)). |
Then sign(Δkτ2(τ1))≠0, since ωk(k=1,2,⋯,N2) are N2 distinct positive roots of (2.21). System (1.5) undergoes a Hopf bifurcation at E2 when τ2=τ(n)2k(τ1).
Define
Sτ2(τ1)={τ2|H(λ,τ1,τ2)=0,Re(λ)<0,τ1∈Sτ1}, |
τ20(τ1)=min{τ(n)2k(τ1)|1≤k≤N2,n=0,1,2,⋯}, |
when τ2∈Sτ2(τ1) the positive equilibrium E2 is local stable. Note that, if (2.21) has more than one positive root, there would be finite stability switches when time delay τ2 passing through the critical points
τ2=τ(n)2k(τ1)(k=1,2,⋯,N2;n=0,1,2,⋯) |
and [0,τ20(τ1))⊆Sτ2(τ1). If f5i>0(i=1,2,⋯,5) and condition C23:fη>KE2 hold, (2.21) has only one positive root, there is no stability switches when time delay τ1 passing through the critical points τ2=τ(n)2(τ1)(n=1,2,⋯) and Sτ2(τ1)=[0,τ(0)2(τ1)).
Theorem 2.5 (i) Suppose (2.21) has at least one positive roots denoted by ωk(1≤k≤N2). There exists a nonempty set Sτ2(τ1) and [0,τ20(τ1))⊆Sτ2(τ1), when τ2∈Sτ2(τ1) the positive equilibrium E2 of system (1.5) is local stable. There is a Hopf bifurcation at E2 when
τ2=τ(n)2k(τ1)(k=1,2,⋯,N2;n=0,1,2,⋯). |
(ii) Suppose (2.21) has only one positive root denoted by ω1. There exists a nonempty set Sτ2(τ1) and Sτ2(τ1)=[0,τ(0)2(τ1)), when τ2(τ1)∈Sτ2(τ1) the positive equilibrium E2 of (1.5) is local stable and unstable when τ2>τ(0)2(τ1). There is a Hopf bifurcation at E2 when τ2=τ(n)2(τ1)(n=0,1,2,⋯).
Note 2.5 If f5i>0(i=1,2,⋯,5) and condition C23:fη>KE2 hold, then (2.21) has only one positive root, and this is a special case of Theorem 2.5 (ii).
We consider following system
{x′1(t)=2.5x2(t)−x1(t)(1.05+0.2x1(t)+1.25y(t)),x′2(t)=0.9x1(t)−0.7x2(t),y′(t)=y(t)(0.75x1(t−τ1)−0.1−1.8y(t−τ2)), | (3.1) |
where α=2.5,γ1=0.15,Ω=0.9,η=0.2,E=1.25,θ1=0.2,a=0.5,K=0.6,d=0.1,f=1.8,X(0)=(4.0,5.0,1.3).
In case 2.2.1, τ1>0,τ2≡0, from (2.6) we have f12=24.6366,f11=84.7024,f10=−5.3829, the unique positive root ω=0.2498 and
τ(n)1=8.4802+0.5nπ,n=0,1,2,⋯, |
sign(Δ1τ1)=1. According to Theorem 2.2.1 (ⅱ),
τ10=8.4802,Sτ1=[0,8.4802). |
The positive equilibrium point E2 is local stable when τ1=8.3<τ10, and unstable when τ1=8.6>τ10 (Figure 1). And increasing time delay τ1, the prey and predator populations can coexist with stable limit cycles when τ2=0 and τ1=8.5,8.6,8.7,8.8,8.9,9,9.3,10,11, 12,13,15,20,25,40,80, respectively (Figure 2). Then, there is a global Hopf bifurcation when time delay τ1 far away from the first bifurcating critical point τ10 [16], and the amplitudes of period oscillation are increasing with time delay τ1 increased. By the fast-slow oscillations, too large time delay τ1 would make the population to be die out, since the populations are very close to zero when time delay τ1 increase to some critical value (Figure 3).
In case 2.2.2, τ2>0,τ1≡0, from (2.10) we have f22=7.5640,f21=−103.9972,f20=5.3829, and there are two positive roots ω1=7.0595,ω2=0.0520,
τ(n)21=0.68+0.2833nπ,τ(n)22=17.4608+38.4615nπ,n=0,1,2,⋯, |
sign(Δ1τ2)=1,sign(Δ2τ2)=−1. Note that τ(0)21<τ(1)21<τ(0)22, there is no stability switches for τ2 passing through the critical points τ(n)21 and τ(n)22. According to Theorem 2.2.2 (ⅰ),
τ20=0.68,Sτ2=[0,0.68). |
The positive equilibrium point E2 is local stable when τ2=0.66<τ20, and unstable when τ2=0.70>τ20 (Figure 4). And increasing time delay τ2, the prey and predator populations can coexist with stable limit cycles when τ1=0 and τ2=0.7,0.8,0.9,1.0,1.1,1.2, respectively (Figure 5), and the amplitudes of period oscillation are increased. And, time delay τ2 would make the population to be die out, because the populations are very close to zero and then tend to unbounded solutions as time delay τ2=1.23 (Figure 6).
In case 2.2.3, τ1=τ2=τ,f32=14.7433,f31=−171.3174,f30=−12.0940, and the unique positive root ω=2.7753,
τ(n)=0.5015+0.7206nπ,n=0,1,2,⋯, |
sign(Δ1τ)=1. Then τ0=0.5017, According to Theorem 2.2.3 (ⅰ),
τ0=0.5017,Sτ=[0,0.5017). |
The positive equilibrium point E2 is local stable when τ=0.48<τ0, and unstable when τ=0.52>τ0 (Figure 7). And increasing time delay τ, the prey and predator populations can coexist with stable limit cycles when τ=0.503,0.505,0.508,0.513,0.518,0.523, 0.526,0.53, respectively (Figure 8), and the amplitudes of period oscillation are increased. And, time delay τ would make the population to be die out, because the populations are very close to zero and then tend to unbounded solution as time delay τ=0.536 (Figure 9).
We plot the stable and unstable regions with τ1×τ2=[0,10]×[0,1.4] (Figure 10) by using the publicly available Matlab package Trace-DDE [22], which by the pseudospectral method for the computation of characteristic roots of delay differential equations introduced in [23,24]. From Figure 10, we see that, if one fixed τ2 about 0.55, there would be stability switches when τ1 increasing from 0 to 10. Let τ2=0.52∈Sτ2, in case 2.2.4, from the Figure 11 we see that Fτ1(τ2)=0 have three positive roots
ω1=2.896366,ω2=2.473462,ω3=0.288596, |
and
τ(n)11=0.338236+0.690520nπ,τ(n)12=0.786103+0.808583nπ, |
τ(n)13=7.871032+6.930103nπ,(n=0,1,2,⋯),sign(Δ1τ1(τ2))=1,sign(Δ2τ1(τ2))=−1,sign(Δ3τ1(τ2))=1. |
Note that,
τ(0)11=0.338236,τ(0)12=0.786103,τ(1)11=2.507569,τ(1)12=3.326342,τ(2)11=4.676903, |
τ(2)12=5.866582,τ(3)11=6.846236,τ(0)13=7.8710317,τ(3)12=8.406821,τ(4)11=9.015570. |
then
τ(0)11<τ(0)12<τ(1)11<τ(1)12<τ(2)11<τ(2)12<τ(3)11<τ(0)13<τ(3)12<τ(4)11, |
and
Sτ1(τ2)=[0,τ(0)11)⋃(τ(0)12,τ(1)11)⋃(τ(1)12,τ(2)11)⋃(τ(2)12,τ(3)11). |
When τ2=0.52,τ1∈Sτ1(τ2), the positive equilibrium point E2 is local stable, where Sτ1(τ2) composed of four an increasing intervals. There are four times stability switches when time delay τ1 crossing Sτ1(τ2). And continuously increasing time delay τ1, the prey and predator populations coexist with period oscillation, quasi-period oscillation, even chaotic oscillation when τ1=8,9,11,14,15,19,37, and tend to unbounded oscillation for τ1=38 (Figure 12).
Let τ1=3.1∈(τ(1)11,τ(1)12) (unstable region). We investigate the effect time delay τ2 on system (3.1). The bifurcation diagrams of time delay τ2 over [0.52,0.68] show that system (3.1) has rich dynamics (Figure 13), including (1) periodic oscillating, (2) period-doubling bifurcations, and (3) chaos, and the solution tend to unbounded oscillation for τ2=0.69 at time t=210 (Figure 14).
Furthermore, increasing τ2 from 0.52 to 1.4, then τ(n)11 decreased and τ(n)12 increased, and the stability switches disappear one by one when τ(n)12>τ(n+1)11 for n=2,1,0 (Figure 15). We plot the stable and unstable regions (Figures 16 and 17) by choose f=0.6,0.8,1.2,1.5,1.9,2.2,2.4,2.9 respectively, and remained other parameters in example 1. By increasing the values of parameter f, the stable and unstable regions showing that τ(0)11 increased and τ(0)21 decreased, and the stable regions changed more and more complexity, which is a connect region from the view of topology. If we increasing the values of parameter f and choose τ2 less than and closed to the first critical point τ(0)21, then there would be more and more stability switches by increasing time delay τ1 from 0 to 15.
We consider following system
{x′1(t)=2.6x2(t)−x1(t)(1.1+0.3x1(t)+1.1y(t)),x′2(t)=0.9x1(t)−0.8x2(t),y′(t)=y(t)(0.88x1(t−τ1)−0.15−fy(t−τ2)), | (3.2) |
where α=2.6,γ1=0.2,Ω=0.9,η=0.3,E=1.1,θ1=0.15,a=0.65,k=0.8,d=0.15,X(0)=(4.0,5.0,1.3). We consider the case 2.2.2 with different value of parameter f.
Let f=0.095, from (2.10) we have ω1=0.3760,ω2=0.2963 and
τ(n)21=6.7472+5.3191nπ,τ(n)22=12.1964+6.7499nπ,sign(Δ1τ2)=1,sign(Δ2τ2)=−1, |
τ(0)21=6.7472,τ(0)22=12.1964,τ(1)21=23.4585,τ(1)22=33.4014, |
τ(2)21=40.1697,τ(2)22=54.6066,τ(3)21=56.8809,τ(4)21=73.5921,τ(3)22=75.8117, |
then
τ(0)21<τ(0)22<τ(1)21<τ(1)22<τ(2)21<τ(2)22<τ(3)21<τ(4)21<τ(3)22, |
and
Sτ2=[0,τ(0)21)⋃(τ(0)22,τ(1)21)⋃(τ(1)22,τ(2)21)⋃(τ(2)22,τ(3)21). |
Let f=0.11, from (2.10) we have ω1=0.4037,ω2=0.2957 and
τ(n)21=6.0146+4.9542nπ,τ(n)22=12.5688+6.7636nπ,sign(Δ1τ2)=1,sign(Δ2τ2)=−1, |
τ(0)21=6.0146,τ(0)22=12.5688,τ(1)21=21.5800, |
τ(1)22=33.8150,τ(2)21=37.1453,τ(3)21=52.7107,τ(2)22=55.0613, |
then
τ(0)21<τ(0)22<τ(1)21<τ(1)22<τ(2)21<τ(3)21<τ(2)22, |
and
Sτ2=[0,τ(0)21)⋃(τ(0)22,τ(1)21)⋃(τ(1)22,τ(2)21). |
Let f=0.16, from (2.10) we have ω1=0.4874,ω2=0.2972 and
τ(n)21=4.6008+4.1034nπ,τ(n)22=13.0647+6.7295nπ,sign(Δ1τ2)=1,sign(Δ2τ2)=−1, |
τ(0)21=4.6008,τ(0)22=13.0647,τ(1)21=17.4916,τ(2)21=30.3824,τ(1)22=34.2025, |
then
τ(0)21<τ(0)22<τ(1)21<τ(2)21<τ(1)22,Sτ2=[0,τ(0)21)⋃(τ(0)22,τ(1)21). |
Let f=0.35, from (2.10) we have ω1=0.7658,ω2=0.2976 and
τ(n)21=2.6501+2.6116nπ,τ(n)22=13.5430+6.7204nπ,sign(Δ1τ2)=1,sign(Δ2τ2)=−1,τ(0)21=2.6501,τ(1)21=10.8544,τ(1)21=13.5430, |
then
τ(0)21<τ(1)21<τ(0)22,Sτ2=[0,τ(0)21). |
From above numerical analysis, we see that, the times of stability switches are decreased from four to one by increasing the values of parameter f from 0.095 to 0.35; and the first critical point τ(0)21 also decreased (Figure 18). From Figure 19, we see the stable regions changed more and more simple by increasing the values of parameter f, and the stable regions from four parts to three parts, and to two parts, finally to one connect region.
We have considered a prey-predator system with three stage structure and two delays, and analyzed the stability of the equilibrium point, obtained the conditions for the positive equilibrium E2 occurring Hopf bifurcation by analyzing the characteristic equation in five cases. From the numerical examples and analysis, we know that the time delays would make the system subject to period oscillation, quasi-period oscillation, chaotic oscillation, finite stability switches, even unbounded oscillation and extinct. That is to say, time delays are important factors to affect the dynamic behaviors of the system.
From the analysis in section 2, we know that f30<0 in (2.14) for case 2.2.3, then (2.14) has at least one positive root, and there is a natural Hopf bifurcation for system (1.5) without any conditions according to theorem 2.2.3 (ⅰ). If condition C13:fη<KE2 holds then f10<0 in (2.6) for case 2.2.1, and that (2.6) has at least one positive root, and there is a Hopf bifurcation for system (1.5) according to theorem 2.2.1 (ⅰ). Similarly, if condition C23:fη>KE2 holds then f20<0 in (2.10) for case 2.2.2, and that (2.10) has at least one positive root, there is a Hopf bifurcation for system (1.5) according to theorem 2.2.2 (ⅰ). Note that conditions C13:fη<KE2 and C23:fη>KE2 cannot hold at the same time, but one of them can hold for any parameter values of the system exclude the special case fη=KE2. Therefore, there is a Hopf bifurcation for system (1.5) with only one time delay τ1 or τ2. And then, one of Sτ1 and Sτ2 is nonempty set. So, there is a natural Hopf bifurcation for system (1.5), and large time delays would make the positive point E2 eventually unstable. These are harmful delays for system (1.5).
From the analysis in section 2, we know that there would be finite stability switches for system (1.5) when the equation has more than one positive roots ωk(k>1). From example 1 in case 2.2.2, only time delay τ2, there are two positive roots and two critical delay sequences τ(n)21 and τ(n)22. But, there is no stability switches since τ(0)21<τ(1)21<τ(0)22. From example 1 in case 2.2.4 fixed τ2=0.52∈Sτ2, there are three positive roots and three critical delay sequences τ(n)11,τ(n)12 and τ(0)13. Note that
τ(0)11<τ(0)12<τ(1)11<τ(1)12<τ(2)11<τ(2)12<τ(3)11<τ(0)13<τ(3)12<τ(4)11, |
and
Sτ1(τ2)=[0,τ(0)11)⋃(τ(0)12,τ(1)11)⋃(τ(1)12,τ(2)11)⋃(τ(2)12,τ(3)11), |
there are four times stability switches when time delay τ1 increasing from 0 to infinity. From the stable and unstable regions in example 1 (Figures 16 and 17), we see that, there is no stability switches on τ1-axis or τ2-axis, but there are several times stability switches on τ2-axis in example 2 for some suitable parameter values (Figure 19). And, the stability regions in examples 1 and 2 are two different types in view of topology. The former is a connected region varying the parameter f from 0.6 to 2.9, and the latter from four parts to three parts, to two parts and to one connected region varying the parameter f from 0.095 to 0.35. That is to say, parameter f would change the stability switches times for some suitable parameter values of the system.
The numerical simulations show that delayed system (1.5) has complicated dynamic behaviors (Figures 12, 13 and 14) when we change the time delays and far away from the first bifurcating critical time delay point, including periodic oscillating, quasi-periodic oscillating, period-doubling bifurcations, chaos, and those behaviors undiscovered if the system (1.5) has only one time delay [16,17,18]. That is to say, time delays are important factor to affect the complex dynamic behaviors of the system, since the positive equilibrium point E2 of the system (1.5) is global asymptotically stable in the absence of time delays [16]. When time delay far away from the first critical point and increased, large time delays would make system (1.5) extinct (unbounded oscillation) undergoing a series of fast-slow oscillations or chaotic oscillations which make the prey and predator populations very closed to zero, and destroyed the permanence of it. And these are not found in [16,17,18]. All of the analysis show that the time delays would destroy the stability of the system, and induced complicated dynamic behaviors, even make the system die out.
All in all, time delays induced Hopf bifurcation, stability switches, and complicated dynamic behaviors for system (1.5), and make the system (1.5) subject to period oscillations and finite times stability switches via local Hopf bifurcation, and quasi-period oscillations, period-doubling bifurcations, chaotic oscillations and unbounded oscillations. Harmful time delays destroy the stability of the system, even make the system die out. How to control the bifurcation, unbounded oscillations and even chaos, arising from the multiple time delays system? The impulsive control strategies and the time-varying control strategies would be considered [25,26], which could both improve the stability of the system and control periodic and chaotic oscillations effectively. We will continue to study these problems in the future.
We are very grateful to anonymous referees for their valuable comments and suggestions.
This work was supported by the Natural Science Foundation of Guizhou Province (No. [2016]1135), the Joint Natural Science Foundation of Guizhou Province (Nos. LH[2014]7437 and LKQS[2013]01).
The author declares that there is no conflict of interest.
[1] |
|
[2] | 1. H. G. Li and Q. Zhang, Test system and automatic control of coal spontaneous combustion tendency oxidation kinetics, Chin. Univ. Min. Techn. Press, (14), 25–49. |
[3] | 2. H. F. Zhang, X. G. Chen and X. L. Sun, Classification research on coal spontaneous combustion based on fuzzy comprehensive evaluation, Coal Techn., (2014), 209–210. |
[4] | 3. T. S. Li, C. J. Lin, P. H. Kuo, et al., Grasping posture control design for a home service robot using an ABC-based adaptive PSO algorithm, Int. J. Adv. Robot. Syst., (2016), 1–15. |
[5] | 4. K. Meng, Z. Y. Dong and D. H. Wang, A self-adaptive RBF neural network classifier for transformer Fault analysis, IEEE Trans. Power Appar. Syst., 22010), 1350–1360. |
[6] | 5. W. C. Gu, B. R. Chai and Y. P. Teng, Research on support vector machine based on particle swarm optimization, T. Beijing Inst. Techn., 34(2014), 705–709. |
[7] | 6. H. G. Bargh and M. H. Sadr, Vibration Optimization of fiber-metal laminated composite shallow shell panels using an adaptive PSO algorithm, Mech. Adv. Compos. Struct., 4(201, 99–110. |
[8] | 7. J. Deng, Z. Xing and L. Ma, Application of multiple regression analysis in coal spontaneous combustion prediction, J. Xi'An Univ. Sci. Technol., 3(2011), 645–64 |
[9] | 8. L. Wang, S.J. Wu and C. Q. Li, Coal spontaneous combustion prediction based on grey-markov model, Comput. S., 31(2014), 416–420. |
[10] | 9. Y. Gao, M. G. Qin and M. J. Li, Analysis on prediction of residual coal spontaneous combustion in goaf based on support vector machine, Coal Sci. Technol., 38(20,50–54. |
[11] | 10. Y. P. Jin, B. Zhang and K. Gao, Application of spherical SVM in forecasting spontaneous combustion of coal, Comput. Appl. Softw., 30(2013), 57–60. |
[12] | 11. A. Sahu and S. Patnaik, Evolving neuro structure using adaptive PSO and modified TLBO for classification, Procedia Computer Sci., 92 (2016), 450–454. |
[13] | 12. W. P. Ding, C. T. Lin, M. Prasad, et al., A layered coevolution based attribute-boosted reduction using adaptive quantum behavior PSO and its consistent segmentation for neonates brain tissue, IEEE T. Fuzzy Syst., (2017),1–16. |
[14] | 13. K. W. Xia, Y. Dong and H. B. Du, Oil layer recognition model of LS-SVM based on improved PSO algorithm, Control Decis., 22(2007), 1385–1389. |
[15] | 14. S. R. Inkollu and V. R. Kota, Optimal setting of FACTS devices for voltage stability improvement using PSO adaptive GSA hybrid algorithm, Eng. Sci. Techn., 19(2016),1166–1176. |
[16] | 15. Y. Zhang, D. W. Gong and J. Cheng, Multi-objective particle swarm optimization approach for cost-based feature selection in classification, IEEE/ACM T. Comput. Bi., 14(2017), 64–75. |
[17] | 16. L. L. Shan, H. J. Zhang, J. Wang, et al., Parameters optimization and implementation of mixed kernels Ε-SVM based on improved PSO algorithm, Appl. Res. Comp., 30(2013), 1636–1639. |
[18] | 17. Y. Zhang, D. W. Gong and Z. H. Ding, Handling multi-objective optimization problems with a multi-swarm cooperative particle swarm optimizer, Expert Syst. Appl., 38(2011): 3933–3941. |
[19] | 18. L. S. Coelho, Gaussian quantum-behaved particle swarm optimization approaches for constrained engineering design problems, Expert Syst. Appl., 37(2010), 1676–1683. |
[20] | 19. Q. Zhang, M. Li, X. S. Wang, et al., Instance-based transfer learning for multi-source domains, Acta Autom. Sin., 40(4), 1176–1183. |
1. | Zhenming Sun, Dong Li, Chi-Hua Chen, Coal Mine Gas Safety Evaluation Based on Adaptive Weighted Least Squares Support Vector Machine and Improved Dempster–Shafer Evidence Theory, 2020, 2020, 1607-887X, 1, 10.1155/2020/8782450 | |
2. | Shangbo Zhou, Yuxiao Han, Long Sha, Shufang Zhu, A multi-sample particle swarm optimization algorithm based on electric field force, 2021, 18, 1551-0018, 7464, 10.3934/mbe.2021369 | |
3. | Jing Bi, Kaiyi Zhang, Haitao Yuan, Qinglong Hu, 2021, Energy-Aware Task Offloading with Genetic Particle Swarm Optimization in Hybrid Edge Computing, 978-1-6654-4207-7, 3194, 10.1109/SMC52423.2021.9658678 | |
4. | Yiqin Bao, Hongbing Lu, Qiang Zhao, Zhongxue Yang, Wenbin Xu, Detection system of dead and sick chickens in large scale farms based on artificial intelligence, 2021, 18, 1551-0018, 6117, 10.3934/mbe.2021306 | |
5. | Jing Bi, Haitao Yuan, Kaiyi Zhang, MengChu Zhou, Energy-Minimized Partial Computation Offloading for Delay-Sensitive Applications in Heterogeneous Edge Networks, 2022, 10, 2168-6750, 1941, 10.1109/TETC.2021.3137980 | |
6. | Wei Liu, Zhenjun Song, Meng Wang, Pengyu Wen, Dynamic prediction of high-temperature points in longwall gobs under a multi-field coupling framework, 2024, 187, 09575820, 1062, 10.1016/j.psep.2024.04.097 | |
7. | Surendra Kumar Dogra, Jitendra Pramanik, Singam Jayanthu, Abhaya Kumar Samal, 2023, Chapter 13, 978-3-031-46965-7, 153, 10.1007/978-3-031-46966-4_13 | |
8. | Liyang Gao, Bo Tan, Feiran Wang, Hao Lu, David Cliff, Xiaomeng Li, Comparison and Optimization of Monitoring and Warning Methods for Coal Spontaneous Combustion Fire Area, 2025, 0010-2202, 1, 10.1080/00102202.2025.2497061 | |
9. | Xuezhao Zheng, Peihua Li, Guobin Cai, Jun Guo, Yin Liu, MICE-PSO-RF Model for Predicting Coal Spontaneous Combustion Temperature Based on Multiple Imputation by Chained Equations, 2025, 0010-2202, 1, 10.1080/00102202.2025.2500516 |