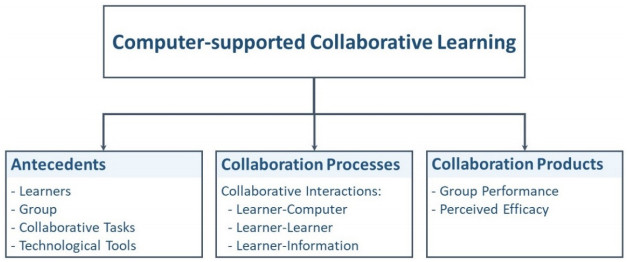
Main elements of CSCL
.We used the quantile vector autoregressive (QVAR) dynamic connectedness framework to examine whether leading stock markets in America and Europe would have any impact on major stock markets in Asia.1 More precisely, we analyzed systematically the stock market connectedness in 15 countries, namely Germany, the UK, the USA, and 12 Asian countries, which include five major ASEAN countries, namely Indonesia, Malaysia, Philippines, Singapore, and Thailand from 1996 to 2023. The findings indicated that Hong Kong and Singaporean stocks were major transmitters of financial shocks at the extreme low price market condition, while Germany and UK were minor transmitters. By contrast, the USA could be considered the major transmitter of financial shock during the extreme high market price returns condition. In the normal market condition, these three countries in Europe and America are important transmitters of financial shock. More interestingly, the empirical findings indicated the centrality of Singapore in the stock market connectedness in Asia.
1 The authors are grateful to Professor David Gabauer who makes available the R codes for all calculations in this paper.
Citation: OlaOluwa S. Yaya, Miao Zhang, Han Xi, Fumitaka Furuoka. How do leading stock markets in America and Europe connect to Asian stock markets? Quantile dynamic connectedness[J]. Quantitative Finance and Economics, 2024, 8(3): 502-531. doi: 10.3934/QFE.2024019
[1] | Soheila Garshasbi, Brian Yecies, Jun Shen . Microlearning and computer-supported collaborative learning: An agenda towards a comprehensive online learning system. STEM Education, 2021, 1(4): 225-255. doi: 10.3934/steme.2021016 |
[2] | Xin Zhang, Longzhu Yi, Huikuan Chen, Jiayu Qian, Xuesong Zhai . Collaborative science experiments based on the educational metaverse: Research on the impact and mechanisms of collaborative science experiments on elementary students' creative thinking. STEM Education, 2025, 5(2): 250-274. doi: 10.3934/steme.2025013 |
[3] | Suhua Wang, Zhiqiang Ma, Hongjie Ji, Tong Liu, Anqi Chen, Dawei Zhao . Personalized exercise recommendation method based on causal deep learning: Experiments and implications. STEM Education, 2022, 2(2): 157-172. doi: 10.3934/steme.2022011 |
[4] | Zhongyan Hu, Yun Fah Chang, Ming Kang Ho . A comparative study on the effectiveness of blended learning, physical learning, and online learning in functional skills training among higher vocational education. STEM Education, 2024, 4(3): 247-262. doi: 10.3934/steme.2024015 |
[5] | Xiangping Cui, Jiangming Qian, Soheila Garshasbi, Susan Zhang, Geng Sun, Juan Wang, Jun Shen, Lin Yue, Yewen Lyu . Enhancing learning effectiveness in livestream teaching: Investigating the impact of teaching, social, and cognitive presences through a community of inquiry lens. STEM Education, 2024, 4(2): 82-105. doi: 10.3934/steme.2024006 |
[6] | Sasha Nikolic, Sarah Grundy, Rezwanul Haque, Sulakshana Lal, Ghulam M. Hassan, Scott Daniel, Marina Belkina, Sarah Lyden, Thomas F. Suesse . A ranking comparison of the traditional, online and mixed laboratory mode learning objectives in engineering: Uncovering different priorities. STEM Education, 2023, 3(4): 331-349. doi: 10.3934/steme.2023020 |
[7] | Tasadduq Imam . Good practices of delivery and teaching leadership for online educators in technical disciplines: A perspective. STEM Education, 2021, 1(2): 92-103. doi: 10.3934/steme.2021007 |
[8] | Sasha Nikolic, Zach Quince, Anna Lidfors Lindqvist, Peter Neal, Sarah Grundy, May Lim, Faham Tahmasebinia, Shannon Rios, Josh Burridge, Kathy Petkoff, Ashfaque Ahmed Chowdhury, Wendy S.L. Lee, Rita Prestigiacomo, Hamish Fernando, Peter Lok, Mark Symes . Project-work Artificial Intelligence Integration Framework (PAIIF): Developing a CDIO-based framework for educational integration. STEM Education, 2025, 5(2): 310-332. doi: 10.3934/steme.2025016 |
[9] | William Guo . Design and implementation of multi-purpose quizzes to improve mathematics learning for transitional engineering students. STEM Education, 2022, 2(3): 245-261. doi: 10.3934/steme.2022015 |
[10] | Yujuan Li, Robert N. Hibbard, Peter L. A. Sercombe, Amanda L. Kelk, Cheng-Yuan Xu . Inspiring and engaging high school students with science and technology education in regional Australia. STEM Education, 2021, 1(2): 114-126. doi: 10.3934/steme.2021009 |
We used the quantile vector autoregressive (QVAR) dynamic connectedness framework to examine whether leading stock markets in America and Europe would have any impact on major stock markets in Asia.1 More precisely, we analyzed systematically the stock market connectedness in 15 countries, namely Germany, the UK, the USA, and 12 Asian countries, which include five major ASEAN countries, namely Indonesia, Malaysia, Philippines, Singapore, and Thailand from 1996 to 2023. The findings indicated that Hong Kong and Singaporean stocks were major transmitters of financial shocks at the extreme low price market condition, while Germany and UK were minor transmitters. By contrast, the USA could be considered the major transmitter of financial shock during the extreme high market price returns condition. In the normal market condition, these three countries in Europe and America are important transmitters of financial shock. More interestingly, the empirical findings indicated the centrality of Singapore in the stock market connectedness in Asia.
1 The authors are grateful to Professor David Gabauer who makes available the R codes for all calculations in this paper.
Following the development of knowledge dynamics and 'ubiquitous computing', new learning systems are being generated. Microlearning is flourishing as a constantly evolving learning trend. It is an effective means of 'learning on the go', especially in the corporate learning space that includes employee onboarding, compliance training, and skills training.
According to Hug [1], microlearning is a digitally focused and multi-modal approach that aims at content generation. It can take the form of short asynchronous learning materials – for instance, short videos, micro-podcasts, or digital texts. Microlearning has been acclaimed as empowering self-directed lifelong learning [2]. Moreover, it supports the development of autonomy in learners [3].
Despite these advantages, Neelen and Kirschner [4] expressed concerns about the operational definitions of microlearning. Also, Jomah, Masoud, Kishore and Aurelia [5] argued that microlearning is not useful when learners need to acquire complex skills, processes, or behaviors. Cutler [6] speculated that microlearning may lead to learning failures as learners may become 'overly dependent on this mode of instruction'. Hence, there is a need for further investigation of microlearning and its systematic theoretical and instructional underpinnings [7-9].
Furthermore, material developers, curriculum designers and instructors should proactively adjust teaching and learning methods to accommodate students' learning needs and preferences. Furthermore, different learning and teaching models should be adopted based on specific course/subject characteristics. Microlearning is a valuable learning model which addresses short attention spans and enhances learning retention; however, it's certainly not a panacea for all courses/subjects, all learners and different periods of the learning process.
Whereas microlearning is a personalized learning mode, which facilitates learning on the go, there is a corresponding requirement to build up interaction, collaboration and teamwork skills in online learning environments. Palloff and Pratt [10] considered collaboration to be the 'heart and soul' of online education. Computer-supported collaborative learning, as an offshoot of the socio-constructivist theory of learning, refers to interactive platforms and methodologies through which learners engage in completing online collaborative tasks in groups. Learners' intensive engagement in real-life collaborative tasks through group interactions results in effective learning outcomes including improved problem-solving skills, enhanced communication skills, and the formation of robust mental models of complex processes [11]. Computer-supported collaborative learning creates a sense of social presence through interactions among learners [12], which enhances learners' intrinsic motivation and engenders positive attitudes toward learning [10, 13, 14]. The integration of computer-supported collaborative learning platforms and technologies has been strongly advocated as an essential element of 21st-century skills [12, 14, 15].
To shed new and comprehensive light on microlearning (ML) and computer-supported collaborative learning (CSCL), first the authors address the granularity of ML frameworks, including design and content, theoretical and pedagogical considerations, and mobile environments. The second part is a synthesis of CSCL's nuances, and its main objectives, divergent theories, and core elements. Next, this study investigates the mobile applications for computer-supported collaborative learning (mCSCL), as well as some of the perceived obstacles to and challenges of effective CSCL. Finally, the recommendation contextualizes ML and CSCL in diverse learning systems in order to provide STEM leaners with well-equipped means to support their effective and varied online learning, which has become even more significant during and after the pandemic.
Theo Hug [1] defines microlearning as 'an abbreviated manner of expression for all sorts of short-time learning activities with micro-content'. For Hug, microlearning takes place through using social media, mobile technology, the internet and all digital learning tools.
Microlearning is best suited for relatively small and time-restricted learning 'chunks' and tasks. It contains bite-sized learning content, which is delivered when and where the learner requires. Although microlearning is short and simple, learning should not be dumbed down just because it is short-term or narrowly focused. In microlearning design, learning content is generated by breaking it to small units to facilitate learners' interaction with the material. To achieve this, curriculum developers need to consider learners' cognitive and learning capacities by chunking and segmenting learning materials [16, 17] in order to reduce cognitive load [18].
To describe, analyze or generate versions of microlearning, various parameters should be considered as important dimensions of the learning process: time, content, curriculum, form, process, mediality and learning type. Learning types can be categorized as repetitive, activist, reflective, pragmatist, conceptionalist, constructivist, connectivist, behaviorist, learning by example, task or exercise, goal or problem-oriented, action learning, classroom learning, and corporate learning [19].
The first and most important step in generating a microlearning system is considering learning content. Designing learning materials for microlearning, if implemented appropriately, can provide deeper encoding, critical/reflective thinking and practical retrieval mechanisms which lead to successful learning outcomes. Hence, learners' interaction with content in online learning environments is a significant factor in e-learning efficiency.
In addition, heutagogy (or self-determined learning) is a new notion which privileges learners to manage their learning process. Heutagogy prepares learners to foster learning skills and move toward a learner-centered learning system. It also enhances learners' motivation and self-determined goal creation. Blaschke [20] believes that along with learner-created micro-content delivery, heutagogy prepares learners to become skillful long-life learners.
Microlearning materials consist of micro-content and microlearning activities. Micro-content refers to nuggets of information on social media like blogs, Facebook, twitter, or YouTube. The size and length of the content depends on the constraints of the subject under study. Microlearning activities are brief learning experiences acquired through dealing with micro-content. Microlearning activities can be generated by learners or extracted through their learning process. However, microlearning content and microlearning activities require pedagogical cohesion in order to cover learning objectives. To achieve this, microlearning can take the form of e-microlearning, which encourages learners to be co-producers of learning content throughout communicative interactions [21]. Applying microlearning and crowdsourcing, Suhonjić et al. [22] investigated the role of learners in creating and sharing short videos for micro-courses on a collaborative learning platform. Learners' engagement in the creation of microlearning content has a significant impact on improving their intrinsic motivation and satisfaction levels. Hence, investigating the impact of learner-created microlearning materials on pedagogical and learning experiences is an active area of research.
Alqurashi [23] investigated the predictor variables in developing learning self-efficacy during microlearning: learner–content interaction, learner–instructor interaction, and learner–learner interaction. She reported that a learner's interaction with the content was the most influential predictor of learner satisfaction in an online learning environment. Also, self-efficacy is a determining predictor in perceived learning. Hence, improving learners' interaction with content and fostering their confidence should be considered key factors in online learning settings.
Microlearning can be regarded as a learner-centered environment which provides learners with opportunities to create course materials and activities from a wide range of options including micro-podcasts, YouTube, and Vines.
There are a number of micro-content delivery approaches on microlearning platforms. "Micro-lectures", short videos less than 15 minutes in length, are the most popular form of microlearning content [24]. Although learners can enjoy the benefits of microlearning on online platforms, instructors need to be actively engaged in guiding the content and put effort and time into micro-lecture development [25, 26]. Other kinds of microlearning materials are brief social media posts [27], short prompts or tests as supplementary instructional resources [28, 29], and learning materials chunked into interactive modules [25, 30, 31]. Moreover, personalized recommendation systems can be applied to tailor-make the microlearning pathways for learners based on their learning history or other contextualized factors like learners' feedback [26, 32, 33]. As a result of these measures, learners have rated positive learning experiences with personalized microlearning environments. Recent studies have explored the advantages of employing educational technologies like gaming, virtual reality, and data-driven and personalized microlearning systems. The impact of technology choice in various microlearning content delivery systems and different fields of study needs to be explored further.
Baumgartner [34] investigated a theoretical model of microlearning which consists of four stages: (1) behaviorism (learners absorb knowledge); (2) cognitivism (active learning with meaningful feedback); (3) constructivism (knowledge construction); and (4) advanced learning.
Göschlberger [35] suggested a social microlearning framework. Learners collect, create and share microlearning content through interaction with the materials and also through social/inter-personal communication.
Bouillion and Gomez [36] examined learning in the context of daily routine as an important factor in sociocultural learning, as proposed by Vygotsky [37] and the constructivist school. Hence, microlearning can provide learning opportunities within the social context of everyday life.
Considering a constructivist view of knowledge, in combination with the significance of language, culture and interpersonal communication in developing higher psychological processes [38], and the notions of collaboration and collective intelligence [39], the development of a ubiquitous online learning environment – i.e., 'the micro-worlds' as suggested by Papert [40] – is required. Micro-worlds are 'experimental learning environments in which learners can navigate, manipulate, or create objects and test their effects on one another' [41].
Furthermore, Khong and Kabilan [42] proposed a theoretical model for microlearning based on three underlying theories: Sweller's Cognitive Load Theory (CLT) [43], Mayer's Cognitive Theory of Multimedia Learning (CTML) [44], and Ryan's Self-Determination Theory (SDT) of motivation [45]. The design and development of micro-content and micro-tasks can be supported by Cognitive Load Theory principles. Meanwhile, the application of microlearning within the digital micro-media and mobile platforms is consistent with Cognitive Theory of Multimedia Learning. In addition, microlearning and the construction of micro-knowledge can be substantially supported by Self-Determination Theory, given the significance of motivational elements in microlearning as a personalized, autonomous learning system.
The pedagogical orientation of microlearning depends on the learning models and media types applied, inclusive of a wide range of pedagogies: reflective, pragmatist, conceptionalist, constructivist, connectivist, behaviourist learning, and goal/problem-oriented learning [46].
According to social learning theory, learning is the outcome of personal–environmental interactions [47]. Social learning is burgeoning today with the widespread use of the internet, social media and smart devices, which are resulting in innovative and intelligent learning systems including mobile learning and microlearning.
With the widespread use of mobile devices, learners have ready access to course content and learning-on-the-go. Hug [19] investigated the application of mobile devices in microlearning platforms with specific features in mind: (1) micro-content, (2) short attention spans, (3) mobile's small screen size, (4) micro-steps for formal and informal learning environments, (5) mobile, physical and social learning environments, and (6) access to micro-platforms.
Microlearning is associated with mobile learning as a result of being situated, authentic, spontaneous and personalized [48]. These factors have inspired researchers to investigate the application of microlearning in mobile Massive Online Open Courses [49, 50].
Cai and Chen [51] proposed a novel approach, applying mobile augmented reality to deliver microlearning content. Also, Trusty and Truong [52] have integrated web browser content to deliver passive microlearning.
Furthermore, Wen et al. [33] and Göschlberger [53] discussed the implementation and evaluation of a smartphone application for ubiquitous micro-lecture delivery. In addition, Cai and Chen [51] referred to improved self-reported motivation and learning performance in a microlearning system via mobile augmented reality.
Collaborative learning involves groups of learners working together to solve a problem, complete a task or create a product [54]. The ultimate goal of collaborative learning is the co-construction of shared knowledge among group members [55, 56].
Computer-supported collaborative learning enhances collaborative learning via information and communication technologies (ICTs), applying tools and technologies that speed up group learning, knowledge sharing and co-construction [57, 58]. CSCL can be developed and applied in face-to-face, online or distance education (synchronous or asynchronous), or on blended learning platforms [59].
According to Harasim [14], online collaborative processes encourage knowledge development and conceptual change. Collaborative learning enhances deeper learning, critical/reflective thinking, shared understanding, and long-term retention of learning materials [60-62]. Thus, collaborative learning fosters learners' communication skills and competence through interactions designed to complete assigned collaborative tasks.
Incorporating computer-supported collaborative learning and team working activities in e-learning frameworks provides further opportunities for learners' engagement, development of communication skills, and results in active learning. CSCL enables learners to foster active learning strategies and enhance their motivation and persistence; moreover, it develops critical thinking skills [63]. Active learning empowers learners through engaging them in dynamic experiences, shared effort with peers and taking responsibility for their learning within collaborative learning environments. Active learning fosters critical thinking skills and develops reflective learning behaviors which turn surface learning into deep learning. In active learning, the responsibility is transferred to learners. Accordingly, material developers are seeking to develop learner-led activities to boost social interaction among learners [64].
CSCL can be effective for vulnerable and passive learners as they can communicate more comfortably in online platforms compared with face-to-face settings. In CSCL platforms, learners can express their thoughts with lower levels of anxiety [65]. Learners boost their self-confidence, autonomy, and motivation through interaction via written language which can lead to durable communication [66], and also through active engagement in cognitively challenging online tasks [67].
The main objective of computer-supported collaborative learning, with a social constructivist orientation, is to switch intentionally from a content-centered and teacher-driven framework to a process-centered, learner-driven design. Moreover, group or social cognition is a robust principle in collaborative learning [58]. Here, the main purpose is to nurture collaborative knowledge construction through reflection on perceptual experiences [68, 69]. Hence, the producer of knowledge is the learner, who seeks to work collaboratively and analytically to suggest explanations for learning experiences for further discussion and potential refutation. Creating a 'collective intelligence' [39] on computer-supported collaborative learning platforms is considered essential to foster inductive-deductive reasoning. Consequently, the role of instructor becomes that of a facilitator with respect to the specific social-constructivist viewpoint chosen [70].
CSCL and its impacts on learners' characteristics and learning outcomes have been widely investigated, both in empirical studies and meta-analyses. Chen et al. [71] referred to three main factors in CSCL in a meta-analysis: (i) collaborative learning as learning in groups, small groups, Jigsaw, dyadic learning; (ii) using computer and applications like Moodle, Google Apps or Facebook, virtual reality or computer games, multi-touch tablets; and (iii) additional learning tools, or strategies which provide a technology-mediated learning environment to trigger and guide learners' active engagement in collaborative activities [66, 72-80]. Learning tools and strategies which have been employed in CSCL include (1) adaptive or intelligent systems like the recommender system; (2) virtual environments such as digital games, simulation, augmented reality, and virtual reality; and (3) guidance from teachers through providing cognitive and affective strategies [81, 82].
Constructivists believe that knowledge cannot be achieved externally; rather, learners construct knowledge internally through developmental stages in their social interactions with learning content, their instructors and peers [37]. Learning is a meaningful personal experience achieved within social interactions. Instructional designers attempt to create communication opportunities to generate collective knowledge-building and refining within a learner-centered system [83]. It is perfectly in line with constructivist theory to say that communication and collaboration can reinforce learning skills.
Moreover, social constructivists consider human beings as social creatures who develop through social skills and interactions with community members. They believe that learning occurs throughout social interactions and that learners acquire knowledge through dialogue and by completing collaborative tasks during team-working activities [84, 85].
In addition, connectivism, a social learning theory including technology (mainly the internet) [86], holds that knowledge is achieved through social networks and connecting nodes. Connectivism values the role of communication and meaningful knowledge construction in applying interaction skills throughout the learning process.
Collaborative learning is supported by social learning theories such as situated learning [87], distributed cognition [88], and learning communities [89] in online platforms.
Furthermore, Collaborative Cognitive Load Theory [90] postulates how cognitive load theory [91] can be employed to provide instructional guidelines that will enhance effective CSCL platforms. Cognitive load theory can thus be extended to review and incorporate collaboration and collaborative features, as well as the concepts of collective working memory, mutual cognitive interdependence, and transactive tasks [91]. According to Asterhan and Schwartz [92], positive interdependence is an important element in effective CSCL. Positive interdependence is required to achieve learning goals and fulfill task requirements in collaborative learning for which learners require the active participation of all group members. To achieve this, not all members need to gain knowledge of the whole in order to complete the task [93]. In other words, several working memories collaborate to perform group tasks, reducing the demands made on an individual's working memory [94]. Moreover, individuals are responsible for assigned subtasks, which call for less effort; however, this approach demands deep processing of learning material. Hence, group members can rely on each other's knowledge and expertise to complete the collaborative task. Group members gain knowledge by communicating with their partners and exchanging knowledge during transactive interactions, thus improving collaboration outcomes and learning quality.
Extensive empirical research has been carried out on multiple aspects of CSCL including individual perceptions, individual knowledge gain, skill acquisition, social interaction and group task performance. Computer-supported collaborative learning involves two main elements, collaboration through the learning process and the technological support of computers in collaborative learning. Accordingly, some studies have explored the process of collaboration on CSCL platforms, while others have focused on the role of computers and technological tools in productive interactions and efficient collaborative learning [71].
According to Janssen et al. [94], CSCL can be studied broadly in terms of antecedents, processes, and the consequences of the collaboration process. The antecedents include learner, group, task, and technological characteristics, each with determining impacts on CSCL platforms. Collaboration processes include all interactions between team members during collaborative activities. The consequences of CSCL refer to collaboration products – for instance, group performance or perceived efficacy. To investigate CSCL effectively, all these elements – antecedents, processes and consequences – must be simultaneously taken into account (Figure 1).
In terms of collaboration processes and products, all mentioned fundamentals must be addressed in the design and implementation of an effective computer-supported collaborative learning framework to offer the required functions and tools, and also to set limitations so as to minimize direct information delivery.
A computer-supported collaborative learning environment requires an interface design which examines the themes of functionalities and the issues of learner-computer and learner-learner interactions. These interactions, the corresponding issues and required tools should all be considered in designing a computer-supported collaborative learning model. According to Desjardins et al. [95], four types of interactions should be addressed in a computer-supported collaborative learning platform:
1. Learner-computer interaction
2. Learner-learner interaction
3. Interacting with information
4. Using information processing tools
According to Zhao, Sullivan and Mellenius [96], three main factors have a direct impact on online collaboration processes and collaboration products: participation, interaction, and social presence. Social presence develops through interaction; moreover, optimal social presence and group performance boost the quality of participation and interaction, which in turn results in effective collaboration processes and collaboration consequences.
One alternative is the social presence model suggested by Whiteside [97], which includes five factors that foster social presence by motivating learners to be active main players in their own and their peers' learning process. The social presence model, with these five integrated elements, acts as a heuristic for instructors and learners. Furthermore, the social presence model is a valuable means for researchers. The integrated elements of the social presence model proposed by Whiteside [97] can be described as follows:
1. Affective association refers to emotional connections among participants, including personal emotion, humor, and self-disclosure.
2. Community cohesion refers to the formation of a cohesive community and individual sharing of additional resources with the group. Individuals are considered approachable group members in cohesive communities.
3. Instructor Engagement: Instructors facilitate social connections, provide community-building activities, encourage constructive engagements among learners, and foster learners' critical analyses and higher-order thinking skills on online platforms.
4. Interaction Density: This element describes the level of interaction among learners. Interaction density includes acknowledgement of peers' input, agreement, disagreement, compliments, and questions.
5. Knowledge and Experience: The team's collective knowledge and experience are significant factors for establishing social presence; moreover, these elements can enhance discussion and collaboration.
It is important to note that a comprehensive study of collaboration antecedents and processes can lead to effective collaboration consequences, thereby increasing the efficacy of the STEM educators' roadmap choices.
The next section explores the antecedents in CSCL, which consist of the learners, groups, collaboration tasks and collaboration tools. Figure 2 below shows these elements in greater detail.
Turning to learner characteristics, the impact of the learner's prior knowledge, self-regulation skills and collaboration skills have been studied in CSCL. Learners with low prior knowledge can benefit from further exposure to information by other group members [98]. According to Retnowati et al. [99], collaborative learning is recommended for learners with incomplete information.
Personal characteristics – for instance, self-efficacy, motivation, attitudes, goals and epistemic beliefs about learning – have significant impacts on self-regulated learning [100]. As collaborative learning tasks are based on socio-cognitive principles, learners' social attributes, such as suitability for leadership roles, should be considered as well. According to Xie et al. [101], leadership roles have distinctive effects on learners' attitudes and motivation, as well as their learning engagement [102]. As a multifaceted attribute, learning engagement encompasses behavioral, affective, and cognitive aspects [103], as well as social features on collaborative learning platforms [104].
Collaborative learning can be examined in the light of general learning objectives. As suggested by Pellegrino and Hilton [105], learning objectives can be classified basically as (1) intrapersonal goals (cognitive, affective, and motivational) and (2) interpersonal/social goals (social interactions and group discourse).
Individual skill acquisition and knowledge gain include higher-order thinking skills, critical/reflective thinking skills, problem-solving skills, and group-learning skills. Cognitive goals are linked with individual cognitive processes and skills, as well as problem-solving skills which can be measured through objective tests. Furthermore, affective/motivational goals are related to learners' attitudes, motivation, self-efficacy, and anxiety levels, which are subjectively measurable through surveys or questionnaires.
Sociocultural and cognitive attributes of the individual and the group must also be taken into account. Several studies have focused on discourse analysis and argumentation quality through content analysis of verbal and textual attributes of collaboration, which can elucidate learners' cognitive load throughout the collaboration process. Khawaja et al. [106] and Yin et al. [107] studied the collaboration process through discourse analysis and reported the significant impact of grammatical and linguistic characteristics on learners' cognitive load.
Regarding the measurement of cognitive load in CSCL, the 9-point rating scale developed by Paas [108] has been widely used. However, Larmuseau et al. [109], Pijeira-Diáz et al. [110], and Paas and Van Merriënboer [111] proposed measuring physiological variables including electrical activity in the brain, skin temperature and heart-rate variability at certain collaboration intervals, along with traditional cognitive load measures, to elucidate collaboration features and processes.
Additionally, individual attributes are linked with learners' perceptions including the perception and evaluation of the overall learning system, attitudes toward a specific discipline, perceived capability (academic self-efficacy or self-concept) and performance in specific skills, perceived individual learning gains and group learning outcomes – i.e., social presence and cooperativeness.
On the other hand, interpersonal goals include group interactions, collaborative tasks, and peer communication to achieve shared knowledge through collaborative activities. There are a number of validated analysis frameworks which measure group discourse and social interaction, such as the framework proposed by Baker et al. [112]. According to Weinberger and Fischer [113], through measuring group discourse, reliable indicators are achieved which represent the learning process in CSCL platforms.
Furthermore, group-level skills can be considered in two main categories: group task performance and social interaction. Group task performance can be evaluated through group reports, essays, assignments or completed group activities. Social interactions includes task-related argumentation, knowledge construction, metacognitive activities, social activities (like greetings) and off-task communication (like technical and nonsense discourse). Social interactions can be measured quantitatively or through discourse content analysis [71].
Hence, investigation of the impacts of CSCL on attributes such as personal skill acquisition, knowledge achievement, social communication skills, and team-working interaction are active areas of research in CSCL. Intelligent algorithms and tools developed by innovative e-learning technologies have resulted in a revolutionary trend in educational technology, fostering learners' communication on collaborative platforms, promoting guided individualized learning models, and transforming the role of instructors to become facilitators [114].
In online collaborative platforms, learners face many emotional challenges generated by factors ranging from individual differences to dysfunctional interaction processes, all of which impede learners' engagement in collaborative tasks [115]. Applying emotion regulation strategies, both individually and together, team members can achieve and maintain effective and enjoyable collaboration during their group working processes [116]. The concept of emotion regulation relates to human agency and adaptiveness in social situations [117], elements which are essential for effective collaboration and group coordination. To face the emotional challenges of collaborative learning, team members employ different emotion regulation strategies including self-regulation, co-regulation, and socially shared regulation [118].
According to Järvenoja et al. [116], learners typically use four emotion regulation strategies in collaborative activities: encouragement, increasing awareness, social reinforcement, and task structuring. Zhang et al. [119] reported use of socially shared regulation, such as joint planning, monitoring, and evaluating processes in collaboration. While emotion regulation does not have a direct impact on group members' emotions, it is an indicator of their ability to regulate the cognitive and social elements of interactions [120].
Learners' self-regulation strategies, on the other hand, feature regulatory behaviors of the learners and are considered to be critical skills for efficient collaboration in CSCL environments [121, 122]. Cho et al. [123] concluded that highly self-regulated learners revealed a stronger sense of community of inquiry and achieved higher affective outcomes than low-regulated learners.
Also, Kilis et al. [124] emphasized the contribution of self-regulation, metacognition, and motivation to the effective development of community of inquiry and its three presence types – social presence, cognitive presence and teaching presence.
There are three types of self-regulation strategies for learning engagement in computer-supported collaborative learning: cognitive strategies, resource regulation strategies and collaborative learning strategies.
Firstly, cognitive strategies can be considered indictors of learners' cognitive engagement and their deep processing of learning material throughout learning efforts [125]. According to Muis [126], there are two main cognitive strategies: elaboration strategies and critical/reflective thinking strategies. Elaboration strategies are employed in identifying main points, paraphrasing, summarizing, and creating examples, while critical/reflective thinking strategies are used when applying relevant knowledge in a new context or critical evaluation of learning material. The application of cognitive strategies benefits learners in various ways. According to Credé and Phillips [127], there is a strong relationship between critical/reflective thinking strategies and learners' motivation. Moreover, Su et al. [128] reported a positive relationship between scales of online self-regulated language learning strategies – i.e., goal setting, self-evaluation, and learners' attitudes toward collaborative learning.
Secondly, resource regulation strategies are learners' self-regulation of time and environmental constraints and their efforts throughout the learning process to achieve learning goals.
Thirdly, collaboration strategies are significant, especially in group-learning settings. Collaborative strategies are of two main types: peer learning and help-seeking. Peer learning strategies foster learning objectives as learners seek to clarify course materials for each other and to complete collaborative tasks [129]. Help-seeking strategies refer to seeking assistance or explanation from other group members, indicating learners' awareness of their knowledge gaps. Help-seeking strategies are considered to be a critical factor in achieving ultimate learning goals. According to Cao [130], learners who practice such strategies are actively engaged in the learning process and tend not to procrastinate. This is critical for STEM educators to understand learners' metacognitive regulation and co-regulation, as well as their socially shared regulation strategies, which shape approaches to both the current and next phases of L & T development in the world.
Previous studies offer valuable insights on learners' metacognitive regulation of their learning process, including self-evaluating, content monitoring and social emotional regulation. All three of these processes are incentives for efficient collaborative learning. There are many challenges in learners' strategic regulation of cognition, emotion, motivation and learning behaviors in computer-mediated collaborative learning [131]. Self-regulated learning has been investigated, both at an individual level and at the interpersonal/group level, as a social process designed to monitor and regulate cognitive/metacognitive demands throughout group learning processes [132]. As the interconnection between self-regulation and social regulation is multifaceted, focused research is required to support material designers and curriculum developers in CSCL [133]. According to Janssen et al. [134], group performance is directly affected by social regulatory activities including the planning, monitoring and evaluation of the collaboration process.
Considering that self-regulation is a task-specific and dynamic process, researchers advocate investigating the temporal order of learners' regulatory behaviors during group learning processes [135]. Thus, applying sequential pattern-mining approaches is required to shed more light on regulation types and processes which will result in efficient collaboration outcomes [133].
Furthermore, in CSCL settings, teamwork is also the target of regulation. In group learning, both individual learning and group learning processes have to be regulated. Group members help each other to regulate their learning toward a group objective, or to regulate group learning jointly at the group level.
Su et al. [136] studied learners' group dynamics from different regulation perspectives and reported the development of both active self and social regulation skills as an outcome of online collaborative language learning. Furthermore, socio-emotional regulation plays an important role in the quality of team-working [137, 138]. According to Ucan et al. [139], focusing on learners' shared responsibilities and informing them about collaboration goals and the regulation of group tasks can foster co-regulation and socio-emotional regulation in the context of CSCL. Thus, Järvelä et al. [121] emphasized providing greater guidance for group learning and co-regulation through the use of intelligent tools in CSCL settings. Hence, such tools are advantageous to establish clear online collaborative learning and teaching pathways – for both individuals and groups.
Group characteristics have significant impacts on a group's distribution advantage and the minimization of transaction costs – i.e., the extra load placed on individuals' working memory capacity throughout the collaboration process. Moreover, group size is an important factor in setting up a collective working memory effect. In larger groups, team members can share more collective information. Task characteristics can control the impact of group size in CSCL processes and consequences considering the role of Cognitive Collaborative Learning Theory, an active research trend in CSCL [140].
Group composition and optimal group formation are of paramount importance in both collaboration processes and collaboration consequences. Learners' prior knowledge, age, and gender are among the factors which have been considered by investigations into group composition [141-144]. However, further research is required that considers multiple characteristics and learning behaviors throughout the collaboration process, such as learners' attitudes, motivation levels, self-regulation skills, and cognitive and metacognitive attributes.
Considering the significant role of learners in collaborative learning environments as participants in knowledge-building communities [145], multiple aspects of individual learners are required to be taken into account in optimal grouping processes.
Thanks to the science of Information Technology, and the application of intelligent models and algorithms, we can design and manage optimal group composition more effectively after considering learners' multiple characteristics and relevant multimodal data. The ultimate goal is to organize groups into different sizes, managing intelligent group formation and continuous group coordination with optimal composition based on the group's performance during the collaboration process. Garshasbi et al. [146] proposed a well-structured and novel algorithm, a multi-objective version of Genetic Algorithms – i.e., Non-dominated Sorting Genetic Algorithm, NSGA-II – to group learners optimally into inter-homogeneous and intra-heterogeneous teams in a computationally cost-effective manner. The algorithm can consider learners' multiple characteristics in achieving optimal group formation. The multi-objective algorithm is a proper and reliable optimization method for grouping any number of learners with various characteristics of any data type and range of variation into optimal inter-homogeneous and intra-heterogeneous learning groups. Given the increasing demand for multi-disciplinary research in CSCL to investigate group dynamics and group learning processes from the educational and technological viewpoints, intelligent computational approaches and technological tools can be of significant benefit. Moreover, enriching learners' profiles through collecting multimedia data – textual, audio-visual or sensory – is of great benefit in developing optimal group formation algorithms and tools.
Group formation criteria play a significant role in determining learners' individual and social regulatory behaviors, including cognitive and metacognitive skills, during the collaboration process. Hence, group size and group formation criteria are required to be considered in studying the dynamics of self-regulation in CSCL. Also, learners' self-regulatory skills are determining factors in social regulation processes as they apply to online collaborative activities. Accordingly, instructors need to consider learners' online self-regulation profiles as an important criterion in optimal cohort formation and for improving social regulatory behaviors [147].
Moreover, group member familiarity is an effective element which can enhance the collective working memory effect, although this may come at the sacrifice of inefficient transaction costs [148]. Hence, the impact of group member familiarity needs to be investigated in collaboration processes and consequences, especially as educators plan specific activities around group dynamics. This is easier said than done.
Regarding task features, task complexity and task interdependence have been investigated mostly in the relevant literature on CSCL. Appropriate tasks with defined levels of complexity are essential for effective collaborative processes and outcomes. Investigating task complexity from a Collaborative Cognitive Load Theory viewpoint is a dynamic research area. Task interdependence consists of two kinds: outcome interdependence and means interdependence [149]. Outcome interdependence refers to team members' overall attempt to complete a collaborative task in order to gain group rewards, while means interdependence refers to the heterogeneous distribution of information among group members, which requires more collaborative activities to achieve effective learning outcomes [150].
There are several positive studies of the effective role of technological tools in CSCL – scaffolds, awareness tools and collaboration scripts – which speed up the process of collaboration and problem solving. According to Lin et al. [151], social awareness tools have positive impacts on learners' collaboration results. Bause et al. [152] stated that the scaffolds they applied in their group tasks supported team members in their collaborative tasks and positively impacted the cognitive load. Further investigation of group awareness tools from a Cognitive Collaborative Learning Theory perspective will be a step forward in reaching positive outcomes for supporting strategies in CSCL.
Computer-mediated communication (CMC) was an attempt to design and implement discussion tools, such as videoconferencing, to enhance group interactions [153]. Also, visual representation tools, such as concept maps, were applied to express complex ideas [51, 148]. Group awareness tools have been used to monitor and manage collaborative tasks [94].
Furthermore, the role of supporting strategies in CSCL has been under study; they include peer feedback [154], collaboration scripts [155, 156], and role assignment [157]. Other studies focus on annotation tools [158] and multi-touch interactive tabletops [159].
All these elements – collaboration tasks, computer supports and extra-learning tools or strategies – need to be employed in CSCL environments to support different aspects of computer-supported collaborative learning [71]. Moreover, more than one technology tool is commonly employed in CSCL to overcome existing challenges. Hence, learning objectives, learners' needs, and the nature of learning activities should all be considered when designing an appropriate CSCL environment with effective tools and strategies.
Finally, considering the characteristics of learning courses in selecting appropriate technological tools and strategies in CSCL is of paramount importance. For instance, in science courses, virtual reality or digital games can be well suited to assist in situated learning settings [71].
In the past decade, intelligent computing algorithms and tools have been designed and implemented to support CSCL to achieve interactive learning purposes. Intelligent mobile software applications have also promoted effective learning performance, learners' active engagement, and interaction in CSCL [160]. Reviewing the literature on the impact of mobile-CSCL, there have been improvements in learners' problem-solving skills, critical thinking strategies and overall learning performance in different disciplines, such as language learning [161-163], nursing [164], math [165], computer programming [166], and natural science [167]. Lai et al. [164] and Liu et al. [167] reported learners' active participation and high motivation on mobile-CSCL platforms. Liu et al. [166] stated that learners were eager to take part in team discussions and were more responsive to activity notifications via mobile phones. Viberg and Kukulska-Hulme [163] concluded that mobile-CSCL assisted language learners to develop collaborative and self-regulation skills. The mobile arena opens additional opportunities for facilitating collaborative tasks, tools, processes, and products.
The main effective interventions of mobile-CSCL can be summarized as presenting assigned learning tasks to help as the focal point of interaction; facilitating the interaction process through various mobile apps; providing feedback for group learning, which can assist with learners' evaluation; decision making; and regulating communication processes [168, 169]. Clearly, this domain of STEM offers some of the most exciting and challenging opportunities for interdisciplinary collaboration, and it is a topic needing further research elsewhere.
Suffice it to say that there are some fundamental challenges to CSCL that offer educators provocative insights on ideal platform attributes for sustaining effective learning environments.
The main objective of CSCL is boosting academic and social outcomes through collaborative interactions [170]. However, several challenges remain in developing an optimal online collaborative platform.
Lee et al. [171] reported four major obstacles to the effectiveness of collaborative learning: learners' lack of collaborative skills, free-riding, competence status, and friendship. Learners' lack of collaboration skills hinders group interaction and affects the process and consequences of collaborative learning [172]. This can lead to free-riding, a phenomenon which has been frequently investigated in the collaborative learning research literature [173, 174]. Also, group members' lack of social competence impedes collaborative learning, as less competent members cannot actively engage in collaborative tasks. Thus, learners' academic and social competency is a determining factor in collaborative task completion [175]. Considering friendship, group members often cannot concentrate on group tasks as they tend to socialize more in such situations [176]. Furthermore, teachers' lack of competencies and/or ignorance in training learners to develop collaborative skills and to assess the outcomes of collaboration processes, negatively impacts the efficiency of collaborative learning [177, 178]. Hence, it is highly recommended that teachers be equipped with the requisite knowledge and techniques, allowing them to set clear cognitive and collaborative objectives; furthermore, efficient teachers can help learners to develop collaboration skills and to gain efficient outcomes throughout the collaboration process.
In addition, Lee et al. [171] referred to further challenges in collaborative learning such as knowledge hoarding/hiding among group members, personal conflicts within the group, and members' unwillingness to develop an understanding of the content and/or the tasks required. Moreover, unequal participation is one of the most common complaints in team activities. Accordingly, Chang et al. [179] proposed applying strategies, including group contracts, role assignments, anonymous peer evaluations, and peer ratings to motivate learners' participation.
One of the main difficulties in the design and implementation of an effective CSCL is group formation criteria. There has been ample research investigating different aspects of group formation. According to Bert [180], between- and within-group differences in task engagement can affect team learning processes – an important factor to consider in designing CSCL platforms, especially where group formation processes are entailed.
Furthermore, material developers and instructors face many challenges in the design and organization of appropriate group tasks that would encourage well-structured group interactions to foster productive collaboration [181]. Nonetheless, monitoring and assessment of groups' progress is a significant concern in CSCL. Also, adequate and proper intervention on the part of the instructor strongly impacts the quality of group discussion and collaborative activities. So, if instructors fail to intervene effectively over the groups' demands or to model proper collaborative behaviors, the collaborative process will be negatively affected [182-184]. According to Chiriac and Granström [185], the criteria and framework for assessment in CSCL lacks transparency and concreteness. Strom et al. [186] concluded that a lack of assessment tools to measure learners' performance in CSCL has led to concerns about the fairness of the assessment process and also loss of motivation. Thus, the design and implementation of proper assessment tools and criteria in CSCL is of paramount significance.
One of the challenges of collaborative learning environments is overcoming participants' lack of communicative and collaborative skills. Thus, focusing on learners' communicative and group-learning skills is at the core of CSCL. Collaborative learning strategies involve team-working behaviors as intrinsic attributes of group learning – i.e., peer learning and help-seeking. Peer learning entails explaining materials for team members and collaborating with them in learning tasks, measures which result in achieving learning objectives uniformly through interaction and sharing views on course content [129]. On the other hand, help-seeking involves seeking peers' assistance and clarification, an indicator of learners' awareness of their lack of comprehension. Thus, there are several unresolved challenges in CSCL: low awareness of social presence and peer contribution, interacting complex ideas, an inadequate sense of collective responsibility, inadequate guidance, and a lack of feedback.
Biasutti et al. [187, 188] studied metacognition in group processes – i.e., planning, monitoring and evaluating in CSCL environments. Concepts such as team/shared cognition, group awareness and transactive memory have been applied to investigate team knowledge and collective mental constructs. Investigation of collective knowledge management processes – how teams create, manage and control knowledge-building processes – is a challenging research area. Zion et al. [189] reported that the study of metacognition at the group level, and the role of socially regulated behaviors in collaborative tasks, have been largely neglected. Also, according to Janssen et al. [134], further investigation is required on the metacognition of group dynamics and group awareness. Hence, nurturing a productive group work dynamic is the ultimate goal of CSCL.
Finally, it is worth noting that interdisciplinary research, applying advanced technologies and integrated AI-assisted intelligent algorithms and tools, can make significant contributions to overcoming the abovementioned barriers on CSCL platforms.
Taken together, the bulk of previous relevant literature informs us that a key aspect of microlearning is that it can be applied in flexible ways, depending on the learning purposes envisaged. For instance, it fits on-demand types of learning needs, supports lifelong learning, and can connect with diverse learning theories and approaches. Microlearning can be implemented in all learning systems with a variety of learning goals. It can also be employed in flexible ways in order to meet different learners' requirements. Hence, it can be linked with multiple learning theories and approaches.
Moreover, microlearning can be applied on diverse learning platforms, such as face-to-face, 'flipped' classrooms, online and/or blended learning settings. Accordingly, Semingson [190] emphasized that microlearning can be effective in different learning settings. Yet, microlearning also can be employed as an independent learning system for corporate learning. It offers learning flexibility and employee autonomy, thereby stimulating learners' motivation and a practice of lifelong learning. It can also support and augment long e-learning courses and even face-to-face learning. Learners can have the opportunity to grasp course content through microlearning modules at their preferred time and location.
Microlearning can absolutely be a proper complement that bridges the gaps for learners with knowledge deficiencies in certain aspects of the curriculum. It can help them to compensate for shortcomings, either independently or under the supervision of an instructor who can direct them purposefully toward the course objectives without hindering the learning flow in the classroom.
Moreover, microlearning modules with single topics can be integrated to build up a robust microlearning library, which can be embedded within a comprehensive learning platform. This forms an effective embedded module which can play a significant role in solidifying course content according to learners' most convenient times and locations.
Employing microlearning as a module integrated in an online learning framework will provide an opportunity for course/material designers to track learners' feedback and progress. It can serve as a valuable and effective means to modify and manage instructional strategies, materials and tools to meet the diverse needs of learners. While it is not suited to complex concepts and in-depth training, microlearning can enhance learners' engagement, independence and knowledge retention. Microlearning and micro-content are well suited to remedial and improvement settings.
Microlearning is extremely adaptable as an effective element of blended learning and as a complement to face-to face settings to enrich the learning goals in the personal learning process [191]. Given that microlearning is learner-centric, it suits learning plans from on-campus learning or even standard e-learning and m-learning settings.
Considering the fact that the focal point of microlearning is personalized learning, it can be integrated within a comprehensive learning framework, whether online, face-to-face or blended. On the other hand, CSCL improves communication and group working skills in learners which are basically among the fundamental values of learning systems.
However, CSCL is not a panacea for all learning processes [71]. It is absolutely an effective remedy for specific learning contents/tasks and certain periods of learning. Moreover, the characteristics of learning subjects and instructional goals are required to be taken into account. To design and implement an effective computer-supported collaborative learning module, technology and material developers, along with professional instructors, are required to investigate aspects of the learning process and diverse needs of learners. Hence, CSCL can also be considered as a complementary module within a comprehensive learning platform, to be utilized over a specified period of the learning process to meet learners' specific needs.
With this in mind, the benefits of computer-supported collaborative learning are investigated from different theoretical and empirical perspectives including the large-scale sharing of information in groups, coordination, navigation and co-construction of knowledge. To build collective intelligence in computer-supported collaborative learning platforms, social connectivity through participation and socially distributed inquiry processes are essential.
Furthermore, microlearning empowers learners through a reduced cognitive overload, improved self-management and motivation, heightened engagement, and reduced development time and costs. In sum, microlearning and CSCL are not one-size-fits-all learning approaches, and should be tailored to suit the individual requirements of each unique learner. Neither microlearning nor CSCL covers instructional designs and materials for all curricula across diverse fields of study. However, they both can be complementary modules in a comprehensive online learning platform.
Hence, a synergistic interplay of microlearning and CSCL is recommended to cover a variety of learning contents that suit learners' requirements in an online learning environment. Accordingly, we propose a combination of microlearning and collaborative learning systems in order to reach multiple learning objectives within an adaptive multimodal online learning environment.
[1] |
Agénor PR, Pereira da Silva LA (2022) Financial spillovers, spillbacks, and the scope for international macroprudential policy coordination. Int Econ Econ Policy 19: 79–127. https://doi.org/10.1007/s10368-021-00522-5 doi: 10.1007/s10368-021-00522-5
![]() |
[2] | Aggarwal R, Rivoli P (1989) The relationship between the US and four Asian stock markets. ASEAN Econ Bull 6: 110–117. |
[3] |
Ando T, Greenwood-Nimmo M, Shin Y (2022) Quantile Connectedness: Modeling Tail Behavior in the Topology of Financial Networks. Manag Sci 68: 2401–2431. https://doi.org/10.1287/mnsc.2021.3984 doi: 10.1287/mnsc.2021.3984
![]() |
[4] |
Anscombe FJ, Glynn WJ (1983) Distribution of the kurtosis statistic b2 for normal samples. Biometrika 70: 227–234. https://doi.org/10.1093/biomet/70.1.227 doi: 10.1093/biomet/70.1.227
![]() |
[5] |
Antonakakis N, Chatziantoniou I, Gabauer D (2020) Refined Measures of Dynamic Connectedness based on Time-Varying Parameter Vector Autoregressions. J Risk Financ Manage 13: 84. https://doi.org/10.3390/jrfm13040084 doi: 10.3390/jrfm13040084
![]() |
[6] |
Baig AS, Butt HA, Haroon O, et al. (2021) Deaths, panic, lockdowns and US equity markets: The case of COVID-19 pandemic. Financ Res Lett 38: 101701. https://doi.org/10.1016/j.frl.2020.101701 doi: 10.1016/j.frl.2020.101701
![]() |
[7] |
Balcilar M, Gabauer D, Umar Z (2021) Crude Oil futures contracts and commodity markets: New evidence from a TVP-VAR extended joint connectedness approach. Resour Policy 73: 102219. https://doi.org/10.1016/j.resourpol.2021.102219 doi: 10.1016/j.resourpol.2021.102219
![]() |
[8] | CEIC (2024) Available from: https://www.ceicdata.com/en (accessed on 7 August 2024) |
[9] |
Cepoi CO (2020) Asymmetric dependence between stock market returns and news during COVID-19 financial turmoil. Financ Res Lett 36: 101658. https://doi.org/10.1016/j.frl.2020.101658 doi: 10.1016/j.frl.2020.101658
![]() |
[10] |
Chatziantoniou I, Gabauer D (2021) EMU risk-synchronisation and financial fragility through the prism of dynamic connectedness. Q Rev Econ Financ 79: 1–14. https://doi.org/10.1016/j.qref.2020.12.003 doi: 10.1016/j.qref.2020.12.003
![]() |
[11] | Chatziantoniou I, Gabauer D, Gupta R (2021) Integration and risk transmission in the market for crude oil: a time-varying parameter frequency connectedness approach. Working Paper, University of Portsmouth, No. 202147. |
[12] |
Chatziantoniou I, Gabauer D, Stenfors A (2021) Interest rate swaps and the transmission mechanism of monetary policy: A quantile connectedness approach. Econ Lett 204: 109891. https://doi.org/10.1016/j.econlet.2021.109891 doi: 10.1016/j.econlet.2021.109891
![]() |
[13] |
Chavleishvili S, Manganelli S (2024) Forecasting and stress testing with quantile vector autoregression. J Appl Economet 39: 66–85. https://doi.org/10.1002/jae.3009 doi: 10.1002/jae.3009
![]() |
[14] |
D'Agostino RB (1970) Transformation to Normality of the Null Distribution of g1. Biometrika 57: 679–681. https://doi.org/10.2307/2334794. doi: 10.2307/2334794
![]() |
[15] |
Dai Z, Zhu J, Zhang X (2022) Time-frequency connectedness and cross-quantile dependence between crude oil, Chinese commodity market, stock market and investor sentiment. Energ Econ 114: 106226. https://doi.org/10.1016/j.eneco.2022.106226. doi: 10.1016/j.eneco.2022.106226
![]() |
[16] |
Diebold FX, Yilmaz K (2009) Measuring financial asset return and volatility spillovers, with application to global equity markets. Econ J 119: 158–171. https://doi.org/10.1111/j.1468-0297.2008.02208.x doi: 10.1111/j.1468-0297.2008.02208.x
![]() |
[17] |
Diebold FX, Yilmaz K (2012) Better to give than to receive: Predictive directional measurement of volatility spillovers. Int J Forecast 28: 57–66. https://doi.org/10.1016/j.ijforecast.2011.02.006 doi: 10.1016/j.ijforecast.2011.02.006
![]() |
[18] |
Diebold FX, Yılmaz K (2014) On the network topology of variance decompositions: Measuring the connectedness of financial firms. J Econometrics 182: 119–134. https://doi.org/10.1016/j.jeconom.2014.04.012 doi: 10.1016/j.jeconom.2014.04.012
![]() |
[19] |
Dornbusch R, Park YC, Claessens S (2000) Contagion: Understanding How It Spreads. World Bank Res Obs 15: 177–197. https://doi.org/10.1093/wbro/15.2.177 doi: 10.1093/wbro/15.2.177
![]() |
[20] | Elliott G, Rothenberg TJ, Stock JH (1992) Efficient Tests for an Autoregressive Unit Root (Working Paper No. 130) National Bureau of Economic Research. https://doi.org/10.3386/t0130 |
[21] | Fermanian JD, Scaillet O (2005) Some statistical pitfalls in copula modeling for financial applications. FAME Research paper No.108. |
[22] | Fleming JM (1962) Domestic financial policies under fixed and under floating exchange rates. Staff Pap Int Monetary Fund 9: 369–380. |
[23] |
Fisher TJ, Gallagher CM (2012) New Weighted Portmanteau Statistics for Time Series Goodness of Fit Testing. J Am Stat Assoc 107: 777–787. https://doi.org/10.1080/01621459.2012.688465 doi: 10.1080/01621459.2012.688465
![]() |
[24] |
Furuoka F, Yaya OS, Ling PK, et al. (2023) Transmission of risks between energy and agricultural commodities: Frequency time-varying VAR, asymmetry and portfolio management. Resour Policy 81: 103339. https://doi.org/10.1016/j.resourpol.2023.103339 doi: 10.1016/j.resourpol.2023.103339
![]() |
[25] | Gautam A, Lepone G (2024) Time Zone Difference and Equity Market Price Efficiency Post Earnings Announcements. http://dx.doi.org/10.2139/ssrn.4815836 |
[26] |
Gabauer D (2021) Dynamic measures of asymmetric & pairwise connectedness within an optimal currency area: Evidence from the ERM I system. J Multinatl Financ M 60: 100680. https://doi.org/10.1016/j.mulfin.2021.100680 doi: 10.1016/j.mulfin.2021.100680
![]() |
[27] |
Grote MH (2007) Mobile marketplaces—consequences of the changing governance of European stock exchanges. Growth Change 38: 260–278. https://doi.org/10.1111/j.1468-2257.2007.00366.x doi: 10.1111/j.1468-2257.2007.00366.x
![]() |
[28] |
Gong C, Tang P, Wang Y (2019) Measuring the network connectedness of global stock markets. Phys A 535: 122351. https://doi.org/10.1016/j.physa.2019.122351 doi: 10.1016/j.physa.2019.122351
![]() |
[29] | Huang Y, Su W, Li X (2010) Comparison of BEKK GARCH and DCC GARCH models: An empirical study, In: Advanced Data Mining and Applications, Springer Berlin Heidelberg, 99–110. https://doi.org/10.1007/978-3-642-17313-4_10 |
[30] | Ito T, Krueger AO (2009) Regional and Global Capital Flows: Macroeconomic Causes and Consequences, University of Chicago Press. |
[31] |
Iwanicz-Drozdowska M, Rogowicz K, Kurowski Ł, et al. (2021) Two decades of contagion effect on stock markets: Which events are more contagious? J Financ Stabil 55: 100907. https://doi.org/10.1016/j.jfs.2021.100907 doi: 10.1016/j.jfs.2021.100907
![]() |
[32] |
Kao EH, Ho TW, Fung HG (2015) Price linkage between the US and Japanese futures across different time zones: An analysis of the minute-by-minute data. J Int Financ Mark I 34: 321–336. https://doi.org/10.1016/j.intfin.2014.12.002 doi: 10.1016/j.intfin.2014.12.002
![]() |
[33] |
Koop G, Pesaran MH, Potter SM (1996) Impulse response analysis in nonlinear multivariate models. J Econometrics 74: 119–147. https://doi.org/10.1016/0304-4076(95)01753-4 doi: 10.1016/0304-4076(95)01753-4
![]() |
[34] | Korkmaz A (2014) Can the Interdependence Effect and the Contagion Phenomena be Related with One Another? J Empirl Stud 1: 38–47. |
[35] |
Lin WL, Engle RF, Ito T (1994) Do bulls and bears move across borders? International transmission of stock returns and volatility. Rev Financ Stud 7: 507–538. https://doi.org/10.1093/rfs/7.3.507 doi: 10.1093/rfs/7.3.507
![]() |
[36] |
Mai JF, Scherer M (2013) What makes dependence modeling challenging? Pitfalls and ways to circumvent them. Statist Risk Model 30: 287–306. https://doi.org/10.1524/strm.2013.2001 doi: 10.1524/strm.2013.2001
![]() |
[37] |
Marfatia HA (2017) A Fresh Look at Integration of Risks in the International Stock Markets: A Wavelet Approach. Rev Financ Econ 34: 33–49. https://doi.org/10.1016/j.rfe.2017.07.003 doi: 10.1016/j.rfe.2017.07.003
![]() |
[38] |
Mensi W, Boubaker FZ, Al-Yahyaee KH, et al. (2018) Dynamic volatility spillovers and connectedness between global, regional, and GIPSI stock markets. Financ Res Lett 25: 230–238. https://doi.org/10.1016/j.frl.2017.10.032 doi: 10.1016/j.frl.2017.10.032
![]() |
[39] |
Mensi W, Ziadat SA, Vo XV, et al. (2024) Extreme quantile connectedness and spillovers between oil and Vietnamese stock markets: a sectoral analysis. Int J Emerg Mark 19: 1586–1625. https://doi.org/10.1108/IJOEM-03-2022-0513 doi: 10.1108/IJOEM-03-2022-0513
![]() |
[40] |
Mishkin FS (1999) Lessons from the Asian crisis. J Int Money Financ 18: 709–723. https://doi.org/10.1016/S0261-5606(99)00020-0 doi: 10.1016/S0261-5606(99)00020-0
![]() |
[41] | Noble GW, Ravenhill J (2000) Causes and Consequences of the Asian Financial Crisis, In: G. W. Noble & J. Ravenhill (Eds.), The Asian Financial Crisis and the Architecture of Global Finance Cambridge University Press, 1st ed., 1–35. https://doi.org/10.1017/CBO9781139171328.002 |
[42] |
Pesaran HH, Shin Y (1998) Generalized impulse response analysis in linear multivariate models. Econ Lett 58: 17–29. https://doi.org/10.1016/S0165-1765(97)00214-0 doi: 10.1016/S0165-1765(97)00214-0
![]() |
[43] | S&P Global (2023) S&Q Capital IQ. https://www.spglobal.com/marketintelligence/. [accessed on 25 February 2023]. |
[44] |
Samarakoon LP (2011) Stock market interdependence, contagion, and the US financial crisis: The case of emerging and frontier markets. J Int Financ Mark I 21: 724–742. https://doi.org/10.1016/j.intfin.2011.05.001 doi: 10.1016/j.intfin.2011.05.001
![]() |
[45] |
Serrano P, Vaello-Sebastià A, Vich-Llompart MM (2023) The international linkages of market risk perception. J Multinatl Financ M 72: 100826. https://doi.org/10.1016/j.mulfin.2023.100826 doi: 10.1016/j.mulfin.2023.100826
![]() |
[46] | Schüler YS (2014) Asymmetric effects of uncertainty over the business cycle: A quantile structural vector autoregressive approach. Universi ty of Konstanz, Department of Economics, Working Paper No. 2014-0. |
[47] |
Tiwari AK, Abakah EJA, Yaya OS, et al. (2022) Tail risk dependence, co-movement and predictability between green bond and green stocks. Appl Econ 55: 201–222. https://doi.org/10.1080/00036846.2022.2085869 doi: 10.1080/00036846.2022.2085869
![]() |
[48] | Tuominen DR (2020) Investigation on how Brexit has impacted IPO listings with Deutsche Börse and the London Stock Exchange. Degree Thesis, Arcada University of Applied Sciences (Finland). Available from: https://www.theseus.fi/handle/10024/353824 (accessed on 6 April 2024) |
[49] | Ullah S (2015) The Impact of Internal Corporate Governance Mechanisms on the Performance of Firms: Evidence from the UK and Germany. Open University (United Kingdom). Available from: https://www.proquest.com/docview/2171038131?pq-origsite = gscholar & fromopenview = true & sourcetype = Dissertations%20 & %20Theses(accessed on 7 February 2024) |
[50] | Wang P, Wang P (2010) Price and volatility spillovers between the Greater China Markets and the developed markets of US and Japan. Global Financ J 21: 304–317. https://doi.org/10.1016/j.gfj.2010.09.007 |
[51] |
Yang Z, Zhou Y (2017) Quantitative easing and volatility spillovers across countries and asset classes. Manage Sci 63: 333–354. https://doi.org/10.1287/mnsc.2015.2305 doi: 10.1287/mnsc.2015.2305
![]() |
[52] |
Yousaf I, Mensi W, Vo XV, et al. (2023) Spillovers and connectedness between Chinese and ASEAN stock markets during bearish and bullish market statuses. Int J Emerg Mark. https://doi.org/10.1108/IJOEM-07-2022-1194 doi: 10.1108/IJOEM-07-2022-1194
![]() |
[53] | Yuce A, Simga-Mugan C (2000) Linkages among Eastern European stock markets and the major stock exchanges. Russian East Eur Financ Trade 36: 54–69. |
[54] |
Zhang Q, Wei R (2024) Carbon reduction attention and financial market stress: A network spillover analysis based on quantile VAR modeling. J Environ Manage 356: 120640. https://doi.org/10.1016/j.jenvman.2024.120640 doi: 10.1016/j.jenvman.2024.120640
![]() |
![]() |
![]() |
1. | William Guo, Design and implementation of multi-purpose quizzes to improve mathematics learning for transitional engineering students, 2022, 2, 2767-1925, 245, 10.3934/steme.2022015 | |
2. | Tom Gorham, Rwitajit Majumdar, Hiroaki Ogata, Analyzing learner profiles in a microlearning app for training language learning peer feedback skills, 2023, 2197-9987, 10.1007/s40692-023-00264-0 | |
3. | Winny Astiwardhani, , Transforming Educational Paradigms: How Micro Learning Shapes Student Understanding, Retention, and Motivation?, 2024, 8, 2549-3272, 300, 10.23887/jear.v8i2.77711 | |
4. | Soheila Garshasbi, Sabine Graf, Mahmood Asgari, Jun Shen, Sarah Howard, 2023, An Optimal Grouping and Regrouping Method for Effective Collaborative Learning: Leveraging the Group Dynamics, 978-1-6654-5331-8, 1, 10.1109/TALE56641.2023.10398311 | |
5. | Wali Khan Monib, Atika Qazi, Rosyzie Anna Apong, Mapping microlearning development and trends across diverse contexts: a bibliometric analysis (2007–2023), 2024, 1049-4820, 1, 10.1080/10494820.2024.2402556 | |
6. | Zhisheng Chen, Responsible AI in Organizational Training: Applications, Implications, and Recommendations for Future Development, 2024, 23, 1534-4843, 498, 10.1177/15344843241273316 | |
7. | Nurul Fitriah Alias, Rafiza Abdul Razak, Revolutionizing learning in the digital age: a systematic literature review of microlearning strategies, 2024, 1049-4820, 1, 10.1080/10494820.2024.2331638 | |
8. | Mariana Madalina Nastase, Elvira Popescu, 2023, Chapter 32, 978-3-031-32882-4, 352, 10.1007/978-3-031-32883-1_32 | |
9. | Ján Skalka, Matúš Valko, Rapid Guessing Behavior Detection in Microlearning: Insights Into Student Performance, Engagement, and Response Accuracy, 2024, 12, 2169-3536, 157996, 10.1109/ACCESS.2024.3485505 | |
10. | Shahid Bashir, Alexander L. Lapshun, E-learning future trends in higher education in the 2020s and beyond, 2025, 12, 2331-186X, 10.1080/2331186X.2024.2445331 | |
11. | Nurul Fitriah Alias, 2025, chapter 7, 9798369384527, 239, 10.4018/979-8-3693-8452-7.ch007 | |
12. | Mª Ángeles López-Cabarcos, Analía López-Carballeira, 2025, chapter 2, 9798337307053, 21, 10.4018/979-8-3373-0705-3.ch002 | |
13. | Haiyan Zhong, Jingbo Xu, The effect of fragmented learning ability on college students’ online learning satisfaction: exploring the mediating role of affective, behavioral, and cognitive engagement, 2025, 1049-4820, 1, 10.1080/10494820.2025.2488142 | |
14. | Haozhun Luo, Weiyan Li, Impact of microlearning on developing soft skills of university students across disciplines, 2025, 16, 1664-1078, 10.3389/fpsyg.2025.1491265 |