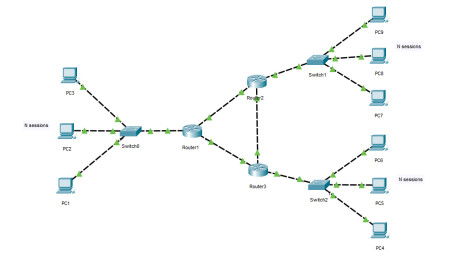
This paper discusses the congestion control challenges in a network employing multi-bottleneck Transmission Control Protocol/Active Queue Management (TCP/AQM). The study specifically focuses on networks characterized by input nonlinearity and unknown disturbances. We regard the network as a whole, and consider the influence between multiple nodes and unknown disturbance, a dynamic model of multi-bottleneck network is established. And the impact of dead zone and saturation on the system is taken into account for the first time in the model, the builded TCP/AQM model is more practicable. Based on the characteristics of fuzzy logic systems (FLS), combined with backstepping technology and Lyapunov function, an adaptive congestion control algorithm is designed to make full use of the link resources of each node and improve the network utilization. Ultimately, the proposed algorithm's efficacy and superiority are substantiated through simulation.
Citation: Yanxin Li, Shangkun Liu, Jia Li, Weimin Zheng. Congestion tracking control of multi-bottleneck TCP networks with input-saturation and dead-zone[J]. AIMS Mathematics, 2024, 9(5): 10935-10954. doi: 10.3934/math.2024535
[1] | Xiaohang Su, Peng Liu, Haoran Jiang, Xinyu Yu . Neighbor event-triggered adaptive distributed control for multiagent systems with dead-zone inputs. AIMS Mathematics, 2024, 9(4): 10031-10049. doi: 10.3934/math.2024491 |
[2] | Wei Zhao, Lei Liu, Yan-Jun Liu . Adaptive neural network control for nonlinear state constrained systems with unknown dead-zones input. AIMS Mathematics, 2020, 5(5): 4065-4084. doi: 10.3934/math.2020261 |
[3] | Shihua Zhang, Xiaohui Qi, Sen Yang . A cascade dead-zone extended state observer for a class of systems with measurement noise. AIMS Mathematics, 2023, 8(6): 14300-14320. doi: 10.3934/math.2023732 |
[4] | Hadil Alhazmi, Mohamed Kharrat . Echo state network-based adaptive control for nonstrict-feedback nonlinear systems with input dead-zone and external disturbance. AIMS Mathematics, 2024, 9(8): 20742-20762. doi: 10.3934/math.20241008 |
[5] | Giovanni G. Soares, Ernesto Estrada . Navigational bottlenecks in nonconservative diffusion dynamics on networks. AIMS Mathematics, 2024, 9(9): 24297-24325. doi: 10.3934/math.20241182 |
[6] | Guijun Xing, Huatao Chen, Zahra S. Aghayan, Jingfei Jiang, Juan L. G. Guirao . Tracking control for a class of fractional order uncertain systems with time-delay based on composite nonlinear feedback control. AIMS Mathematics, 2024, 9(5): 13058-13076. doi: 10.3934/math.2024637 |
[7] | Miao Xiao, Zhe Lin, Qian Jiang, Dingcheng Yang, Xiongfeng Deng . Neural network-based adaptive finite-time tracking control for multiple inputs uncertain nonlinear systems with positive odd integer powers and unknown multiple faults. AIMS Mathematics, 2025, 10(3): 4819-4841. doi: 10.3934/math.2025221 |
[8] | Le You, Chuandong Li, Xiaoyu Zhang, Zhilong He . Edge event-triggered control and state-constraint impulsive consensus for nonlinear multi-agent systems. AIMS Mathematics, 2020, 5(5): 4151-4167. doi: 10.3934/math.2020266 |
[9] | Fang Zhu, Pengtong Li . Adaptive fuzzy consensus tracking control of multi-agent systems with predefined time. AIMS Mathematics, 2025, 10(3): 5307-5331. doi: 10.3934/math.2025245 |
[10] | Mohsen Bakouri, Abdullah Alqarni, Sultan Alanazi, Ahmad Alassaf, Ibrahim AlMohimeed, Mohamed Abdelkader Aboamer, Tareq Alqahtani . Robust dynamic control algorithm for uncertain powered wheelchairs based on sliding neural network approach. AIMS Mathematics, 2023, 8(11): 26821-26839. doi: 10.3934/math.20231373 |
This paper discusses the congestion control challenges in a network employing multi-bottleneck Transmission Control Protocol/Active Queue Management (TCP/AQM). The study specifically focuses on networks characterized by input nonlinearity and unknown disturbances. We regard the network as a whole, and consider the influence between multiple nodes and unknown disturbance, a dynamic model of multi-bottleneck network is established. And the impact of dead zone and saturation on the system is taken into account for the first time in the model, the builded TCP/AQM model is more practicable. Based on the characteristics of fuzzy logic systems (FLS), combined with backstepping technology and Lyapunov function, an adaptive congestion control algorithm is designed to make full use of the link resources of each node and improve the network utilization. Ultimately, the proposed algorithm's efficacy and superiority are substantiated through simulation.
The rapid proliferation of cloud computing, the Internet of Things, and online video applications has led to an exponential surge in network traffic. This causes network capacity to quickly reach its limits, making congestion control a critical issue in ensuring efficient network operation. Since the birth of the Internet, the congestion problem of network transmission has received widespread attention[1,2,3,4,5]. For this reason, many methods to solve network congestion came into being. Traditional queue management policies adopt the "Drop-Tail" policy, which is easy to produce continuous full queue state, and even lead to data flow deadlock and global synchronization. Therefore, active queue management (AQM) has attracted the attention of many scholars[6]. It can effectively mitigates congestion, diminishes packet loss rates, enhances network utilization, and averts network crashes. The initial AQM algorithm introduced is Random Early Detection (RED), which computes the likelihood of packet loss based on the average queue length[7]. Although RED algorithm can effectively control the congestion in the network, the performance of RED algorithm can not adapt to the change of network load because it is sensitive to static parameters. In the following decades, many scholars have improved the RED algorithm[8,9], but these improved RED algorithms have high requirements for parameter tuning and are easily affected by the environment[10]. Due to the lack of systematic theory, the algorithm basically relies on intuition and inspiration, which leads to some problems in stability and robustness. To avoid this problem, Misra established a new TCP/AQM model based on fluid flow theory combined with stochastic differential equations, which laid the foundation for applying control theory to settle the corresponding congestion problem[11]. Due to the complexity of the network, scholars focus on different issues. Ren introduced an AQM algorithm grounded in linear control principles[12]. However, the AQM controller based on linear theory can not well compensate the nonlinearity of the network system, and is vulnerable to the disturbance of unresponsive flow and other factors, its robustness is poor, the stability of the algorithm is difficult to be guaranteed. [13] uses linear matrix inequality (LMI) to linearize the nonlinear model, and compensates the influence of uncertainty in the network through the designed sliding surface. These studies are based on linear systems, ignoring the nonlinear dynamic nature of TCP networks. Therefore, many scholars have proposed congestion control algorithms for nonlinear TCP networks. Liu applies the prescribed performance technology to the TCP/AQM congestion problem, and designs congestion control combined with H∞ control, which can estimate the unknown link capacity[14]. Considering the dynamic nature of the session count during network transmission, Chen adapted the TCP/AQM model into a switching model. Subsequently, a network congestion controller was devised, integrating prescribed performance technology. This designed controller adeptly manages the frequent fluctuations in the number of sessions[15]. Furthermore, certain studies also use intelligent control methods[16], data segmentation methods[17] and various other control methodologies to formulate network congestion controllers. The author develops an AQM algorithm using Neural Network (NN) approaches and fuzzy variable structure control respectively[18,19]. The output weight of radial basis function NN was obtained by particle swarm optimization, and then AQM controller was implemented in [20].
The dynamic nonlinear system of TCP network has saturation characteristics, which may deteriorate the performance of the network system and inducing instability of the system. Therefore, accounting for the saturation characteristics of the control input becomes imperative when designing AQM algorithm[21,22,23,24]. [21] considers the input constraint of the TCP network system, and replaces the non-differentiable saturation function with the smooth differentiable function, the designed AQM algorithm enables the system to obtain better asymptotic stability. [22] designs robust enhanced proportional derivatives affected by input saturation, and solves the controller design problem for linear systems with asymmetric constraints by the scaling small gain theorem. Considering the input saturation within the TCP/AQM system, Shen proposed a new AQM control combined with the prescribed performance control. Employing FLS, the approach effectively addresses error disturbances stemming from input saturation, thereby enhancing the overall control effectiveness of the system.[23]. Similarly, dead zone may exist in the actual dynamic system[25,26,27], although many scholars have noticed the input nonlinearity such as dead zone and saturation, have also proposed corresponding solutions, the existing researches on TCP network congestion control only consider the dynamic characteristics of a single characteristic. So the control problems of multi-type input nonlinearity, particularly those associated with uncertain nonlinearities, remain relatively scarce. In this paper, the effects of dead zone and saturation inputs concurrently on TCP network congestion control are considered at the same time, and the performance of the system is controlled effectively.
In the Internet, under the influence of the environment, there are always some uncertainties in practical TCP network, such as the uncertainty of network parameters and unresponsive User Datagram Protocol (UDP) flows. At present, the most effective solution is to learn the uncertain functions in the nonlinear systems with the neural networks or the fuzzy logical systems[28,29,30,31,32]. Based on the approximate characteristics of FLS, Liu et al. introduced an adaptive fuzzy control scheme to improve the robustness and convergence of nonlinear stochastic switching systems[33]. In the context of a nonlinear system characterized by uncertain function constraints, a fuzzy state observer is devised to estimate the unmeasurable state variables, so that the system state is no longer constrained by the function[34]. Based on the above analysis, the application of FLS in TCP/AQM system can effectively alleviate the congestion problem. Mohammadi et al. proposed a PID controller for TCP/AQM systems with saturated input delay, which reduces packet loss and improves network utilization. In the design process of the controller, fuzzy algorithm is used to approximate the optimal PID control gain[35]. In view of the time-varying number of sessions during network transmission, Chen modified the TCP/AQM model into a switching model and established a network congestion controller combined FLS with prescribed performance technology, the designed controller can cope with frequent changes in the number of sessions[36]. The incorporation of FLS is undertaken to address unknown elements, accompanied by the formulation of a novel practical control law. The designed adaptive tracking controller ensures the actual boundary of all signals in the TCP/AQM network system [37].
At present, in the big data environment, the number of network nodes and data traffic are very large, especially UDP flow represented by audio and video occupy a large amount of bandwidth. Therefore, Controlling traffic for a single node proves challenging in mitigating network congestion. Recognizing this, in the development of a congestion control algorithm, a holistic approach that considers the entire network becomes imperative to effectively avert congestion issues within the expansive realm of big data. Based on this, this paper studies delves into an exploration of an adaptive fuzzy control for AQM network nonlinear systems, incorporating considerations for both saturated input and dead zones. The principal contributions of this study can be summarized in the following three key points.
(1) Considering the unknown response flow and the interplay among network nodes, this paper takes the TCP network as a whole and builds a multi-bottleneck TCP/AQM network model, which can more accurately describe the real network and improve the window utilization.
(2) In this paper, for the first time, the dead zone and saturation input nonlinear characteristics of the network model are considered at the same time, by confining the input within the permissible range, this approach renders the model in this study notably more comprehensive and inclusive.
(3) Combined with the backstepping technique, this paper designs the adaptive fuzzy control algorithm, which guarantees the steady-state and transient performance of the tracking error, allowing the queue length of nodes to effectively track the desired queue length.
The subsequent sections of this paper are structured as follows: Section 2 provides the TCP network model and outlines the preliminaries. Section 3 presents the primary result. To illustrate the effectiveness of the proposed method, Section 4 conducts simulation experiments. Finally, Section 5 summarizes the conclusion.
Building upon Misra's fluid model in 2001, this paper considers the following multi-bottleneck TCP network
{˙Ws,i(t)=Ni(t)Ri(t)−W2s,i(t)2Ni(t)Ri(t)pi(t)˙qi(t)=−Ci(t)+Ws,i(t)Ri(t)−∑nj=1Ws,j(t)Nj(t)+ωi(t) | (2.1) |
where Ws,i(t) is the total congestion window size, Ri(t) is the round-trip delay, qi(t) is the queue length in the router, pi(t) is the probability of packet loss, Ni(t) and Ci(t) is the number of TCP sessions and available link capacity, respectively. ωi(t) is the external disturbance caused by unresponsive flows like UDP flows. i is the i-th network node.
Remark 1. A multi-bottleneck TCP/AQM network is different from a single-bottleneck network in that it contains multiple bottleneck nodes. In the transmission process, the upstream node acts as the sender of the downstream node, and its link capacity is affected by the adjustment of the downstream node to the size of the sender window. Hence, in formulating the network model, this paper takes into account how downstream nodes affect the link capacity of upstream nodes. It is worth noting that the queue capacity of each bottleneck node is usually not the same, making its transmission model different.
Due to the complexity of multi-bottleneck networks, the queue length in single-bottleneck networks is no longer suitable. Therefore, in the congestion control design, this paper considers tracking the queue usage rate qu,i. Assuming the known total number of queues that a single router can accommodate, denoted as qmax, and the queue usage rate of a single router as qu,i=qiqmax, model (2.1) can be reformulated as follows:
{˙Ws,i(t)=Ni(t)Ri(t)−W2s,i(t)2Ni(t)Ri(t)pi(t)˙qu,i(t)=˙qi(t)qmax,i=Ws,i(t)Ri(t)qmax,i−−Ci+∑nj=1aijWs,j(t)Nj(t)qmax,i+ωi(t). | (2.2) |
Let x1,i=qu,i, x2,i=Ws,i(t), Ni∈N+, The network dynamics model (2.2) can be written as
{˙x1,i=fi(x2,i)−−Ci+∑nj=1aijx2,j(t)Nj(t)qmax,i+ωi(t)˙x2,i=gσ(t),i(x2,i)+hσ(t),i(x2,i)ui(t)yi=x1,i | (2.3) |
where fi(x2,i)=x2,iRi(t)qmax,i, gσ(t),i=Nσ(t),i(t)Ri(t), hσ(t),i(x2,i)=−x22,i(t)2Nσ(t),i(t)Ri(t), ui(t)=pi(t), σ(t):[0,∞)→N=1,⋯,Nmax is switching signal.
Assumption 1. Suppose that the unknown disturbance ωi(t) is bounded, and 0≤ωi≤ωmax.
ui(t) is a nonsymmetric dead-zone input nonlinearity which is defined as follows:
D(u)={mr(u−ur),u≥ur0,ul<u<urml(u−ul),u≤ul | (2.4) |
where the parameters ur>0 and ul<0 represent the breakpoints of control signal nonlinearity. ml>0 and mr>0 denote the right slope and the left slope of the dead zone.
Given that the control signal ui(t) for TCP/AQM represents the marking probability, with a value range of [0,1], the system input is constrained by nonlinear saturation as defined by
sat(u)={umax,u>umaxu,−umin≤u≤umax−umin,u<−umin=℘(u)u | (2.5) |
where umax>0 and umin>0 are unknown constants, respectively.
Let's assume umax>mrur and umin>mlul. Defined ℘(u) as follows:
℘(u)={umaxu,u>umax1,−umin≤u≤umax−uminu,u<−umin. | (2.6) |
Obviously, ℘(u)∈R and η≤℘(u)≤1, where η>0 is an unknown constant.
Remark 2. Significantly, both the input dead zone and saturation characteristics of the network model are considered in this paper, which makes the application more extensive.
Thus, according to (2.4–2.6), sat(D(u)) can be characterized as
sat(D(u))={umaxD(u)D(u)=℘(D(u))mr(u−ur),u>umaxmr+urmr(u−ur),umaxmr+ur≥u>umax0,−umin≤u≤umaxml(u−ul),−uminml+ul≤u<−ul−uminD(u)D(u)=℘(D(u))ml(u−ul),u<−uminml+ul. | (2.7) |
Consider the jth IF-THEN rule of the following form:
Rℓ: IF x1 is Fℓ1 and … and xn is Fℓn.
Then y is Gℓ, l=1,2,…,N, where x=[x1,x2,…,xn]T∈Rn, and y∈R are input and output of the FLS, respectively. Fℓi and Gℓ are fuzzy sets in R. By using the singleton fuzzification, the product inference and the center-average defuzzification, the FLS can be given as
y(x)=∑Nℓ=1φℓ∏ni=1μFℓi(ˉxi)∑nℓ=1[∏ni=1μFℓi(ˉxi)] |
where N is the number of IF-THEN rules, ϖj is the point at which fuzzy membership function μPℓ(ϖℓ)=1.
Let
ζℓ(x)=∏ni=1μFℓi(xi)∑Nℓ=1[∏ni=1μFℓi(xi)] |
where ζ(x)=[ζ1(x),ζ2(x),…,ζN(x)]T Then the FLS can be described as
y=φTζ(x). | (2.8) |
Lemma 1. [38] Let f(x) be a continuous function defined on a compact set Ω. Then, for ∀ϵ>0, there exists a FLS (2.8) such that
supx∈Ω|f(x)−φTζ(x)|≤ϵ. | (2.9) |
Lemma 2. (Young's inequality) For ∀(x,y)∈R2, the following inequality holds:
xy≤αpp|x|p+1qαq|y|q, | (2.10) |
where α>0, p>1, q>1, and (p−1)(q−1)=1.
Lemma 3. For 1≤i≤n, there is an unknown constant b>0 that satisfies:
|hσ(t),i|≤b. | (2.11) |
This section introduces an adaptive fuzzy control scheme utilizing the backstepping method for system (2.3). The backstepping design scheme includes n steps. First of all, the transfer error of network nodes is given as follows:
{z1,i=∑nj=1aij(yi−yj)+(yi−yref)z2,i=x2,i−α1,i | (3.1) |
where α1,i is the virtual control law and yref is the tracking objective function.
Theorem 1. Under assumption 1, using virtual control law (3.2), adaptive law (3.3), (3.4) and control law (3.12), multi-bottleneck TCP/AQM system (2.2) exhibits the following characteristics:
(1) The queue length required for the output tracking of the system.
(2) All signals within the closed-loop system are semi-globally uniform and ultimately bounded.
(3) The tracking error of each bottleneck node converges to a small neighborhood near the origin.
Before designing the controller, it is necessary to establish the constants θk,i=‖Φ∗k,i‖2,k=1,2,⋯,n, where ˆθk,i represents the estimation of θk,i, with the estimation error denoted as ˜θk,i=θk,i−ˆθk,i.
Select the virtual control law and adaptive law as follows:
α1,i=Rqmax,idi(Ci+∑nj=1aijxi,jNjqmax,idi+˙qref−12a21z1,iˆθ1,iξT1,iξ1,i). | (3.2) |
˙ˆθ1,i=r12a21z21,iξT1,iξ1,i−τ1,iˆθ1,i. | (3.3) |
˙ˆθ2,i=r22a22z22,iξT2,iξ2,i−τ2,iˆθ2,i. | (3.4) |
Step 1. Consider the following Lyapunov function:
V1,i=12z21,i+12r1˜θ21,i. | (3.5) |
Then, differentiating V1,i with respect to time results in
˙V1,i=z1,i[n∑j=1,j≠iaij(yi−yj)+(yi−yref)]−1r1˜θ1,i˙ˆθ1,i=z1,i[n∑j=1,j≠iaij(fi(x2,i)−Ci+∑nj=1aijx2,jNjqmax,i+ωi(t))−n∑j=1,j≠iaij(fj(x2,j)−Cj+∑nk=1ajkx2,kNkqmax,j−ωi(t))+(fi(x2,i)−Ci+∑nj=1aijx2,jNjqmax,i+ωj(t)−˙qref)]−1r1˜θ1,i˙ˆθ1,i. | (3.6) |
Let ∑Nj=1aij+1=di, then (3.6) can be written as
˙V1,i=z1,i[di(fi(x2,i)−Ci+∑nj=1aijx2,jNjqmax,i)−˙qref−n∑j=1,j≠iaij(fj(x2,j)−Cj+∑nk=1ajkx2,kNkqmax,j+ωj(t))+diωi(t)]−1r1˜θ1,i˙ˆθ1,i. | (3.7) |
The following nonlinear functions are approximated by FLS. According to Lemma 1, there are fuzzy logic functions ΦT1,iξ1,i(X) that satisfy
F1,i(X)=−n∑j=1,j≠iaij(fj(x2,j)−Cj+∑nk=1ajkx2,kNkqmax,j+ωj(t))+diωi(t)=ΦT1,iξ1,i(X)+ϵ1,i(X),ϵ1,i(X)≤ε1,i. |
From young's inequality of Lemma 2
z1,iF1,i(X)=z1,iΦT1,iξ1,i(X)+z1,iϵ1,i(X)≤12a21z21,iθ1,iξT1,iξ1,i+12a21+12z21,i+12ε21,i. |
Therefore, (3.7) can be reformulated as
˙V1,i≤z1,i[di(fi(x2,i)−Ci+∑nj=1aijx2,jNjqmax,i)−˙qref]+12a21z21,iθ1,iξT1,iξ1,i+12a21+12z21,i+12ε21,i−1r1˜θ1,i˙ˆθ1,i. | (3.8) |
According to the (3.3), we have
1r1˜θ1,i˙ˆθ1,i=12a21z21,i˜θ1,iξT1,iξ1,i−τ1,ir1˜θ1,iˆθ1,i. |
It is noted that
τ1,ir1˜θ1,iˆθ1,i≤−τ1,i2r1˜θ21,i+τ1,i2r1θ21,i. |
Due to z2,i=x2,i−α1,i⇒x2,i=z2,i+α1,i. Substituting (3.2) into (3.8), the calculation of V1,i can be determined as:
˙V1,i≤diRqmax,iz1,iz2,i+12a21+12z21,i+12ε21,i−τ1,i2r1˜θ21,i+τ1,i2r1θ21,i. | (3.9) |
Step 2. Consider the following Lyapunov function:
V2,i=V1,i+12z22,i+12r2˜θ22,i. | (3.10) |
The computation of the derivative of V2,i is expressed as follows:
˙V2,i=˙V1,i+z2,i[gσ(t),i(x2,i)+hσ(t),i(x2,i)sat(D(ui))−˙α1,i]−1r2˜θ2,i˙ˆθ2,i. | (3.11) |
Define the following control laws:
ui={u′iˆθ2,i+ur,i,z2,i<0−u′iˆθ2,i+ul,i,z2,i≥0 | (3.12) |
where u′i≥0, θ2,i=hσ(t),iηimi and mi=min{mr,i,ml,i}.
We'll delve into the discussion of the following two cases:
Case 1:z2,i<0
Since z2,i<0, It follows from (3.12) that one has
ui=u′iˆθ2,i+ur,i. |
Since ui>ur,i, from (2.4), one has
D(u)=mr,i(ui−ur,i). |
For the analysis of sat(D(ui)), we have the following two cases:
(1) ui≤umax,imr,i+ur,i
From (2.7), it gives
sat(D(ui))=mr,i(ui−ur,i). | (3.13) |
According to Lemma 3 and combine (3.12) and (3.13), we have
z2,ihσ(t),isat(D(ui))≤z2,ibmr,i(ui−ur,i)=z2,ibmr,iu′iˆθ2,i. | (3.14) |
This case is based on z2,i<0 discussion, one has z2,i=−|z2,i|. Then, (3.14) can be rewritten as
z2,ihσ(t),isat(D(ui))≤z2,ibmr,iu′iˆθ2,i≤−|z2,i|ηibmr,iu′iˆθ2,i | (3.15) |
where 0<ηi≤℘(D(ui))≤1.
Substituting (3.15) into (3.11) yields
˙V2,i≤˙V1,i+z2,igσ(t),i(x2,i)−|z2,i|ηibmr,iu′iˆθ2,i−z2,i˙α1,i−1r2˜θ2,i˙ˆθ2,i. | (3.16) |
(2) ui>umax,imr,i+ur,i
From (2.7), it gives
sat(D(ui))=umax,iD(ui)D(ui)=℘(D(ui))mr,i(ui−ur,i). | (3.17) |
According to Lemma 3 and combine (3.12), (3.17), we have
z2,ihσ(t),isat(D(ui))≤z2,ib℘(D(ui))mr,i(ui−ur,i)=z2,ib℘(D(ui))mr,iu′iˆθ2,i. | (3.18) |
Since 0<ηi<℘(D(ui))≤1, similar to (3.15), we have
z2,ihσ(t),isat(D(ui))≤−|z2,i|bηimr,iu′iˆθ2,i. | (3.19) |
Substituting (3.19) into (3.11) yields
˙V2,i≤˙V1,i+z2,igσ(t),i(x2,i)−|z2,i|ηibmr,iu′iˆθ2,i−z2,i˙α1,i−1r2˜θ2,i˙ˆθ2,i. | (3.20) |
Based on the above discussion of (1) and (2), in Case 1, when subjected to the control law defined in (3.12), (3.11) can be formulated as
˙V2,i≤˙V1,i+z2,igσ(t),i(x2,i)−|z2,i|ηibmr,iu′iˆθ2,i−z2,i˙α1,i−1r2˜θ2,i˙ˆθ2,i. | (3.21) |
Case 2:z2,i≥0
Since z2,i≥0, It follows from (3.12) that one has
ui=−u′iˆθ2,i+ul,i. |
Since ui≤ur,i, from (2.4), one has
D(u)=ml,i(ui−ul,i). |
For the analysis of sat(D(ui)), we have the following two cases:
(1) ui≥−umin,iml,i+ul,i
From (2.7), it gives
sat(D(ui)=ml,i(ui−ul,i). | (3.22) |
According to Lemma 3 and combine (3.12), (3.22), we have
z2,ihσ(t),isat(D(ui))≤z2,ibml,i(ui−ul,i)=z2,ibml,iu′iˆθ2,i. | (3.23) |
This case is based on z2,i<0 discussion, one has z2,i=−|z2,i|. Then, (3.23) can be rewritten as
z2,ihσ(t),isat(D(ui))≤z2,ibml,iu′iˆθ2,i≤−|z2,i|ηibml,iu′iˆθ2,i, | (3.24) |
where 0<ηi≤℘(D(ui))≤1.
Substituting (3.24) into (3.11) yields
˙V2,i≤˙V1,i+z2,igσ(t),i(x2,i)−|z2,i|ηibml,iu′iˆθ2,i−z2,i˙α1,i−1r2˜θ2,i˙ˆθ2,i. | (3.25) |
(2) ui<−umin,iml,i+ul,i
From (2.7), it gives
sat(D(ui))=umax,iD(ui)D(ui)=℘(D(ui))ml,i(ui−ul,i). | (3.26) |
According to Lemma 3 and combine (3.12), (3.26), we have
z2,ihσ(t),isat(D(ui))≤z2,ib℘(D(ui))ml,i(ui−ul,i)=z2,ib℘(D(ui))ml,iu′iˆθ2,i. | (3.27) |
Since 0<ηi<℘(D(ui))≤1, similar to (3.24), we have
z2,ihσ(t),isat(D(ui))≤−|z2,i|bηiml,iu′iˆθ2,i. | (3.28) |
Substituting (3.28) into (3.11) yields
˙V2,i≤˙V1,i+z2,igσ(t),i(x2,i)−|z2,i|ηibml,iu′iˆθ2,i−z2,i˙α1,i−1r2˜θ2,i˙ˆθ2,i. | (3.29) |
Based on the above discussion of (1) and (2), in Case 2, when subjected to the control law defined in (3.12), (3.11) can be formulated as
˙V2,i≤˙V1,i+z2,igσ(t),i(x2,i)−|z2,i|ηibml,iu′iˆθ2,i−z2,i˙α1,i−1r2˜θ2,i˙ˆθ2,i. | (3.30) |
Due to θ2,i=hσ(t),iηimi and mi=min{mr,i,ml,i}, the following inequalities are obtained:
−|z2,i|ηibmr,iu′iˆθ2,i≤−|z2,i|θ2,iu′iˆθ2,i−|z2,i|ηibml,iu′iˆθ2,i≤−|z2,i|θ2,iu′iˆθ2,i. |
Therefore, according to the discussions in Cases 1 and 2, from (3.20) and (3.29), we have
˙V2,i≤˙V1,i+z2,igσ(t),i(x2,i)−|z2,i|θ2,iu′iˆθ2,i−z2,i˙α1,i−1r2˜θ2,i˙ˆθ2,i. | (3.31) |
According to Lemma 1, we can approximate the unknown function F2,i(X) using fuzzy logic functions ΦT2,iξ2,i(X). Similarly, one easily obtains
−z2,i˙α1,i≤12a22z22,iθ2,iξT2,iξ2,i+12a22+12z22,i+12ε22,i. | (3.32) |
Following a procedure akin to Step 1, we can derive the subsequent inequality:
1r2˜θ2,i˙ˆθ2,i=12a22z22,i˜θ2,iξT2,iξ2,i−τ2,ir2˜θ2,iˆθ2,iτ2,ir2˜θ2,iˆθ2,i≤−τ2,i2r2,i˜θ22,i+τ2,i2r2θ22,i. | (3.33) |
It follows from the young's inequality that we have
z2,igσ(t),i=z2,iNσ(t),iR≤z22,iN2σ(t),i2R2+12. | (3.34) |
Applying (3.32)–(3.34), we have
˙V2,i≤˙V1,i−|z2,i|θ2,iu′iˆθ2,i+12z22,i(Nσ(t),iR)2+12a22z22,iθ2,iξT2,iξ2,i−12a22z22,i˜θ2,iξT2,iξ2,i+12a22+12z22,i+12ε22,i+12−τ2,i2r2˜θ22,i+τ2,i2r2θ22,i. | (3.35) |
Now, u′i in control law ui can be defined by
u′i=1θ2,iˆθ2,i[12(diRqmax,i)2z21,iz2,i+12z2,i(Nσ(t),iR)2−12a22z2,iˆθ2,iξT2,iξ2,i]. | (3.36) |
Substituting (3.9) and (3.36) into (3.35) yields
˙V2,i≤12z21,i+12a21+12ε21,i−τ1,i2r1˜θ21,i+τ1,i2r1θ21,i+12z22,i+12a22+12ε22,i−τ2,i2r2˜θ22,i+τ2,i2r2θ22,i≤KiV2,i+△i | (3.37) |
where Ki=min{1,−τ1,i,−τ2,i}, △i=∑2j=1(12a2j+12ε2j,i+τj,i2rjθ2j,i).
Consider the following Lyapunov function:
V=n∑i=1V2,i. | (3.38) |
Substitute V2,i, the derivative of (3.38) can be given by
˙V≤KV+△ | (3.39) |
where K=∑ni=1Ki, △=∑ni=1△i.
Obviously, V satisfies the following inequality
0≤V≤e−KtV(0)+△K. | (3.40) |
It is readily apparent from (3.40) that V is bounded. The demonstration of Theorem 1 is now concluded.
This chapter simulates the proposed AQM network congestion control algorithm and evaluates the efficiency and superiority of the controller. A multi-bottleneck network featuring three bottlenecks is examined, and its topology is illustrated in Figure 1. Matlab is used to simulate the presented method, the parameters of the TCP/AQM network system are set as follows:
c1,1=3;c1,2=3;c1,3=3;c2,1=15000;c2,2=9000;c2,3=9000;a1=1;a2=5;r1=1;r2=0.003;d1=1;d2=2;d3=2;R=0.08;N1=85;N2=85;N3=80;C=3500;qref=0.25;qmax,1=730;qmax,2=750;qmax,3=720;ω=cos(t). |
The initial state of the system is x0=[0.205,1,0.205,1,0.205,1,1,1,1,1, 1,1,1], and the results of the simulations are depicted in Figures 2–8.
In Figure 2, the dynamic evolution of the queue length for each node is illustrated. Notably, as the target queue length is set at 180, the queue length for each node converges to a stable state, demonstrating a rapid response and effective control. This underscores the robustness and superiority of the AQM scheme devised in this paper.
Figure 3 showcases the trajectory of the tracking error for each node. As depicted in the Figure 3, the tracking error remains stable, confined within the specified upper and lower bounds, and converges in close proximity to the origin. This observation underscores the superior transient performance of the controller devised in this paper.
In Figure 4, the packet loss probability is introduced, and it is obvious that the packet loss probability is always in p∈[0,1].
Figure 5 represents the variation of the adaptive law. After 1s, the adaptive law tends to be stable and bounded, indicating that the controller formulated in this paper can make good use of the adaptive law for precise estimation of link capacity.
In order to verify the effectiveness of the proposed algorithm, the tracking error and queue length are compared respectively. The comparison results are shown in Figures 6–11. The comparison objects are the proposed algorithm, single bottleneck network (different topographs)[31], EO-PID[39], PSO-PID, and RED.
In Figures 6-8, obviously, the single-bottleneck network and the multi-bottleneck network in this paper are the most stable in the experimental results. The single-bottleneck network tends to be stable at 0.88s, while the multi-bottleneck network in this paper tends to be stable at 1.56s. However, it is worth noting that the queue length of the multi-bottleneck network in this paper is 2.5 times that of the single bottleneck, so the multi-bottleneck network in this paper is more suitable for actual congestion control.
In Figures 6–8, the tracking error of the five algorithms tends to 0 stably, but EO-PID and PSO-PID have obvious jitter, while the tracking error of RED is stable but larger than that of other algorithms. Compared with other algorithms, the tracking error of the multi-bottleneck network and the single-bottleneck network in this paper is the most stable and the smallest. Careful comparison shows that the multi-bottleneck network in this paper is superior to the single-bottleneck network. It shows that the multi-bottleneck AQM algorithm in this paper has good robustness.
In this paper, a novel adaptive congestion control algorithm is developed specifically for multi-bottleneck TCP/AQM network system, considering both dead zone and saturated input interference for the first time. Combined with the approximation characteristics of the FLS and the backstepping technology, the algorithm regards the multi-bottleneck network as a cohesive entity. The adaptive fuzzy controller enhances the robustness of individual nodes within the multi-bottleneck network, and all the network nodes can track the required queue length according to different queue capacity. Finally, through simulation, the feasibility of the designed AQM network congestion control algorithm is verified.
The authors declare they have not used Artificial Intelligence (AI) tools in the creation of this article.
This project is funded by the National Natural Science Foundation of China, No. 61932005.
The authors have declared no conflicts of interest.
[1] |
V. Jacobson, Congestion avoidance and control, ACM SIGCOMM Comput. Commun. Rev., 18 (1988), 314–329. https://doi.org/10.1145/52325.52356 doi: 10.1145/52325.52356
![]() |
[2] | M. Mathis, J. Mahdavi, S. Floyd, A. Romanow, TCP selective acknowledgment options, Tech. Rep., 1996. |
[3] |
S. Ryu, C. Rump, C. Qiao, Advances in internet congestion control, IEEE Commun. Surv. Tut., 5 (2003), 28–39. https://doi.org/10.1109/COMST.2003.5342228 doi: 10.1109/COMST.2003.5342228
![]() |
[4] |
G. Chatranon, M. A. Labrador, S. Banerjee, A survey of tcp-friendly router-based AQM schemes, Comput. Commun., 27 (2004), 1424–1440. https://doi.org/10.1016/j.comcom.2004.05.001 doi: 10.1016/j.comcom.2004.05.001
![]() |
[5] | T. A. N. Nguyen, S. Gangadhar, J. P. Sterbenz, Performance evaluation of TCP congestion control algorithms in data center networks, In: Proceedings of the 11th International Conference on Future Internet Technologies, 2016, 21–28. https://doi.org/10.1145/2935663.2935669 |
[6] | B. Braden, D. Clark, J. Crowcroft, B. Davie, S. Deering, D. Estrin, et al., Recommendations on queue management and congestion avoidance in the internet, Tech. Rep., 1998. |
[7] |
S. Floyd, V. Jacobson, Random early detection gateways for congestion avoidance, IEEE/ACM T. Network., 1 (1993), 397–413. https://doi.org/10.1109/90.251892 doi: 10.1109/90.251892
![]() |
[8] | T. J. Ott, T. Lakshman, L. H. Wong, SRED: stabilized RED, In: IEEE INFOCOM'99. Conference on Computer Communications. Proceedings. Eighteenth Annual Joint Conference of the IEEE Computer and Communications Societies, 3 (1999), 1346–1355. |
[9] | D. Lin, R. Morris, Dynamics of random early detection, In: Proceedings of the ACM SIGCOMM'97 Conference on Applications, Technologies, Architectures, and Protocols for Computer Communication, 1997,127–137. |
[10] |
R. Adams, Active queue management: a survey, IEEE Commun. Surv. Tut., 15 (2012), 1425–1476. https://doi.org/10.1109/SURV.2012.082212.00018 doi: 10.1109/SURV.2012.082212.00018
![]() |
[11] | V. Misra, W. B. Gong, D. Towsley, Fluid-based analysis of a network of AQM routers supporting TCP flows with an application to RED, In: Proceedings of the Conference on Applications, Technologies, Architectures, and Protocols for Computer Communication, 2000,151–160. https://doi.org/10.1145/347059.347421 |
[12] |
F. Ren, C. Lin, X. Yin, Design a congestion controller based on sliding mode variable structure control, Comput. Commun., 28 (2005), 1050–1061. https://doi.org/10.1016/j.comcom.2004.06.011 doi: 10.1016/j.comcom.2004.06.011
![]() |
[13] | M. Yan, Y. Jing, X. Shen, Sliding mode aqm algorithm for TCP networks with uncertainty, J. Northeast. Uni. (Natural Science), 29 (2008), 157. |
[14] |
Y. Liu, X. Liu, Y. Jing, S. Zhou, Adaptive backstepping h∞ tracking control with prescribed performance for internet congestion, ISA Transact., 72 (2018), 92–99. https://doi.org/10.1016/j.isatra.2017.10.004 doi: 10.1016/j.isatra.2017.10.004
![]() |
[15] |
X. Qi, H. Ma, Y. Jing, A novel congestion controller with prescribed settling time for TCP/AQM network system, IEEE Transact. Network Sci. Eng., 9 (2022), 4065–4074. https://doi.org/10.1109/TNSE.2022.3195749 doi: 10.1109/TNSE.2022.3195749
![]() |
[16] |
M. Sheikhan, R. Shahnazi, E. Hemmati, Adaptive active queue management controller for tcp communication networks using pso-rbf models, Neural Comput. Applic., 22 (2013), 933–945. https://doi.org/10.1007/s00521-011-0786-0 doi: 10.1007/s00521-011-0786-0
![]() |
[17] |
P. Wang, D. Zhu, X. Lu, Active queue management algorithm based on data-driven predictive control, Telecommun. Syst., 64 (2017), 103–111. https://doi.org/10.1007/s11235-016-0162-6 doi: 10.1007/s11235-016-0162-6
![]() |
[18] |
W. Chang, Y. Meng, K. Tsai, AQM router design for TCP network via input constrained fuzzy control of time-delay affine takagi-sugeno fuzzy models, Int. J. Syst. Sci., 43 (2012), 2297–2313. https://doi.org/10.1080/00207721.2011.572197 doi: 10.1080/00207721.2011.572197
![]() |
[19] |
S. Xu, M. Fei, X. Yang, L. Wu, AQM scheme design for TCP network via takagi-sugeno fuzzy method, Complexity, 21 (2016), 606–612. https://doi.org/10.1002/cplx.21838 doi: 10.1002/cplx.21838
![]() |
[20] |
M. Sheikhan, R. Shahnazi, E. Hemmati, Adaptive active queue management controller for tcp communication networks using pso-rbf models, Neural Comput. Applic., 22 (2013), 933–945. https://doi.org/10.1007/s00521-011-0786-0 doi: 10.1007/s00521-011-0786-0
![]() |
[21] | M. Lin, T. Ren, H. Yuan, M. Li, The congestion control for TCP network based on input/output saturation, In: 2017 29th Chinese Control And Decision Conference (CCDC), 2017, 1166–1171. https://doi.org/10.1109/CCDC.2017.7978695 |
[22] |
S. Belamfedel Alaoui, E. H. Tissir, N. Chaibi, F. El Haoussi, Regional stabilization and h∞ congestion control with input saturation, Transact. Inst. Meas. Control, 43 (2021), 2196–2212. https://doi.org/10.1177/0142331221992739 doi: 10.1177/0142331221992739
![]() |
[23] |
J. Shen, Y. Jing, T. Ren, Adaptive finite time congestion tracking control for TCP/AQM system with input-saturation, Int. J. Syst. Sci., 53 (2022), 253–264. https://doi.org/10.1080/00207721.2021.1947412 doi: 10.1080/00207721.2021.1947412
![]() |
[24] |
B. Sadek, T. El Houssaine, K. Barbosa, A. J. Rojas, Consensus congestion control for ad hoc networks: Time-delay and saturation, IEEE Transact. Network Sci. Eng., 10 (2023), 1809–1821. https://doi.org/10.1109/TNSE.2023.3235303 doi: 10.1109/TNSE.2023.3235303
![]() |
[25] |
J. Yan, K. Shyu, J. Lin, Adaptive variable structure control for uncertain chaotic systems containing dead-zone nonlinearity, Chaos Solitons Fract., 25 (2005), 347–355. https://doi.org/10.1016/j.chaos.2004.11.013 doi: 10.1016/j.chaos.2004.11.013
![]() |
[26] |
K. Shyu, W. Liu, K. Hsu, Design of large-scale time-delayed systems with dead-zone input via variable structure control, Automatica, 41 (2005), 1239–1246. https://doi.org/10.1016/j.automatica.2005.03.004 doi: 10.1016/j.automatica.2005.03.004
![]() |
[27] |
W. Zhao, L. Liu, Y. Liu, Adaptive neural network control for nonlinear state constrained systems with unknown dead-zones input, AIMS Math., 5 (2020), 4065–4084. https://doi.org/10.3934/math.2020261 doi: 10.3934/math.2020261
![]() |
[28] |
W. Lv, J. Park, J. Lu, R. Guo, Adaptive fuzzy output feedback control for a class of uncertain nonlinear systems in the presence of sensor attacks, J. Franklin Instit., 360 (2023), 2326–2343. https://doi.org/10.1016/j.jfranklin.2022.10.047 doi: 10.1016/j.jfranklin.2022.10.047
![]() |
[29] |
R. Guo, S. Xu, J. Guo, Sliding-mode synchronization control of complex-valued inertial neural networks with leakage delay and time-varying delays, IEEE Transact. Syst. Man Cybernet. Syst., 1095–1103, 2023. https://doi.org/10.1109/TSMC.2022.3193306 doi: 10.1109/TSMC.2022.3193306
![]() |
[30] |
S. Arbatsofla, A. Mazinan, M. Mahmoodabadi, M. A. Nekoui, Fuzzy fractional-order adaptive robust feedback linearization control optimized by the multi-objective artificial hummingbird algorithm for a nonlinear ball–wheel system, J. Braz. Soc. Mech. Sci. Eng., 45 (2023), 575. https://doi.org/10.1007/s40430-023-04455-9 doi: 10.1007/s40430-023-04455-9
![]() |
[31] |
W. Zheng, Y. Li, X. Jing, S. Liu, Adaptive finite-time congestion control for uncertain TCP/AQM network with unknown hysteresis, Complexity, 2020 (2020), 4138390. https://doi.org/10.1155/2020/4138390 doi: 10.1155/2020/4138390
![]() |
[32] |
O. Kahouli, A. Turki, M. Ksantini, M. Ali Hammami, A. Aloui, On the boundedness of solutions of some fuzzy dynamical control systems, AIMS Math., 9 (2024), 5330–5348. https://doi.org/10.3934/math.2024257 doi: 10.3934/math.2024257
![]() |
[33] |
X. Liu, Y. Li, Y. Li, Adaptive tracking control for a class of uncertain switched stochastic nonlinear systems, Adv. Differ. Equ., 2019 (2019), 33. https://doi.org/10.1186/s13662-019-1975-x doi: 10.1186/s13662-019-1975-x
![]() |
[34] |
T. Gao, T. Li, Y. Liu, S. Tong, F. Sun, Observer-based adaptive fuzzy control of non-strict feedback nonlinear systems with function constraints, IEEE Transact. Fuzzy Syst., 31 (2022), 2556–2567. https://doi.org/10.1109/TFUZZ.2022.3228319 doi: 10.1109/TFUZZ.2022.3228319
![]() |
[35] | S. Mohammadi, H. Pour, M. Jafari, A. Javadi, Fuzzy-based pid active queue manager for TCP/IP networks, In: 10th International Conference on Information Science, Signal Processing and their Applications (ISSPA 2010), 2010,434–439. https://doi.org/10.1109/ISSPA.2010.5605462 |
[36] | J. Chen, Y. Jing, X. Qi, TCP/AQM network congestion prescribed performance event-triggered control with input saturation, In: 2021 33rd Chinese Control and Decision Conference (CCDC), 2021, 6602–6607. https://doi.org/10.1109/CCDC52312.2021.9602224 |
[37] |
Z. Zhang, Z. Liu, N. Liu, W. He, F. Yang, Adaptive tracking control for nonlinear systems with uncertain control gains and its application to a TCP/AQM network, Sci. Rep., 13 (2023), 14625. https://doi.org/10.1038/s41598-023-41799-7 doi: 10.1038/s41598-023-41799-7
![]() |
[38] | L. Wang, Adaptive Fuzzy Systems and Control: Design and Stability Analysis, Upper Saddle River: Prentice-Hall, Inc., 1994. |
[39] |
O. Micev, M. Calasan, Design and robustness analysis of an automatic voltage regulator system controller by using equilibrium optimizer algorithm, Compute. Elect. Eng., 89 (2021), 106930. https://doi.org/10.1016/j.compeleceng.2020.106930 doi: 10.1016/j.compeleceng.2020.106930
![]() |
1. | Yanxin Li, Jiqing Chen, Shangkun Liu, Weimin Zheng, Runan Guo, Fixed-Time Congestion Control for a Class of Uncertain Multi-Bottleneck TCP/AWM Networks, 2024, 13, 2076-0825, 388, 10.3390/act13100388 |