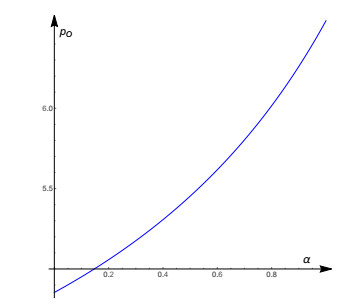
In this article, we investigate the Wong-Zakai approximations of a class of second order non-autonomous stochastic lattice systems with additive white noise. We first prove the existence and uniqueness of tempered pullback random attractors for the original stochastic system and its Wong-Zakai approximation. Then, we establish the upper semicontinuity of these attractors for Wong-Zakai approximations as the step-length of the Wiener shift approaches zero.
Citation: Xintao Li. Wong-Zakai approximations and long term behavior of second order non-autonomous stochastic lattice dynamical systems with additive noise[J]. AIMS Mathematics, 2022, 7(5): 7569-7594. doi: 10.3934/math.2022425
[1] | Cahya Sutowo, Galih Senopati, Andika W Pramono, Sugeng Supriadi, Bambang Suharno . Microstructures, mechanical properties, and corrosion behavior of novel multi-component Ti-6Mo-6Nb-xSn-xMn alloys for biomedical applications. AIMS Materials Science, 2020, 7(2): 192-202. doi: 10.3934/matersci.2020.2.192 |
[2] | Kipkurui N Ronoh, Fredrick M Mwema, Stephen A Akinlabi, Esther T Akinlabi, Nancy W Karuri, Harrison T Ngetha . Effects of cooling conditions and grinding depth on sustainable surface grinding of Ti-6Al-4V: Taguchi approach. AIMS Materials Science, 2019, 6(5): 697-712. doi: 10.3934/matersci.2019.5.697 |
[3] | Daniela Šuryová, Igor Kostolný, Roman Koleňák . Fluxless ultrasonic soldering of SiC ceramics and Cu by Bi–Ag–Ti based solder. AIMS Materials Science, 2020, 7(1): 24-32. doi: 10.3934/matersci.2020.1.24 |
[4] | Muhammad Awwaluddin, Sri Hastuty, Djoko Hadi Prajitno, Makmuri, Budi Prasetiyo, Yudi Irawadi, Jekki Hendrawan, Harry Purnama, Eko Agus Nugroho . Effect of Yttrium on corrosion resistance of Zr-based alloys in Ringer's lactate solution for biomaterial applications. AIMS Materials Science, 2024, 11(3): 565-584. doi: 10.3934/matersci.2024028 |
[5] | Harish Mooli, Srinivasa Rao Seeram, Satyanarayana Goteti, Nageswara Rao Boggarapu . Optimal weld bead profiles in the conduction mode LBW of thin Ti–6Al–4V alloy sheets. AIMS Materials Science, 2021, 8(5): 698-715. doi: 10.3934/matersci.2021042 |
[6] | Jie Sun, Tzvetanka Boiadjieva-Scherzer, Hermann Kronberger, Kevin Staats, Johannes Holinka, Reinhard Windhager . Surface modification of Ti6Al4V alloy for implants by anodization and electrodeposition. AIMS Materials Science, 2019, 6(5): 713-729. doi: 10.3934/matersci.2019.5.713 |
[7] | Muhammad Awwaluddin, Djoko Hadi Prajitno, Wisnu Ari Adi, Maman Kartaman, Tresna P. Soemardi . Mechanical properties and corrosion behavior of novel β-type biomaterial Zr-6Mo-4Ti-xY alloys in simulated body fluid Ringer's lactate solution for implant applications. AIMS Materials Science, 2020, 7(6): 887-901. doi: 10.3934/matersci.2020.6.887 |
[8] | Nikolay A. Voronin . Analysis of the mechanisms of deformation of topocomposites by modeling of the indentation load-displacement curves. AIMS Materials Science, 2019, 6(3): 397-405. doi: 10.3934/matersci.2019.3.397 |
[9] | Liang Zhao, Weimian Guan, Jiwen Xu, Zhiyuan Sun, Maoda Zhang, Junjie Zhang . Atomistic investigation of effect of twin boundary on machinability in diamond cutting of nanocrystalline 3C-SiC. AIMS Materials Science, 2024, 11(6): 1149-1164. doi: 10.3934/matersci.2024056 |
[10] | Valeri S. Harutyunyan, Ashot P. Aivazyan, Andrey N. Avagyan . Stress field of a near-surface basal screw dislocation in elastically anisotropic hexagonal crystals. AIMS Materials Science, 2017, 4(6): 1202-1219. doi: 10.3934/matersci.2017.6.1202 |
In this article, we investigate the Wong-Zakai approximations of a class of second order non-autonomous stochastic lattice systems with additive white noise. We first prove the existence and uniqueness of tempered pullback random attractors for the original stochastic system and its Wong-Zakai approximation. Then, we establish the upper semicontinuity of these attractors for Wong-Zakai approximations as the step-length of the Wiener shift approaches zero.
The models involving entry-exit decisions apply to many situations, as stated in [1,2]. Such models may be described simply as follows. A firm decides when to invest in or when to abandon a project that can bring profit. There is plenty of literature on models concerning how to schedule the timing under the assumption that exiting the project does not take time, so we do not list them in detail, but refer to [1,2,3,4,5,6,7,8,9]. The ideas of entry-exit decisions apply to many concrete issues, for example, relationships of globalization and entrepreneurial entry-exit [10], when to invest or expand a start-up firm [11], when to enter or exit stock markets [12], and how to make a schedule for buying carbon emission rights [13].
In practice, the exiting process may take a long time, so ignoring it is not reasonable. For example, Brexit took more than 3 years, from June 23, 2016 to January 31, 2020 (https://www.britannica.com/topic/Brexit). The models [14,15] take account of the time, but with equal output rates in the regular production and exit periods. It is an apparent feature that the output rate of the project is usually reduced during the exit period. In this paper, we introduce a parameter to describe the output rate during the exit period and completely discuss the effects of output reduction on entry-exit decisions, under the assumption that the commodity price of the project follows a geometric Brownian motion.
We use the optimal stopping theory to carry out the study and obtain the explicit expressions of the optimal activating time and start time of the exit, which is one of the contributions of the paper. With these explicit expressions in hand, we can carefully analyze the effects of output reduction on entry-exit decisions, which is another contribution of the paper.
If the optimal choice is never to exit the project, the output reduction has no effect on the optimal entry-exit decision.
If the optimal exit is in a finite time, the situation becomes complicated. However, we obtain complete criterions, which determine the effects of output reduction on entry-exit decisions (see Section 4).
We outline the structure of this paper. In Section 2, we describe the model in detail. In Section 3, we determine an optimal entry-exit decision. In Section 4, we discuss the effects of output reduction during exit period on entry-exit decisions. Some conclusions are drawn in Section 5.
Assume that the price process P of one unit product follows
dP(t)=μP(t)dt+σP(t)dB(t)andP(0)=p, | (2.1) |
where μ∈R, σ,p>0, and B is a one dimensional standard Brownian motion, which denotes uncertainty. In this paper, all times are stopping times w.r.t. the filtration generated by the Brownian motion B.
Since involving the construction period leads to complicated calculations and distracts us from analyzing the effects of reduction on entry-exit decisions, and the effects of the construction period have been discussed in [3,15], we assume that there is no construction period (it may happen when the firm buys a project). The firm activates the project at time τI with the entry cost KI and completes the abandonment of the project during the time interval [τO,τO+δ], with the exit cost valued at KO at time τO+δ. Without loss of generality, we assume that the firm produces one unit product per unit time during the period [τI,τO] at the marginal cost C and α (0≤α≤1) unit products per unit time during the time interval of exiting the project [τO,τO+δ].
To answer the two questions, what time is optimal to activate the project and what time is optimal to start the abandonment procedure, we solve the optimization problem
J(p)=supτI≤τOEp[∫τOτIexp(−rt)(P(t)−C)dt+α∫τO+δτOexp(−rt)(P(t)−C)dt−exp(−rτI)KI−exp(−r(τO+δ))KO]. | (2.2) |
We call stopping times τI and τO the activating times and start times of the exit, respectively, and we call the function J the maximal expected present value of the project.
If r≤μ, some straight calculations show us that
Ep[∫+∞0exp(−rt)(P(t)−C)dt]=+∞. |
Thus, we obtain the following result.
Theorem 3.1. Assume that r≤μ, then τ∗I: =0 is an optimal activating time and τ∗O: =+∞ is an optimal start time of the exit, i.e., the firm should never exit the project. In addition, the function J in (2.2) is given by J≡+∞.
In the rest of this section, we assume r>μ.
Taking τI=τO: =0 in (2.2), we have
J(p)≥−KI+α(1−exp((μ−r)δ))r−μp−exp(−rδ)KO−αCr(1−exp(−rδ)), |
thus, in the remains of this section, we always assume that
rKI+exp(−rδ)rKO+α(1−exp(−rδ))C≥0 |
to avoid arbitrage opportunities.
Let λ1 and λ2 be the solutions to the equation
r−μλ−12σ2λ(λ−1)=0 |
with λ1<λ2, then we have λ1<0 and λ2>1.
Theorem 3.2. Assume that r>μ. The following are true:
(ⅰ) If
(1−α)C+(αC−rKO)exp(−rδ)≤0, |
then (τ∗I,τ∗O) is a solution to (2.2), where
τ∗I=inf{t:t>0,P(t)≥pI} |
and τ∗O=+∞. Here,
pI=λ2λ2−1(r−μ)(Cr+KI). |
In addition,
J(p)={Bpλ2,ifp<pI,pr−μ−Cr−KI,ifp≥pI, |
where
B=pI1−λ2λ2(r−μ). |
(ⅱ) If
(1−α)C+(αC−rKO)exp(−rδ)>0 |
and
α(1−exp((μ−r)δ))pO−αC(1−exp(−rδ))−exp(−rδ)rKO−rKI≤0, | (3.1) |
where
pO=λ1λ1−1r−μ1−α+αexp((μ−r)δ)((1−α)Cr+exp(−rδ)(αCr−KO)), |
then (τ∗I,τ∗O) is a solution to (2.2), where
τ∗I=inf{t:t>0,P(t)≥pI} |
and
τ∗O=inf{t:t>τ∗I,P(t)≤pO}. |
Here, pI is the largest solution of the algebraic equation
A(λ2−λ1)pλ1I+(λ2−1)r−μpI−λ2(Cr+KI)=0. |
In addition,
J(p)={Bpλ2,ifp<pI,Apλ1+pr−μ−Cr−KI,ifp≥pI, |
where
A=1−α+αexp((μ−r)δ)λ1(μ−r)pO1−λ1 |
and
B=λ1λ−12Apλ1−λ2I+pI1−λ2λ2(r−μ). |
Remark 3.3. We propose condition (3.1) to eliminate the possibility that the firm enters the project at a trigger price lower than the optimal trigger price of the exit, i.e., the firm enters the project and then immediately decides to exit the project.
In light of [14, Theorems 3.1 and 5.2], we have the following Lemma 3.4, which serves as preparation for the proof of Theorem 3.2.
Lemma 3.4. If (τ∗1,τ∗2) is a solution to the optimization problem
˜J(p):=supτI≤τOEp[∫τOτIexp(−rt)(P(t)−C)dt−exp(−rτI)KI−exp(−rτO)(l1P(τO)+l0)], | (3.2) |
it is also a solution to (2.2) and J(x)=˜J(x), where
l1:=α(1−exp((μ−r)δ))μ−r |
and
l0:=αCr(1−exp(−rδ))+exp(−rδ)KO. |
Proof. By the strong Markov property of the process {(s+t,P(t)),t≥0}, where s∈R, we have
Ep[∫τOτIexp(−rt)(P(t)−C)dt+α∫τO+δτOexp(−rt)(P(t)−C)dt−exp(−rτI)KI−exp(−r(τO+δ))KO]=Ep[∫τOτIexp(−rt)(P(t)−C)dt+αexp(−rτO)EP(τO)[∫δ0exp(−rt)(P(t)−C)dt] −exp(−rτI)KI−exp(−r(τO+δ))KO]. |
Thus, we need to calculate
αEP(τO)[∫δ0exp(−rt)(P(t)−C)dt]−exp(−rδ)KO=α∫δ0exp(−rt)(exp(μt)P(τO)−C)dt−exp(−rδ)KO=−l1P(τO)−l0. |
The proof is complete.
Remark 3.5. The proof of Lemma 3.4 has an economic meaning as follows. We first discount the benefit during the abandonment period to time τO, then discount this value to time zero.
With the help of Lemma 3.4, we can prove Theorem 3.2.
Proof of Theorem 3.2. (1) By Lemma 3.4, we solve problem (3.2),
supτI≤τOEp[∫τOτIexp(−rt)(P(t)−C)dt−exp(−rτI)KI−exp(−rτO)(l1P(τO)+l0)]=supτI≤τOEp[exp(−rτI)∫τO−τI0exp(−rt)(P(t+τI)−C)dt−exp(−rτI)KI−exp(−rτO)(l1P(τO)+l0)]=supτIEp[exp(−rτI)(G(P(τI))−KI)]=:H(p), | (3.3) |
where
G(p):=supτOEp[∫τO0exp(−rt)(P(t)−C)dt−exp(−rτO)(l1P(τO)+l0)]. | (3.4) |
(2) Assume
(1−α)C+(αC−rKO)exp(−rδ)≤0. |
We first solve problem (3.4) and then problem (3.3).
For problem (3.4), noting
Ep[∫∞0exp(−rt)(P(t)−C)dt]=pr−μ−Cr, |
we see that
Ep[∫∞0exp(−rt)(P(t)−C)dt]≥−l1p−l0, |
which implies
τ∗O=+∞ |
and
G(p)=pr−μ−Cr. |
Set
h(p):=pr−μ−Cr−KI. |
Since
{p:p>0andrh(p)−μph′(p)≥0}={p:p≥C+rKI}, |
the exercise region of problem (3.3) takes the form [pI,+∞) for some
pI≥C+rKI. |
The function H satisfies
rH−μpH′−12σ2p2H″=0 |
on the continuation region (0,pI) and is Lipschitz continuous on (0,+∞) and C1 continuous at pI. Thus, we get
H(p)=Bpλ2 |
and B and pI solve
{Bpλ2I=pIr−μ−Cr−KI,λ2Bpλ2−1I=1r−μ, |
by which we finish the proof of (ⅰ).
(3) Assume
(1−α)C+(αC−rKO)exp(−rδ)>0 |
and (3.1) hold. We again first solve problem (3.4) and then problem (3.3).
Set
g(p):=−l1p−l0. |
A straight calculation shows that
{p:p>0andrg−μpg′(p)−p+C≥0}=(0,(1−α)C+(αC−rKO)exp(−rδ)1−α(1−exp((μ−r)δ))), |
which means the exercise region of problem (3.4) takes the form (0,pO] for some
pO≤(1−α)C+(αC−rKO)exp(−rδ)1−α(1−exp((μ−r)δ)). |
The function G satisfies
rG−μpG′−12σ2p2G″−p+C=0 |
on the continuation region (pO,+∞) and is Lipschitz continuous on (0,+∞) and C1 continuous at pO. Thus, we get
G(p)=Apλ1+pr−μ−Cr, |
and A and pO solve
{Apλ1O+pOr−μ−Cr=−l1pO−l0,λ1Apλ1−1O+1r−μ=−l1, |
which implies coefficient A and the optimal exit trigger pricer of (ⅱ).
To solve problem (3.4), we define
h(p):=G(p)−KI. |
In light of (3.1),
{p:p>0andrh(p)−μph′(p)≥0}={p:p≥C+rKI}, |
thus, the exercise region of problem (3.3) takes the form [pI,+∞) for some
pI≥C+rKI. |
The function H satisfies
rH−μpH′−12σ2p2H″=0 |
on the continuation region (0,pI) and is Lipschitz continuous on (0,+∞) and C1 continuous at pI. Thus, we get
H(p)=Bpλ2, |
and B and pI solve
{Bpλ2I=Apλ1I+pIr−μ−Cr−KI,λ2Bpλ2−1I=λ1Apλ1−1I+1r−μ, |
by which we finish the proof of (ⅱ).
We analyze the effects of output reduction during the exit period on entry-exit decisions.
If r≤μ, the firm has an optimal time τ∗I=0 to activate the project and should never exit the project. Thus, the reduction does not affect entry-exit decisions.
If r>μ and
(1−α)C+(αC−rKO)exp(−rδ)≤0, |
the optimal time to activate the project is given by
τ∗I=inf{t:t>0,P(t)≥pI}, |
where
pI=λ2λ2−1(r−μ)(Cr+KI). |
Thus, the reduction does not affect the optimal activating time. As same as the case of r≤μ, the firm should never exit the project and the reduction does not affect exit decisions.
Assume that r>μ and
(1−α)C+(αC−rKO)exp(−rδ)>0. |
We first analyze the effects of reduction on the optimal start time of the exit. By (ⅱ) of Theorem 3.2, the optimal trigger price is an increasing function of α if
exp(−μδ)(C−rKO)>C−exp(−rδ)rKO, |
a decreasing function if
exp(−μδ)(C−rKO)<C−exp(−rδ)rKO, |
and a constant function if
exp(−μδ)(C−rKO)=C−exp(−rδ)rKO. |
We list some examples to illustrate the analysis. Taking
r=0.2,μ=−0.1,σ=0.3,δ=2,C=5,KI=20,andKO=−10, |
we have
exp(−μδ)(C−rKO)>C−exp(−rδ)rKO, |
then the optimal trigger price is an increasing function of α. See Figure 1. If we replace μ=−0.1 with μ=0.1, we have a decreasing function. See Figure 2.
We conclude that:
(1) If
exp(−μδ)(C−rKO)>C−exp(−rδ)rKO, |
as the reduction increases (i.e., α decreases), the firm will postpone the exit.
(2) If
exp(−μδ)(C−rKO)<C−exp(−rδ)rKO, |
as the reduction increases, the firm will advance the exit.
(3) If
exp(−μδ)(C−rKO)=C−exp(−rδ)rKO, |
the reduction does not affect the exit.
To analyze the effects of reduction on the optimal time to activate the project, we define two functions as follows:
f(p):=A(λ2−λ1)pλ1 |
and
g(p):=−(λ2−1)r−μp+λ2(Cr+KI) |
according to (ⅱ) of Theorem 3.2. Thus, the functions f and g intersect at two points, say, (p1,f(p1)) and (p2,f(p2)) with p1<p2, and the optimal trigger price pI of activating the project is given by pI=p2.
To capture the behavior of pI as α varies, we only need to examine the behavior of the coefficient A as α varies. Setting
M:=(C−exp(−rδ)rKO)(1−exp((μ−r)δ))+(1−λ1)exp(−rδ)(C−rKO−Cexp(μδ)+exp((μ−r)δ)rKO) |
and
N:=−C(1−exp(−rδ))(1−exp((μ−r)δ)), |
after some calculations, we find that:
(1) If M/N≤0, A is increasing on [0,1] and pI is decreasing on [0,1].
(2) If M/N≥1, A is decreasing on [0,1] and pI is increasing on [0,1].
(3) If 0<M/N<1, A is decreasing on [0,M/N] and increasing on [M/N,1] and pI is increasing on [0,M/N] and decreasing on [M/N,1].
Numerical examples help us understand the analysis above.
Figure 3 demonstrates the decreasing of pI (r=0.2, μ=−0.1, σ=0.3, δ=0.6, C=5, KI=20, and KO=−10). Figure 4 demonstrates the increasing of pI (r=0.2, μ=0.1, σ=0.3, δ=2, C=5, KI=20, and KO=−10), and Figure 5 demonstrates the non-monotonicity of pI (r=0.2, μ=−0.1, σ=0.3, δ=0.6, C=5, KI=20, and KO=−10).
In other words, here are the effects of the reduction on the optimal time of activating the project:
(1) If M/N≤0, as the reduction increases (i.e., α decreases), the firm will postpone activating the project.
(2) If M/N≥0, as the reduction increases, the firm will advance activating the project.
(3) If 0<M/N<1, as the reduction increases, the firm will postpone activating the project then advance activating the project.
There is an apparent phenomenon that firms usually reduce their output rate during stopping the regular production of a project. We intend to analyze the effects of reduction on the optimal entry-exit decision. Since the papers [3,15] have investigated the effects of the construction period on entry-exit decisions, we assume that the project has been constructed and the production immediately starts for concentrating on the study of the effects of reduction.
We introduce the output rate into the models [14,15] and describe the problem using the optimal stopping theory, obtaining explicit solutions in Theorems 3.1 and 3.2. These explicit solutions help us in discovering the effects of reduction on the optimal entry-exit decision.
We carefully examine the effects of reduction. According the analysis listed in Section 4, we come to the effects of reduction as follows. If the firm should never exit the project to obtain the maximal profit, the reduction does not affect the optimal entry-exit decision. However, if the firm exits the project in finite time, the effects show in a complicated manner. We study the effects analytically and numerically, and provide the relations of the parameters involved in the model that can determine the effects.
The author declares he has not used Artificial Intelligence (AI) tools in the creation of this article.
Many thanks are due to the editors and reviewers for their constructive suggestions and valuable comments. This work is partially supported by the Fundamental Research Funds for the Central Universities (Grant No. N2023034).
No potential conflict of interest exists regarding the publication of this paper.
[1] | L. Arnold, Random dynamical systems, 1 Eds., Berlin: Springer, 1998. http://dx.doi.org/10.1007/978-3-662-12878-7 |
[2] |
P. W. Bates, H. Lisei, K. Lu, Attractors for stochastic lattice dynamical systems, Stoch. Dyn., 6 (2006), 1–21. http://dx.doi.org/10.1142/S0219493706001621 doi: 10.1142/S0219493706001621
![]() |
[3] |
Z. Brzeźniak, U. Manna, D. Mukherjee, Wong-Zakai approximation for the stochastic Landau-Lifshitz-Gilbert equations, J. Differ. Equations, 267 (2019), 776–825. http://dx.doi.org/10.1016/j.jde.2019.01.025 doi: 10.1016/j.jde.2019.01.025
![]() |
[4] |
T. L. Carrol, L. M. Pecora, Synchronization in chaotic systems, Phys. Rev. Lett., 64 (1990), 821–824. http://dx.doi.org/10.1103/PhysRevLett.64.821 doi: 10.1103/PhysRevLett.64.821
![]() |
[5] |
P. Chen, R. Wang, X. Zhang, Long-time dynamics of fractional nonclassical diffusion equations with nonlinear colored noise and delay on unbounded domains, B. Sci. Math., 173 (2021), 103071. http://dx.doi.org/10.1016/j.bulsci.2021.103071 doi: 10.1016/j.bulsci.2021.103071
![]() |
[6] |
C. Cheng, Z. Feng, Y. Su, Global stability of traveling wave fronts for a reaction-diffusion system with a quiescent stage on a one-dimensional spatial lattice, Appl. Anal., 97 (2018), 2920–2940. http://dx.doi.org/10.1080/00036811.2017.1395864 doi: 10.1080/00036811.2017.1395864
![]() |
[7] | L. O. Chua, T. Roska, The CNN paradigm, IEEE Trans. Circuits Syst., 40 (1993), 147–156. http://dx.doi.org/10.1109/81.222795 |
[8] |
X. Ding, J. Jiang, Random attractors for stochastic retarded lattice dynamical systems, Abstract. Appl. Anal., 2012 (2012), 409282. http://dx.doi.org/10.1155/2012/409282 doi: 10.1155/2012/409282
![]() |
[9] |
A. Gu, Asymptotic behavior of random lattice dynamical systems and their wong-zakai approximations, Discrete Contin. Dyn. Syst. B, 24 (2019), 5737–5767. http://dx.doi.org/10.3934/dcdsb.2019104 doi: 10.3934/dcdsb.2019104
![]() |
[10] |
A. Gu, K. Lu, B. Wang, Asymptotic behavior of random Navier-Stokes equations driven by Wong-Zakai approximations, Discrete Contin. Dyn. Syst., 39 (2019), 185–218. http://dx.doi.org/10.3934/dcds.2019008 doi: 10.3934/dcds.2019008
![]() |
[11] |
A. Gu, B. Guo, B. Wang, Long term behavior of random Navier-Stokes equations driven by colored noise, Discrete Contin. Dyn. Syst. B, 25 (2020), 2495–2532. http://dx.doi.org/10.3934/dcdsb.2020020 doi: 10.3934/dcdsb.2020020
![]() |
[12] |
J. Guo, C. Wu, The existence of traveling wave solutions for a bistable three-component lattice dynamical system, J. Differ. Equations, 260 (2016), 1445–1455. http://dx.doi.org/10.1016/j.jde.2015.09.036 doi: 10.1016/j.jde.2015.09.036
![]() |
[13] |
Z. Han, S. Zhou, Random uniform exponential attractors for non-autonomous stochastic lattice systems and FitzHugh-Nagumo lattice systems with quasi-periodic forces and multiplicative noise, Stoch. Dyn., 20 (2020), 2050036. http://dx.doi.org/10.1142/S0219493720500367 doi: 10.1142/S0219493720500367
![]() |
[14] |
R. Kapral, Discrete models for chemically reacting systems, J. Math. Chem., 6 (1991), 113–163. http://dx.doi.org/10.1007/BF01192578 doi: 10.1007/BF01192578
![]() |
[15] |
D. Li, L. Shi, Upper semicontinuity of random attractors of stochastic discrete complex Ginzburg-Landau equations with time-varying delays in the delay, J. Differ. Equ. Appl., 24 (2018), 872–897. http://dx.doi.org/10.1080/10236198.2018.1437913 doi: 10.1080/10236198.2018.1437913
![]() |
[16] |
D. Li, L. Shi, X. Wang, Long term behavior of stochastic discrete complex ginzburg-landau equations with time delays in weighted spaces, Discrete Contin. Dyn. Syst. B, 24 (2019), 5121–5148. http://dx.doi.org/10.3934/dcdsb.2019046 doi: 10.3934/dcdsb.2019046
![]() |
[17] |
K. Lu, B. Wang, Wong-Zakai approximations and long term behavior of stochastic partial differential equations, J. Dyn. Diff. Equat., 31 (2019), 1341–1371. http://dx.doi.org/10.1007/s10884-017-9626-y doi: 10.1007/s10884-017-9626-y
![]() |
[18] |
K. Lu, Q. Wang, Chaotic behavior in differential equations driven by a Brownian motion, J. Differ. Equations, 251 (2011), 2853–2895. http://dx.doi.org/10.1016/j.jde.2011.05.032 doi: 10.1016/j.jde.2011.05.032
![]() |
[19] |
J. Malletparet, S. Chow, Pattern formation and spatial chaos in lattice dynamical systems I, IEEE Transactions on Circuits Systems I Fundamental Theory Applications, 42 (2002), 746–751. http://dx.doi.org/10.1109/81.473583 doi: 10.1109/81.473583
![]() |
[20] |
U. Manna, D. Mukherjee, A. A. Panda, Wong-Zakai approximation for the stochastic Landau-Lifshitz-Gilbert equations with anisotropy energy, J. Math. Anal. Appl., 480 (2019), 123384. http://dx.doi.org/10.1016/j.jmaa.2019.123384 doi: 10.1016/j.jmaa.2019.123384
![]() |
[21] |
L. She, R. Wang, Regularity, forward-compactness and measurability of attractors for non-autonomous stochastic lattice systems, J. Math. Anal. Appl., 479 (2019), 2007–2031. http://dx.doi.org/10.1016/j.jmaa.2019.07.038 doi: 10.1016/j.jmaa.2019.07.038
![]() |
[22] |
J. Shen, K. Lu, W. Zhang, Heteroclinic chaotic behavior driven by a Brownian motion, J. Differ. Equations, 255 (2013), 4185–4225. http://dx.doi.org/10.1016/j.jde.2013.08.003 doi: 10.1016/j.jde.2013.08.003
![]() |
[23] |
H. Su, S. Zhou, L. Wu, Random exponential attractor for second-order nonautonomous stochastic lattice systems with multiplicative white noise, Stoch. Dyn., 19 (2019), 1950044. http://dx.doi.org/10.1142/S0219493719500448 doi: 10.1142/S0219493719500448
![]() |
[24] |
B. Wang, Existence and upper semicontinuity of attractors for stochastic equations with deterministic non-autonomous terms, Stoch. Dyn., 14 (2014), 1450009. http://dx.doi.org/10.1142/S0219493714500099 doi: 10.1142/S0219493714500099
![]() |
[25] |
X. Wang, D. Li, J. Shen, Wong-Zakai approximations and attractors for stochastic wave equations driven by additive noise, Discrete Contin. Dyn. Syst. B, 26 (2021), 2829–2855. http://dx.doi.org/10.3934/dcdsb.2020207 doi: 10.3934/dcdsb.2020207
![]() |
[26] |
R. Wang, Y. Li, B. Wang, Random dynamics of fractional nonclassical diffusion equations driven by colored noise, Discrete Contin. Dyn. Syst., 39 (2019), 4091–4126. http://dx.doi.org/10.3934/dcds.2019165 doi: 10.3934/dcds.2019165
![]() |
[27] |
X. Wang, S. Li, D. Xu, Random attractors for second-order stochastic lattice dynamical systems, Nonlinear. Anal. Theor., 72 (2010), 483–494. http://dx.doi.org/10.1016/j.na.2009.06.094 doi: 10.1016/j.na.2009.06.094
![]() |
[28] |
X. Wang, K. Lu, B. Wang, Wong-Zakai approximations and attractors for stochastic reaction-diffusion equations on unbounded domains, J. Differ. Equations, 264 (2018), 378–424. http://dx.doi.org/10.1016/j.jde.2017.09.006 doi: 10.1016/j.jde.2017.09.006
![]() |
[29] | X. Wang, K. Lu, B. Wang, Stationary approximations of stochastic wave equations on unbounded domains with critical exponents, J. Math. Phys., 62 (2021) 092702. http://dx.doi.org/10.1063/5.0011987 |
[30] |
X. Wang, J. Shen, K. Lu, B. Wang, Wong-Zakai approximations and random attractors for non-autonomous stochastic lattice systems, J. Differ. Equations, 280 (2021), 477–516. http://dx.doi.org/10.1016/j.jde.2021.01.026 doi: 10.1016/j.jde.2021.01.026
![]() |
[31] |
R. Wang, L. Shi, B. Wang, Asymptotic behavior of fractional nonclassical diffusion equations driven by nonlinear colored noise on RN, Nonlinearity, 32 (2019), 4524–4556. http://dx.doi.org/10.1088/1361-6544/ab32d7 doi: 10.1088/1361-6544/ab32d7
![]() |
[32] |
R. Wang, B. Wang, Random dynamics of P-Laplacian lattice systems driven by infinite-dimensional nonlinear noise, Stoch. Proc. Appl., 130 (2020), 7431–7462. http://dx.doi.org/10.1016/j.spa.2020.08.002 doi: 10.1016/j.spa.2020.08.002
![]() |
[33] |
R. L. Winalow, A. L. Kimball, A. Varghese, Simulating cartidiac sinus and atrial network dynamics on connection machine, Physica D, 64 (1993), 281–298. http://dx.doi.org/10.1016/0167-2789(93)90260-8 doi: 10.1016/0167-2789(93)90260-8
![]() |
[34] |
C. Wu, A general approach to the asymptotic behavior of traveling waves in a class of three-component lattice dynamical systems, J. Dyn. Differ. Equ., 28 (2016), 317–338. http://dx.doi.org/10.1007/s10884-016-9524-8 doi: 10.1007/s10884-016-9524-8
![]() |
[35] |
X. Xiang, S. Zhou, Random attractor for stochastic second-order non-autonomous stochastic lattice equations with dispersive term, J. Differ. Equ. Appl., 22 (2016), 235–252. http://dx.doi.org/10.1080/10236198.2015.1080694 doi: 10.1080/10236198.2015.1080694
![]() |
[36] |
L. Xu, W. Yan, Stochastic FitzHugh-Nagumo systems with delay, Taiwan. J. Math., 16 (2012), 1079–1103. http://dx.doi.org/10.11650/twjm/1500406680 doi: 10.11650/twjm/1500406680
![]() |
[37] |
W. Yan, Y. Li, S. Ji, Random attractors for first order stochastic retarded lattice dynamical systems, J. Math. Phys., 51 (2010), 032702. http://dx.doi.org/10.1063/1.3319566 doi: 10.1063/1.3319566
![]() |
[38] |
C. Zhang, L. Zhao, The attractors for 2nd-order stochastic delay lattice systems, Discrete Contin. Dyn. Sys., 37 (2017), 575–590. http://dx.doi.org/10.3934/dcds.2017023 doi: 10.3934/dcds.2017023
![]() |
[39] |
S. Zhou, Attractors for second-order lattice dynamical systems with damping, J. Math. Phys., 43 (2002), 452–465. http://dx.doi.org/10.1063/1.1418719 doi: 10.1063/1.1418719
![]() |
[40] |
S. Zhou, Random exponential attractor for cocycle and application to non-autonomous stochastic lattice systems with multiplicative white noise, J. Differ. Equations, 263 (2020), 2247–2279. http://dx.doi.org/10.1016/j.jde.2017.03.044 doi: 10.1016/j.jde.2017.03.044
![]() |